SATURN An Overview Shrawan Kumar Shrawan kumartcs com
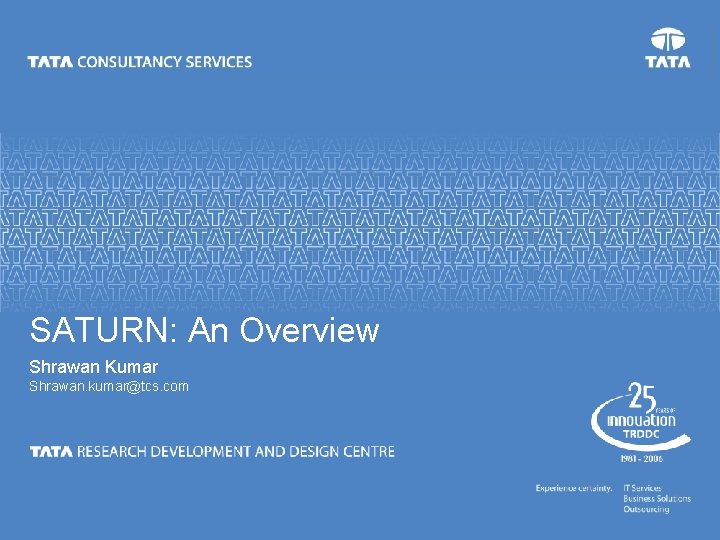
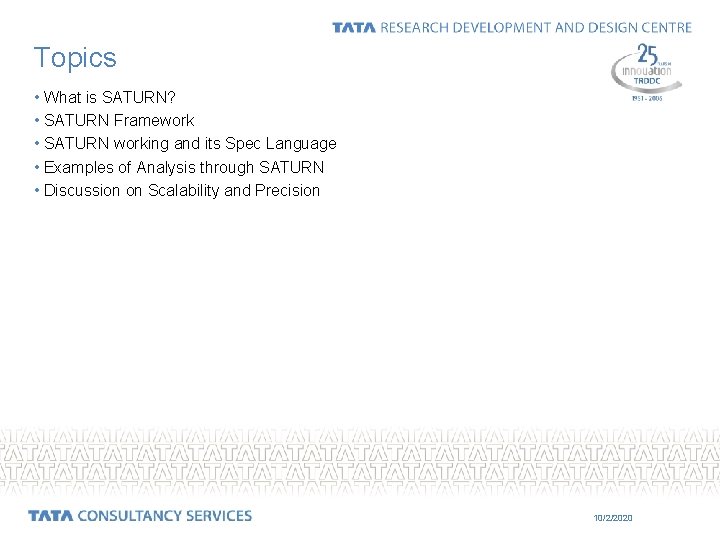
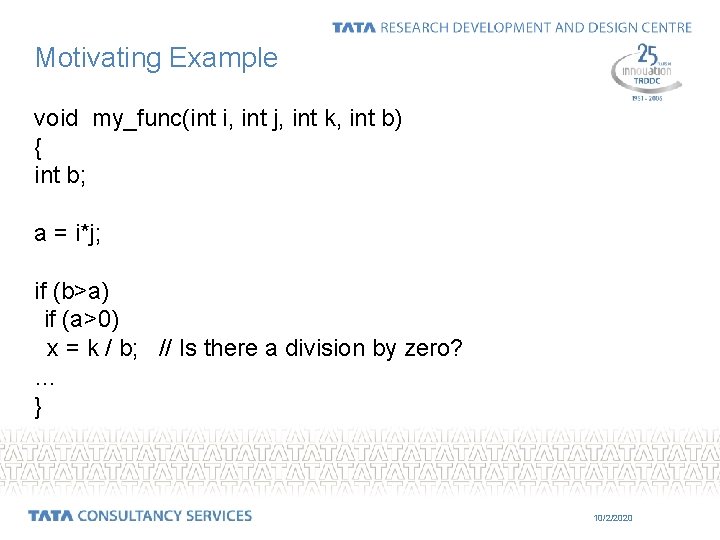
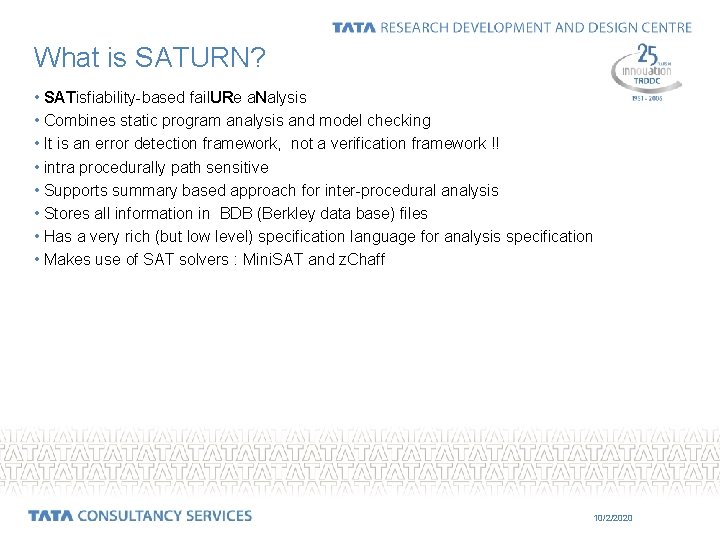
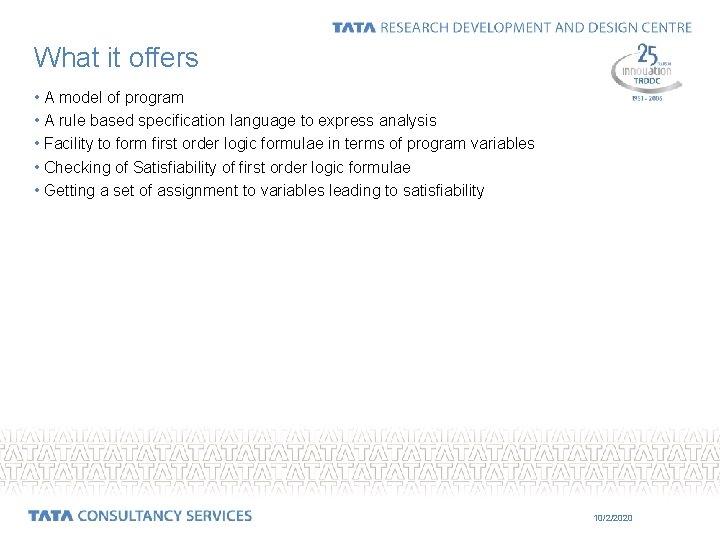
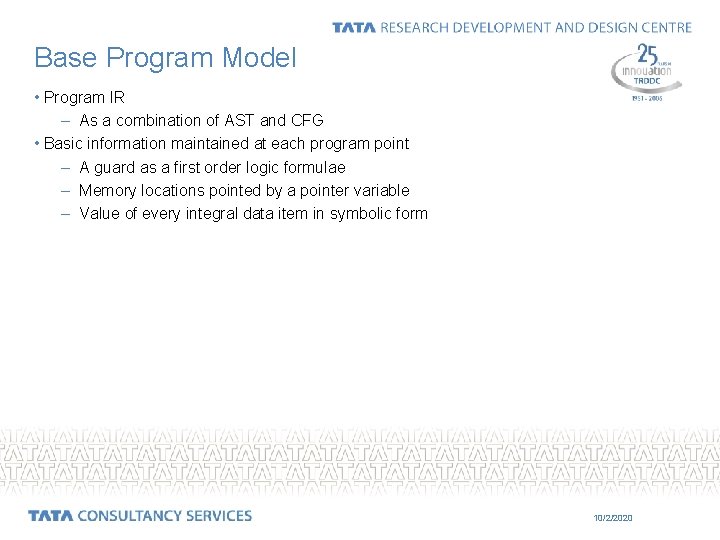
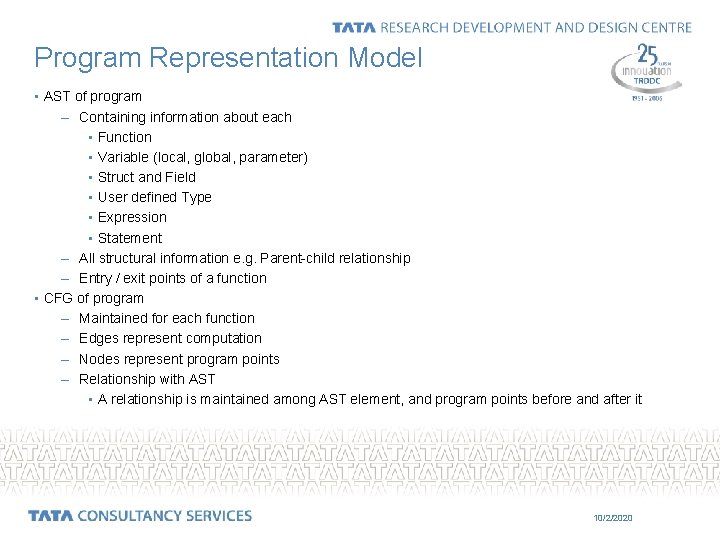
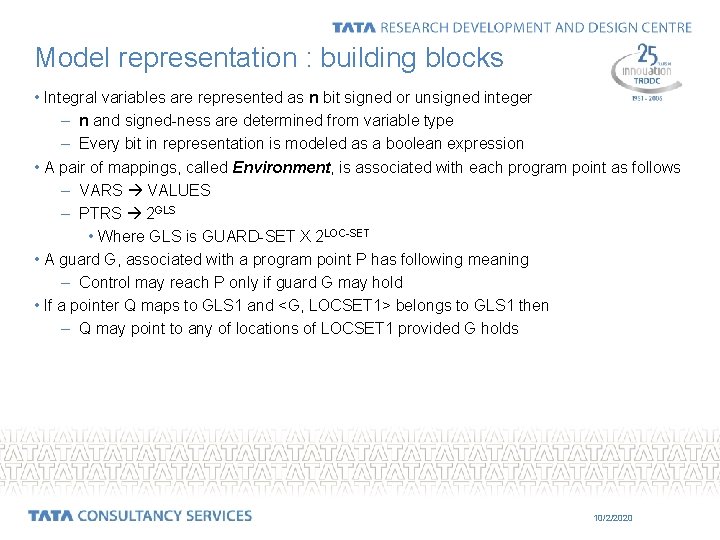
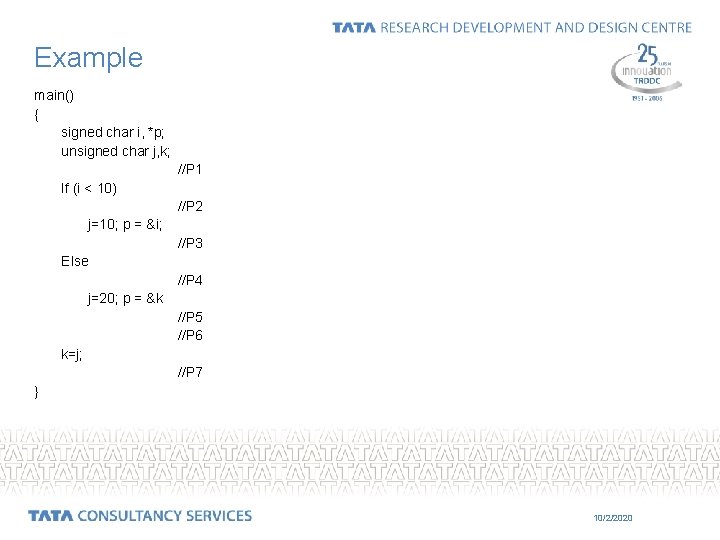
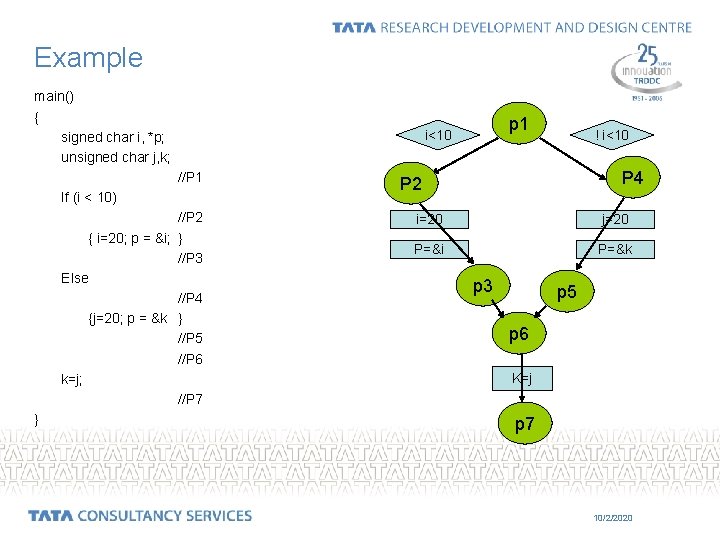
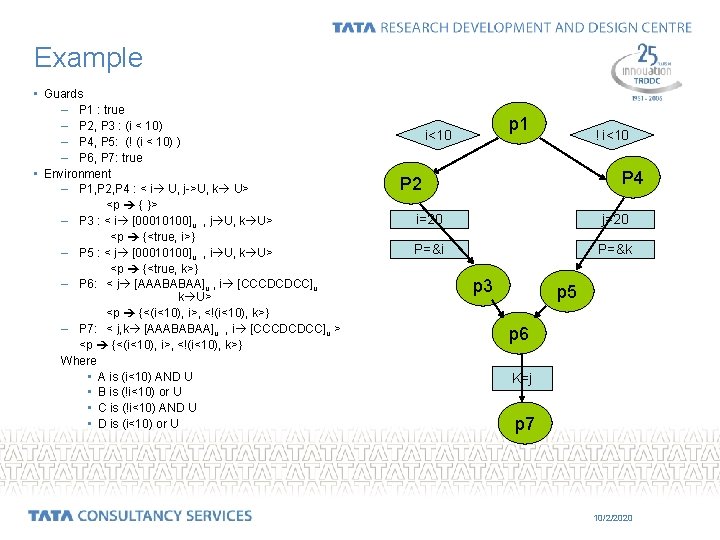
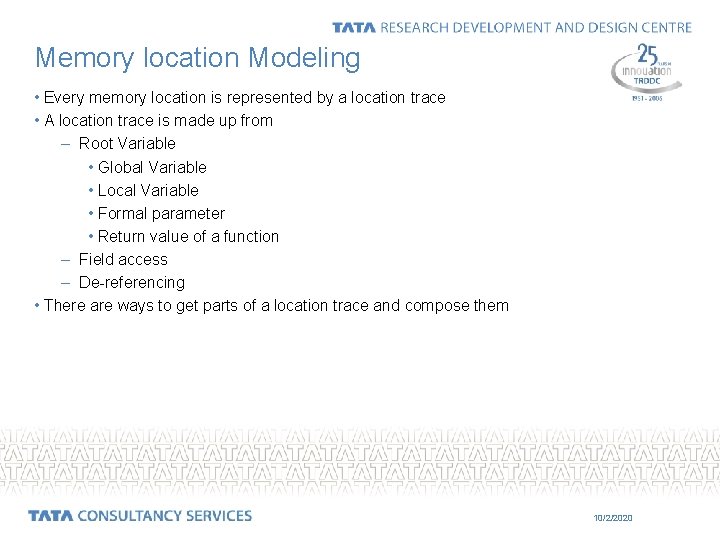
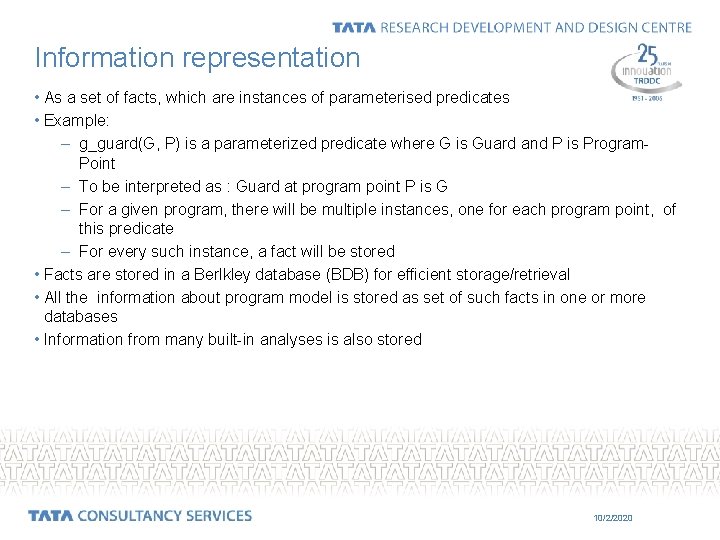
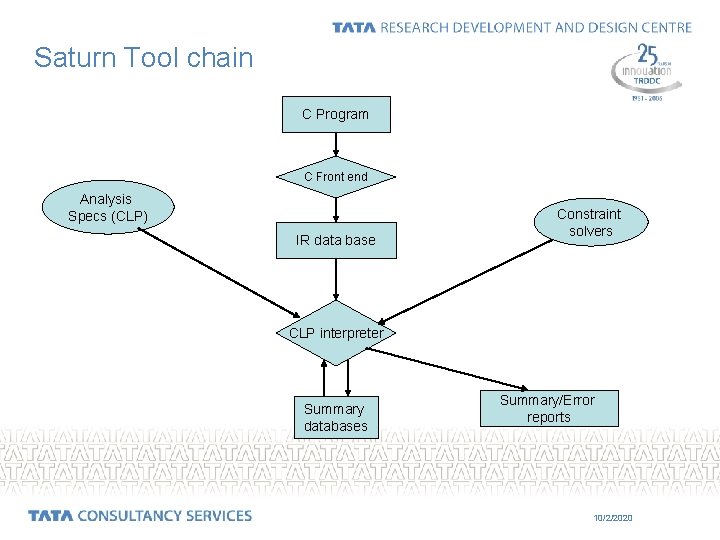
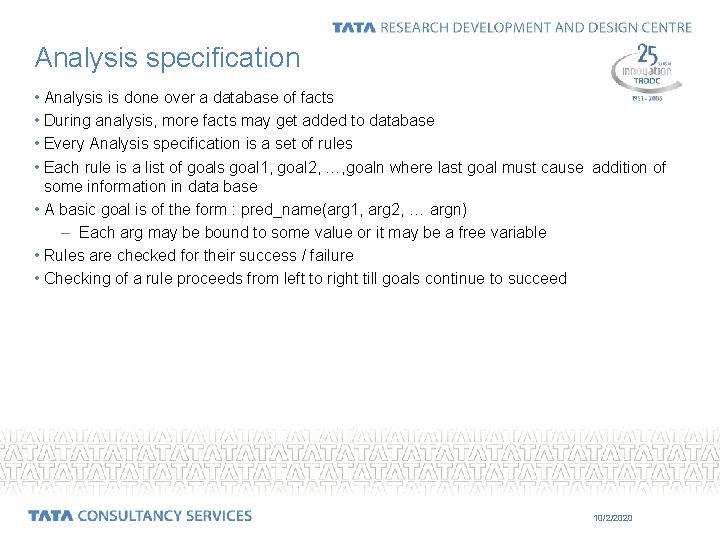
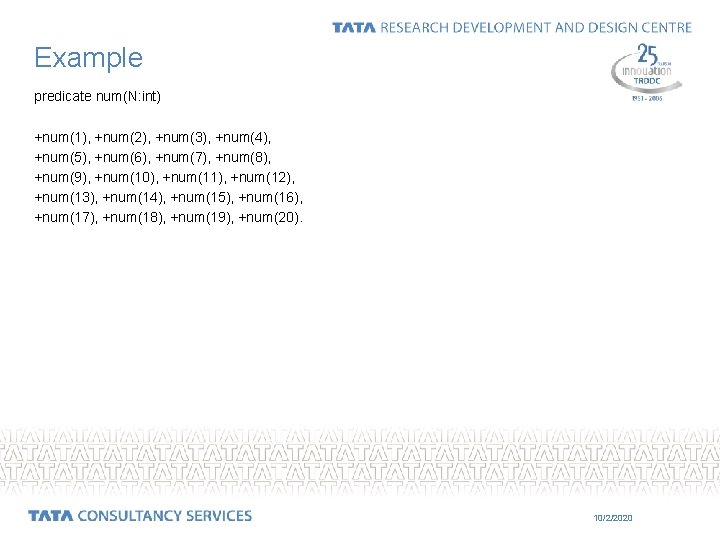
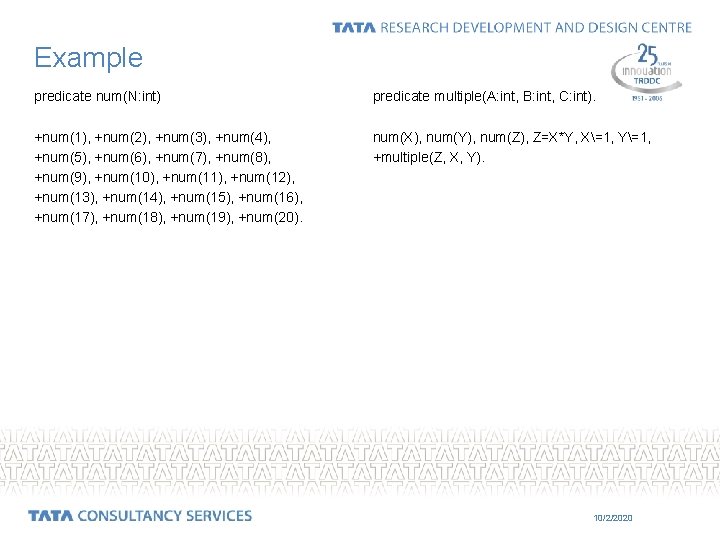
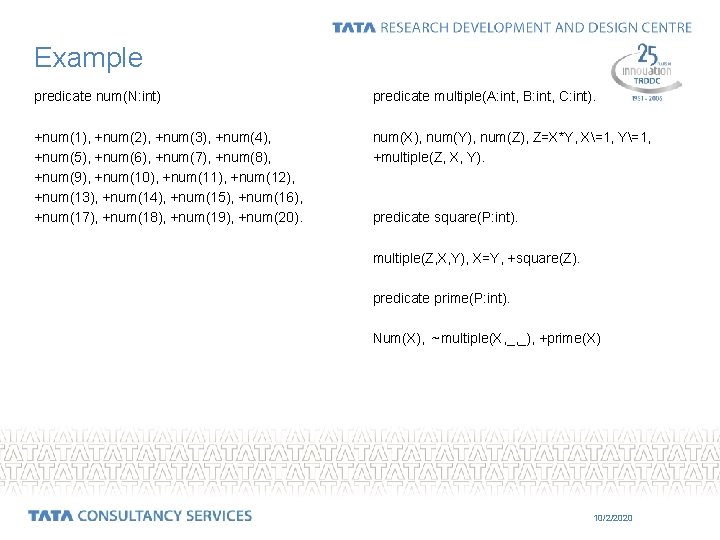
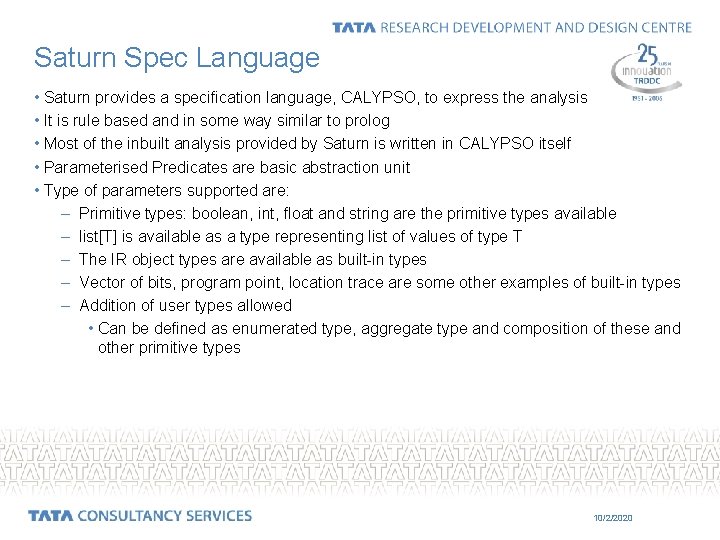
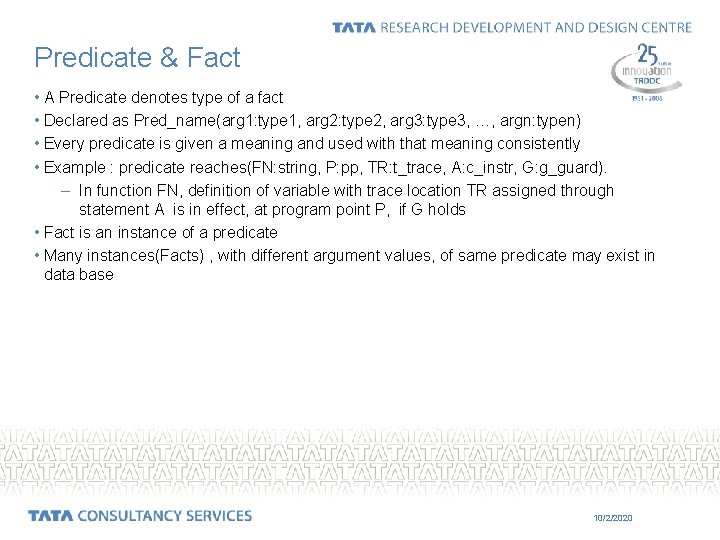
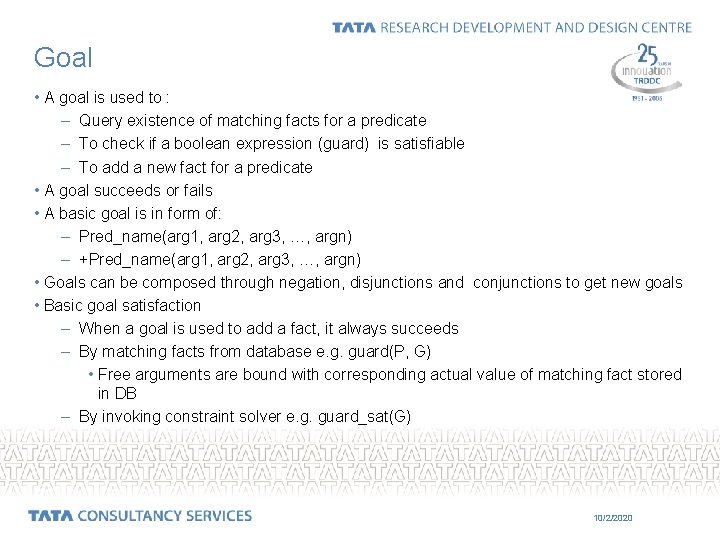
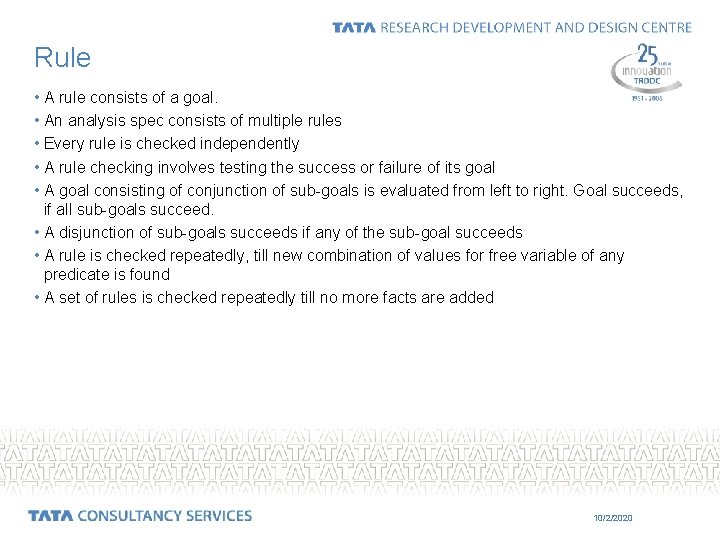
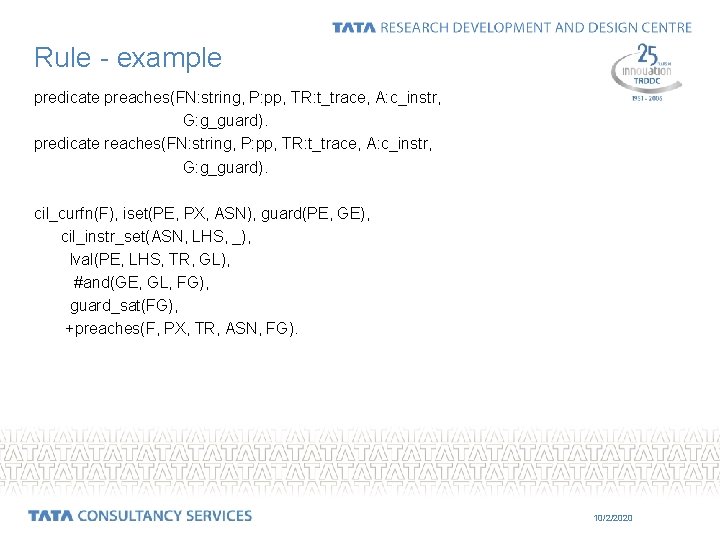
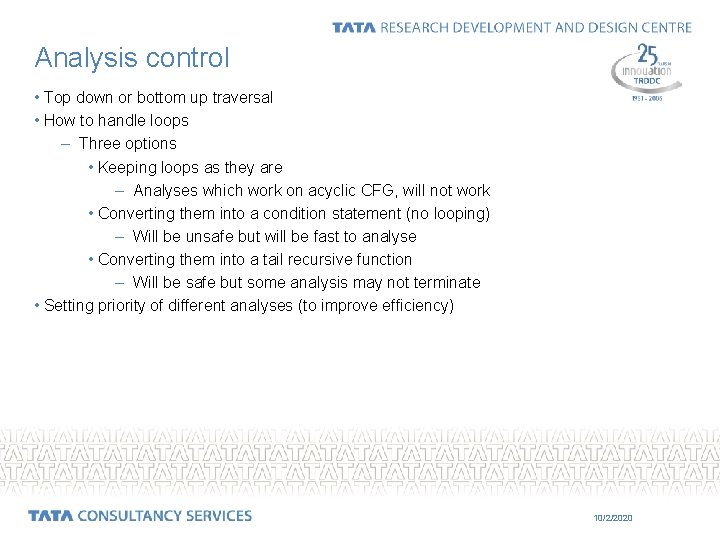
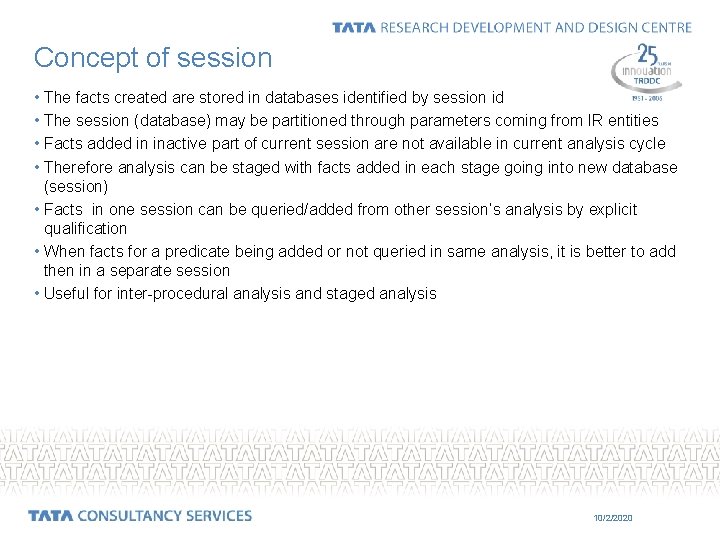
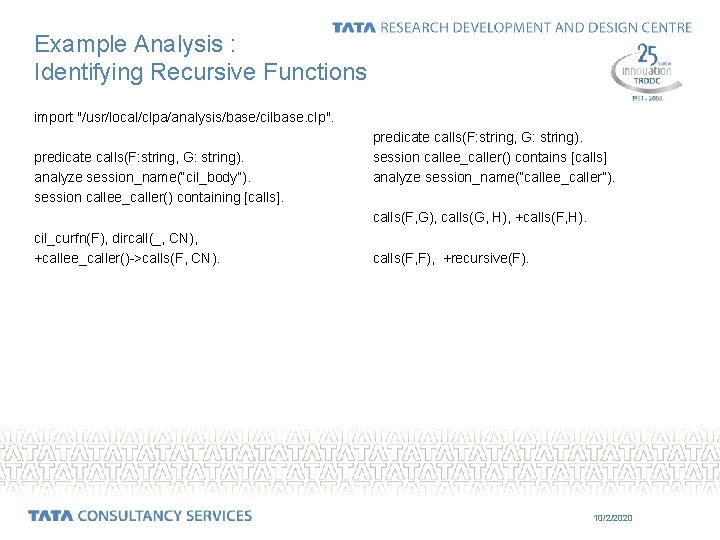
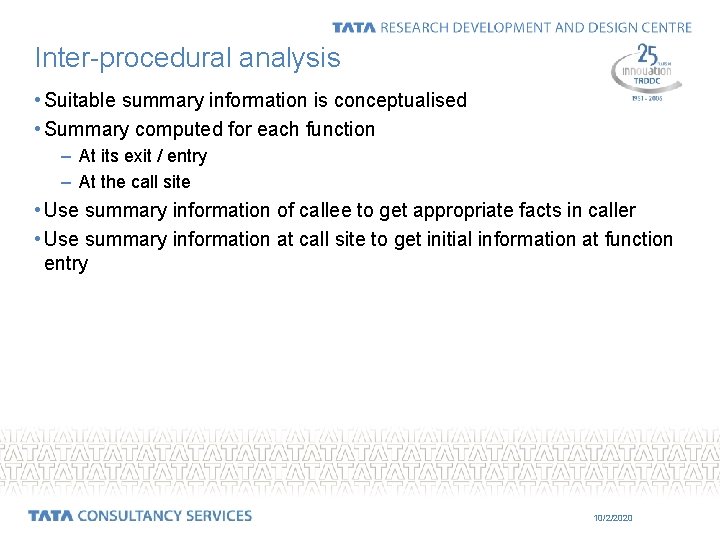
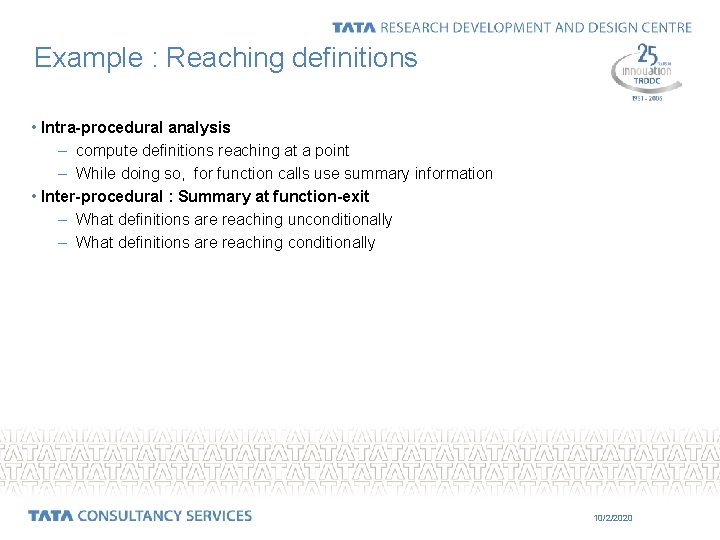
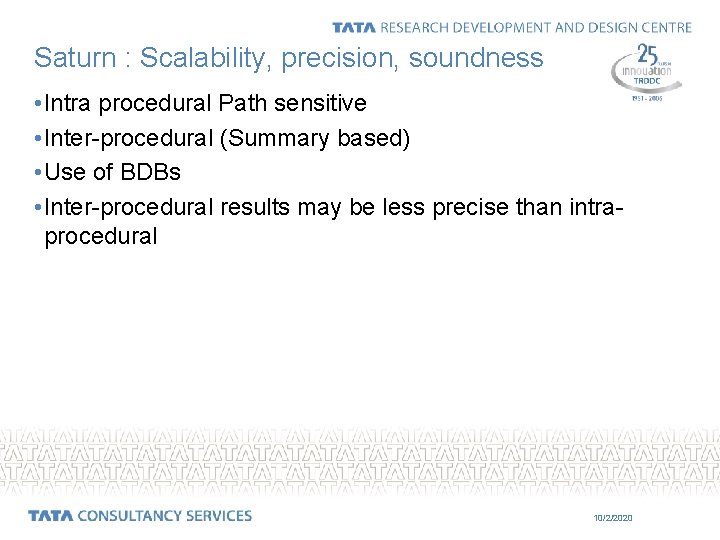
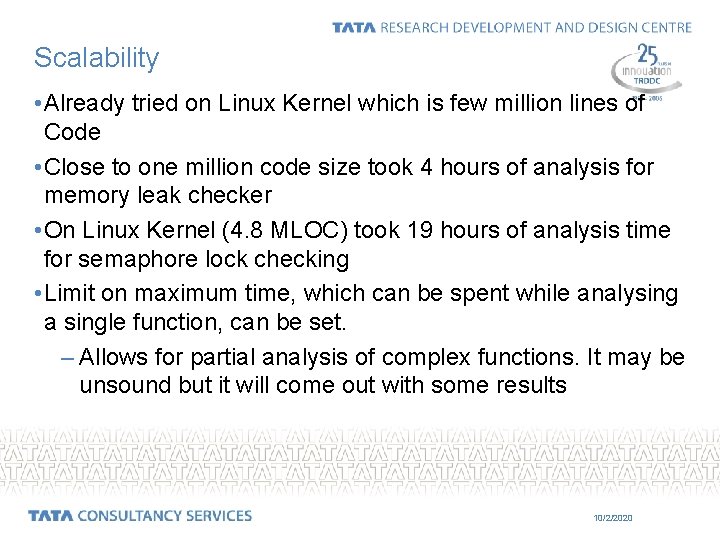
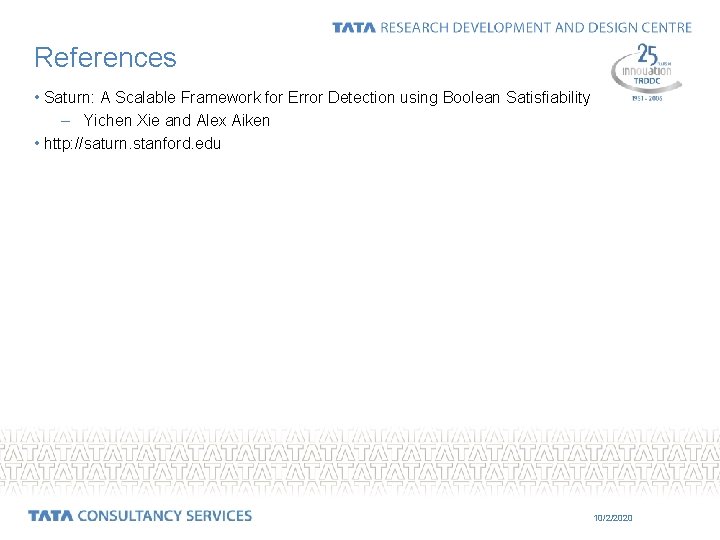
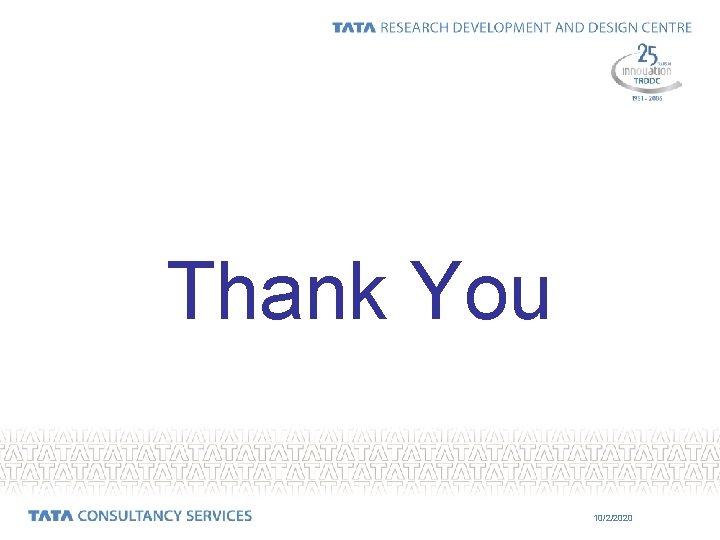
- Slides: 32
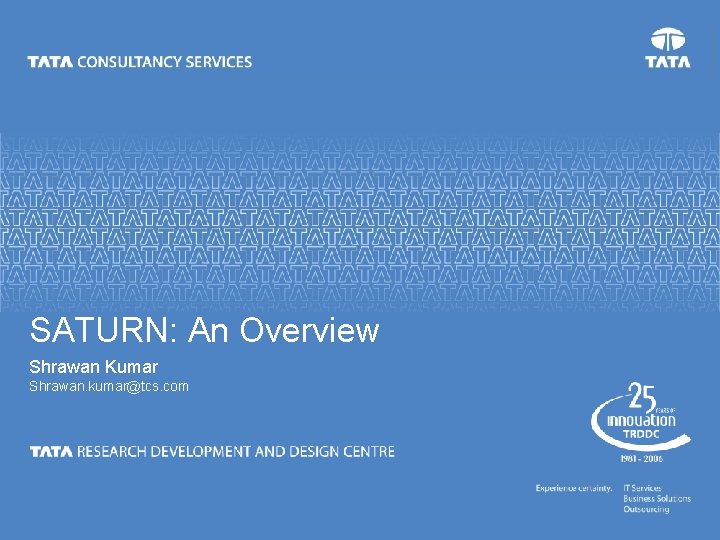
SATURN: An Overview Shrawan Kumar Shrawan. kumar@tcs. com
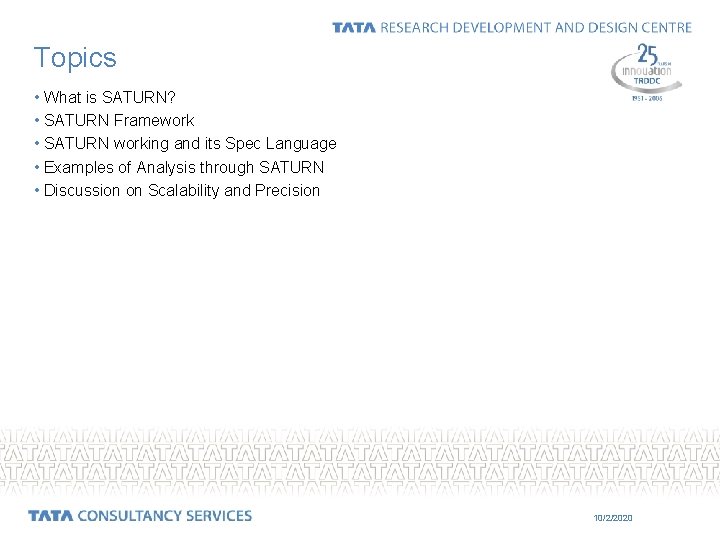
Topics • What is SATURN? • SATURN Framework • SATURN working and its Spec Language • Examples of Analysis through SATURN • Discussion on Scalability and Precision 10/2/2020
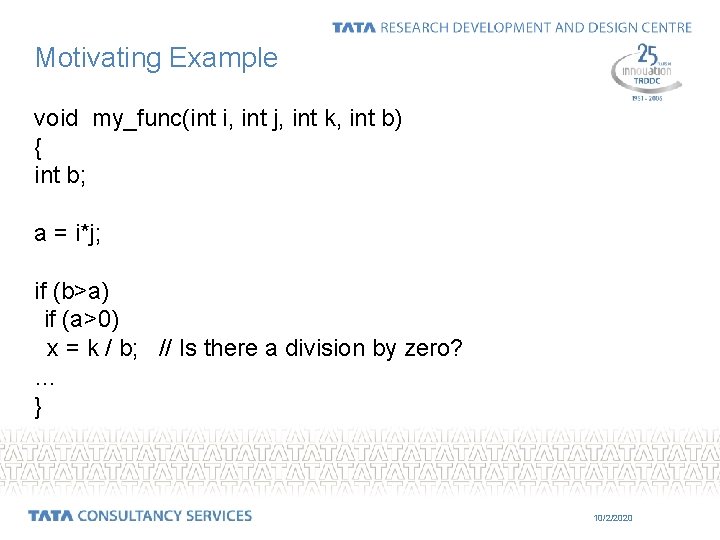
Motivating Example void my_func(int i, int j, int k, int b) { int b; a = i*j; if (b>a) if (a>0) x = k / b; // Is there a division by zero? … } 10/2/2020
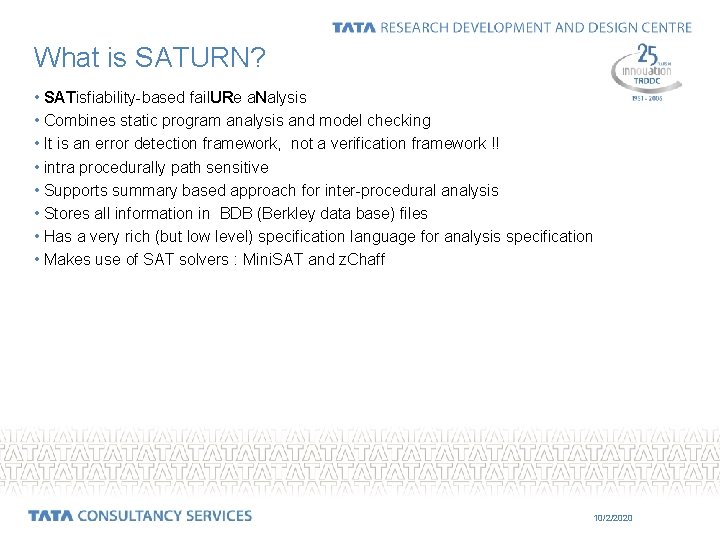
