1 KPAs KPIs and metrics Performance measurement in
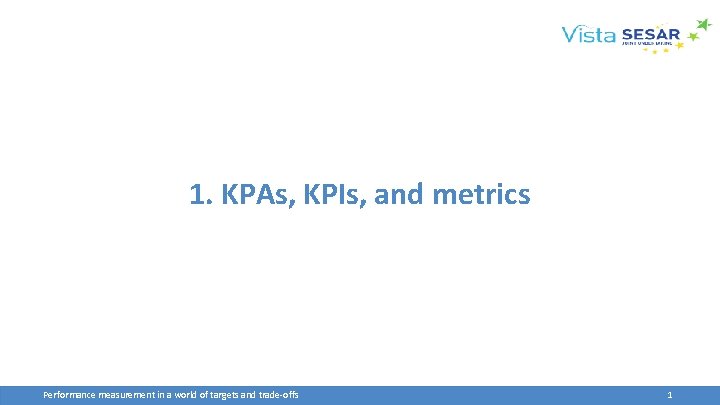
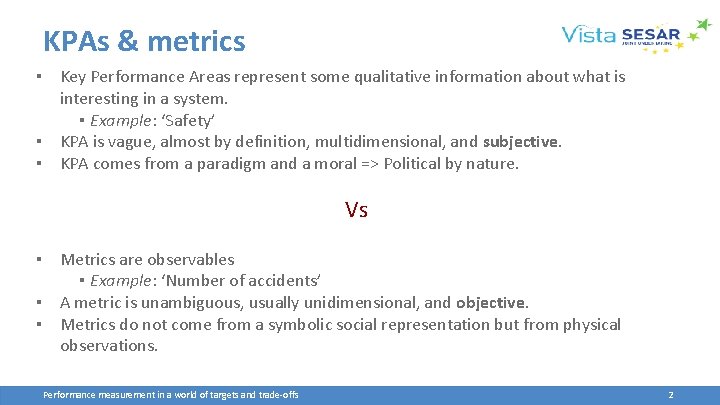
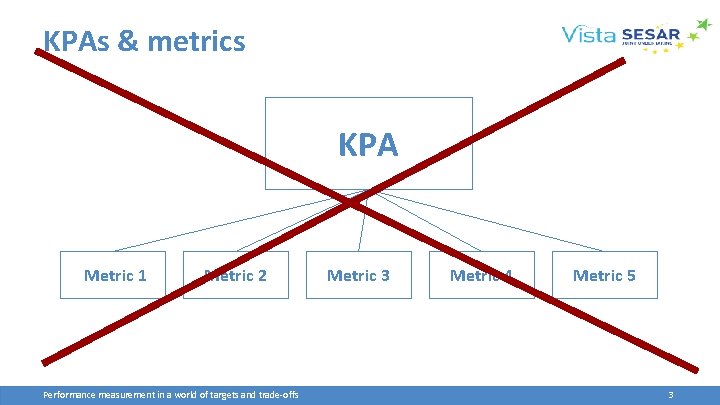
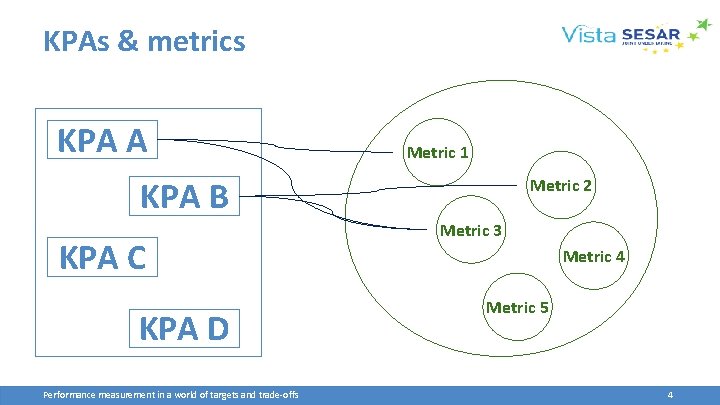
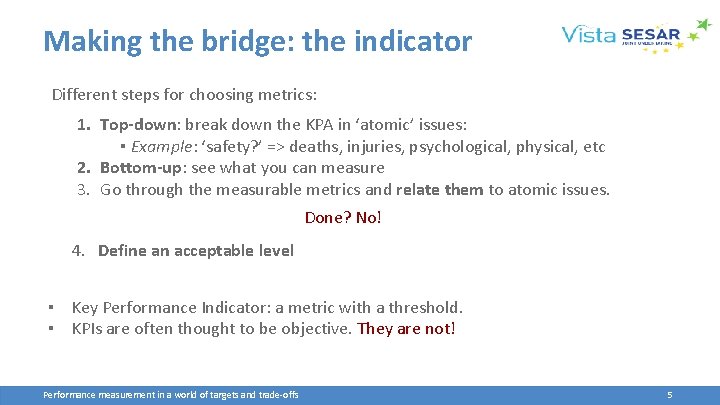
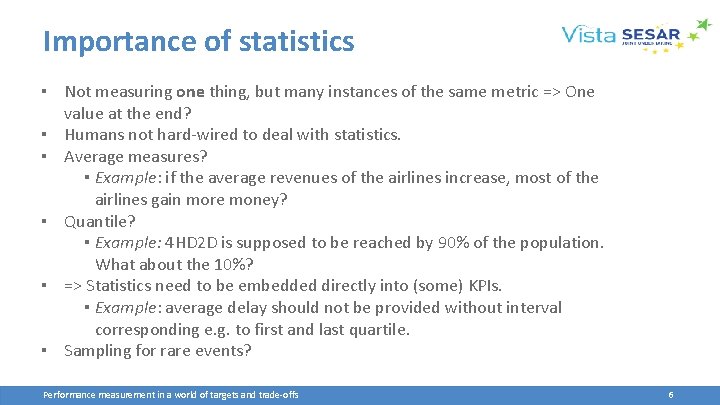
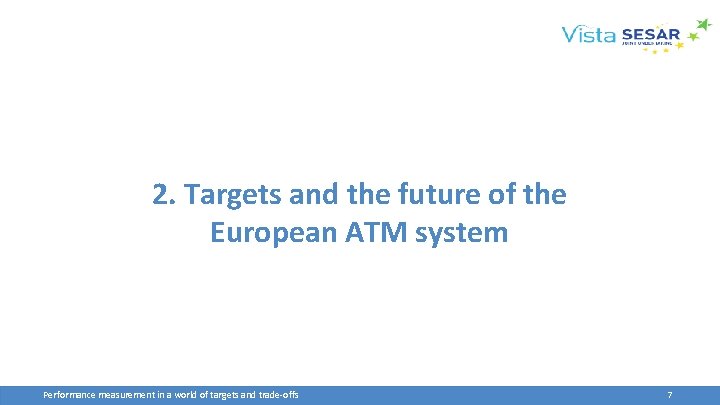
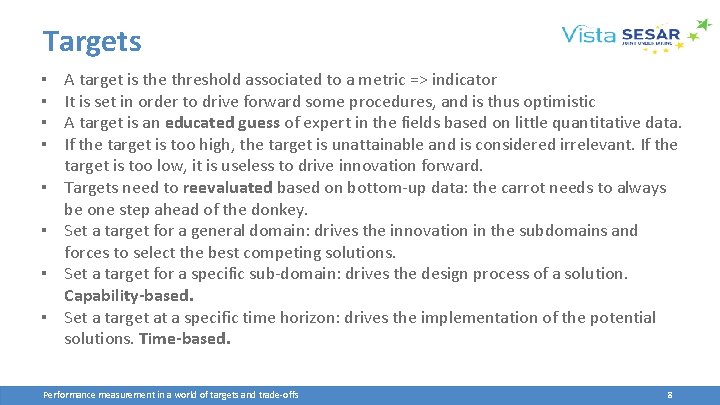
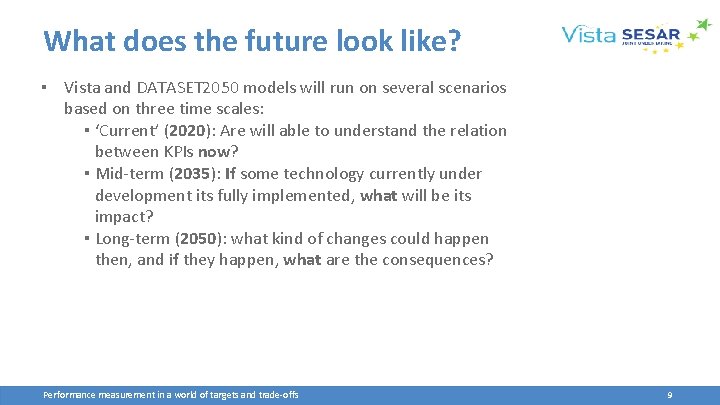
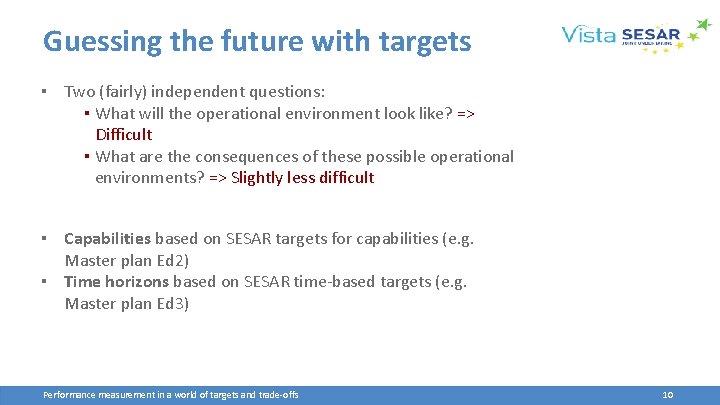
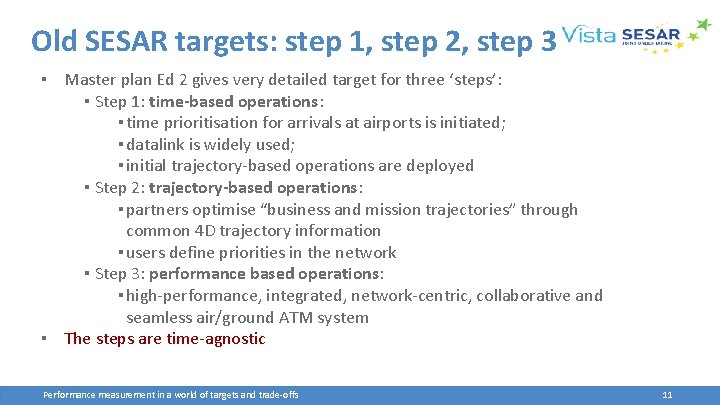
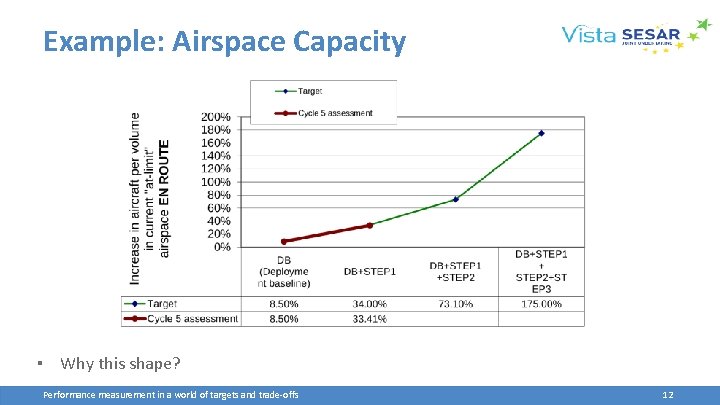
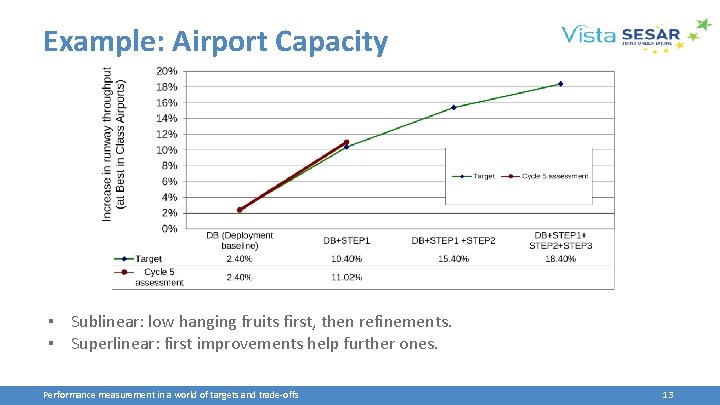
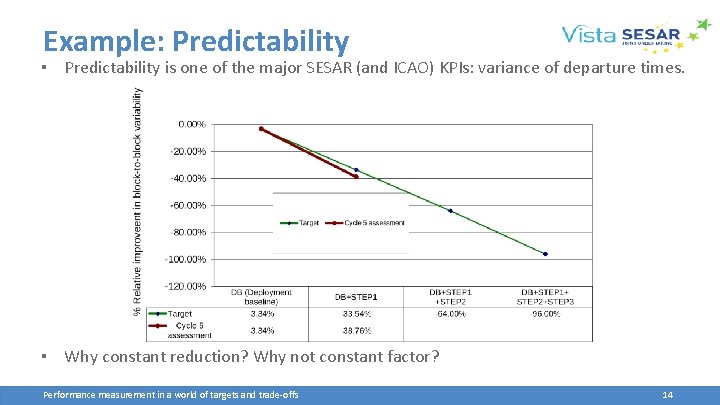
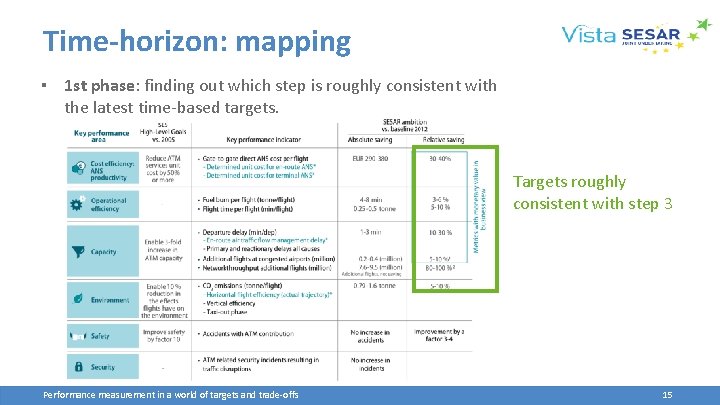
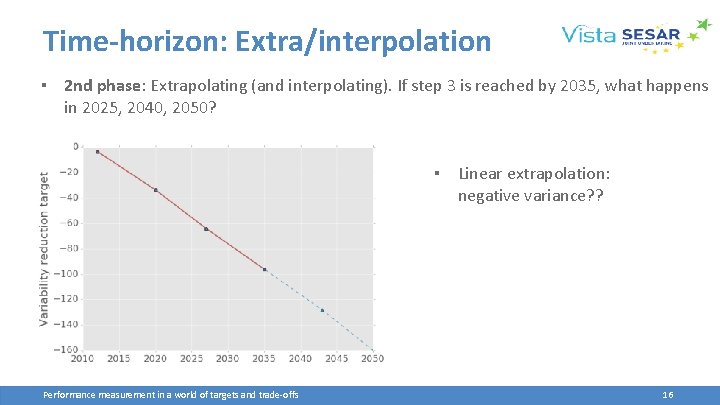
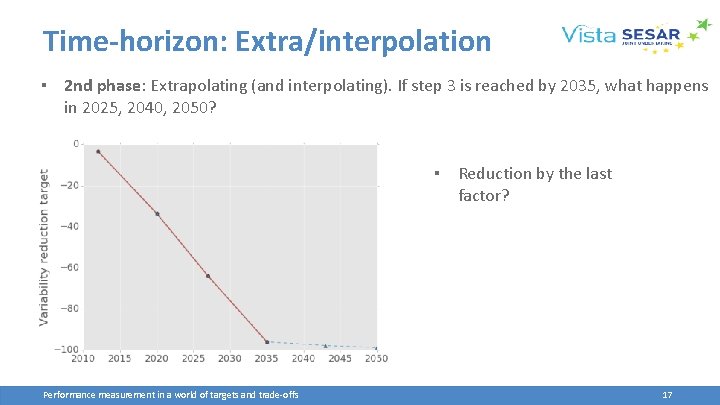
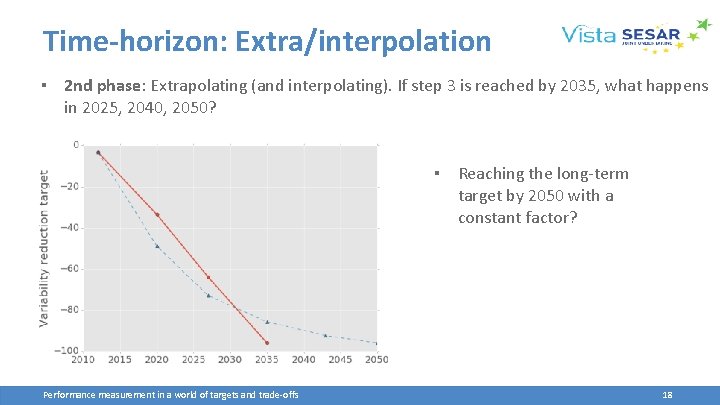
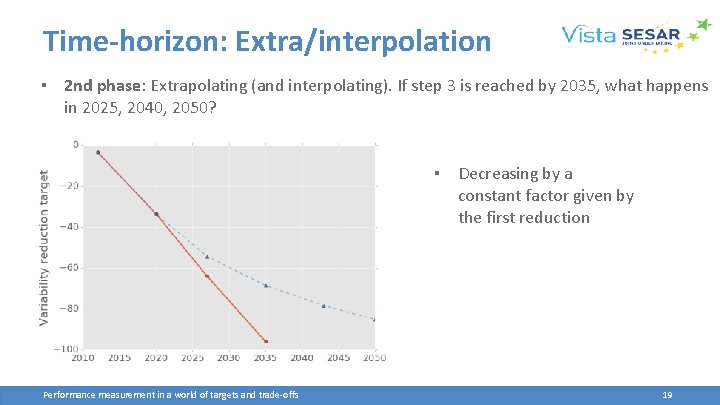
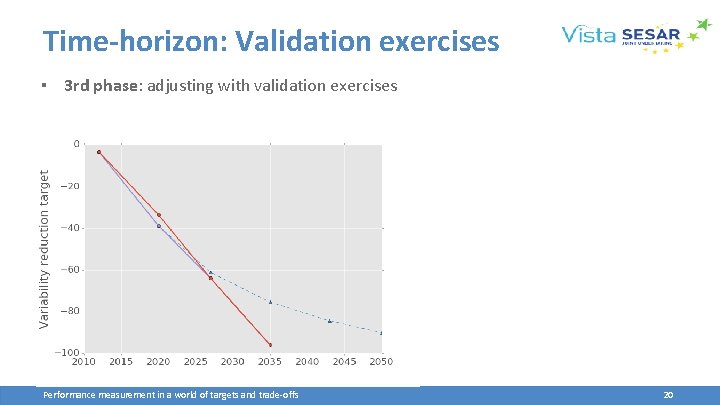
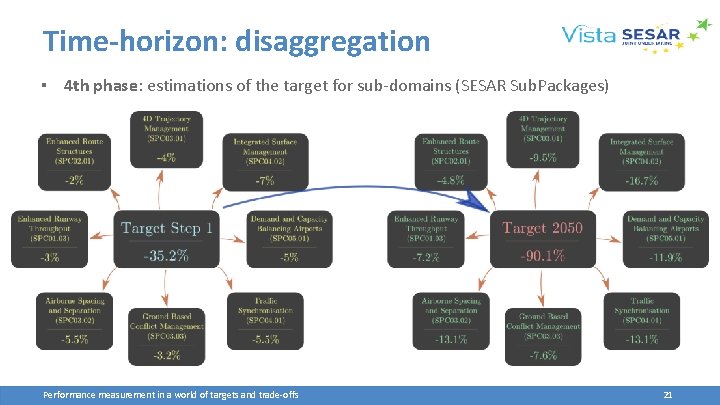
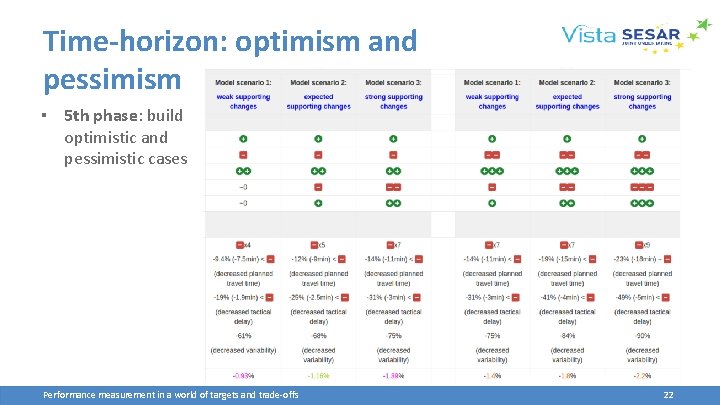
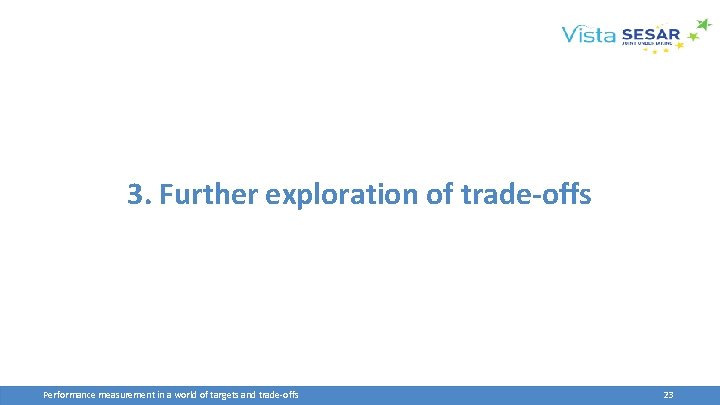
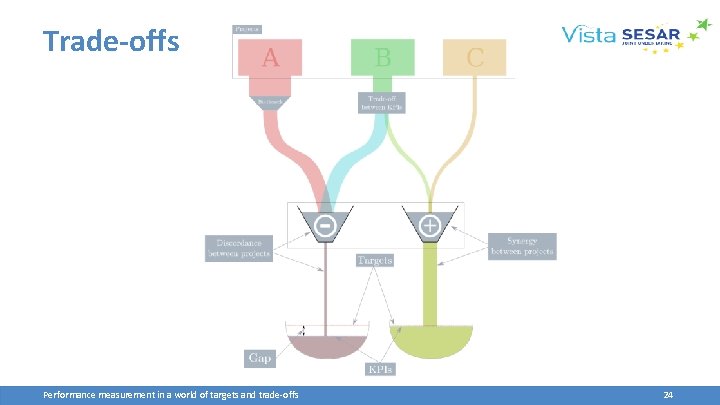
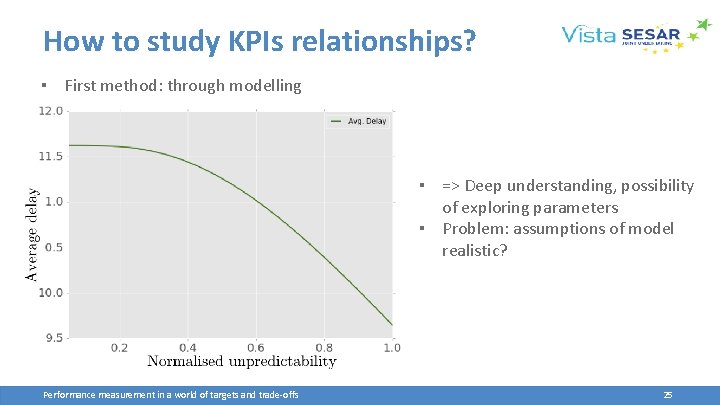
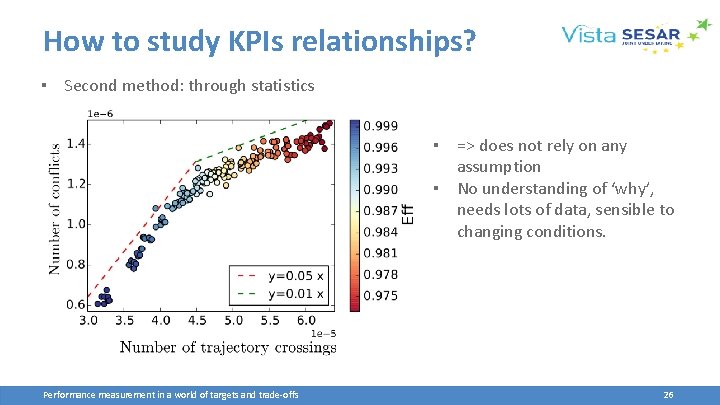
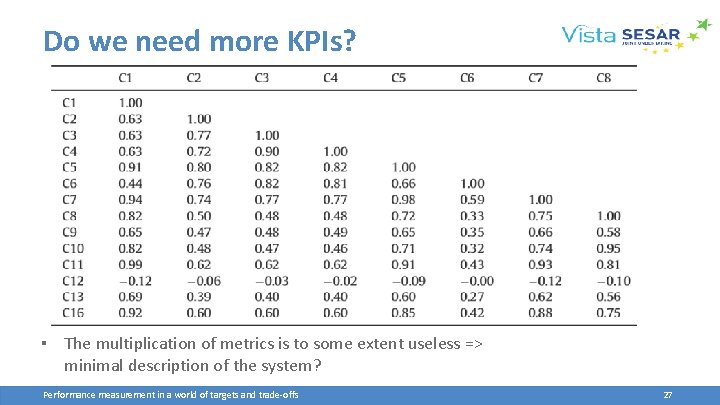
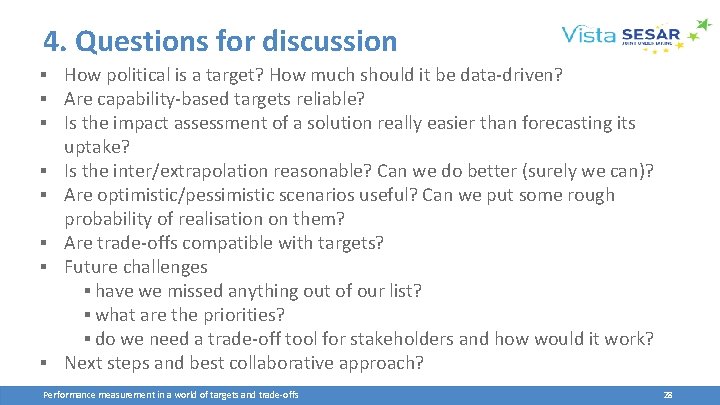
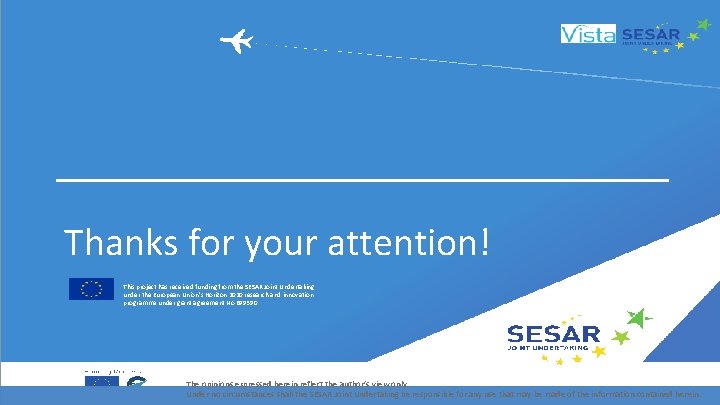
- Slides: 29
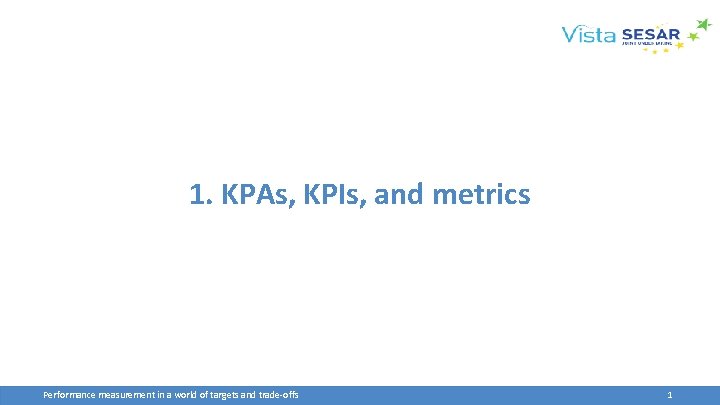
1. KPAs, KPIs, and metrics Performance measurement in a world of targets and trade‐offs 1
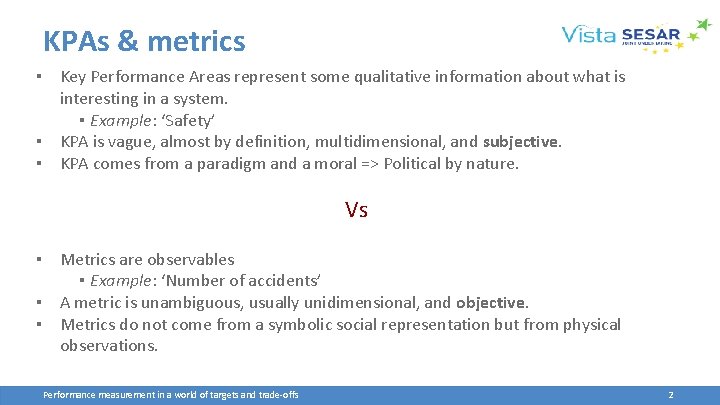
