Intercomparison of Model Simulations of Mixed Phase Clouds
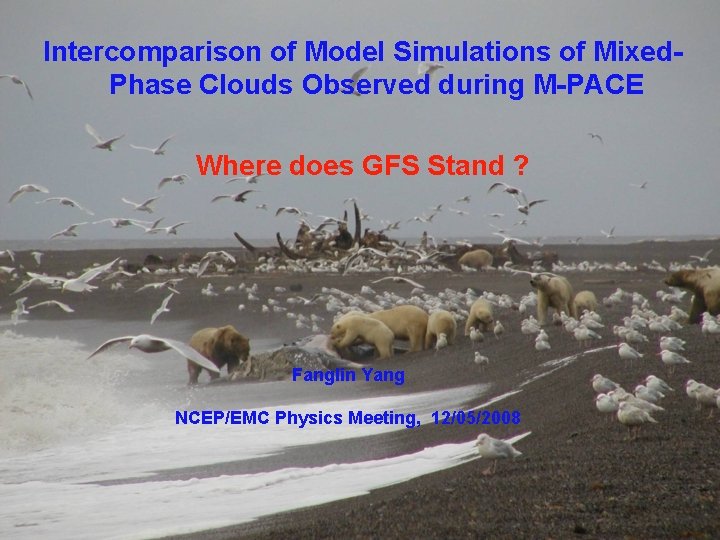
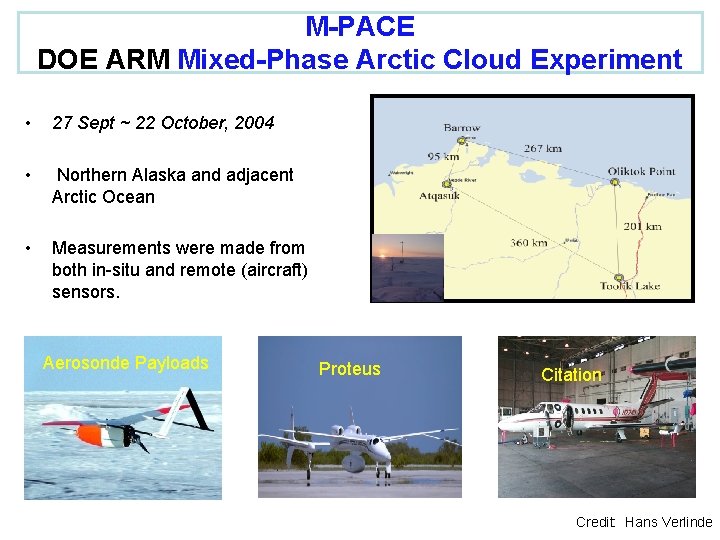
