Optimization of the United States Postal Retail Network
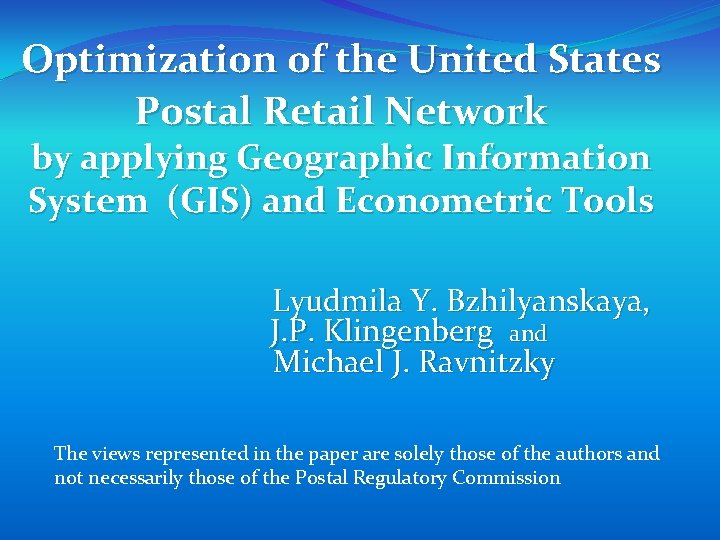
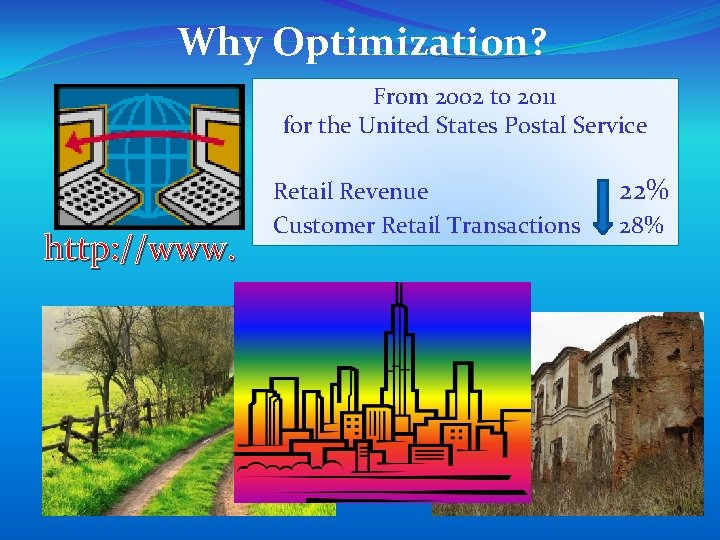
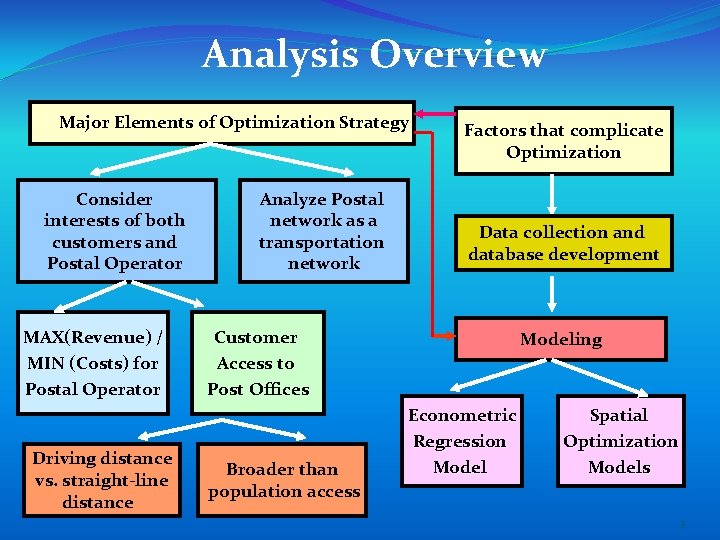
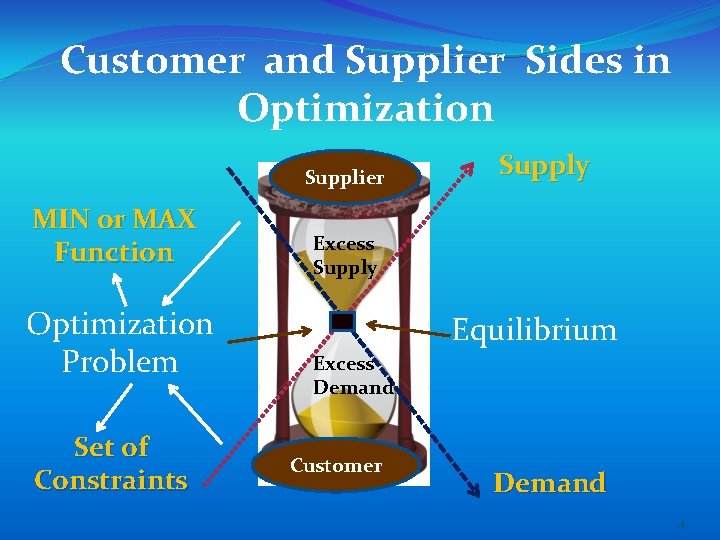
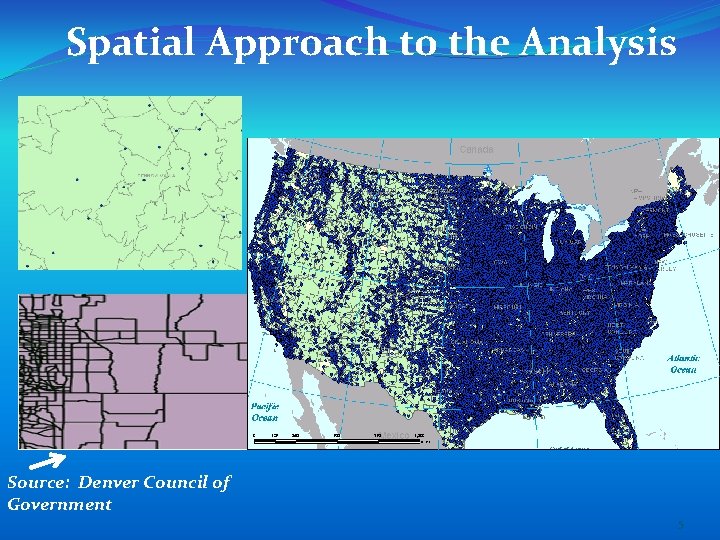
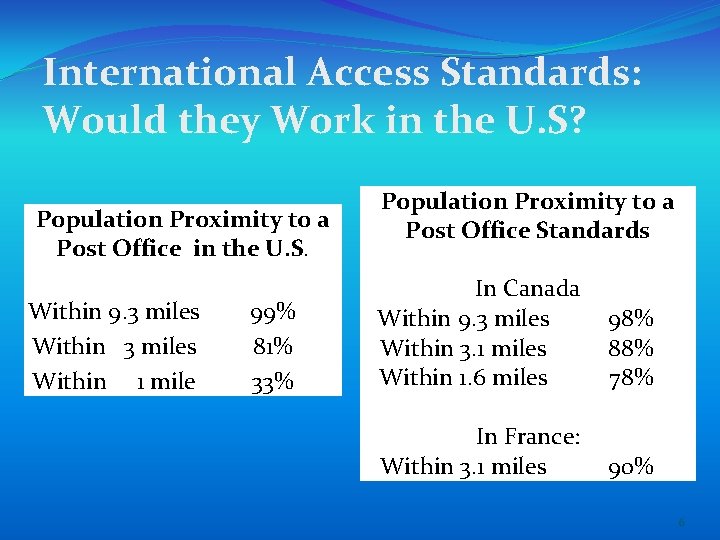
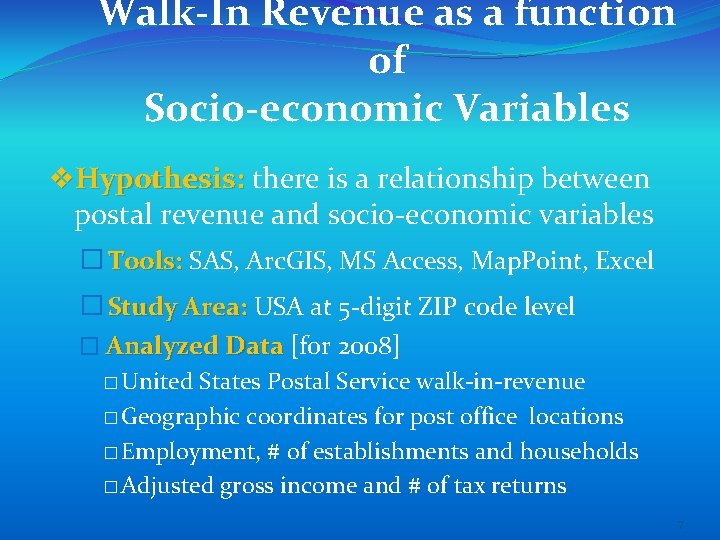
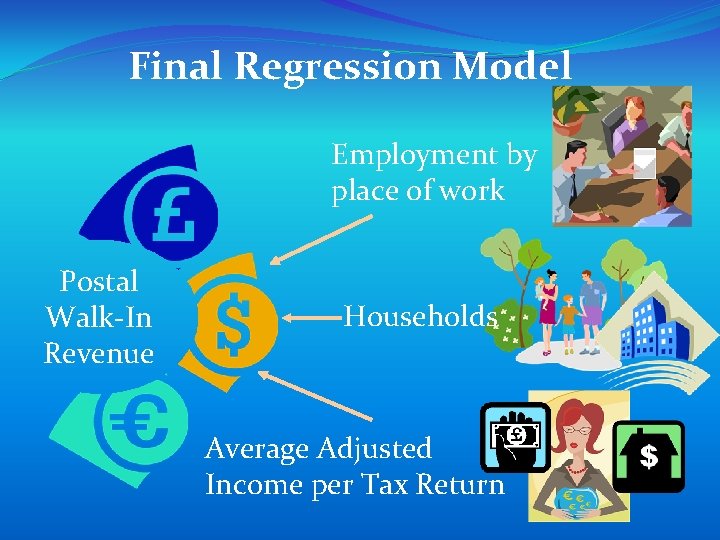
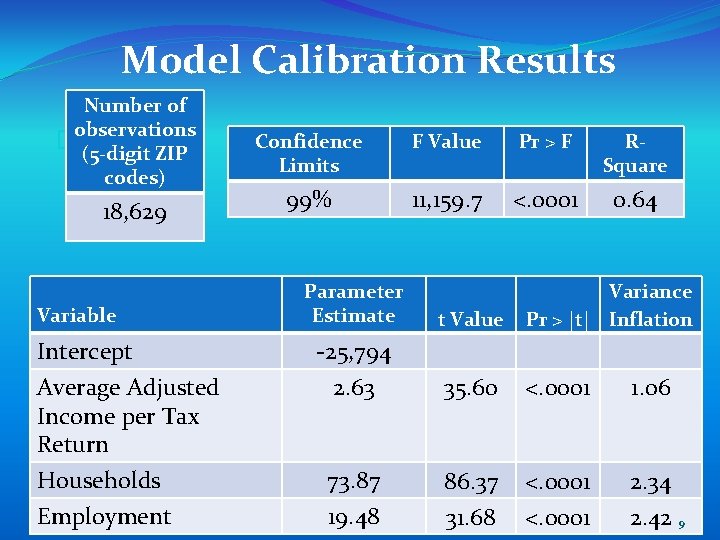
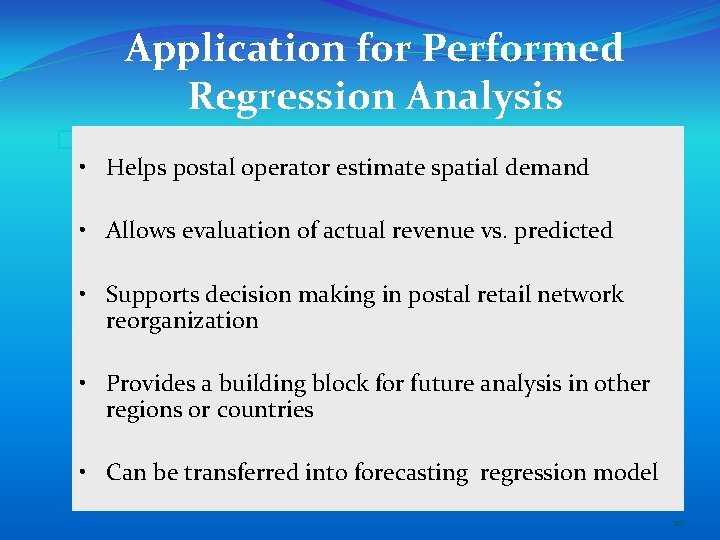
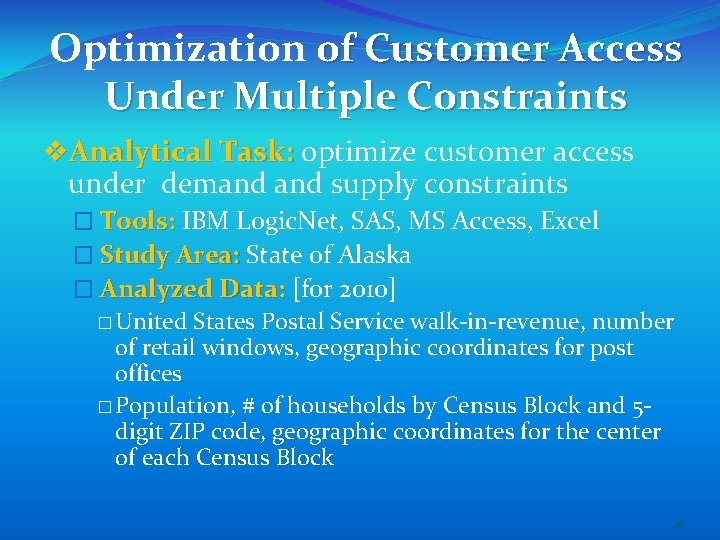
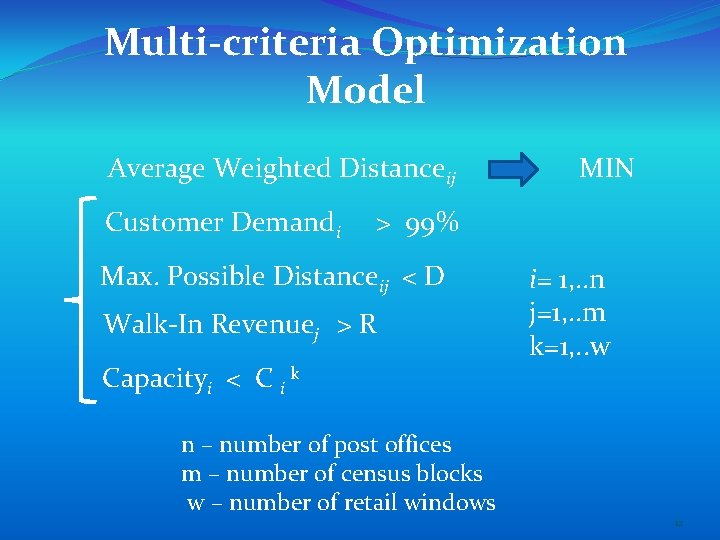
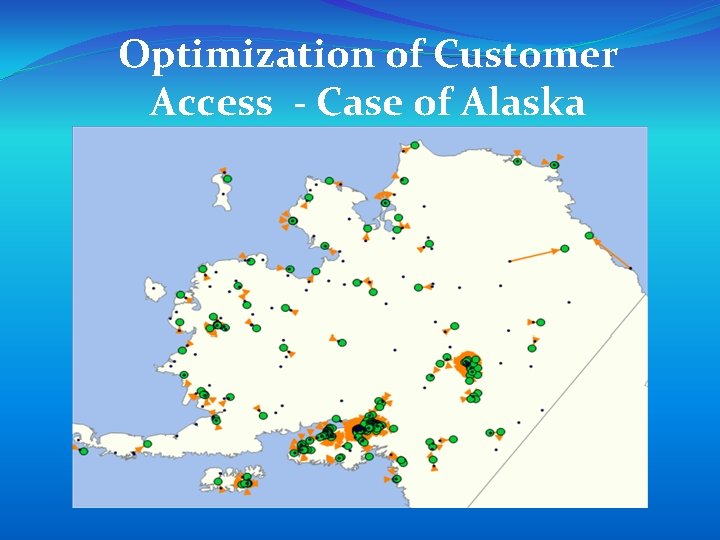
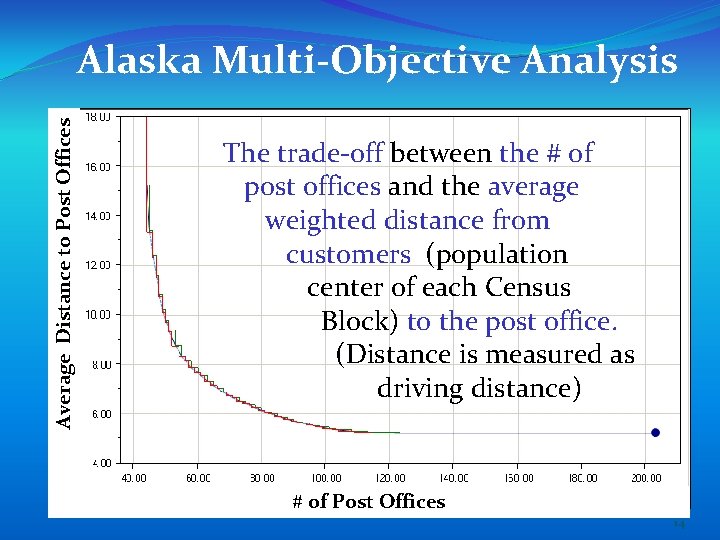
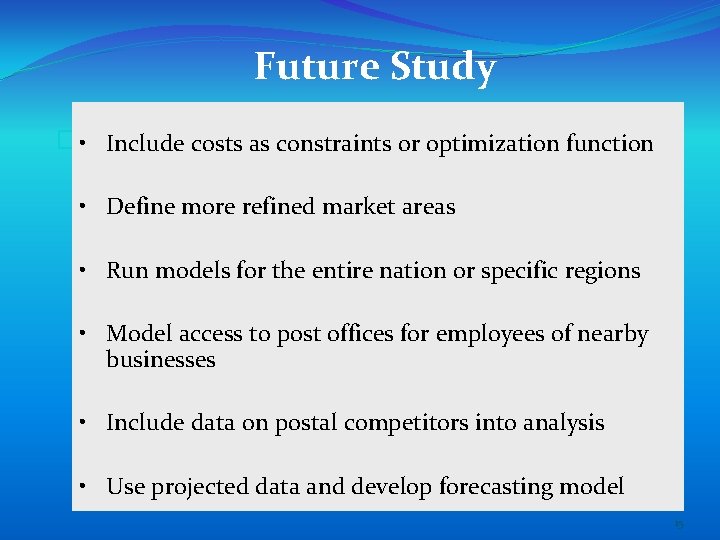
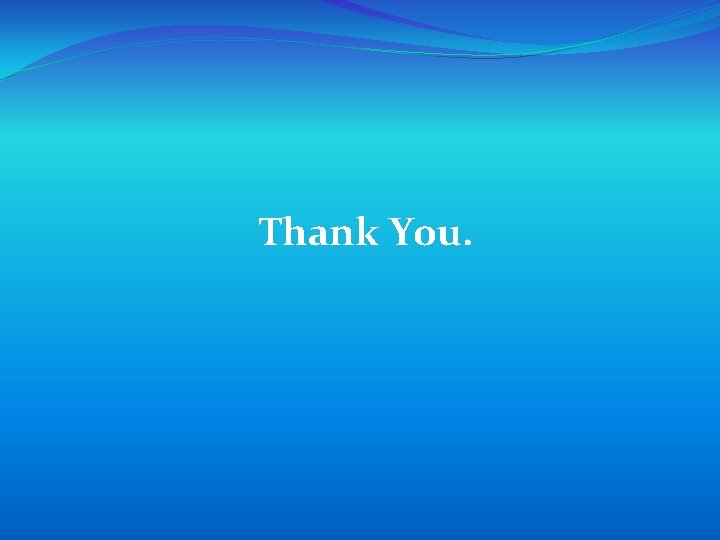
- Slides: 16
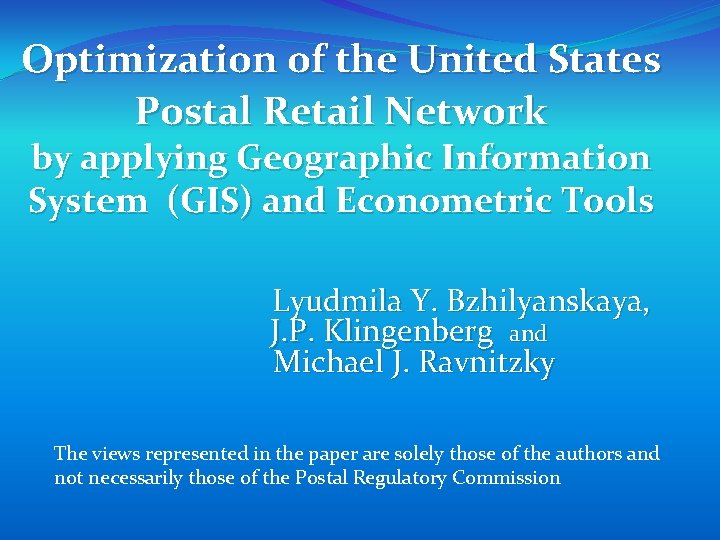