KPAs & metrics ▪ Key Performance Areas represent some qualitative information about what is interesting in a system. ▪ Example: ‘Safety’ ▪ KPA is vague, almost by definition, multidimensional, and subjective. ▪ KPA comes from a paradigm and a moral => Political by nature. Vs ▪ Metrics are observables ▪ Example: ‘Number of accidents’ ▪ A metric is unambiguous, usually unidimensional, and objective. ▪ Metrics do not come from a symbolic social representation but from physical observations. Performance measurement in a world of targets and trade‐offs 2
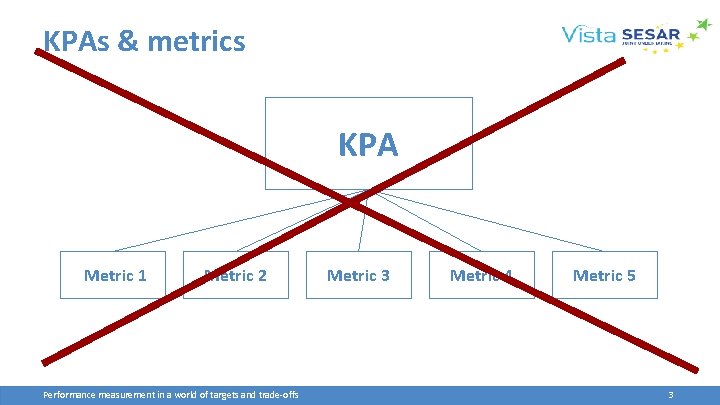
KPAs & metrics KPA Metric 1 Metric 2 Performance measurement in a world of targets and trade‐offs Metric 3 Metric 4 Metric 5 3
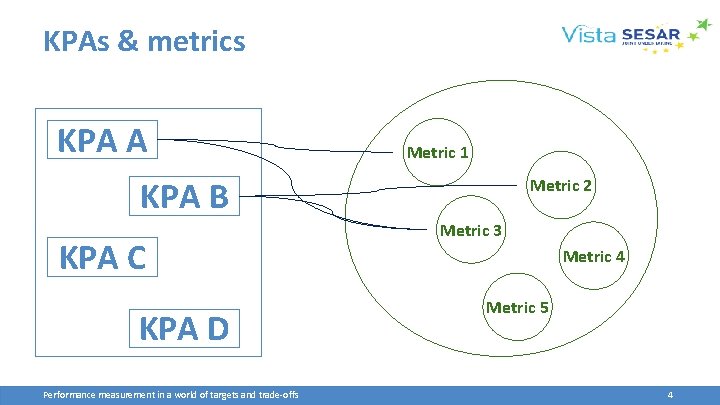
KPAs & metrics KPA A Metric 1 KPA B KPA C KPA D Performance measurement in a world of targets and trade‐offs Metric 2 Metric 3 Metric 4 Metric 5 4
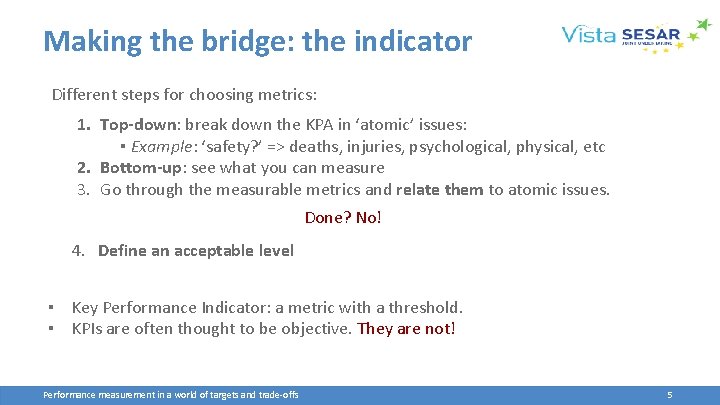
Making the bridge: the indicator Different steps for choosing metrics: 1. Top-down: break down the KPA in ‘atomic’ issues: ▪ Example: ‘safety? ’ => deaths, injuries, psychological, physical, etc 2. Bottom-up: see what you can measure 3. Go through the measurable metrics and relate them to atomic issues. Done? No! 4. Define an acceptable level ▪ Key Performance Indicator: a metric with a threshold. ▪ KPIs are often thought to be objective. They are not! Performance measurement in a world of targets and trade‐offs 5
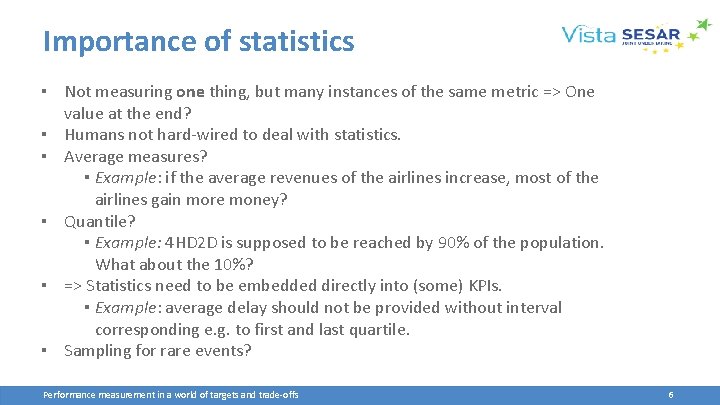
