See Also Auto Generated Recommendations Mislav Cimperak Marija
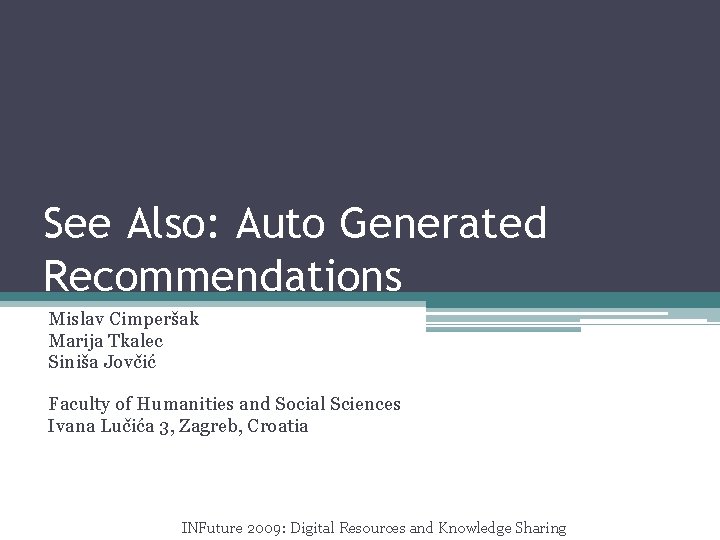
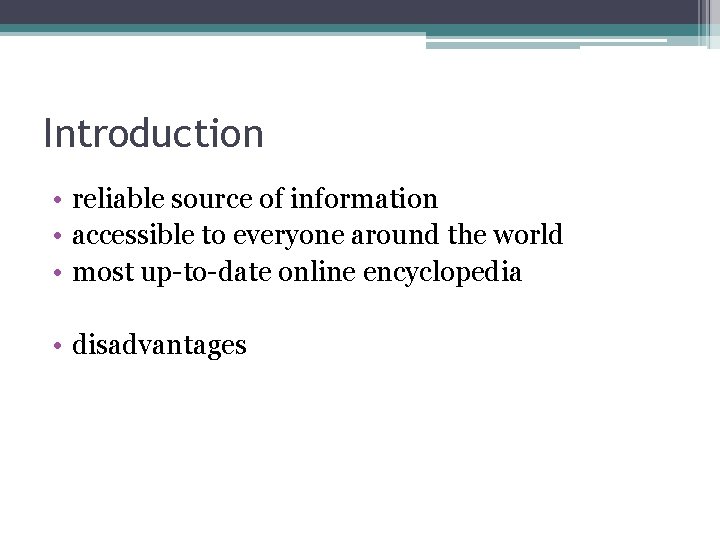
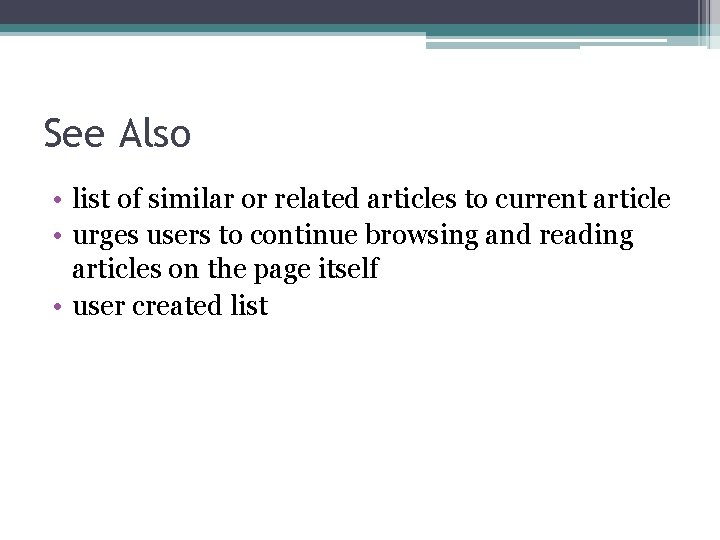
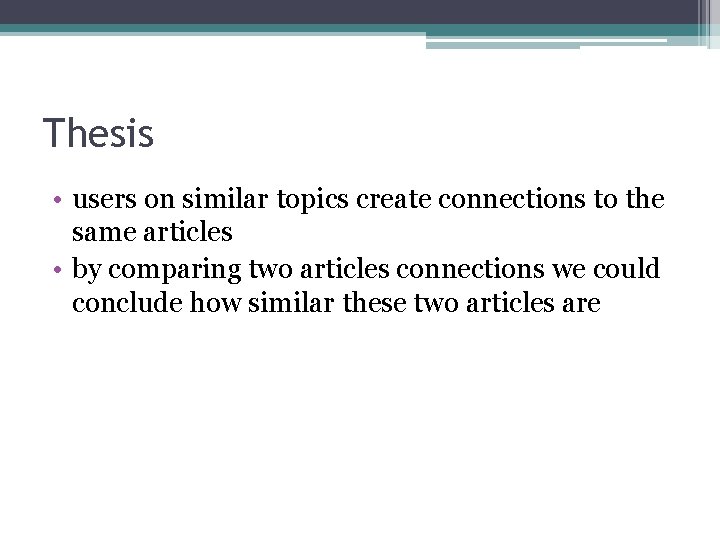
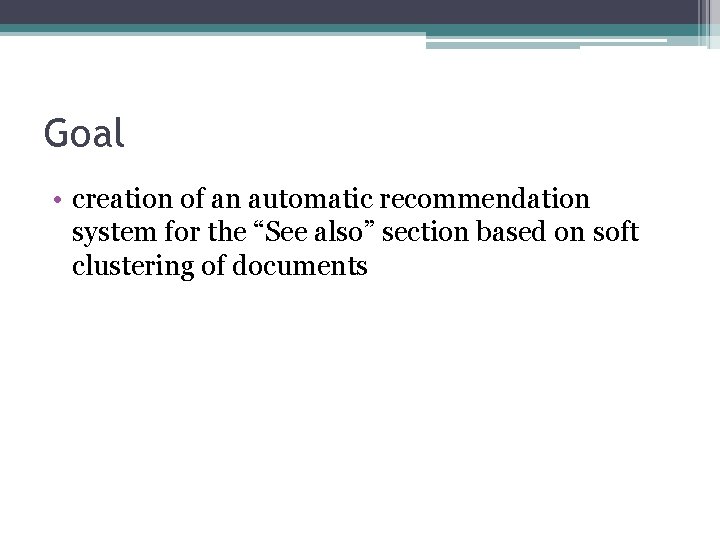
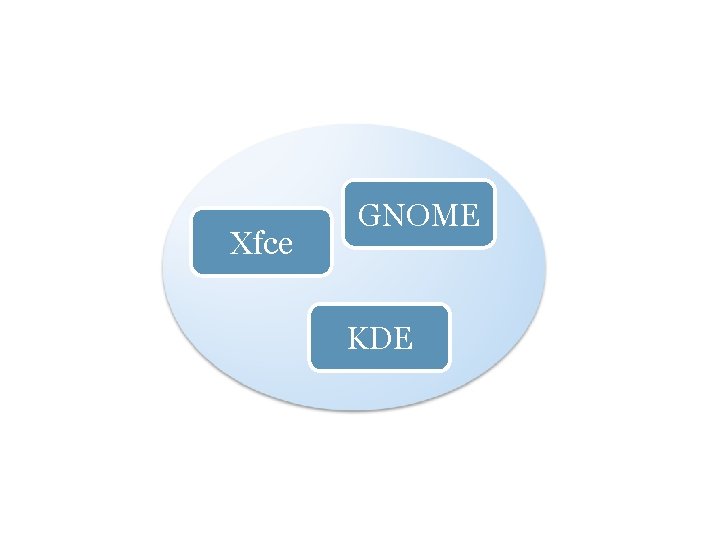
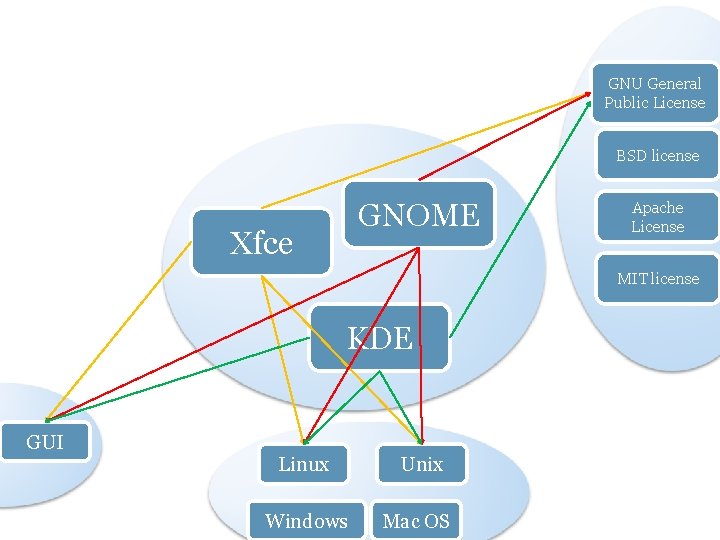
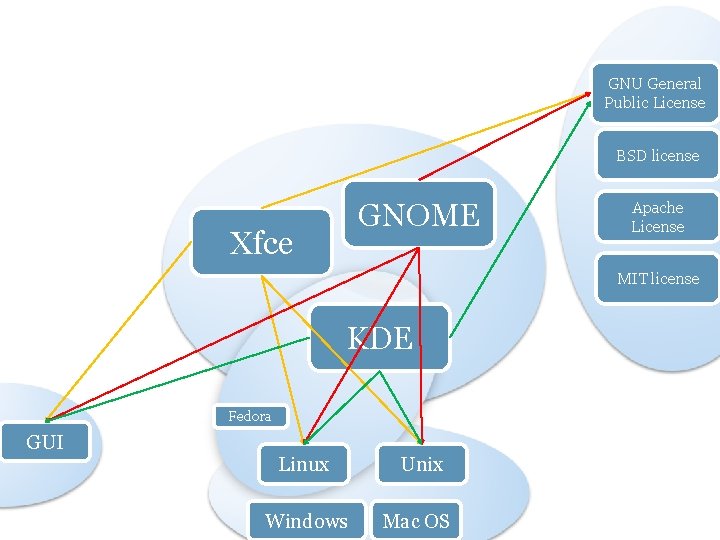
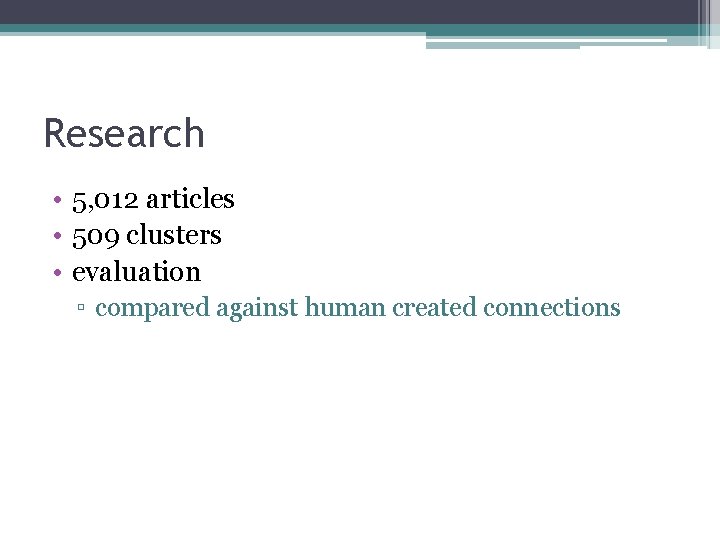
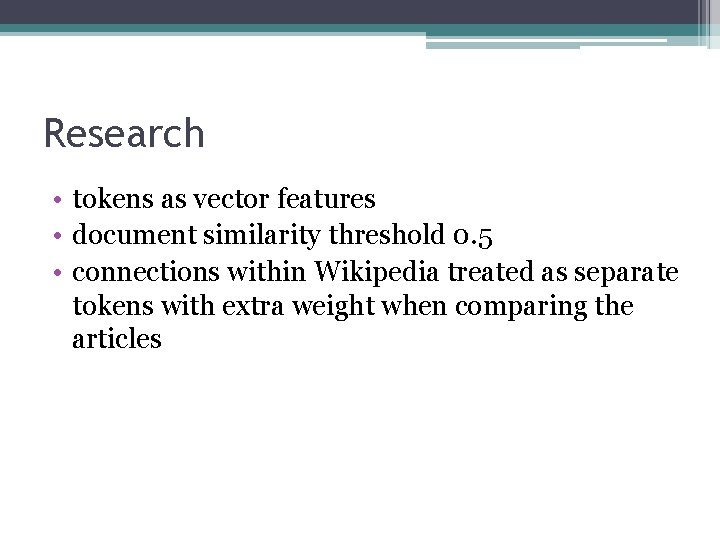
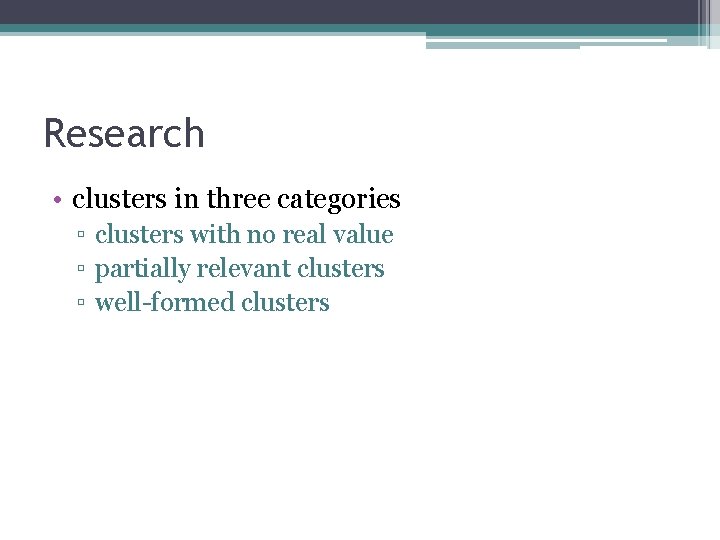
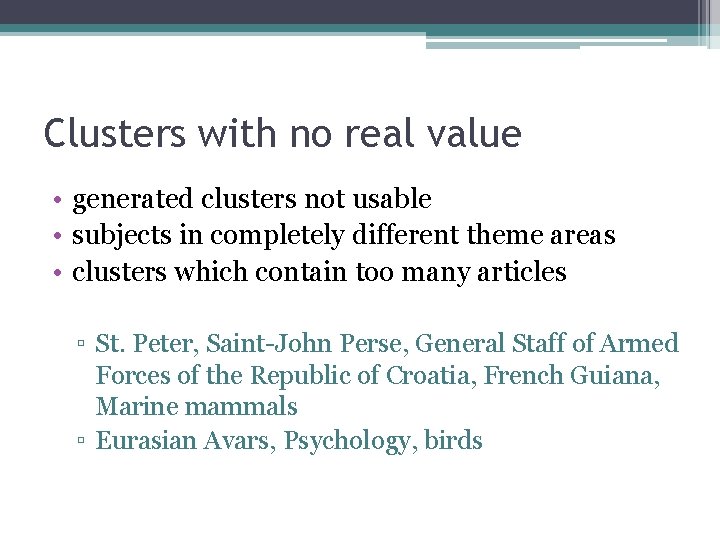