Optimization of the United States Postal Retail Network by applying Geographic Information System (GIS) and Econometric Tools Lyudmila Y. Bzhilyanskaya, J. P. Klingenberg and Michael J. Ravnitzky The views represented in the paper are solely those of the authors and not necessarily those of the Postal Regulatory Commission
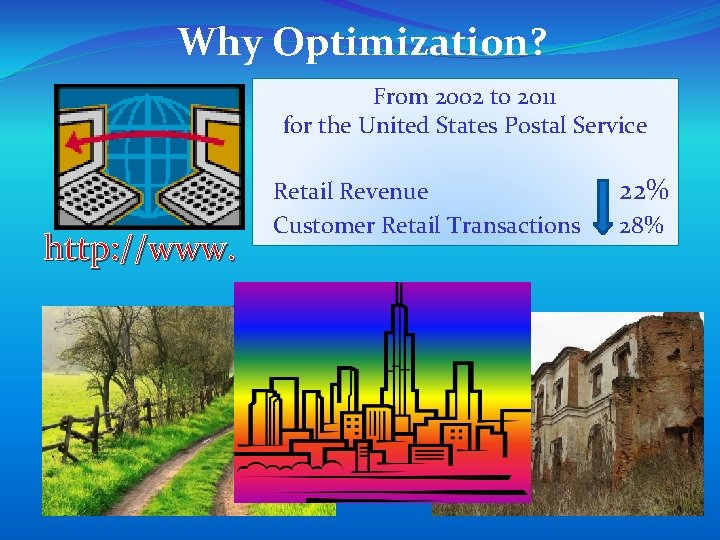
Why Optimization? From 2002 to 2011 for the United States Postal Service http: //www. Retail Revenue Customer Retail Transactions 22% 28%
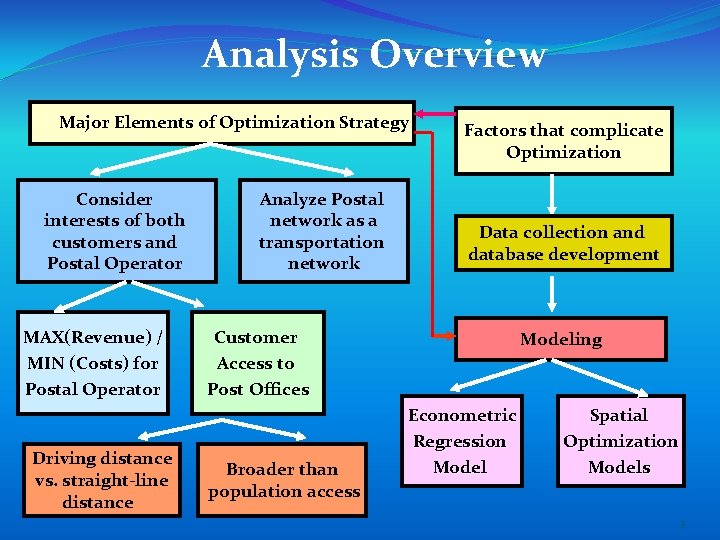
Analysis Overview Major Elements of Optimization Strategy Consider interests of both customers and Postal Operator MAX(Revenue) / MIN (Costs) for Postal Operator Driving distance vs. straight-line distance Analyze Postal network as a transportation network