Methods and Metrics for Cold Start Recommendations Andrew
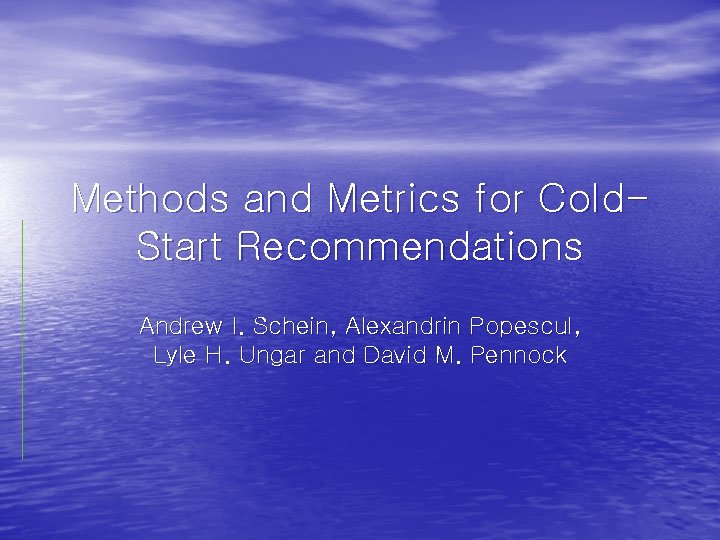
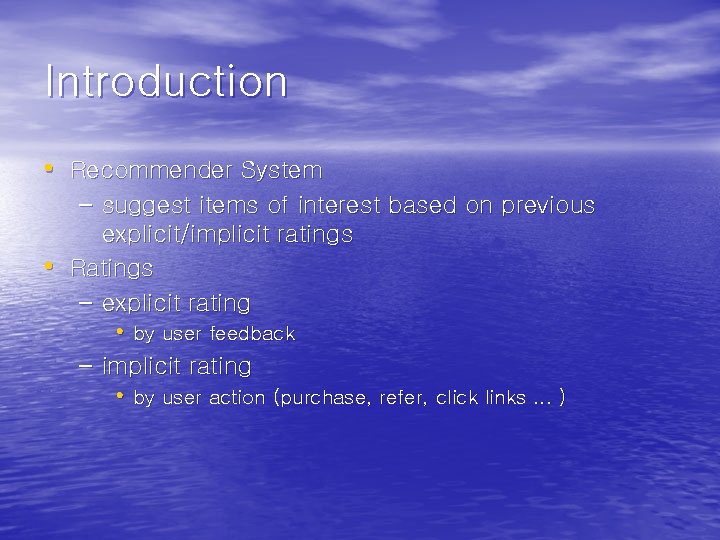
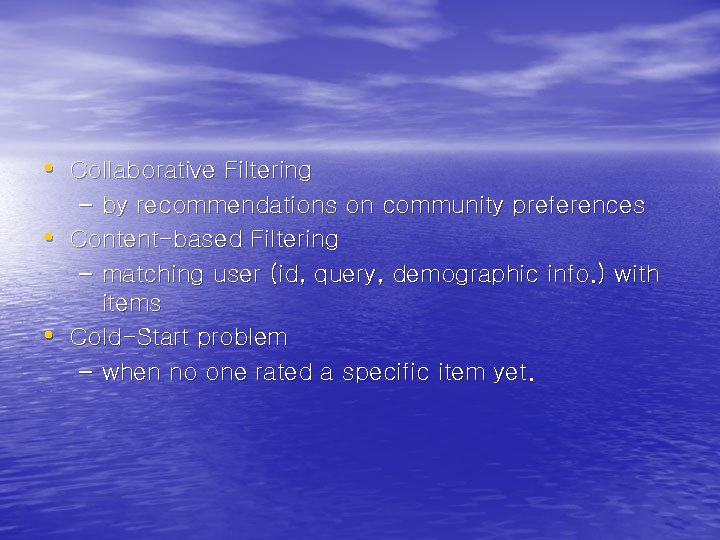
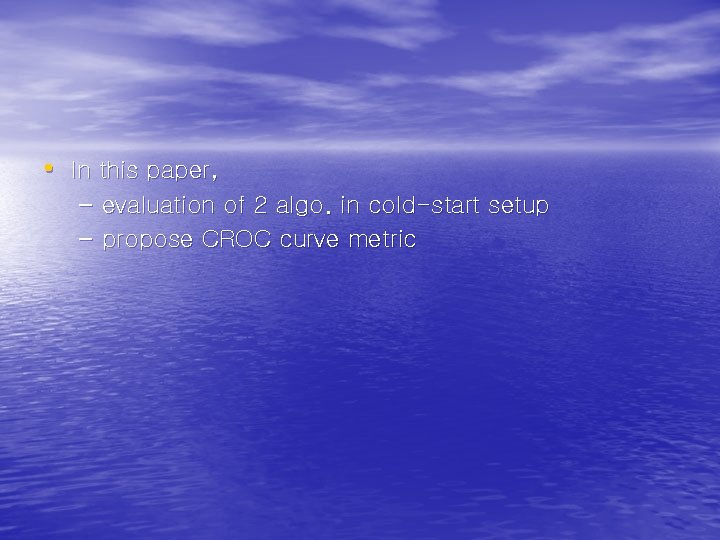
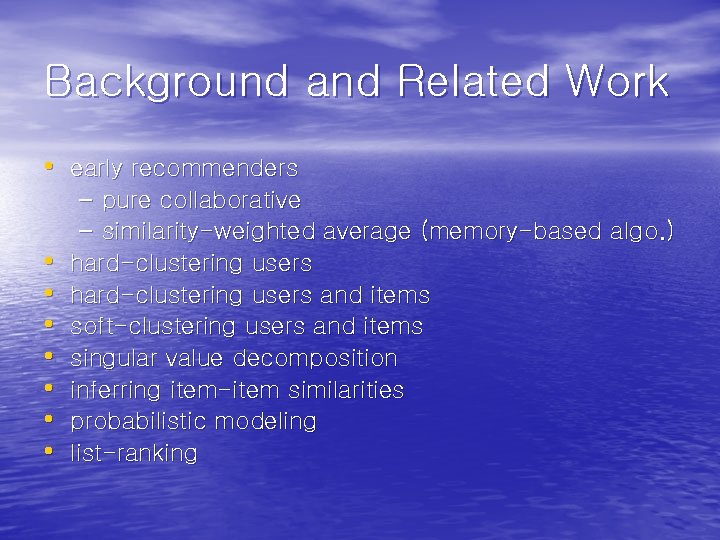
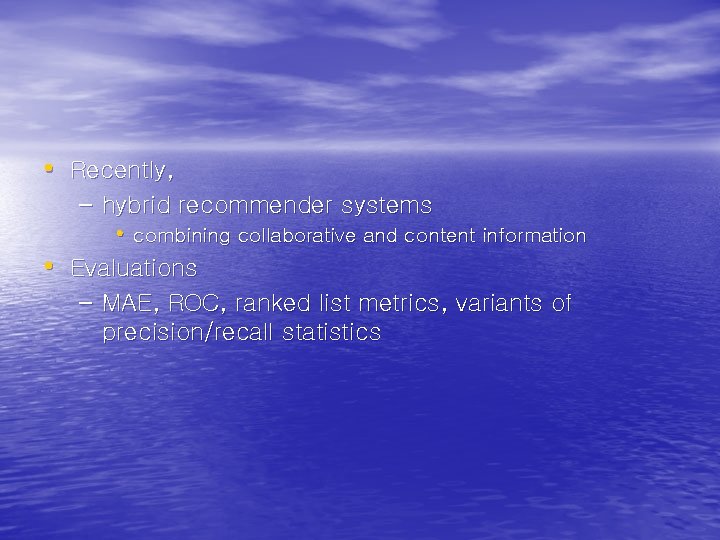
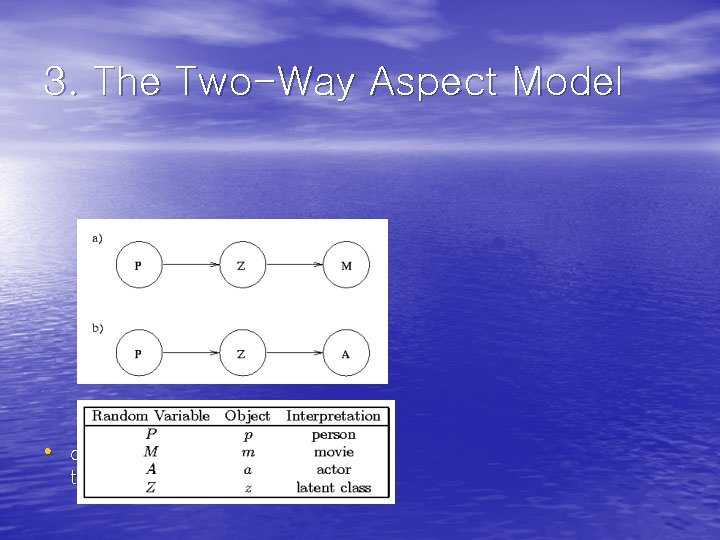
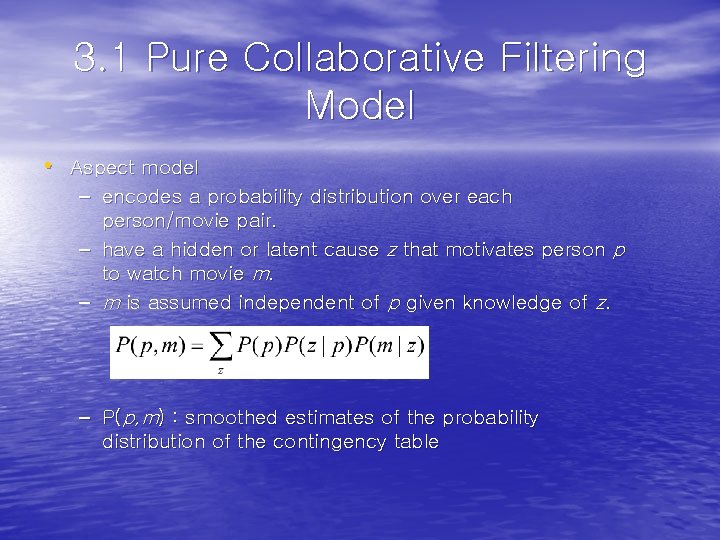
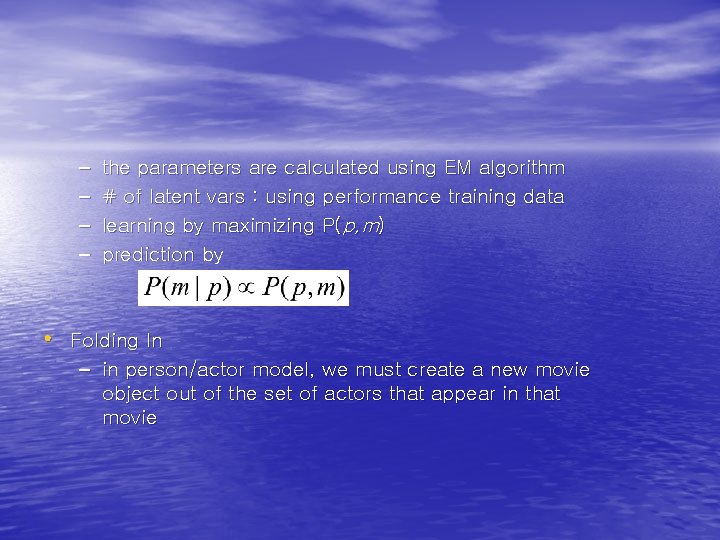
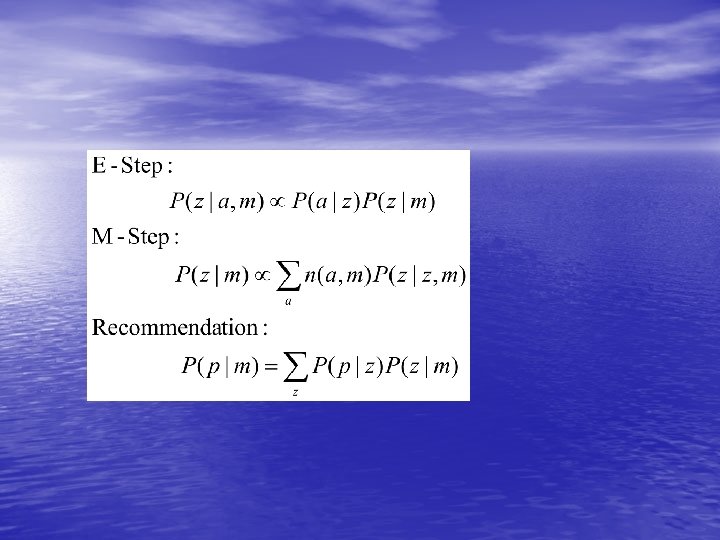
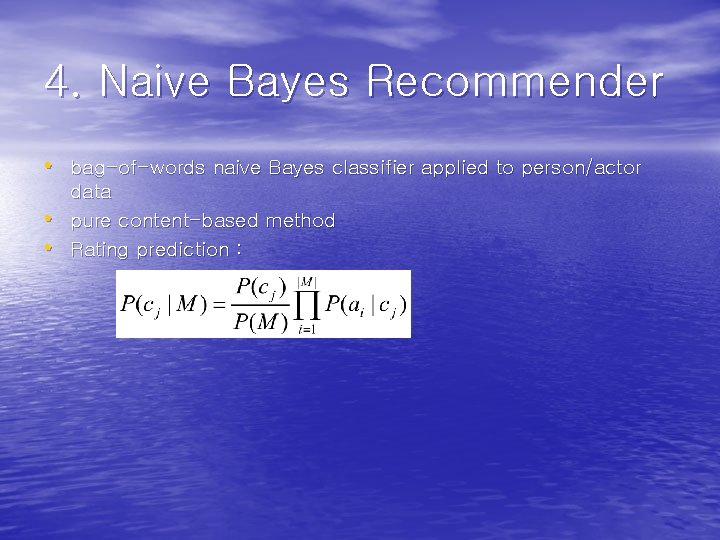
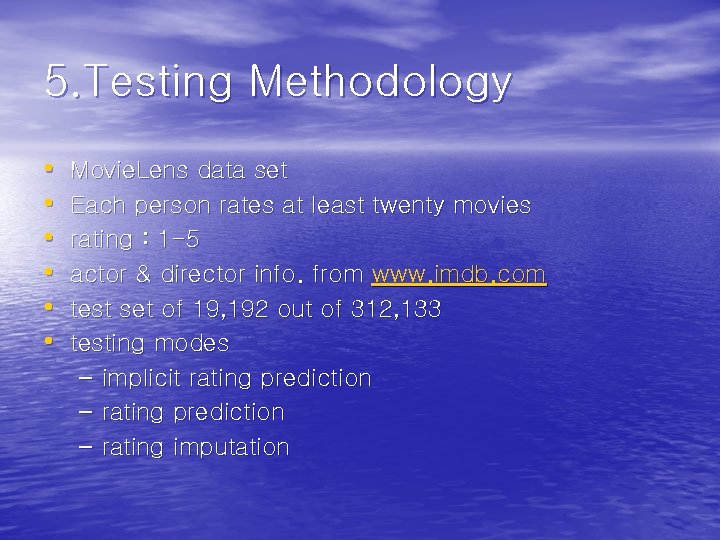
![6. Evaluation Metrics • Receiver Operator Characteristic (ROC) curve [Herlocker] – showing hit/miss rates 6. Evaluation