Modelling inflows for water valuation Dr Geoffrey Pritchard
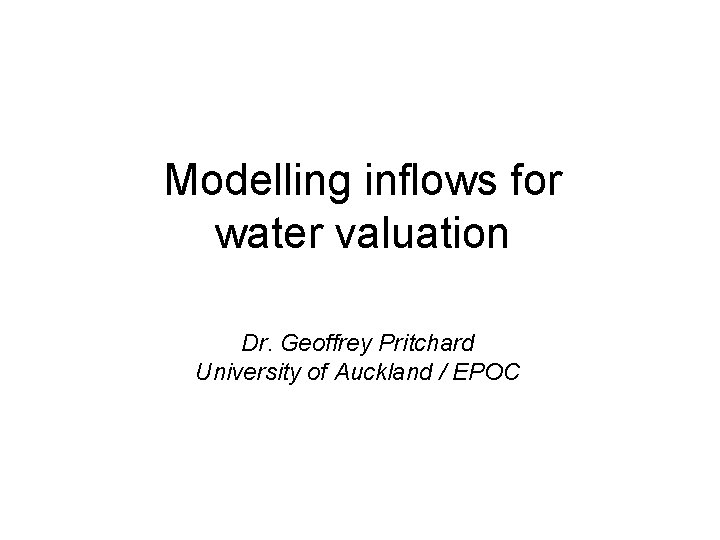
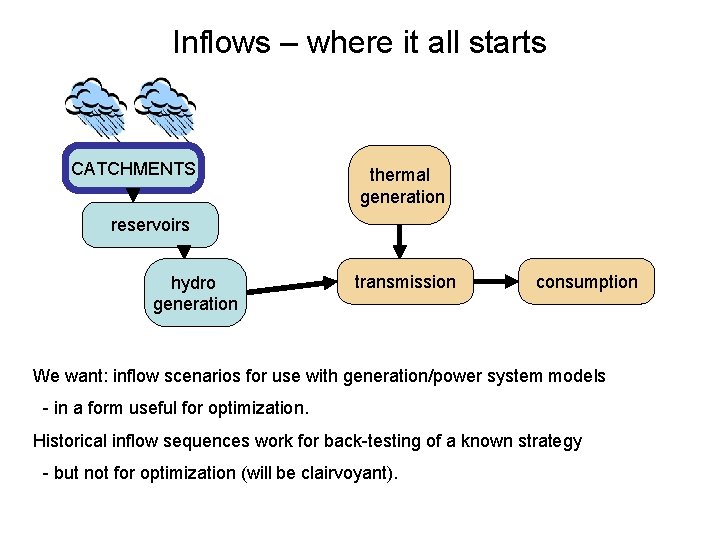
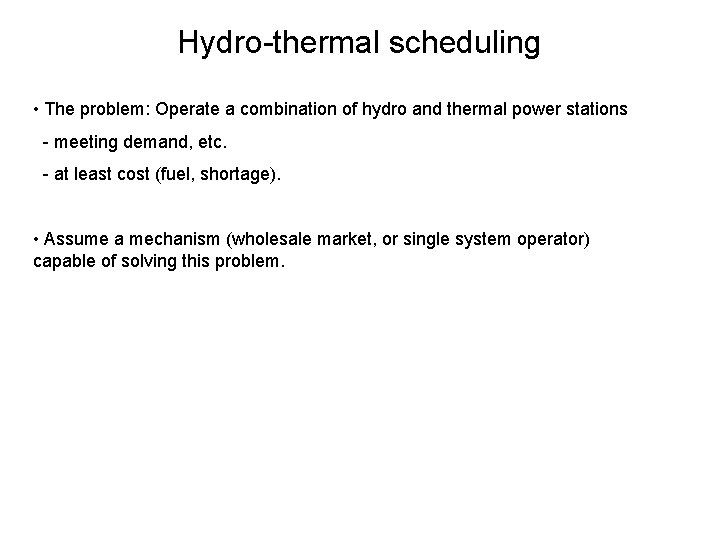
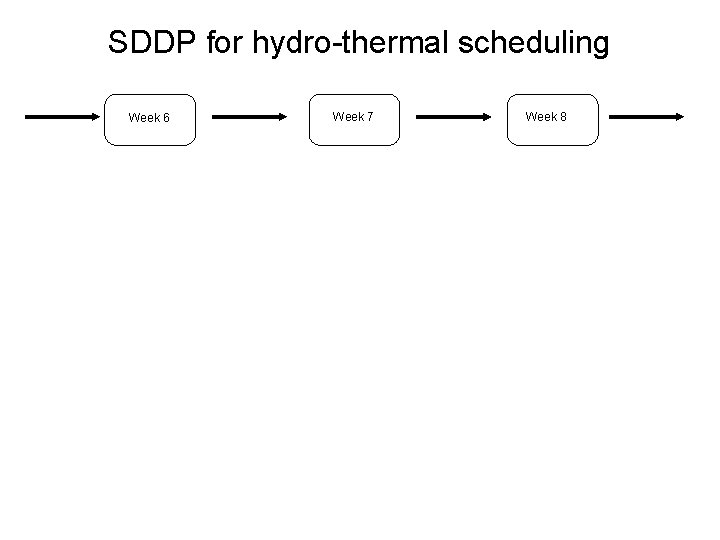
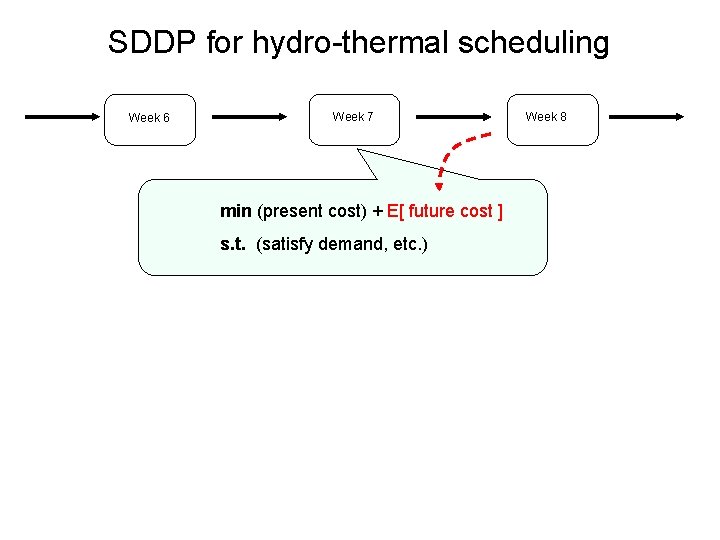
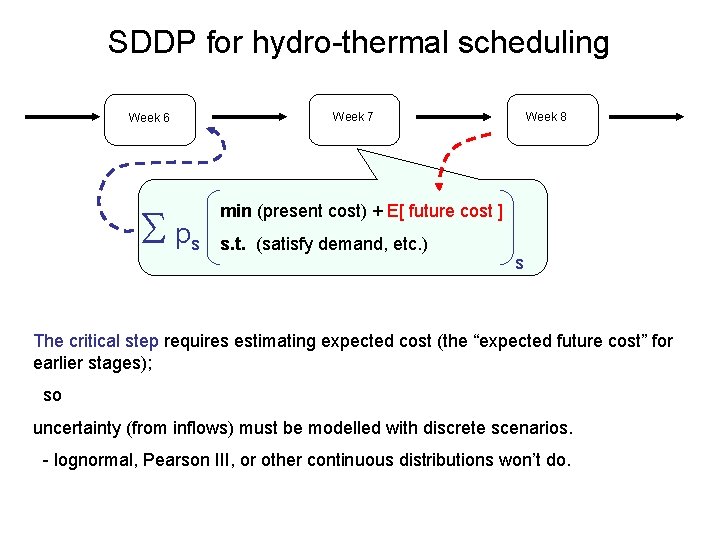
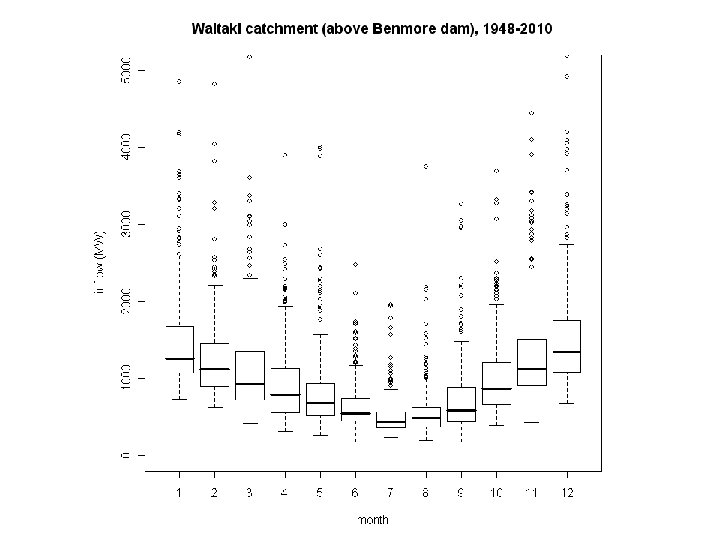
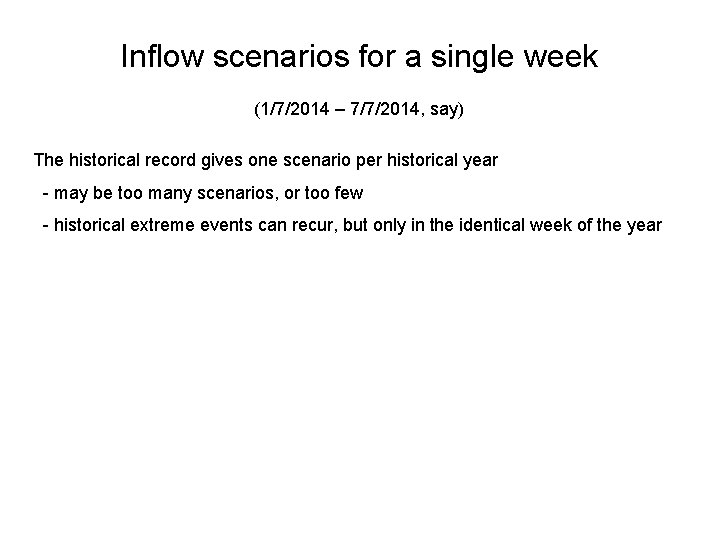
