Twenty Second Annual Meeting 20192020 Developments in Modeling
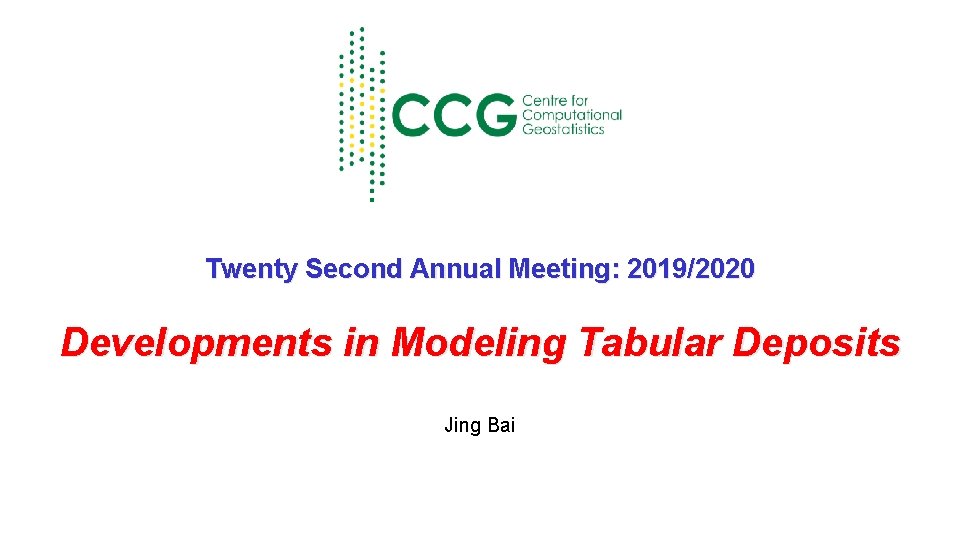
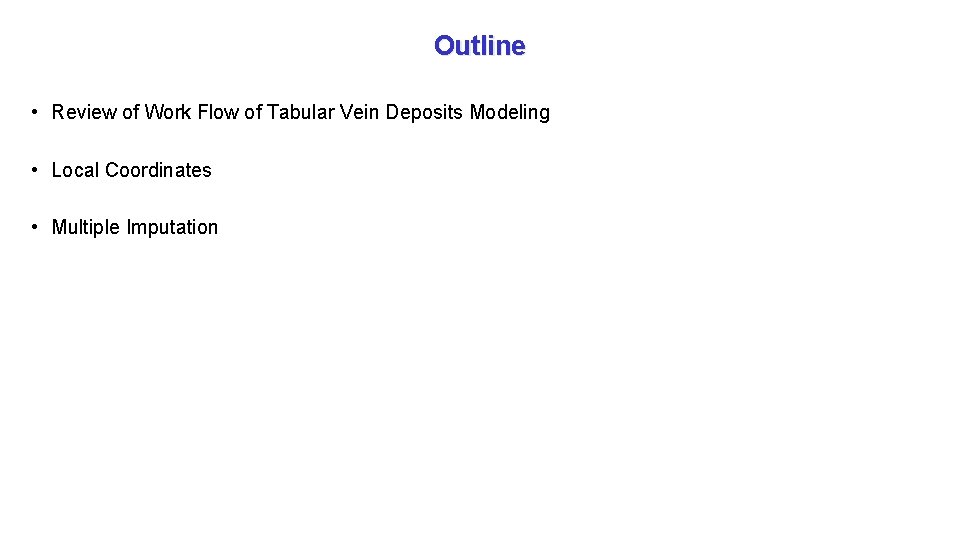
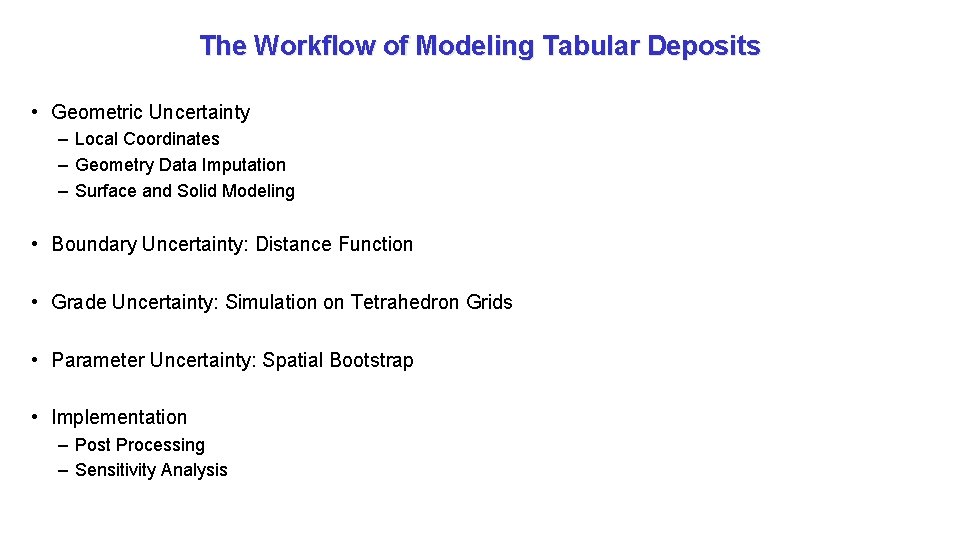
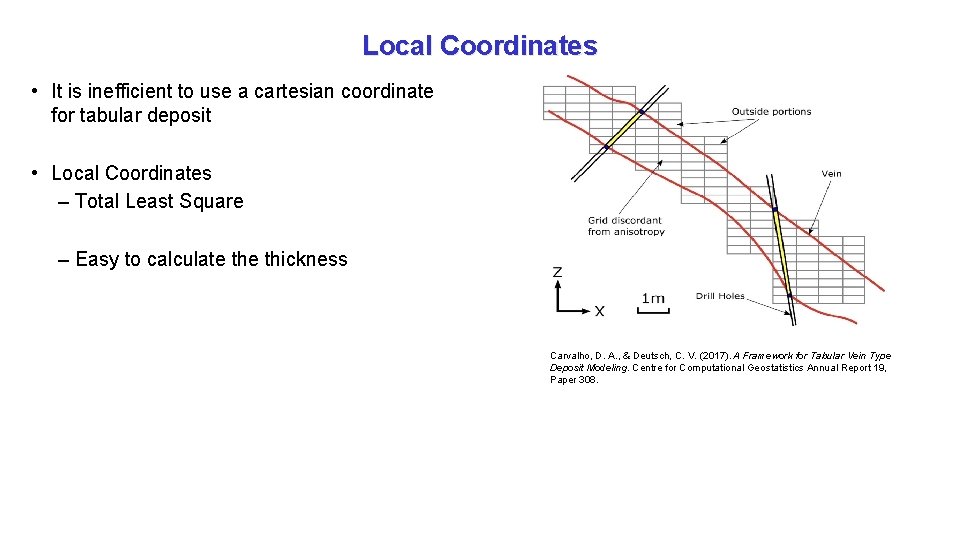
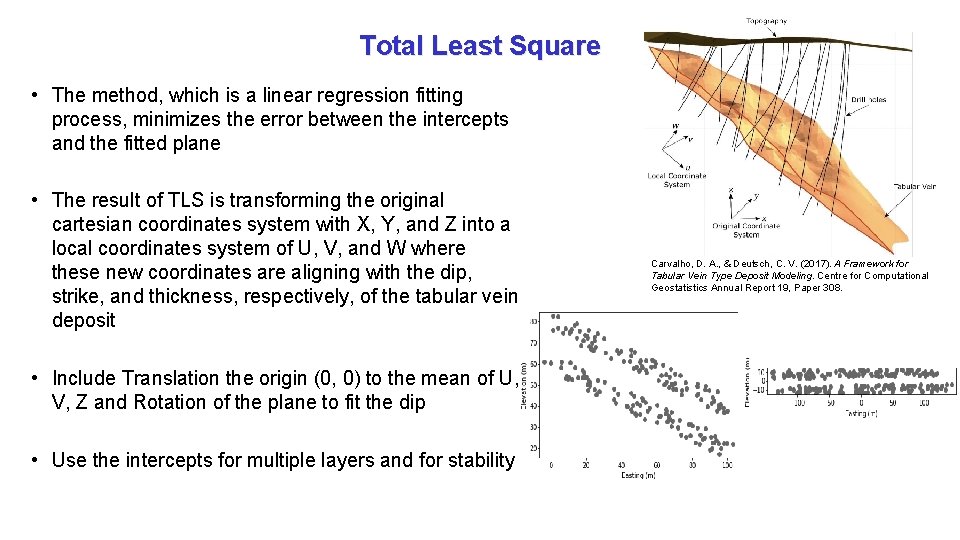
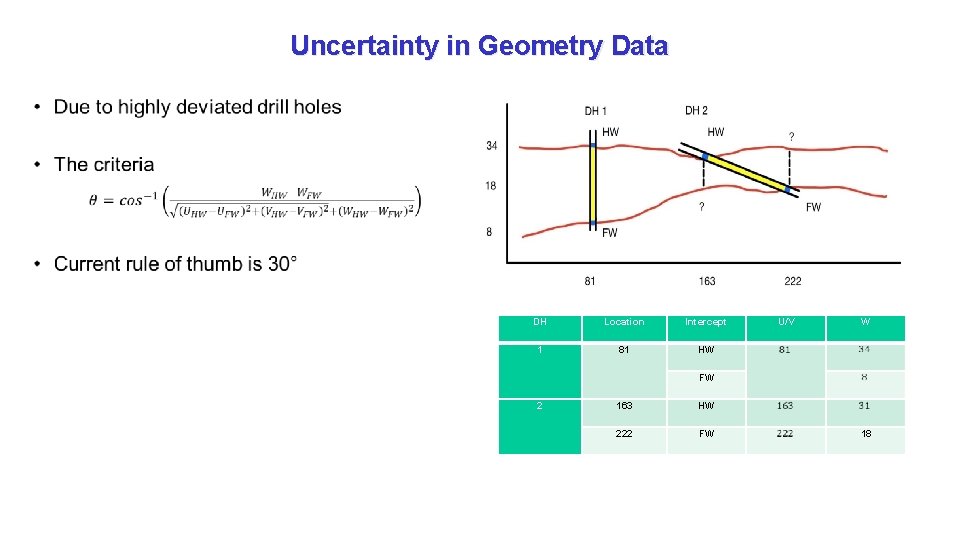
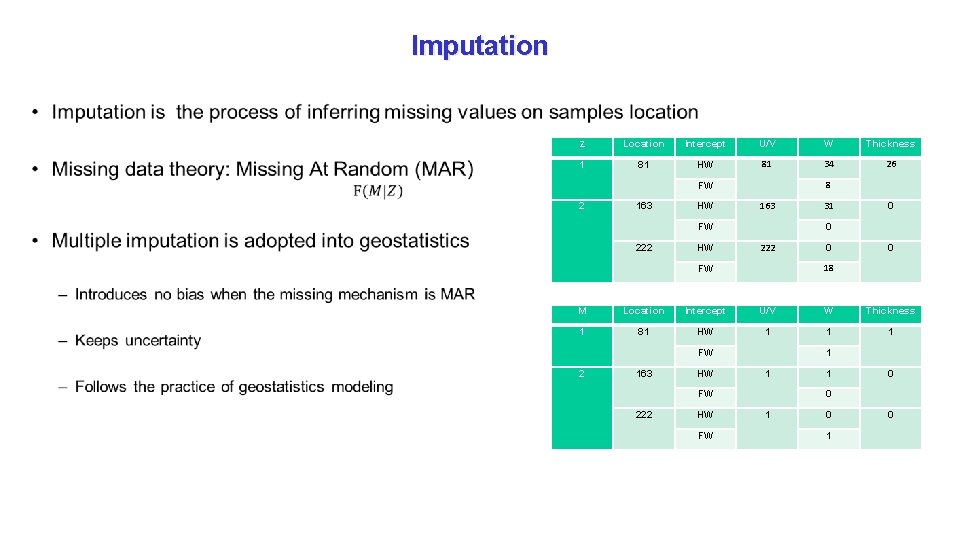
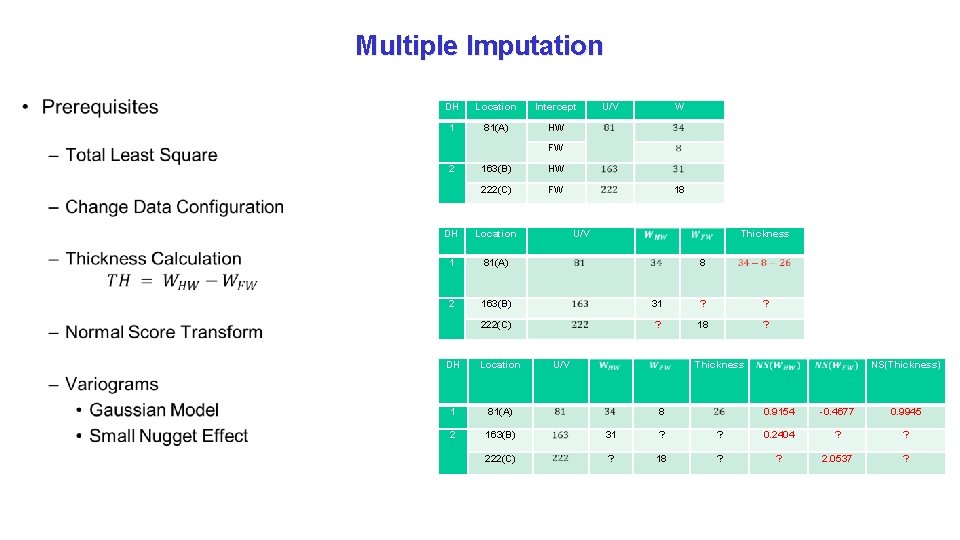
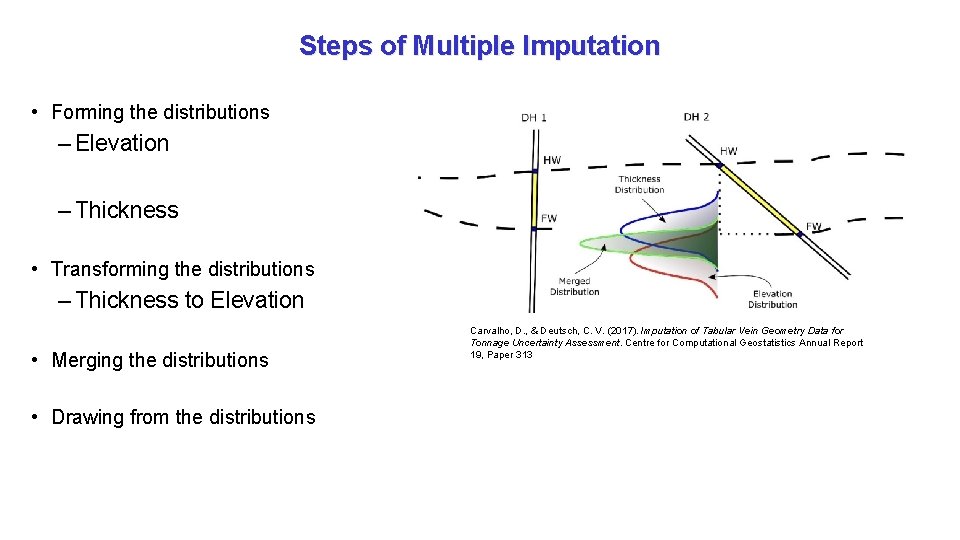
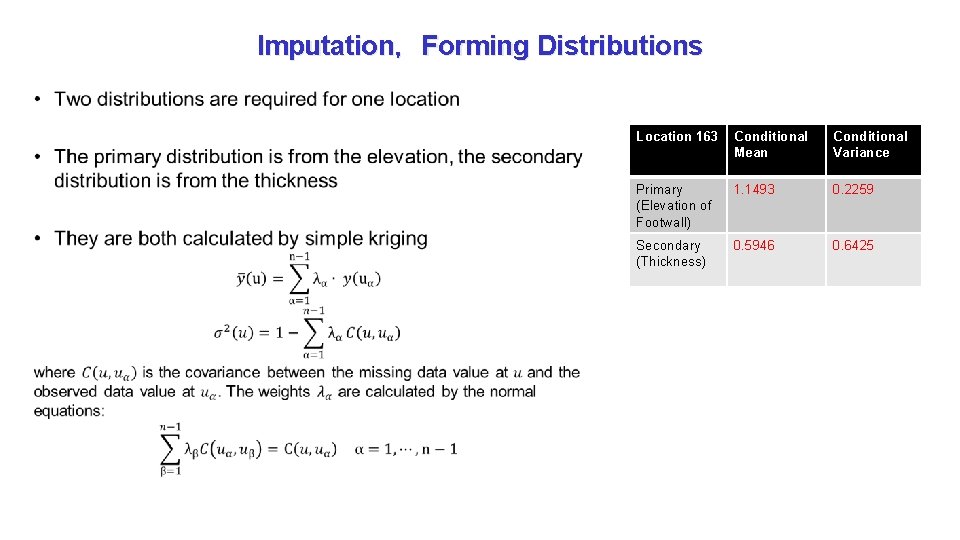
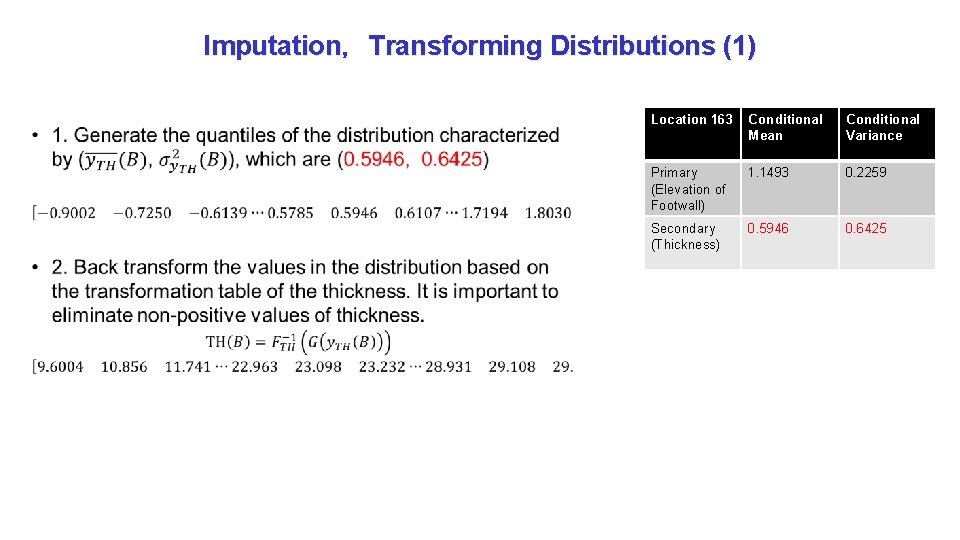