What is SATURN? • SATisfiability-based fail. URe a. Nalysis • Combines static program analysis and model checking • It is an error detection framework, not a verification framework !! • intra procedurally path sensitive • Supports summary based approach for inter-procedural analysis • Stores all information in BDB (Berkley data base) files • Has a very rich (but low level) specification language for analysis specification • Makes use of SAT solvers : Mini. SAT and z. Chaff 10/2/2020
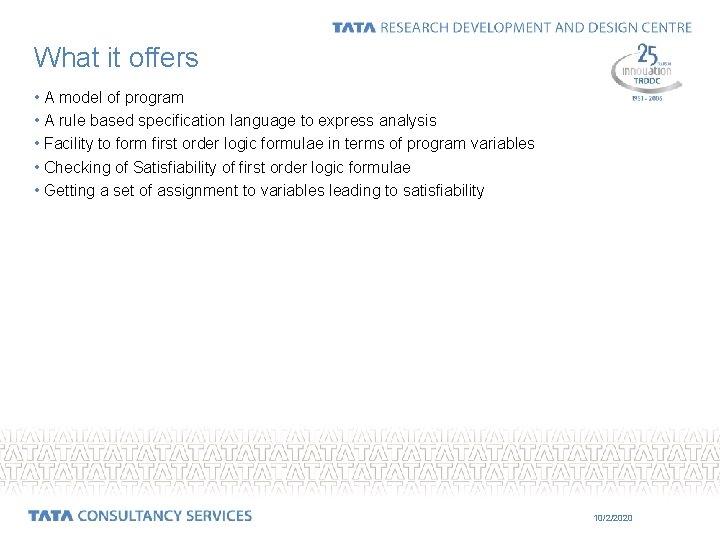
What it offers • A model of program • A rule based specification language to express analysis • Facility to form first order logic formulae in terms of program variables • Checking of Satisfiability of first order logic formulae • Getting a set of assignment to variables leading to satisfiability 10/2/2020
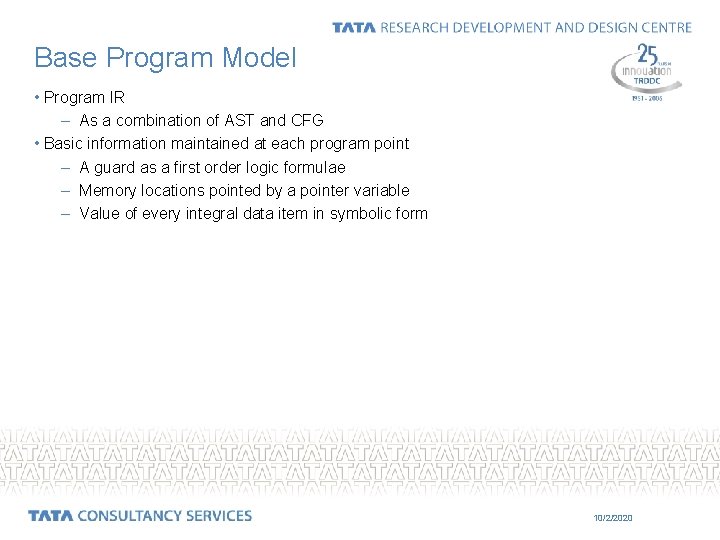
Base Program Model • Program IR – As a combination of AST and CFG • Basic information maintained at each program point – A guard as a first order logic formulae – Memory locations pointed by a pointer variable – Value of every integral data item in symbolic form 10/2/2020
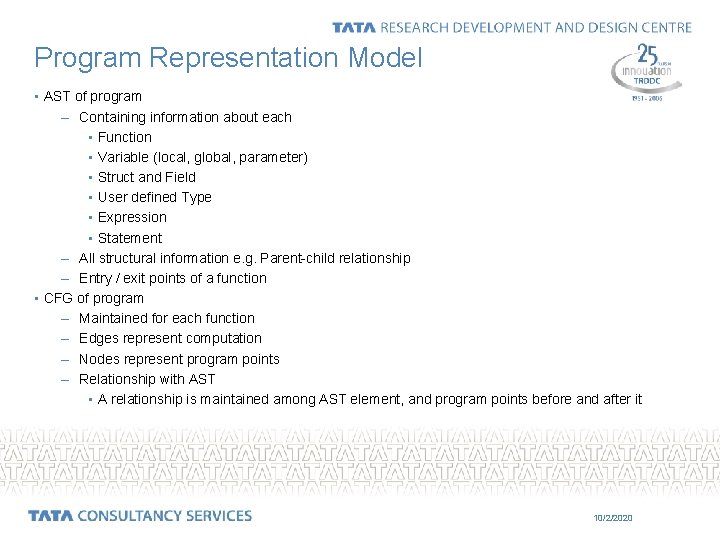
Program Representation Model • AST of program – Containing information about each • Function • Variable (local, global, parameter) • Struct and Field • User defined Type • Expression • Statement – All structural information e. g. Parent-child relationship – Entry / exit points of a function • CFG of program – Maintained for each function – Edges represent computation – Nodes represent program points – Relationship with AST • A relationship is maintained among AST element, and program points before and after it 10/2/2020
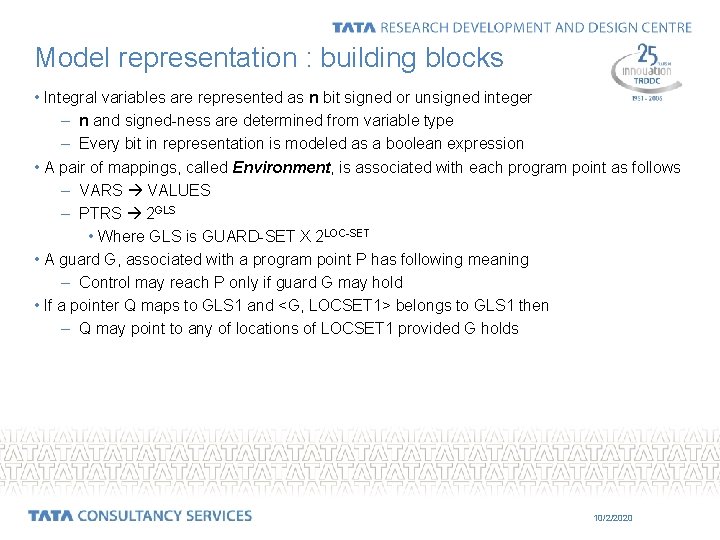
