SIRP MODELING of IFO DATA ResearchTeam Prof Innocenzo
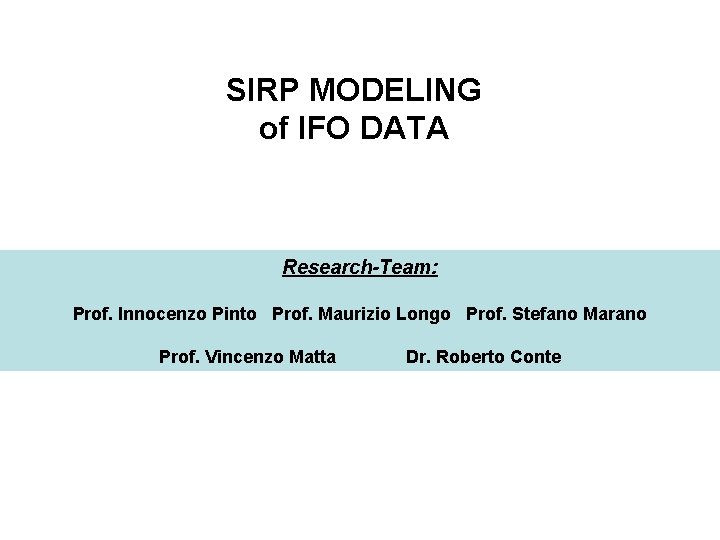
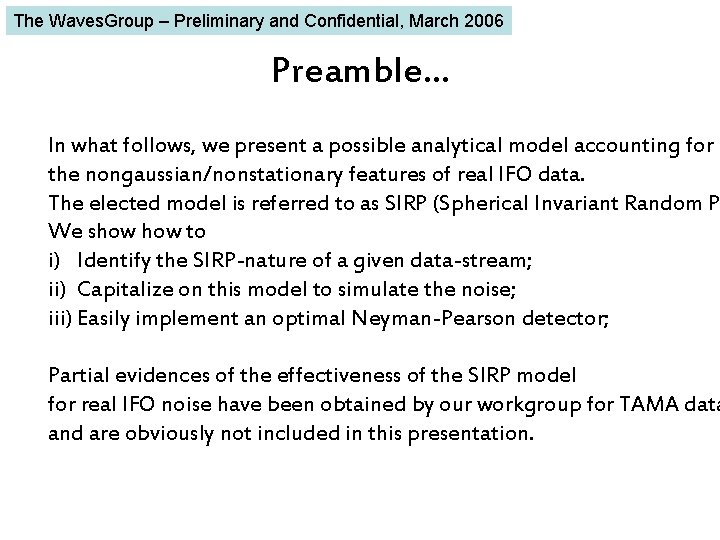
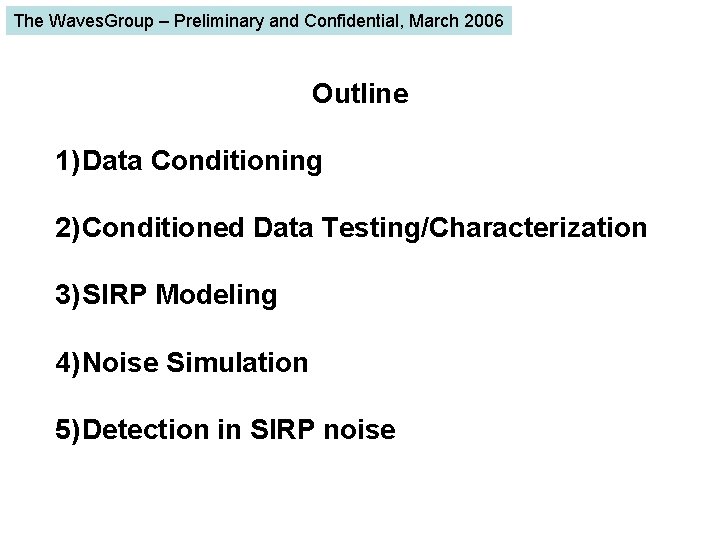
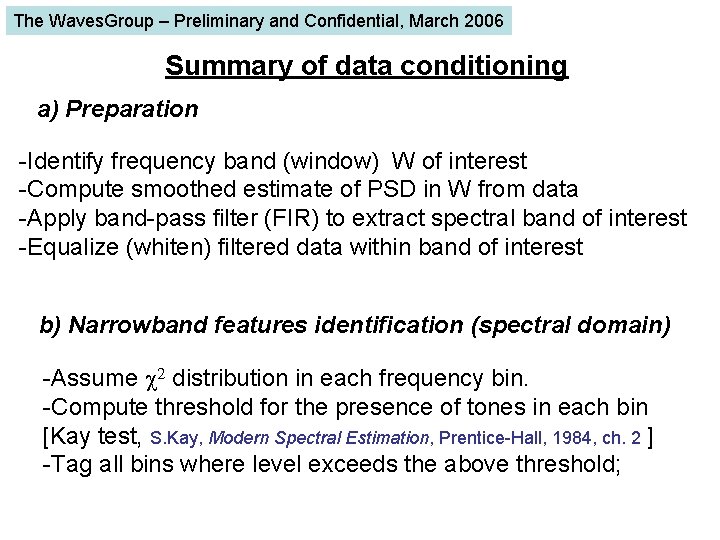
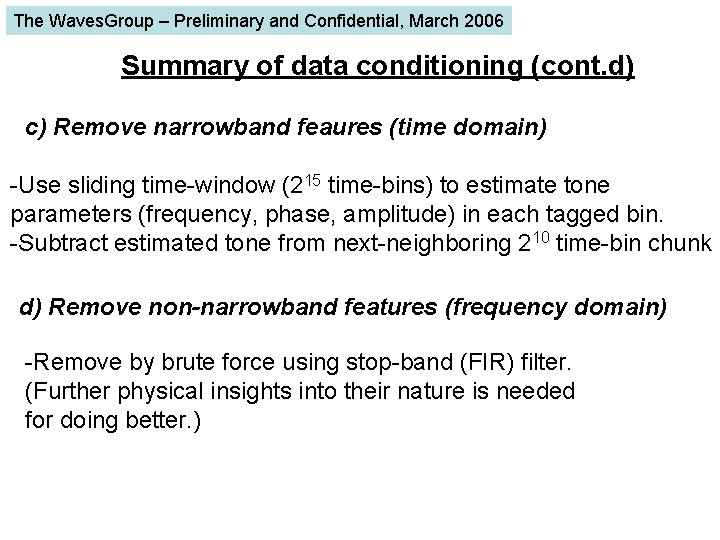
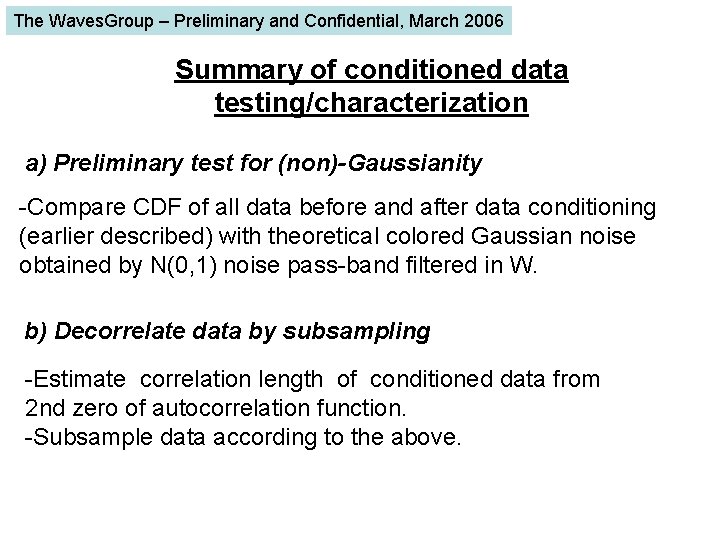
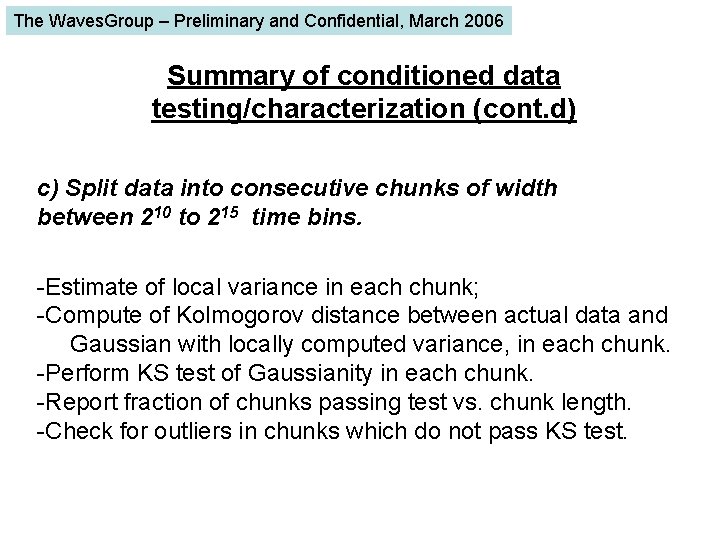
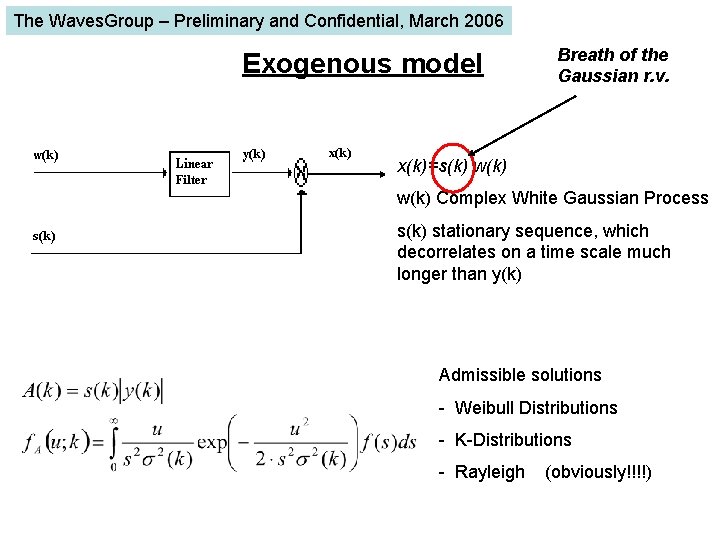
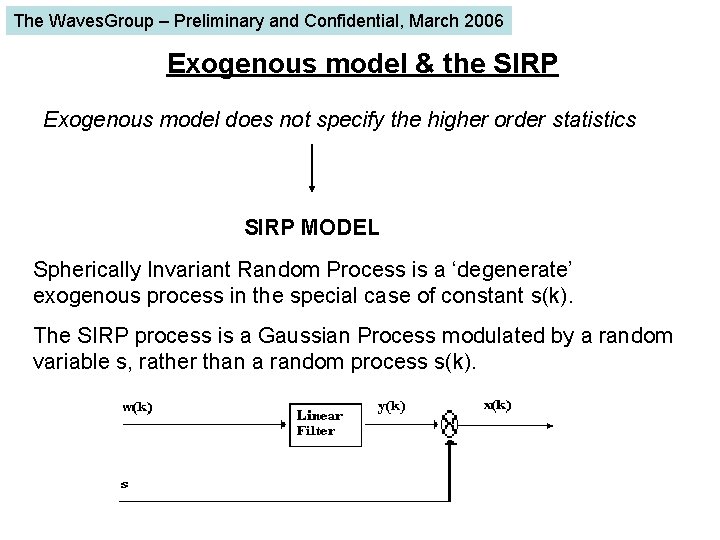
