Neuroelectronics The Neuron Neuron The Device Synapse Dendrites
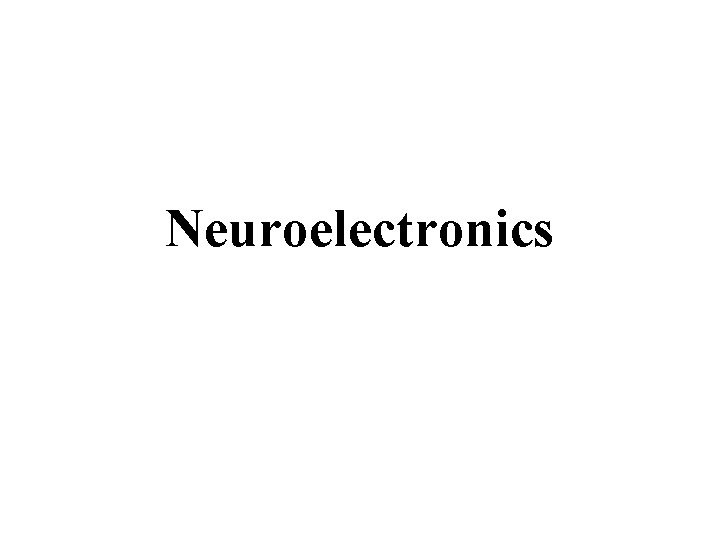
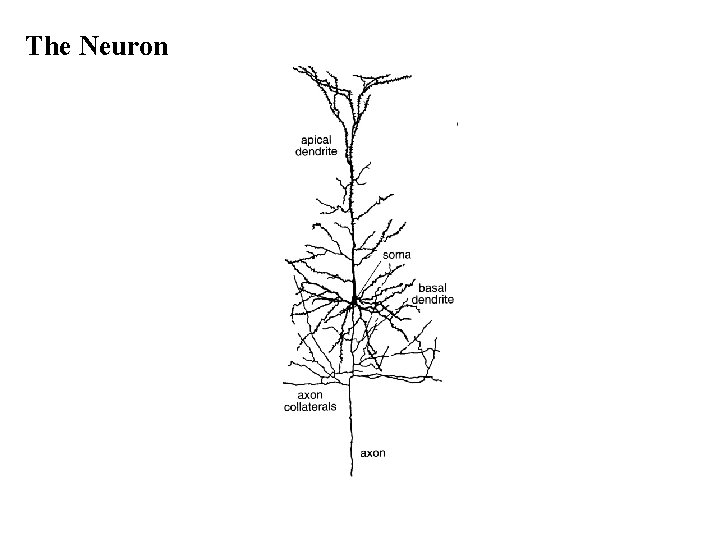
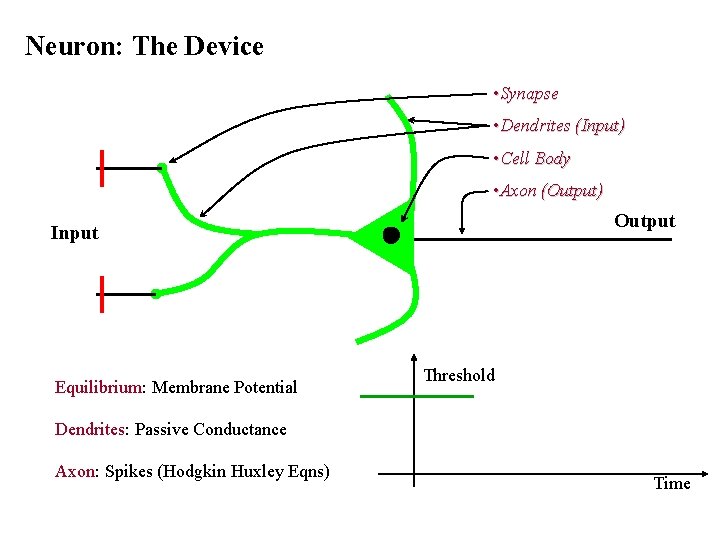
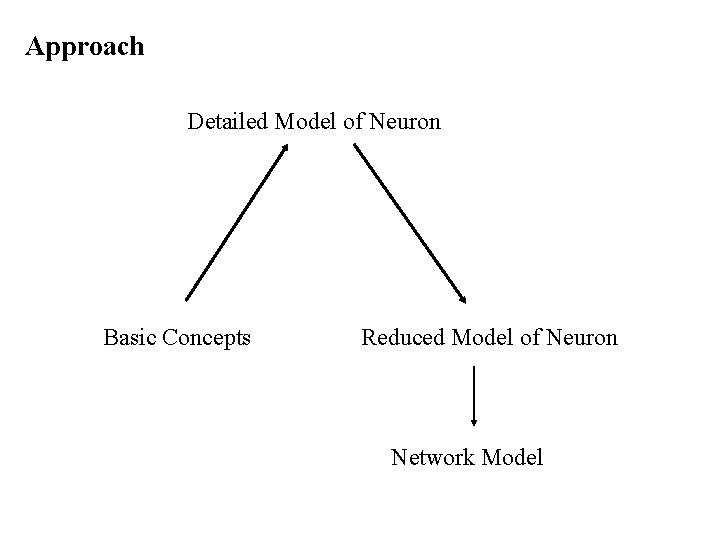
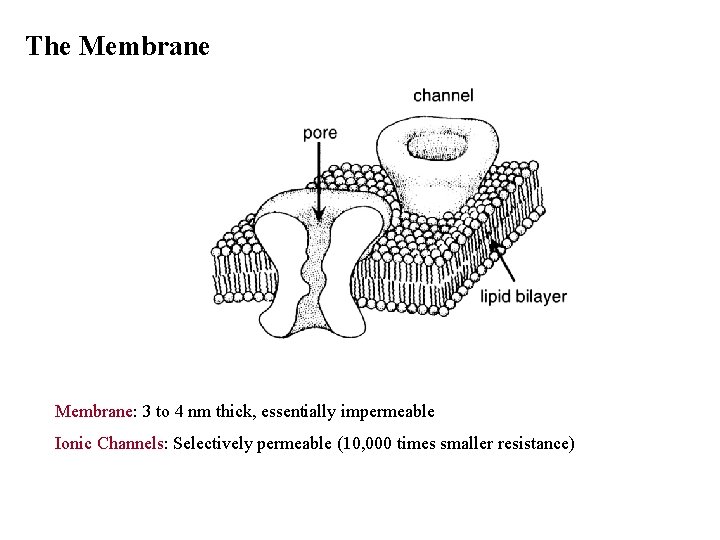
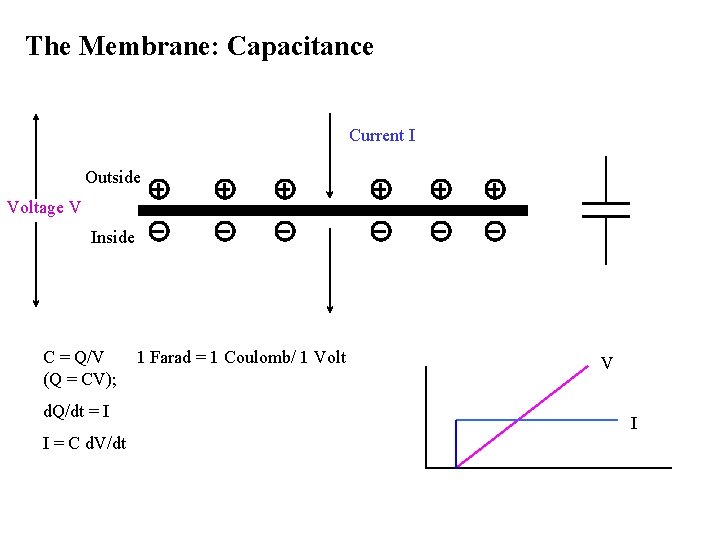
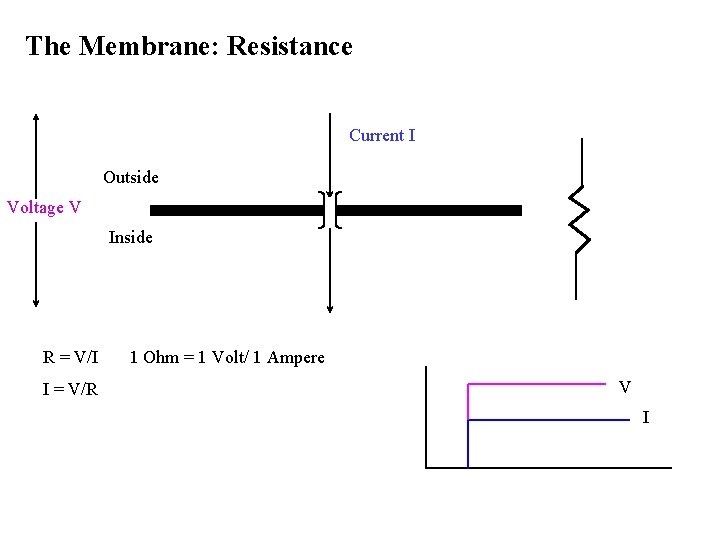
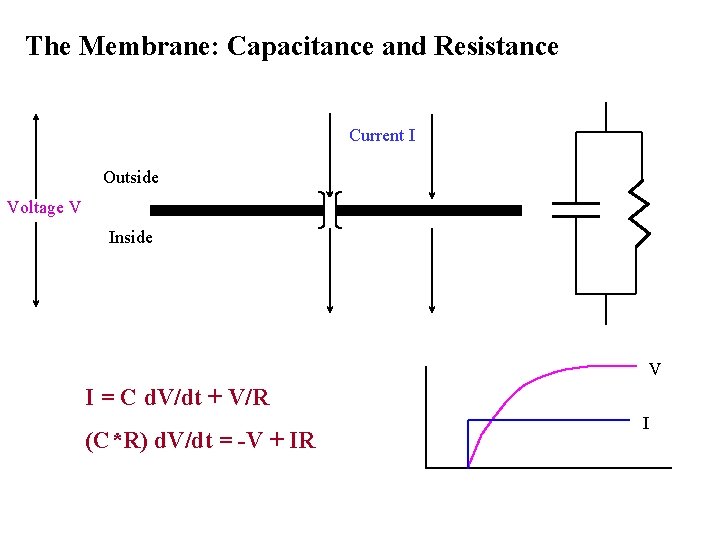
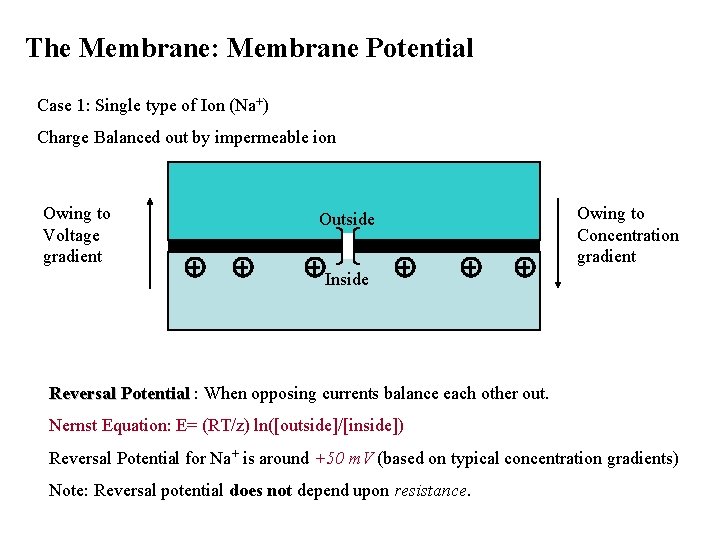
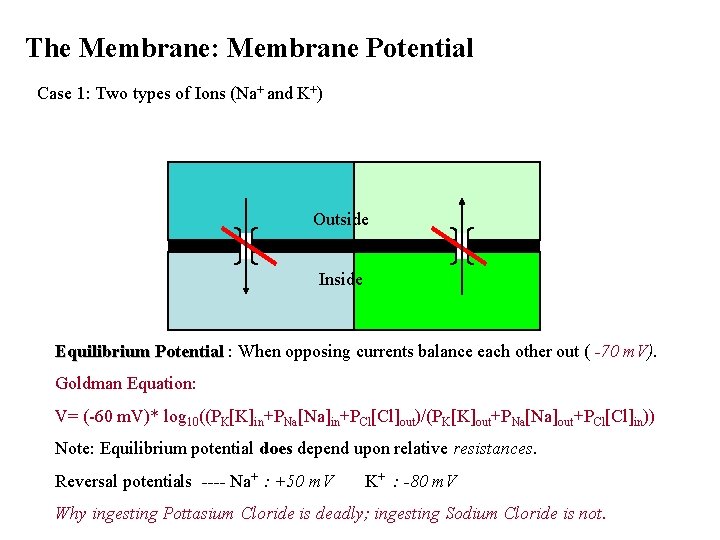
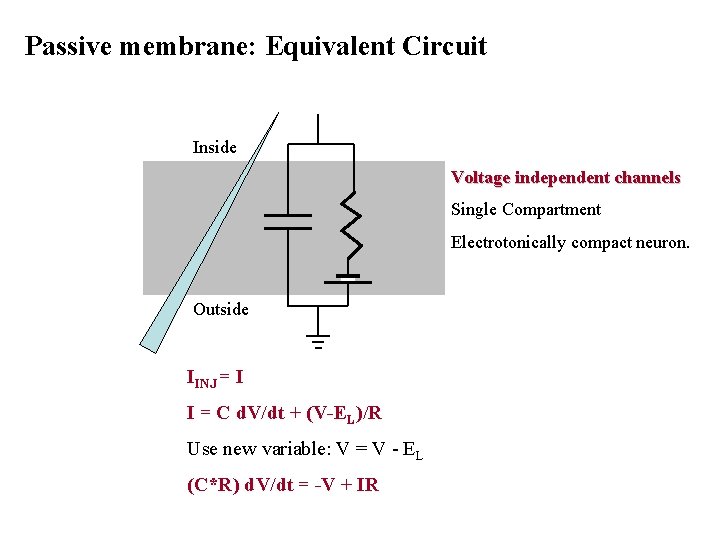
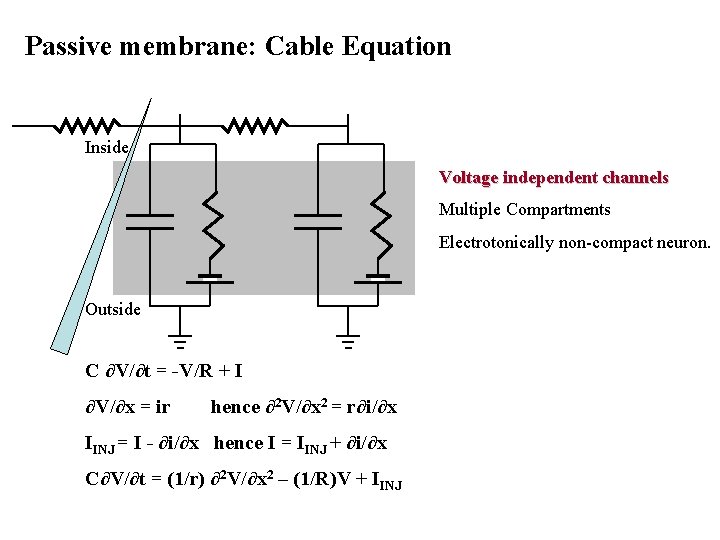