Metrics • Receiver Operator Characteristic (ROC) curve [Herlocker] – showing hit/miss rates](https://slidetodoc.com/presentation_image_h/04c2bb20a1aeb8bcb07c457fadbba8eb/image-13.jpg)
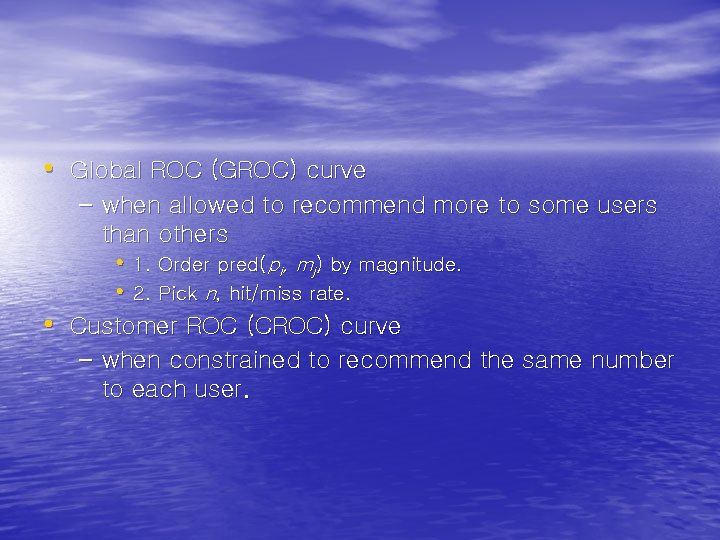
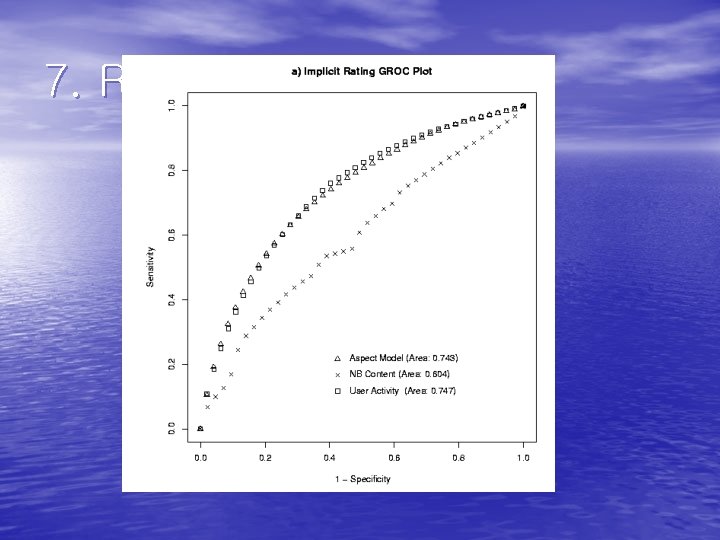
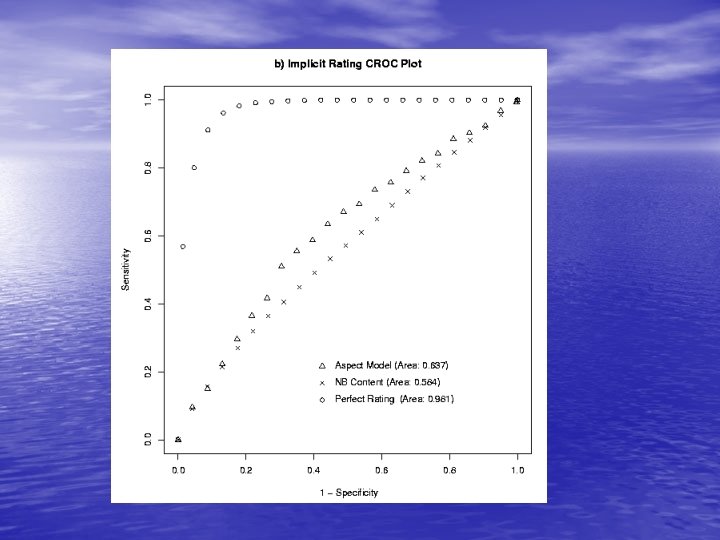
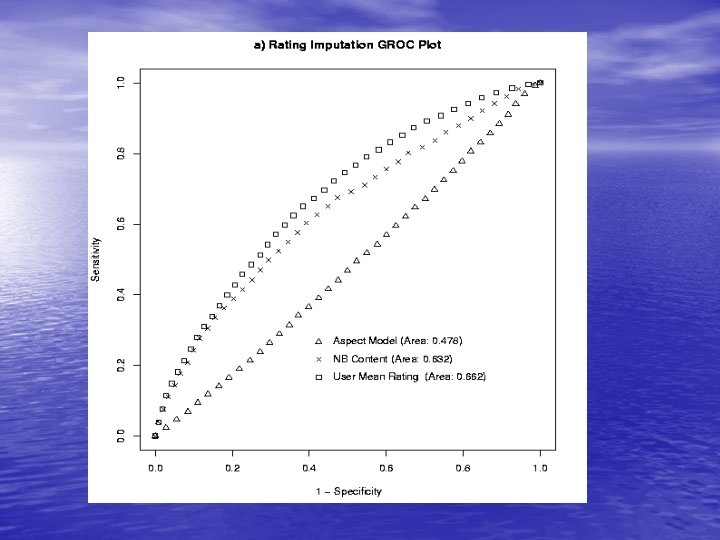
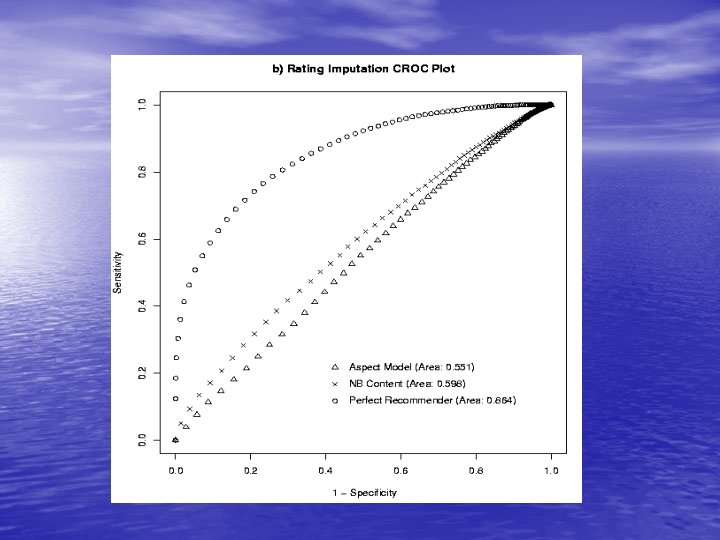
- Slides: 18
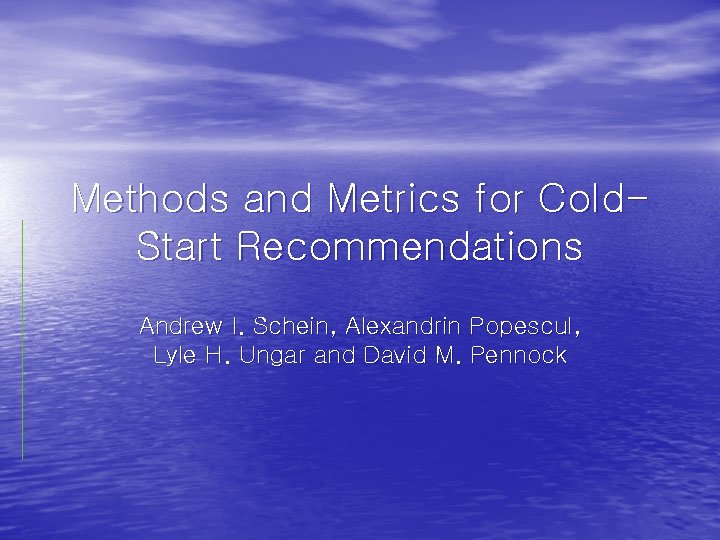
Methods and Metrics for Cold. Start Recommendations Andrew I. Schein, Alexandrin Popescul, Lyle H. Ungar and David M. Pennock
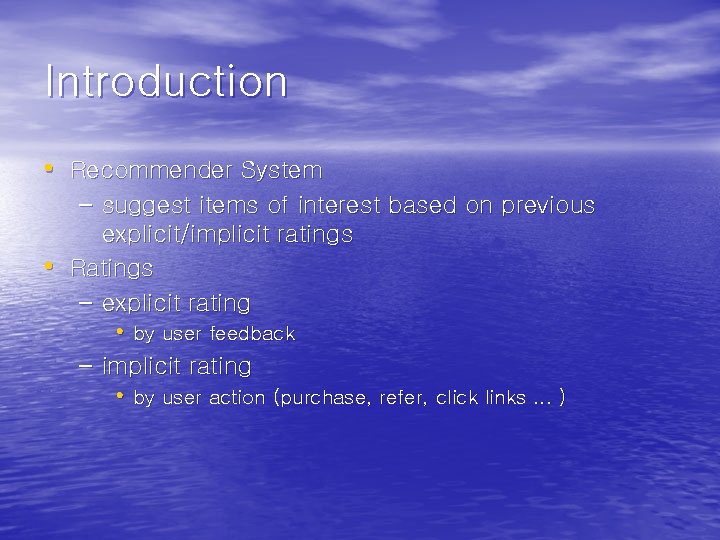