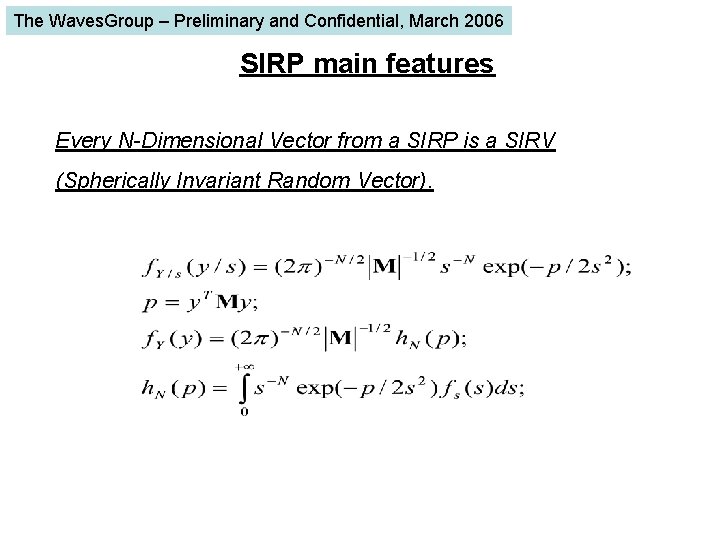
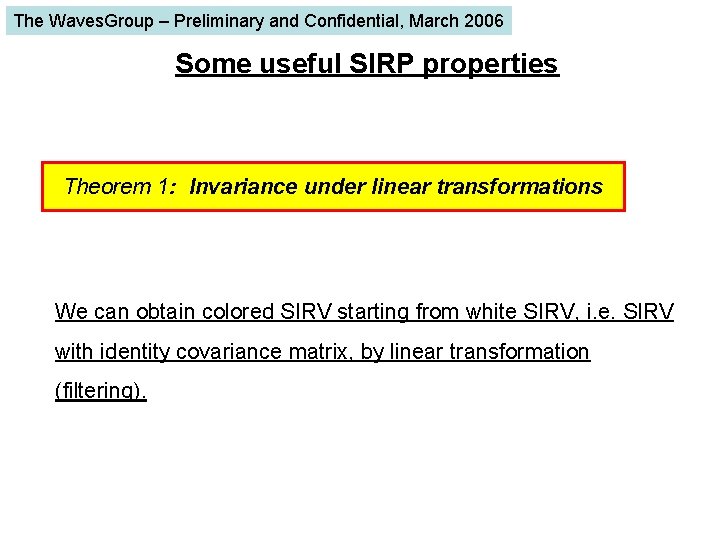
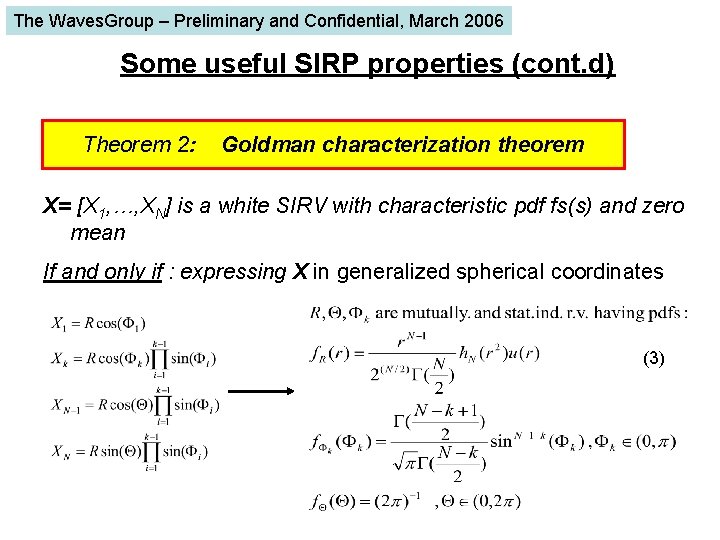
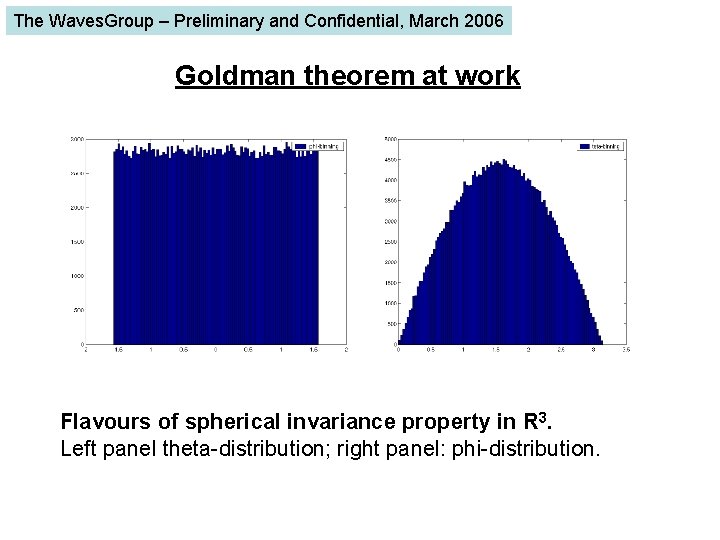
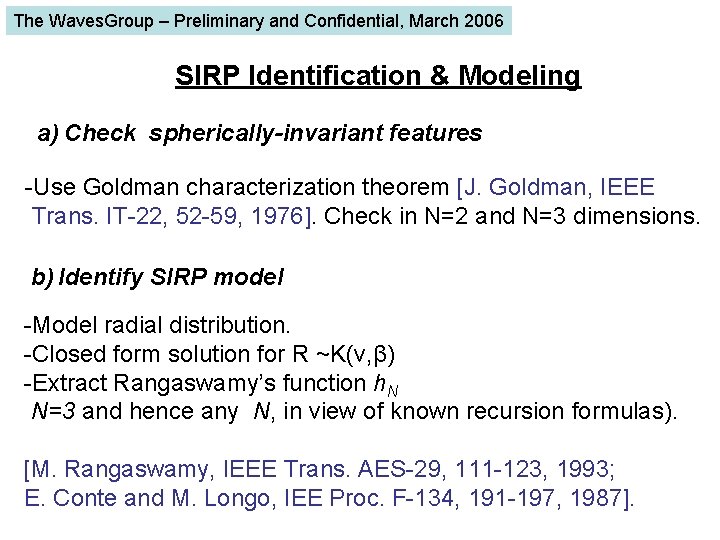
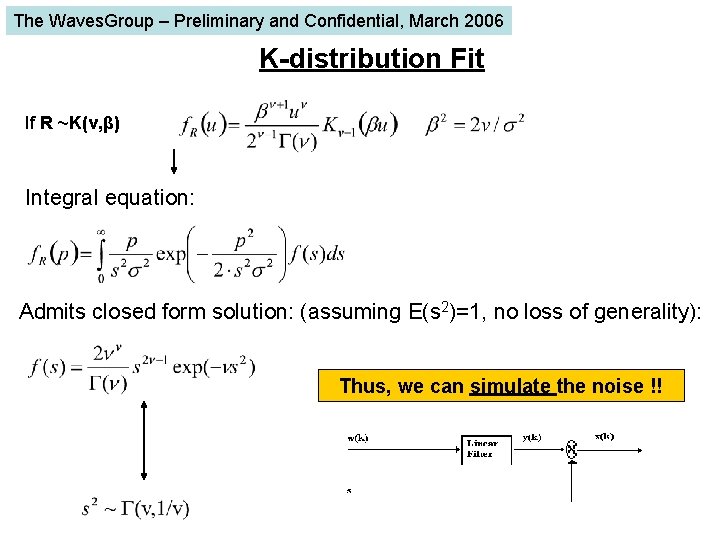
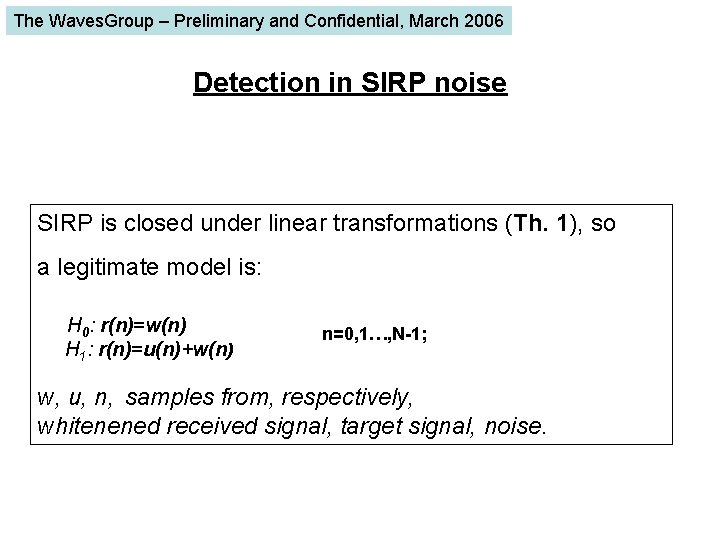
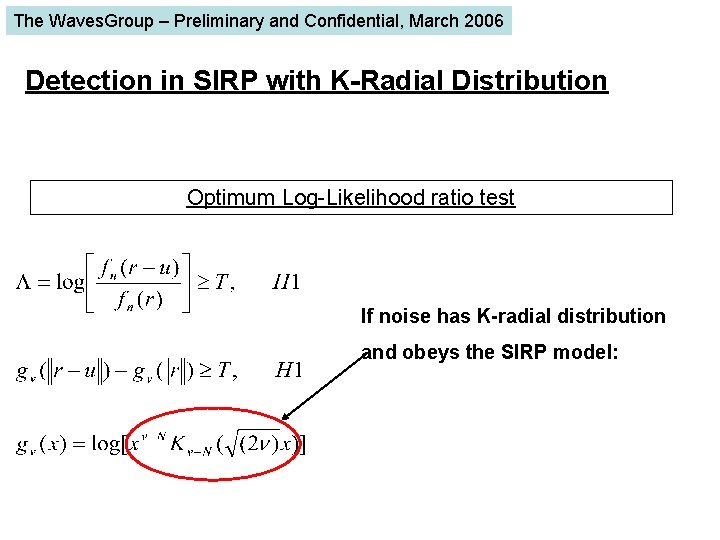
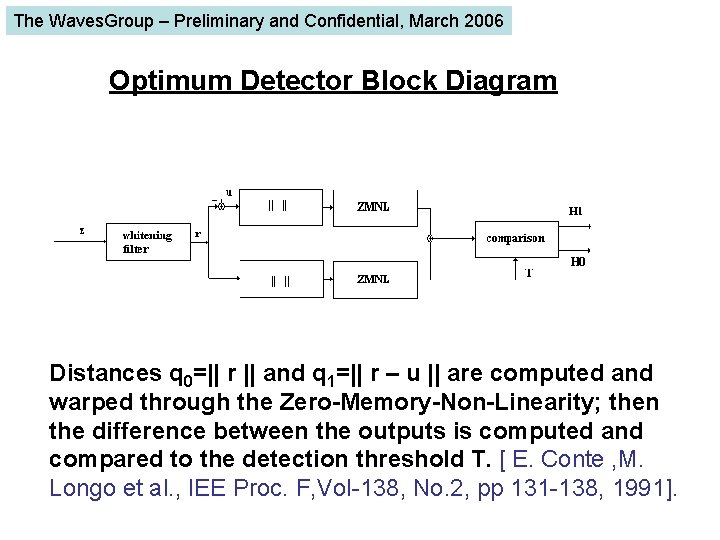
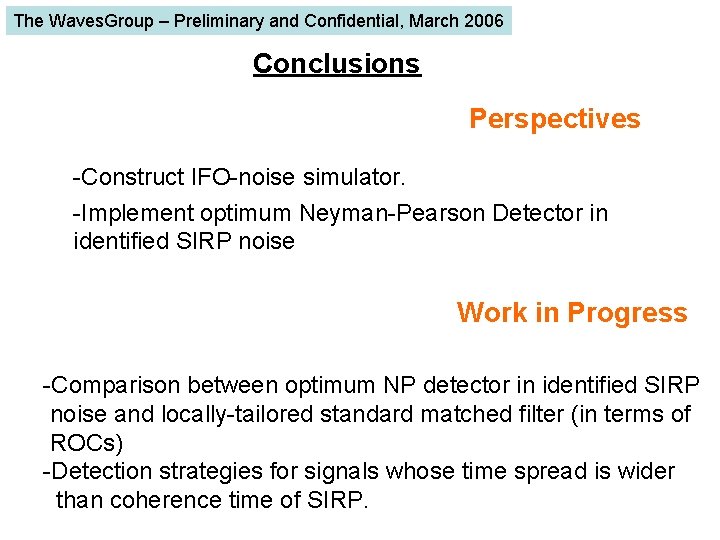
- Slides: 19