Model representation : building blocks • Integral variables are represented as n bit signed or unsigned integer – n and signed-ness are determined from variable type – Every bit in representation is modeled as a boolean expression • A pair of mappings, called Environment, is associated with each program point as follows – VARS VALUES – PTRS 2 GLS • Where GLS is GUARD-SET X 2 LOC-SET • A guard G, associated with a program point P has following meaning – Control may reach P only if guard G may hold • If a pointer Q maps to GLS 1 and <G, LOCSET 1> belongs to GLS 1 then – Q may point to any of locations of LOCSET 1 provided G holds 10/2/2020
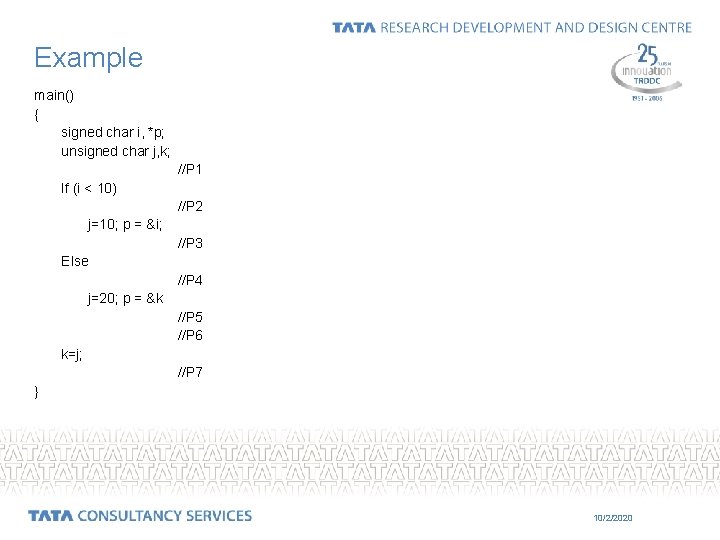
Example main() { signed char i, *p; unsigned char j, k; //P 1 If (i < 10) //P 2 j=10; p = &i; //P 3 Else //P 4 j=20; p = &k //P 5 //P 6 k=j; //P 7 } 10/2/2020
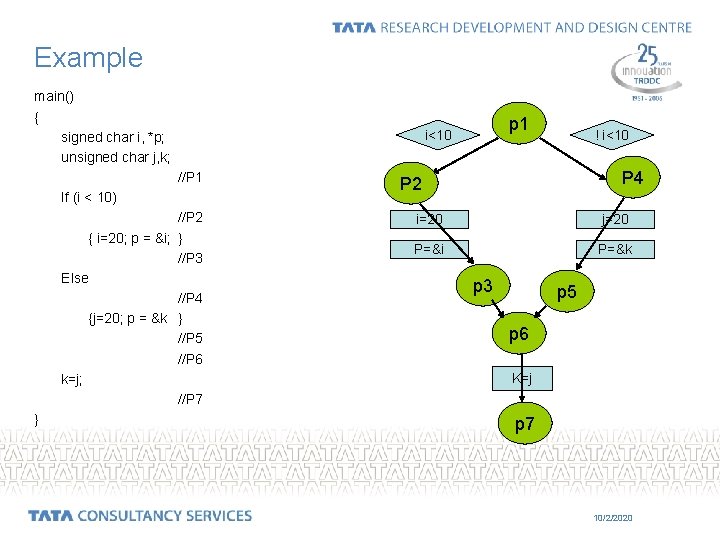
Example main() { signed char i, *p; unsigned char j, k; //P 1 If (i < 10) //P 2 { i=20; p = &i; } //P 3 Else //P 4 {j=20; p = &k } //P 5 //P 6 k=j; //P 7 } p 1 i<10 ! i<10 P 4 P 2 i=20 j=20 P=&i P=&k p 3 p 5 p 6 K=j p 7 10/2/2020
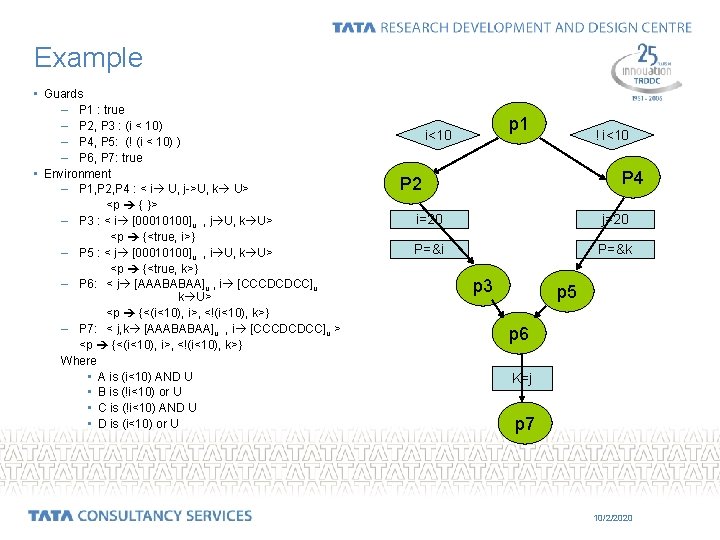
Example • Guards – P 1 : true – P 2, P 3 : (i < 10) – P 4, P 5: (! (i < 10) ) – P 6, P 7: true • Environment – P 1, P 2, P 4 : < i U, j->U, k U> <p { }> – P 3 : < i [00010100]u , j U, k U> <p {<true, i>} – P 5 : < j [00010100]u , i U, k U> <p {<true, k>} – P 6: < j [AAABABAA]u , i [CCCDCDCC]u k U> <p {<(i<10), i>, <!(i<10), k>} – P 7: < j, k [AAABABAA]u , i [CCCDCDCC]u > <p {<(i<10), i>, <!(i<10), k>} Where • A is (i<10) AND U • B is (!i<10) or U • C is (!i<10) AND U • D is (i<10) or U p 1 i<10 ! i<10 P 4 P 2 i=20 j=20 P=&i P=&k p 3 p 5 p 6 K=j p 7 10/2/2020
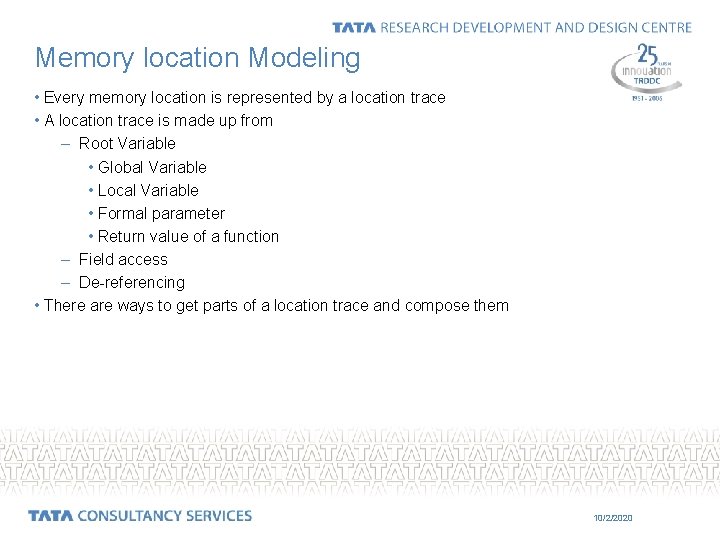
Memory location Modeling • Every memory location is represented by a location trace • A location trace is made up from – Root Variable • Global Variable • Local Variable • Formal parameter • Return value of a function – Field access – De-referencing • There are ways to get parts of a location trace and compose them 10/2/2020
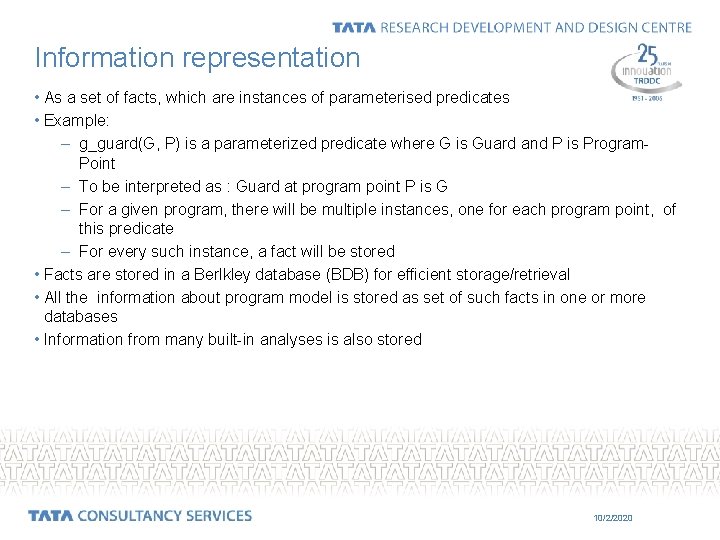
