Intro to SEM images z SEM Scanning electron
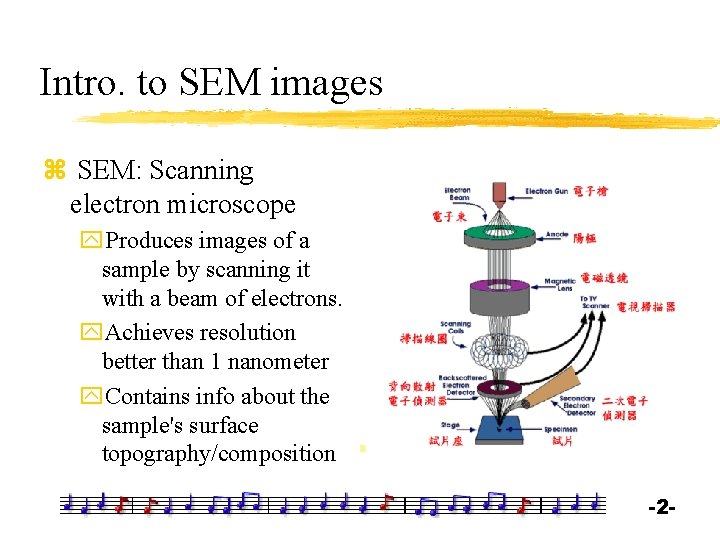
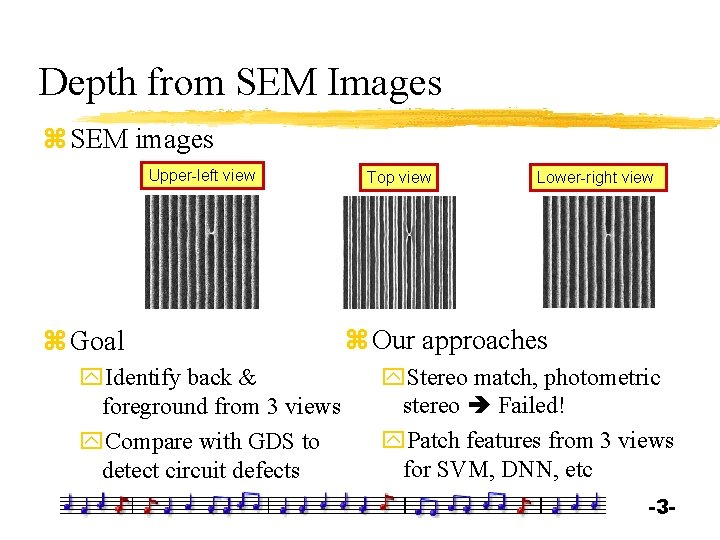
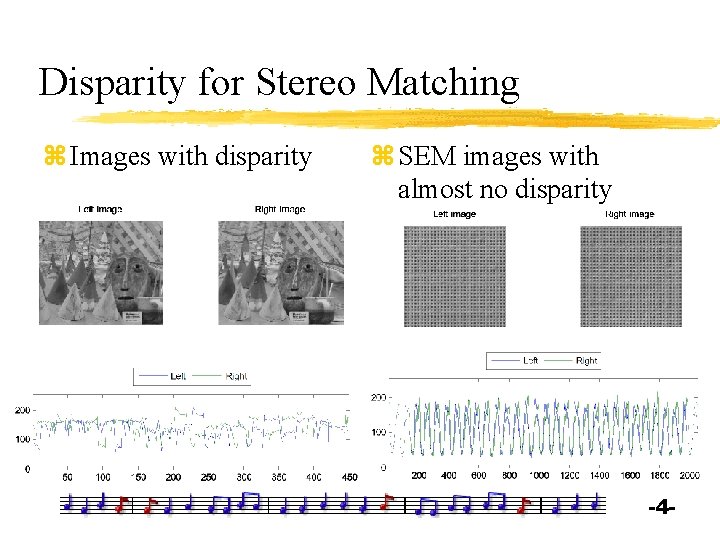
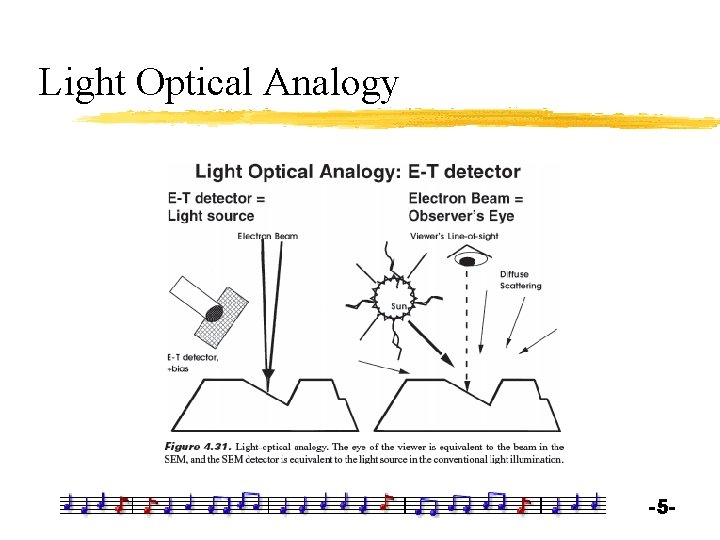
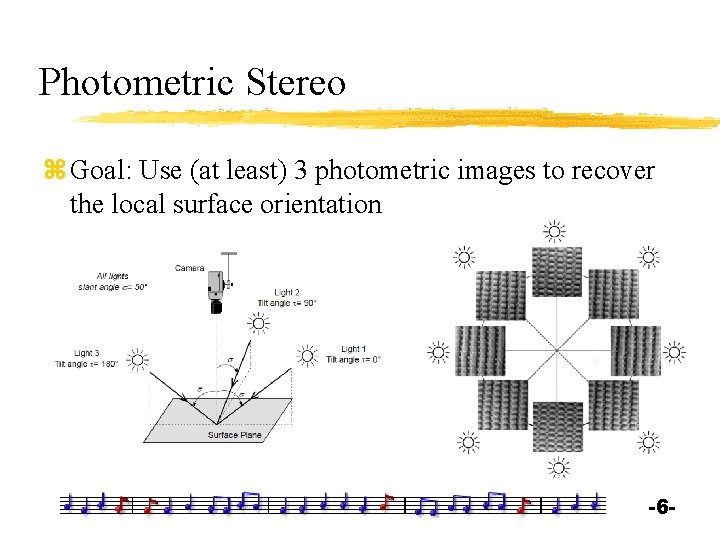
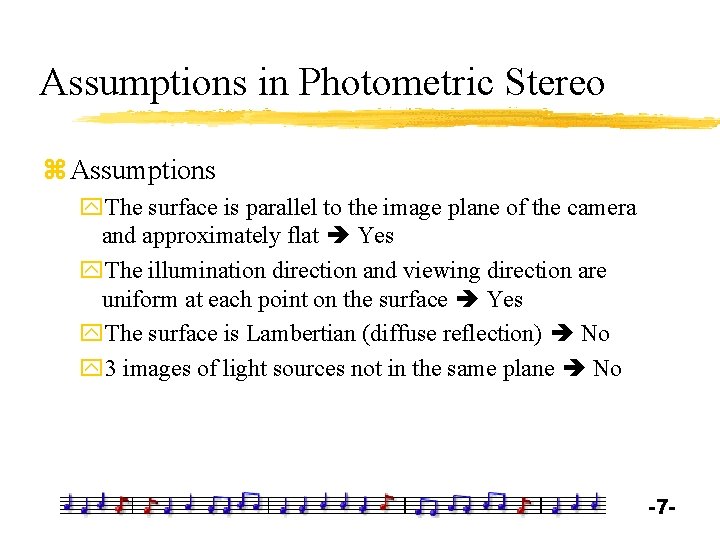
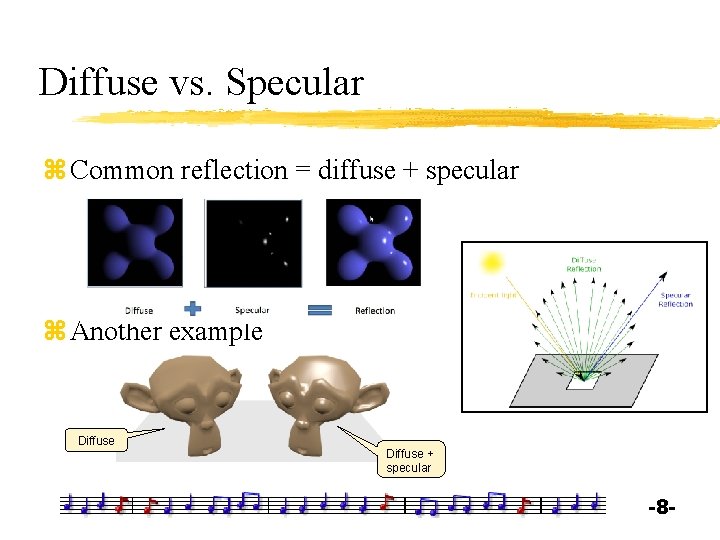
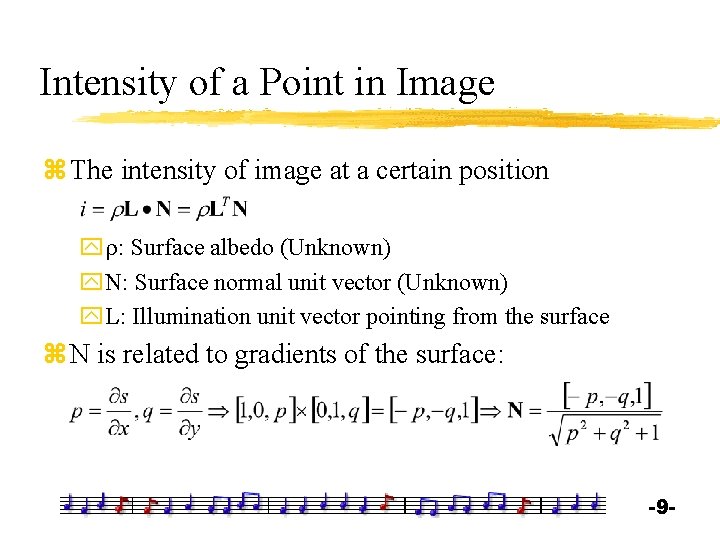
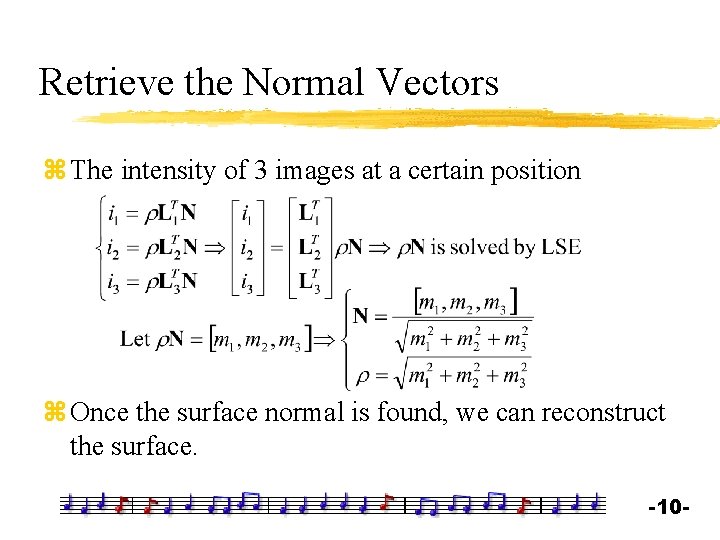
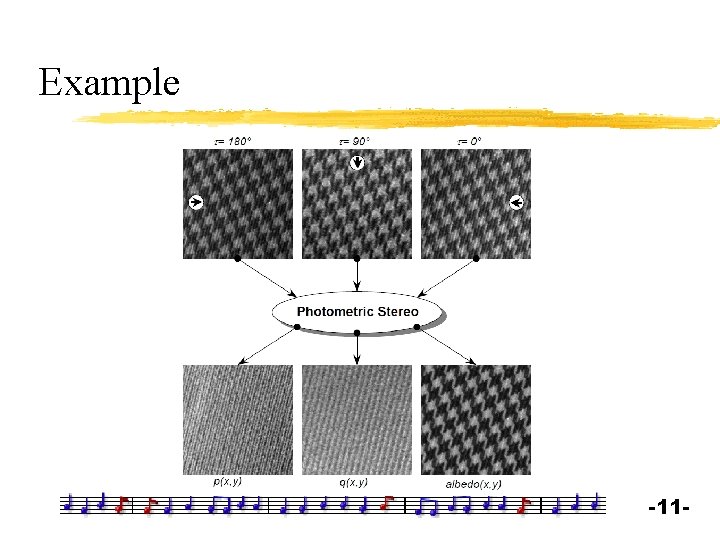