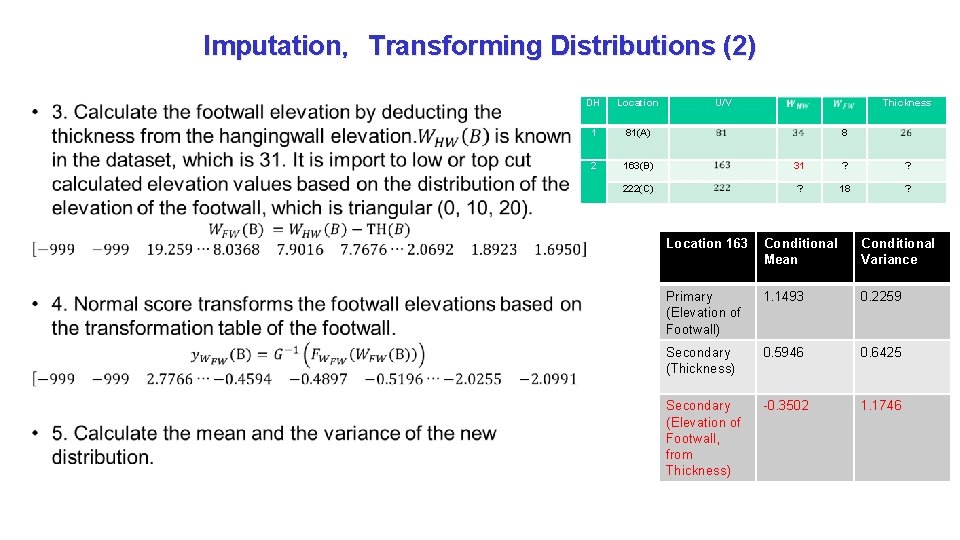
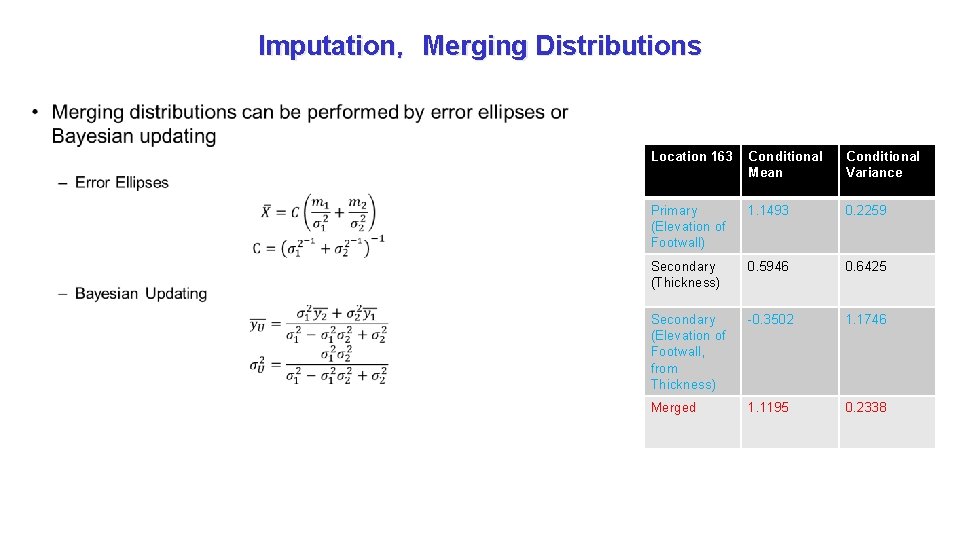
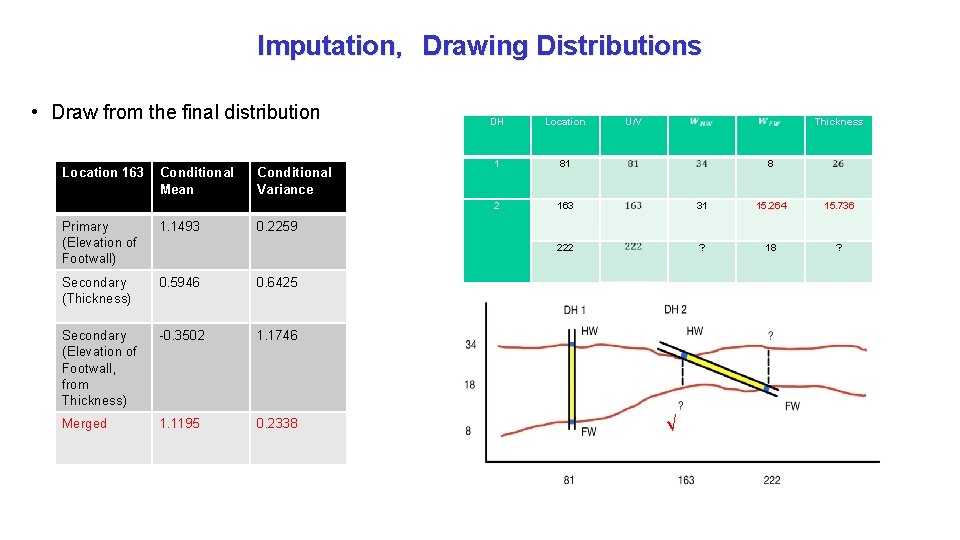
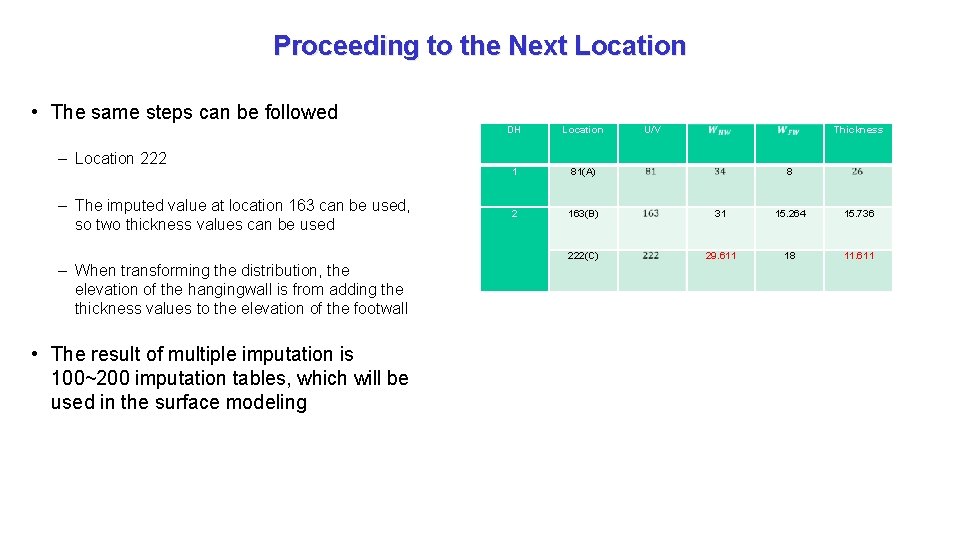
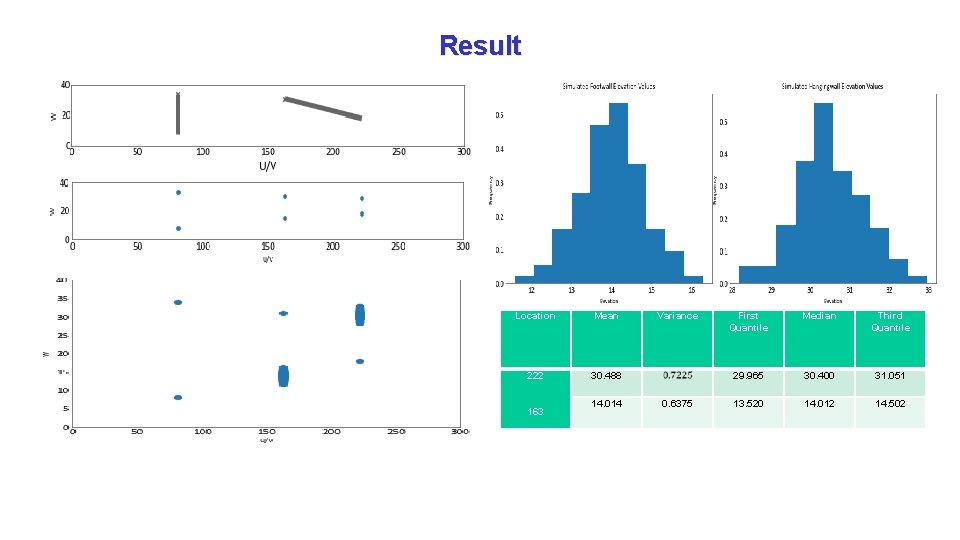
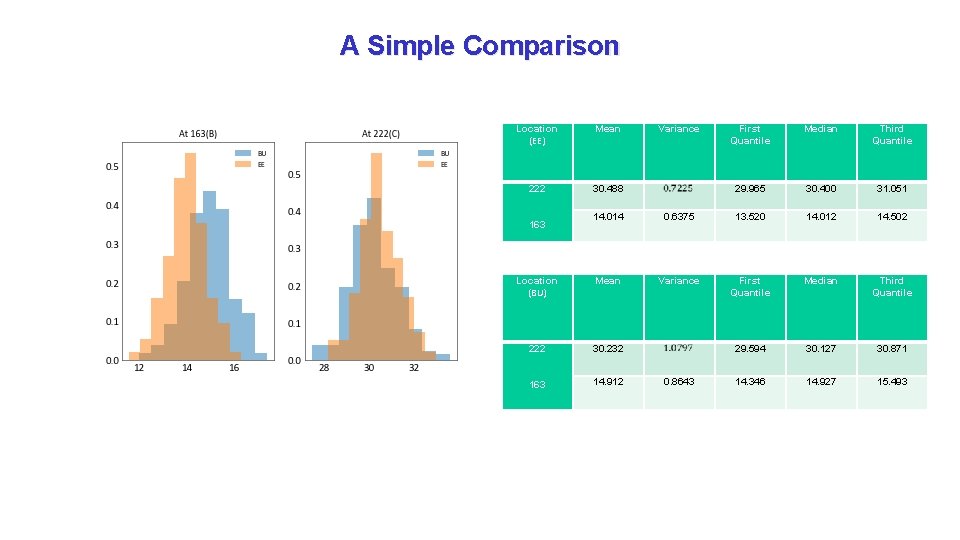
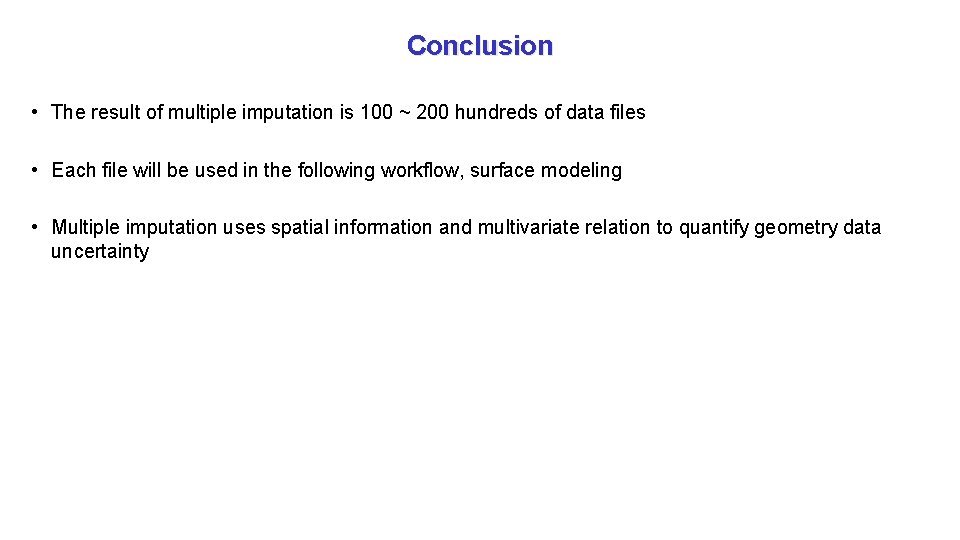
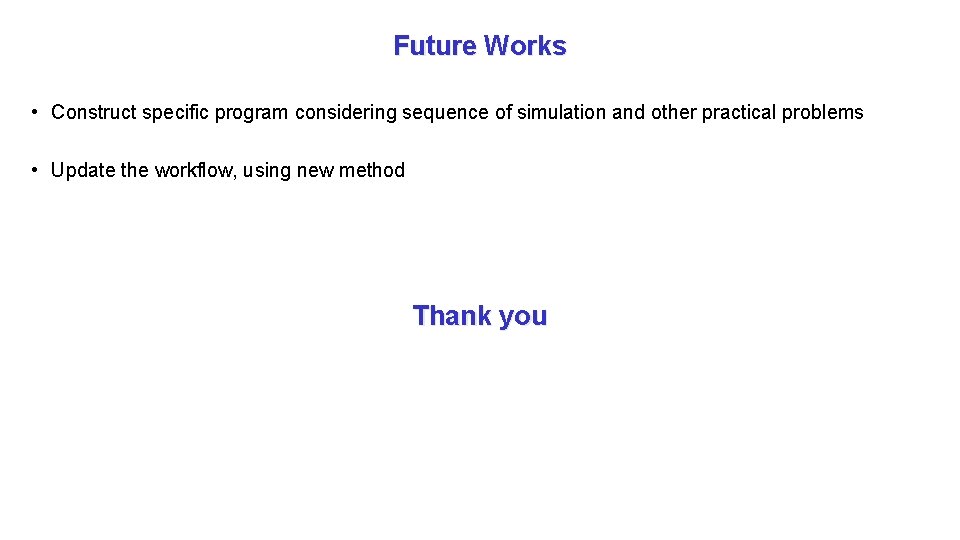
- Slides: 19
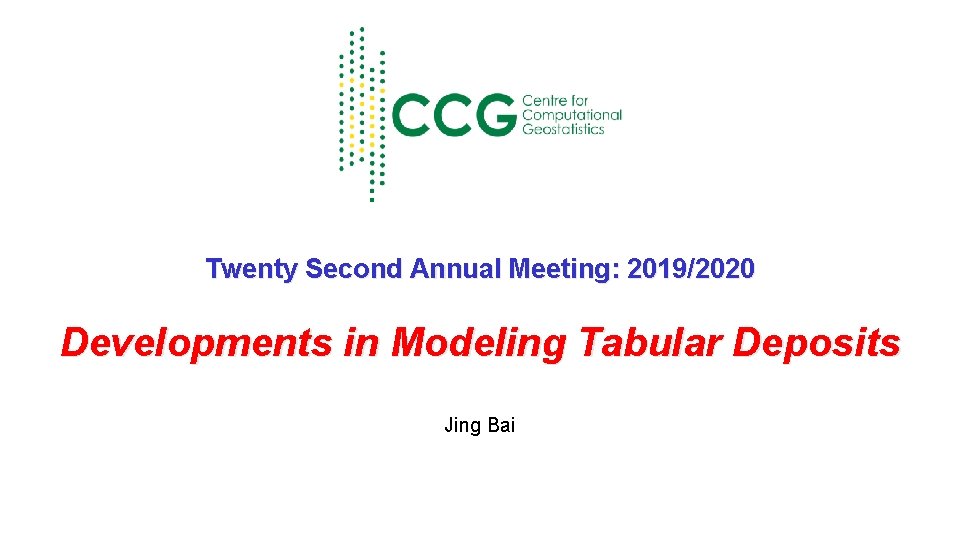
Twenty Second Annual Meeting: 2019/2020 Developments in Modeling Tabular Deposits Jing Bai
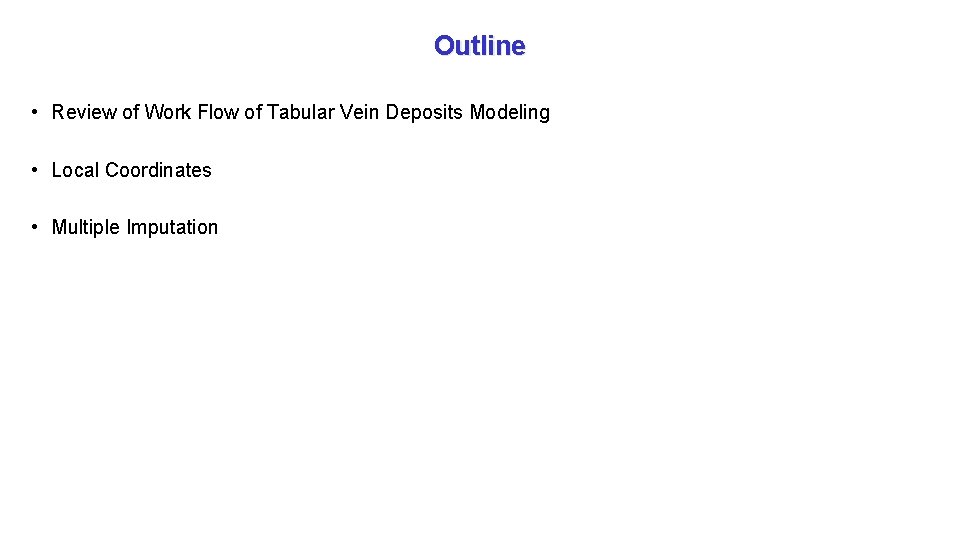