Importance of statistics ▪ Not measuring one thing, but many instances of the same metric => One value at the end? ▪ Humans not hard‐wired to deal with statistics. ▪ Average measures? ▪ Example: if the average revenues of the airlines increase, most of the airlines gain more money? ▪ Quantile? ▪ Example: 4 HD 2 D is supposed to be reached by 90% of the population. What about the 10%? ▪ => Statistics need to be embedded directly into (some) KPIs. ▪ Example: average delay should not be provided without interval corresponding e. g. to first and last quartile. ▪ Sampling for rare events? Performance measurement in a world of targets and trade‐offs 6
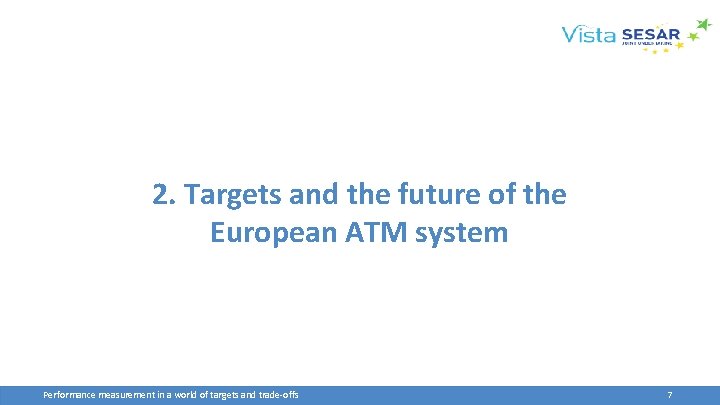
2. Targets and the future of the European ATM system Performance measurement in a world of targets and trade‐offs 7
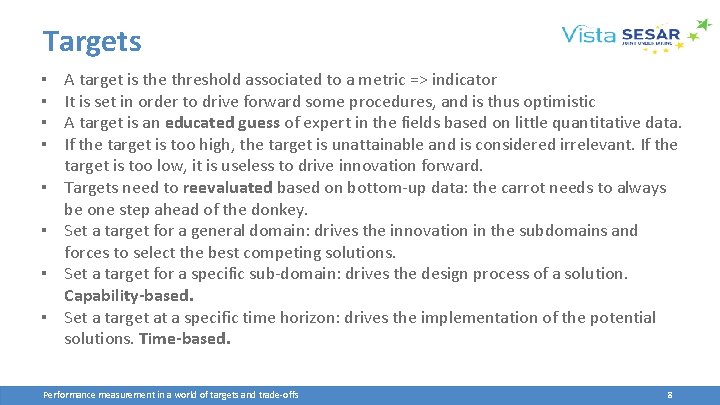
Targets ▪ ▪ ▪ ▪ A target is the threshold associated to a metric => indicator It is set in order to drive forward some procedures, and is thus optimistic A target is an educated guess of expert in the fields based on little quantitative data. If the target is too high, the target is unattainable and is considered irrelevant. If the target is too low, it is useless to drive innovation forward. Targets need to reevaluated based on bottom‐up data: the carrot needs to always be one step ahead of the donkey. Set a target for a general domain: drives the innovation in the subdomains and forces to select the best competing solutions. Set a target for a specific sub‐domain: drives the design process of a solution. Capability-based. Set a target at a specific time horizon: drives the implementation of the potential solutions. Time-based. Performance measurement in a world of targets and trade‐offs 8