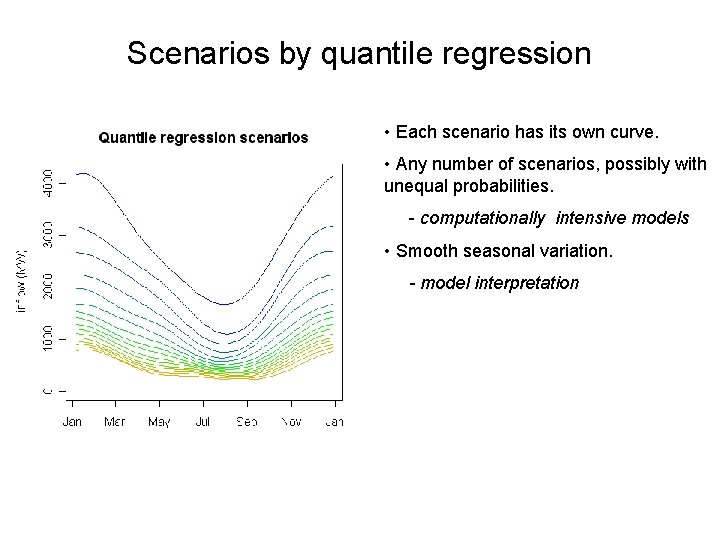
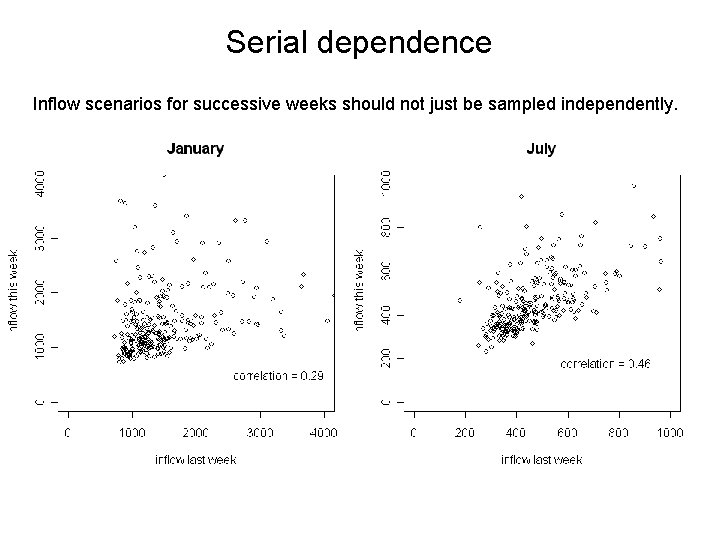
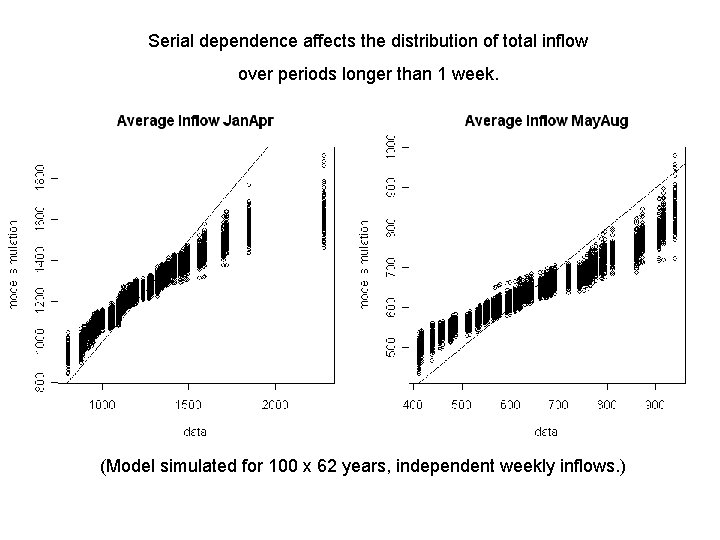
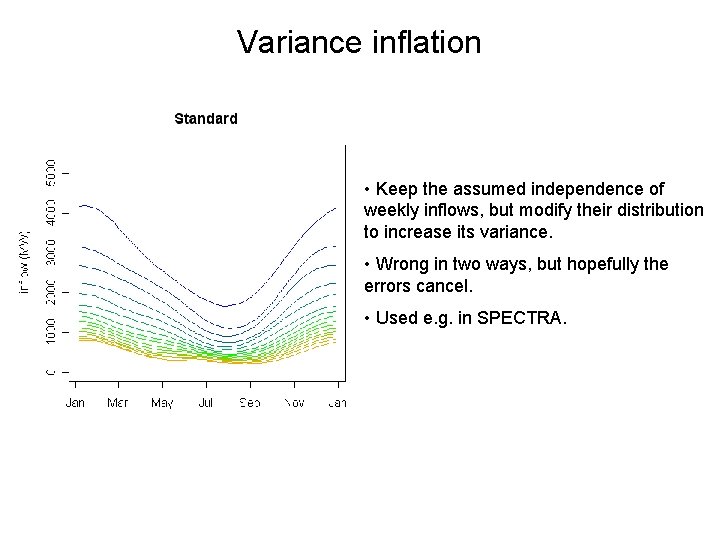
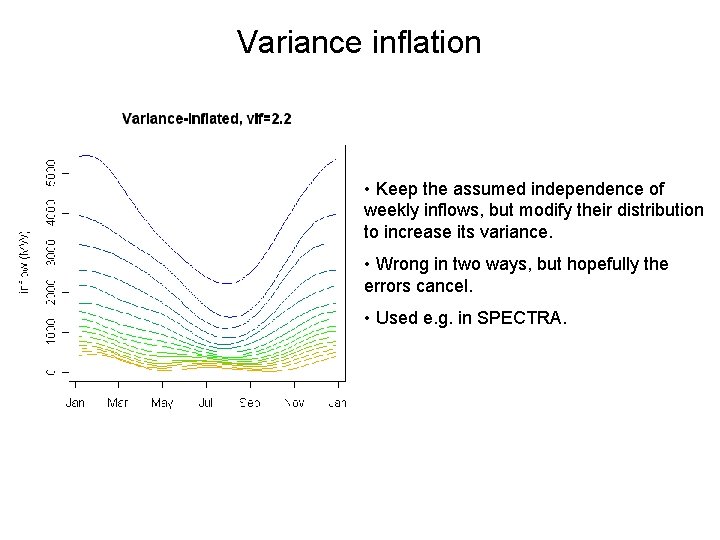
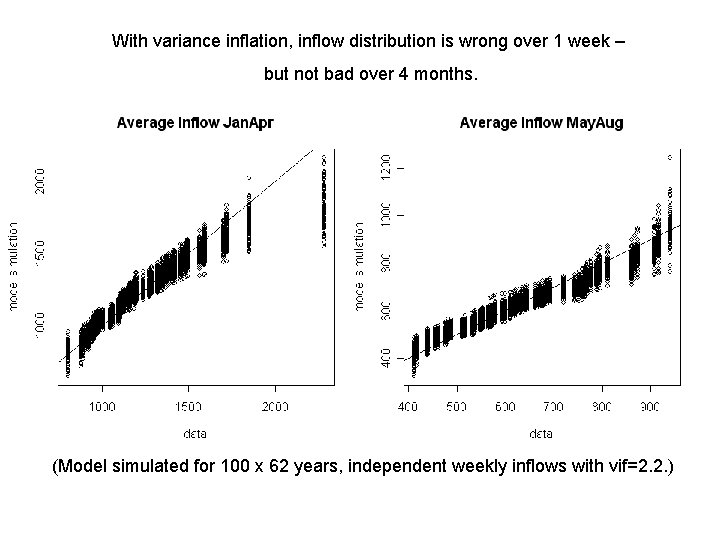
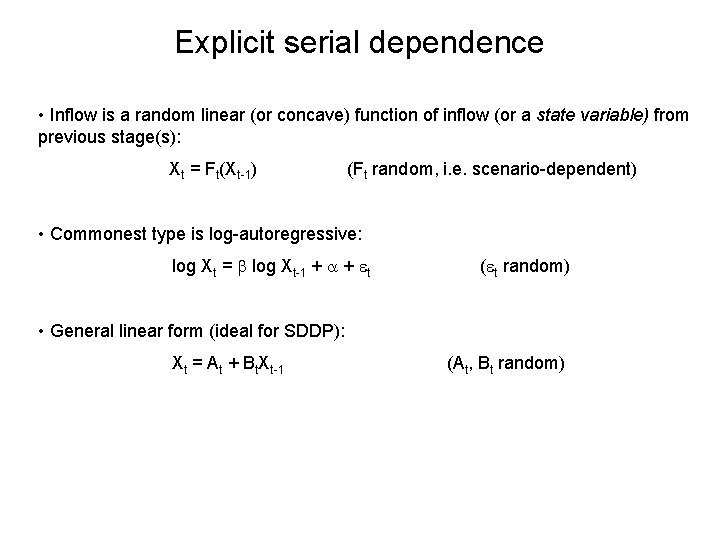
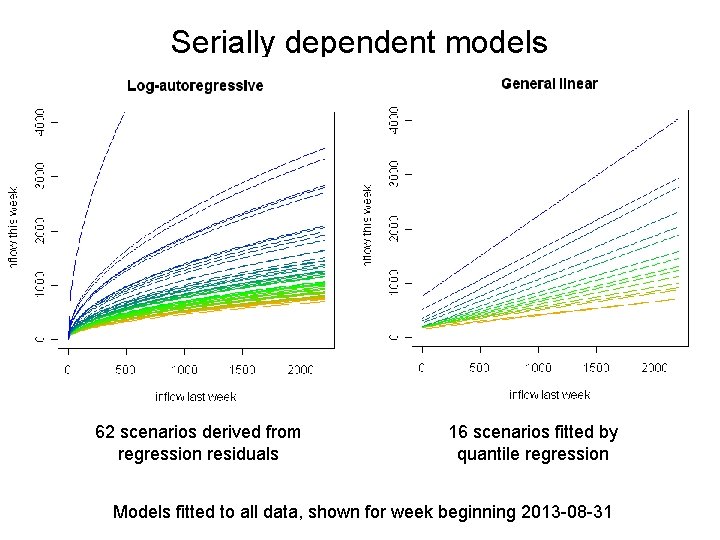
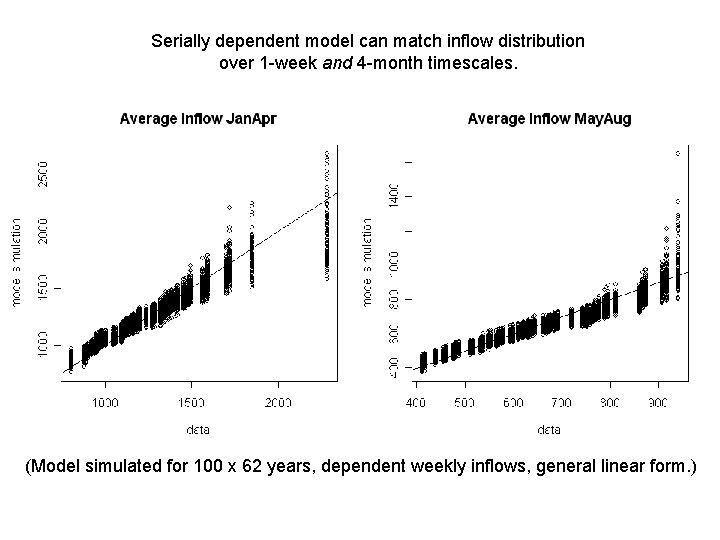
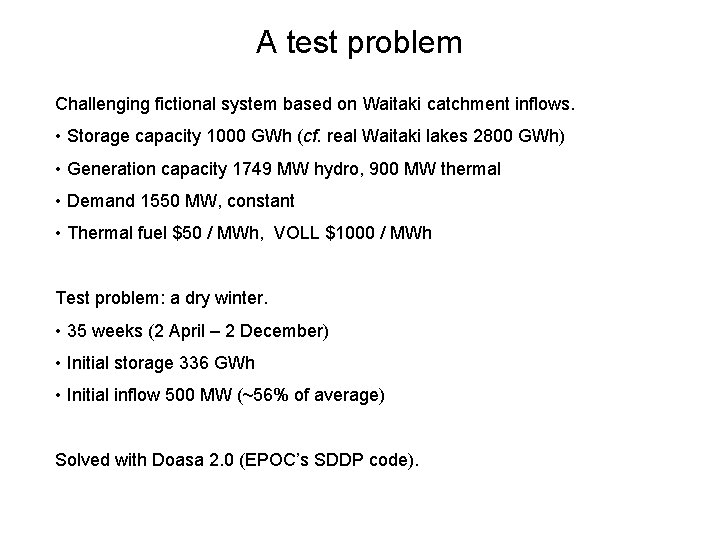