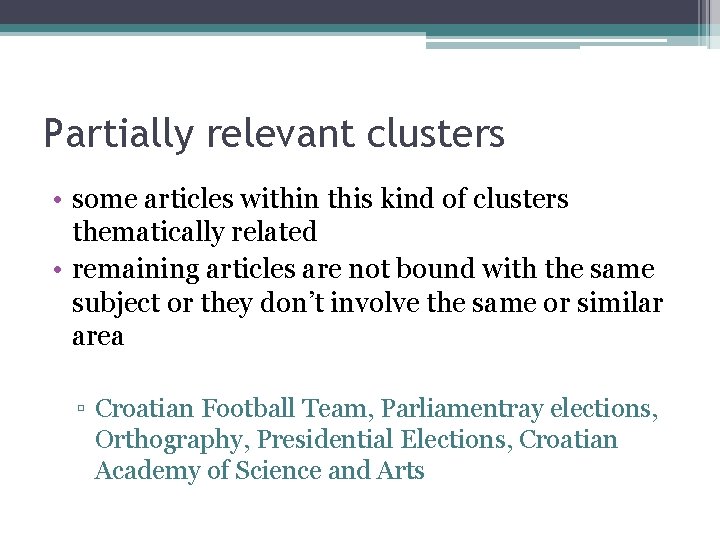
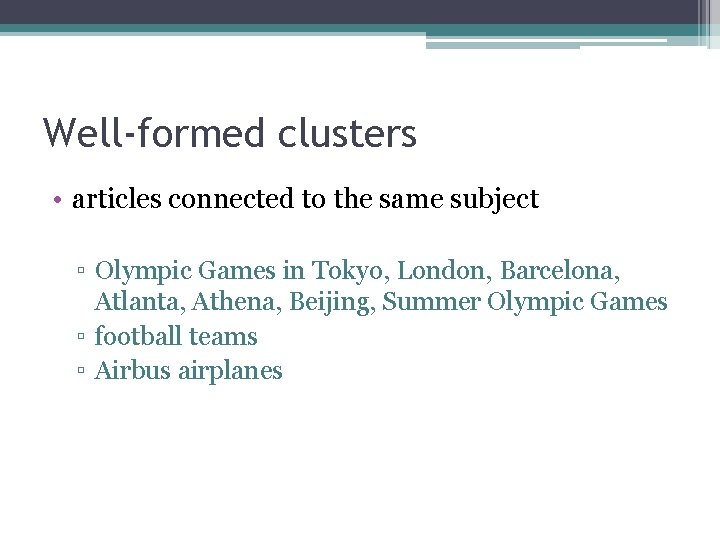
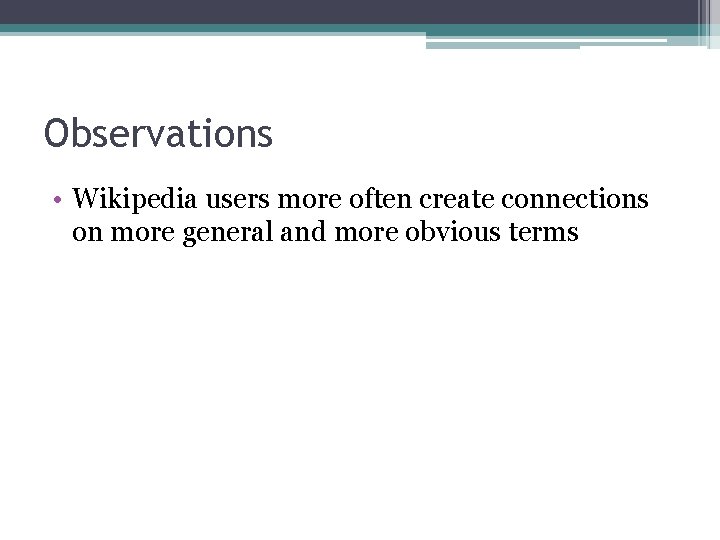
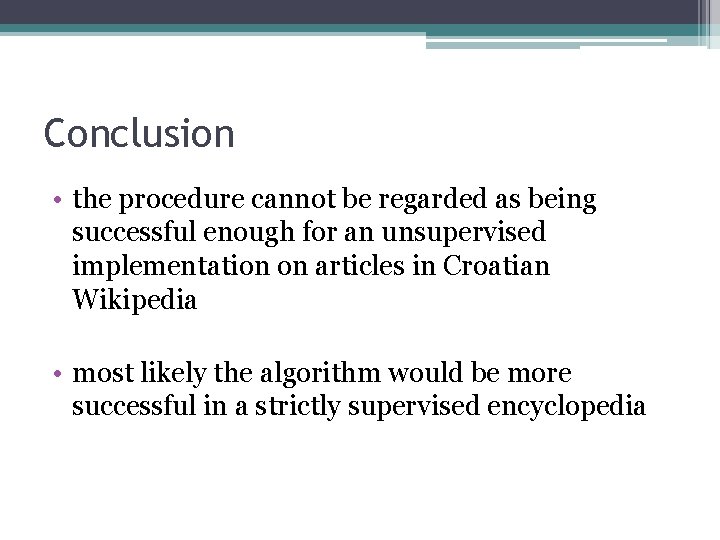
- Slides: 16
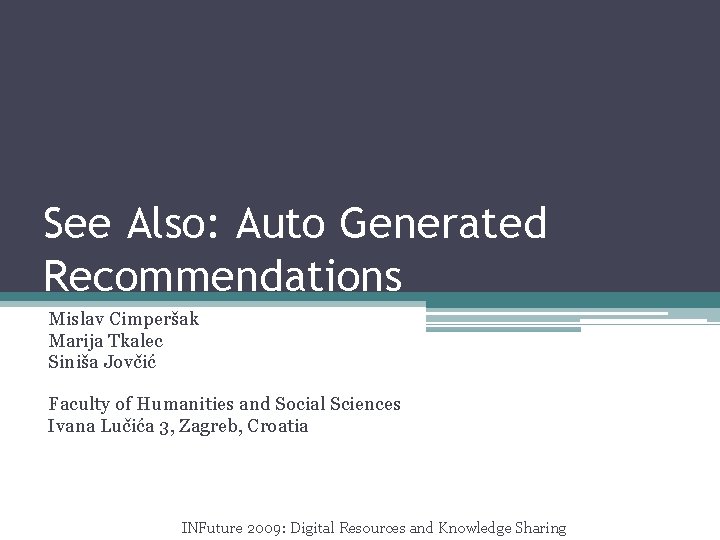
See Also: Auto Generated Recommendations Mislav Cimperšak Marija Tkalec Siniša Jovčić Faculty of Humanities and Social Sciences Ivana Lučića 3, Zagreb, Croatia INFuture 2009: Digital Resources and Knowledge Sharing
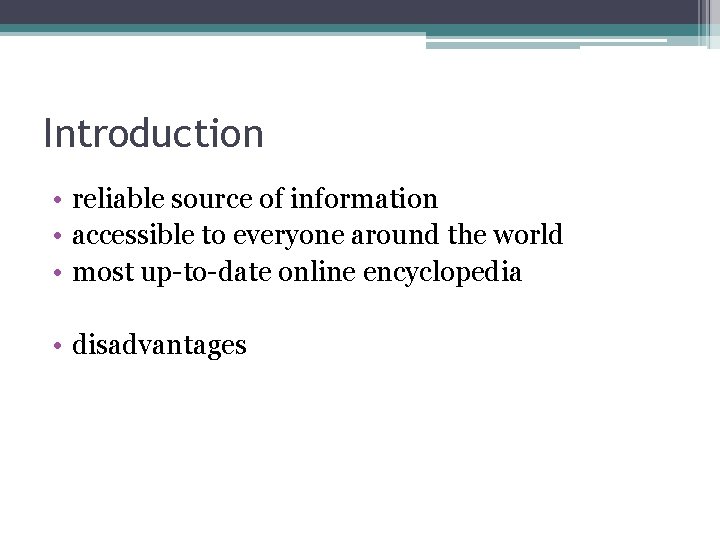
Introduction • reliable source of information • accessible to everyone around the world • most up-to-date online encyclopedia • disadvantages
