Standardising industrialising end to end flows of statistical
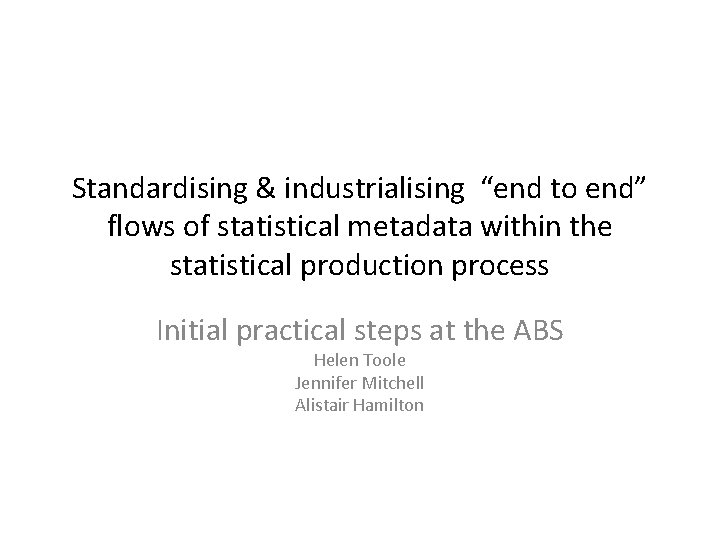
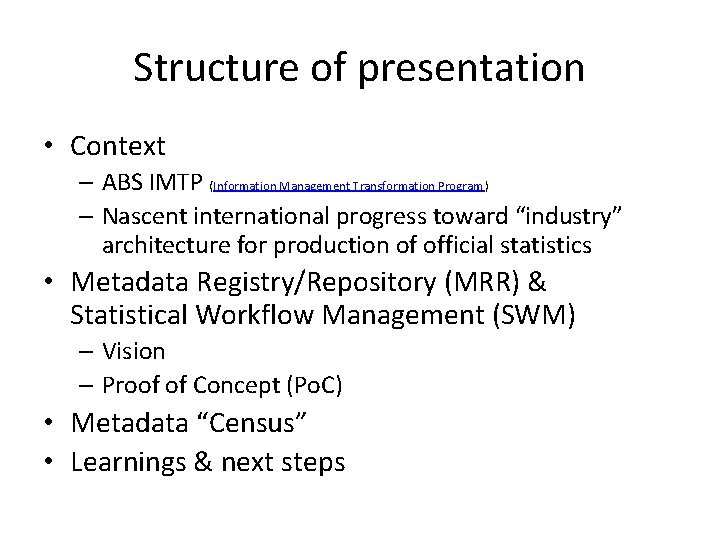
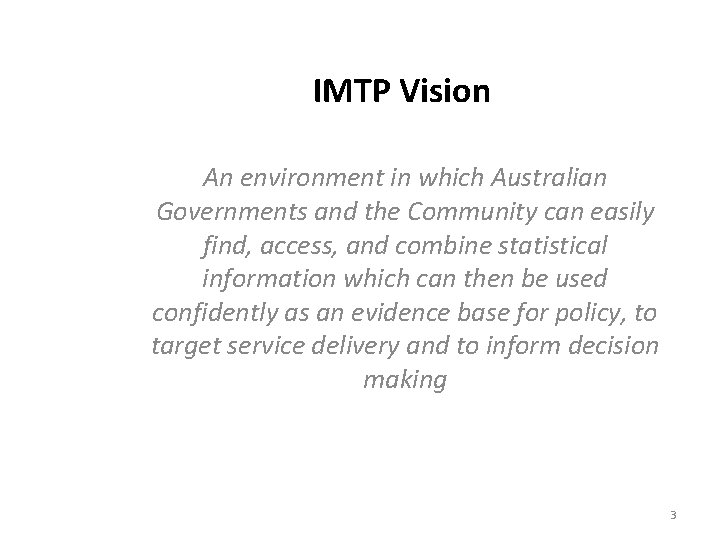
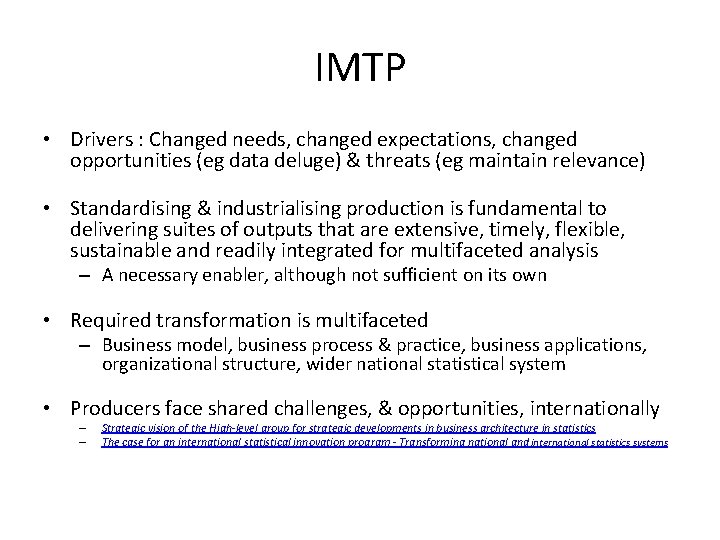
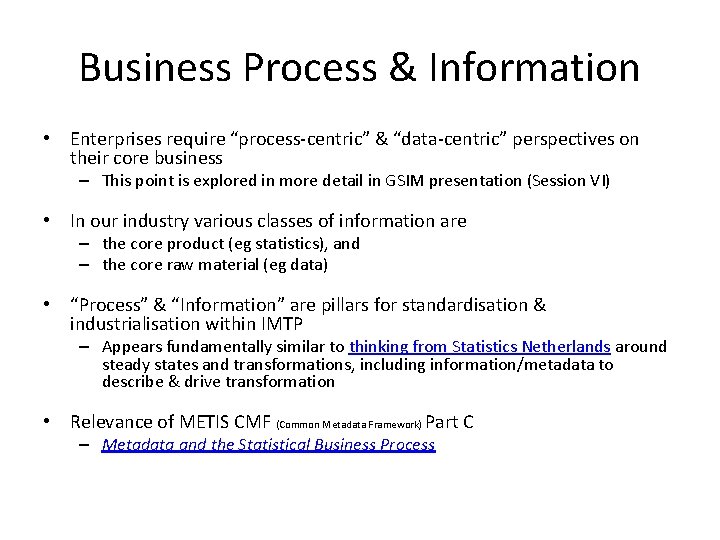
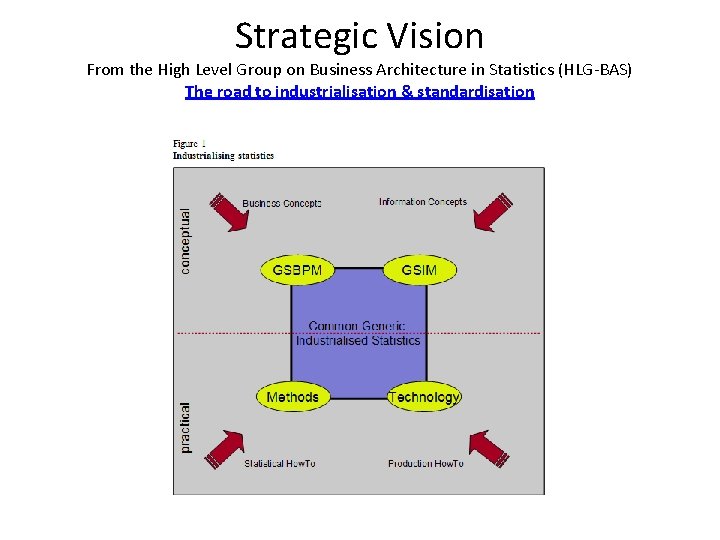
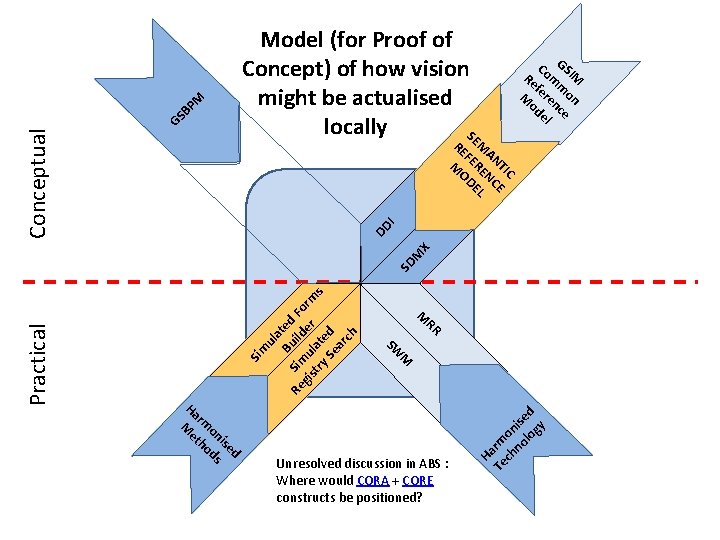
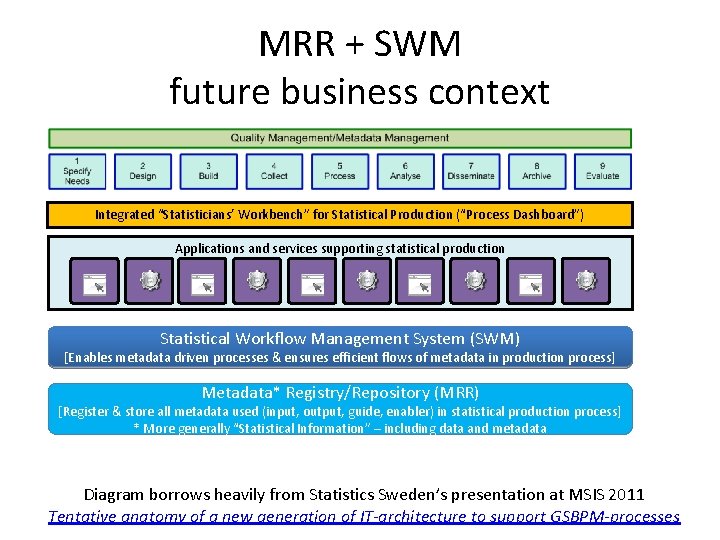
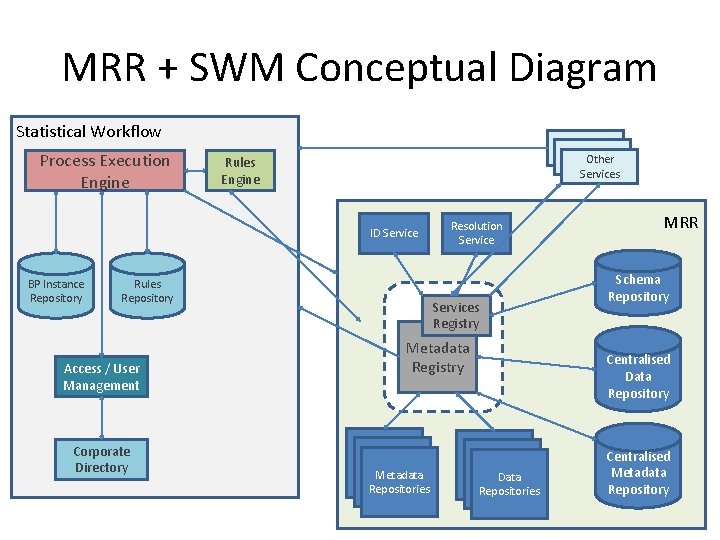
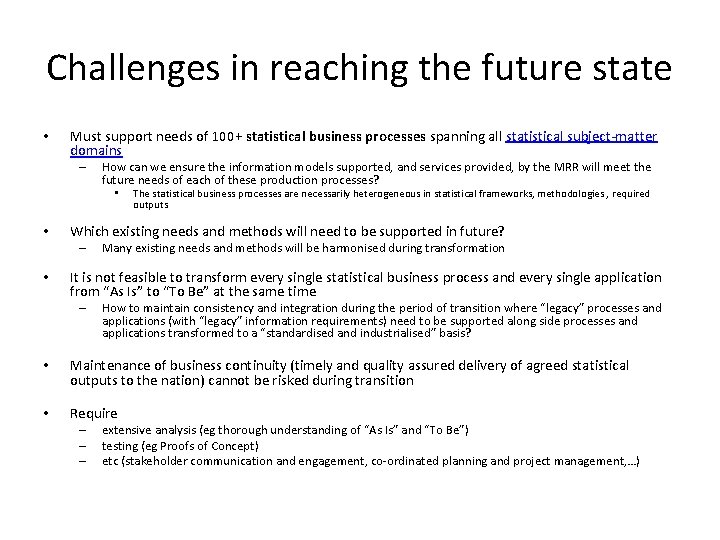
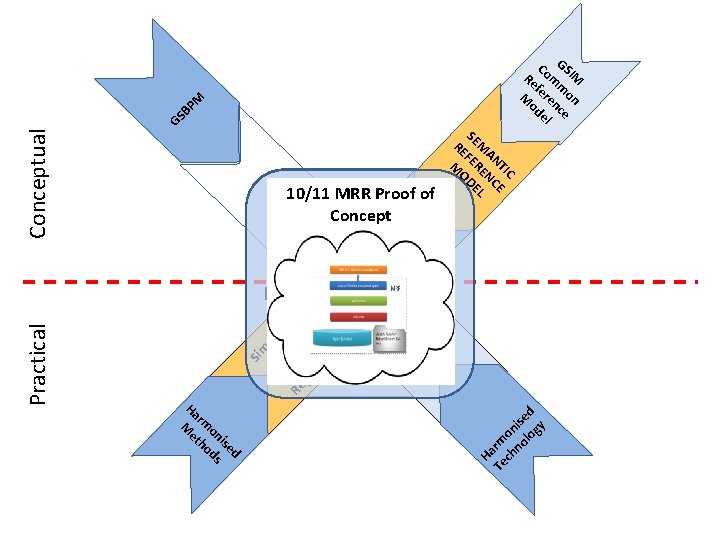
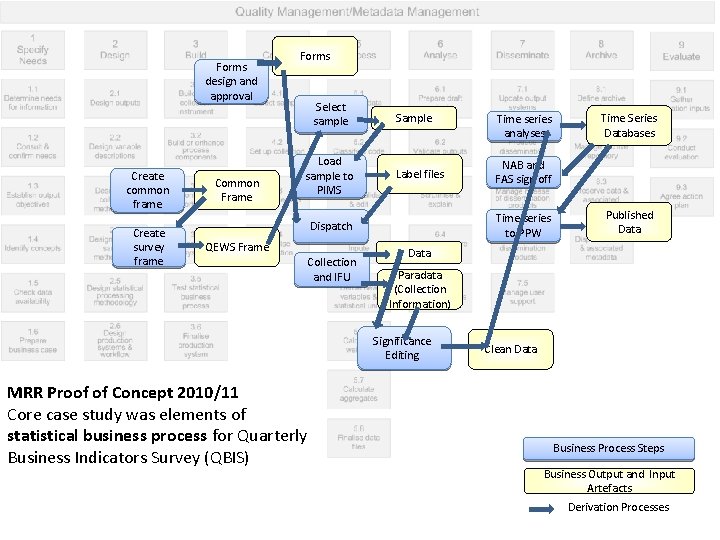
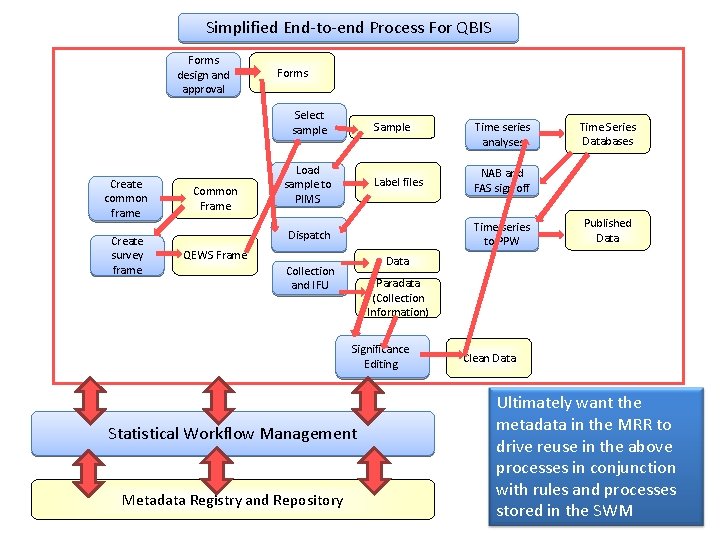
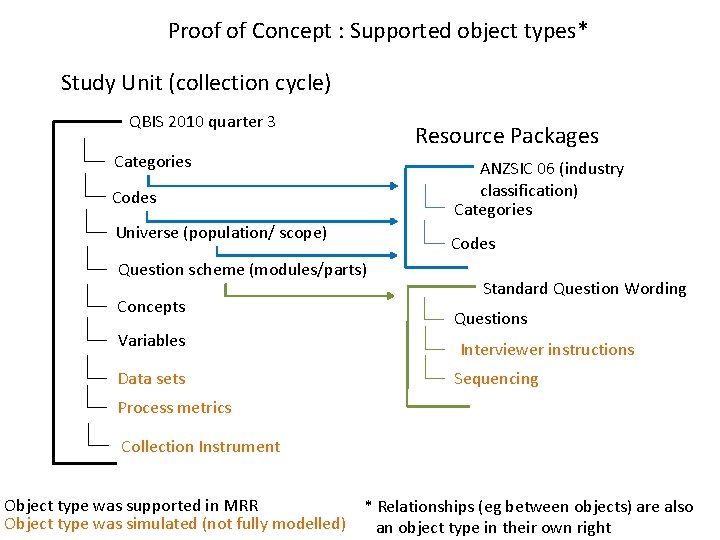
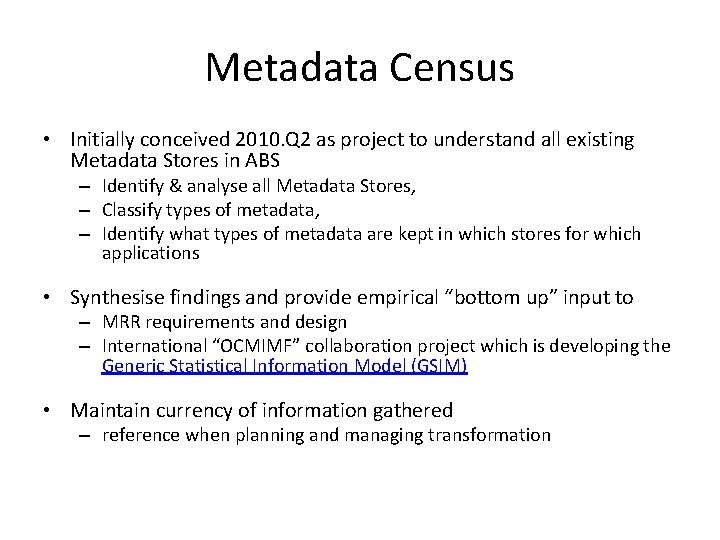
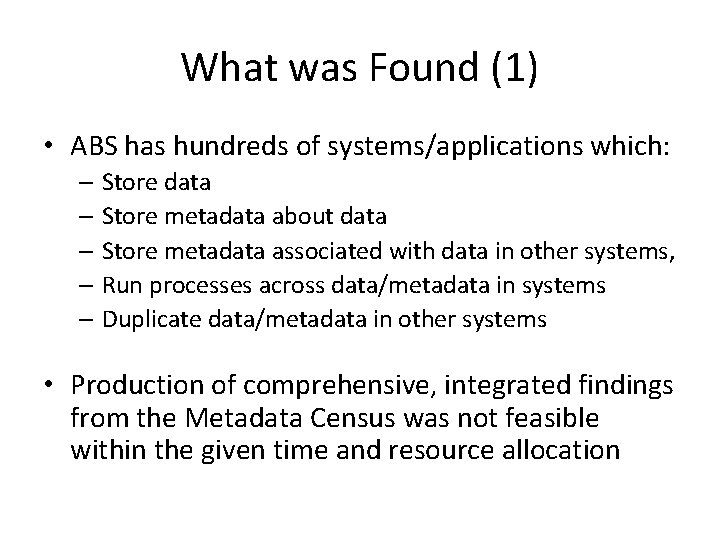
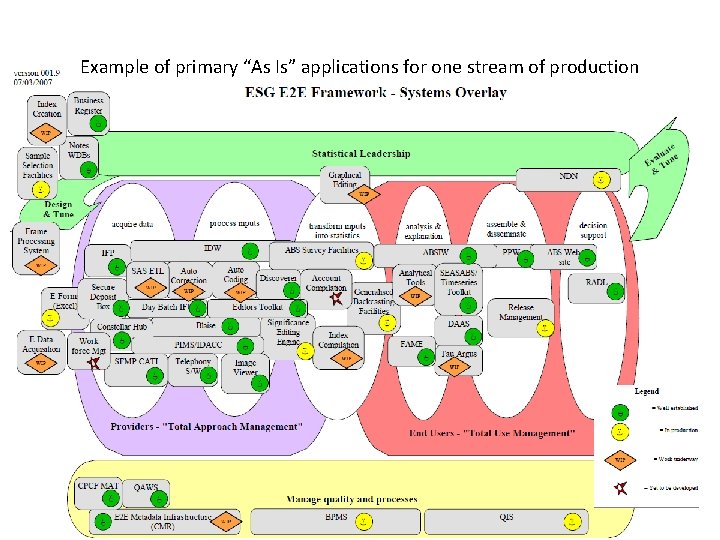
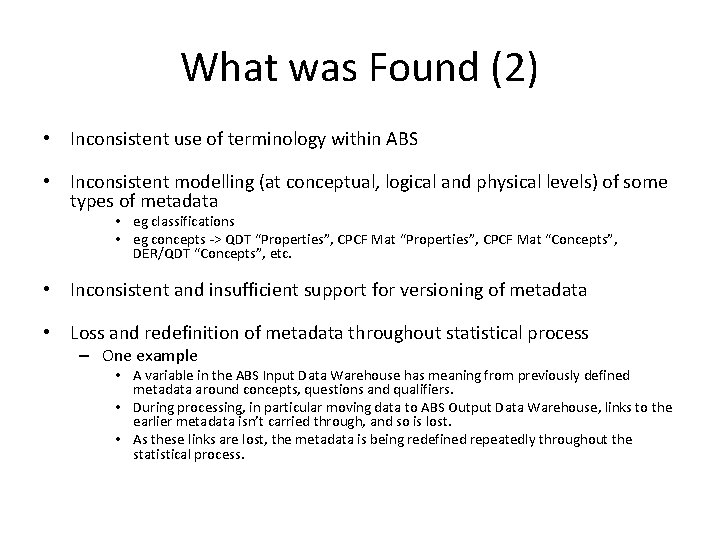
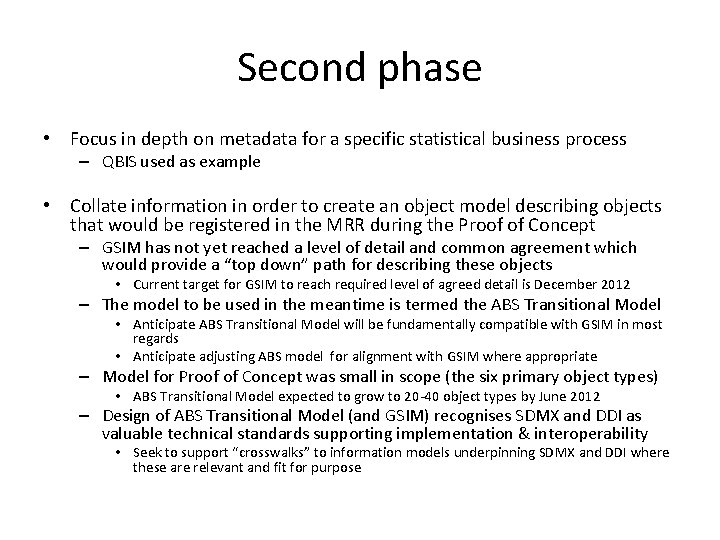
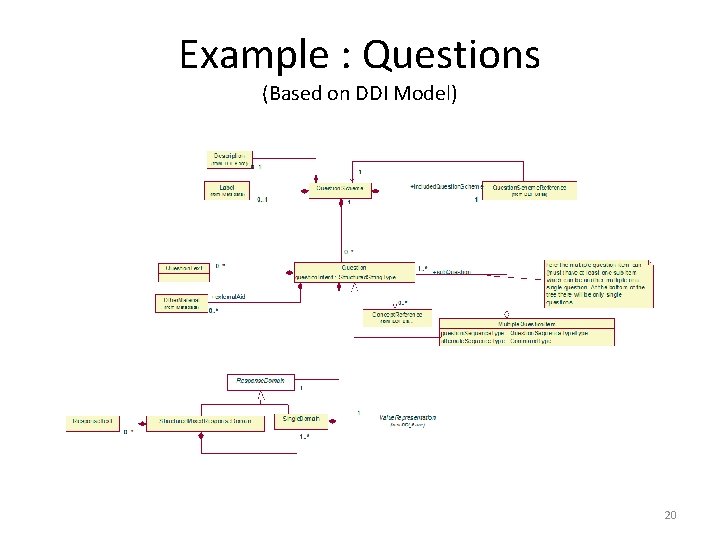
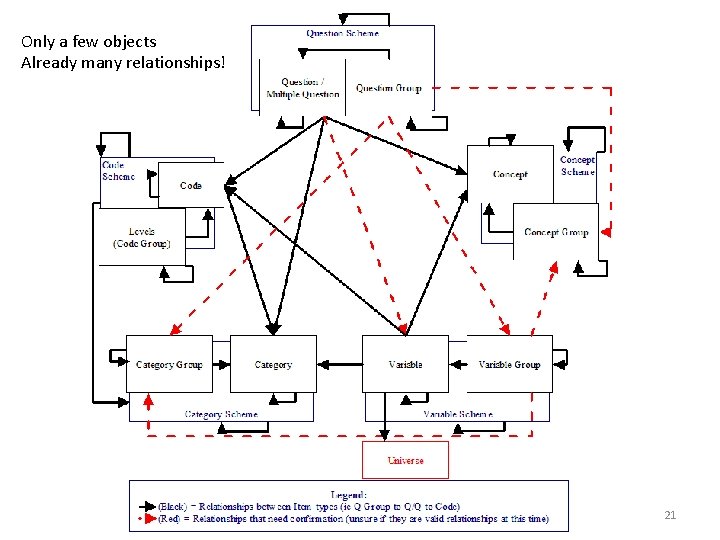
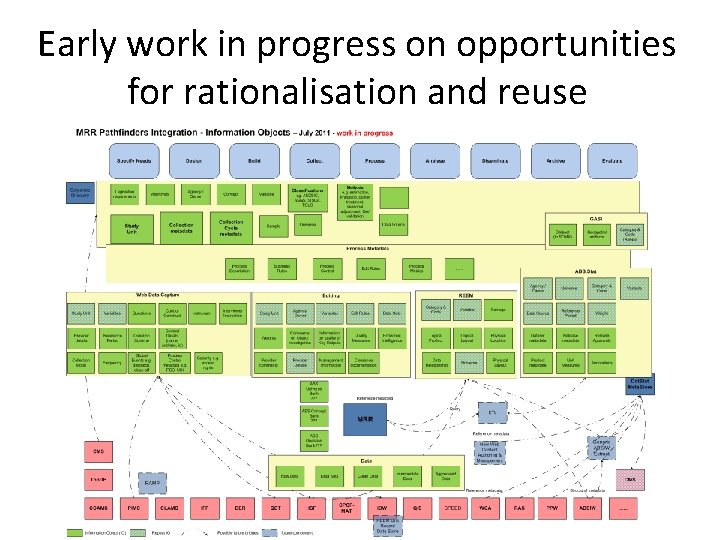
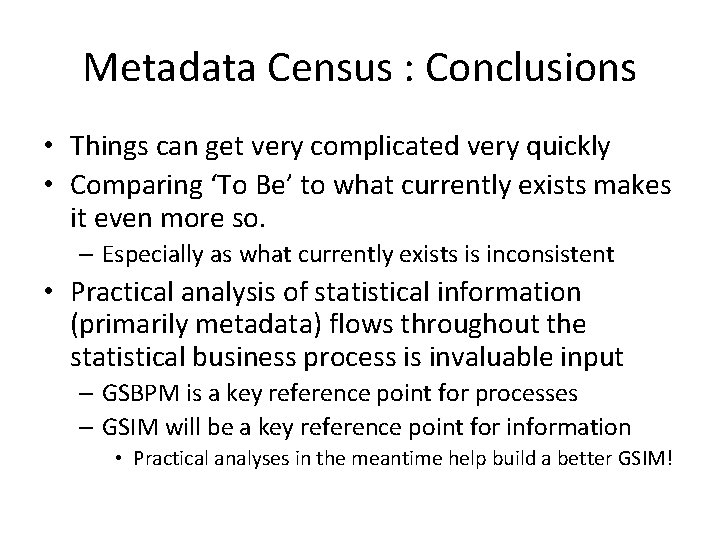
- Slides: 23
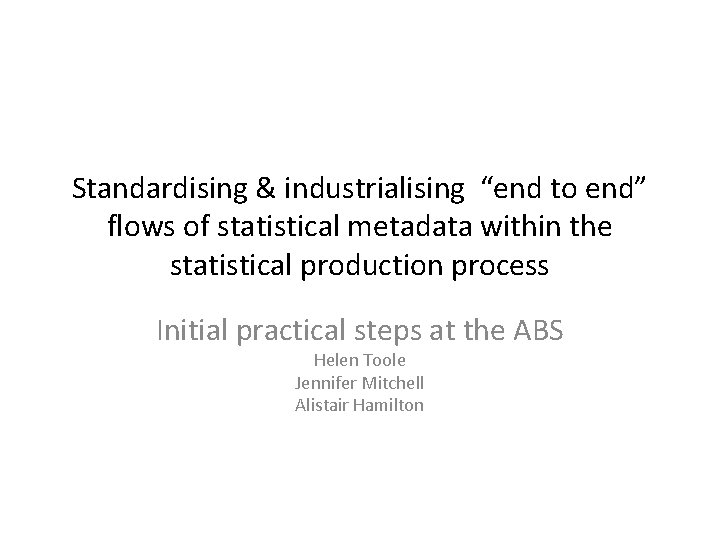
Standardising & industrialising “end to end” flows of statistical metadata within the statistical production process Initial practical steps at the ABS Helen Toole Jennifer Mitchell Alistair Hamilton
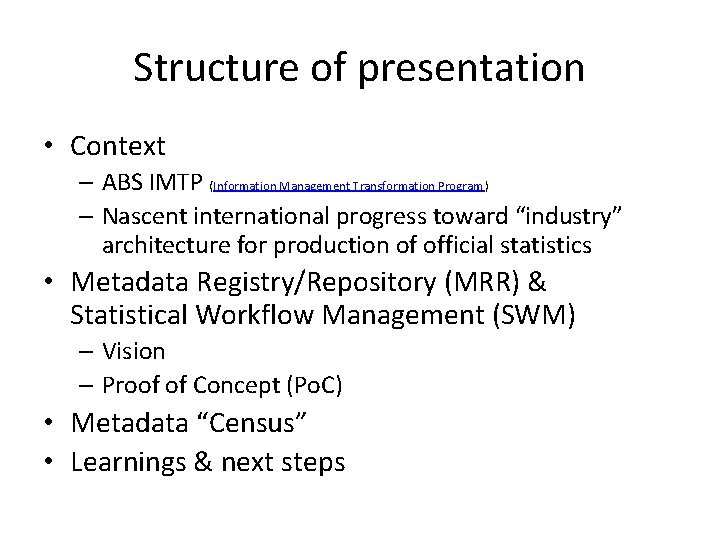
Structure of presentation • Context – ABS IMTP (Information Management Transformation Program) – Nascent international progress toward “industry” architecture for production of official statistics • Metadata Registry/Repository (MRR) & Statistical Workflow Management (SWM) – Vision – Proof of Concept (Po. C) • Metadata “Census” • Learnings & next steps
