Twenty Second Annual Meeting 20192020 Locally weighted machine
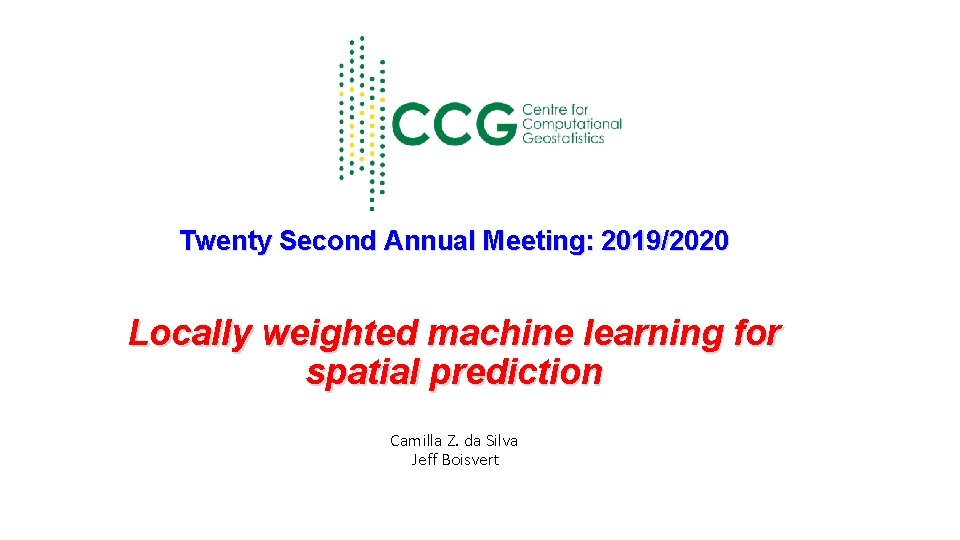
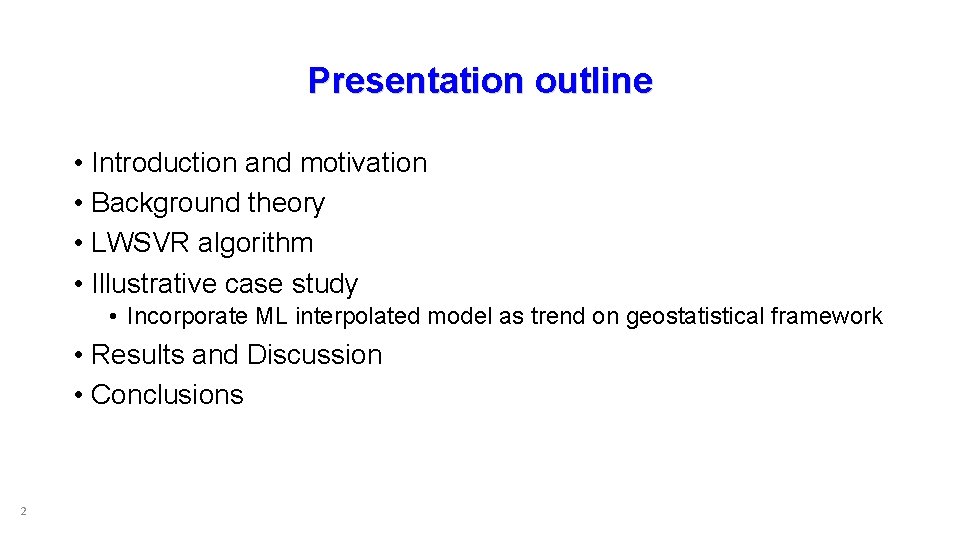
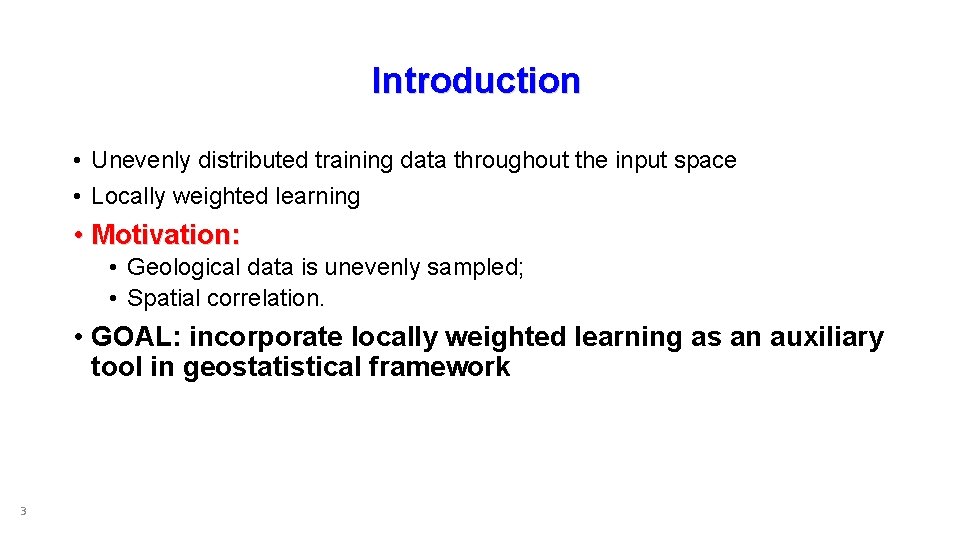
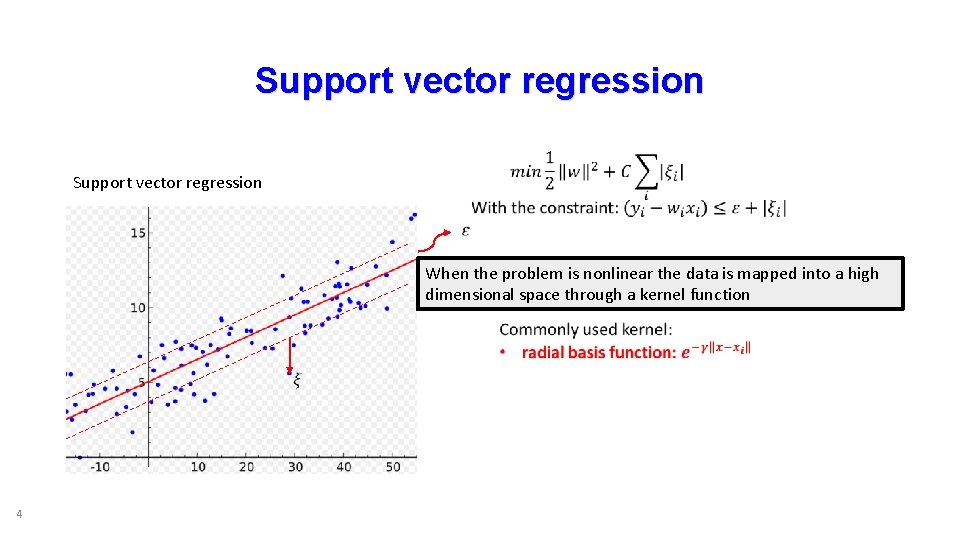
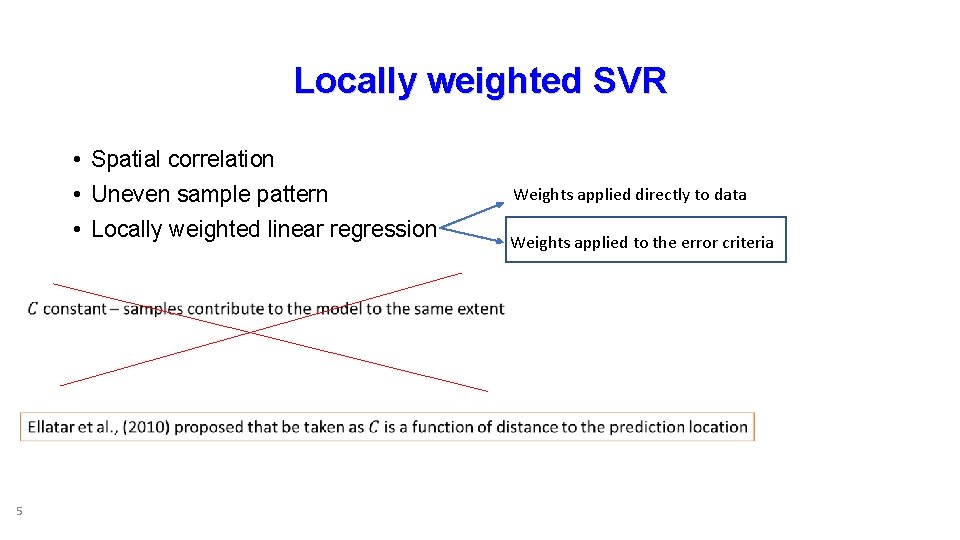
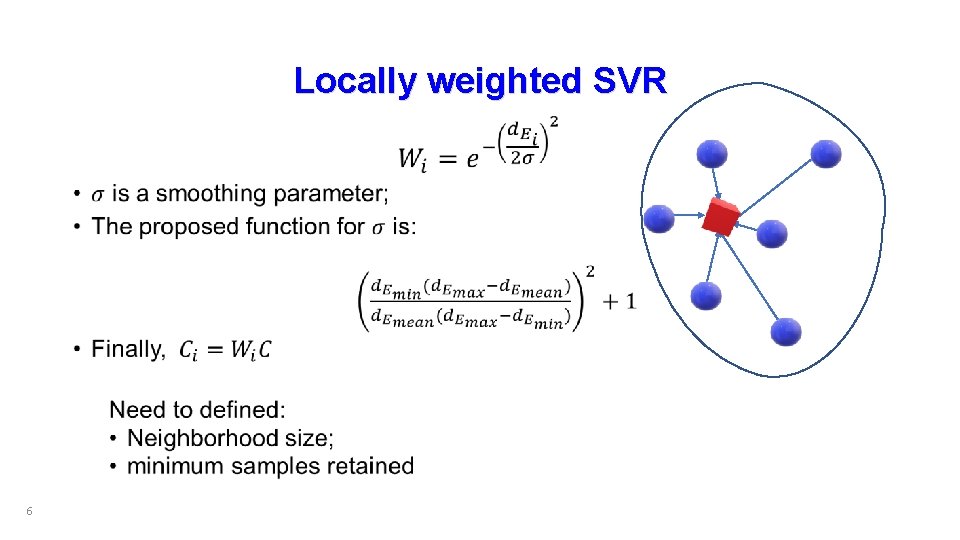
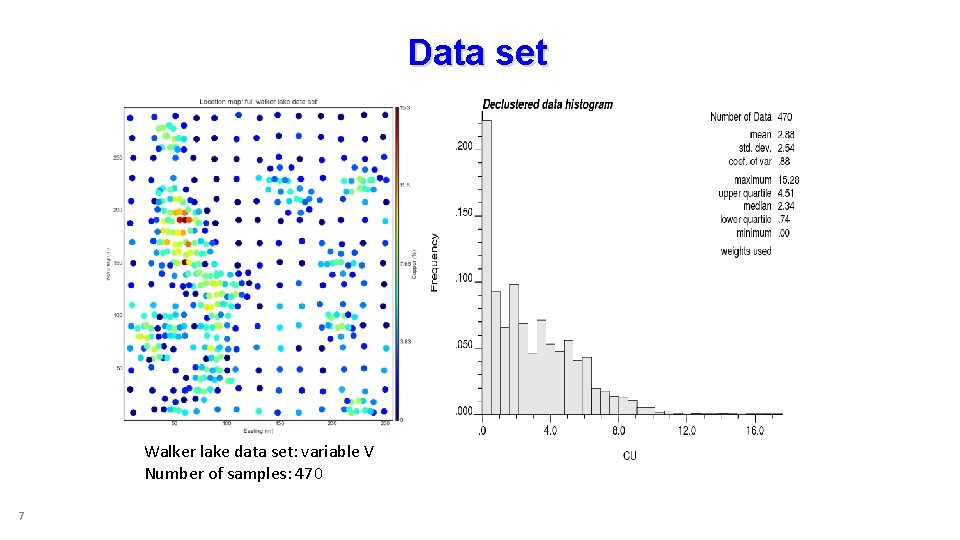
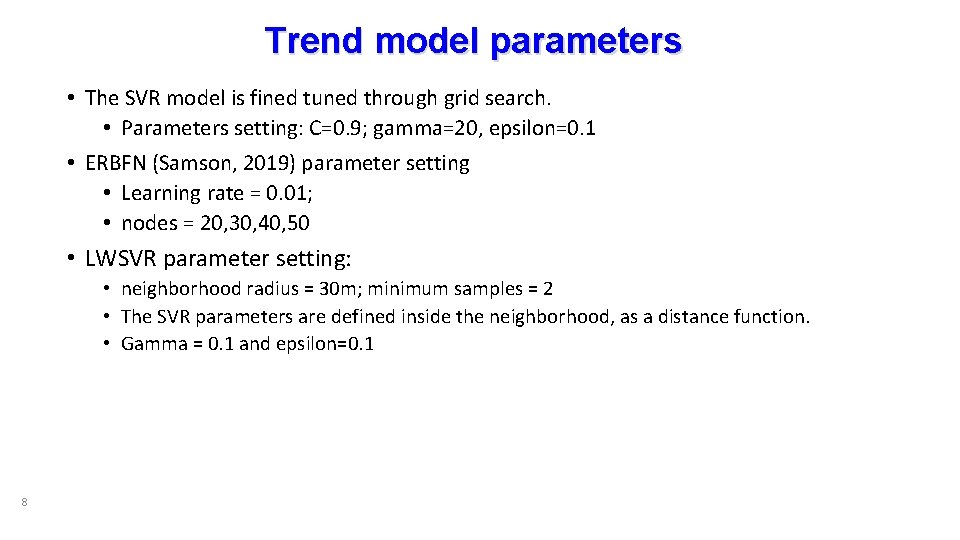
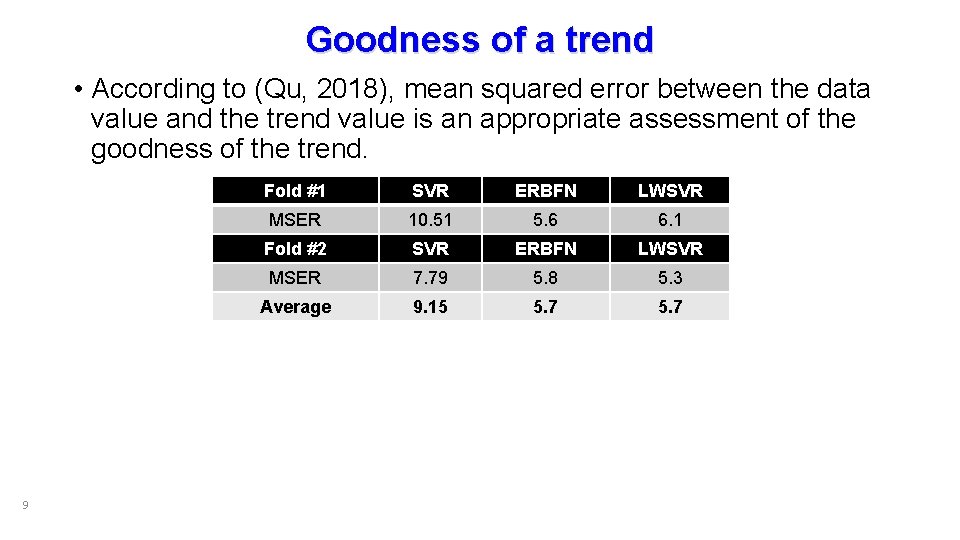
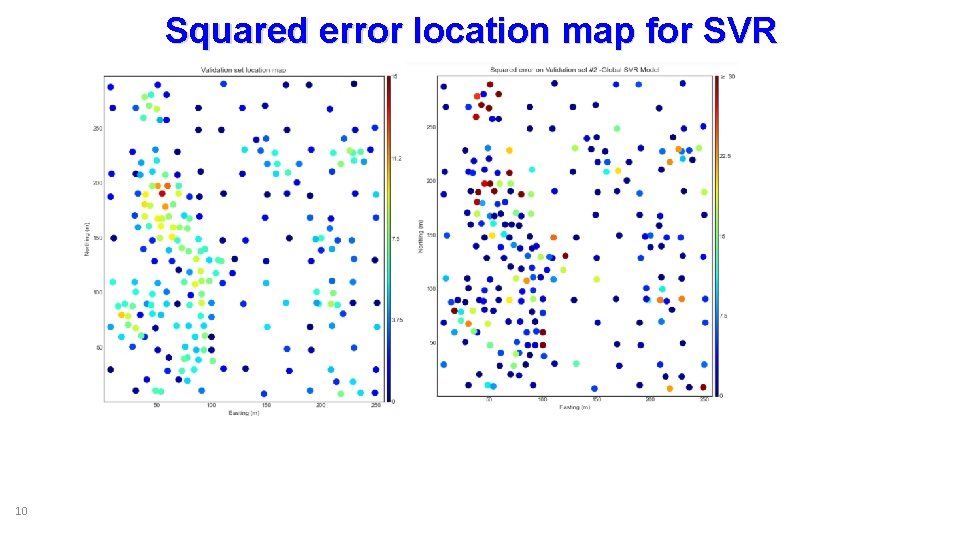
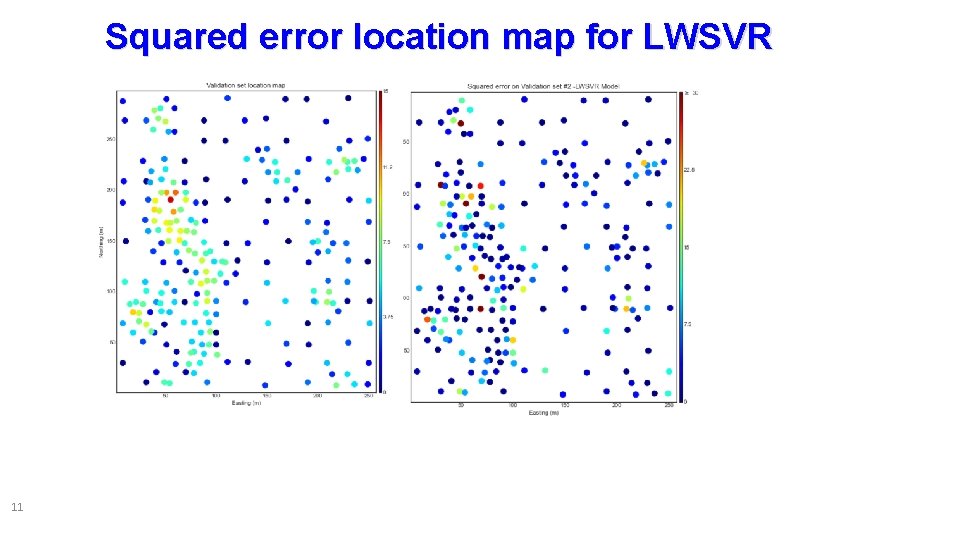
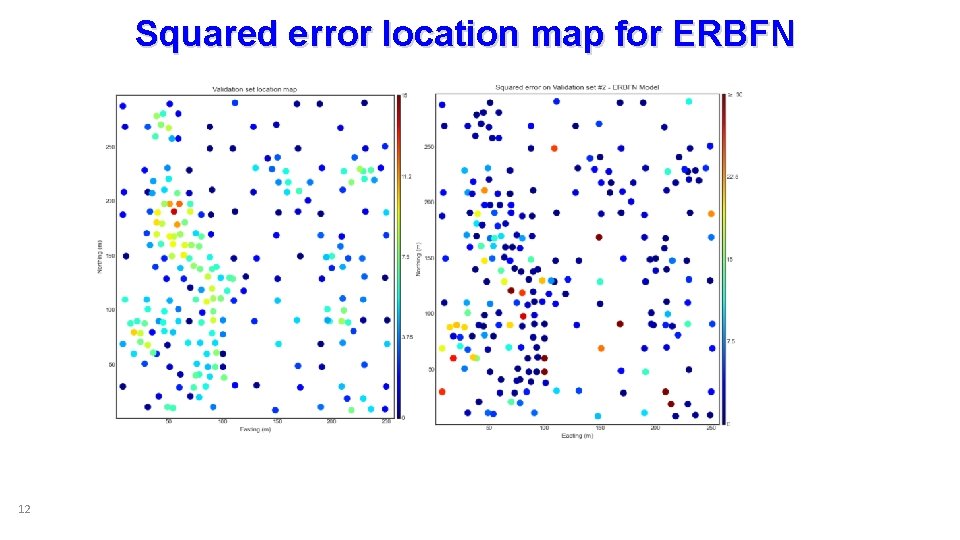
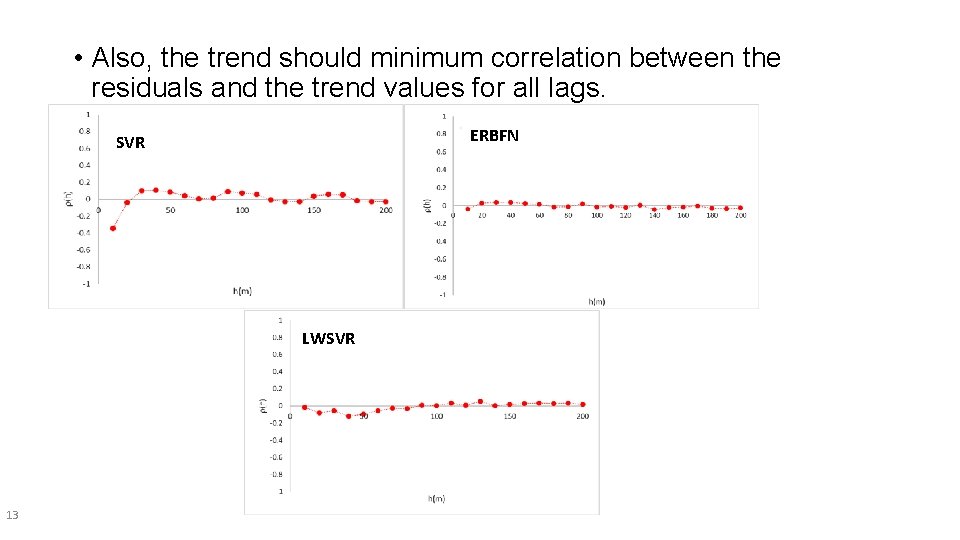
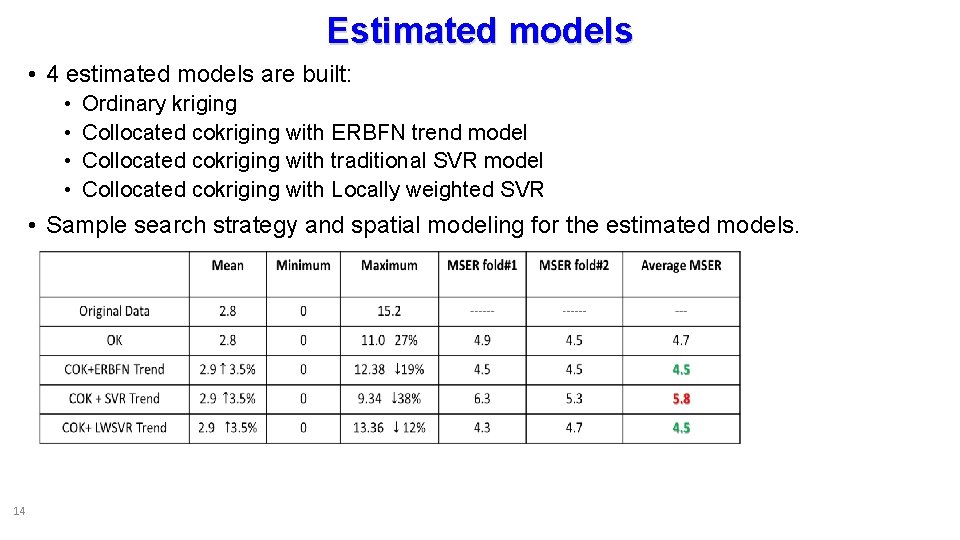