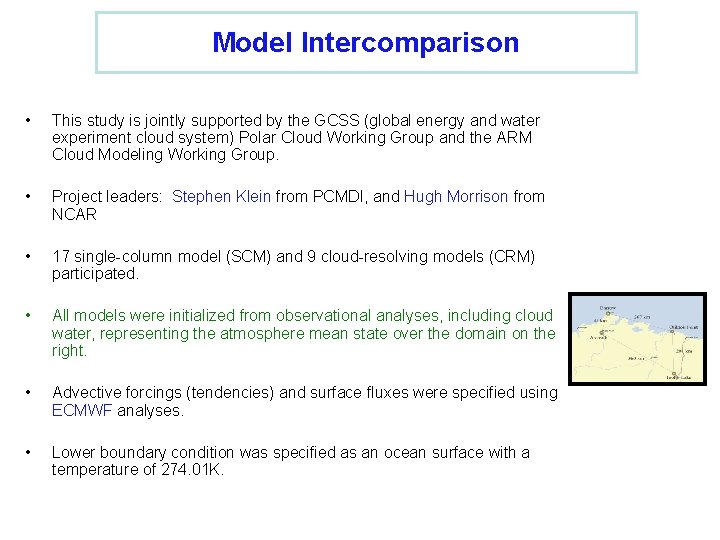
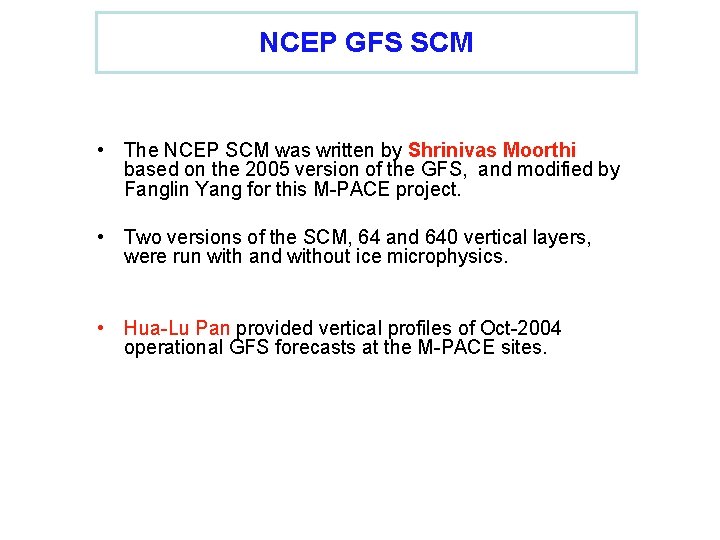
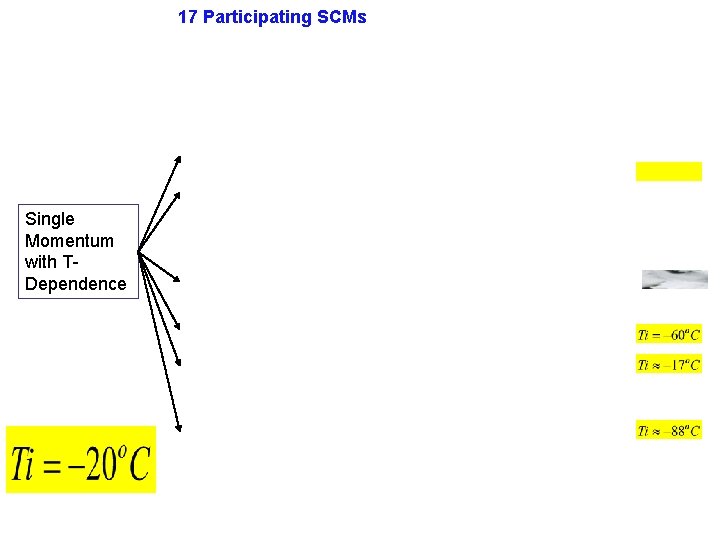