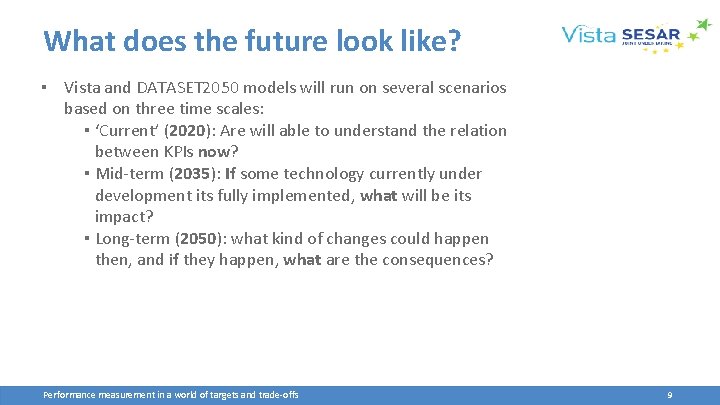
What does the future look like? ▪ Vista and DATASET 2050 models will run on several scenarios based on three time scales: ▪ ‘Current’ (2020): Are will able to understand the relation between KPIs now? ▪ Mid‐term (2035): If some technology currently under development its fully implemented, what will be its impact? ▪ Long‐term (2050): what kind of changes could happen then, and if they happen, what are the consequences? Performance measurement in a world of targets and trade‐offs 9
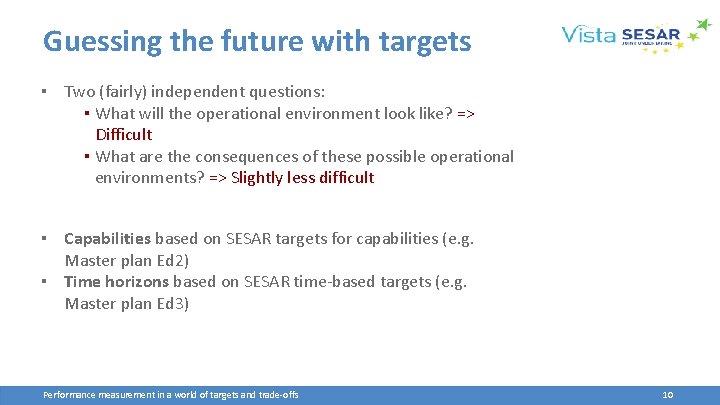
Guessing the future with targets ▪ Two (fairly) independent questions: ▪ What will the operational environment look like? => Difficult ▪ What are the consequences of these possible operational environments? => Slightly less difficult ▪ Capabilities based on SESAR targets for capabilities (e. g. Master plan Ed 2) ▪ Time horizons based on SESAR time‐based targets (e. g. Master plan Ed 3) Performance measurement in a world of targets and trade‐offs 10
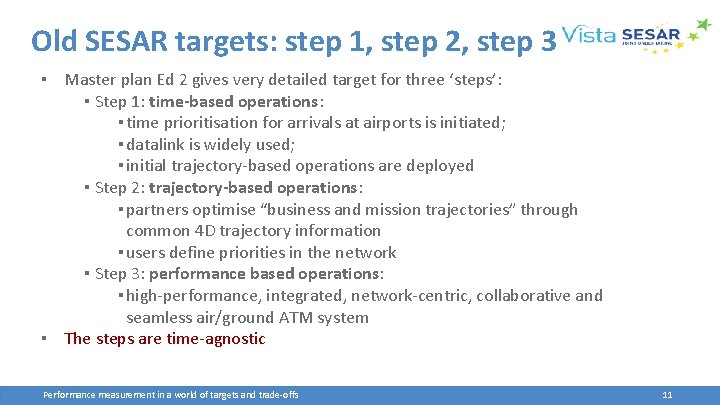
Old SESAR targets: step 1, step 2, step 3 ▪ Master plan Ed 2 gives very detailed target for three ‘steps’: ▪ Step 1: time-based operations: ▪ time prioritisation for arrivals at airports is initiated; ▪ datalink is widely used; ▪ initial trajectory‐based operations are deployed ▪ Step 2: trajectory-based operations: ▪ partners optimise “business and mission trajectories” through common 4 D trajectory information ▪ users define priorities in the network ▪ Step 3: performance based operations: ▪ high‐performance, integrated, network‐centric, collaborative and seamless air/ground ATM system ▪ The steps are time‐agnostic Performance measurement in a world of targets and trade‐offs 11
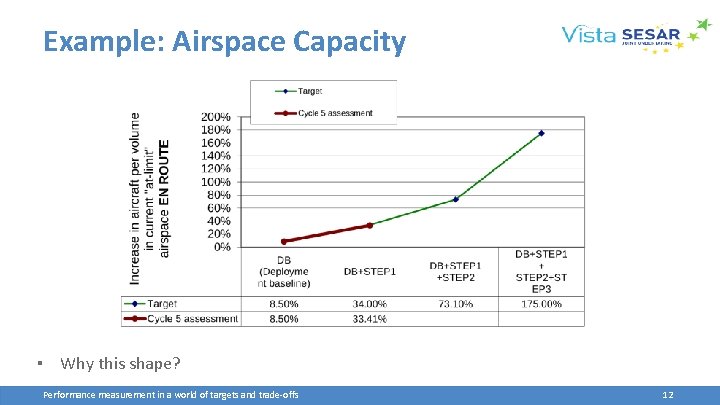
Example: Airspace Capacity ▪ Why this shape? Performance measurement in a world of targets and trade‐offs 12
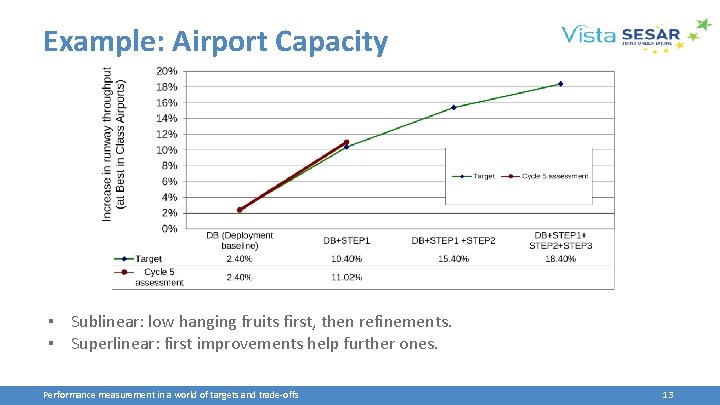
Example: Airport Capacity ▪ Sublinear: low hanging fruits first, then refinements. ▪ Superlinear: first improvements help further ones. Performance measurement in a world of targets and trade‐offs 13
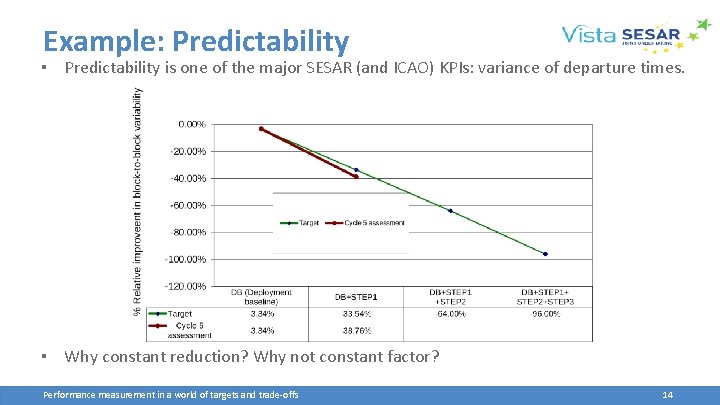
Example: Predictability ▪ Predictability is one of the major SESAR (and ICAO) KPIs: variance of departure times. ▪ Why constant reduction? Why not constant factor? Performance measurement in a world of targets and trade‐offs 14
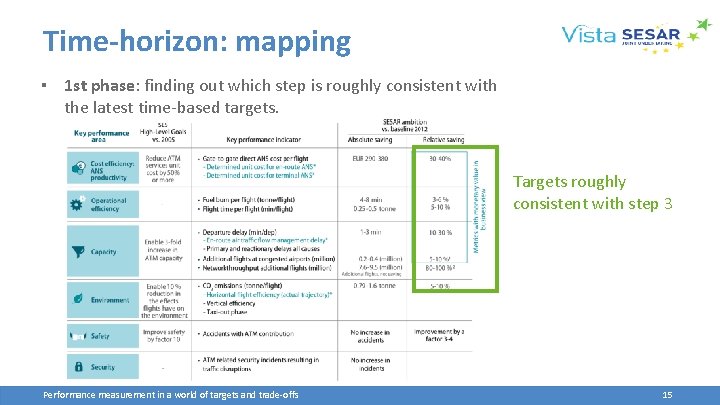
Time-horizon: mapping ▪ 1 st phase: finding out which step is roughly consistent with the latest time‐based targets. Targets roughly consistent with step 3 Performance measurement in a world of targets and trade‐offs 15
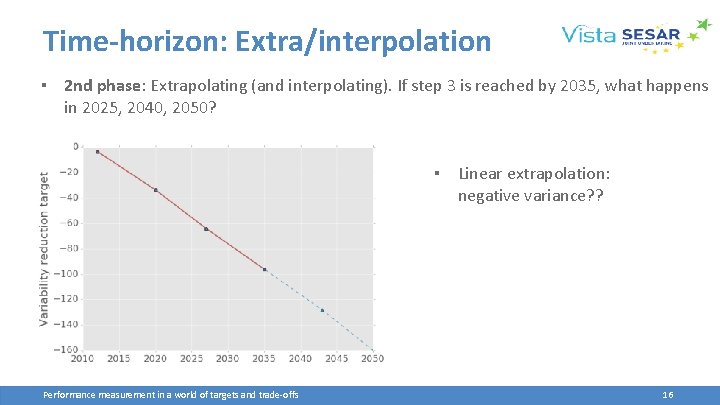
Time-horizon: Extra/interpolation ▪ 2 nd phase: Extrapolating (and interpolating). If step 3 is reached by 2035, what happens in 2025, 2040, 2050? ▪ Linear extrapolation: negative variance? ? Performance measurement in a world of targets and trade‐offs 16