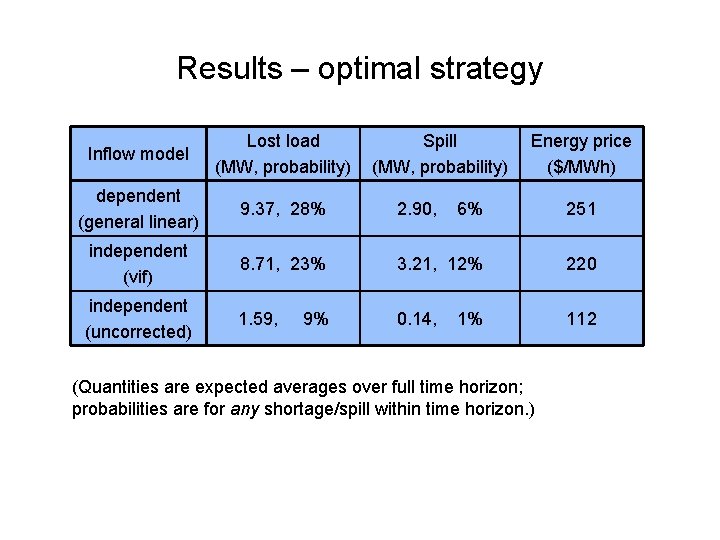
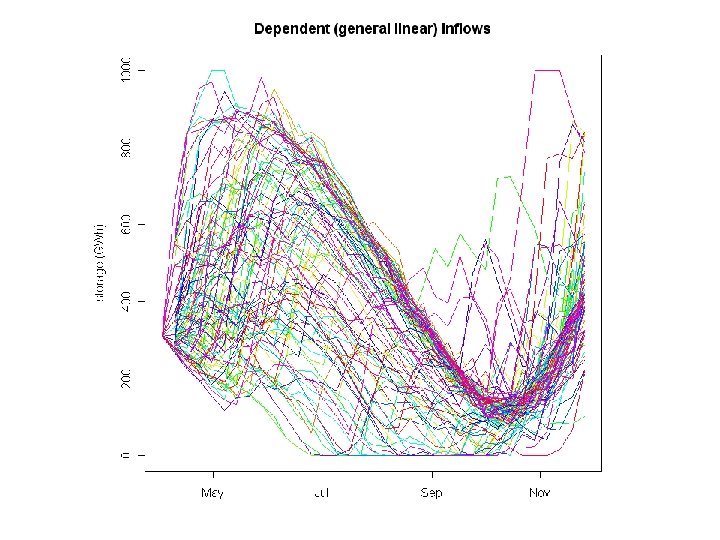
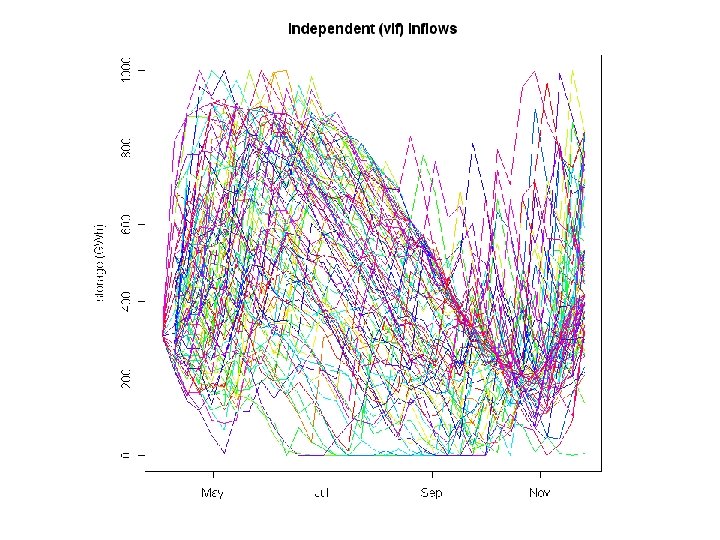
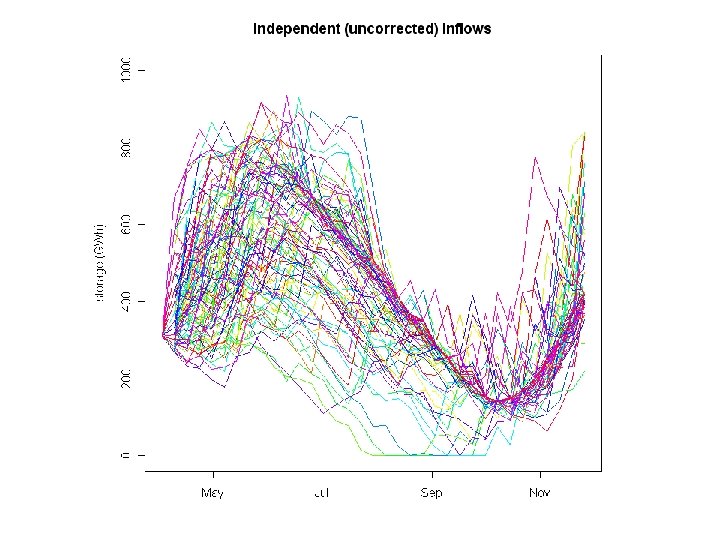
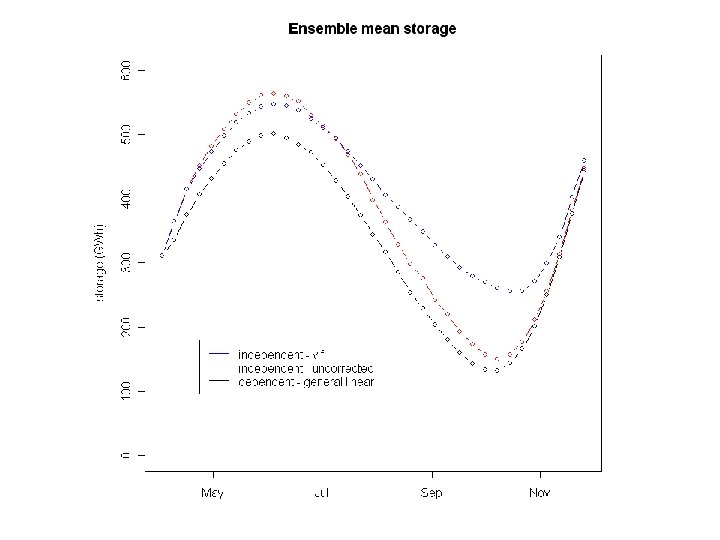
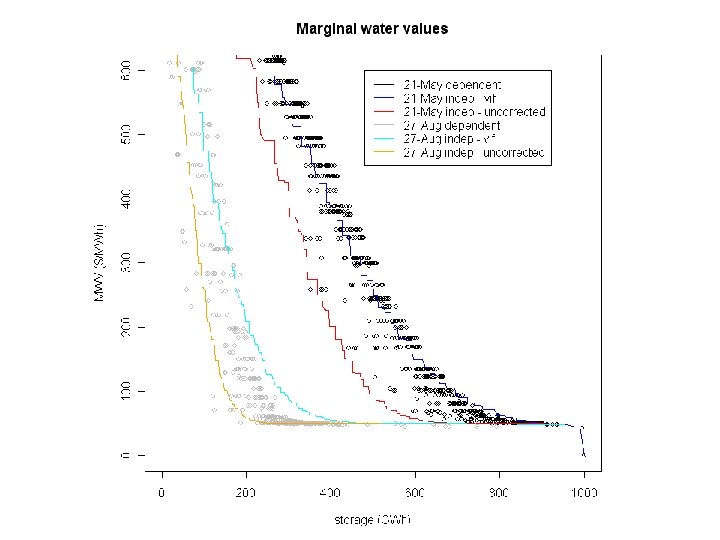
- Slides: 24
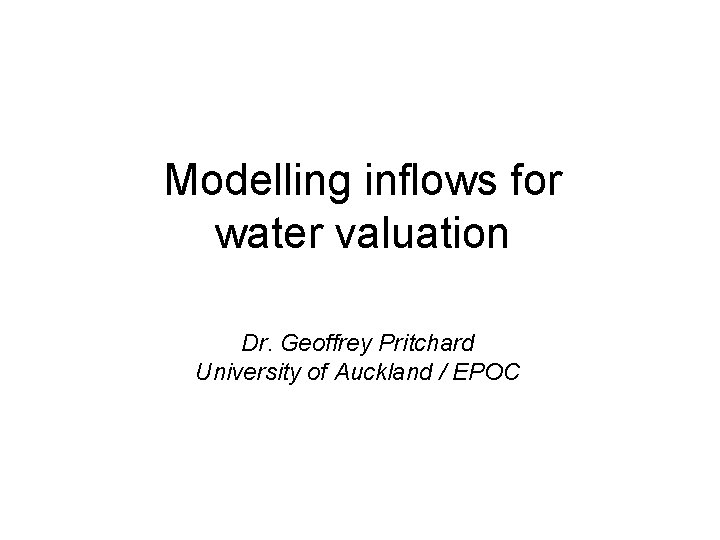
Modelling inflows for water valuation Dr. Geoffrey Pritchard University of Auckland / EPOC