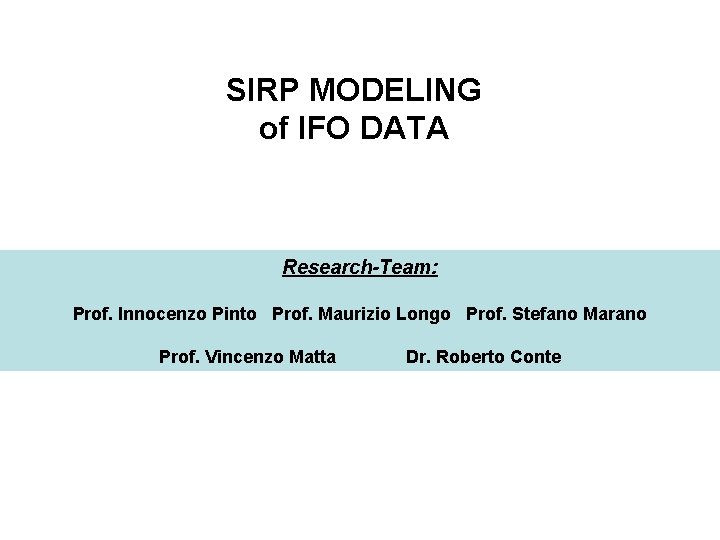
SIRP MODELING of IFO DATA Research-Team: Prof. Innocenzo Pinto Prof. Maurizio Longo Prof. Stefano Marano Prof. Vincenzo Matta Dr. Roberto Conte
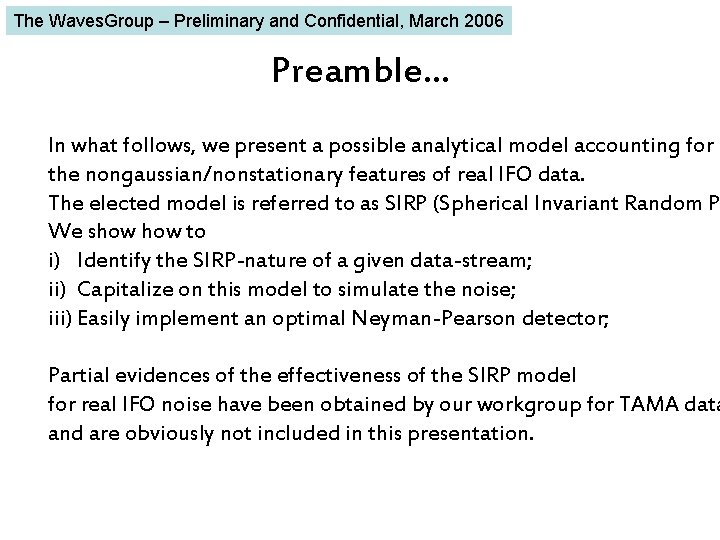
The Waves. Group – Preliminary and Confidential, March 2006 Preamble. . . In what follows, we present a possible analytical model accounting for the nongaussian/nonstationary features of real IFO data. The elected model is referred to as SIRP (Spherical Invariant Random P We show to i) Identify the SIRP-nature of a given data-stream; ii) Capitalize on this model to simulate the noise; iii) Easily implement an optimal Neyman-Pearson detector; Partial evidences of the effectiveness of the SIRP model for real IFO noise have been obtained by our workgroup for TAMA data and are obviously not included in this presentation.