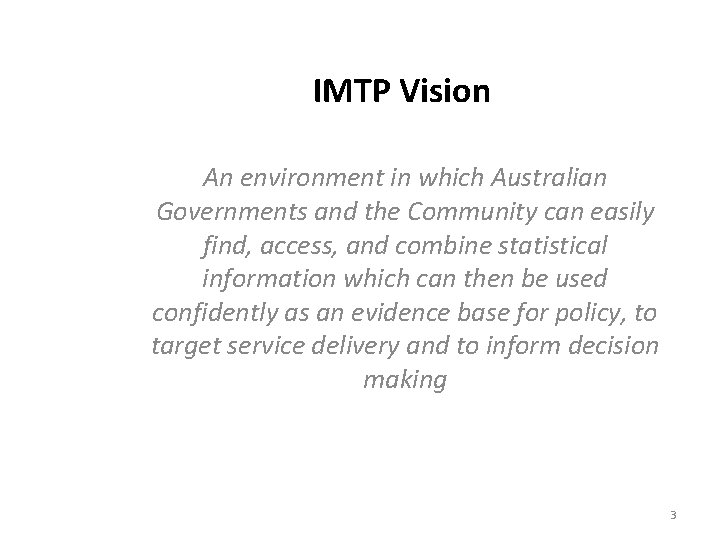
IMTP Vision An environment in which Australian Governments and the Community can easily find, access, and combine statistical information which can then be used confidently as an evidence base for policy, to target service delivery and to inform decision making 3
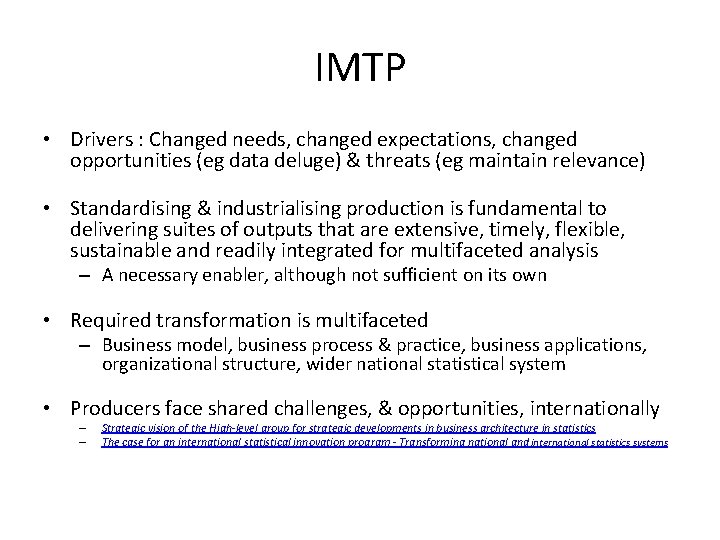
IMTP • Drivers : Changed needs, changed expectations, changed opportunities (eg data deluge) & threats (eg maintain relevance) • Standardising & industrialising production is fundamental to delivering suites of outputs that are extensive, timely, flexible, sustainable and readily integrated for multifaceted analysis – A necessary enabler, although not sufficient on its own • Required transformation is multifaceted – Business model, business process & practice, business applications, organizational structure, wider national statistical system • Producers face shared challenges, & opportunities, internationally – – Strategic vision of the High-level group for strategic developments in business architecture in statistics The case for an international statistical innovation program - Transforming national and international statistics systems
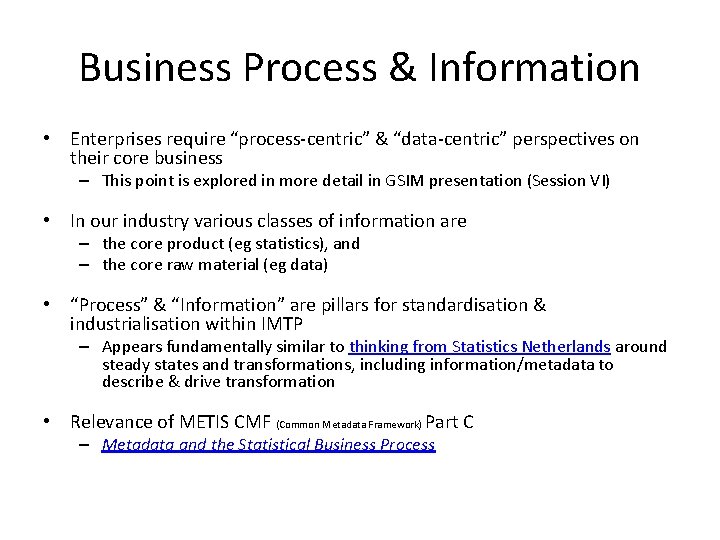