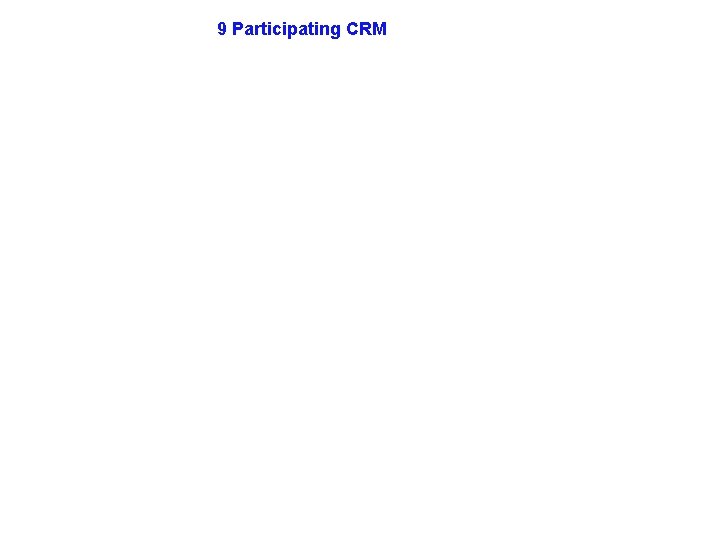
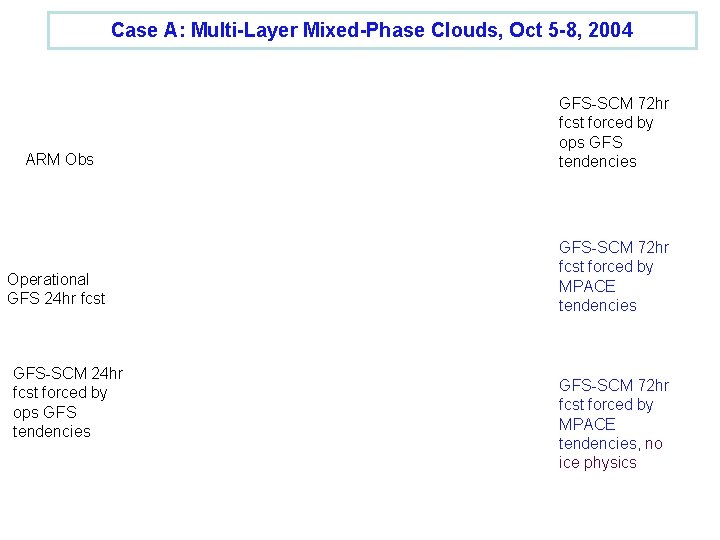
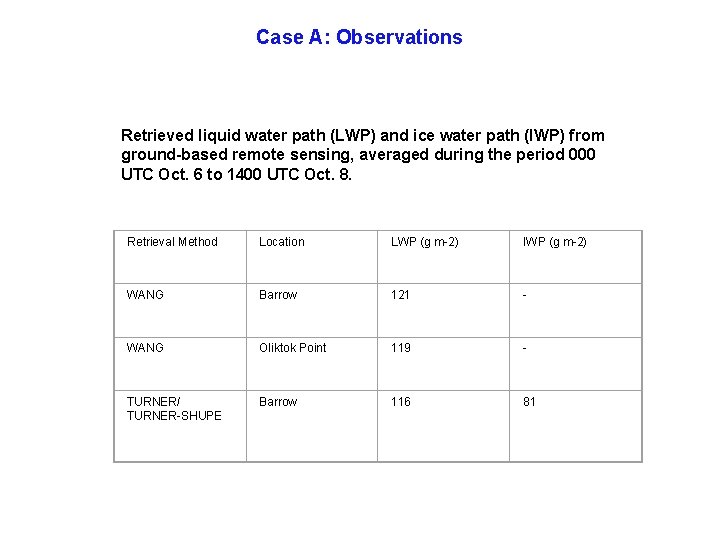