Factors that complicate Optimization Data collection and database development Customer Access to Post Offices Broader than population access Modeling Econometric Regression Model Spatial Optimization Models 3
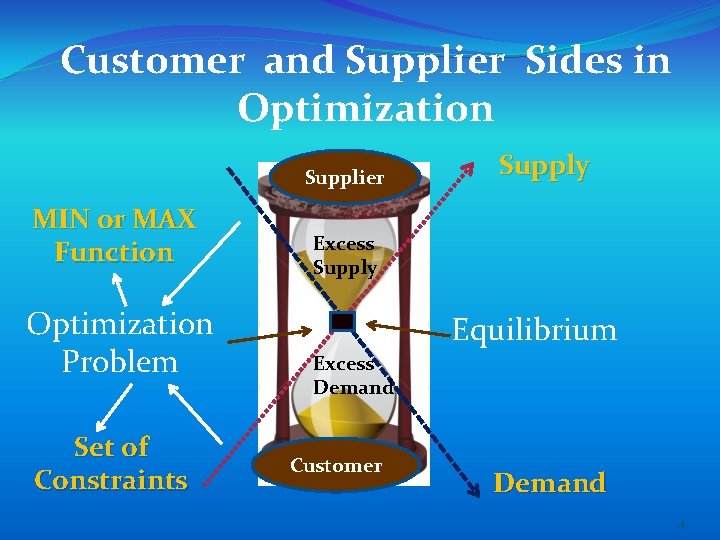
Customer and Supplier Sides in Optimization Supplier MIN or MAX Function Optimization Problem Set of Constraints Supply Excess Supply Equilibrium Excess Demand Customer Demand 4
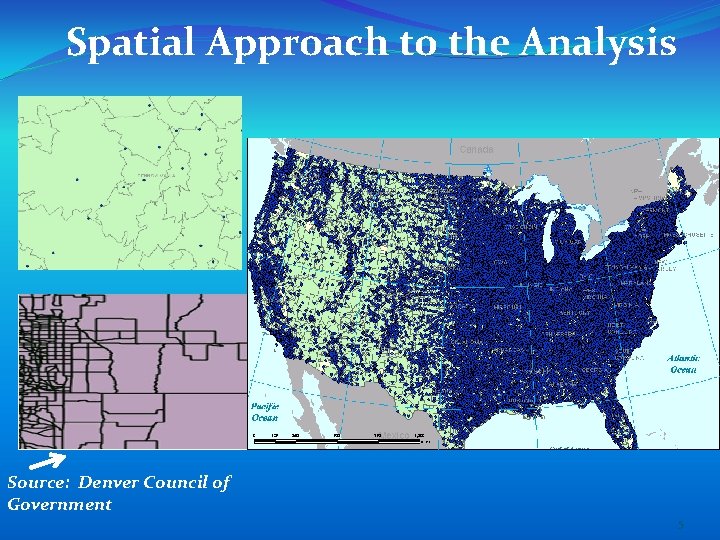
Spatial Approach to the Analysis Source: Denver Council of Government 5
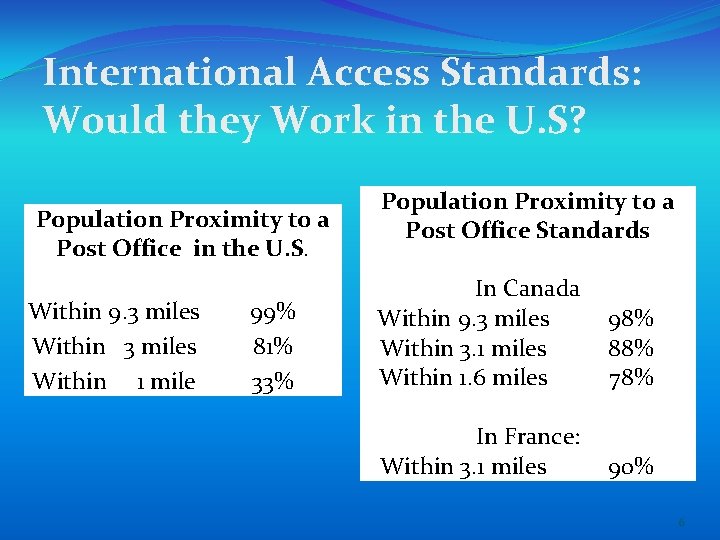
International Access Standards: Would they Work in the U. S? Population Proximity to a Post Office in the U. S. Within 9. 3 miles Within 1 mile 99% 81% 33% Population Proximity to a Post Office Standards In Canada Within 9. 