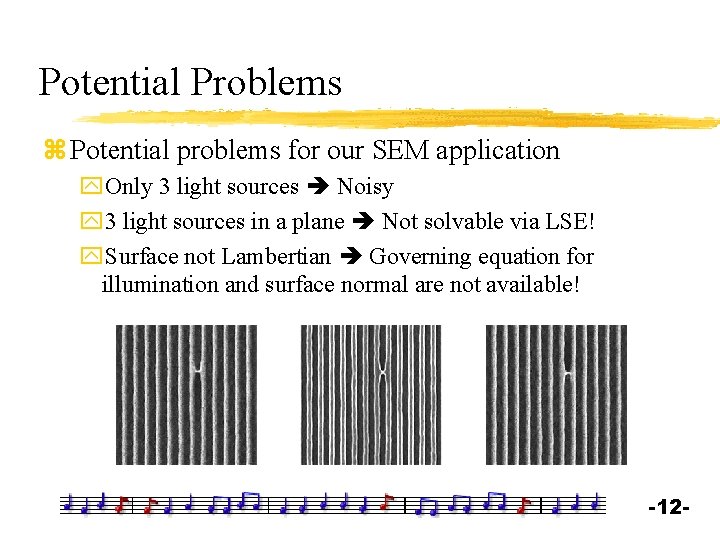
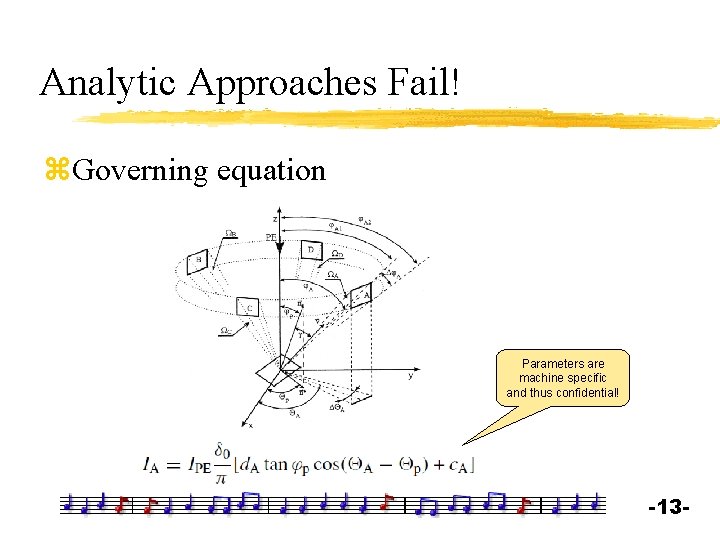
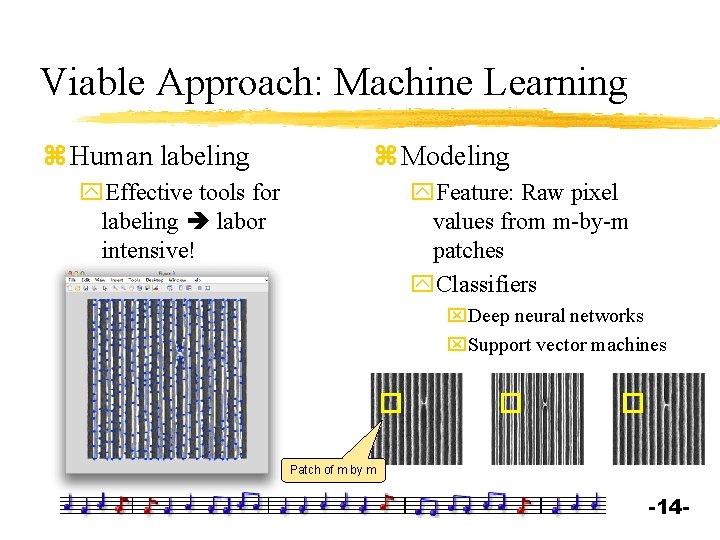
- Slides: 13
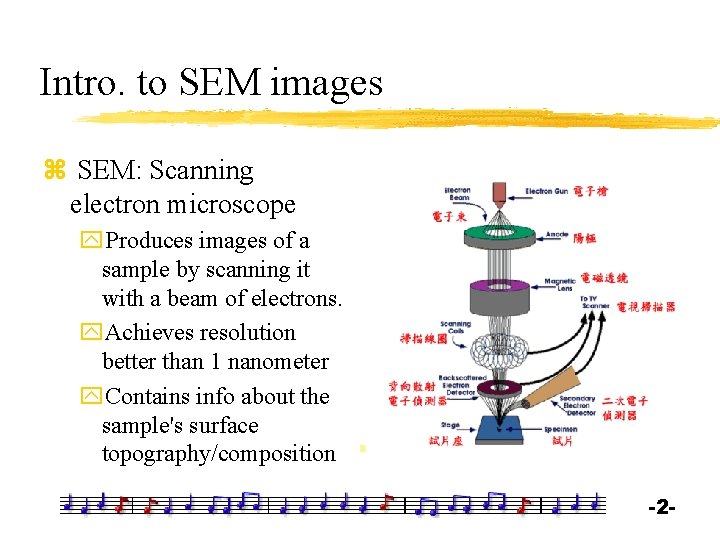
Intro. to SEM images z SEM: Scanning electron microscope y. Produces images of a sample by scanning it with a beam of electrons. y. Achieves resolution better than 1 nanometer y. Contains info about the sample's surface topography/composition -2 -
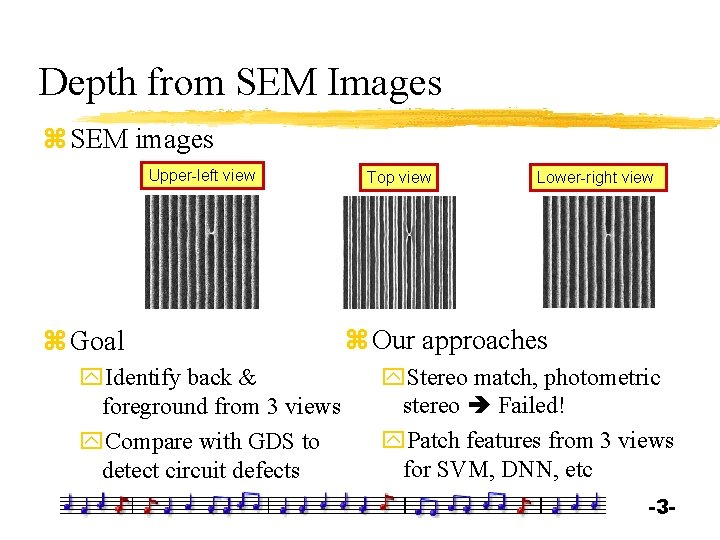