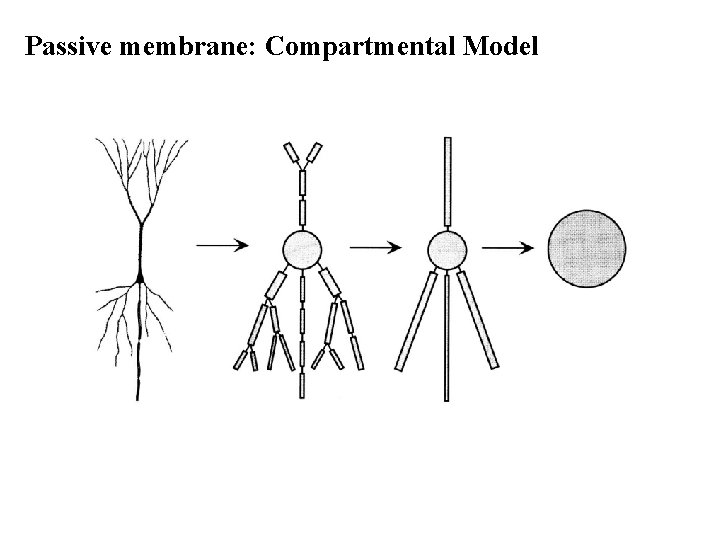
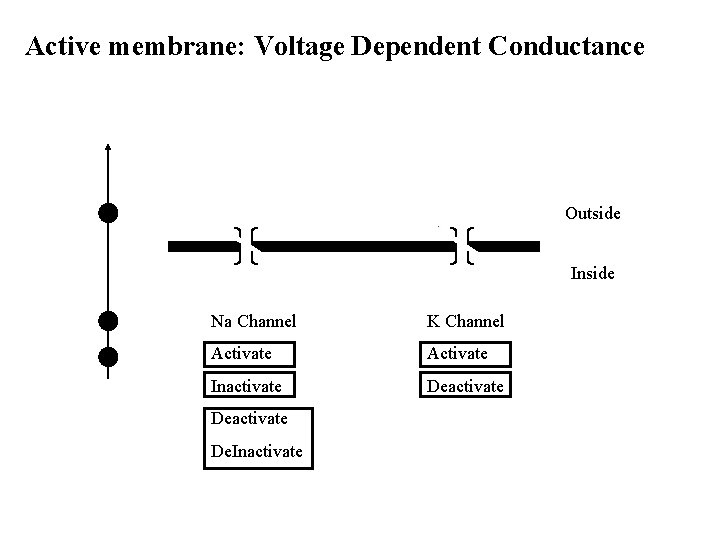
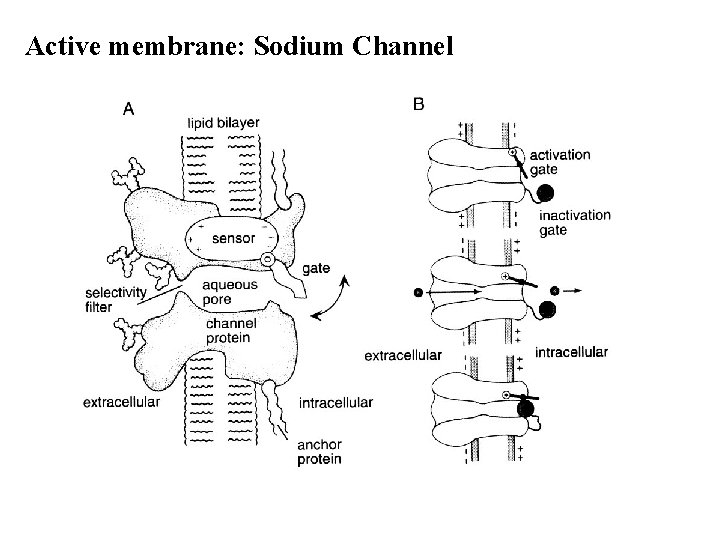
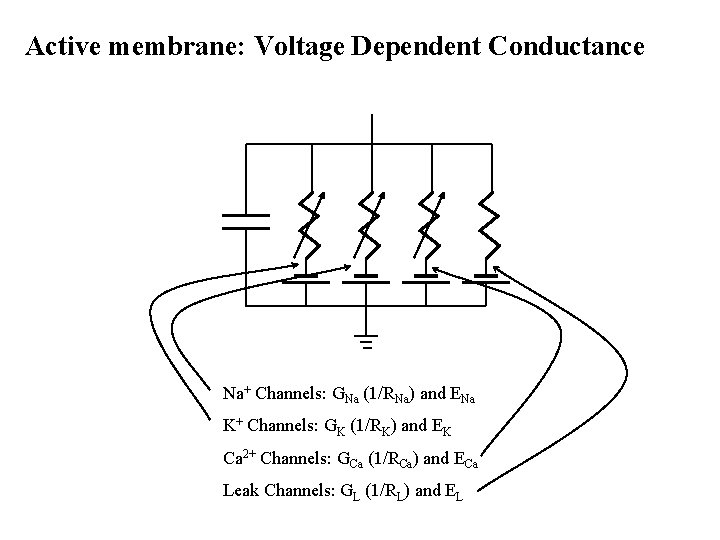
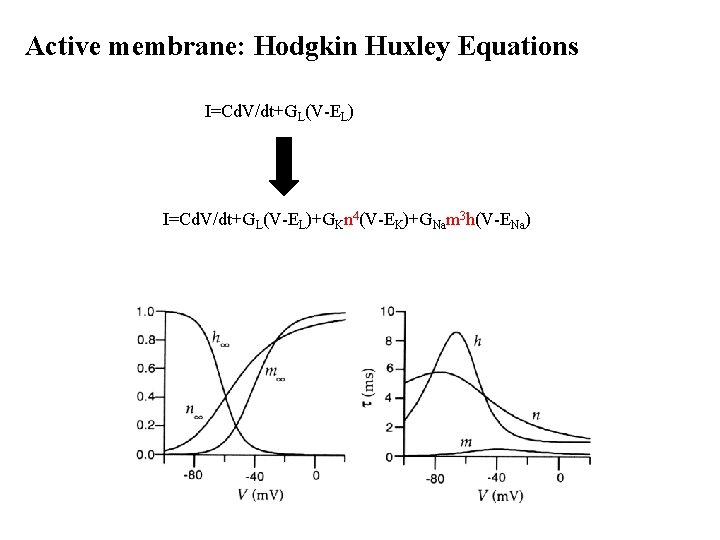
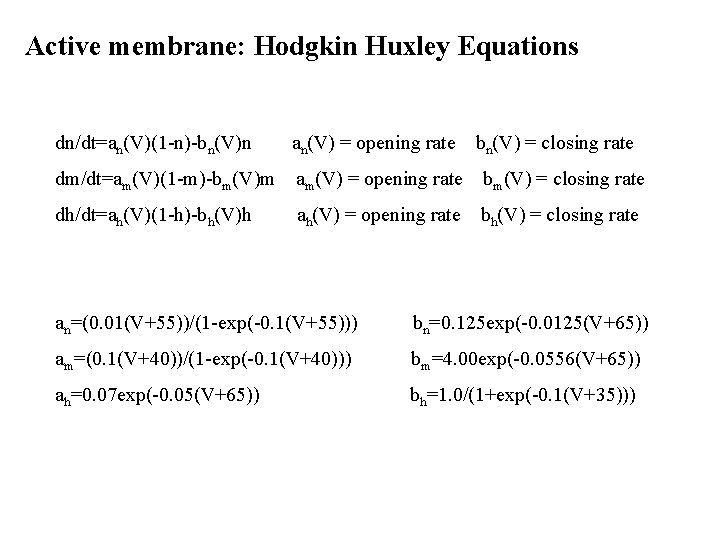
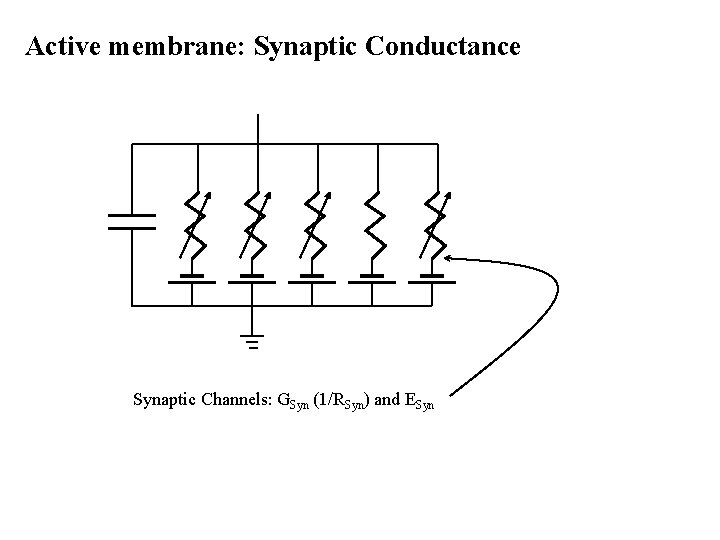
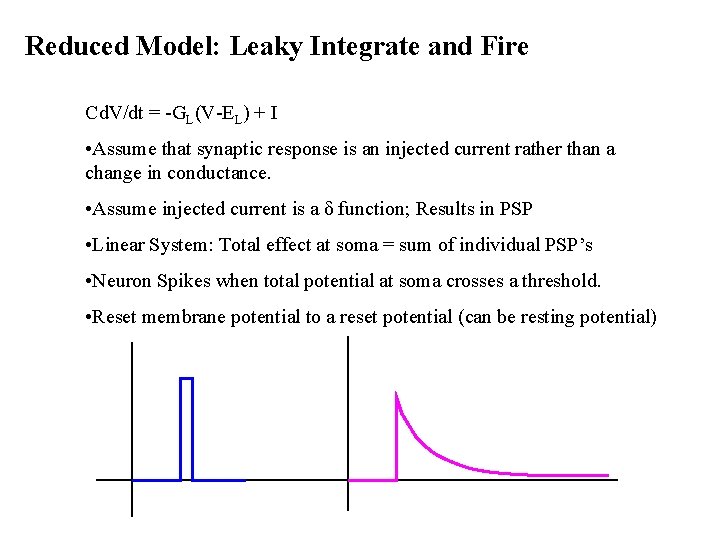
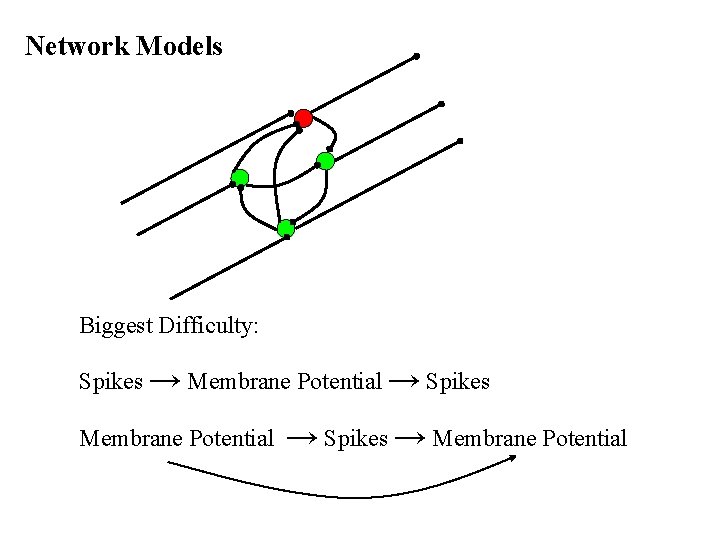
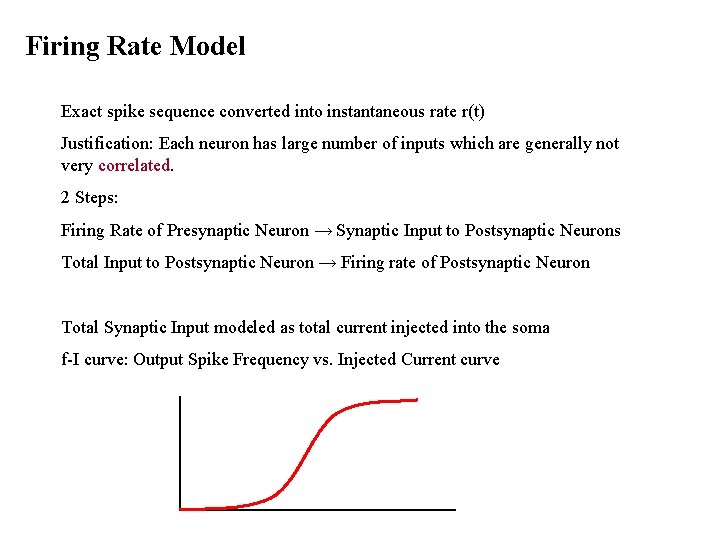
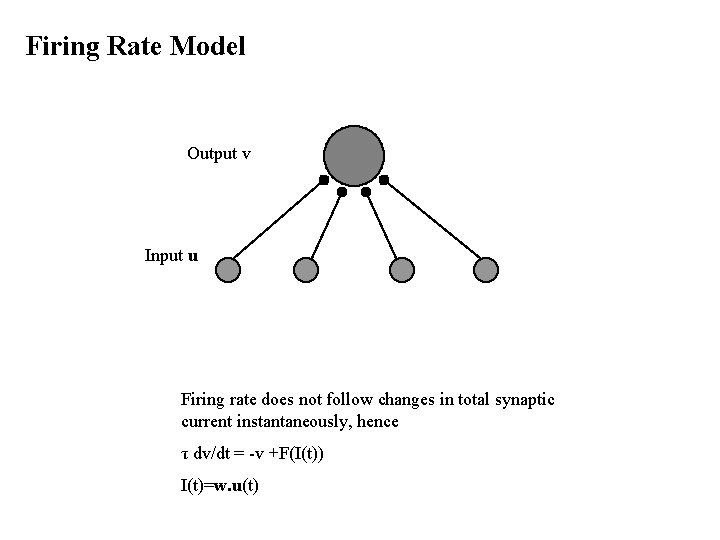
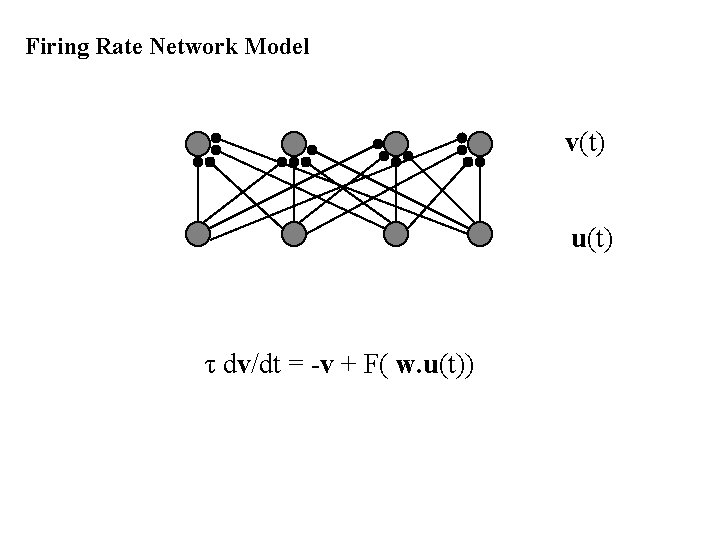
- Slides: 24