3 miles Within 3. 1 miles Within 1. 6 miles 98% 88% 78% In France: Within 3. 1 miles 90% 6
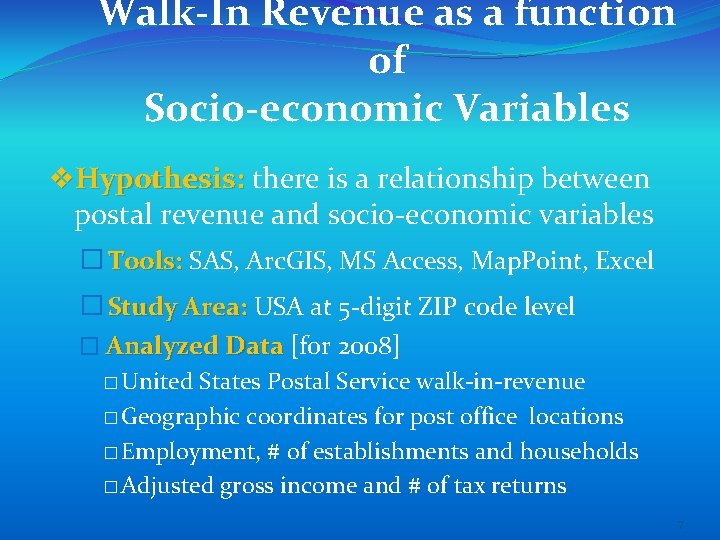
Walk-In Revenue as a function of Socio-economic Variables v. Hypothesis: there is a relationship between postal revenue and socio-economic variables � Tools: SAS, Arc. GIS, MS Access, Map. Point, Excel � Study Area: USA at 5 -digit ZIP code level � Analyzed Data [for 2008] � United States Postal Service walk-in-revenue � Geographic coordinates for post office locations � Employment, # of establishments and households � Adjusted gross income and # of tax returns 7
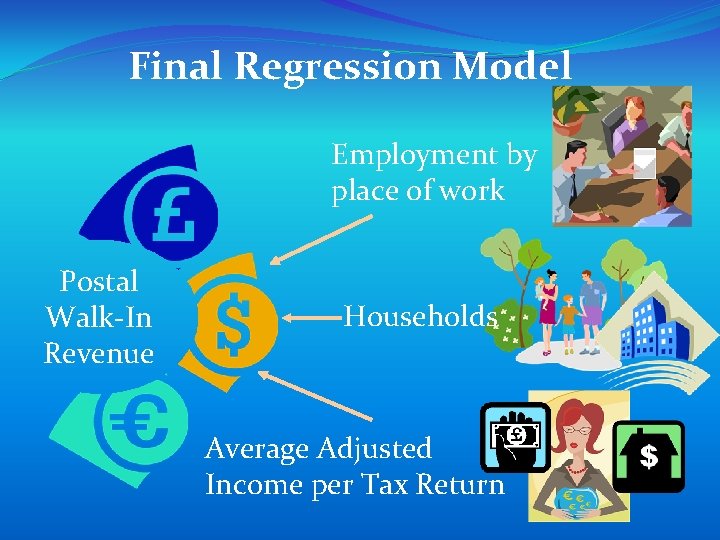
Final Regression Model Employment by place of work Postal Walk-In Revenue Households Average Adjusted Income per Tax Return