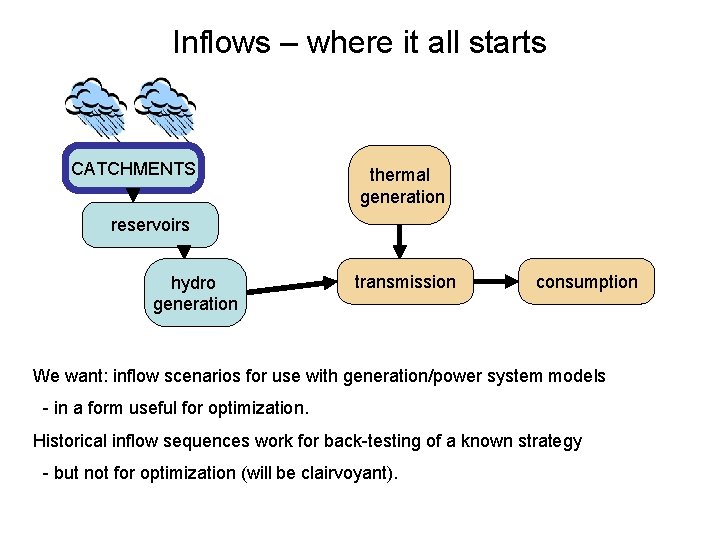
Inflows – where it all starts CATCHMENTS thermal generation reservoirs hydro generation transmission consumption We want: inflow scenarios for use with generation/power system models - in a form useful for optimization. Historical inflow sequences work for back-testing of a known strategy - but not for optimization (will be clairvoyant).