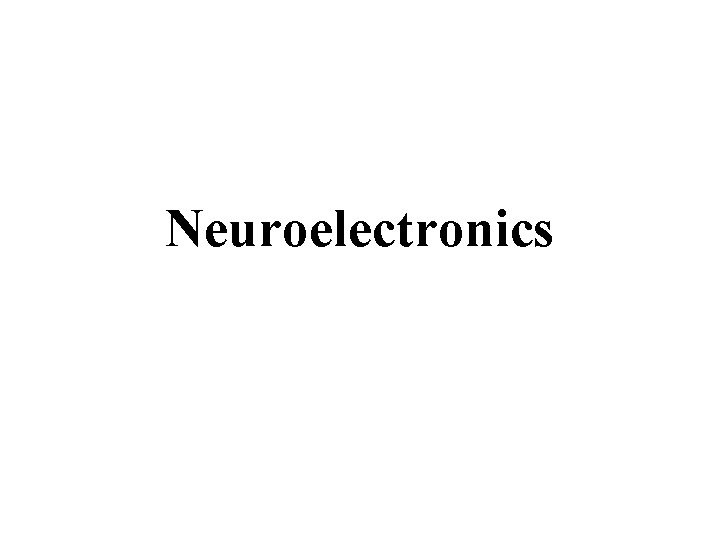
Neuroelectronics
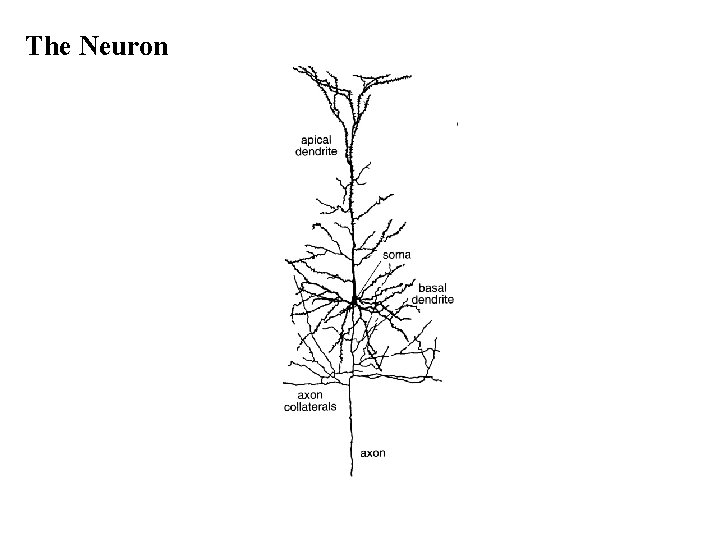
The Neuron
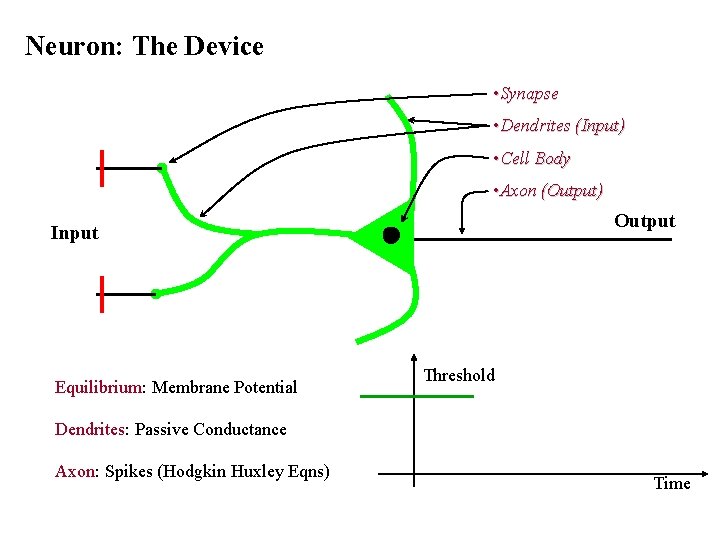
Neuron: The Device • Synapse • Dendrites (Input) • Cell Body • Axon (Output) Output Input Equilibrium: Membrane Potential Threshold Dendrites: Passive Conductance Axon: Spikes (Hodgkin Huxley Eqns) Time
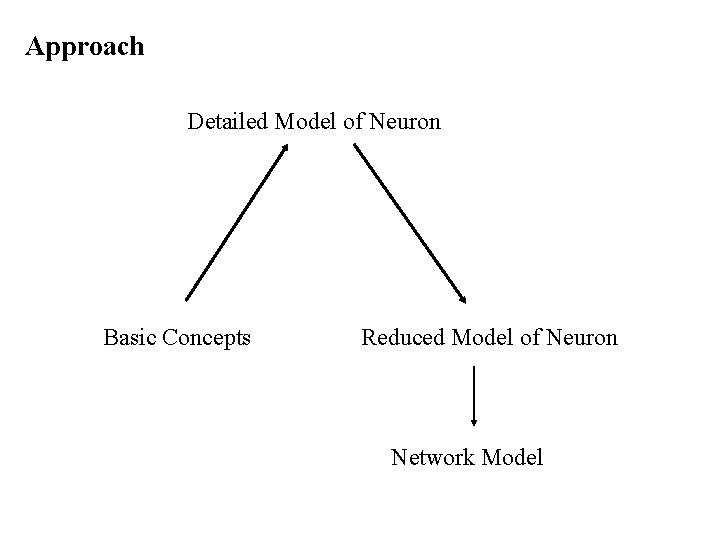
Approach Detailed Model of Neuron Basic Concepts Reduced Model of Neuron Network Model
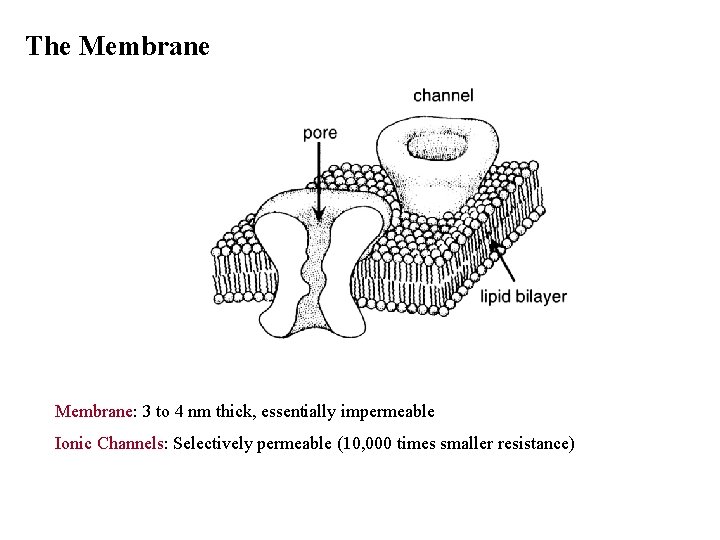