Introduction • Recommender System – suggest items of interest based on previous explicit/implicit ratings • Ratings – explicit rating • by user feedback – implicit rating • by user action (purchase, refer, click links. . . )
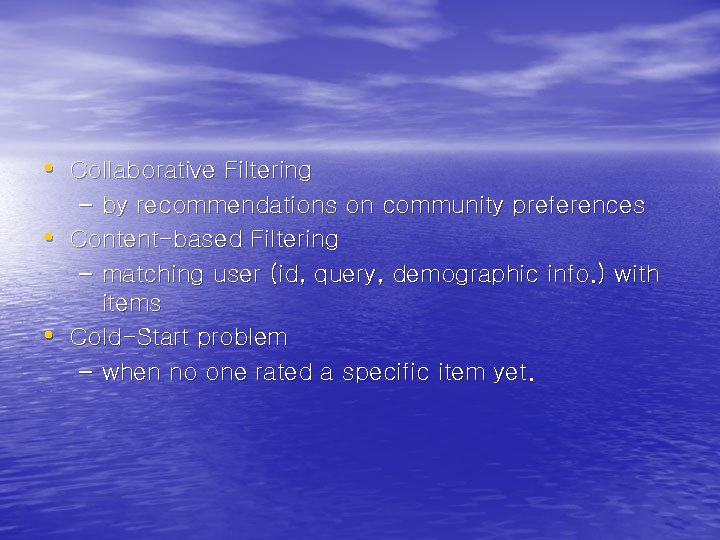
• Collaborative Filtering – by recommendations on community preferences • Content-based Filtering – matching user (id, query, demographic info. ) with items • Cold-Start problem – when no one rated a specific item yet.
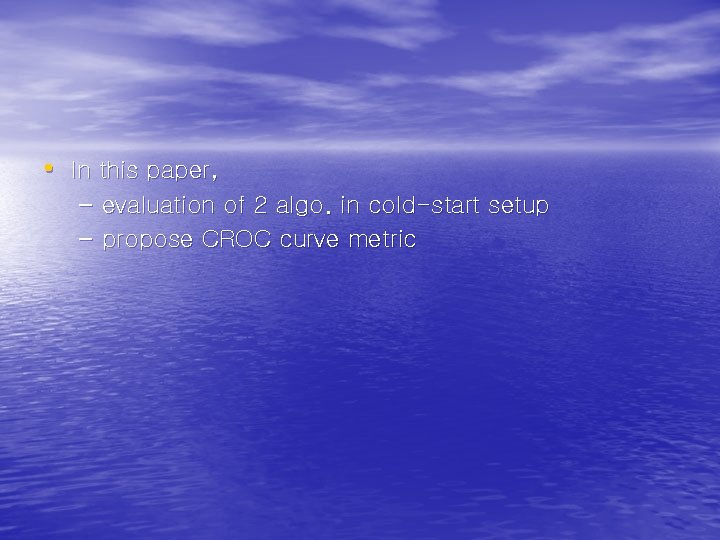
• In this paper, – evaluation of 2 algo. in cold-start setup – propose CROC curve metric
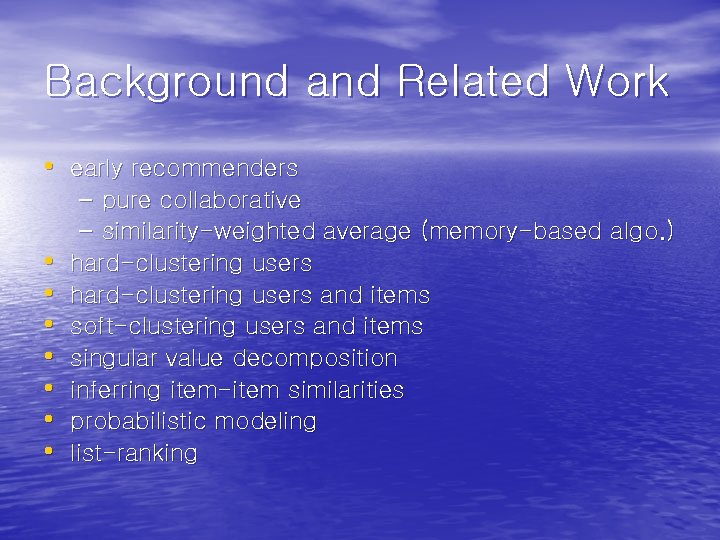