Depth from SEM Images z SEM images Upper-left view z Goal y. Identify back & foreground from 3 views y. Compare with GDS to detect circuit defects Top view Lower-right view z Our approaches y. Stereo match, photometric stereo Failed! y. Patch features from 3 views for SVM, DNN, etc -3 -
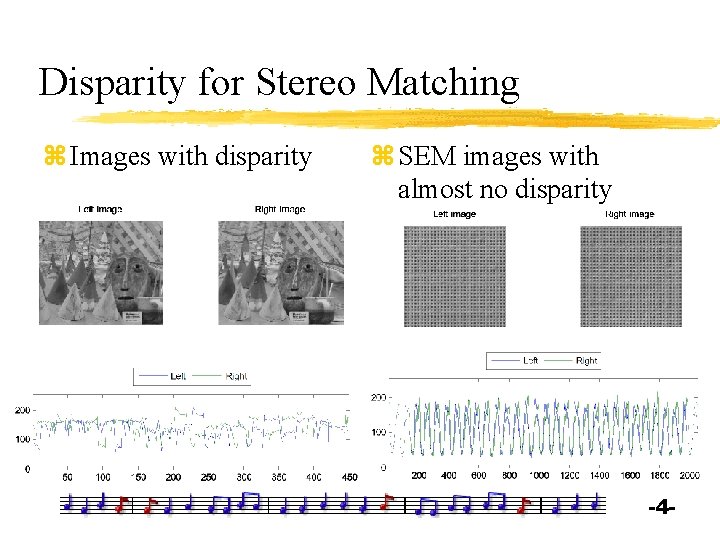
Disparity for Stereo Matching z Images with disparity z SEM images with almost no disparity -4 -
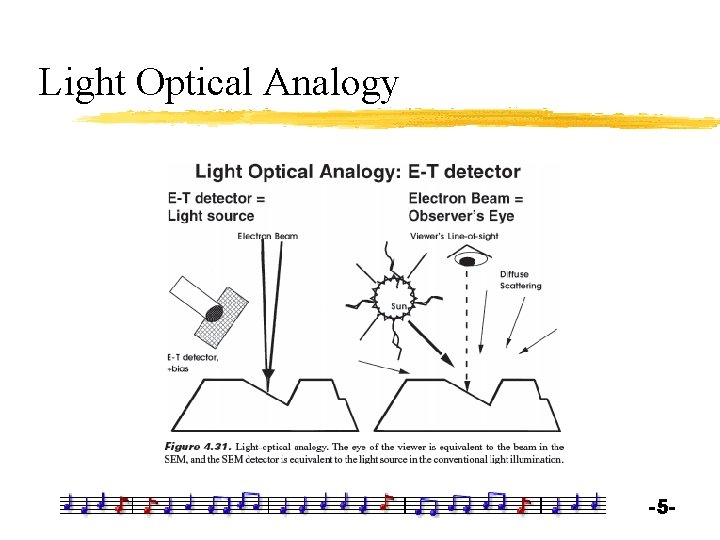