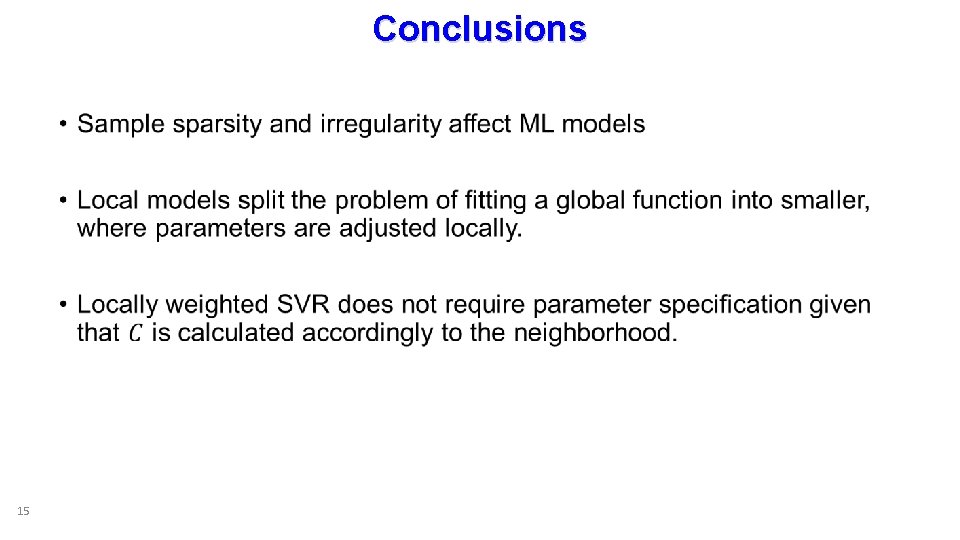
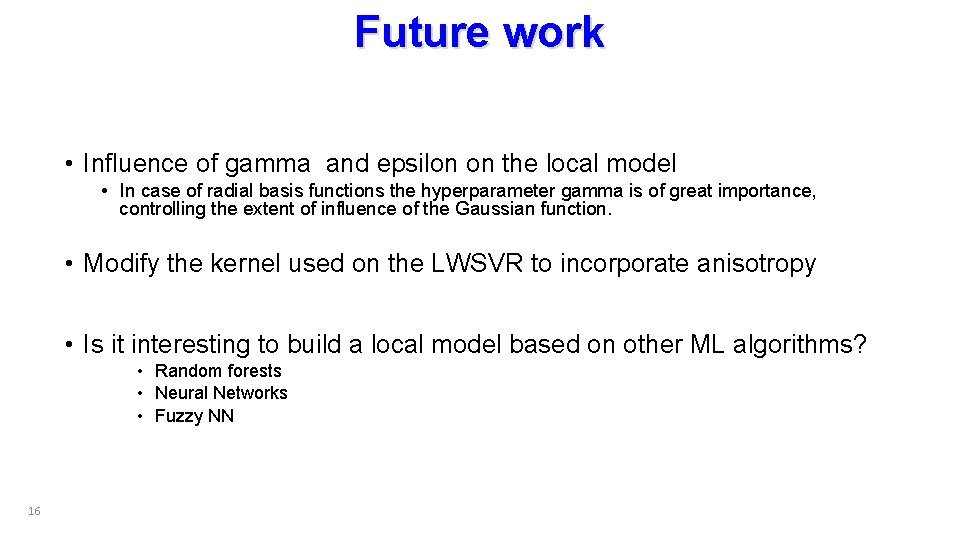
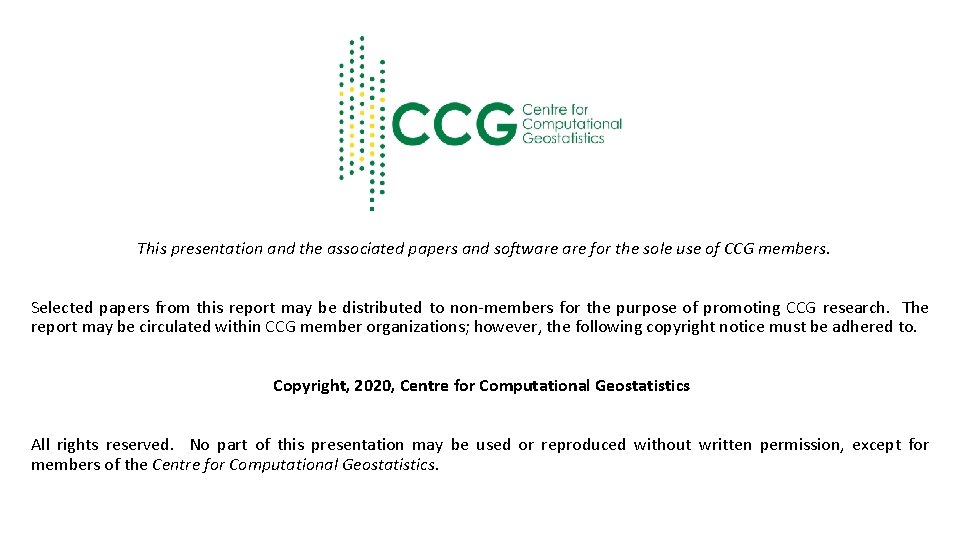
- Slides: 17
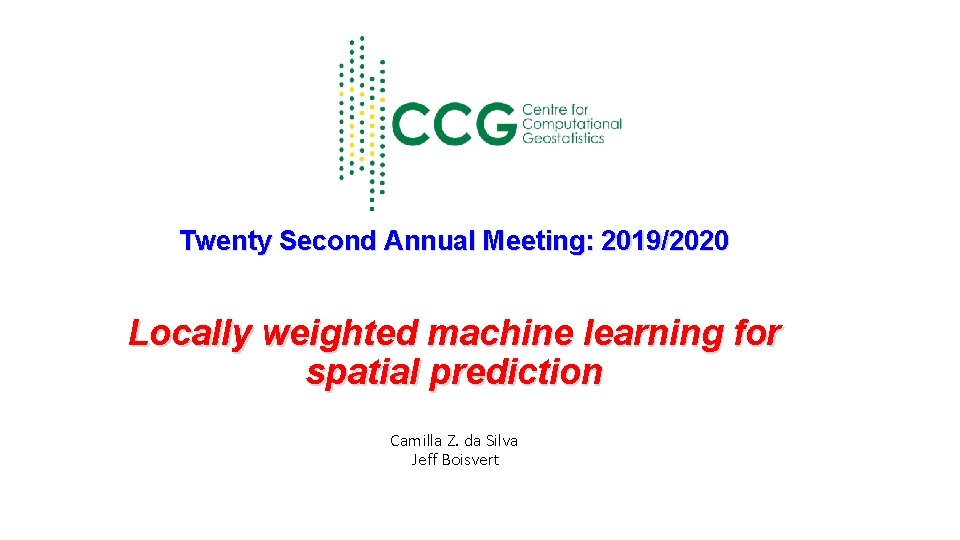
Twenty Second Annual Meeting: 2019/2020 Locally weighted machine learning for spatial prediction Camilla Z. da Silva Jeff Boisvert
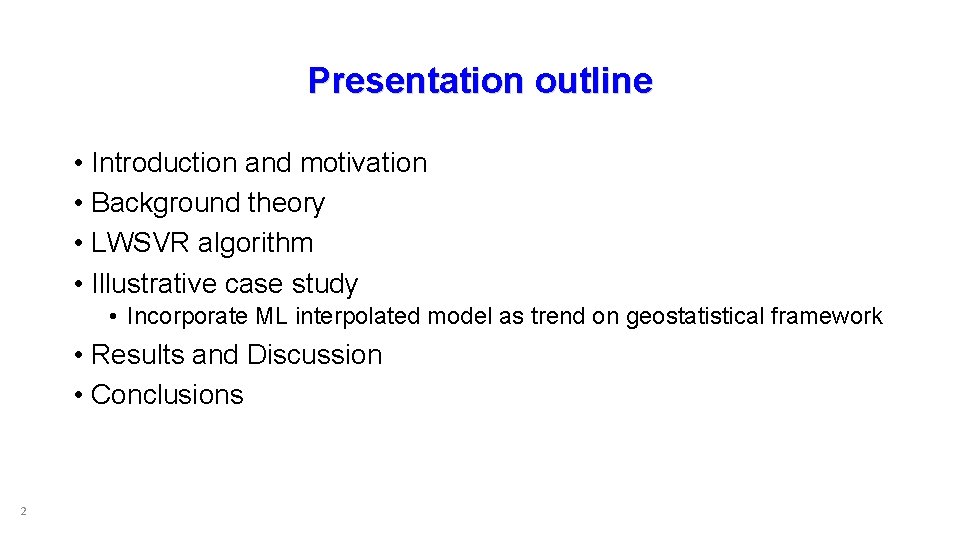
Presentation outline • Introduction and motivation • Background theory • LWSVR algorithm • Illustrative case study • Incorporate ML interpolated model as trend on geostatistical framework • Results and Discussion • Conclusions 2
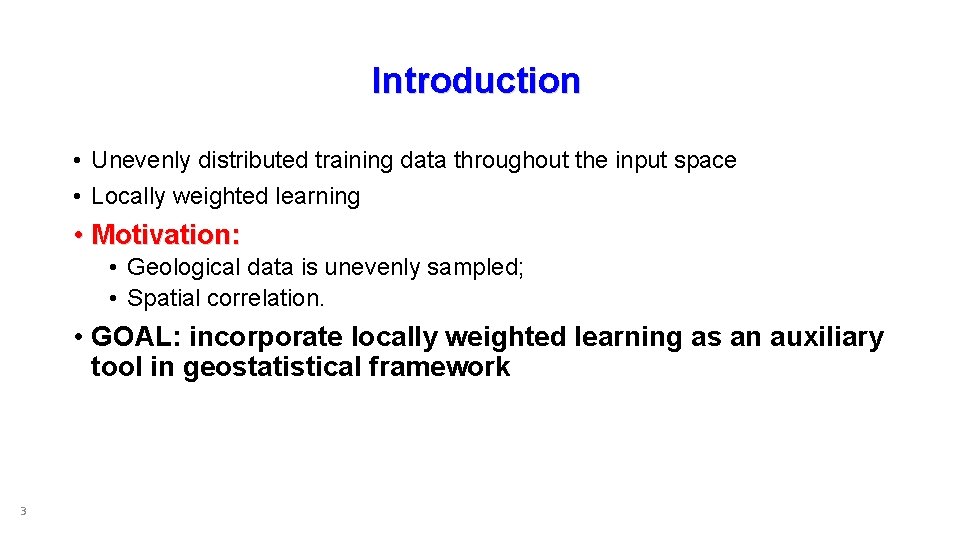