Background and Related Work • early recommenders • • – pure collaborative – similarity-weighted average (memory-based algo. ) hard-clustering users and items soft-clustering users and items singular value decomposition inferring item-item similarities probabilistic modeling list-ranking
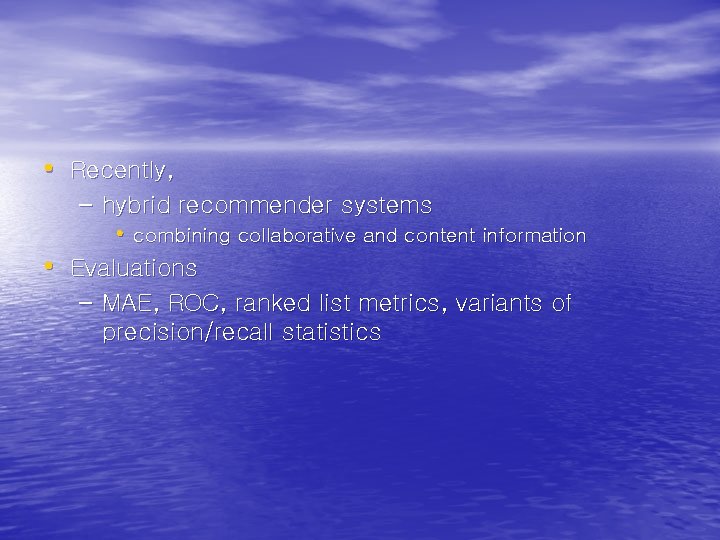
• Recently, – hybrid recommender systems • combining collaborative and content information • Evaluations – MAE, ROC, ranked list metrics, variants of precision/recall statistics
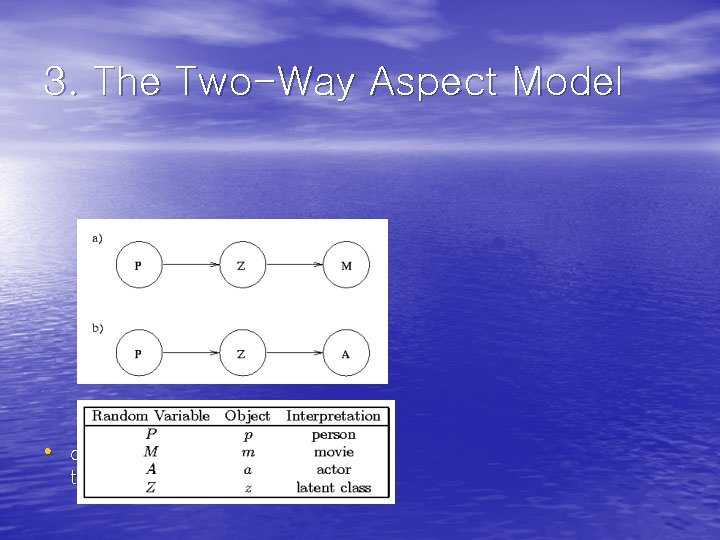
3. The Two-Way Aspect Model • designed for contingency table smoothing
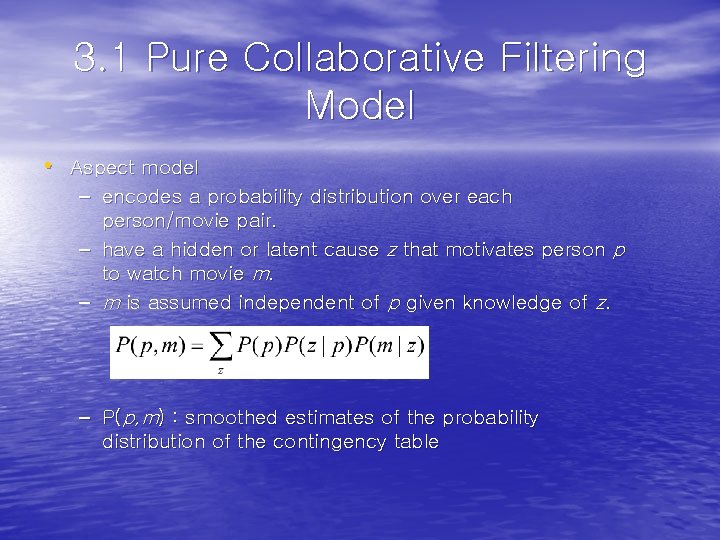