Light Optical Analogy -5 -
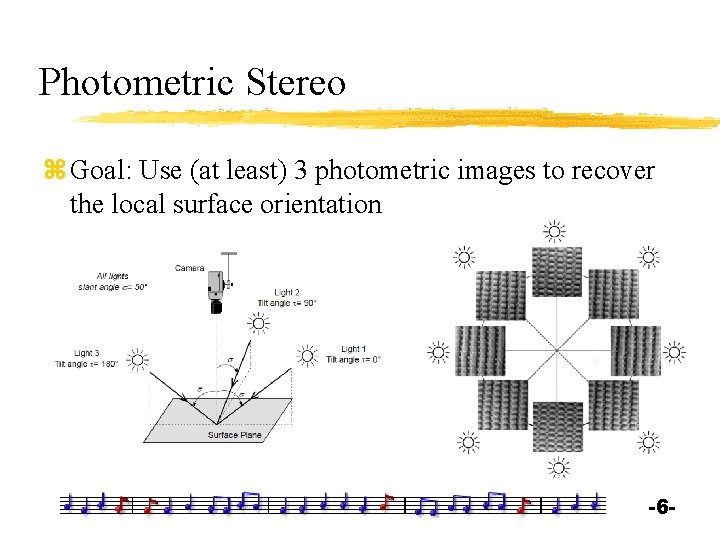
Photometric Stereo z Goal: Use (at least) 3 photometric images to recover the local surface orientation -6 -
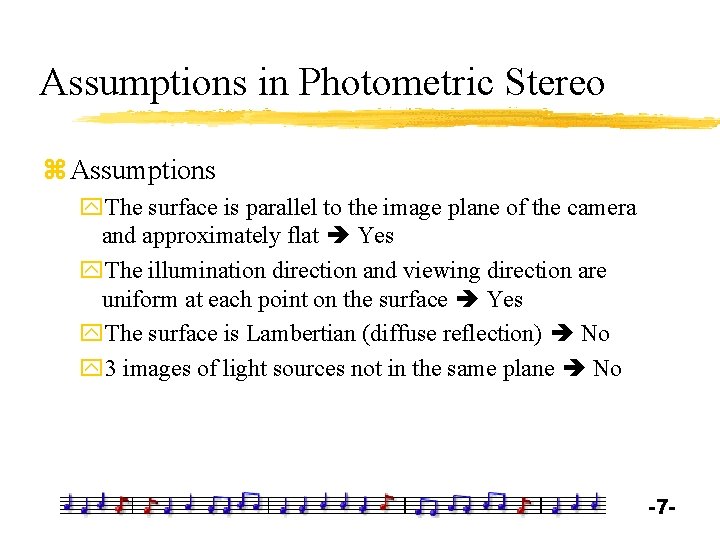
Assumptions in Photometric Stereo z Assumptions y. The surface is parallel to the image plane of the camera and approximately flat Yes y. The illumination direction and viewing direction are uniform at each point on the surface Yes y. The surface is