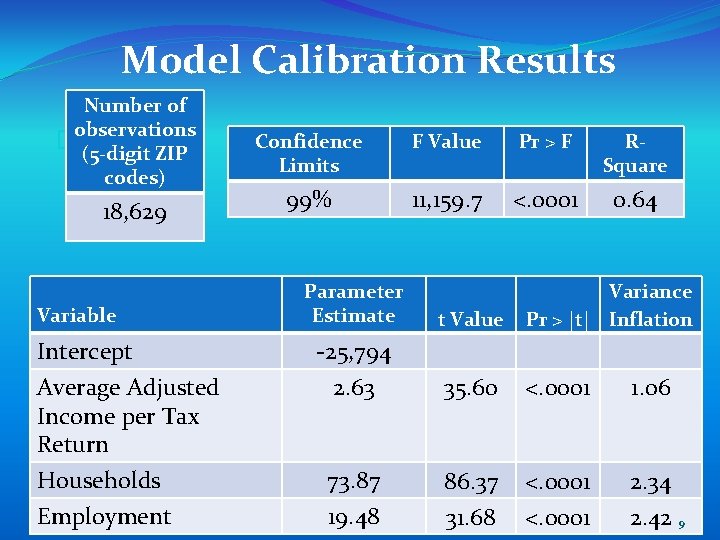
Model Calibration Results Number of �observations (5 -digit ZIP codes) 18, 629 Variable Intercept Average Adjusted Income per Tax Return Households Employment Confidence Limits F Value Pr > F RSquare 99% 11, 159. 7 <. 0001 0. 64 Variance Pr > |t| Inflation Parameter Estimate t Value -25, 794 2. 63 35. 60 <. 0001 1. 06 73. 87 19. 48 86. 37 31. 68 <. 0001 2. 34 2. 42 9
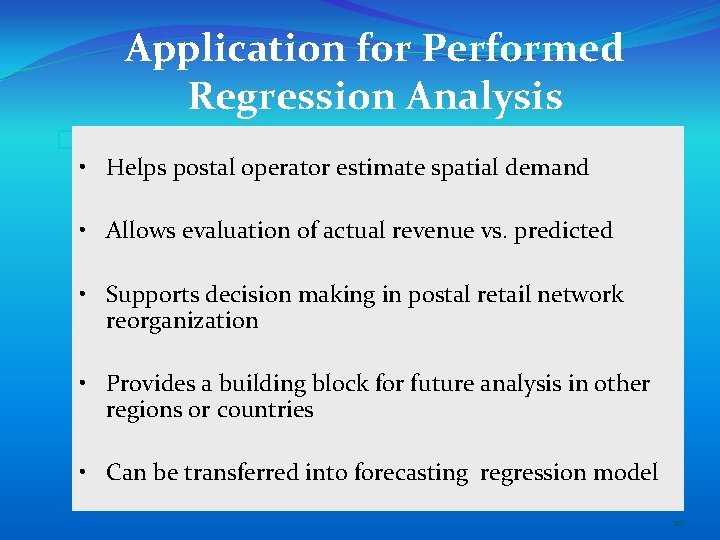
Application for Performed Regression Analysis � • Helps postal operator estimate spatial demand • Allows evaluation of actual revenue vs. predicted • Supports decision making in postal retail network reorganization • Provides a building block for future analysis in other regions or countries • Can be transferred into forecasting regression model 10