The Membrane: 3 to 4 nm thick, essentially impermeable Ionic Channels: Selectively permeable (10, 000 times smaller resistance)
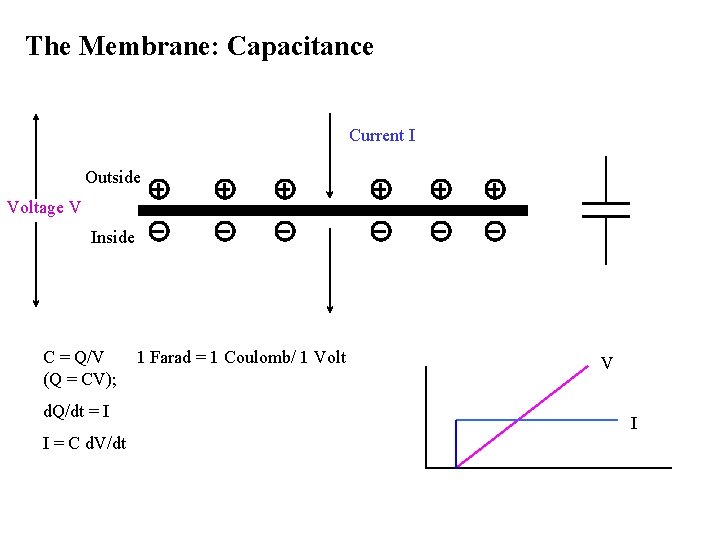
The Membrane: Capacitance Current I Outside Voltage V Inside C = Q/V (Q = CV); d. Q/dt = I I = C d. V/dt 1 Farad = 1 Coulomb/ 1 Volt V I
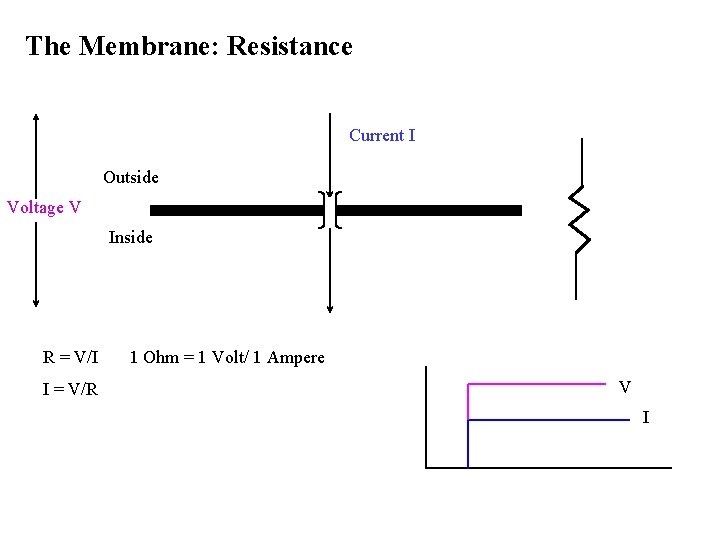
The Membrane: Resistance Current I Outside Voltage V Inside R = V/I I = V/R 1 Ohm = 1 Volt/ 1 Ampere V I
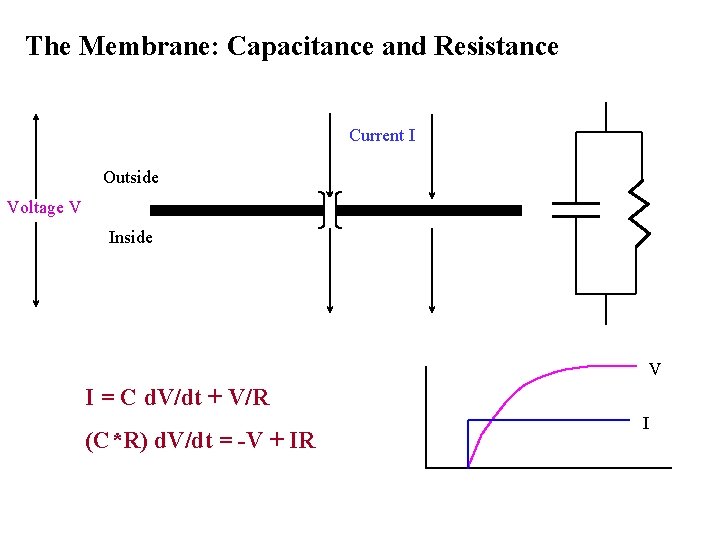
The Membrane: Capacitance and Resistance Current I Outside Voltage V Inside V I = C d. V/dt + V/R (C*R) d. V/dt = -V + IR I