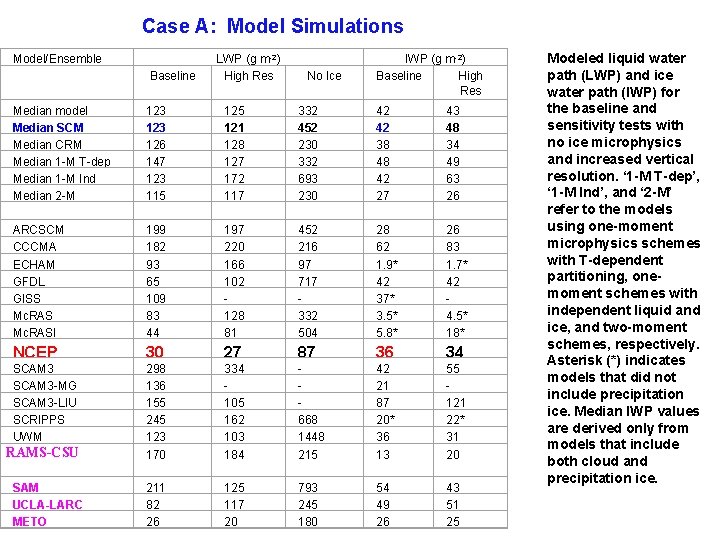
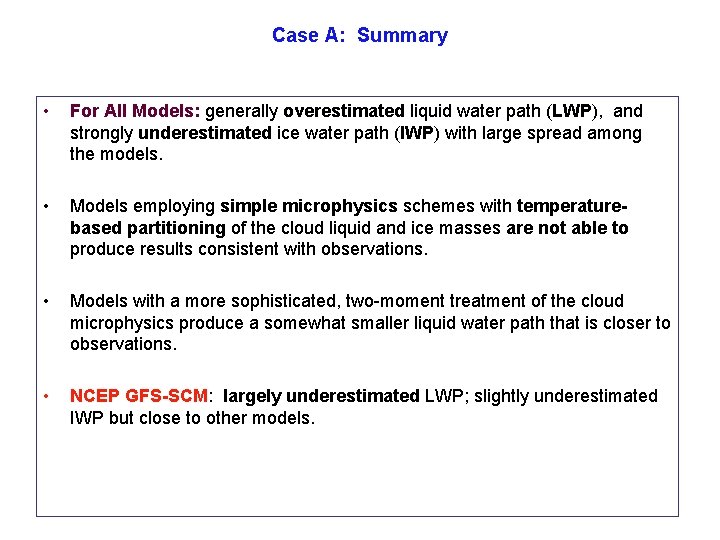
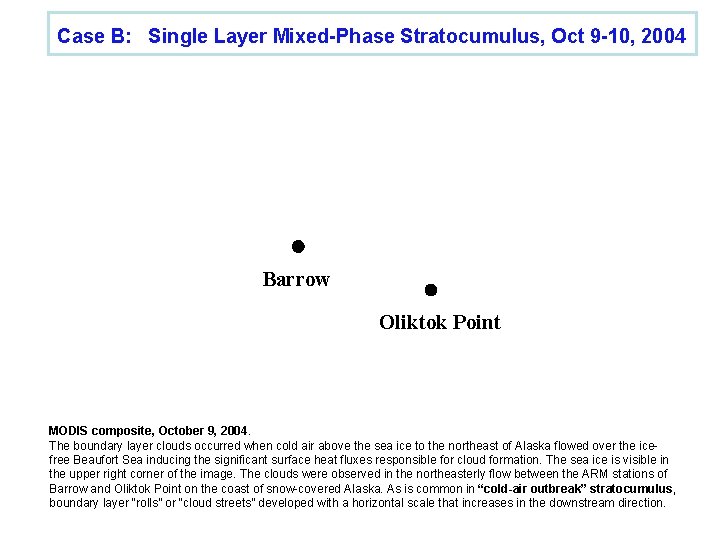
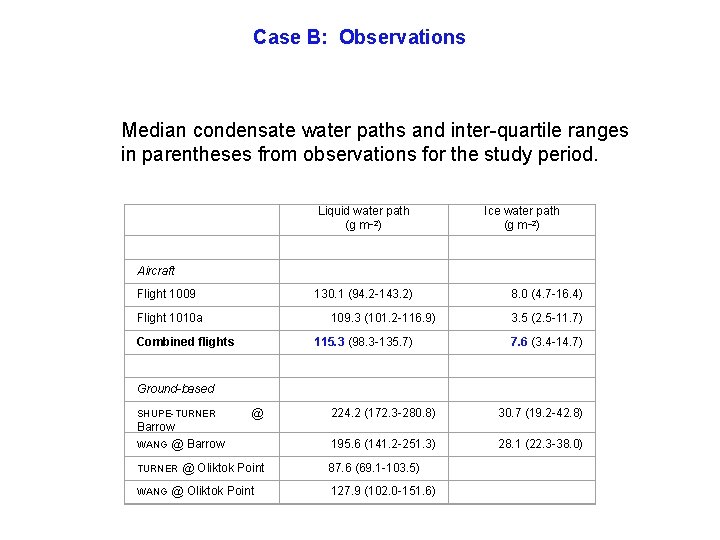