Business Process & Information • Enterprises require “process-centric” & “data-centric” perspectives on their core business – This point is explored in more detail in GSIM presentation (Session VI) • In our industry various classes of information are – the core product (eg statistics), and – the core raw material (eg data) • “Process” & “Information” are pillars for standardisation & industrialisation within IMTP – Appears fundamentally similar to thinking from Statistics Netherlands around steady states and transformations, including information/metadata to describe & drive transformation • Relevance of METIS CMF (Common Metadata Framework) Part C – Metadata and the Statistical Business Process
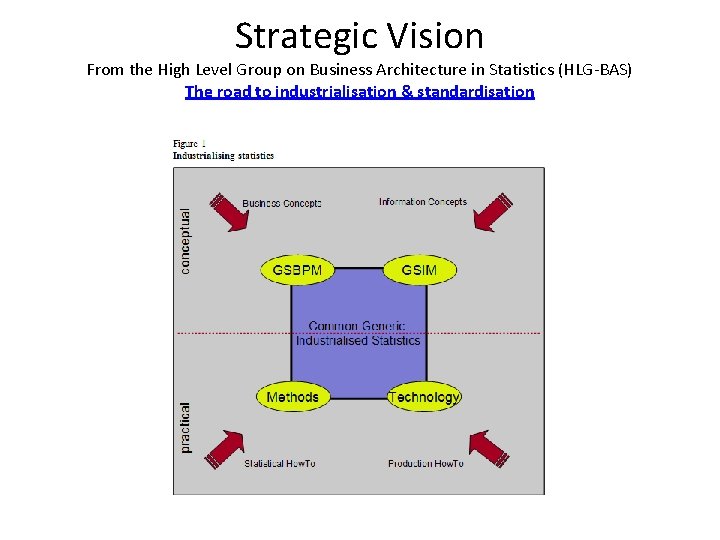
Strategic Vision From the High Level Group on Business Architecture in Statistics (HLG-BAS) The road to industrialisation & standardisation
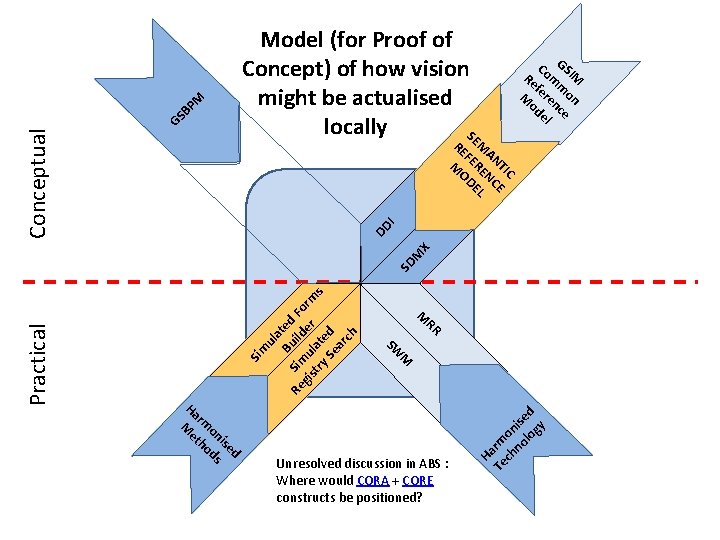
M BP GS Conceptual Model (for Proof of Concept) of how vision might be actualised locally SE C GS Re om IM f m M ere on od nc el e Unresolved discussion in ABS : Where would CORA + CORE constructs be positioned? Ha Te rm ch on no is lo ed gy a Bu ted Re Sim ild F gis u er orm try lat s Se ed ar ch ul Sim RR M M Ha r M mo et ni ho se ds d SW Practical SD M X DD I RE M F A M ERE NTI OD N C EL CE
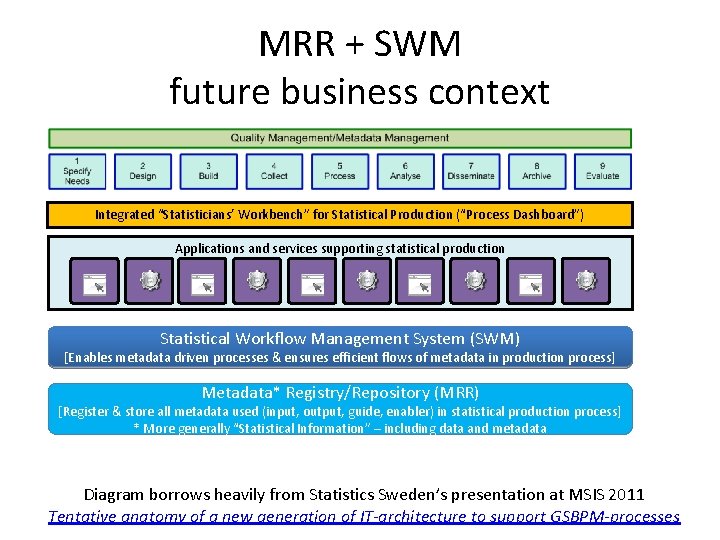
MRR + SWM future business context Integrated “Statisticians’ Workbench” for Statistical Production (“Process Dashboard”) Applications and services supporting statistical production Statistical Workflow Management System (SWM) [Enables metadata driven processes & ensures efficient flows of metadata in production process] Metadata* Registry/Repository (MRR) [Register & store all metadata used (input, output, guide, enabler) in