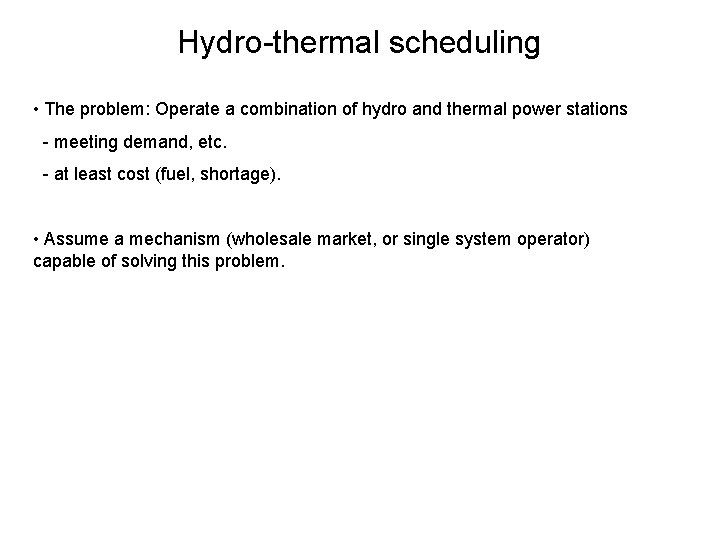
Hydro-thermal scheduling • The problem: Operate a combination of hydro and thermal power stations - meeting demand, etc. - at least cost (fuel, shortage). • Assume a mechanism (wholesale market, or single system operator) capable of solving this problem.
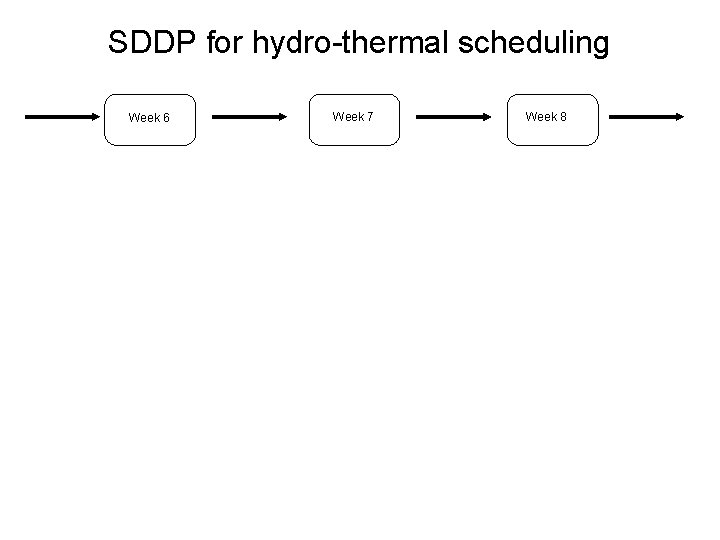
SDDP for hydro-thermal scheduling Week 6 Week 7 Week 8
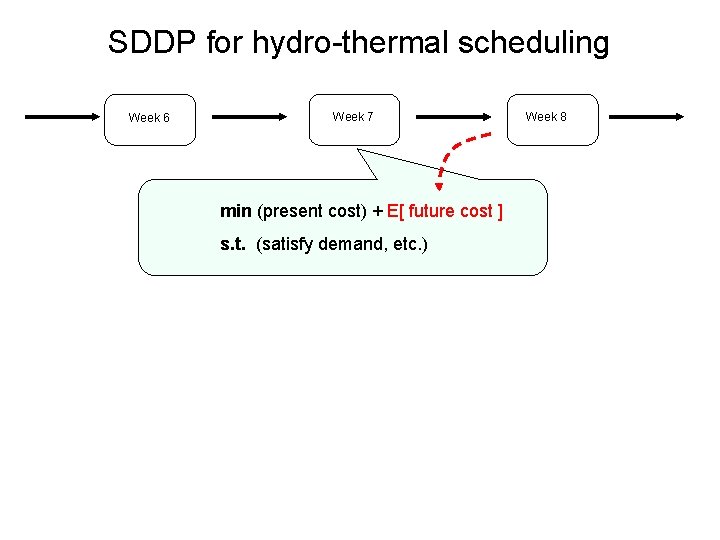
SDDP for hydro-thermal scheduling Week 6 Week 7 min (present cost) + E[ future cost ] s. t. (satisfy demand, etc. ) Week 8
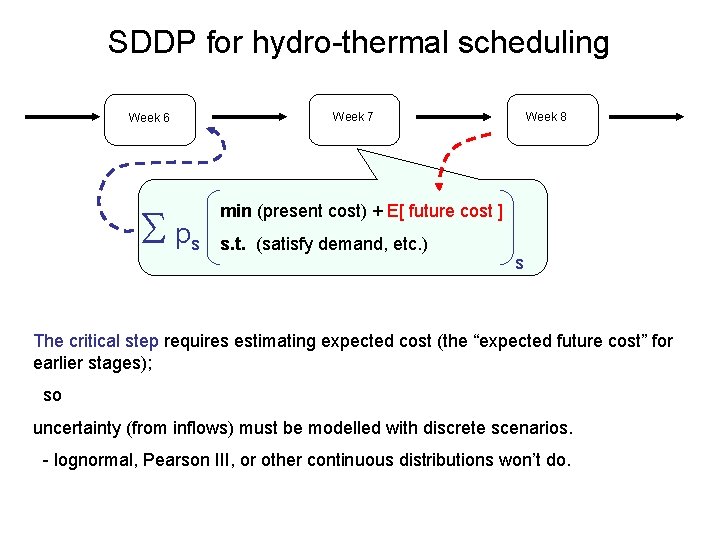
SDDP for hydro-thermal scheduling Week 6 S ps Week 7 Week 8 min (present cost) + E[ future cost ] s. t. (satisfy demand, etc. ) s The critical step requires estimating expected cost (the “expected future cost” for earlier stages); so uncertainty (from inflows) must be modelled with discrete scenarios. - lognormal, Pearson III, or other continuous distributions won’t do.