3. 1 Pure Collaborative Filtering Model • Aspect model – encodes a probability distribution over each person/movie pair. – have a hidden or latent cause z that motivates person p to watch movie m. – m is assumed independent of p given knowledge of z. – P(p, m) : smoothed estimates of the probability distribution of the contingency table
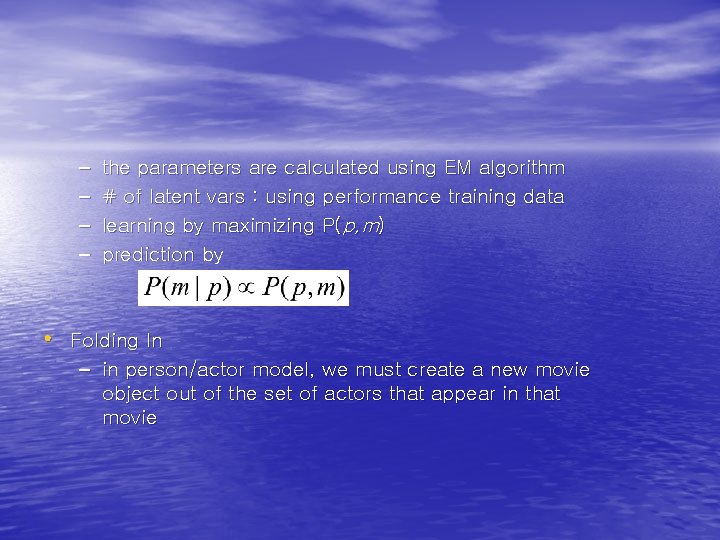
– – the parameters are calculated using EM algorithm # of latent vars : using performance training data learning by maximizing P(p, m) prediction by • Folding In – in person/actor model, we must create a new movie object out of the set of actors that appear in that movie
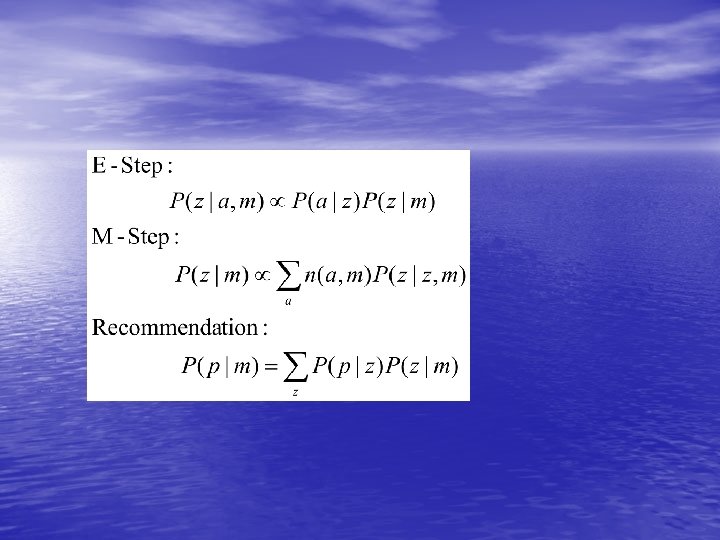
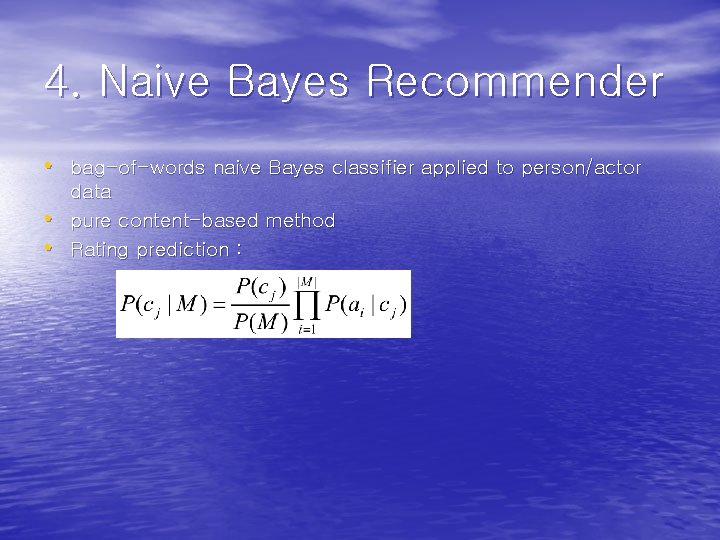