Introduction • Unevenly distributed training data throughout the input space • Locally weighted learning • Motivation: • Geological data is unevenly sampled; • Spatial correlation. • GOAL: incorporate locally weighted learning as an auxiliary tool in geostatistical framework 3
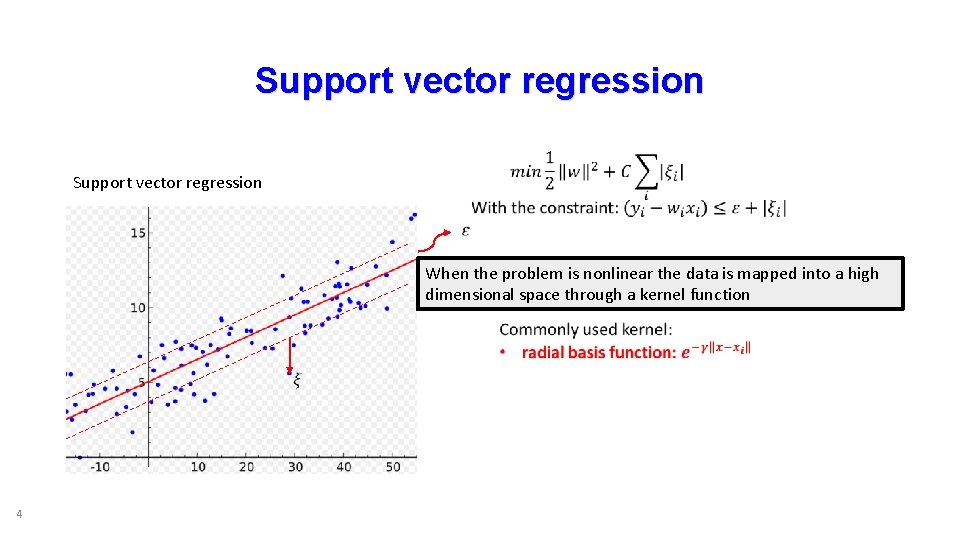
Support vector regression When the problem is nonlinear the data is mapped into a high dimensional space through a kernel function 4
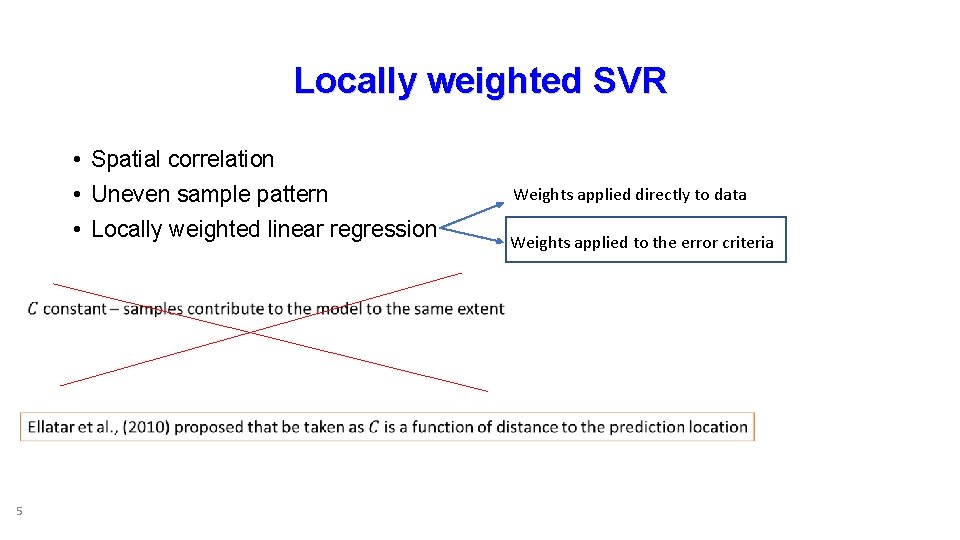
Locally weighted SVR • Spatial correlation • Uneven sample pattern • Locally weighted linear regression 5 Weights applied directly to data Weights applied to the error criteria
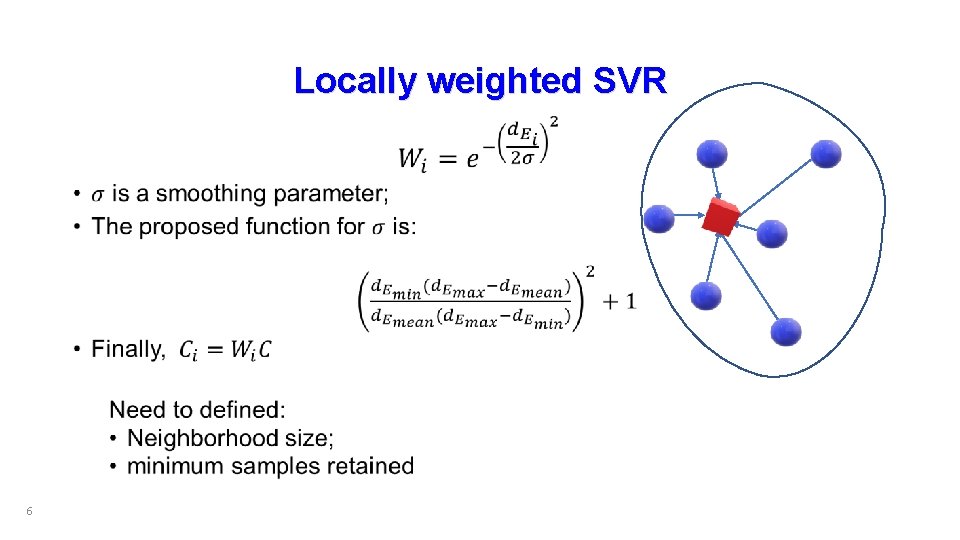