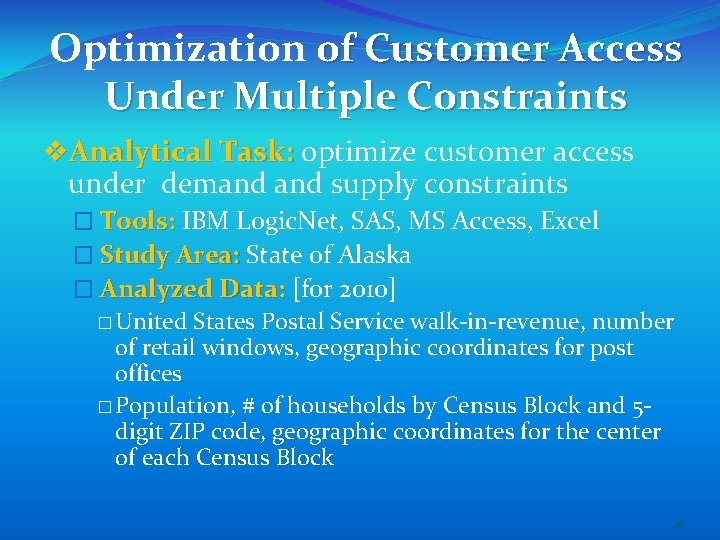
Optimization of Customer Access Under Multiple Constraints v. Analytical Task: optimize customer access under demand supply constraints � Tools: IBM Logic. Net, SAS, MS Access, Excel � Study Area: State of Alaska � Analyzed Data: [for 2010] � United States Postal Service walk-in-revenue, number of retail windows, geographic coordinates for post offices � Population, # of households by Census Block and 5 digit ZIP code, geographic coordinates for the center of each Census Block 11
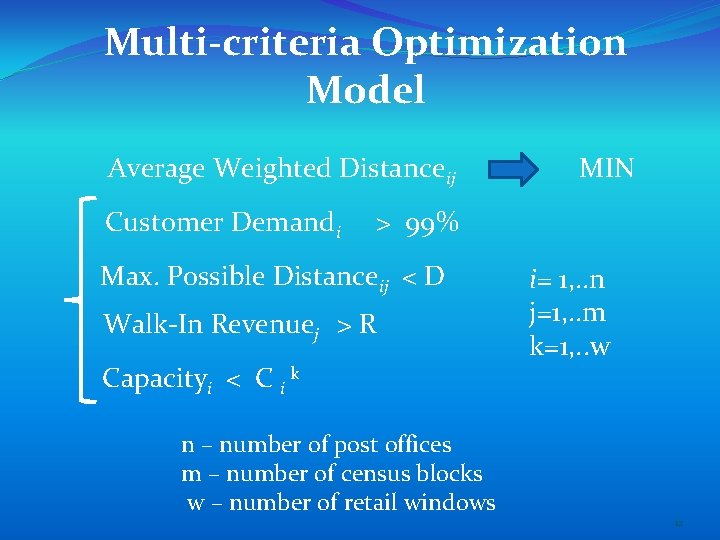
Multi-criteria Optimization Model Average Weighted Distanceij Customer Demandi MIN > 99% Max. Possible Distanceij < D Walk-In Revenuej > R Capacityi < C i k n – number of post offices m – number of census blocks w – number of retail windows i= 1, . . n j=1, . . m k=1, . . w 12