4. Naive Bayes Recommender • bag-of-words naive Bayes classifier applied to person/actor • • data pure content-based method Rating prediction :
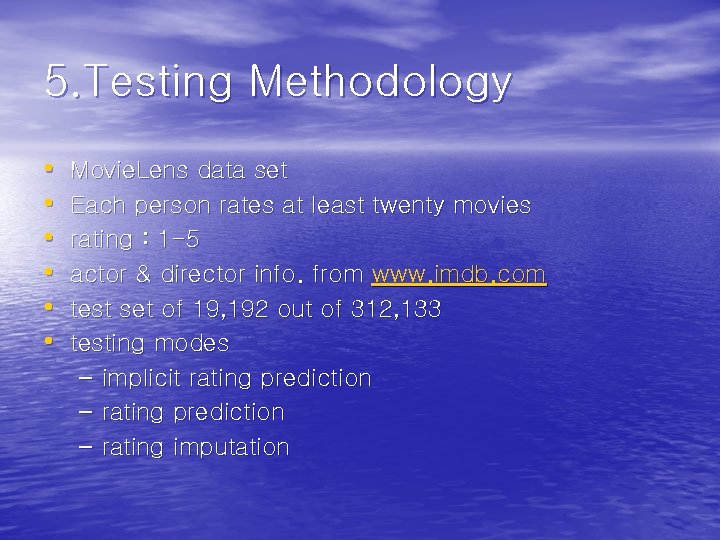
5. Testing Methodology • • • Movie. Lens data set Each person rates at least twenty movies rating : 1 -5 actor & director info. from www. imdb. com test set of 19, 192 out of 312, 133 testing modes – implicit rating prediction – rating imputation
![6 Evaluation Metrics Receiver Operator Characteristic ROC curve Herlocker showing hitmiss rates 6. Evaluation Metrics • Receiver Operator Characteristic (ROC) curve [Herlocker] – showing hit/miss rates](https://slidetodoc.com/presentation_image_h/04c2bb20a1aeb8bcb07c457fadbba8eb/image-13.jpg)