Locally weighted SVR • 6
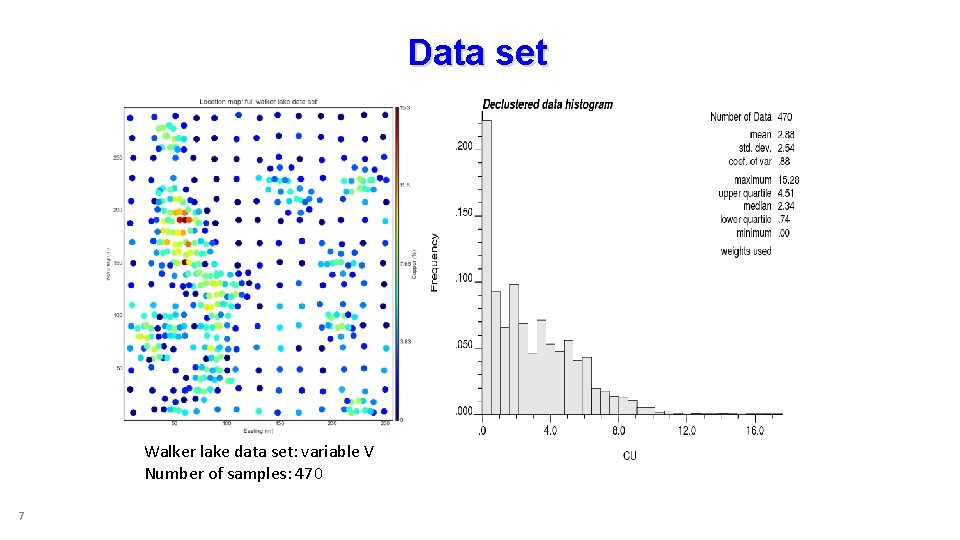
Data set Walker lake data set: variable V Number of samples: 470 7
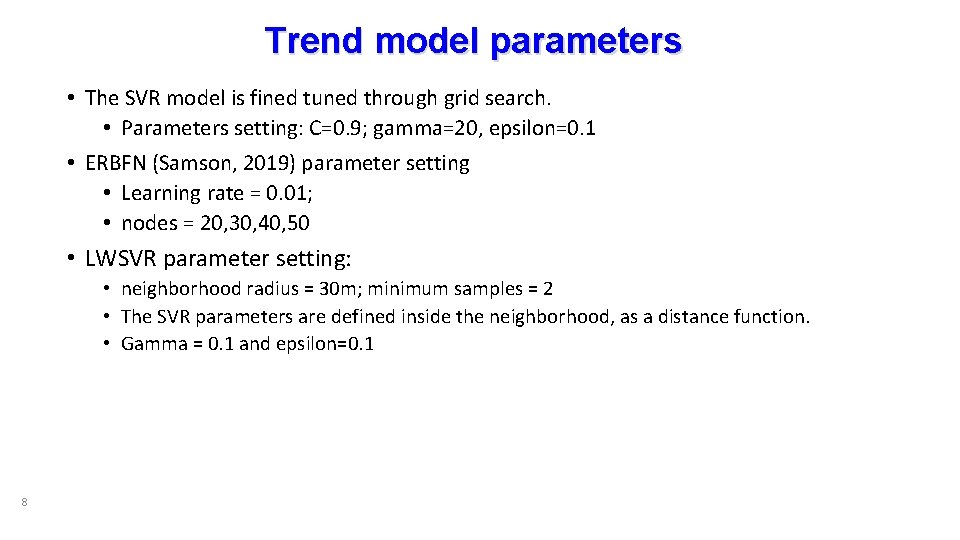
Trend model parameters • The SVR model is fined tuned through grid search. • Parameters setting: C=0. 9; gamma=20, epsilon=0. 1 • ERBFN (Samson, 2019) parameter setting • Learning rate = 0. 01; • nodes = 20, 30, 40, 50 • LWSVR parameter setting: • neighborhood radius = 30 m; minimum samples = 2 • The SVR parameters are defined inside the neighborhood, as a distance function. • Gamma = 0. 1 and epsilon=0. 1 8
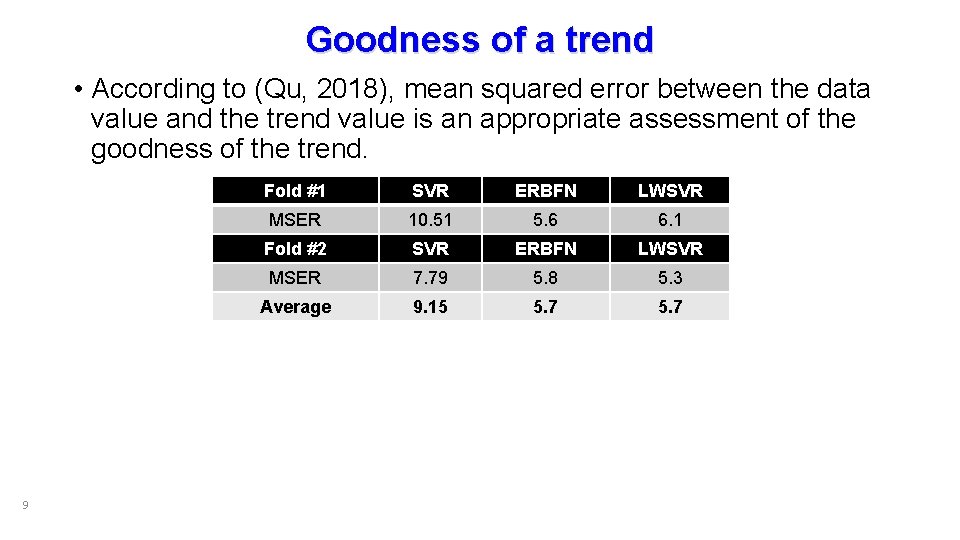