Information representation • As a set of facts, which are instances of parameterised predicates • Example: – g_guard(G, P) is a parameterized predicate where G is Guard and P is Program. Point – To be interpreted as : Guard at program point P is G – For a given program, there will be multiple instances, one for each program point, of this predicate – For every such instance, a fact will be stored • Facts are stored in a Berlkley database (BDB) for efficient storage/retrieval • All the information about program model is stored as set of such facts in one or more databases • Information from many built-in analyses is also stored 10/2/2020
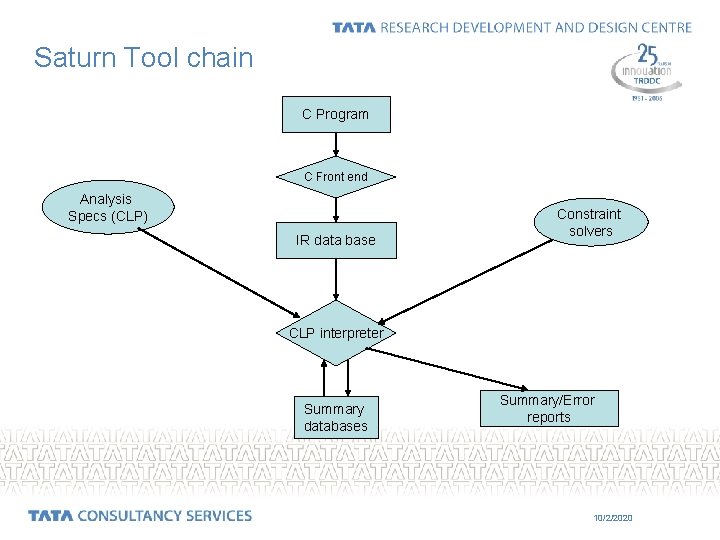
Saturn Tool chain C Program C Front end Analysis Specs (CLP) IR data base Constraint solvers CLP interpreter Summary databases Summary/Error reports 10/2/2020
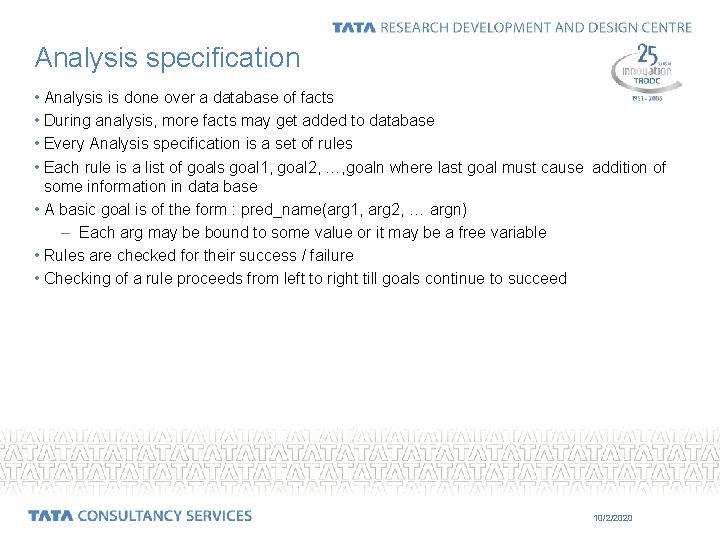
Analysis specification • Analysis is done over a database of facts • During analysis, more facts may get added to database • Every Analysis specification is a set of rules • Each rule is a list of goals goal 1, goal 2, …, goaln where last goal must cause addition of some information in data base • A basic goal is of the form : pred_name(arg 1, arg 2, … argn) – Each arg may be bound to some value or it may be a free variable • Rules are checked for their success / failure • Checking of a rule proceeds from left to right till goals continue to succeed 10/2/2020
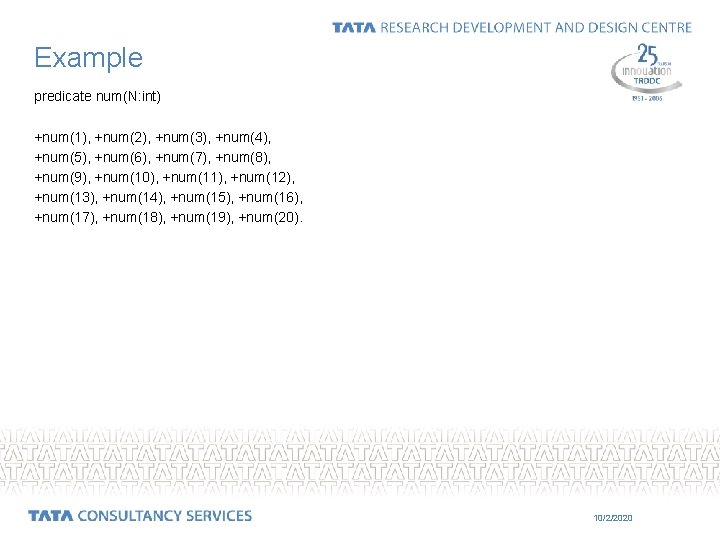
Example predicate num(N: int) +num(1), +num(2), +num(3), +num(4), +num(5), +num(6), +num(7), +num(8), +num(9), +num(10), +num(11), +num(12), +num(13), +num(14), +num(15), +num(16), +num(17), +num(18), +num(19), +num(20). 10/2/2020
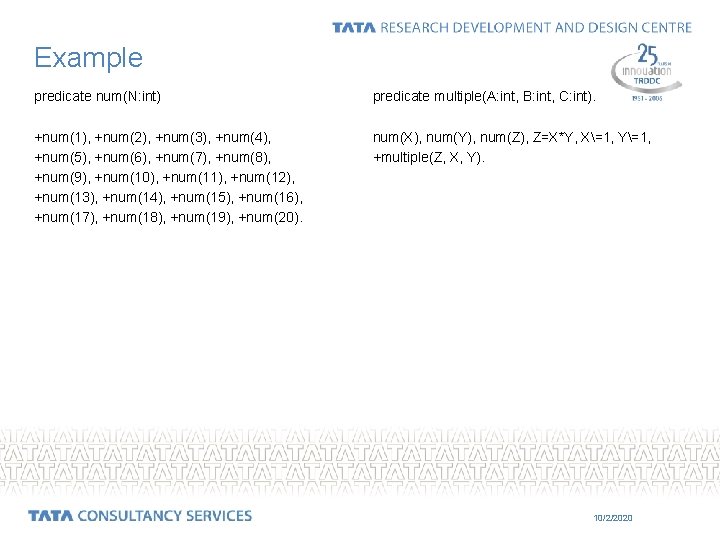
Example predicate num(N: int) predicate multiple(A: int, B: int, C: int). +num(1), +num(2), +num(3), +num(4), +num(5), +num(6), +num(7), +num(8), +num(9), +num(10), +num(11), +num(12), +num(13), +num(14), +num(15), +num(16), +num(17), +num(18), +num(19), +num(20). num(X), num(Y), num(Z), Z=X*Y, X=1, Y=1, +multiple(Z, X, Y). 10/2/2020