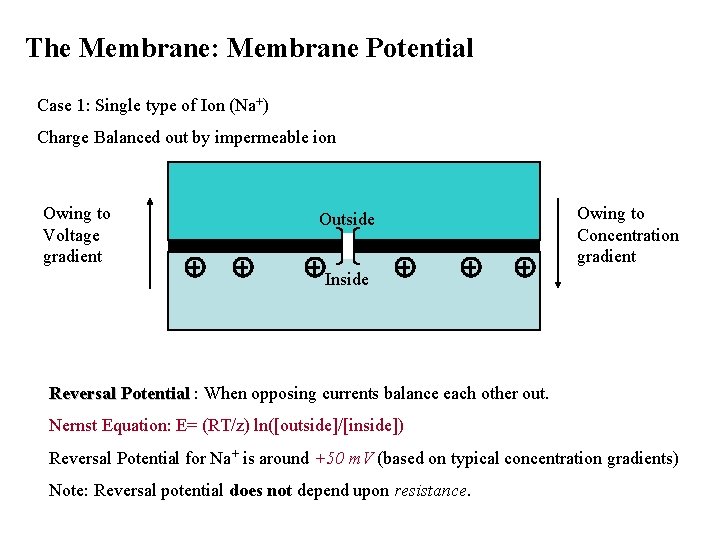
The Membrane: Membrane Potential Case 1: Single type of Ion (Na+) Charge Balanced out by impermeable ion Owing to Voltage gradient Outside Owing to Concentration gradient Inside Reversal Potential : When opposing currents balance each other out. Nernst Equation: E= (RT/z) ln([outside]/[inside]) Reversal Potential for Na+ is around +50 m. V (based on typical concentration gradients) Note: Reversal potential does not depend upon resistance.