Outline • Review of Work Flow of Tabular Vein Deposits Modeling • Local Coordinates • Multiple Imputation
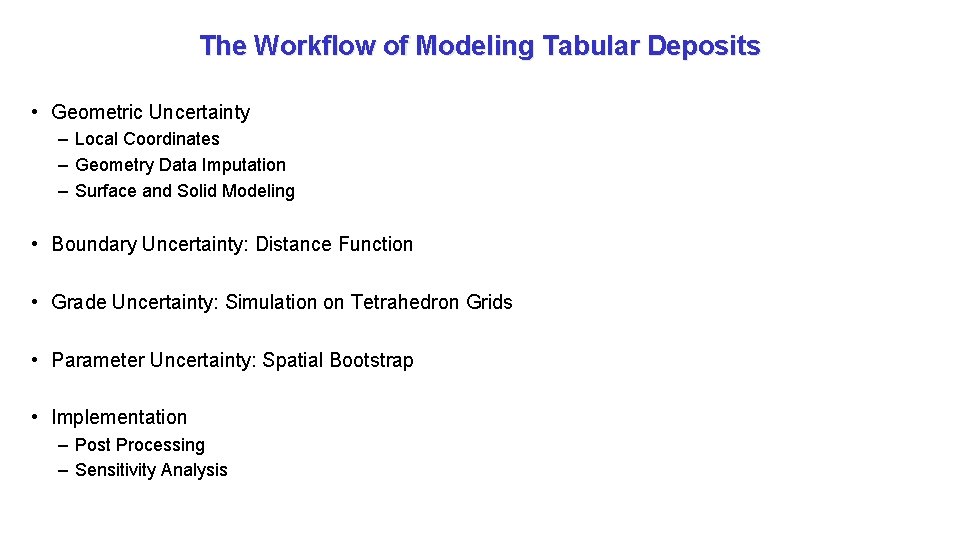
The Workflow of Modeling Tabular Deposits • Geometric Uncertainty – Local Coordinates – Geometry Data Imputation – Surface and Solid Modeling • Boundary Uncertainty: Distance Function • Grade Uncertainty: Simulation on Tetrahedron Grids • Parameter Uncertainty: Spatial Bootstrap • Implementation – Post Processing – Sensitivity Analysis
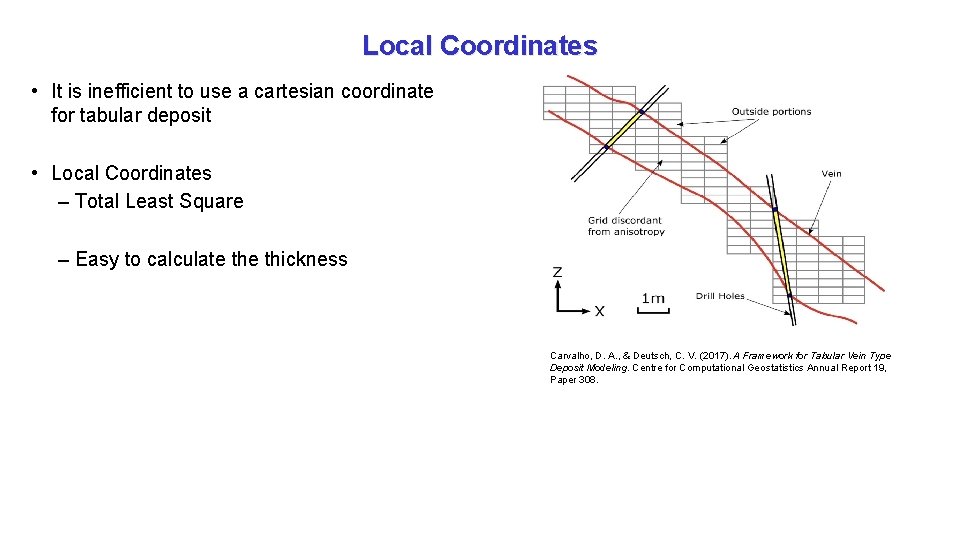