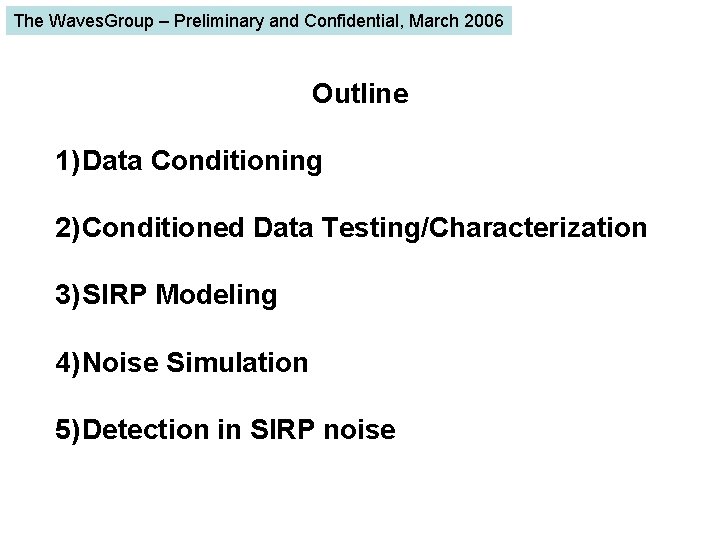
The Waves. Group – Preliminary and Confidential, March 2006 Outline 1) Data Conditioning 2) Conditioned Data Testing/Characterization 3) SIRP Modeling 4) Noise Simulation 5) Detection in SIRP noise
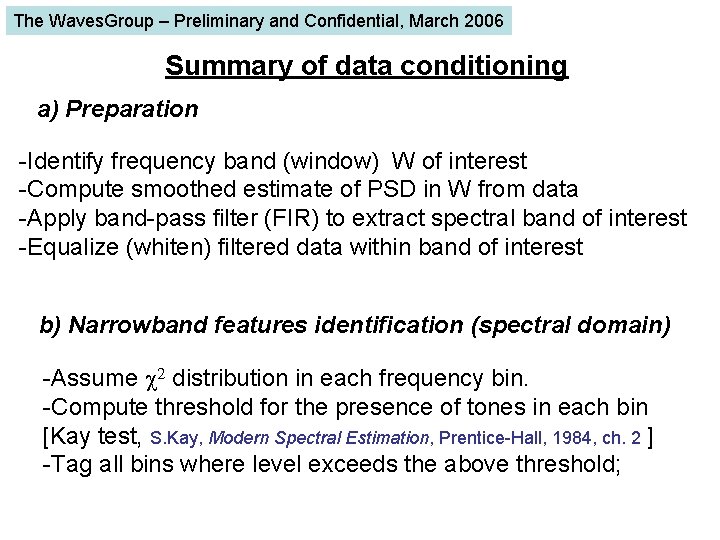
The Waves. Group – Preliminary and Confidential, March 2006 Summary of data conditioning a) Preparation -Identify frequency band (window) W of interest -Compute smoothed estimate of PSD in W from data -Apply band-pass filter (FIR) to extract spectral band of interest -Equalize (whiten) filtered data within band of interest b) Narrowband features identification (spectral domain) -Assume distribution in each frequency bin. -Compute threshold for the presence of tones in each bin [Kay test, S. Kay, Modern Spectral Estimation, Prentice-Hall, 1984, ch. 2 ] -Tag all bins where level exceeds the above threshold;
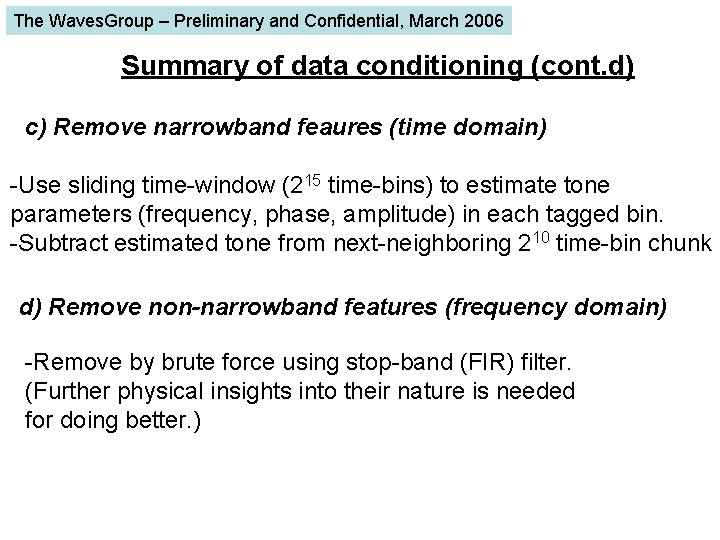
The Waves. Group – Preliminary and Confidential, March 2006 Summary of data conditioning (cont. d) c) Remove narrowband feaures (time domain) -Use sliding time-window (215 time-bins) to estimate tone parameters (frequency, phase, amplitude) in each tagged bin. -Subtract estimated tone from next-neighboring 210 time-bin chunk d) Remove non-narrowband features (frequency domain) -Remove by brute force using stop-band (FIR) filter. (Further physical insights into their nature is needed for doing better. )
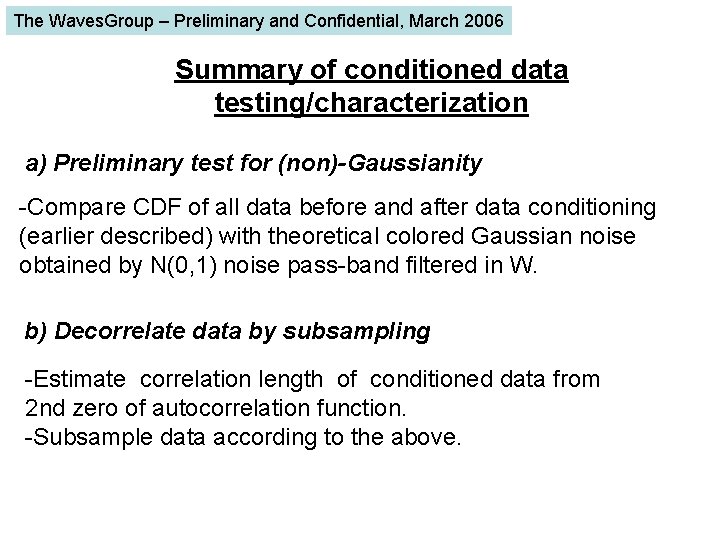
The Waves. Group – Preliminary and Confidential, March 2006 Summary of conditioned data testing/characterization a) Preliminary test for (non)-Gaussianity -Compare CDF of all data before and after data conditioning (earlier described) with theoretical colored Gaussian noise obtained by N(0, 1) noise pass-band filtered in W. b) Decorrelate data by subsampling -Estimate correlation length of conditioned data from 2 nd zero of autocorrelation function. -Subsample data according to the above.