statistical production process] * More generally “Statistical Information” – including data and metadata Diagram borrows heavily from Statistics Sweden’s presentation at MSIS 2011 Tentative anatomy of a new generation of IT-architecture to support GSBPM-processes
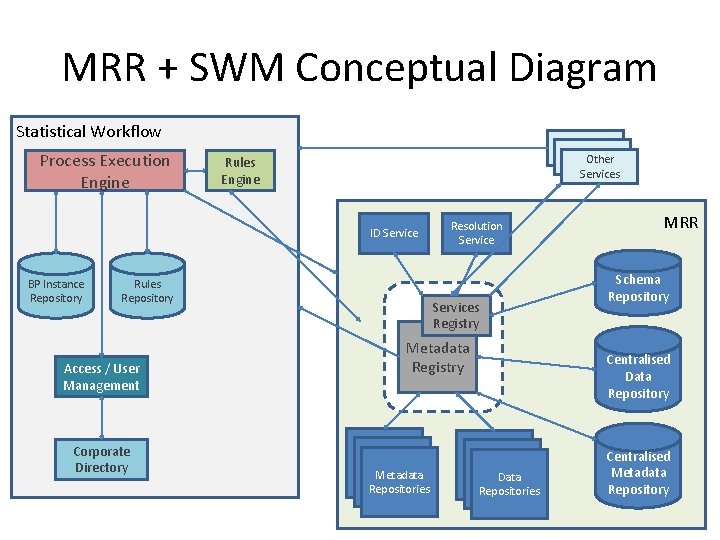
MRR + SWM Conceptual Diagram Statistical Workflow Process Execution Engine Other Services Rules Engine ID Service BP Instance Repository Rules Repository Access / User Management Corporate Directory Resolution Services Registry Metadata Repositories MRR Schema Repository Centralised Data Repository Data Repositories Centralised Metadata Repository
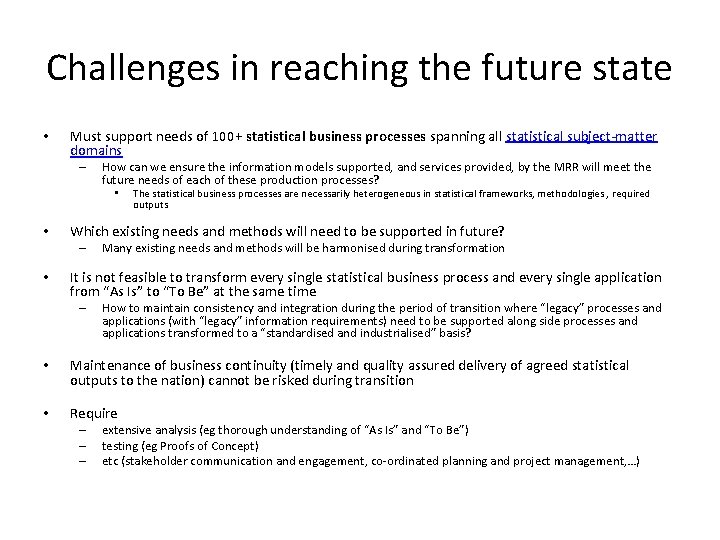
Challenges in reaching the future state • Must support needs of 100+ statistical business processes spanning all statistical subject-matter domains – How can we ensure the information models supported, and services provided, by the MRR will meet the future needs of each of these production processes? • • Which existing needs and methods will need to be supported in future? – • The statistical business processes are necessarily heterogeneous in statistical frameworks, methodologies , required outputs Many existing needs and methods will be harmonised during transformation It is not feasible to transform every single statistical business process and every single application from “As Is” to “To Be” at the same time – How to maintain consistency and integration during the period of transition where “legacy” processes and applications (with “legacy” information requirements) need to be supported along side processes and applications transformed to a “standardised and industrialised” basis? • Maintenance of business continuity (timely and quality assured delivery of agreed statistical outputs to the nation) cannot be risked during transition • Require – – – extensive analysis (eg thorough understanding of “As Is” and “To Be”) testing (eg Proofs of Concept) etc (stakeholder communication and engagement, co-ordinated planning and project management, …)
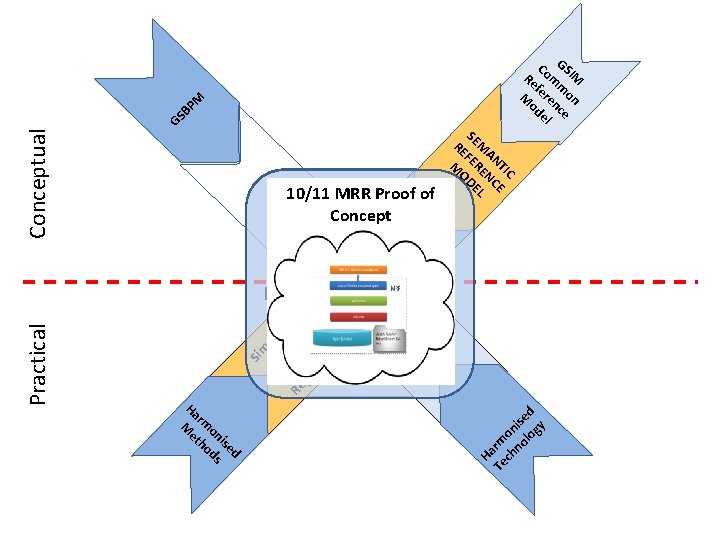
M BP GS Conceptual M X DD I 10/11 MRR Proof of Concept S RE EM F A M ERE NTI OD N C EL CE C GS Re om IM f m M ere on od nc el e Ha Te rm ch on no is lo ed gy a Bu ted Re Sim ild F gis u er orm try lat s Se ed ar ch ul Sim RR M M Ha r M mo et ni ho se ds d SW Practical SD Common Generic Industrialised Statistics
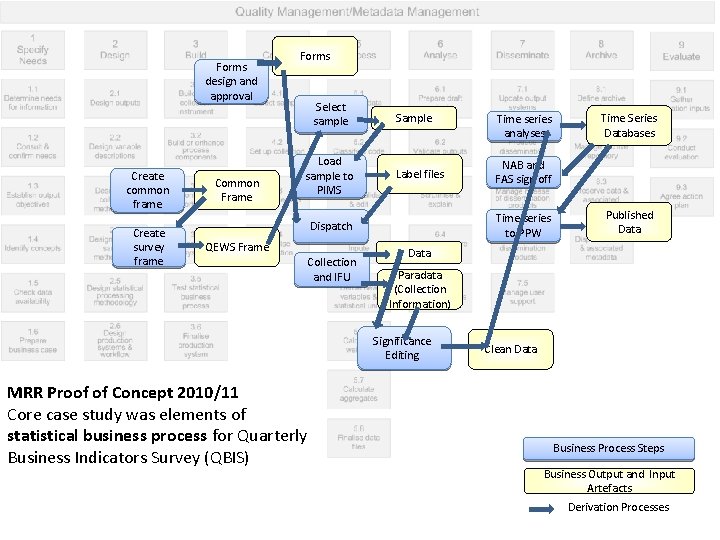
Forms design and approval Create common frame Create survey frame Common Frame Forms Select sample Load sample to PIMS Sample Label files Collection and IFU Published Data Paradata (Collection Information) Significance Editing MRR Proof of Concept 2010/11 Core case study was elements of statistical business process for Quarterly Business Indicators Survey (QBIS) Time Series Databases NAB and FAS sign off Time series to PPW Dispatch QEWS Frame Time series analyses Clean Data Business Process Steps Business Output and Input Artefacts Derivation Processes
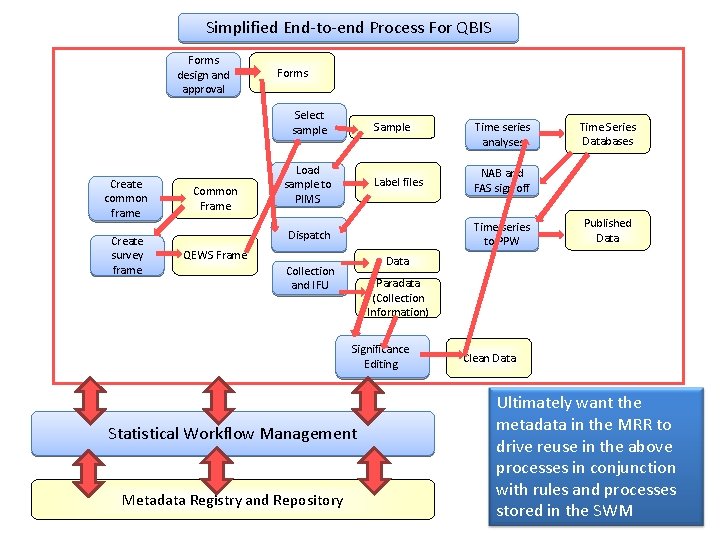