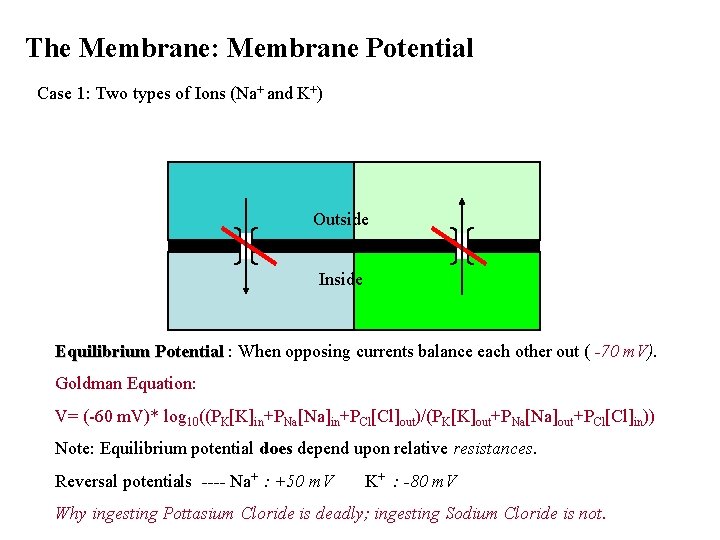
The Membrane: Membrane Potential Case 1: Two types of Ions (Na+ and K+) Outside Inside Equilibrium Potential : When opposing currents balance each other out ( -70 m. V). Goldman Equation: V= (-60 m. V)* log 10((PK[K]in+PNa[Na]in+PCl[Cl]out)/(PK[K]out+PNa[Na]out+PCl[Cl]in)) Note: Equilibrium potential does depend upon relative resistances. Reversal potentials ---- Na+ : +50 m. V K+ : -80 m. V Why ingesting Pottasium Cloride is deadly; ingesting Sodium Cloride is not.
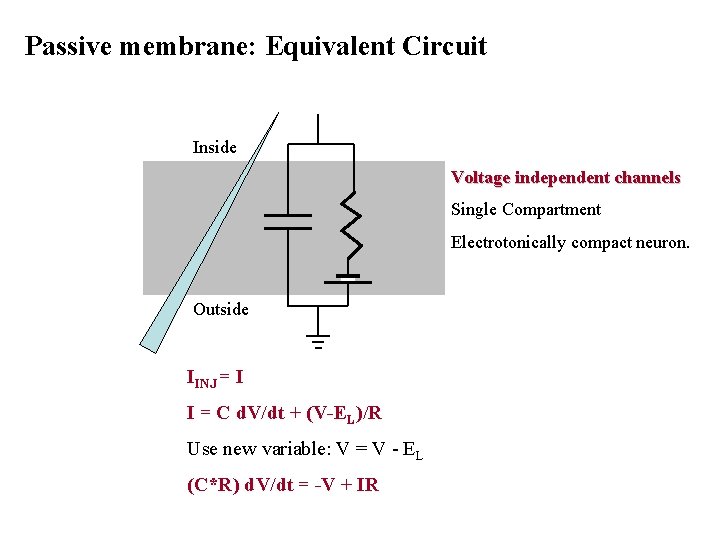
Passive membrane: Equivalent Circuit Inside Voltage independent channels Single Compartment Electrotonically compact neuron. Outside IINJ = I I = C d. V/dt + (V-EL)/R Use new variable: V = V - EL (C*R) d. V/dt = -V + IR
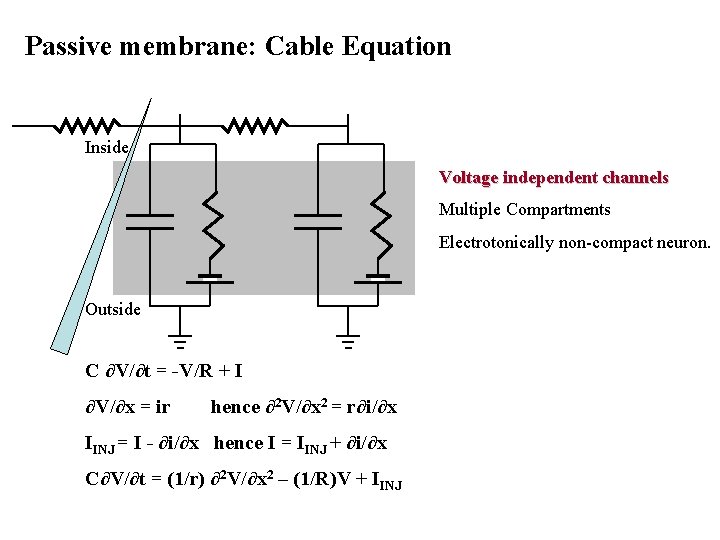
Passive membrane: Cable Equation Inside Voltage independent channels Multiple Compartments Electrotonically non-compact neuron. Outside C ∂V/∂t = -V/R + I ∂V/∂x = ir hence ∂2 V/∂x 2 = r∂i/∂x IINJ = I - ∂i/∂x hence I = IINJ + ∂i/∂x C∂V/∂t = (1/r) ∂2 V/∂x 2 – (1/R)V + IINJ
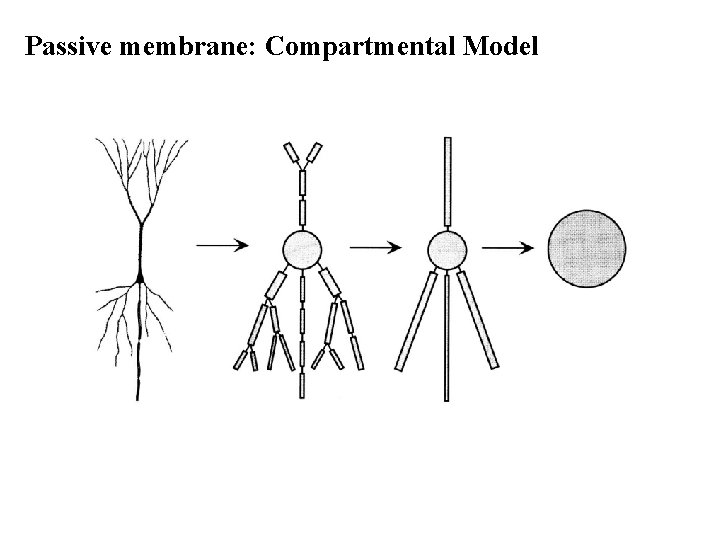
Passive membrane: Compartmental Model
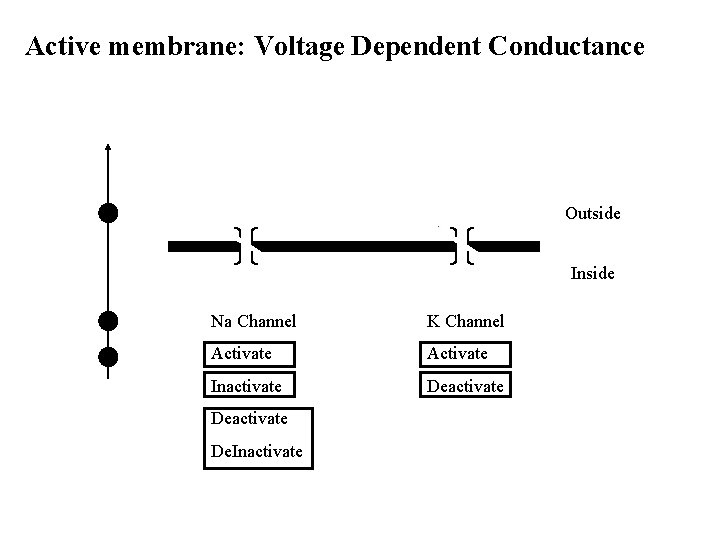
Active membrane: Voltage Dependent Conductance Outside Inside Na Channel K Channel Activate Inactivate Deactivate De. Inactivate
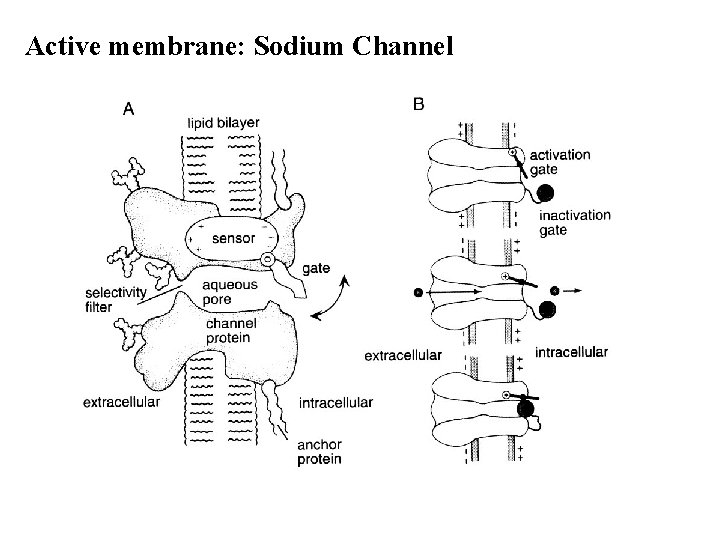
Active membrane: Sodium Channel