6. Evaluation Metrics • Receiver Operator Characteristic (ROC) curve [Herlocker] – showing hit/miss rates for different classification thresholds – sensitivity (hit rate) • percentage of all positive values found above some threshold – 1 -specificity (miss rate) • the fraction of all negative values found above some threshold
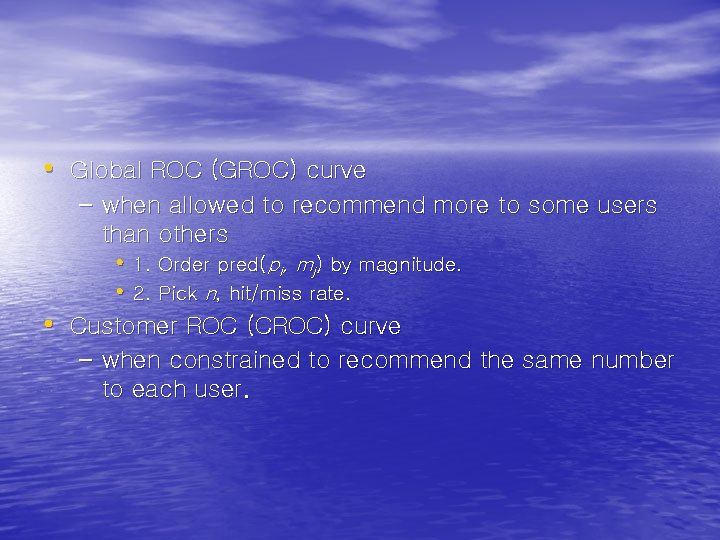
• Global ROC (GROC) curve – when allowed to recommend more to some users than others • 1. Order pred(pi, mj) by magnitude. • 2. Pick n, hit/miss rate. • Customer ROC (CROC) curve – when constrained to recommend the same number to each user.