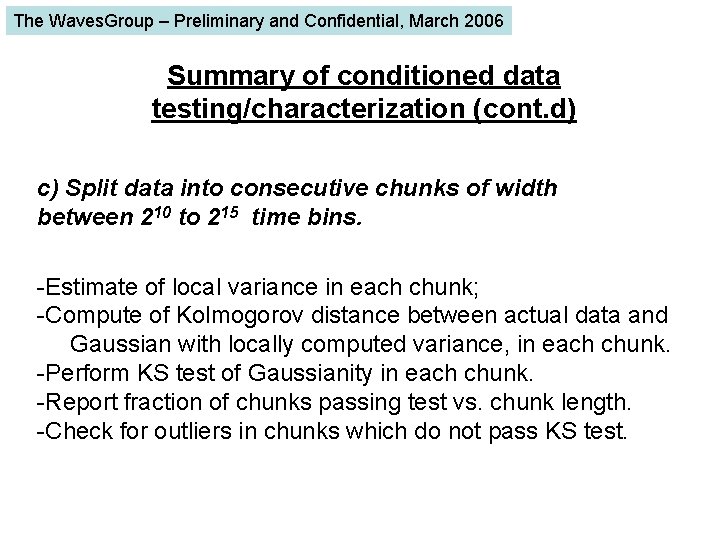
The Waves. Group – Preliminary and Confidential, March 2006 Summary of conditioned data testing/characterization (cont. d) c) Split data into consecutive chunks of width between 210 to 215 time bins. -Estimate of local variance in each chunk; -Compute of Kolmogorov distance between actual data and Gaussian with locally computed variance, in each chunk. -Perform KS test of Gaussianity in each chunk. -Report fraction of chunks passing test vs. chunk length. -Check for outliers in chunks which do not pass KS test.
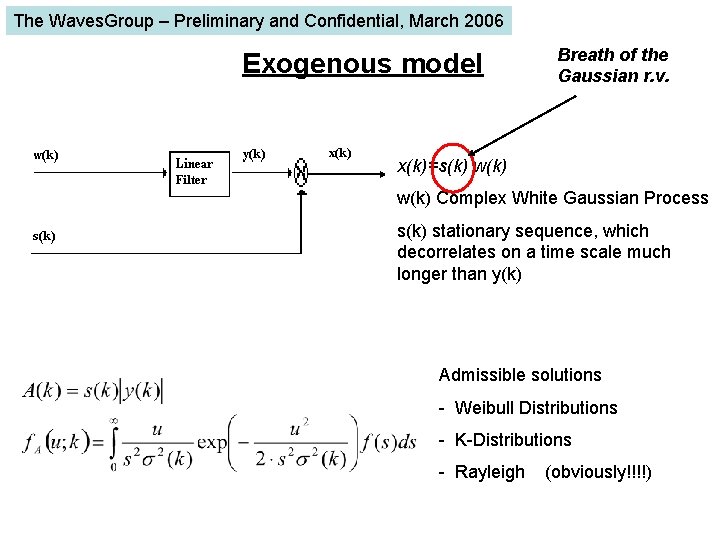
The Waves. Group – Preliminary and Confidential, March 2006 Exogenous model Breath of the Gaussian r. v. x(k)=s(k) w(k) Complex White Gaussian Process s(k) stationary sequence, which decorrelates on a time scale much longer than y(k) Admissible solutions - Weibull Distributions - K-Distributions - Rayleigh (obviously!!!!)
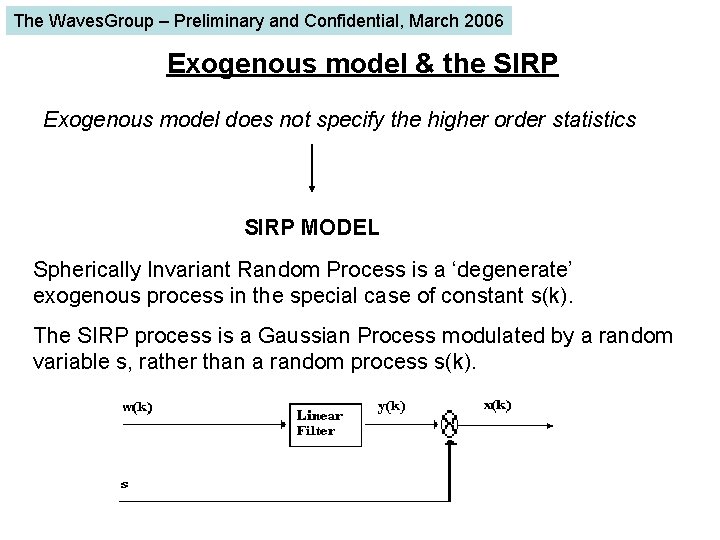
The Waves. Group – Preliminary and Confidential, March 2006 Exogenous model & the SIRP Exogenous model does not specify the higher order statistics SIRP MODEL Spherically Invariant Random Process is a ‘degenerate’ exogenous process in the special case of constant s(k). The SIRP process is a Gaussian Process modulated by a random variable s, rather than a random process s(k).
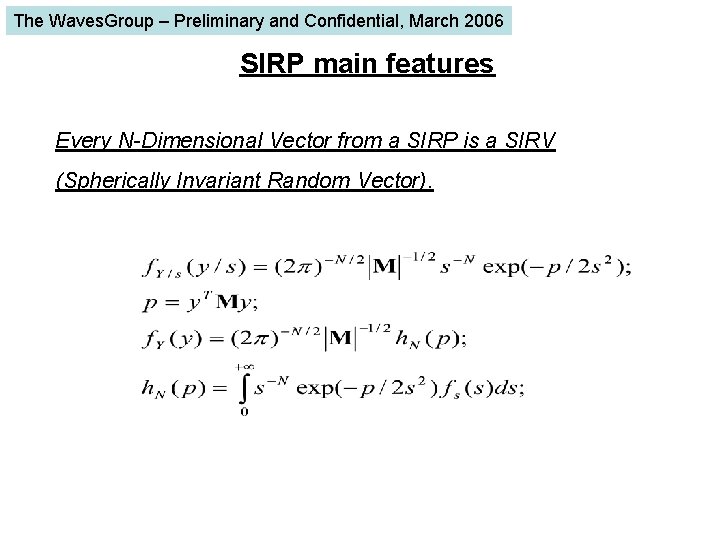
The Waves. Group – Preliminary and Confidential, March 2006 SIRP main features Every N-Dimensional Vector from a SIRP is a SIRV (Spherically Invariant Random Vector).
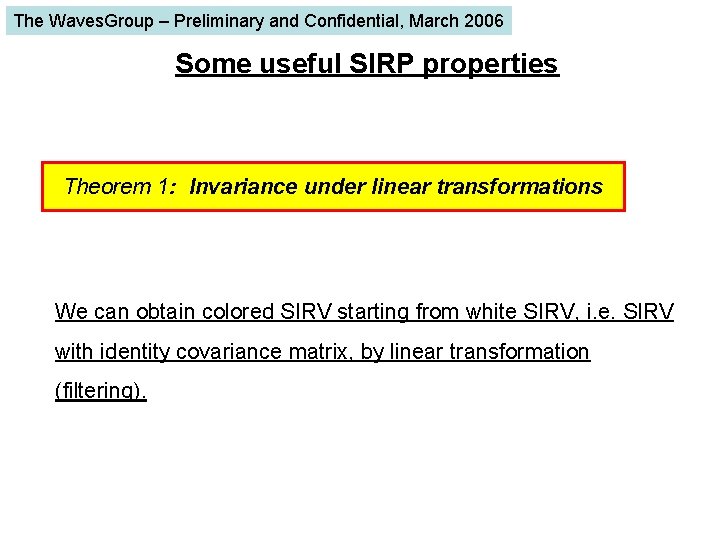
The Waves. Group – Preliminary and Confidential, March 2006 Some useful SIRP properties Theorem 1: Invariance under linear transformations We can obtain colored SIRV starting from white SIRV, i. e. SIRV with identity covariance matrix, by linear transformation (filtering).