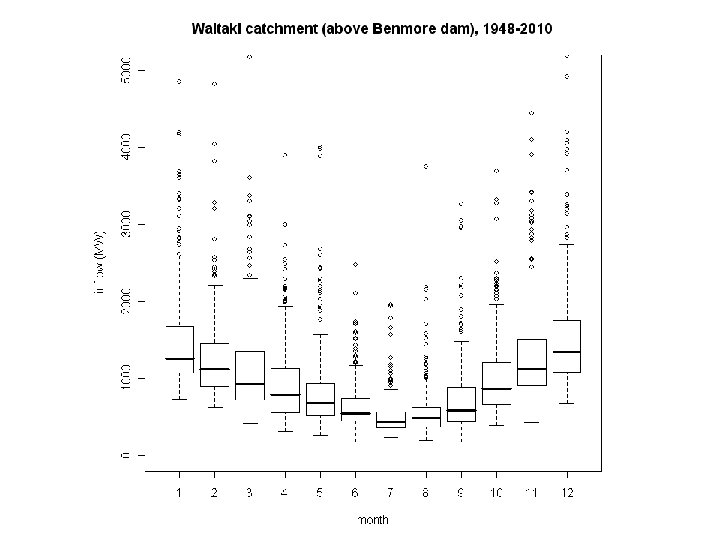
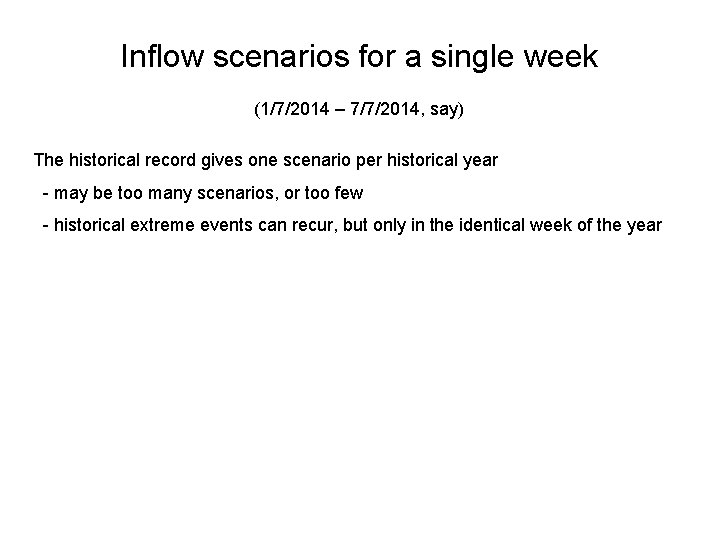
Inflow scenarios for a single week (1/7/2014 – 7/7/2014, say) The historical record gives one scenario per historical year - may be too many scenarios, or too few - historical extreme events can recur, but only in the identical week of the year
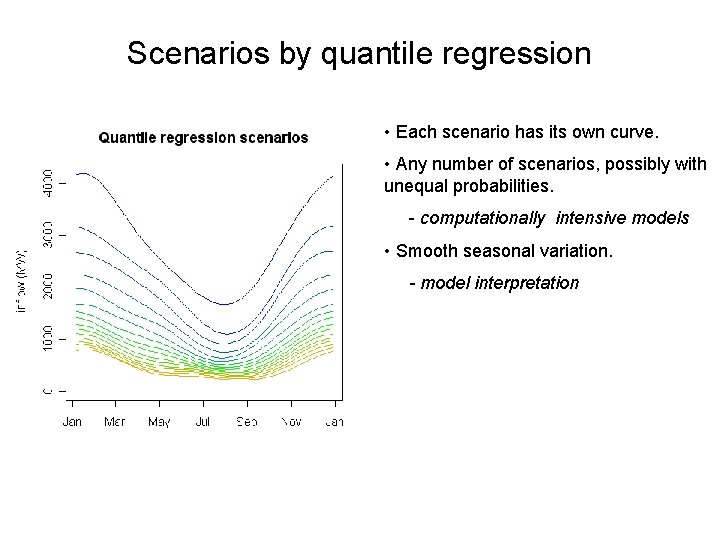
Scenarios by quantile regression • Each scenario has its own curve. • Any number of scenarios, possibly with unequal probabilities. - computationally intensive models • Smooth seasonal variation. - model interpretation
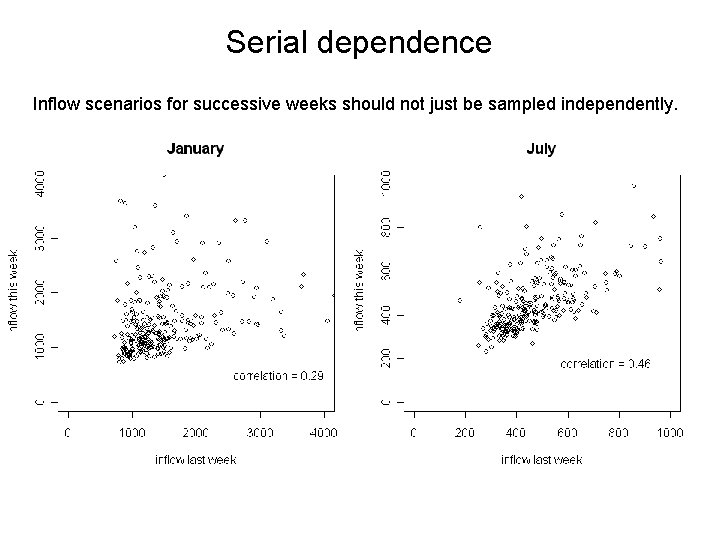
Serial dependence Inflow scenarios for successive weeks should not just be sampled independently.
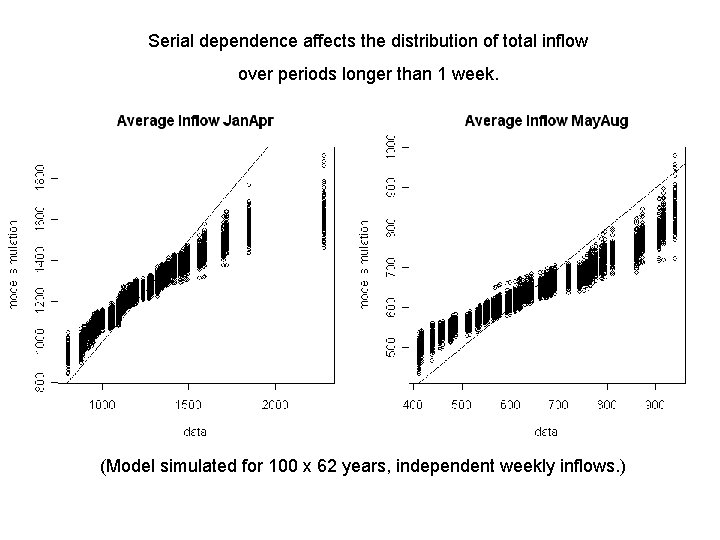
Serial dependence affects the distribution of total inflow over periods longer than 1 week. (Model simulated for 100 x 62 years, independent weekly inflows. )
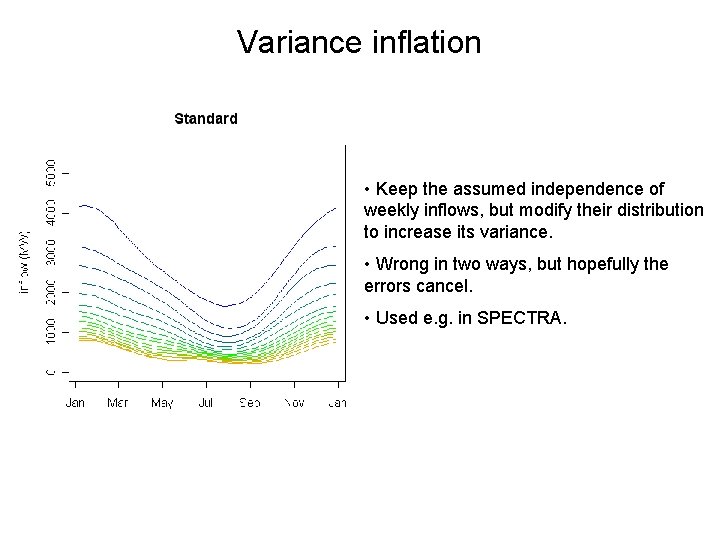
Variance inflation • Keep the assumed independence of weekly inflows, but modify their distribution to increase its variance. • Wrong in two ways, but hopefully the errors cancel. • Used e. g. in SPECTRA.
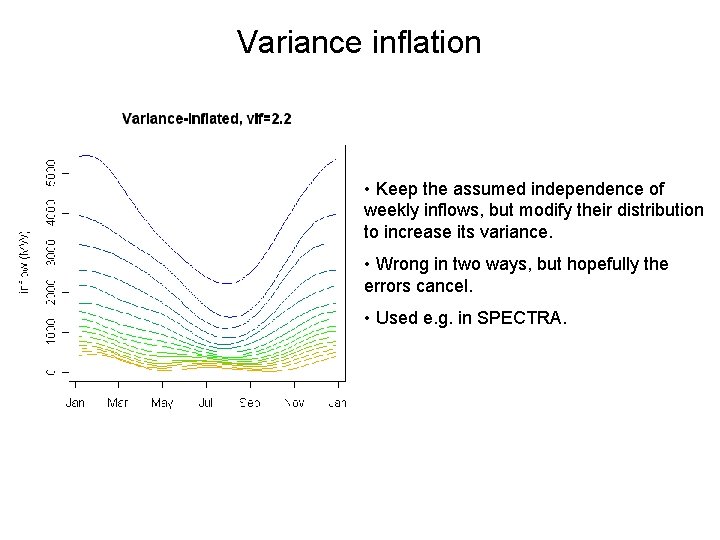
Variance inflation • Keep the assumed independence of weekly inflows, but modify their distribution to increase its variance. • Wrong in two ways, but hopefully the errors cancel. • Used e. g. in SPECTRA.