Simplified End-to-end Process For QBIS Forms design and approval Forms Select sample Create common frame Create survey frame Common Frame Sample Load sample to PIMS Label files QEWS Frame Time Series Databases NAB and FAS sign off Time series to PPW Dispatch Published Data Collection and IFU Paradata (Collection Information) Significance Editing Statistical Workflow Management Metadata Registry and Repository Time series analyses Clean Data Ultimately want the metadata in the MRR to drive reuse in the above processes in conjunction with rules and processes stored in the SWM
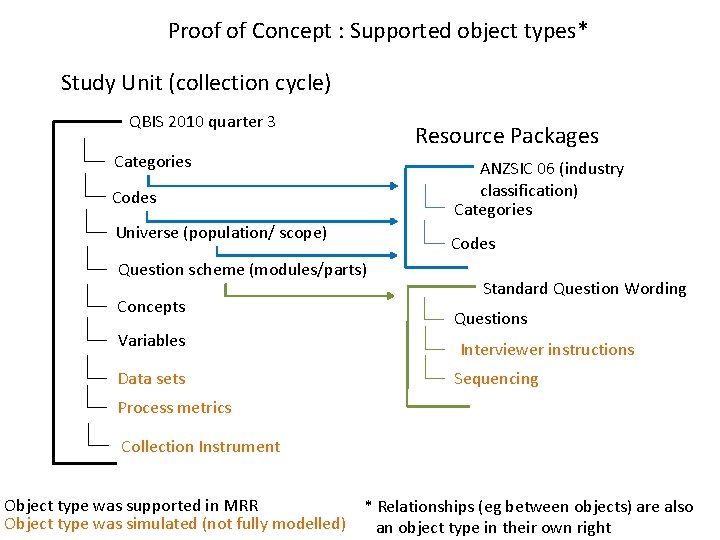
Proof of Concept : Supported object types* Study Unit (collection cycle) QBIS 2010 quarter 3 Categories Codes Universe (population/ scope) Question scheme (modules/parts) Concepts Variables Data sets Resource Packages ANZSIC 06 (industry classification) Categories Codes Standard Question Wording Questions Interviewer instructions Sequencing Process metrics Collection Instrument Object type was supported in MRR * Relationships (eg between objects) are also Object type was simulated (not fully modelled) an object type in their own right
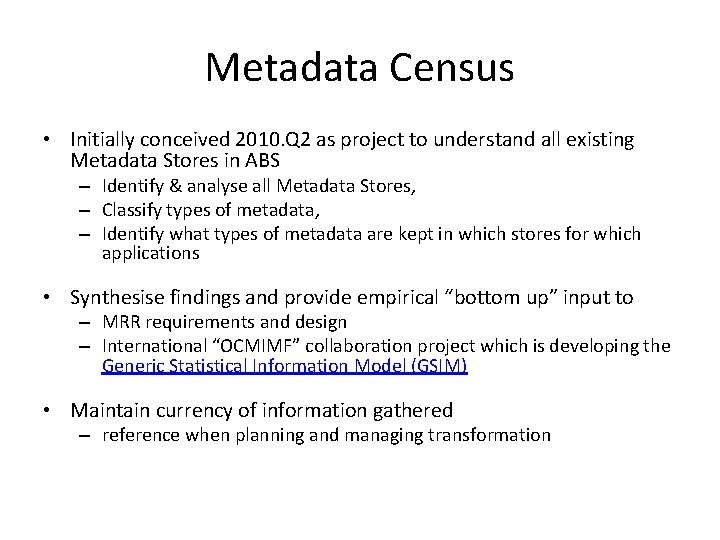
Metadata Census • Initially conceived 2010. Q 2 as project to understand all existing Metadata Stores in ABS – Identify & analyse all Metadata Stores, – Classify types of metadata, – Identify what types of metadata are kept in which stores for which applications • Synthesise findings and provide empirical “bottom up” input to – MRR requirements and design – International “OCMIMF” collaboration project which is developing the Generic Statistical Information Model (GSIM) • Maintain currency of information gathered – reference when planning and managing transformation