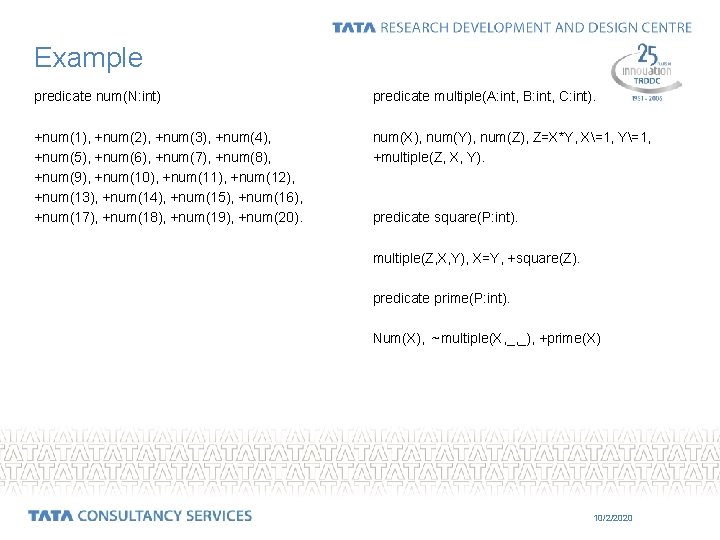
Example predicate num(N: int) predicate multiple(A: int, B: int, C: int). +num(1), +num(2), +num(3), +num(4), +num(5), +num(6), +num(7), +num(8), +num(9), +num(10), +num(11), +num(12), +num(13), +num(14), +num(15), +num(16), +num(17), +num(18), +num(19), +num(20). num(X), num(Y), num(Z), Z=X*Y, X=1, Y=1, +multiple(Z, X, Y). predicate square(P: int). multiple(Z, X, Y), X=Y, +square(Z). predicate prime(P: int). Num(X), ~multiple(X, _, _), +prime(X) 10/2/2020
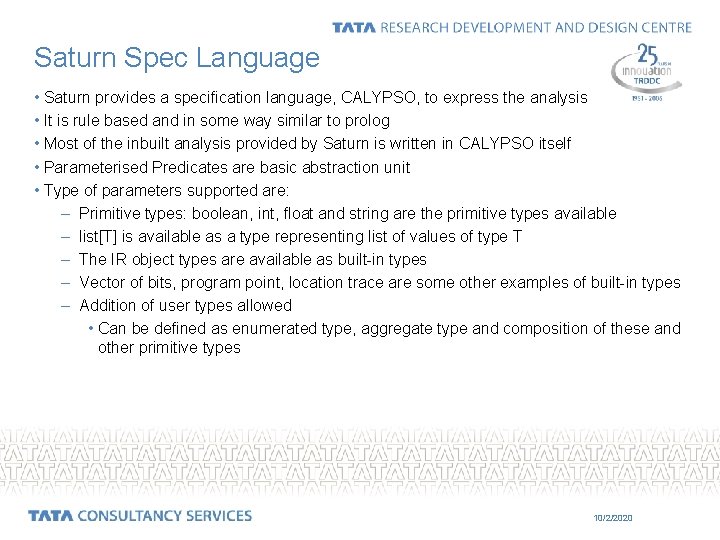
Saturn Spec Language • Saturn provides a specification language, CALYPSO, to express the analysis • It is rule based and in some way similar to prolog • Most of the inbuilt analysis provided by Saturn is written in CALYPSO itself • Parameterised Predicates are basic abstraction unit • Type of parameters supported are: – Primitive types: boolean, int, float and string are the primitive types available – list[T] is available as a type representing list of values of type T – The IR object types are available as built-in types – Vector of bits, program point, location trace are some other examples of built-in types – Addition of user types allowed • Can be defined as enumerated type, aggregate type and composition of these and other primitive types 10/2/2020
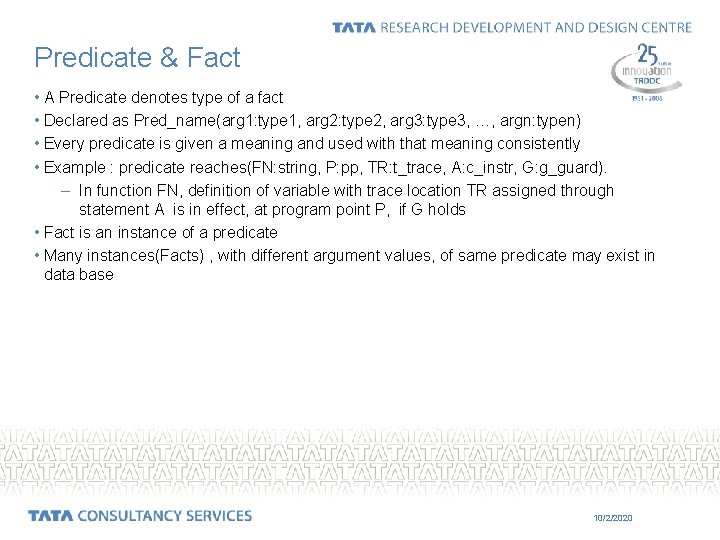
Predicate & Fact • A Predicate denotes type of a fact • Declared as Pred_name(arg 1: type 1, arg 2: type 2, arg 3: type 3, …, argn: typen) • Every predicate is given a meaning and used with that meaning consistently • Example : predicate reaches(FN: string, P: pp, TR: t_trace, A: c_instr, G: g_guard). – In function FN, definition of variable with trace location TR assigned through statement A is in effect, at program point P, if G holds • Fact is an instance of a predicate • Many instances(Facts) , with different argument values, of same predicate may exist in data base 10/2/2020