Local Coordinates • It is inefficient to use a cartesian coordinate for tabular deposit • Local Coordinates – Total Least Square – Easy to calculate thickness Carvalho, D. A. , & Deutsch, C. V. (2017). A Framework for Tabular Vein Type Deposit Modeling. Centre for Computational Geostatistics Annual Report 19, Paper 308.
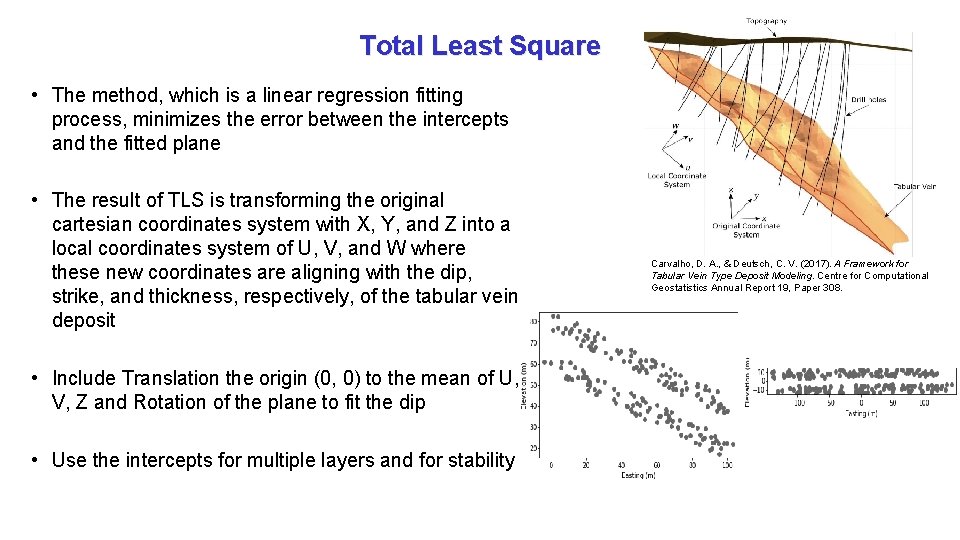
Total Least Square • The method, which is a linear regression fitting process, minimizes the error between the intercepts and the fitted plane • The result of TLS is transforming the original cartesian coordinates system with X, Y, and Z into a local coordinates system of U, V, and W where these new coordinates are aligning with the dip, strike, and thickness, respectively, of the tabular vein deposit • Include Translation the origin (0, 0) to the mean of U, V, Z and Rotation of the plane to fit the dip • Use the intercepts for multiple layers and for stability Carvalho, D. A. , & Deutsch, C. V. (2017). A Framework for Tabular Vein Type Deposit Modeling. Centre for Computational Geostatistics Annual Report 19, Paper 308.