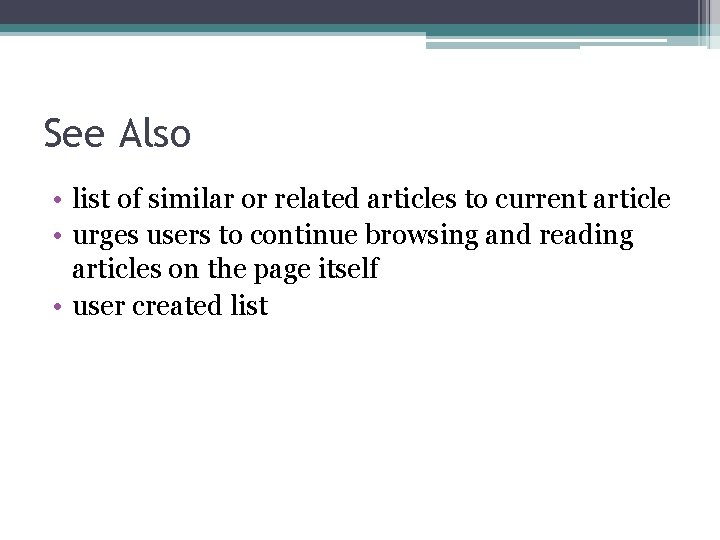
See Also • list of similar or related articles to current article • urges users to continue browsing and reading articles on the page itself • user created list
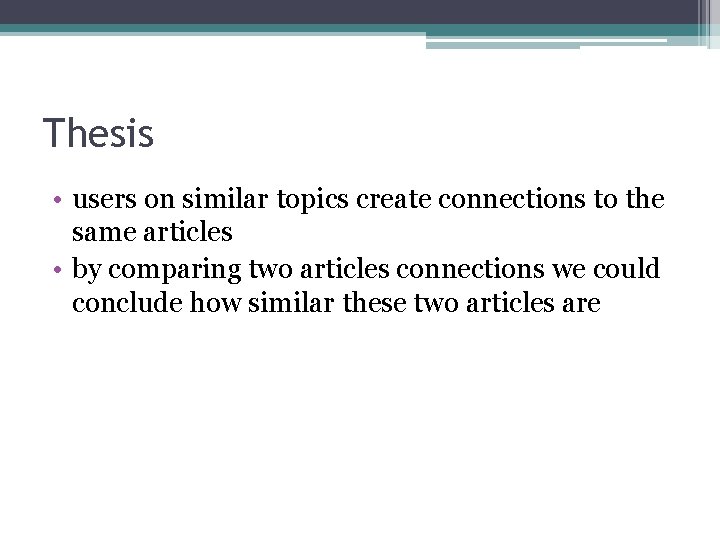
Thesis • users on similar topics create connections to the same articles • by comparing two articles connections we could conclude how similar these two articles are
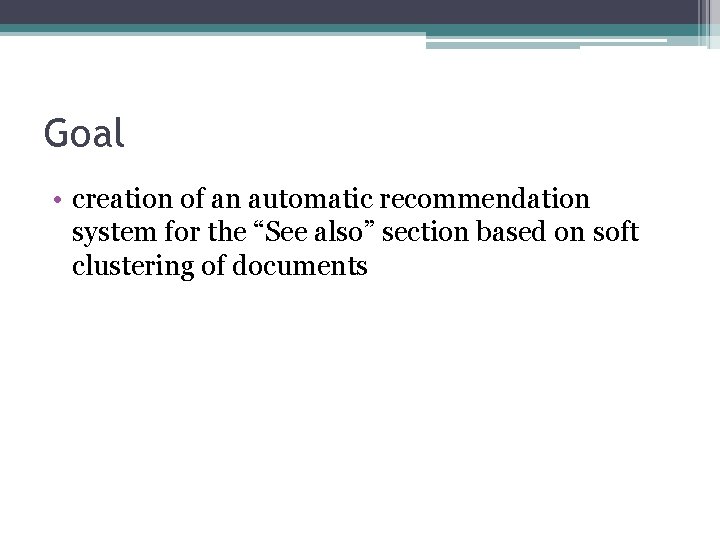