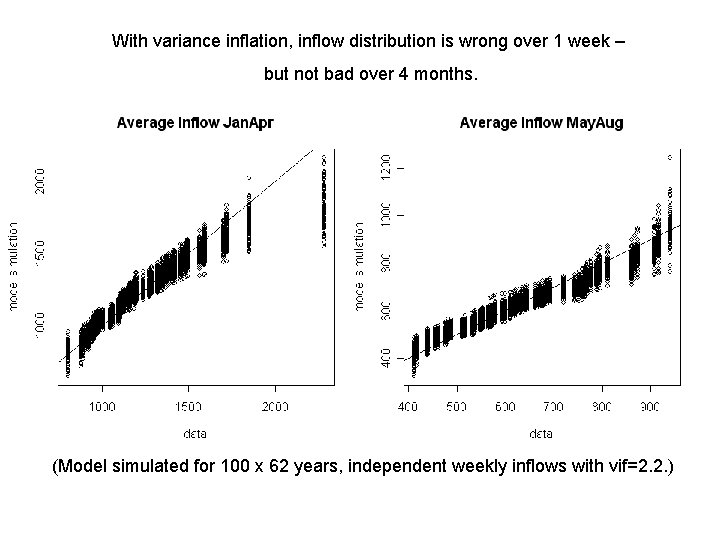
With variance inflation, inflow distribution is wrong over 1 week – but not bad over 4 months. (Model simulated for 100 x 62 years, independent weekly inflows with vif=2. 2. )
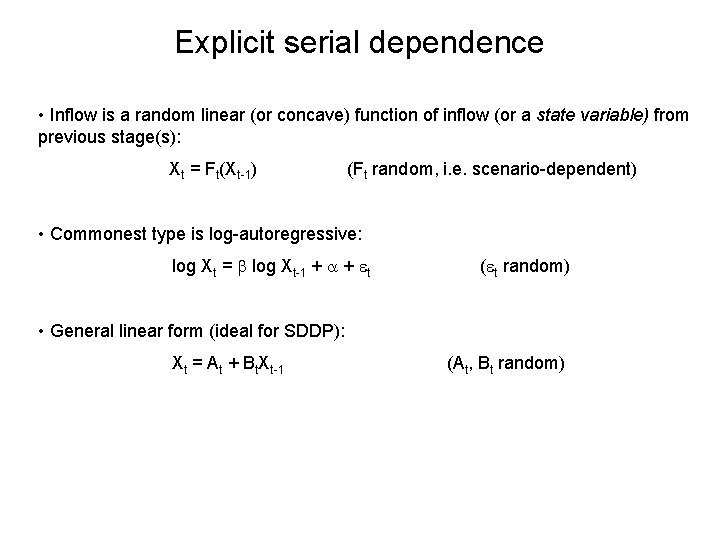
Explicit serial dependence • Inflow is a random linear (or concave) function of inflow (or a state variable) from previous stage(s): Xt = Ft(Xt-1) (Ft random, i. e. scenario-dependent) • Commonest type is log-autoregressive: log Xt = b log Xt-1 + a + et (et random) • General linear form (ideal for SDDP): Xt = At + Bt. Xt-1 (At, Bt random)
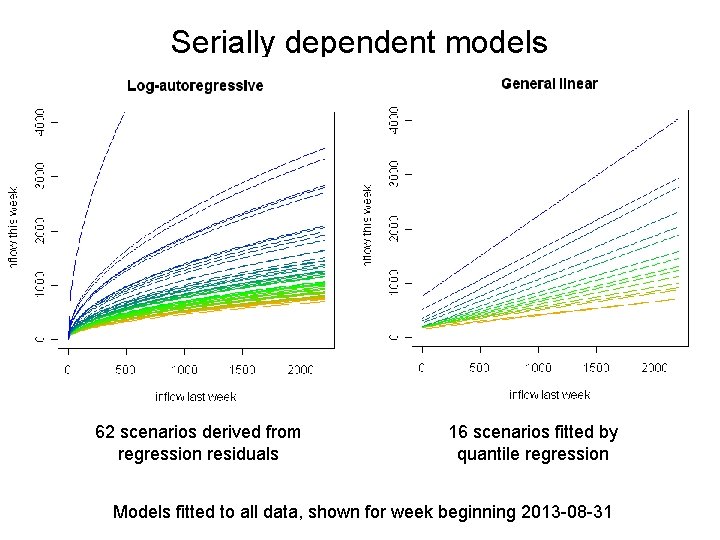
Serially dependent models 62 scenarios derived from regression residuals 16 scenarios fitted by quantile regression Models fitted to all data, shown for week beginning 2013 -08 -31
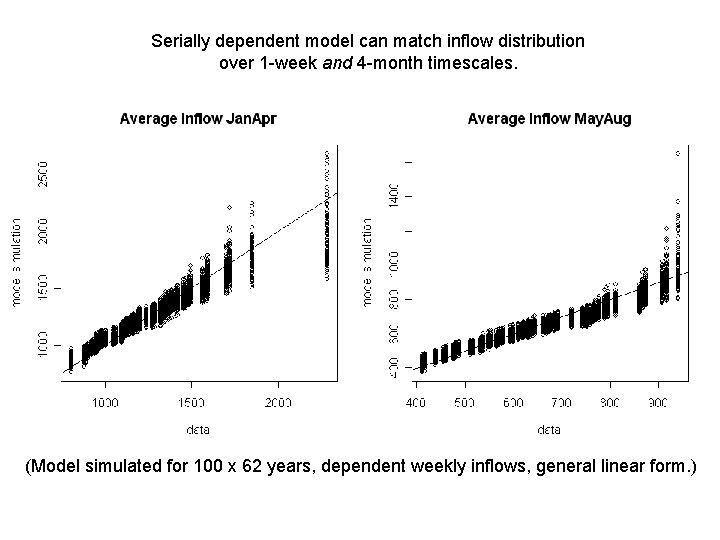
Serially dependent model can match inflow distribution over 1 -week and 4 -month timescales. (Model simulated for 100 x 62 years, dependent weekly inflows, general linear form. )
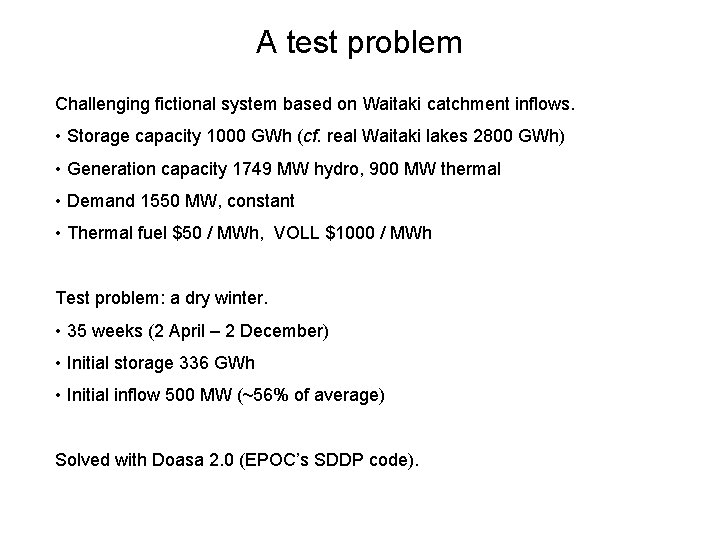
A test problem Challenging fictional system based on Waitaki catchment inflows. • Storage capacity 1000 GWh (cf. real Waitaki lakes 2800 GWh) • Generation capacity 1749 MW hydro, 900 MW thermal • Demand 1550 MW, constant • Thermal fuel $50 / MWh, VOLL $1000 / MWh Test problem: a dry winter. • 35 weeks (2 April – 2 December) • Initial storage 336 GWh • Initial inflow 500 MW (~56% of average) Solved with Doasa 2. 0 (EPOC’s SDDP code).