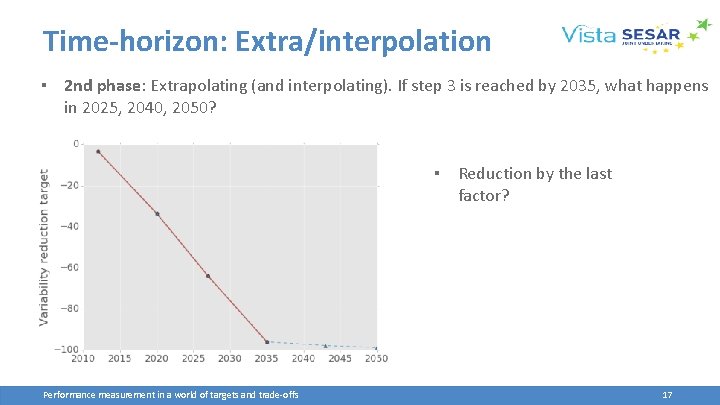
Time-horizon: Extra/interpolation ▪ 2 nd phase: Extrapolating (and interpolating). If step 3 is reached by 2035, what happens in 2025, 2040, 2050? ▪ Reduction by the last factor? Performance measurement in a world of targets and trade‐offs 17
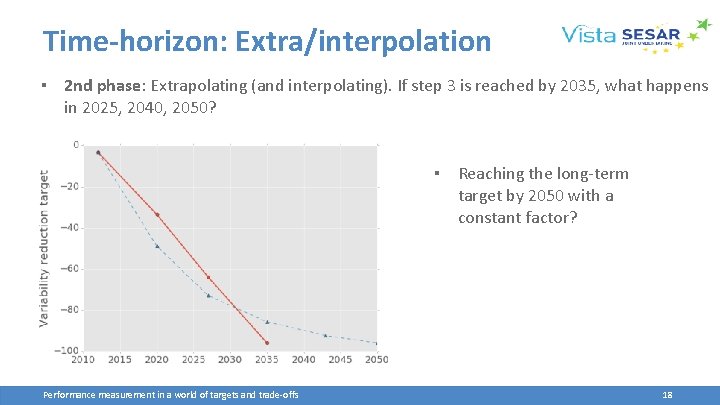
Time-horizon: Extra/interpolation ▪ 2 nd phase: Extrapolating (and interpolating). If step 3 is reached by 2035, what happens in 2025, 2040, 2050? ▪ Reaching the long‐term target by 2050 with a constant factor? Performance measurement in a world of targets and trade‐offs 18
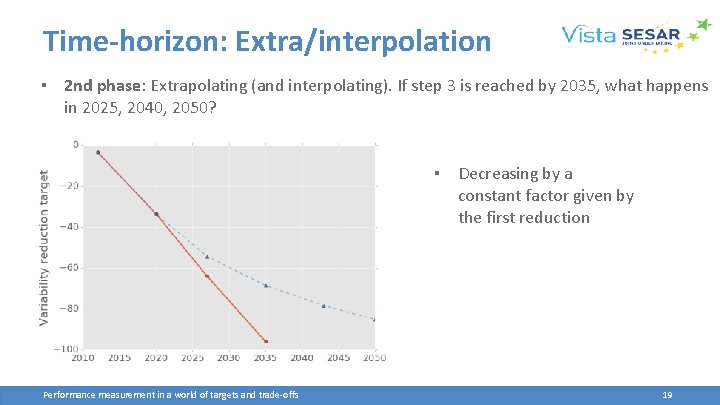
Time-horizon: Extra/interpolation ▪ 2 nd phase: Extrapolating (and interpolating). If step 3 is reached by 2035, what happens in 2025, 2040, 2050? ▪ Decreasing by a constant factor given by the first reduction Performance measurement in a world of targets and trade‐offs 19
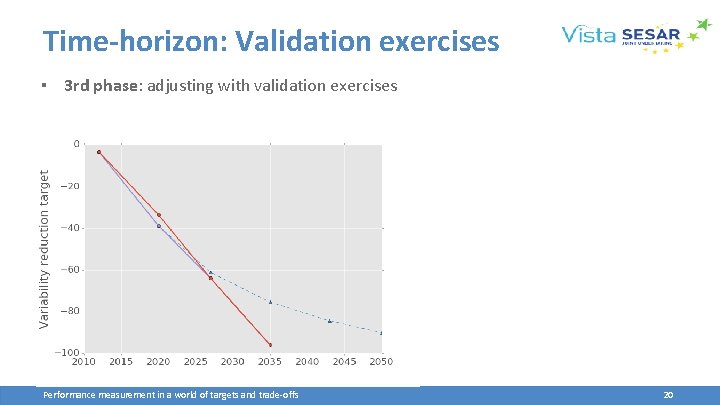
Time-horizon: Validation exercises ▪ 3 rd phase: adjusting with validation exercises Performance measurement in a world of targets and trade‐offs 20
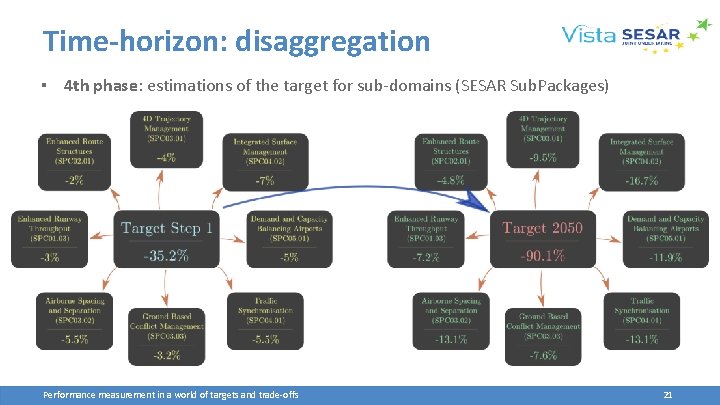
Time-horizon: disaggregation ▪ 4 th phase: estimations of the target for sub‐domains (SESAR Sub. Packages) Performance measurement in a world of targets and trade‐offs 21
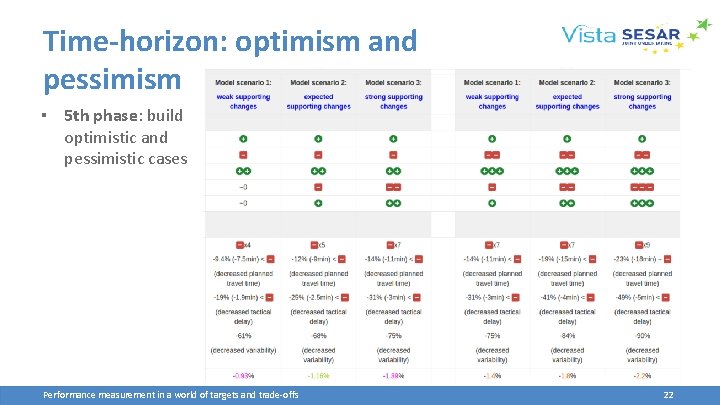
Time-horizon: optimism and pessimism ▪ 5 th phase: build optimistic and pessimistic cases Performance measurement in a world of targets and trade‐offs 22