Goal • creation of an automatic recommendation system for the “See also” section based on soft clustering of documents
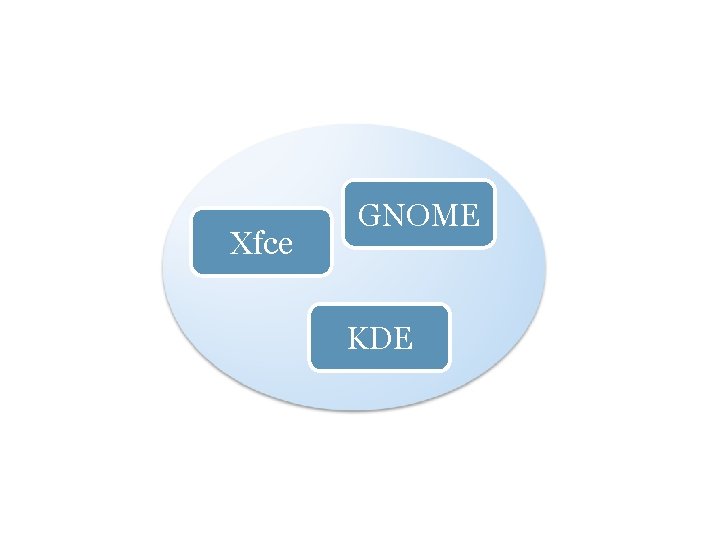
Xfce GNOME KDE
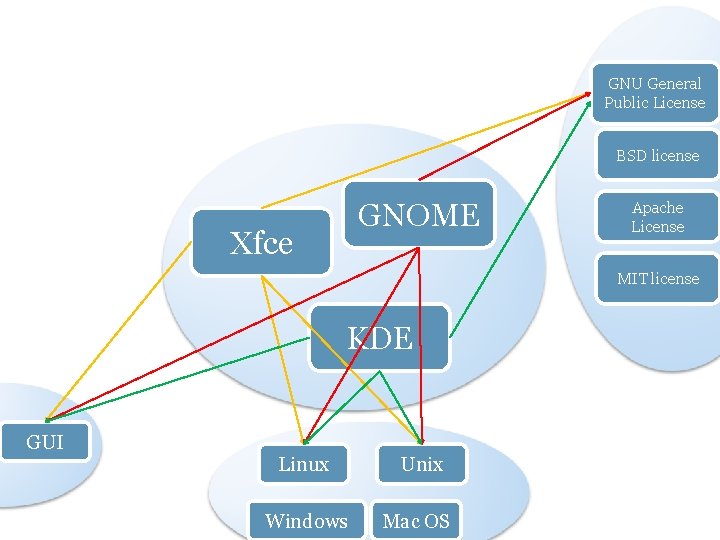
GNU General Public License BSD license GNOME Xfce Apache License MIT license KDE GUI Linux Unix Windows Mac OS
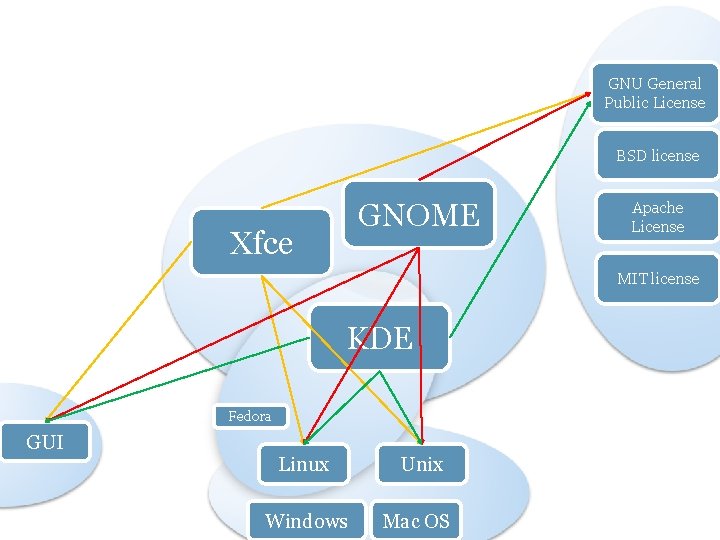
GNU General Public License BSD license GNOME Xfce Apache License MIT license KDE Fedora GUI Linux Unix Windows Mac OS
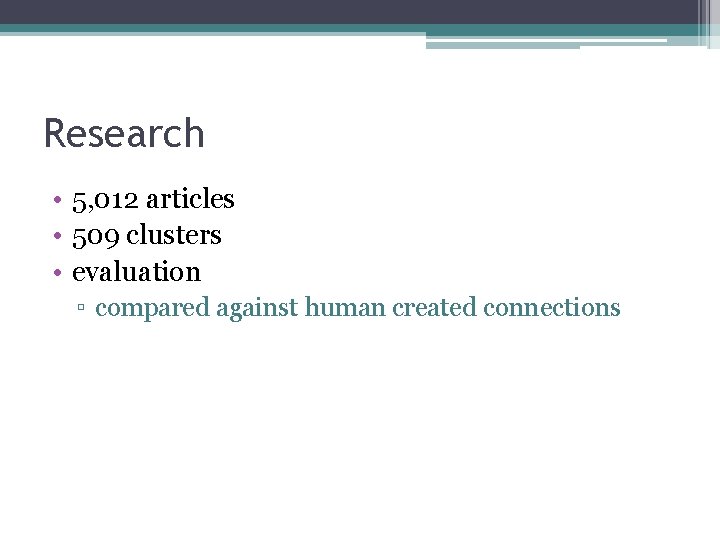
Research • 5, 012 articles • 509 clusters • evaluation ▫ compared against human created connections