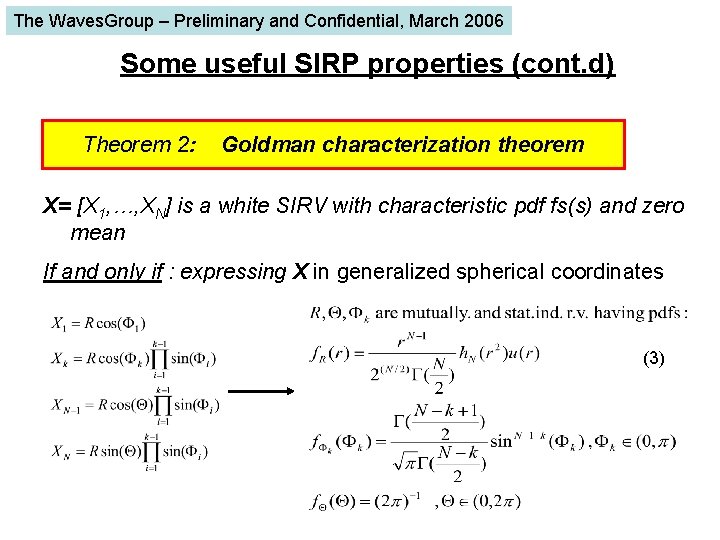
The Waves. Group – Preliminary and Confidential, March 2006 Some useful SIRP properties (cont. d) Theorem 2: Goldman characterization theorem X= [X 1, …, XN] is a white SIRV with characteristic pdf fs(s) and zero mean If and only if : expressing X in generalized spherical coordinates (3)
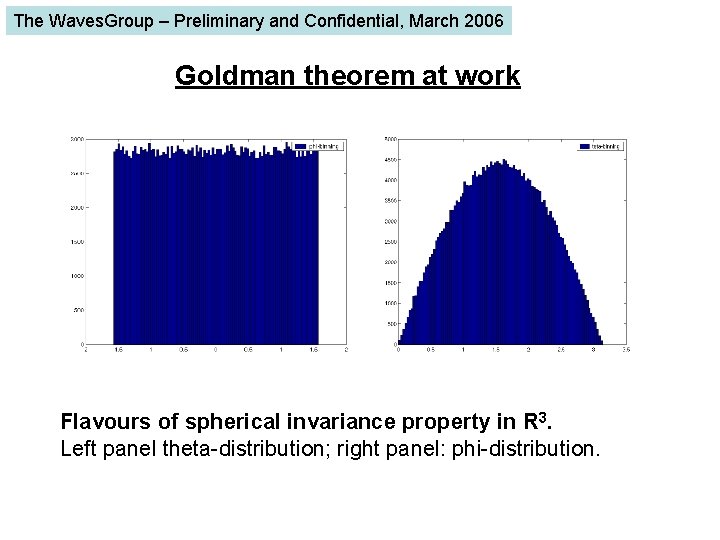
The Waves. Group – Preliminary and Confidential, March 2006 Goldman theorem at work Flavours of spherical invariance property in R 3. Left panel theta-distribution; right panel: phi-distribution.
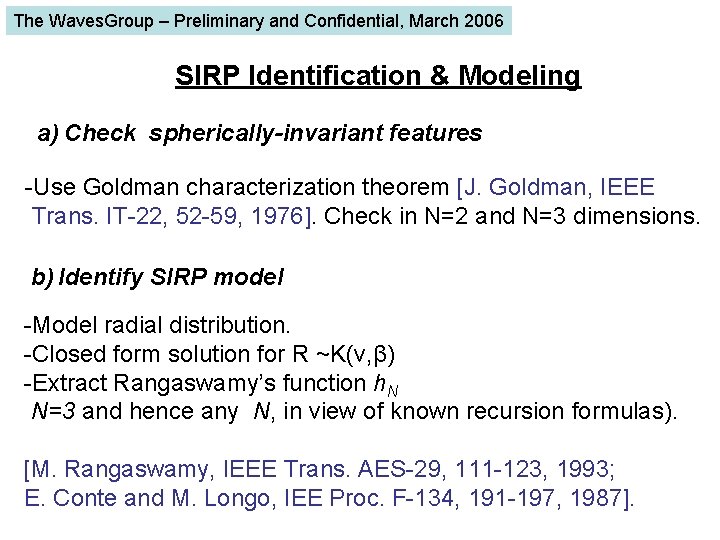
The Waves. Group – Preliminary and Confidential, March 2006 SIRP Identification & Modeling a) Check spherically-invariant features -Use Goldman characterization theorem [J. Goldman, IEEE Trans. IT-22, 52 -59, 1976]. Check in N=2 and N=3 dimensions. b) Identify SIRP model -Model radial distribution. -Closed form solution for R ~K(v, β) -Extract Rangaswamy’s function h. N N=3 and hence any N, in view of known recursion formulas). [M. Rangaswamy, IEEE Trans. AES-29, 111 -123, 1993; E. Conte and M. Longo, IEE Proc. F-134, 191 -197, 1987].
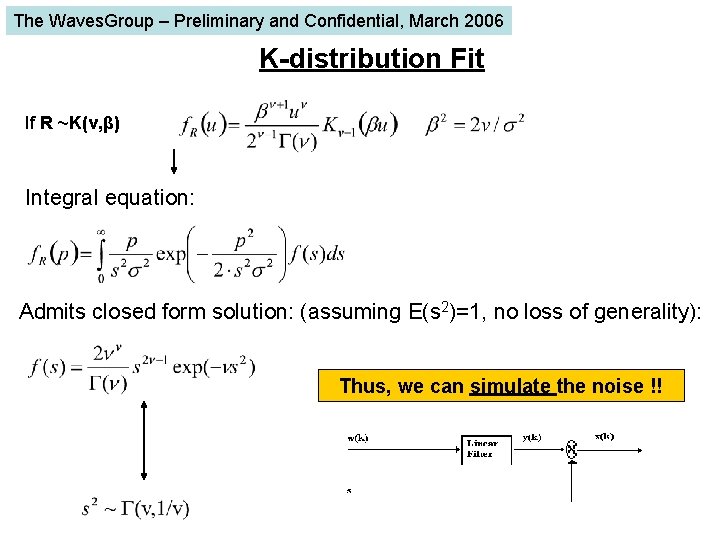
The Waves. Group – Preliminary and Confidential, March 2006 K-distribution Fit If R ~K(v, β) Integral equation: Admits closed form solution: (assuming E(s 2)=1, no loss of generality): Thus, we can simulate the noise !!