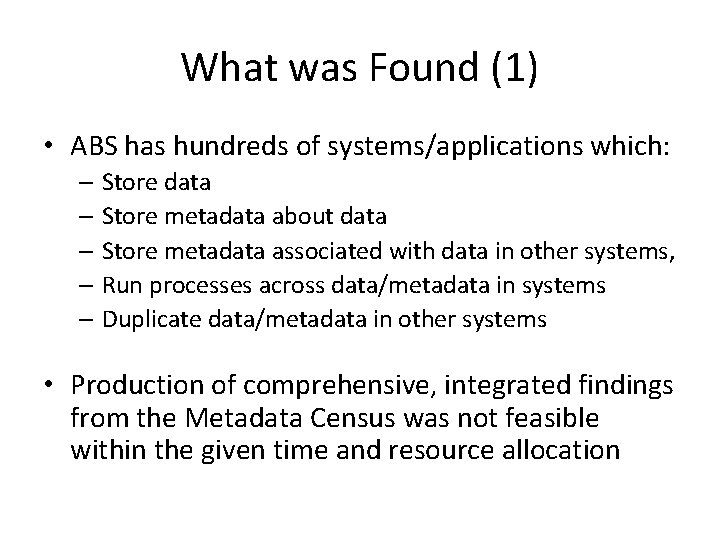
What was Found (1) • ABS has hundreds of systems/applications which: – Store data – Store metadata about data – Store metadata associated with data in other systems, – Run processes across data/metadata in systems – Duplicate data/metadata in other systems • Production of comprehensive, integrated findings from the Metadata Census was not feasible within the given time and resource allocation
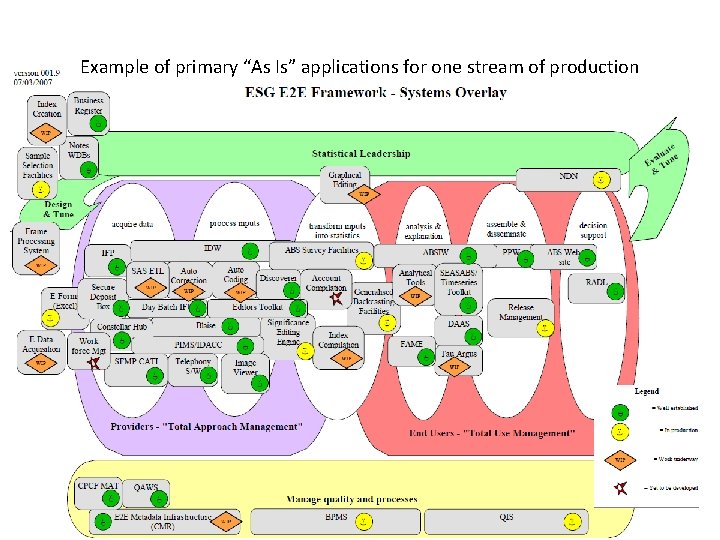
Example of primary “As Is” applications for one stream of production
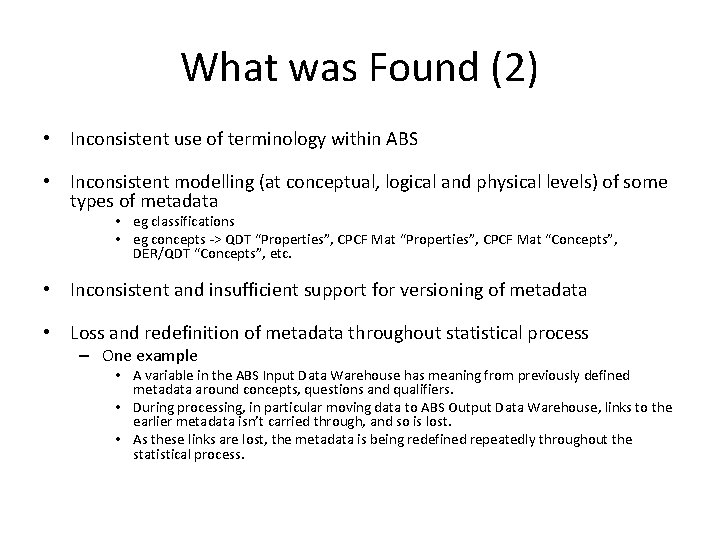
What was Found (2) • Inconsistent use of terminology within ABS • Inconsistent modelling (at conceptual, logical and physical levels) of some types of metadata • eg classifications • eg concepts -> QDT “Properties”, CPCF Mat “Concepts”, DER/QDT “Concepts”, etc. • Inconsistent and insufficient support for versioning of metadata • Loss and redefinition of metadata throughout statistical process – One example • A variable in the ABS Input Data Warehouse has meaning from previously defined metadata around concepts, questions and qualifiers. • During processing, in particular moving data to ABS Output Data Warehouse, links to the earlier metadata isn’t carried through, and so is lost. • As these links are lost, the metadata is being redefined repeatedly throughout the statistical process.