Lambertian (diffuse reflection) No y 3 images of light sources not in the same plane No -7 -
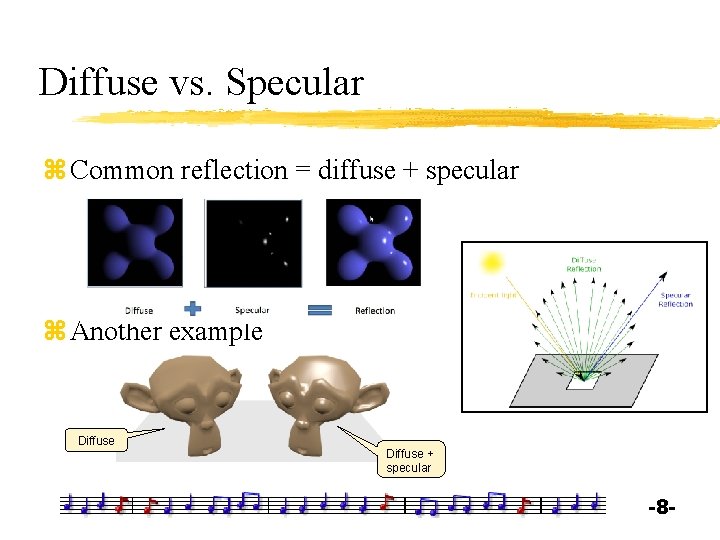
Diffuse vs. Specular z Common reflection = diffuse + specular z Another example Diffuse + specular -8 -
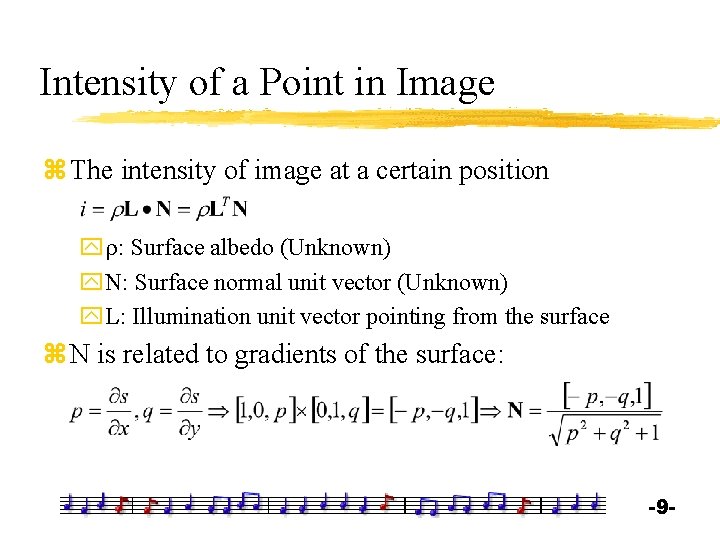
Intensity of a Point in Image z The intensity of image at a certain position yr: Surface albedo (Unknown) y. N: Surface normal unit vector (Unknown) y. L: Illumination unit vector pointing from the surface z N is related to gradients of the surface: -9 -