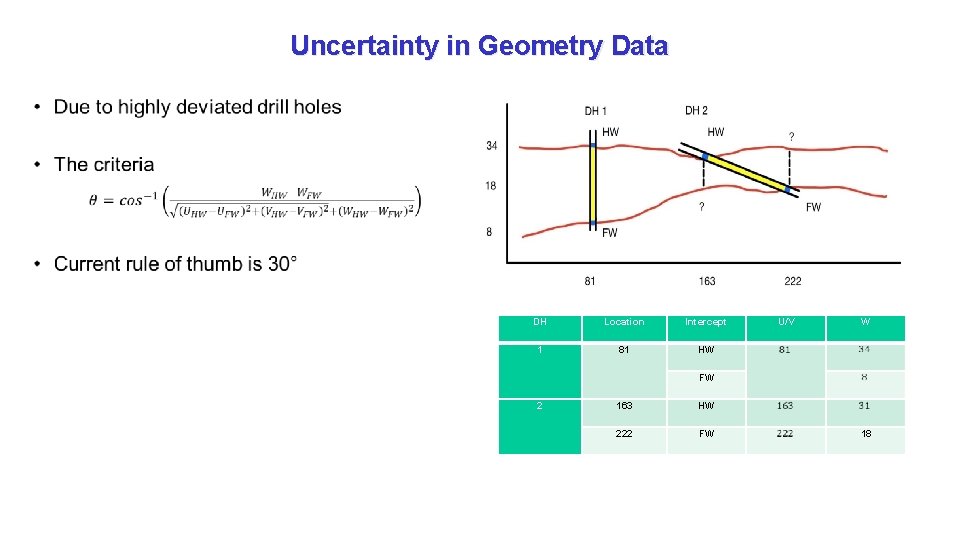
Uncertainty in Geometry Data DH Location Intercept 1 81 HW U/V W FW 2 163 HW 222 FW 18
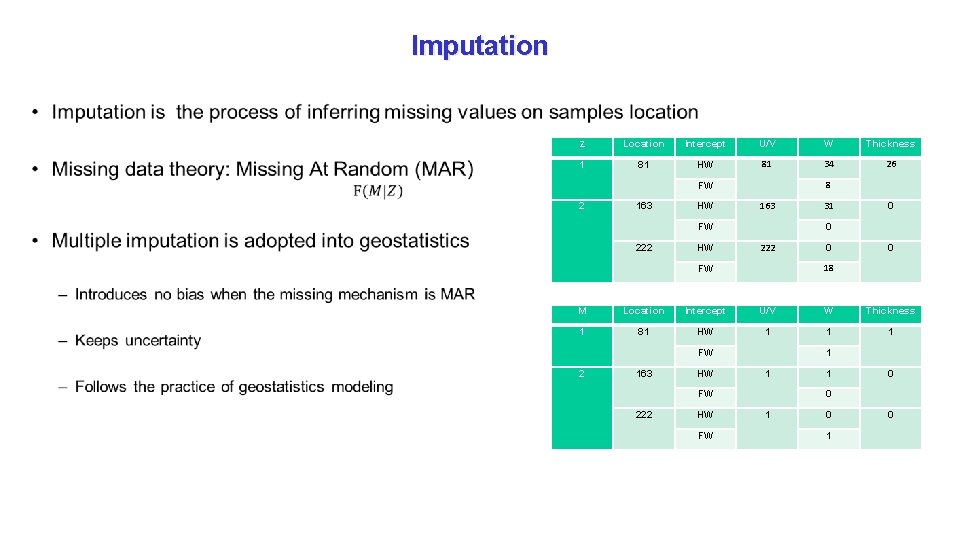
Imputation • Z Location Intercept U/V W Thickness 1 81 HW 81 34 26 8 FW 2 163 HW 163 FW 222 HW 31 0 0 222 0 0 18 FW M Location Intercept U/V W Thickness 1 81 HW 1 1 1 FW 2 163 HW 1 1 FW 222 HW FW 1 0 0 1 0
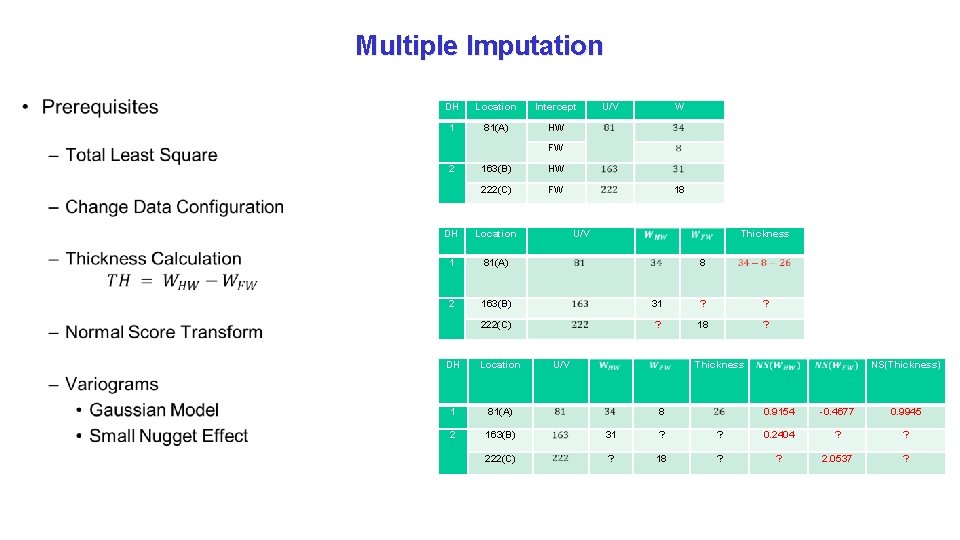
Multiple Imputation • DH Location Intercept 1 81(A) HW U/V W FW 2 163(B) HW 222(C) FW 18 DH Location U/V Thickness 1 81(A) 2 163(B) 31 ? ? 222(C) ? 18 ? 8 DH Location U/V Thickness 1 81(A) 2 163(B) 31 ? 222(C) ? 18 8 NS(Thickness) 0. 9154 -0. 4677 0. 9945 ? 0. 2404 ? ? 2. 0537 ?
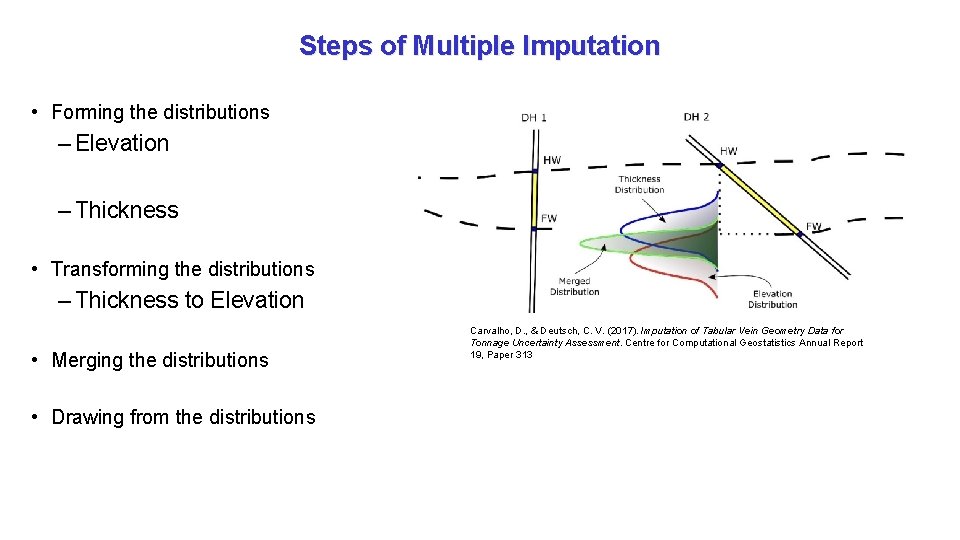
Steps of Multiple Imputation • Forming the distributions – Elevation – Thickness • Transforming the distributions – Thickness to Elevation • Merging the distributions • Drawing from the distributions Carvalho, D. , & Deutsch, C. V. (2017). Imputation of Tabular Vein Geometry Data for Tonnage Uncertainty Assessment. Centre for Computational Geostatistics Annual Report 19, Paper 313
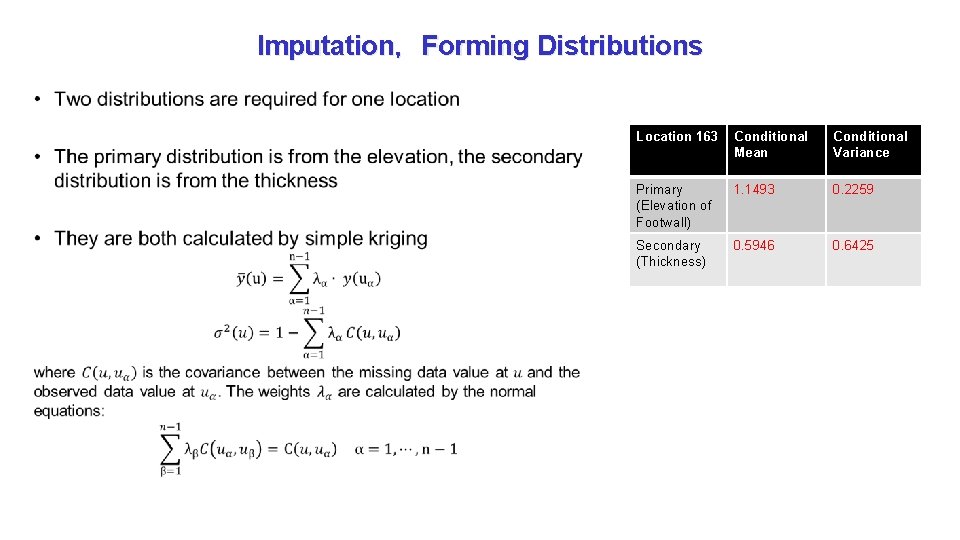
Imputation,Forming Distributions • Location 163 Conditional Mean Conditional Variance Primary (Elevation of Footwall) 1. 1493 0. 2259 Secondary (Thickness) 0. 5946 0. 6425
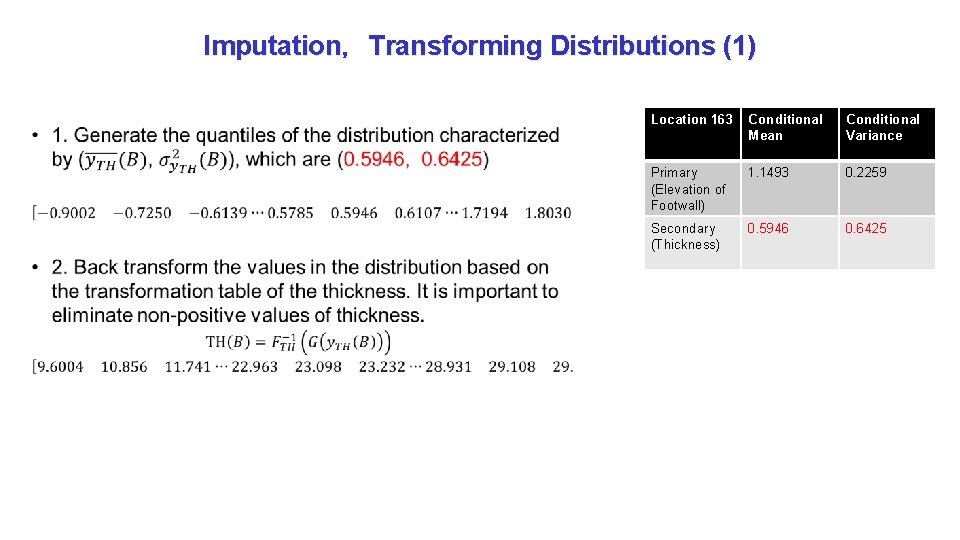
Imputation,Transforming Distributions (1) • Location 163 Conditional Mean Conditional Variance Primary (Elevation of Footwall) 1. 1493 0. 2259 Secondary (Thickness) 0. 5946 0. 6425
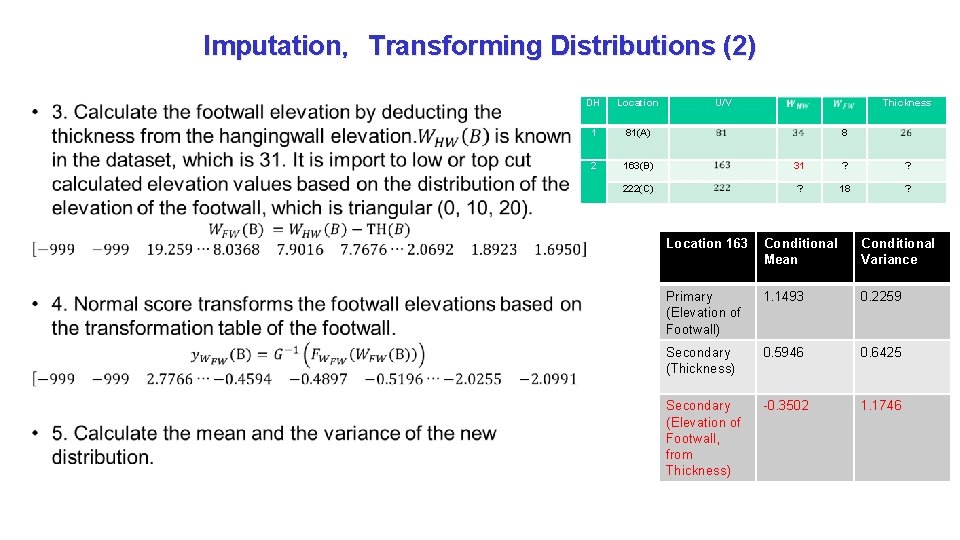