Goodness of a trend • According to (Qu, 2018), mean squared error between the data value and the trend value is an appropriate assessment of the goodness of the trend. 9 Fold #1 SVR ERBFN LWSVR MSER 10. 51 5. 6 6. 1 Fold #2 SVR ERBFN LWSVR MSER 7. 79 5. 8 5. 3 Average 9. 15 5. 7
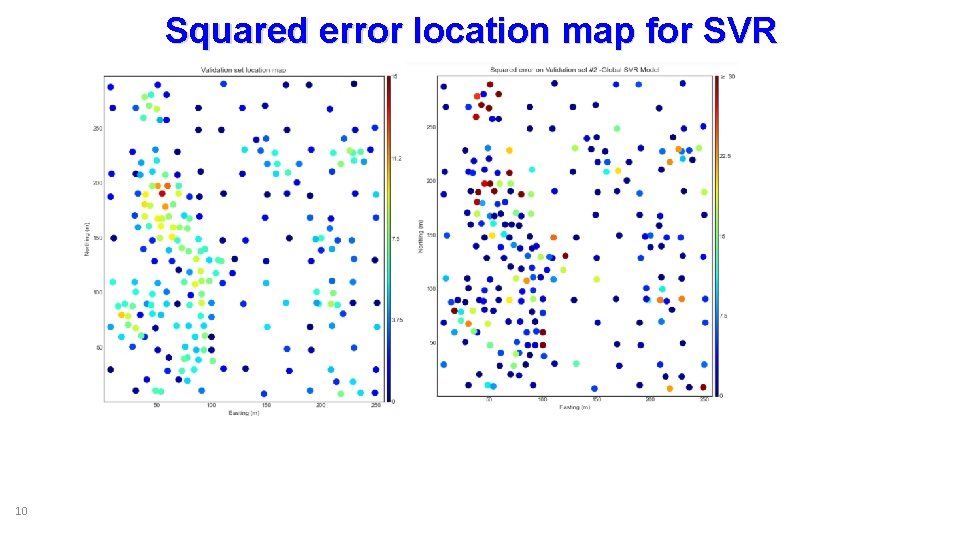
Squared error location map for SVR 10
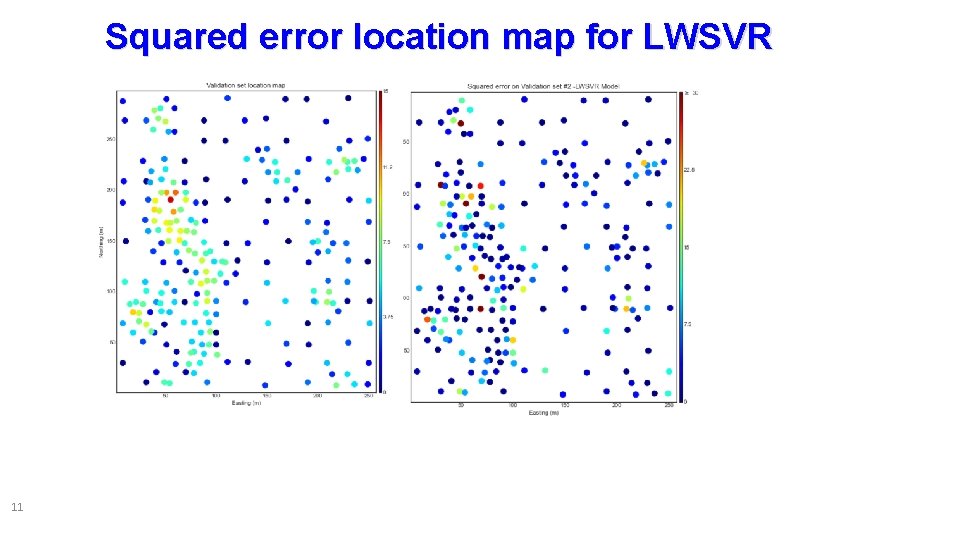
Squared error location map for LWSVR 11
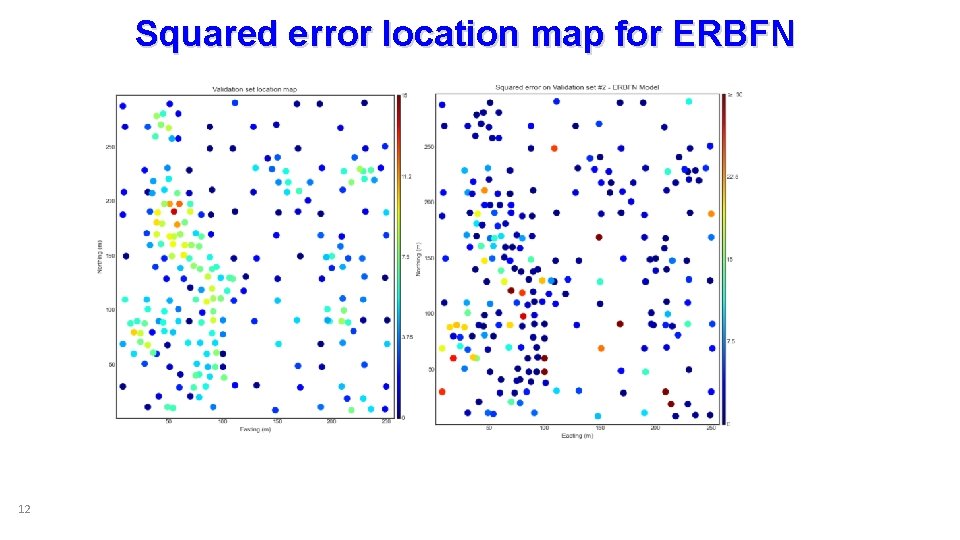
Squared error location map for ERBFN 12
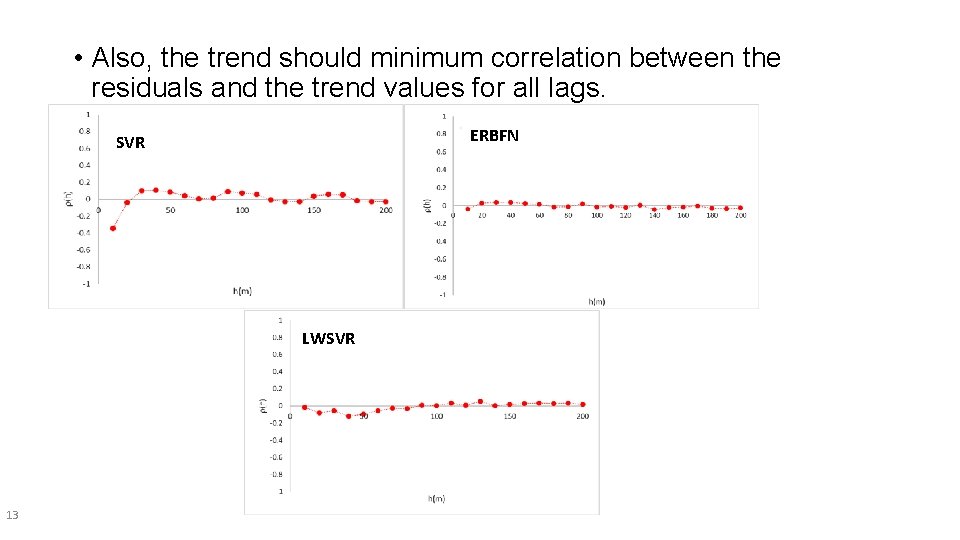
• Also, the trend should minimum correlation between the residuals and the trend values for all lags. ERBFN SVR LWSVR 13