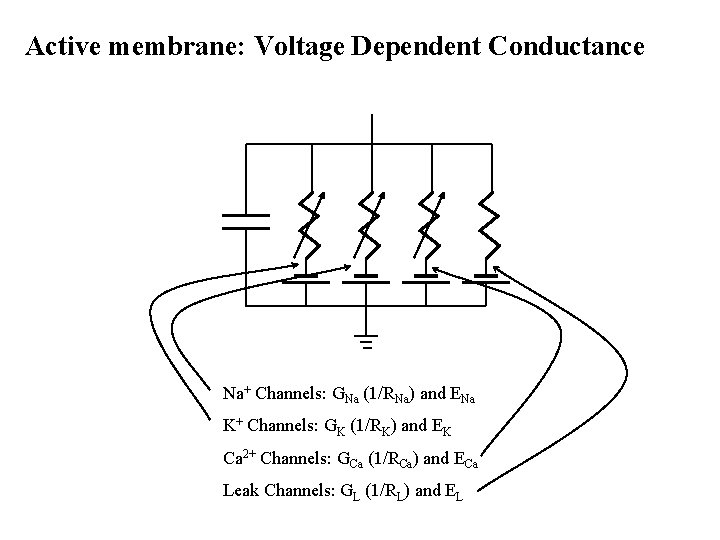
Active membrane: Voltage Dependent Conductance Na+ Channels: GNa (1/RNa) and ENa K+ Channels: GK (1/RK) and EK Ca 2+ Channels: GCa (1/RCa) and ECa Leak Channels: GL (1/RL) and EL
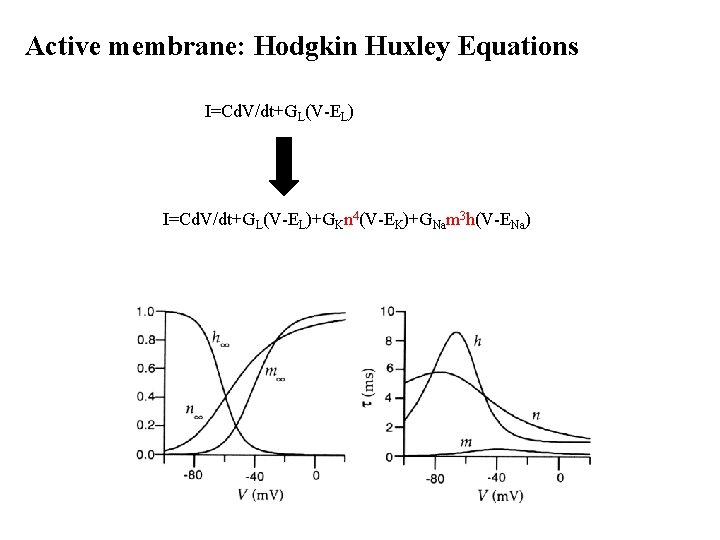
Active membrane: Hodgkin Huxley Equations I=Cd. V/dt+GL(V-EL)+GKn 4(V-EK)+GNam 3 h(V-ENa)
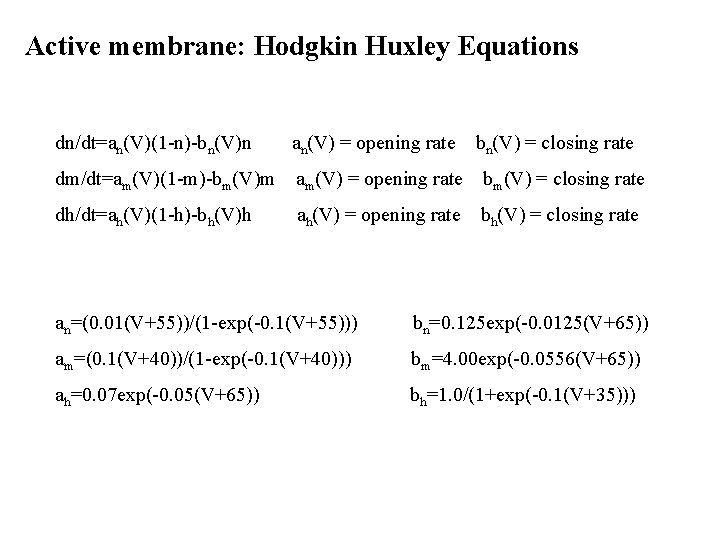
Active membrane: Hodgkin Huxley Equations dn/dt=an(V)(1 -n)-bn(V)n an(V) = opening rate bn(V) = closing rate dm/dt=am(V)(1 -m)-bm(V)m am(V) = opening rate bm(V) = closing rate dh/dt=ah(V)(1 -h)-bh(V)h ah(V) = opening rate bh(V) = closing rate an=(0. 01(V+55))/(1 -exp(-0. 1(V+55))) bn=0. 125 exp(-0. 0125(V+65)) am=(0. 1(V+40))/(1 -exp(-0. 1(V+40))) bm=4. 00 exp(-0. 0556(V+65)) ah=0. 07 exp(-0. 05(V+65)) bh=1. 0/(1+exp(-0. 1(V+35)))
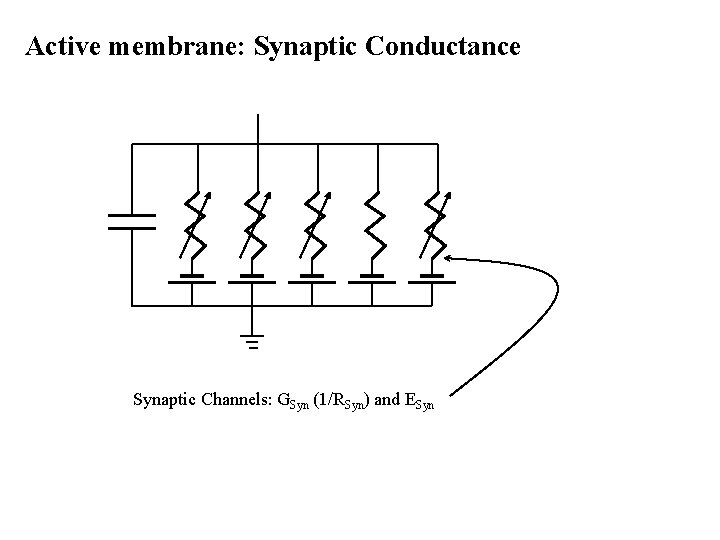
Active membrane: Synaptic Conductance Synaptic Channels: GSyn (1/RSyn) and ESyn
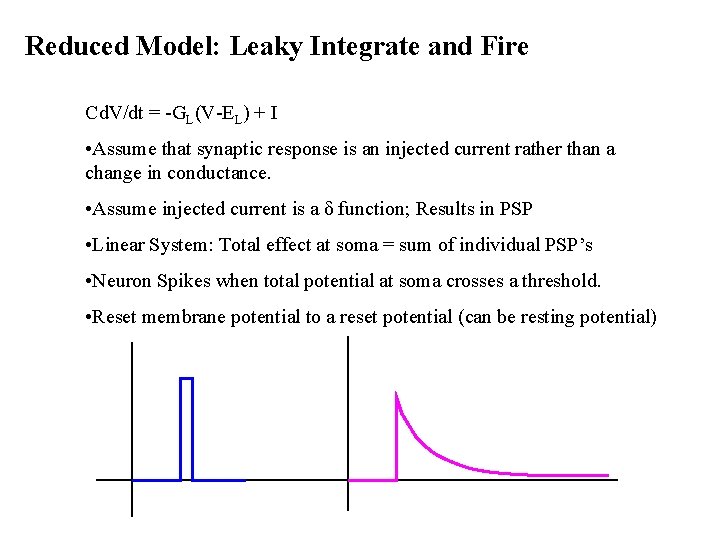
Reduced Model: Leaky Integrate and Fire Cd. V/dt = -GL(V-EL) + I • Assume that synaptic response is an injected current rather than a change in conductance. • Assume injected current is a δ function; Results in PSP • Linear System: Total effect at soma = sum of individual PSP’s • Neuron Spikes when total potential at soma crosses a threshold. • Reset membrane potential to a reset potential (can be resting potential)
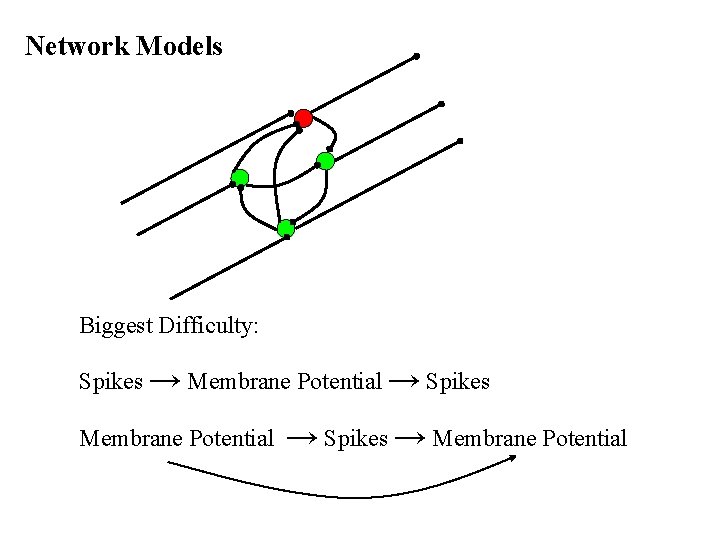