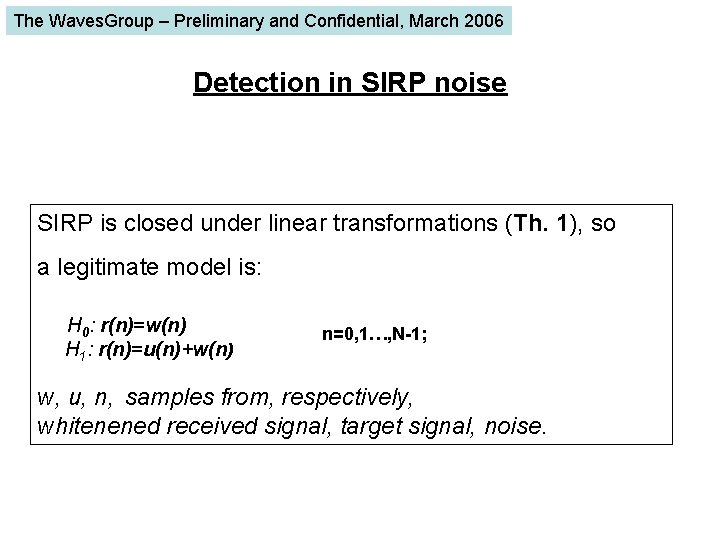
The Waves. Group – Preliminary and Confidential, March 2006 Detection in SIRP noise SIRP is closed under linear transformations (Th. 1), so a legitimate model is: H 0: r(n)=w(n) H 1: r(n)=u(n)+w(n) n=0, 1…, N-1; w, u, n, samples from, respectively, whitenened received signal, target signal, noise.
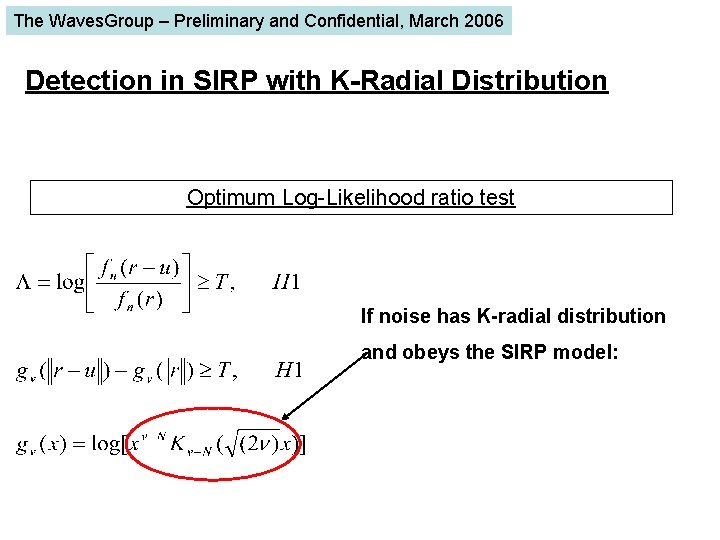
The Waves. Group – Preliminary and Confidential, March 2006 Detection in SIRP with K-Radial Distribution Optimum Log-Likelihood ratio test If noise has K-radial distribution and obeys the SIRP model:
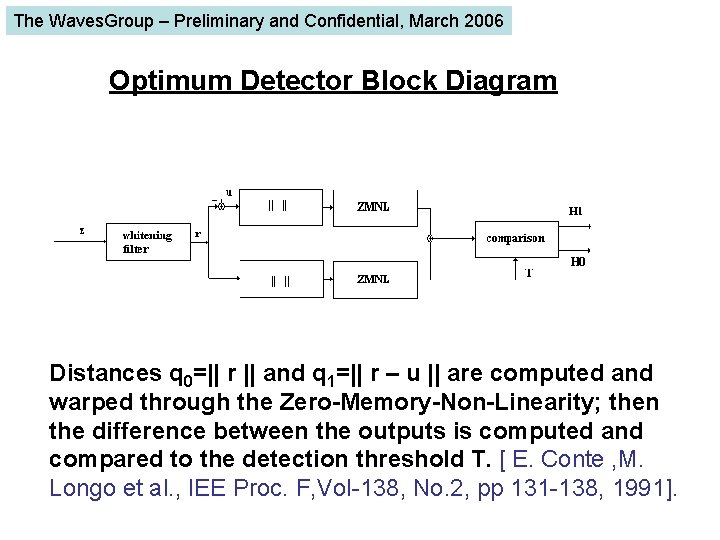
The Waves. Group – Preliminary and Confidential, March 2006 Optimum Detector Block Diagram Distances q 0=|| r || and q 1=|| r – u || are computed and warped through the Zero-Memory-Non-Linearity; then the difference between the outputs is computed and compared to the detection threshold T. [ E. Conte , M. Longo et al. , IEE Proc. F, Vol-138, No. 2, pp 131 -138, 1991].
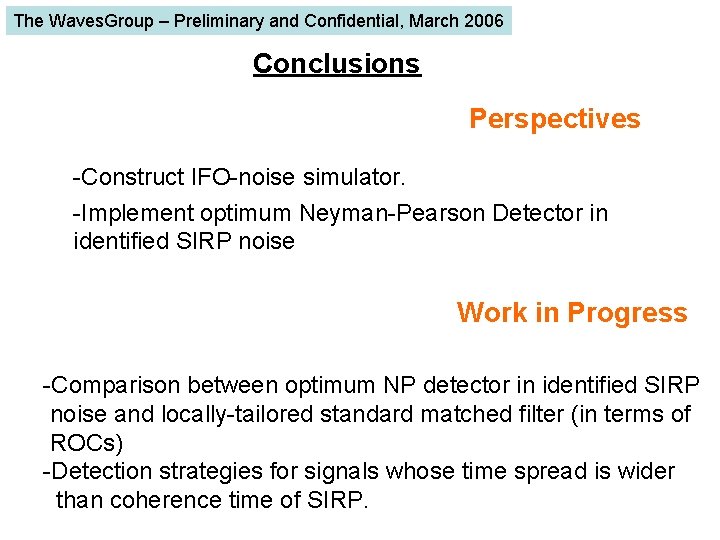
The Waves. Group – Preliminary and Confidential, March 2006 Conclusions Perspectives -Construct IFO-noise simulator. -Implement optimum Neyman-Pearson Detector in identified SIRP noise Work in Progress -Comparison between optimum NP detector in identified SIRP noise and locally-tailored standard matched filter (in terms of ROCs) -Detection strategies for signals whose time spread is wider than coherence time of SIRP.
Innocenzo iii
Innocenzo ix baceno
Istoria telefonului
Role modeling theory
Relational vs dimensional data modeling
Data modeling best practices for data warehousing
Modeling relational data with graph convolutional networks
Idefix notation
Data modeling using entity relationship model
Data warehouse modeling tutorial
Modeling data in the organization
Modeling data in the organization
Qlik circular reference
Vhdl data flow modeling
Oltp data model
Real world sinusoidal functions
Modeling data distributions
Er modeler
Data flow modeling in verilog
Data vault modeling pros and cons