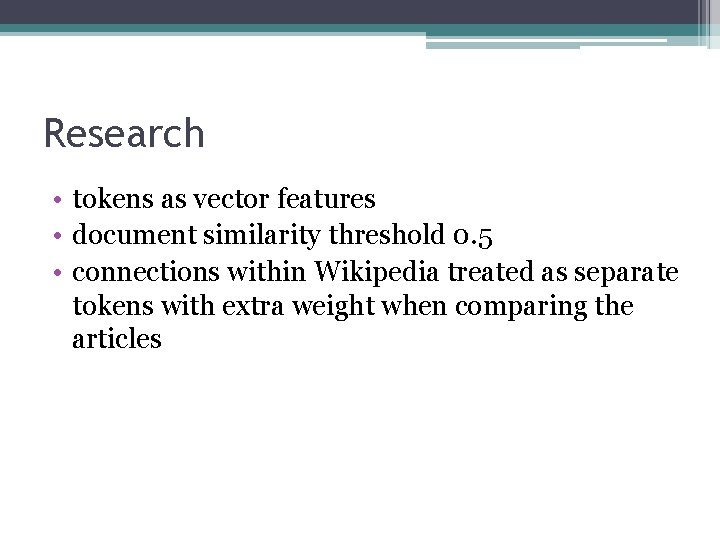
Research • tokens as vector features • document similarity threshold 0. 5 • connections within Wikipedia treated as separate tokens with extra weight when comparing the articles
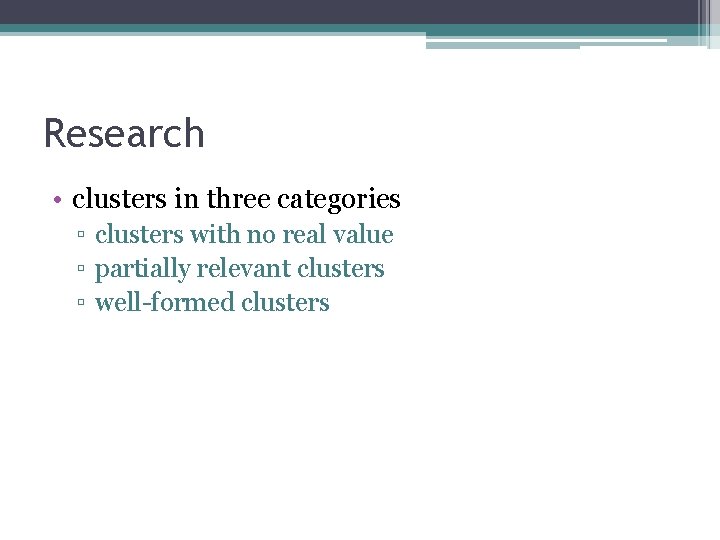
Research • clusters in three categories ▫ clusters with no real value ▫ partially relevant clusters ▫ well-formed clusters
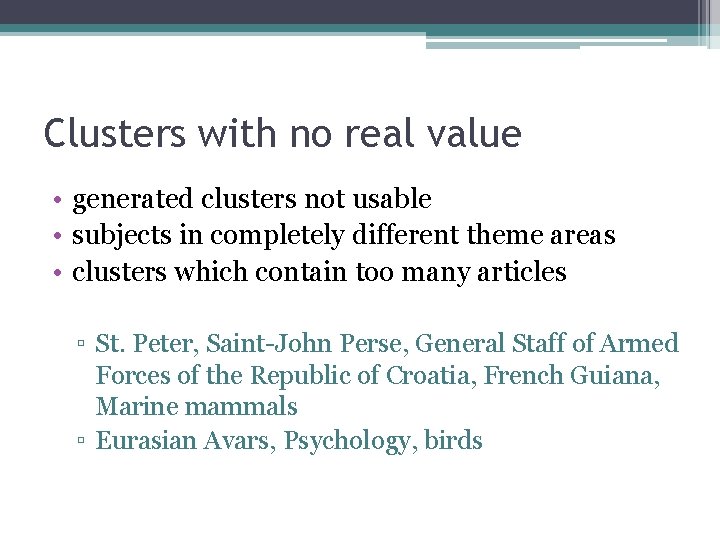
Clusters with no real value • generated clusters not usable • subjects in completely different theme areas • clusters which contain too many articles ▫ St. Peter, Saint-John Perse, General Staff of Armed Forces of the Republic of Croatia, French Guiana, Marine mammals ▫ Eurasian Avars, Psychology, birds
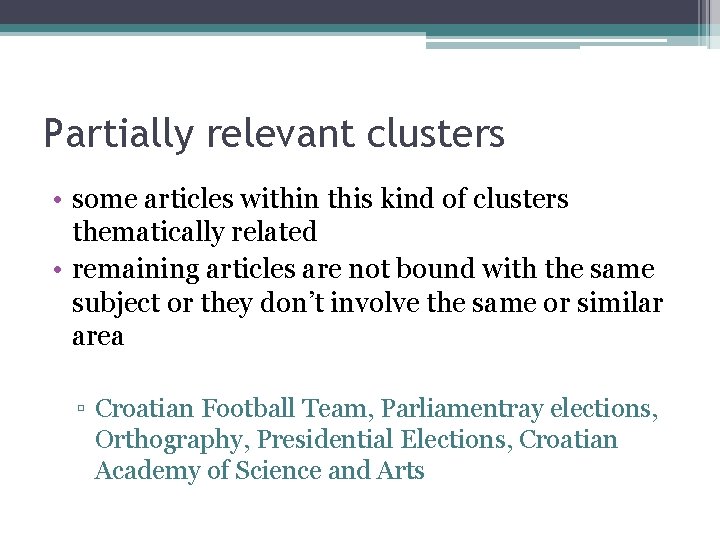
Partially relevant clusters • some articles within this kind of clusters thematically related • remaining articles are not bound with the same subject or they don’t involve the same or similar area ▫ Croatian Football Team, Parliamentray elections, Orthography, Presidential Elections, Croatian Academy of Science and Arts