Network Models Biggest Difficulty: Spikes → Membrane Potential → Spikes → Membrane Potential
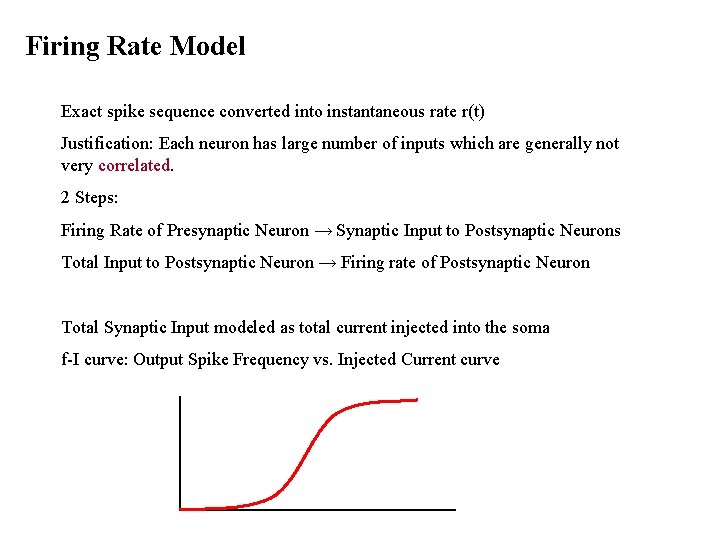
Firing Rate Model Exact spike sequence converted into instantaneous rate r(t) Justification: Each neuron has large number of inputs which are generally not very correlated. 2 Steps: Firing Rate of Presynaptic Neuron → Synaptic Input to Postsynaptic Neurons Total Input to Postsynaptic Neuron → Firing rate of Postsynaptic Neuron Total Synaptic Input modeled as total current injected into the soma f-I curve: Output Spike Frequency vs. Injected Current curve
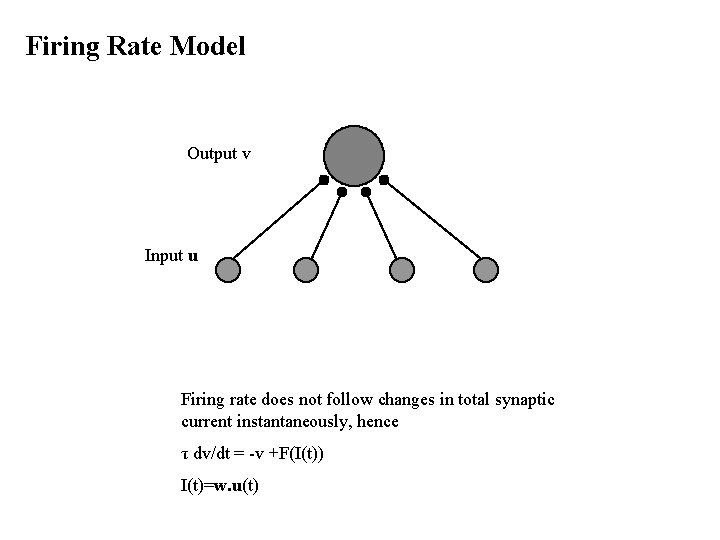
Firing Rate Model Output v Input u Firing rate does not follow changes in total synaptic current instantaneously, hence τ dv/dt = -v +F(I(t)) I(t)=w. u(t)
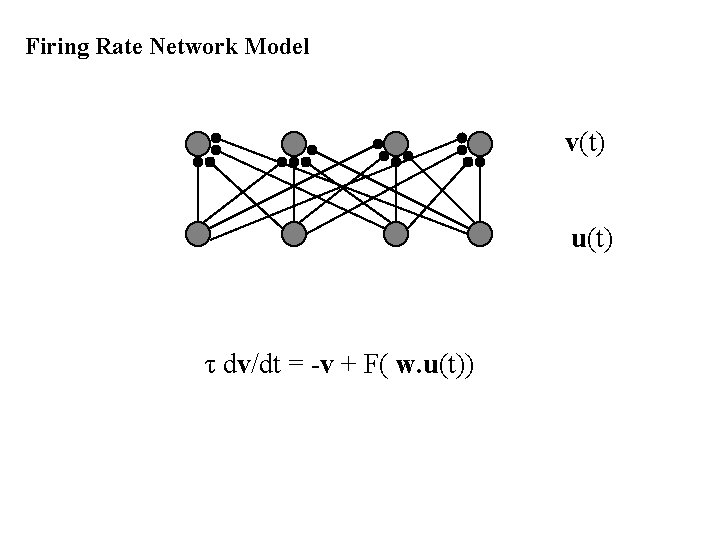
Firing Rate Network Model v(t) u(t) τ dv/dt = -v + F( w. u(t))
Electrical synapse vs chemical synapse
Electrical synapse vs chemical synapse
Neuron synapse
Dendrites analogy
Dendrites
Dendrites et axones
Dendrites
Input output storage devices
Sensorische nervenzelle bau und funktion
Synapse decompiler
Limitless analytics with azure synapse pdf
Electrical synapse
Conjoint synapse example
Chemical synapse
Synapse
Synapse histology
Synapse
Anaxonic neuron
Difference between ipsp and epsp
Hypopolarization
Synapse
Functions of the synapse
Axoaxonic synapse
Synapse vs databricks
Synapse