Imputation,Transforming Distributions (2) • DH Location U/V Thickness 1 81(A) 2 163(B) 31 ? ? 222(C) ? 18 ? 8 Location 163 Conditional Mean Conditional Variance Primary (Elevation of Footwall) 1. 1493 0. 2259 Secondary (Thickness) 0. 5946 0. 6425 Secondary (Elevation of Footwall, from Thickness) -0. 3502 1. 1746
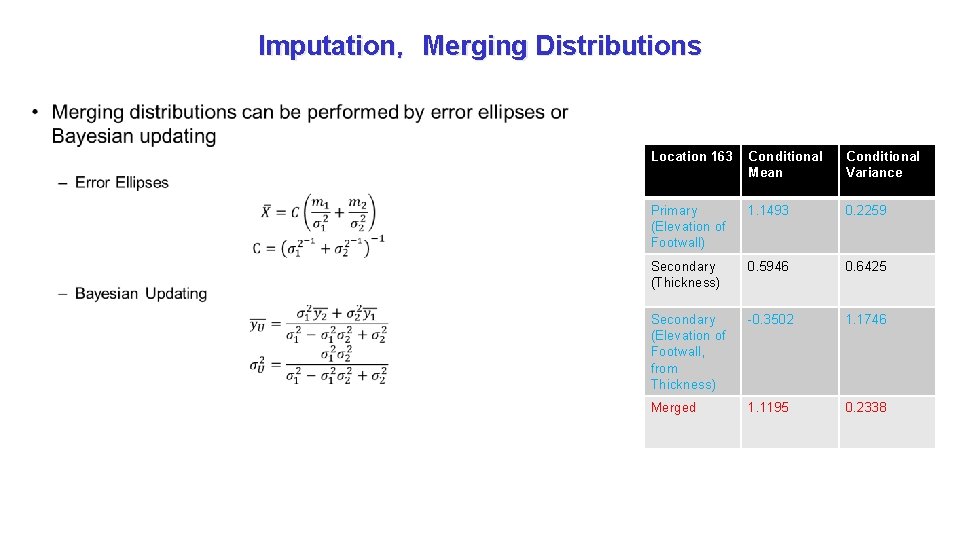
Imputation,Merging Distributions • Location 163 Conditional Mean Conditional Variance Primary (Elevation of Footwall) 1. 1493 0. 2259 Secondary (Thickness) 0. 5946 0. 6425 Secondary (Elevation of Footwall, from Thickness) -0. 3502 1. 1746 Merged 1. 1195 0. 2338
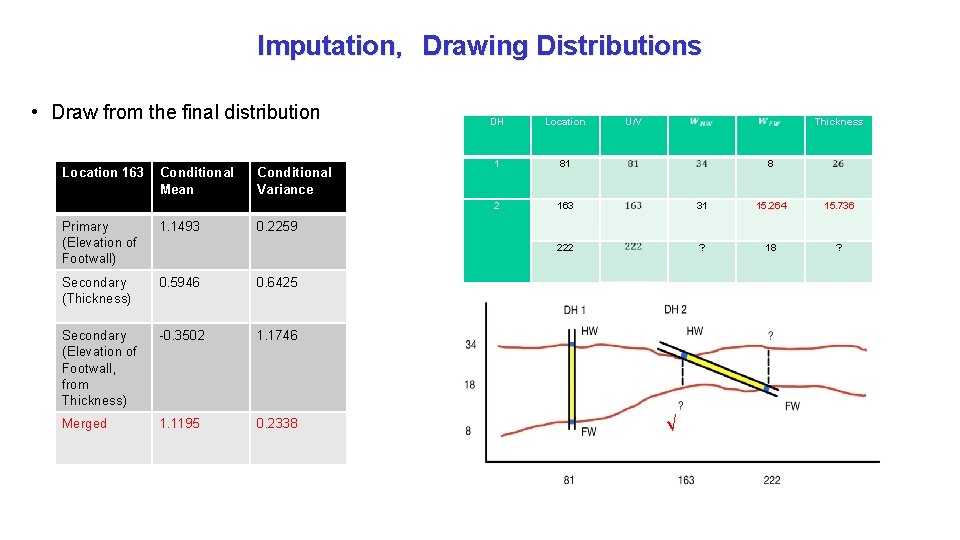
Imputation,Drawing Distributions • Draw from the final distribution Location 163 Conditional Mean Conditional Variance Primary (Elevation of Footwall) 1. 1493 Secondary (Thickness) 0. 5946 0. 6425 Secondary (Elevation of Footwall, from Thickness) -0. 3502 1. 1746 Merged 1. 1195 0. 2338 DH Location U/V Thickness 1 81 2 163 31 15. 264 15. 736 222 ? 18 ? 8 0. 2259 √
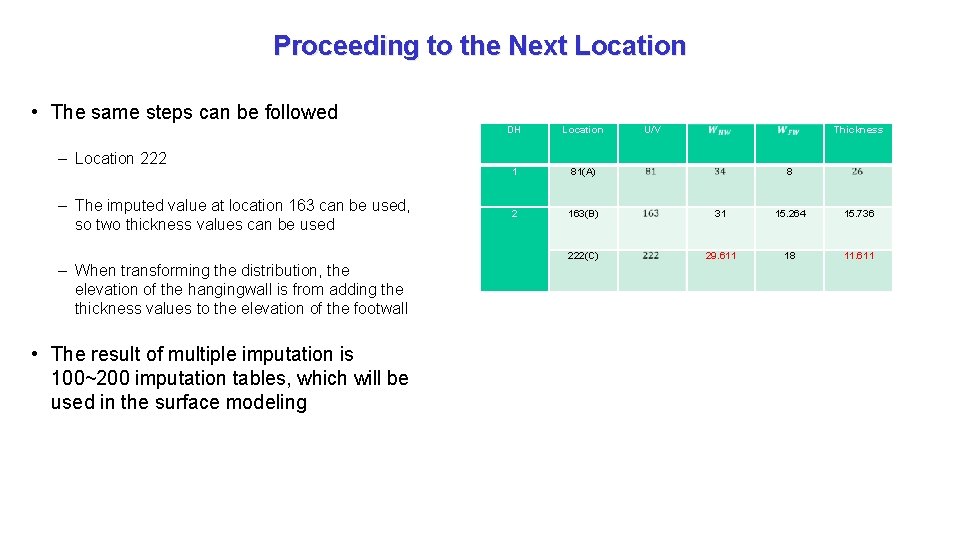
Proceeding to the Next Location • The same steps can be followed – Location 222 – The imputed value at location 163 can be used, so two thickness values can be used – When transforming the distribution, the elevation of the hangingwall is from adding the thickness values to the elevation of the footwall • The result of multiple imputation is 100~200 imputation tables, which will be used in the surface modeling DH Location U/V Thickness 1 81(A) 2 163(B) 31 15. 264 15. 736 222(C) 29. 611 18 11. 611 8