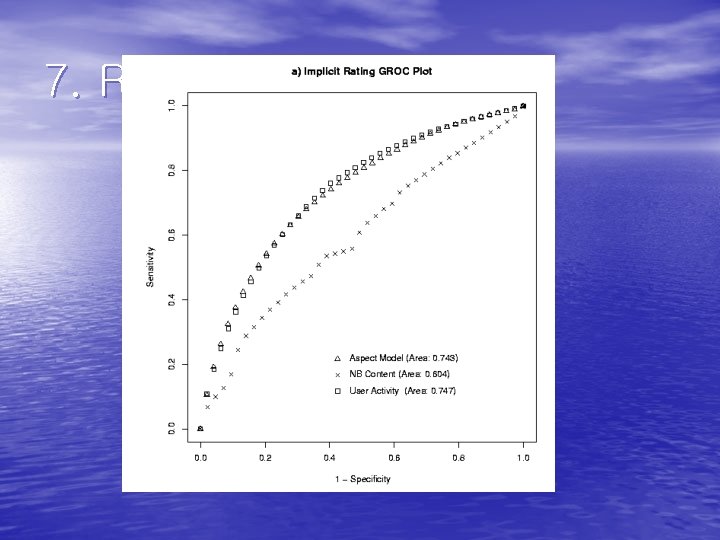
7. Results
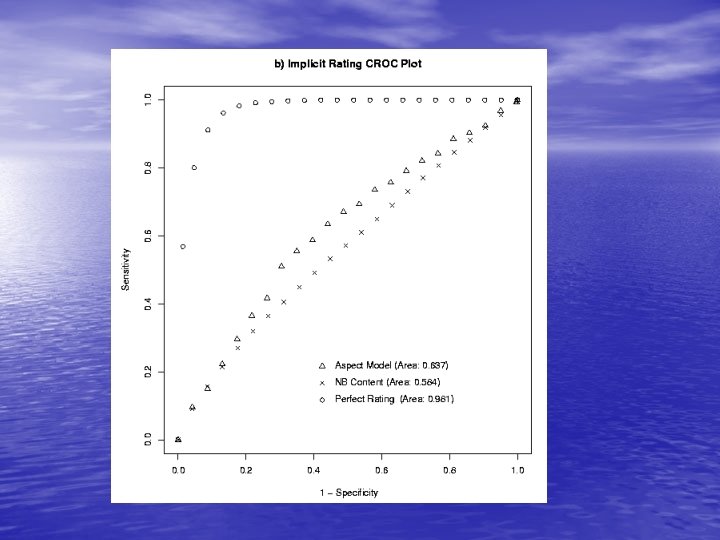
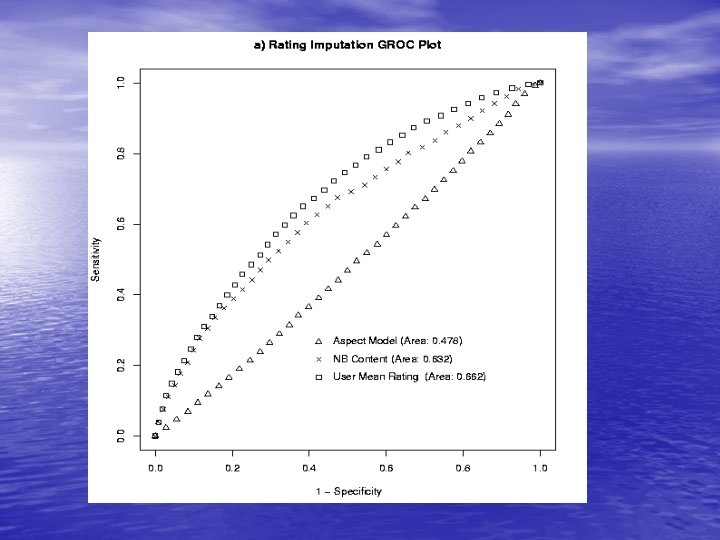
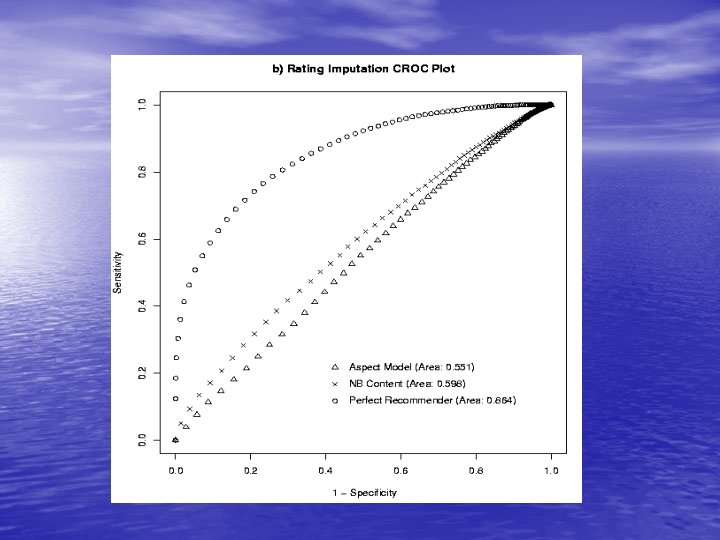
Methods and metrics for cold-start recommendations
Karttecken brant
But we gotta start somewhere
Jumpstart pediatric triage
Cold start
The cold war lesson 1
Wax pattern fabrication pdf
Ipcr comments
Giving recommendations and opinions
Asking and giving recommendations
Making suggestions and recommendations
Concluding sentence for lamb to the slaughter
Fspos
Novell typiska drag
Nationell inriktning för artificiell intelligens
Returpilarna
Varför kallas perioden 1918-1939 för mellankrigstiden
En lathund för arbete med kontinuitetshantering
Underlag för särskild löneskatt på pensionskostnader