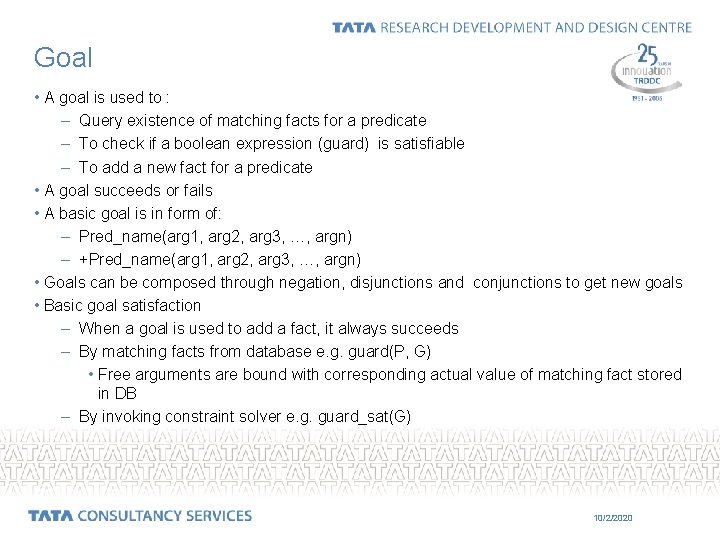
Goal • A goal is used to : – Query existence of matching facts for a predicate – To check if a boolean expression (guard) is satisfiable – To add a new fact for a predicate • A goal succeeds or fails • A basic goal is in form of: – Pred_name(arg 1, arg 2, arg 3, …, argn) – +Pred_name(arg 1, arg 2, arg 3, …, argn) • Goals can be composed through negation, disjunctions and conjunctions to get new goals • Basic goal satisfaction – When a goal is used to add a fact, it always succeeds – By matching facts from database e. g. guard(P, G) • Free arguments are bound with corresponding actual value of matching fact stored in DB – By invoking constraint solver e. g. guard_sat(G) 10/2/2020
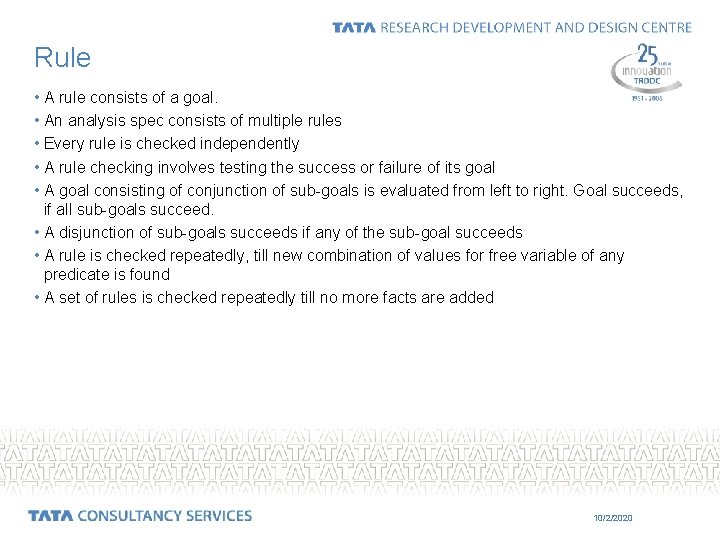
Rule • A rule consists of a goal. • An analysis spec consists of multiple rules • Every rule is checked independently • A rule checking involves testing the success or failure of its goal • A goal consisting of conjunction of sub-goals is evaluated from left to right. Goal succeeds, if all sub-goals succeed. • A disjunction of sub-goals succeeds if any of the sub-goal succeeds • A rule is checked repeatedly, till new combination of values for free variable of any predicate is found • A set of rules is checked repeatedly till no more facts are added 10/2/2020
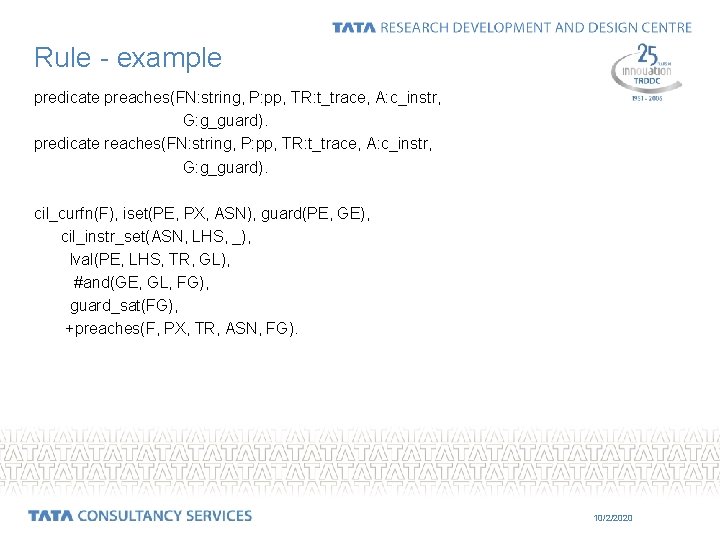
Rule - example predicate preaches(FN: string, P: pp, TR: t_trace, A: c_instr, G: g_guard). predicate reaches(FN: string, P: pp, TR: t_trace, A: c_instr, G: g_guard). cil_curfn(F), iset(PE, PX, ASN), guard(PE, GE), cil_instr_set(ASN, LHS, _), lval(PE, LHS, TR, GL), #and(GE, GL, FG), guard_sat(FG), +preaches(F, PX, TR, ASN, FG). 10/2/2020
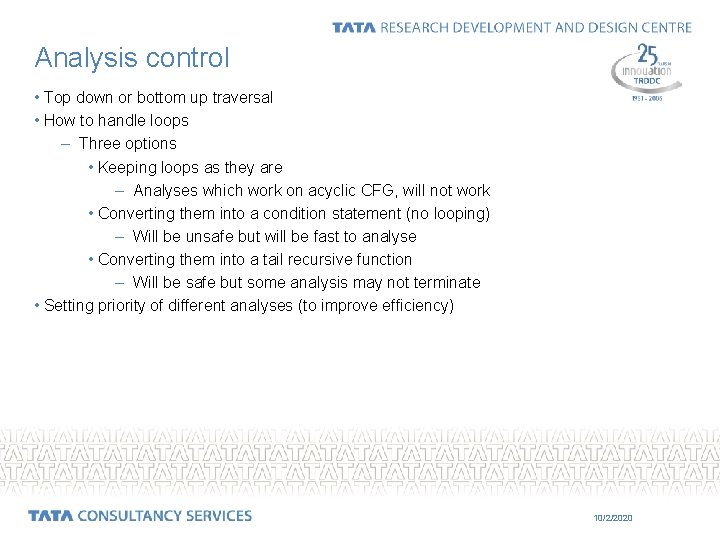