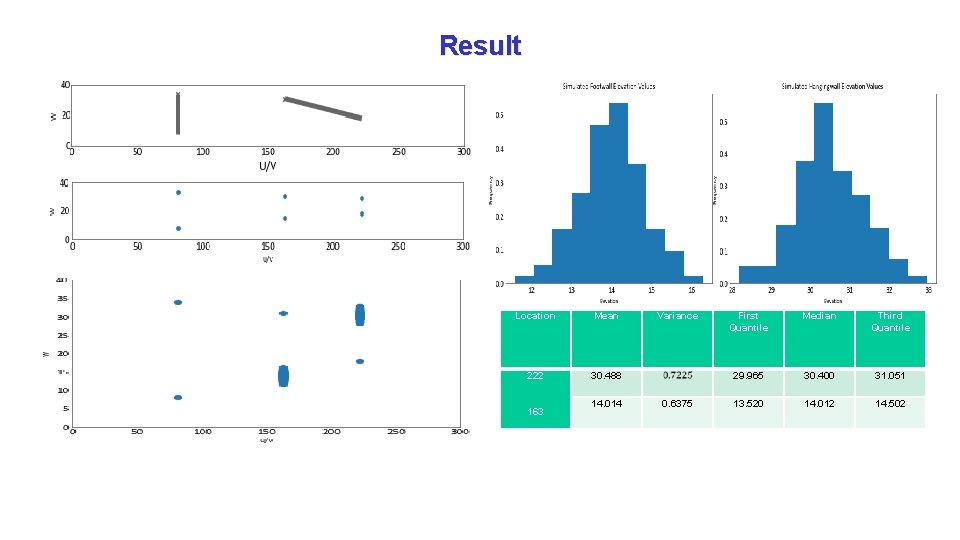
Result Location Mean 222 30. 488 163 14. 014 Variance 0. 6375 First Quantile Median Third Quantile 29. 965 30. 400 31. 051 13. 520 14. 012 14. 502
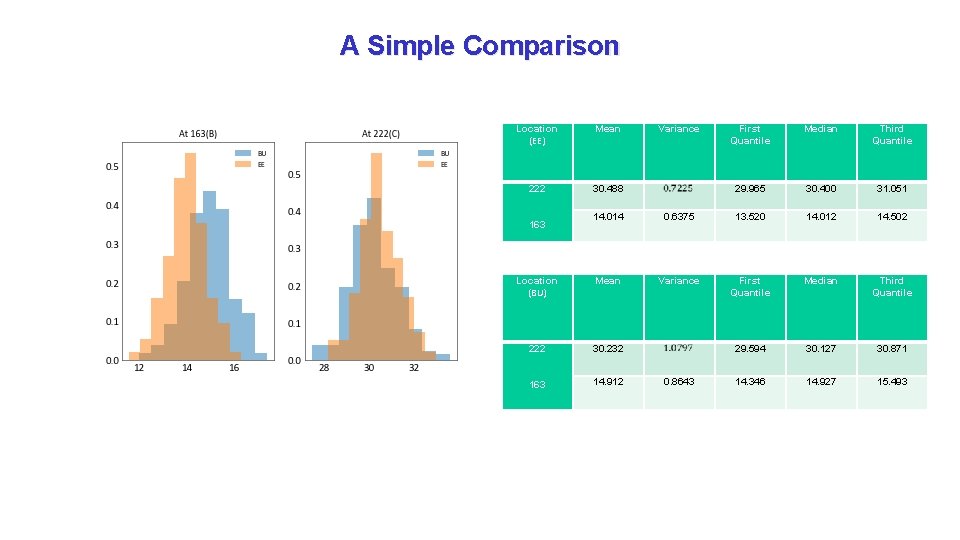
A Simple Comparison Location (EE) Mean 222 30. 488 Variance First Quantile Median Third Quantile 29. 965 30. 400 31. 051 14. 014 0. 6375 13. 520 14. 012 14. 502 Location (BU) Mean Variance First Quantile Median Third Quantile 222 30. 232 29. 594 30. 127 30. 871 163 14. 912 14. 346 14. 927 15. 493 163 0. 8643
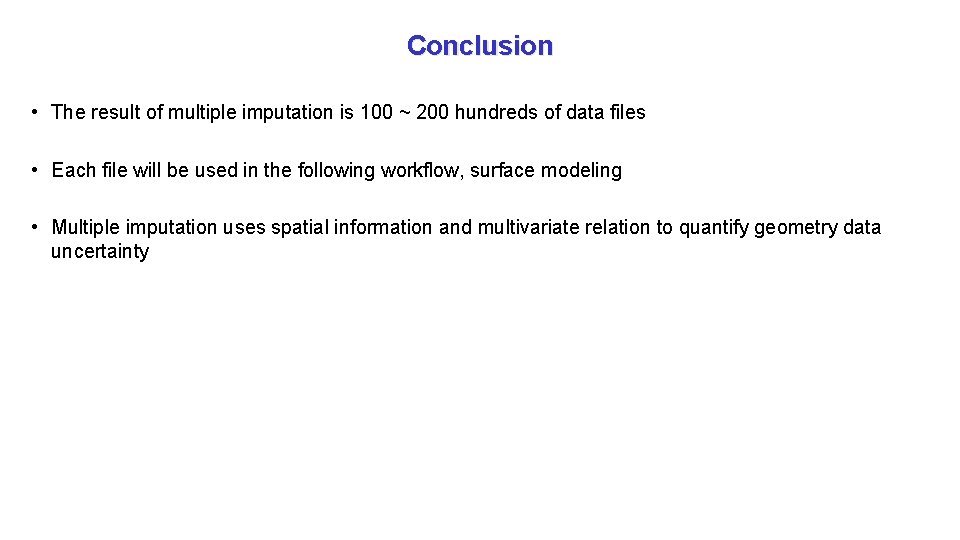
Conclusion • The result of multiple imputation is 100 ~ 200 hundreds of data files • Each file will be used in the following workflow, surface modeling • Multiple imputation uses spatial information and multivariate relation to quantify geometry data uncertainty
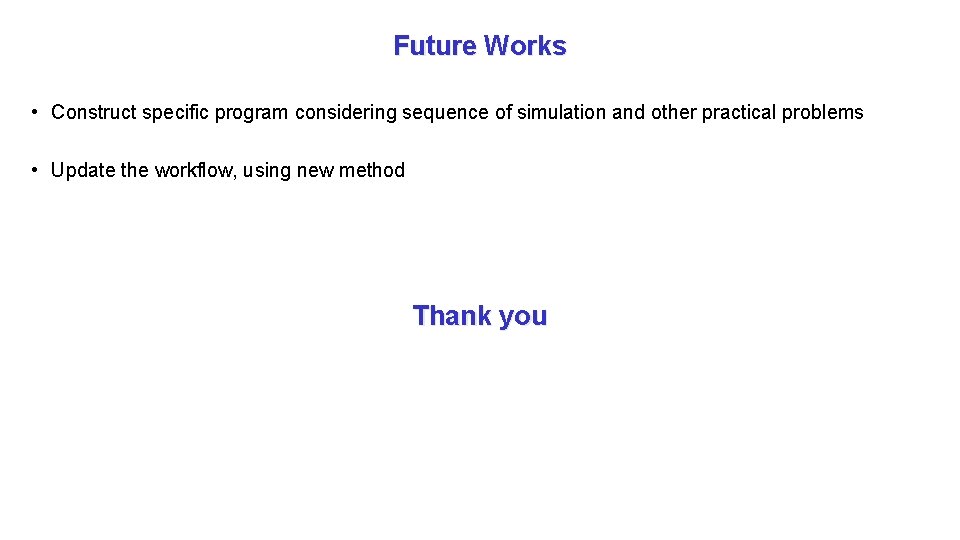
Future Works • Construct specific program considering sequence of simulation and other practical problems • Update the workflow, using new method Thank you
Occurrence exceedance probability
Helen c erickson
Dimensional modeling vs relational modeling
Aashto annual meeting 2015
Aupha annual meeting
Cwemf
Scts membership
American psychiatric association annual meeting 2020
How to run an annual general meeting
Nrg oncology meeting 2016
Kentucky grand lodge
Nrg oncology meeting 2017
Informs annual meeting
American epilepsy society annual meeting 2017
Positron vs proton
27 miles per gallon into kilometers per liter
For todays meeting
Meeting objective
What is meeting and types of meeting
What is meeting and types of meeting