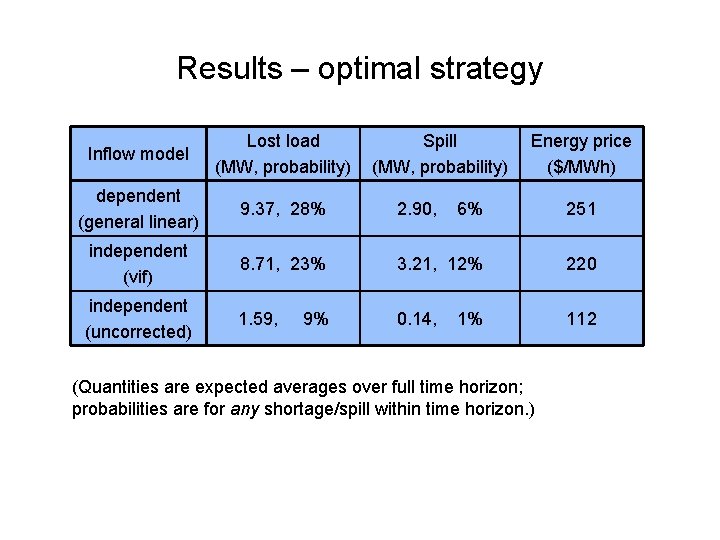
Results – optimal strategy Inflow model Lost load (MW, probability) dependent (general linear) 9. 37, 28% 2. 90, 6% 251 independent (vif) 8. 71, 23% 3. 21, 12% 220 independent (uncorrected) 1. 59, 0. 14, 112 9% Spill (MW, probability) Energy price ($/MWh) 1% (Quantities are expected averages over full time horizon; probabilities are for any shortage/spill within time horizon. )
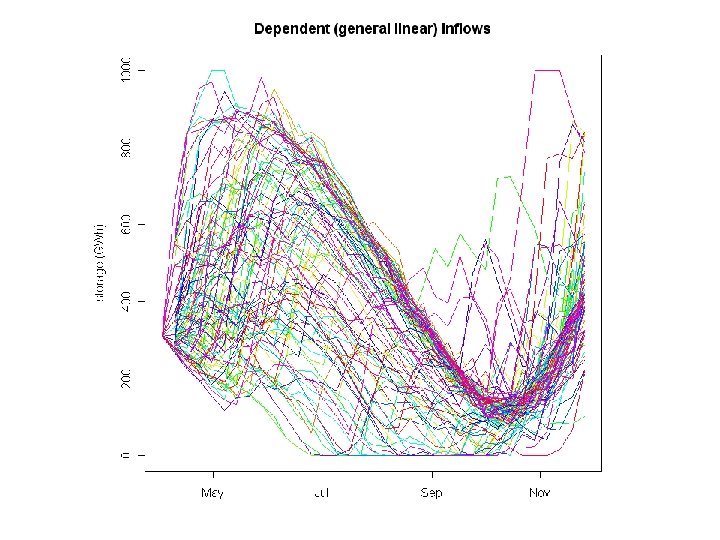
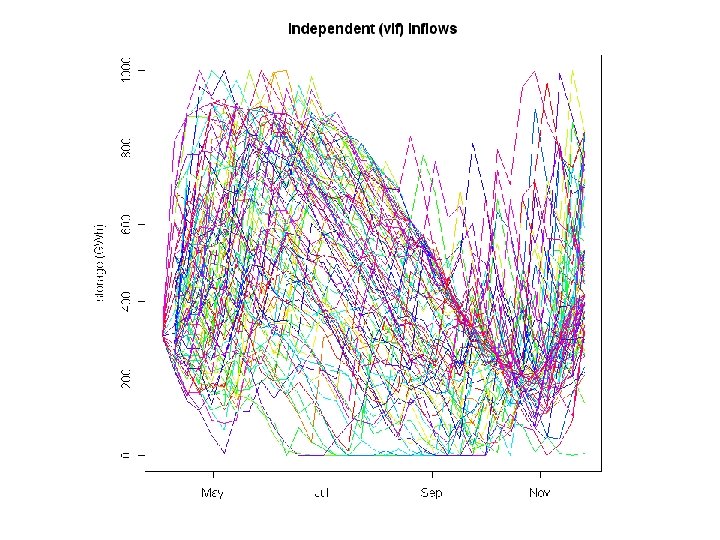
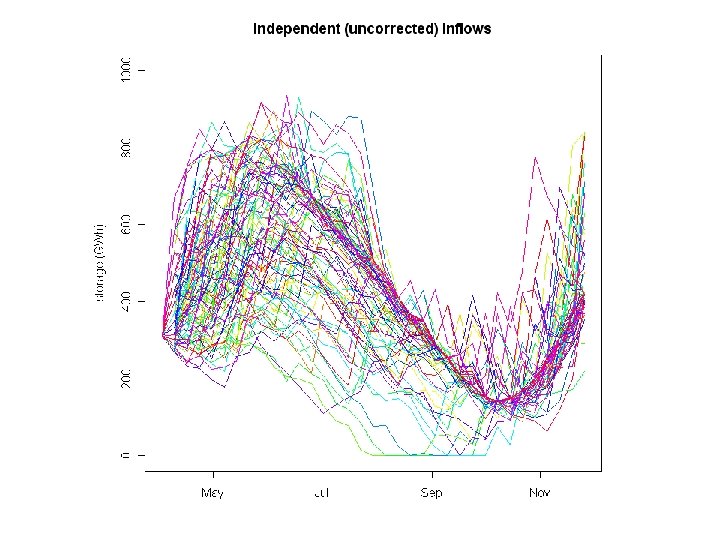
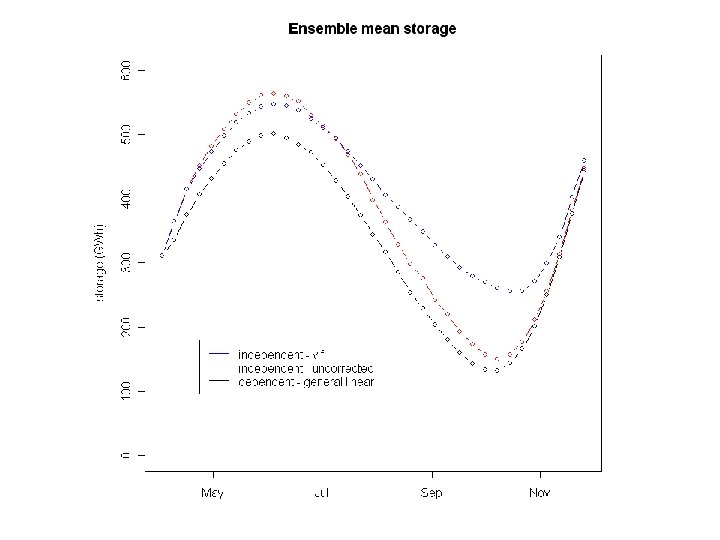
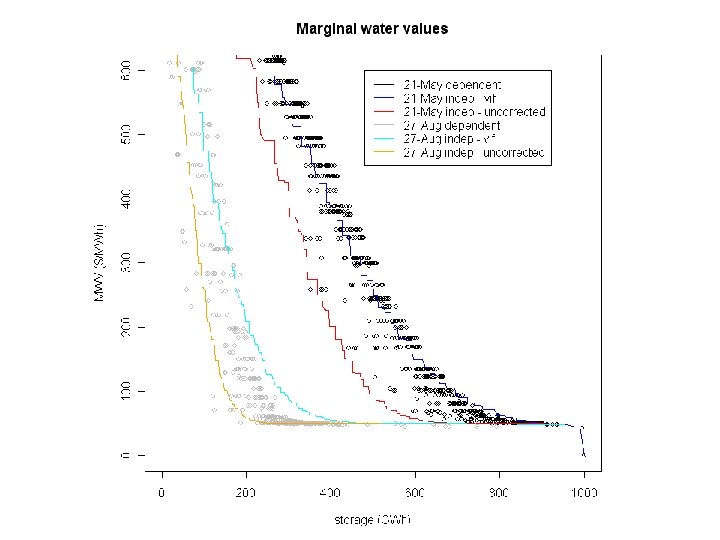
Geoffrey pritchard
Water and water and water water
Fixed income valuation methods
Margaret pritchard houston
Pritchard capital partners
Margaret pritchard houston
Emergncia
Rosalind pritchard
Ivor pritchard
Pritchard regimen
Geoffrey brown nsf
Dr geoffrey cohen
Geoffrey ye li
Geoffrey howe resignation speech youtube
Geoffrey fox
Mixed quantifiers
Leech 1981
Professor geoffrey smith fiu
Sports med udel
Performer heritage geoffrey chaucer
Backpropagation and the brain
Geoffrey brown iu
Prince royce manager
Geoffrey bawa awards
Geoffrey chaucer notes