Analysis control • Top down or bottom up traversal • How to handle loops – Three options • Keeping loops as they are – Analyses which work on acyclic CFG, will not work • Converting them into a condition statement (no looping) – Will be unsafe but will be fast to analyse • Converting them into a tail recursive function – Will be safe but some analysis may not terminate • Setting priority of different analyses (to improve efficiency) 10/2/2020
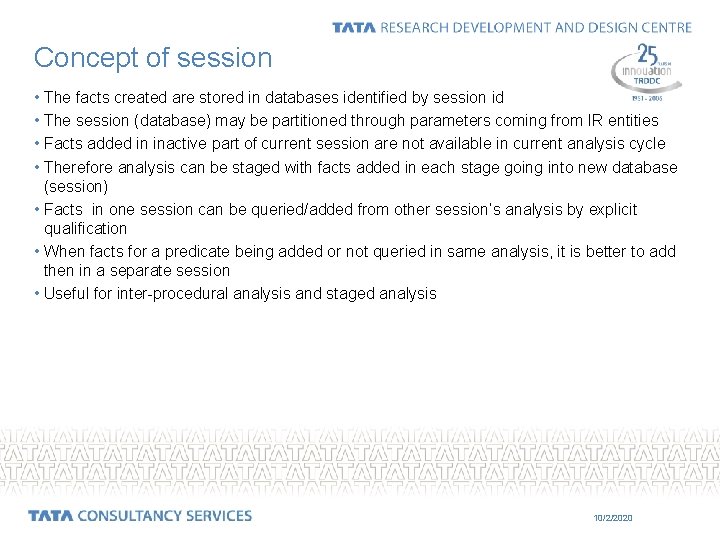
Concept of session • The facts created are stored in databases identified by session id • The session (database) may be partitioned through parameters coming from IR entities • Facts added in inactive part of current session are not available in current analysis cycle • Therefore analysis can be staged with facts added in each stage going into new database (session) • Facts in one session can be queried/added from other session’s analysis by explicit qualification • When facts for a predicate being added or not queried in same analysis, it is better to add then in a separate session • Useful for inter-procedural analysis and staged analysis 10/2/2020
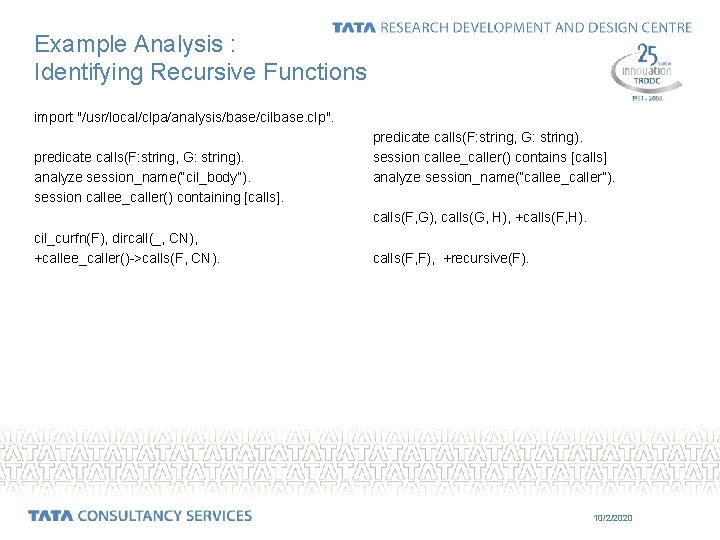
Example Analysis : Identifying Recursive Functions import "/usr/local/clpa/analysis/base/cilbase. clp". predicate calls(F: string, G: string). analyze session_name(“cil_body”). session callee_caller() containing [calls]. predicate calls(F: string, G: string). session callee_caller() contains [calls] analyze session_name(“callee_caller”). calls(F, G), calls(G, H), +calls(F, H). cil_curfn(F), dircall(_, CN), +callee_caller()->calls(F, CN). calls(F, F), +recursive(F). 10/2/2020
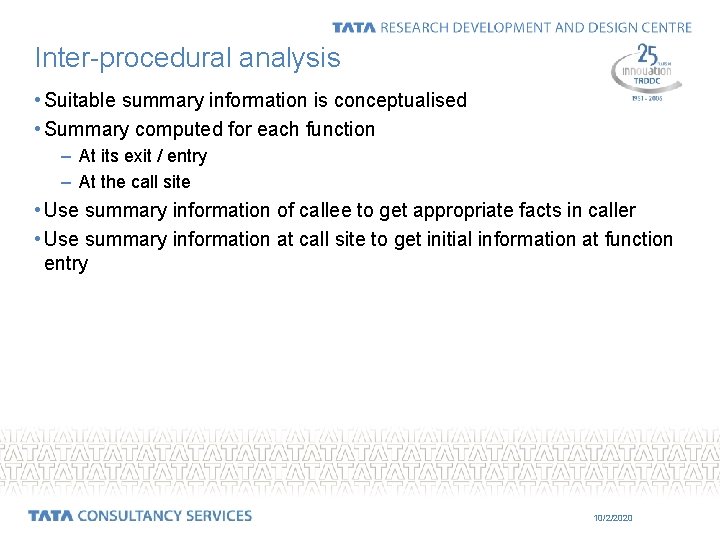
Inter-procedural analysis • Suitable summary information is conceptualised • Summary computed for each function – At its exit / entry – At the call site • Use summary information of callee to get appropriate facts in caller • Use summary information at call site to get initial information at function entry 10/2/2020
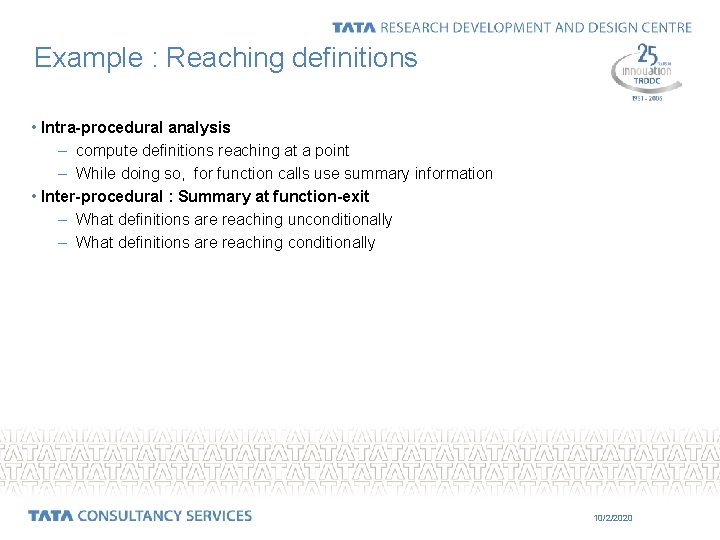