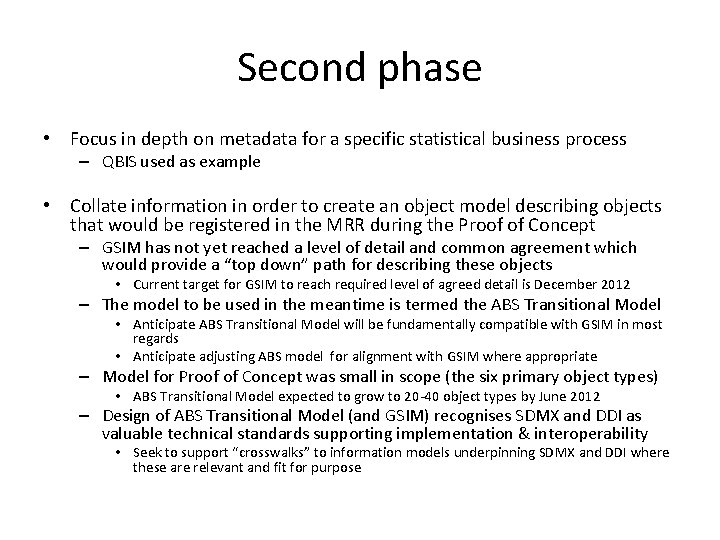
Second phase • Focus in depth on metadata for a specific statistical business process – QBIS used as example • Collate information in order to create an object model describing objects that would be registered in the MRR during the Proof of Concept – GSIM has not yet reached a level of detail and common agreement which would provide a “top down” path for describing these objects • Current target for GSIM to reach required level of agreed detail is December 2012 – The model to be used in the meantime is termed the ABS Transitional Model • Anticipate ABS Transitional Model will be fundamentally compatible with GSIM in most regards • Anticipate adjusting ABS model for alignment with GSIM where appropriate – Model for Proof of Concept was small in scope (the six primary object types) • ABS Transitional Model expected to grow to 20 -40 object types by June 2012 – Design of ABS Transitional Model (and GSIM) recognises SDMX and DDI as valuable technical standards supporting implementation & interoperability • Seek to support “crosswalks” to information models underpinning SDMX and DDI where these are relevant and fit for purpose
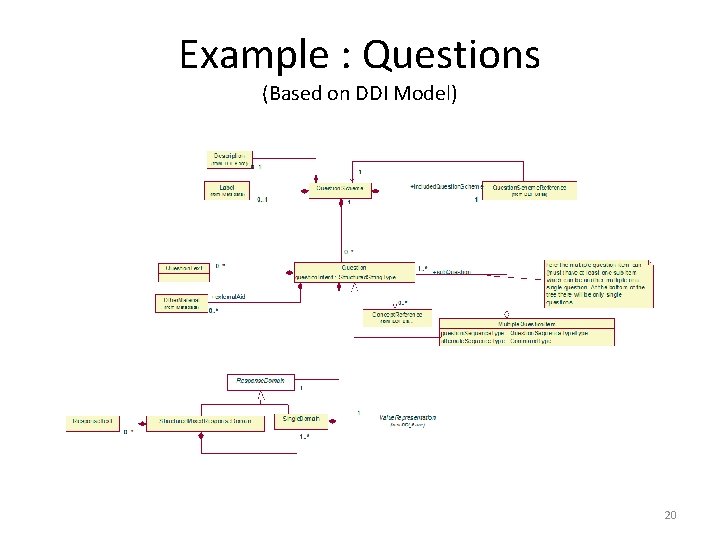
Example : Questions (Based on DDI Model) 20
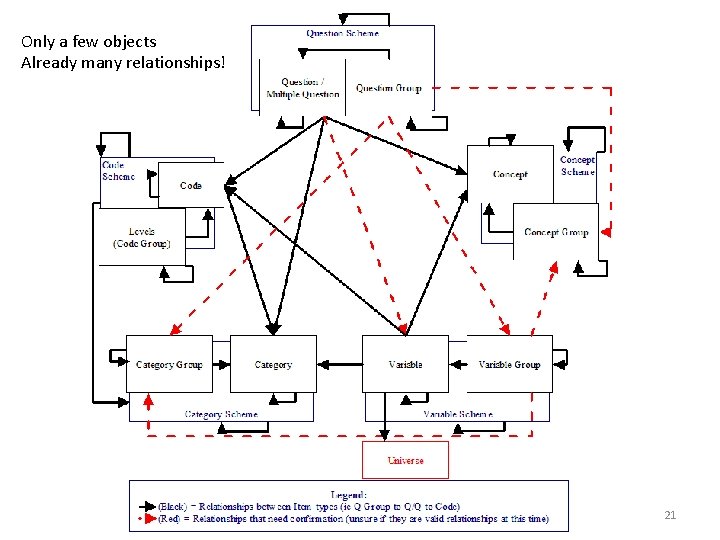
Only a few objects Already many relationships! 21
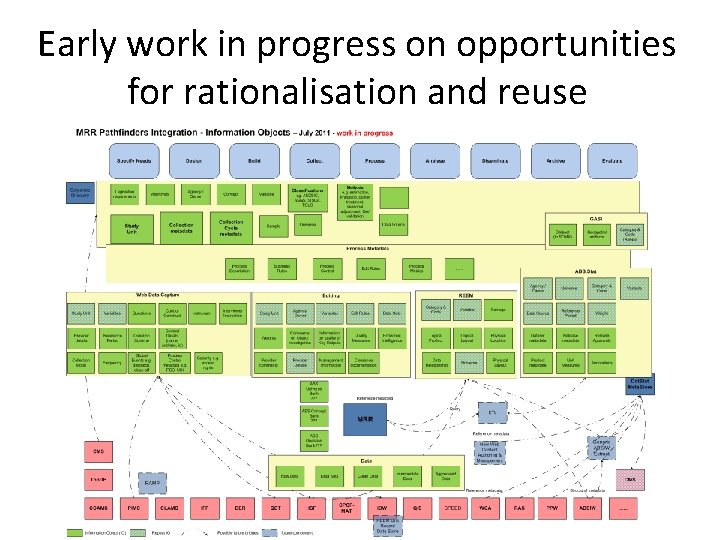
Early work in progress on opportunities for rationalisation and reuse
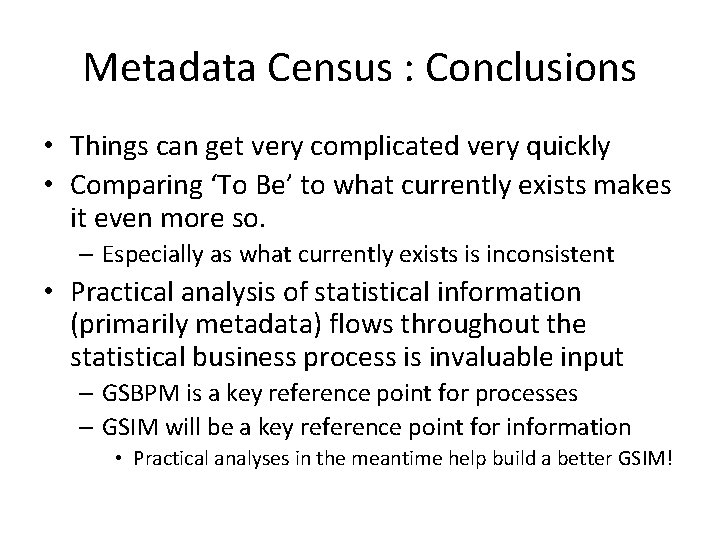
Metadata Census : Conclusions • Things can get very complicated very quickly • Comparing ‘To Be’ to what currently exists makes it even more so. – Especially as what currently exists is inconsistent • Practical analysis of statistical information (primarily metadata) flows throughout the statistical business process is invaluable input – GSBPM is a key reference point for processes – GSIM will be a key reference point for information • Practical analyses in the meantime help build a better GSIM!
Eight generic channel flows
Supporting facility
Flow chapter 13
Structure of flows in a modern exchange economy
Cmos process flows
Ammortisation schedule
Intermediate accounting chapter 23
Mutual fund flows and performance in rational markets
Statement of cash flows order
Intermediate cash flows
The great tao
Water flows in the branching pipe shown
Structure of flows in a modern exchange economy
Relevant cash flows definition
Granting credit letter
Future value of multiple cash flows example
Cash flow indirect method
Chapter 13 statement of cash flows
Cash received from customers
Amg fund flows
What countries does the mekong river flow through
Marketing channel concept
Combines elements of linear and parallel process flows
The statement of cash flows helps users