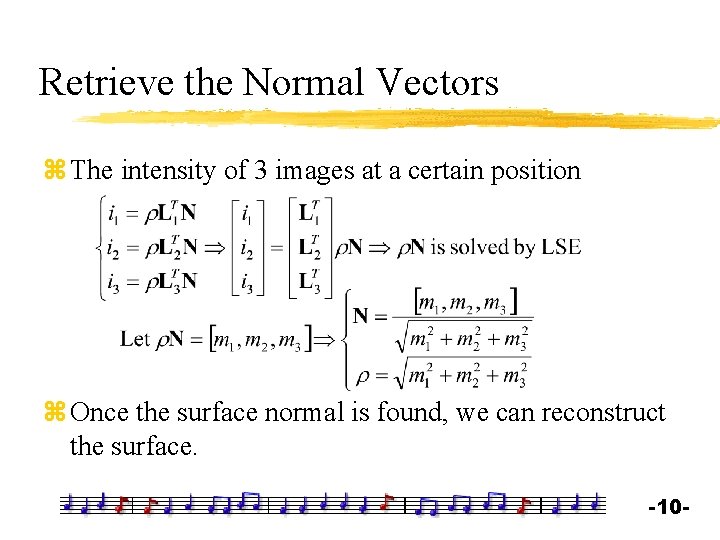
Retrieve the Normal Vectors z The intensity of 3 images at a certain position z Once the surface normal is found, we can reconstruct the surface. -10 -
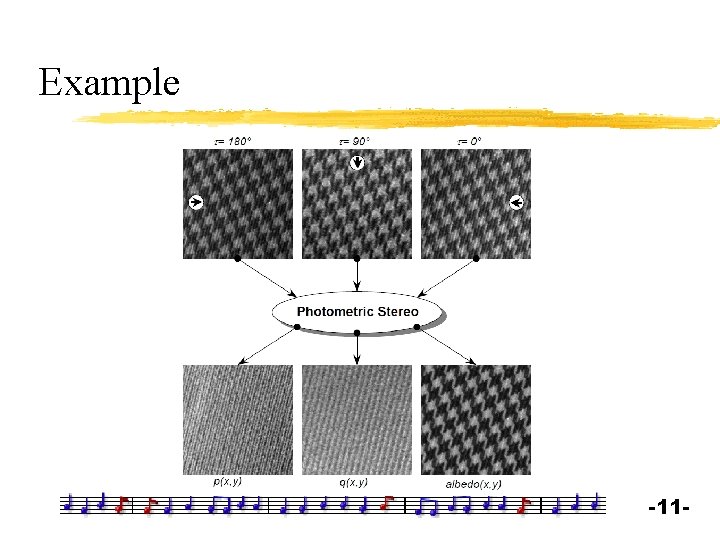
Example -11 -
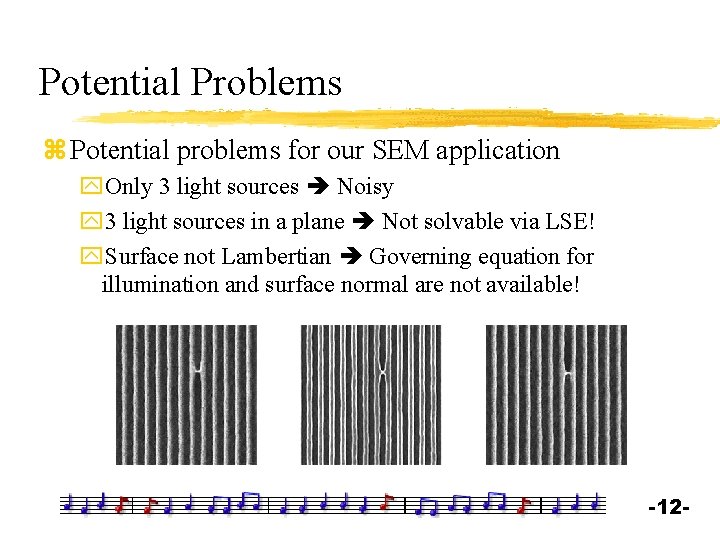
Potential Problems z Potential problems for our SEM application y. Only 3 light sources Noisy y 3 light sources