Example : Reaching definitions • Intra-procedural analysis – compute definitions reaching at a point – While doing so, for function calls use summary information • Inter-procedural : Summary at function-exit – What definitions are reaching unconditionally – What definitions are reaching conditionally 10/2/2020
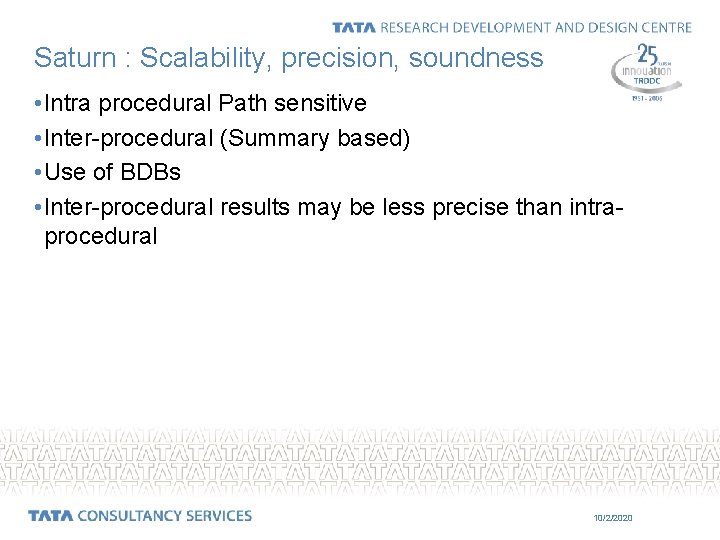
Saturn : Scalability, precision, soundness • Intra procedural Path sensitive • Inter-procedural (Summary based) • Use of BDBs • Inter-procedural results may be less precise than intraprocedural 10/2/2020
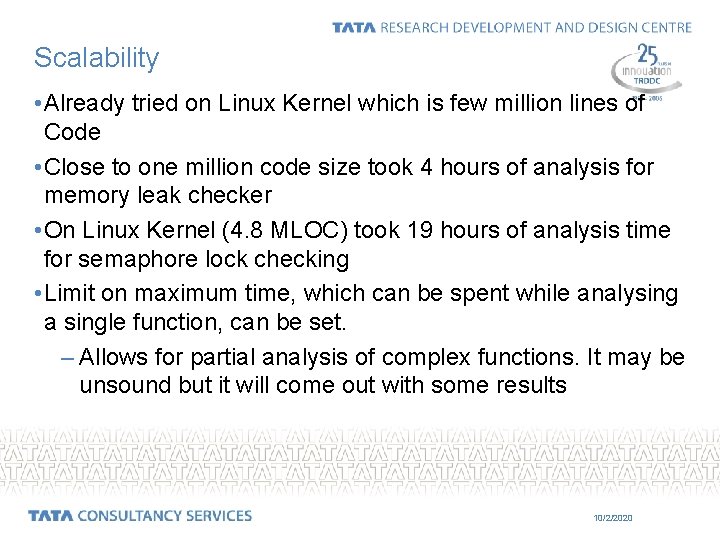
Scalability • Already tried on Linux Kernel which is few million lines of Code • Close to one million code size took 4 hours of analysis for memory leak checker • On Linux Kernel (4. 8 MLOC) took 19 hours of analysis time for semaphore lock checking • Limit on maximum time, which can be spent while analysing a single function, can be set. – Allows for partial analysis of complex functions. It may be unsound but it will come out with some results 10/2/2020
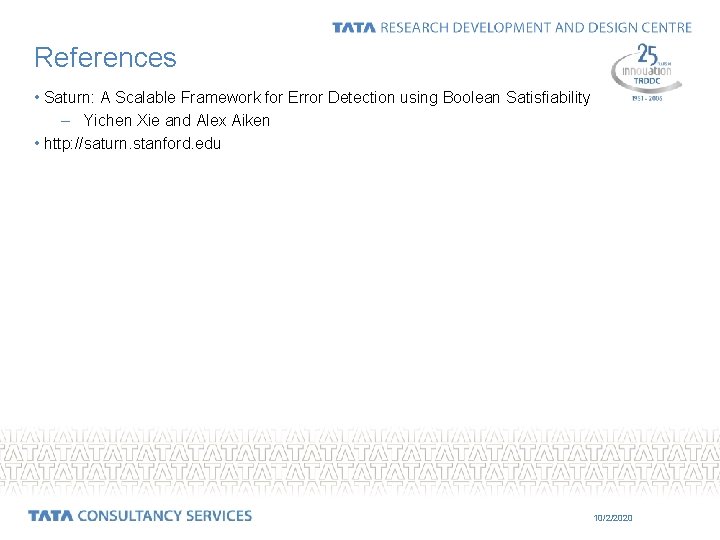
References • Saturn: A Scalable Framework for Error Detection using Boolean Satisfiability – Yichen Xie and Alex Aiken • http: //saturn. stanford. edu 10/2/2020
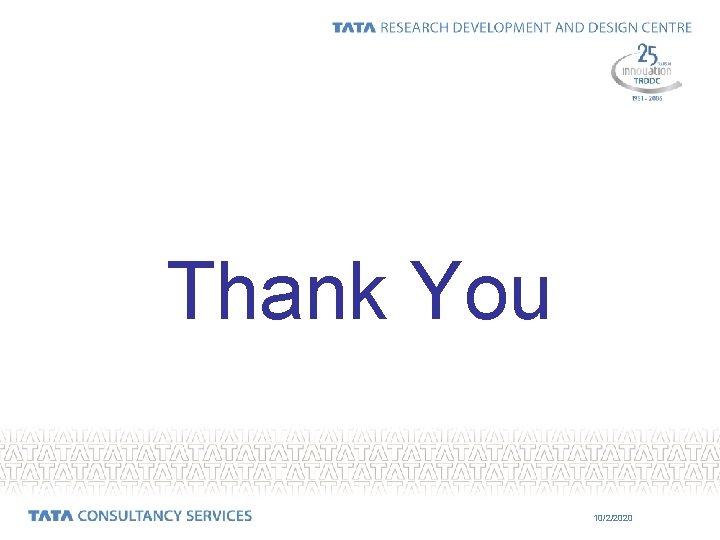
Thank You 10/2/2020
Saturn
Dậy thổi cơm mua thịt cá
Cơm
Saturn 4 billion years ago
Jupiter outline
The solar system consists of
What are characteristics of saturn
Lot saturn
Jupiter is noticeably oblate because
Solar saturn overhaul
: l
Mars, jupiter, and saturn show retrograde motion because
Saturn zeus
Saturn is noticeably oblate because
Outer planets
What is the 2nd smallest planet in our solar system
Neptun saturn
Saturn prezentacja
Saturn v wiki
Saturn facts
Stonehenge planetary orbits
Fun facts about saturn
Saturn x mc
Saturn presentation
Why does saturn have rings
Saturn prezentace
Bůh saturn
Write about solar system
Uranus distance from sun
Outer planets
Mercury planet features
Zweitgrößter planet
Saturn geo