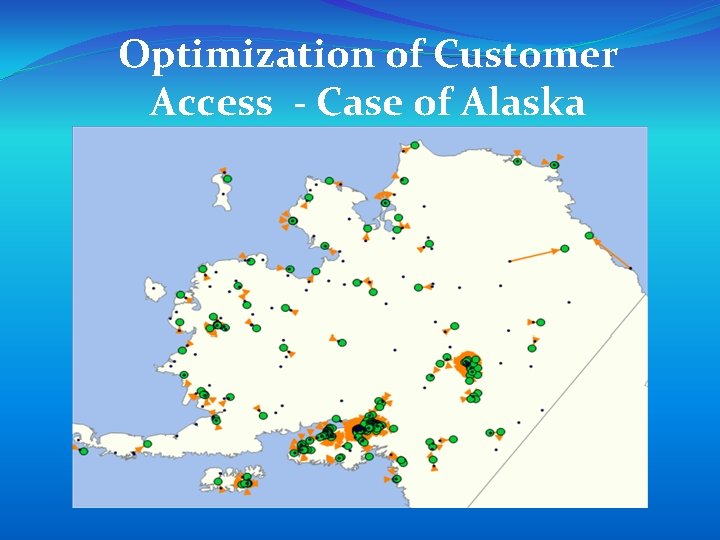
Optimization of Customer Access - Case of Alaska
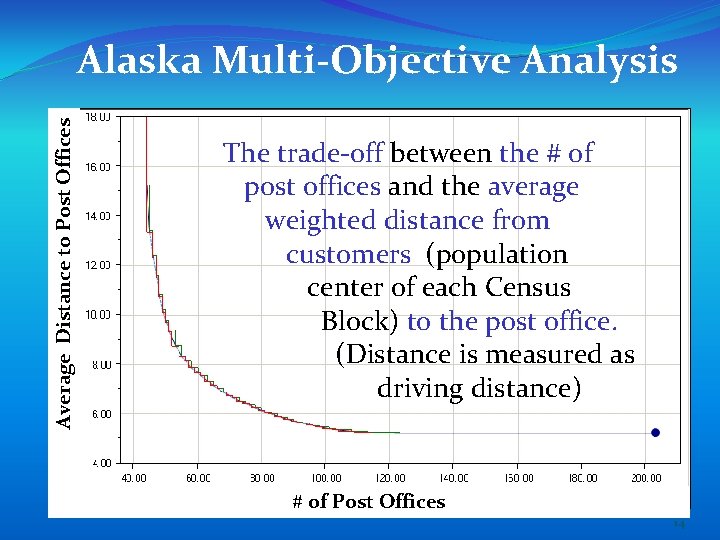
Average Distance to Post Offices Alaska Multi-Objective Analysis The trade-off between the # of post offices and the average weighted distance from customers (population center of each Census Block) to the post office. (Distance is measured as driving distance) # of Post Offices 14
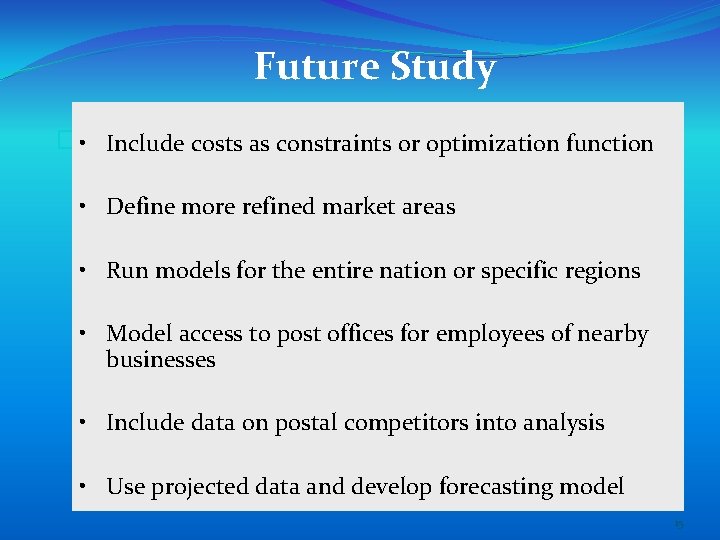
Future Study � • Include costs as constraints or optimization function • Define more refined market areas • Run models for the entire nation or specific regions • Model access to post offices for employees of nearby businesses • Include data on postal competitors into analysis • Use projected data and develop forecasting model 15