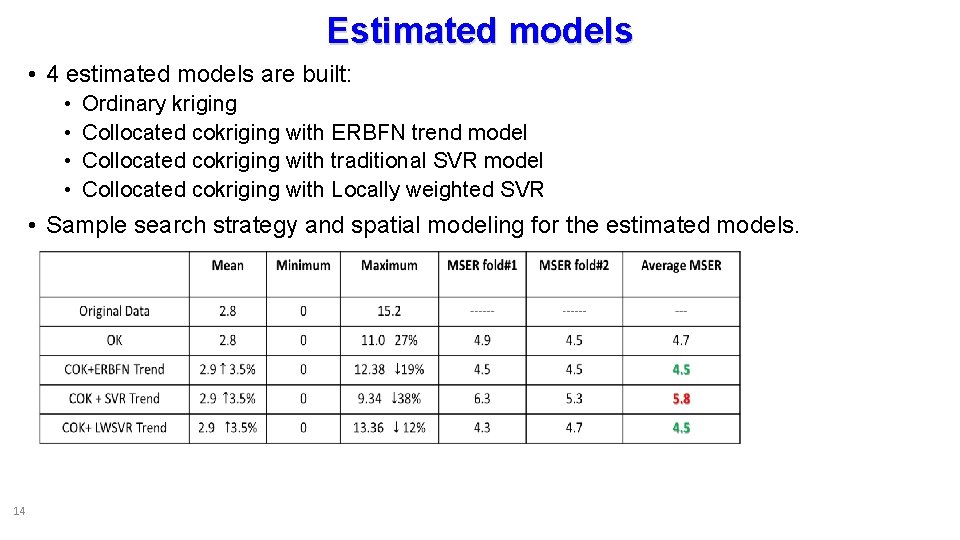
Estimated models • 4 estimated models are built: • • Ordinary kriging Collocated cokriging with ERBFN trend model Collocated cokriging with traditional SVR model Collocated cokriging with Locally weighted SVR • Sample search strategy and spatial modeling for the estimated models. 14
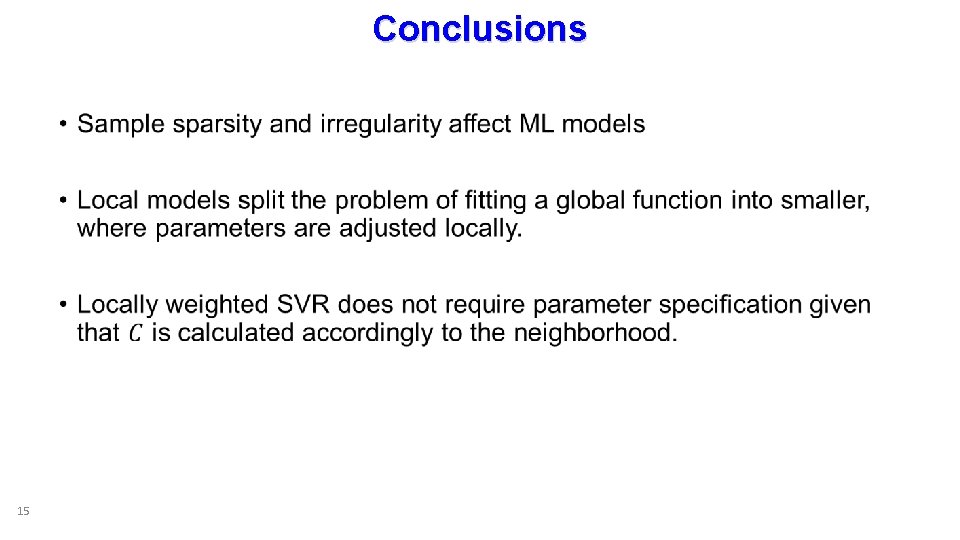
Conclusions • 15
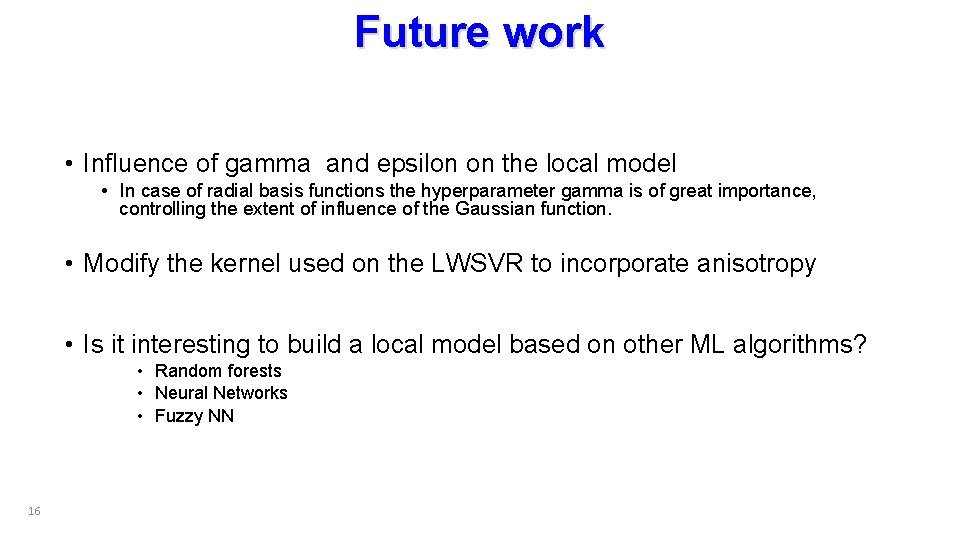
Future work • Influence of gamma and epsilon on the local model • In case of radial basis functions the hyperparameter gamma is of great importance, controlling the extent of influence of the Gaussian function. • Modify the