in a plane Not solvable via LSE! y. Surface not Lambertian Governing equation for illumination and surface normal are not available! -12 -
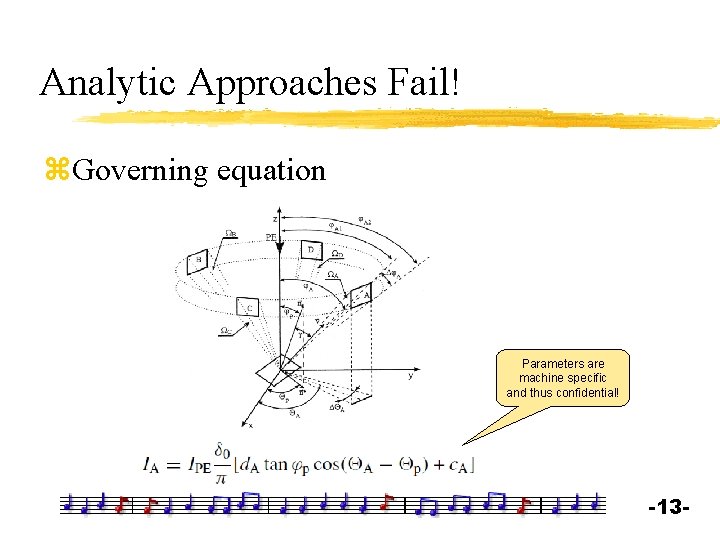
Analytic Approaches Fail! z. Governing equation Parameters are machine specific and thus confidential! -13 -
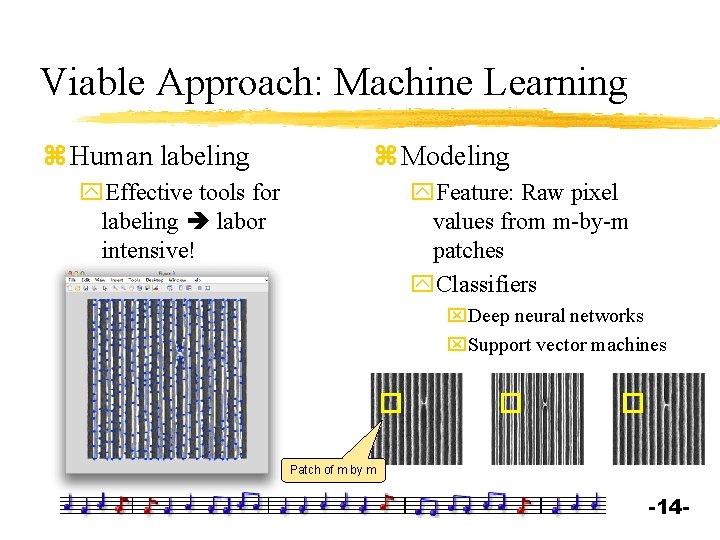
Viable Approach: Machine Learning z Human labeling z Modeling y. Effective tools for labeling labor intensive! y. Feature: Raw pixel values from m-by-m patches y. Classifiers x. Deep neural networks x. Support vector machines Patch of m by m -14 -
Sem sem sem
Scanning electron microscope main idea
Scanning electron microscopy
Scanning electron microscope explained
Components of tem
Scanning electron
Detailed reading examples
Intertextualidade 7 ano
Releia voltou a criar histórias
Which organelle breaks down organelles
Virtual vs real image
Yahoo.com.yw
Real images vs virtual images
Httpstw