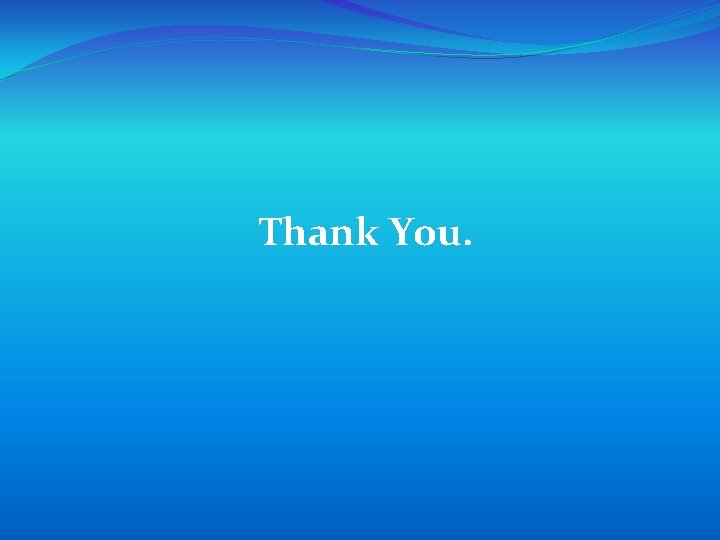
Thank You.
Chemdraw stationery document
Communication mix in retail
Retail management retail store organizational chart
Was the united states on the axis powers or allied powers?
Mediterranean climate in us
36 30 line
Soccer league hierarchy
United states v nixon outcome
United states student association
The united states ought to provide a universal basic income
Preamble of the constitution
The united states in the caribbean 1776 to 1985
Physical features of the southeast region
Expansion of the united states of america 1607 to 1853 map
Why did truman believe greece needed aid
Who was the previous owner of texas
Awake united states song