kernel used on the LWSVR to incorporate anisotropy • Is it interesting to build a local model based on other ML algorithms? • Random forests • Neural Networks • Fuzzy NN 16
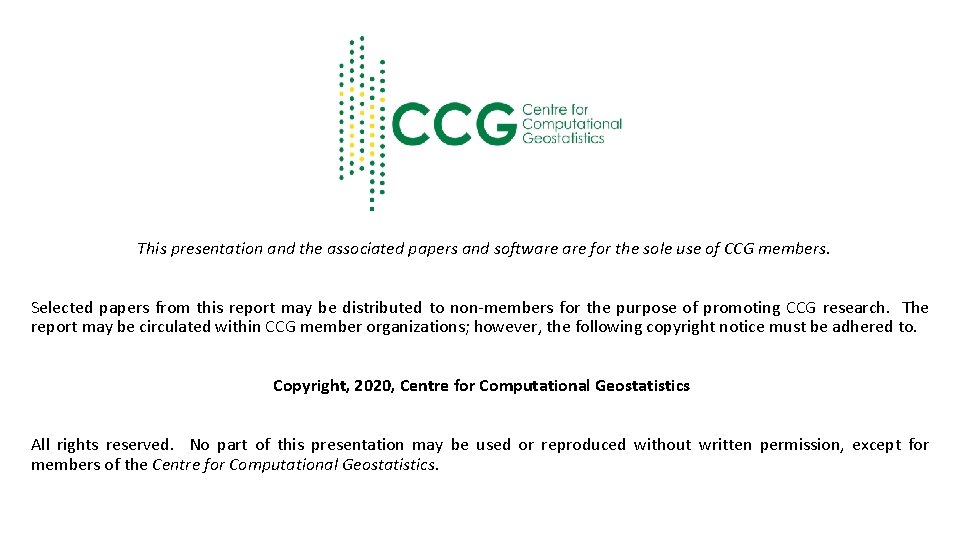
This presentation and the associated papers and software for the sole use of CCG members. Selected papers from this report may be distributed to non-members for the purpose of promoting CCG research. The report may be circulated within CCG member organizations; however, the following copyright notice must be adhered to. Copyright, 2020, Centre for Computational Geostatistics All rights reserved. No part of this presentation may be used or reproduced without written permission, except for members of the Centre for Computational Geostatistics.
Locally weighted regression python
Weighted code in digital electronics
Informs annual meeting
Aupha annual meeting
American psychiatric association annual meeting 2020
Grand lodge of ky annual meeting
Annual theory meeting
Cwemf annual meeting
How to run an annual general meeting
Nrg oncology meeting 2017
Aashto annual meeting 2015
Scts membership
Nrg oncology meeting 2017
American epilepsy society annual meeting 2017
The name tinikling is a reference to birds locally known as
Species that vary locally
Species vary locally
Species vary locally