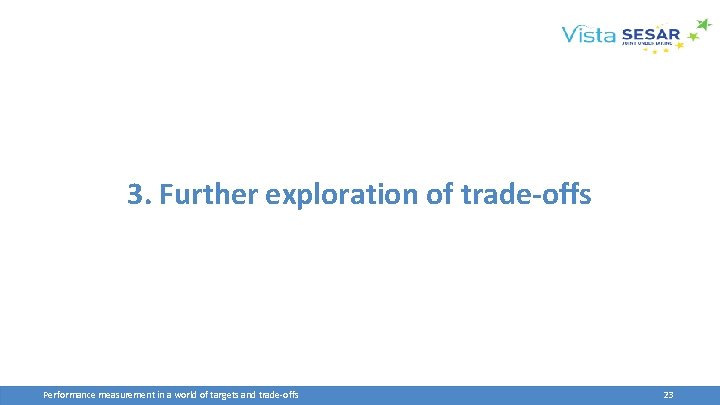
3. Further exploration of trade-offs Performance measurement in a world of targets and trade‐offs 23
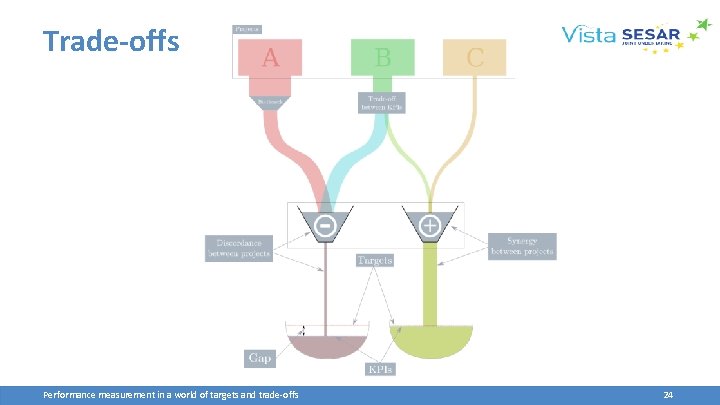
Trade-offs Performance measurement in a world of targets and trade‐offs 24
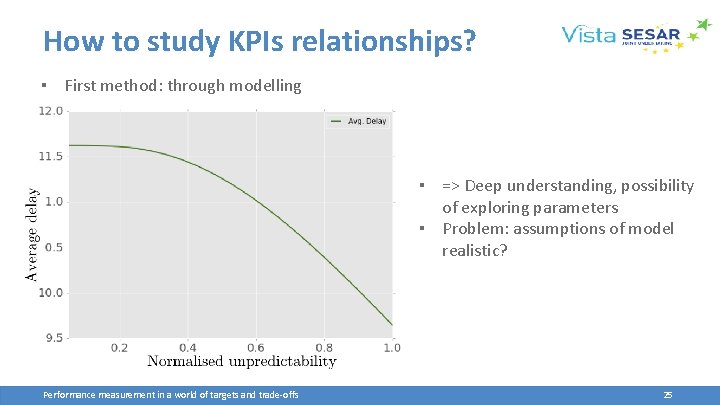
How to study KPIs relationships? ▪ First method: through modelling ▪ => Deep understanding, possibility of exploring parameters ▪ Problem: assumptions of model realistic? Performance measurement in a world of targets and trade‐offs 25
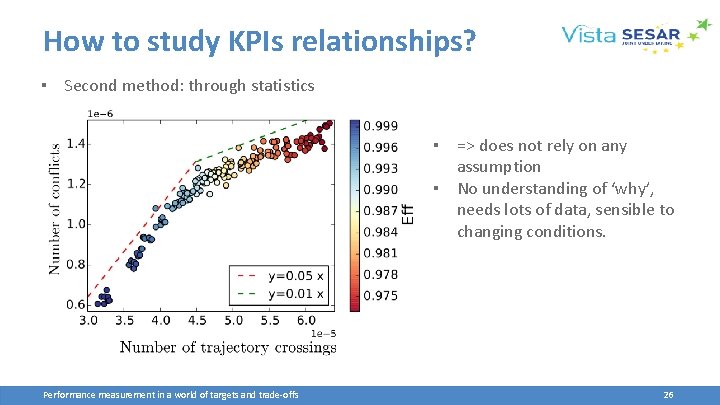
How to study KPIs relationships? ▪ Second method: through statistics ▪ => does not rely on any assumption ▪ No understanding of ‘why’, needs lots of data, sensible to changing conditions. Performance measurement in a world of targets and trade‐offs 26
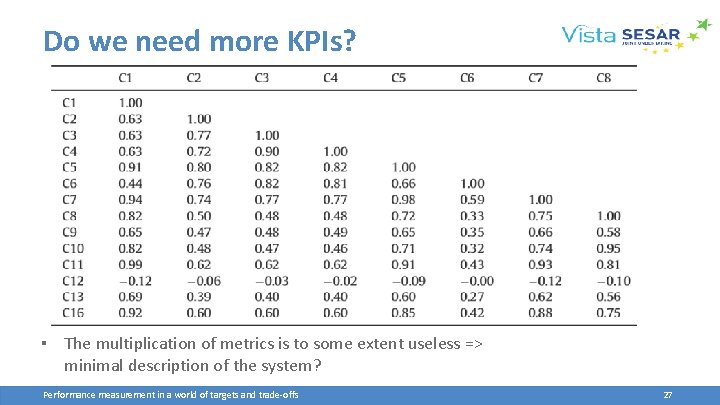
Do we need more KPIs? ▪ The multiplication of metrics is to some extent useless => minimal description of the system? Performance measurement in a world of targets and trade‐offs 27
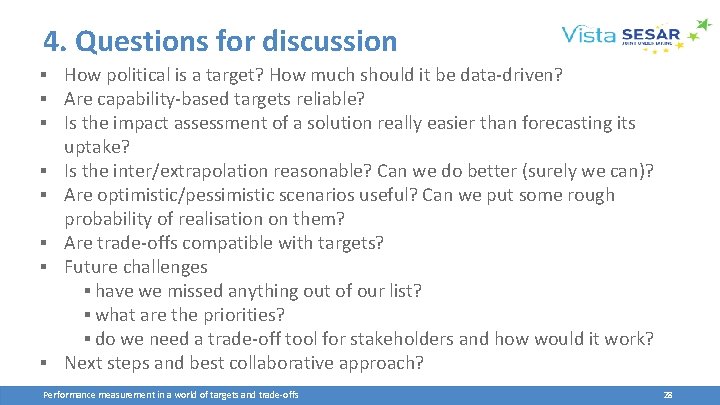
4. Questions for discussion ▪ How political is a target? How much should it be data‐driven? ▪ Are capability‐based targets reliable? ▪ Is the impact assessment of a solution really easier than forecasting its uptake? ▪ Is the inter/extrapolation reasonable? Can we do better (surely we can)? ▪ Are optimistic/pessimistic scenarios useful? Can we put some rough probability of realisation on them? ▪ Are trade‐offs compatible with targets? ▪ Future challenges ▪ have we missed anything out of our list? ▪ what are the priorities? ▪ do we need a trade‐off tool for stakeholders and how would it work? ▪ Next steps and best collaborative approach? Performance measurement in a world of targets and trade‐offs 28
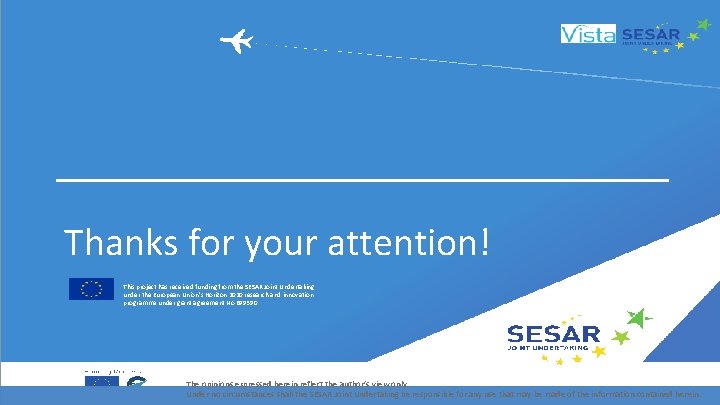
Thanks for your attention! This project has received funding from the SESAR Joint Undertaking under the European Union’s Horizon 2020 research and innovation programme under grant agreement No 699390. The opinions expressed herein reflect the author’s view only. Under no circumstances shall the SESAR Joint Undertaking be responsible for any use that may be made of the information contained herein.
Software measurement and metrics
27000 series
Health and safety kpi
Kpi profit margin
Chapter 2
Strategic e-marketing and performance metrics
Kpis de seguridad
Kpis de awareness
Supply chain kpi scorecard
Performance indicator template
Revenue cycle management indicators
Automation coverage metrics
L2name
Digital marketing kpis 2020
Sem kpis
Ap kpis
Progress and performance measurement and evaluation
Evaluation in progress
Intranet performance metrics
Infrastructure performance metrics
Asqp
Engineering performance metrics
Suzuki kasami broadcast algorithm
Characteristics of good performance metrics
Cache performance metrics
Lo performance metrics
Software performance metrics
Treasury performance metrics
Datapower performance metrics
Computer performance metrics