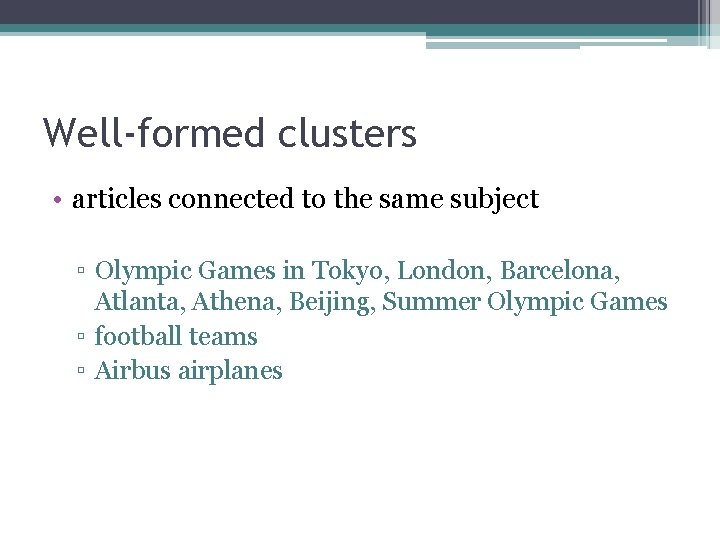
Well-formed clusters • articles connected to the same subject ▫ Olympic Games in Tokyo, London, Barcelona, Atlanta, Athena, Beijing, Summer Olympic Games ▫ football teams ▫ Airbus airplanes
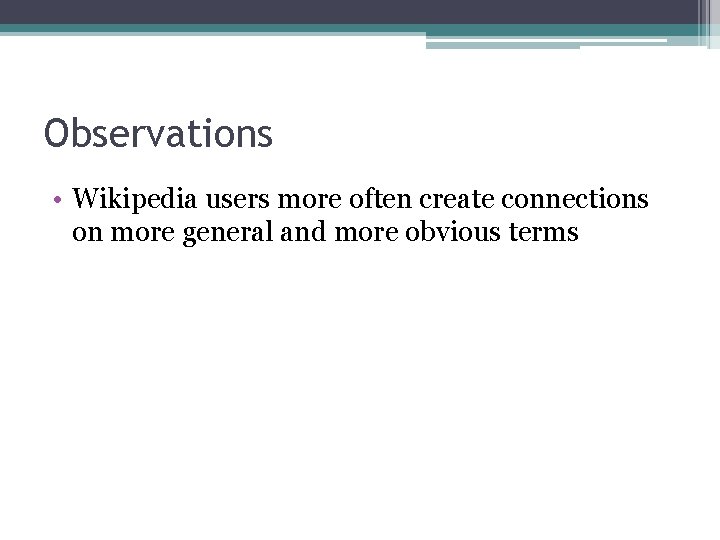
Observations • Wikipedia users more often create connections on more general and more obvious terms
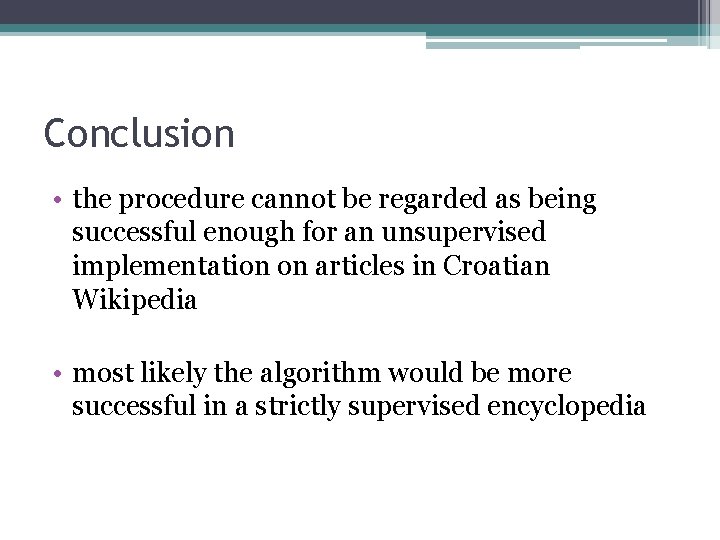
Conclusion • the procedure cannot be regarded as being successful enough for an unsupervised implementation on articles in Croatian Wikipedia • most likely the algorithm would be more successful in a strictly supervised encyclopedia
Mislav herman
Mislav radić
Carnet sigma
Energija plime i oseke
An 8 pole dc shunt generator with 778 wave
Image by generated photos
Chemistry of life
What is presentation graphics software
How does a battery work
Computer generated report
User generated content mobile
Microprocessor are
What is the angle generated by a clockwise rotation?
Can backdated udin be generated
User generated content journalism
How lift is generated
Electric current is generated due to