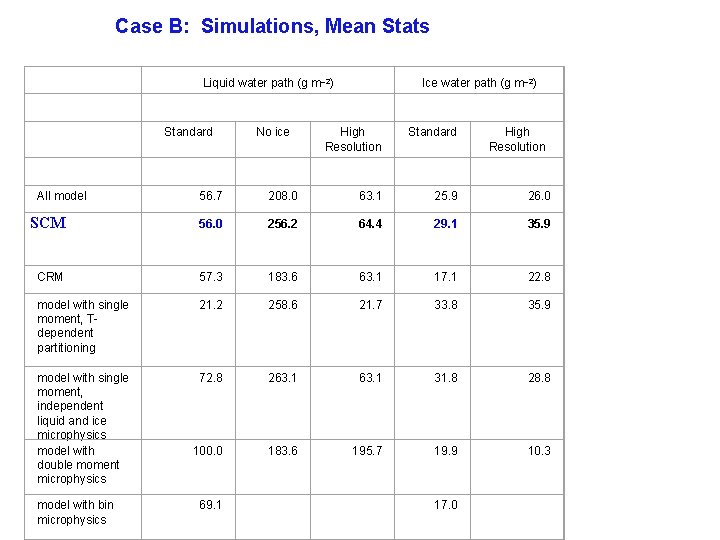
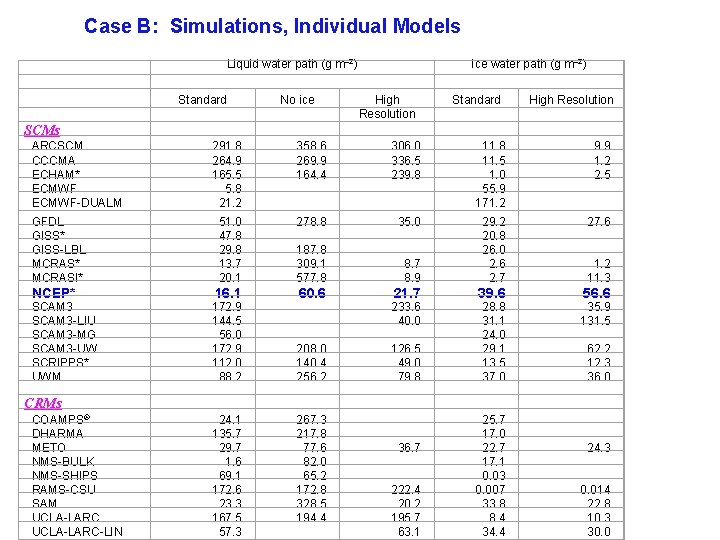
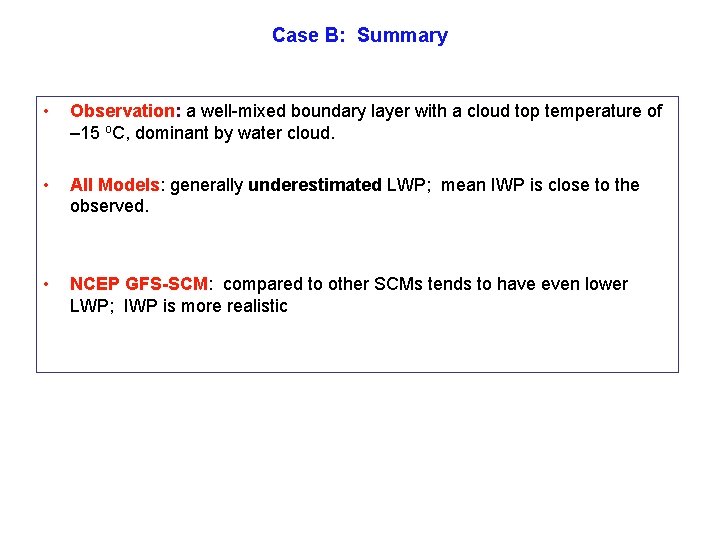
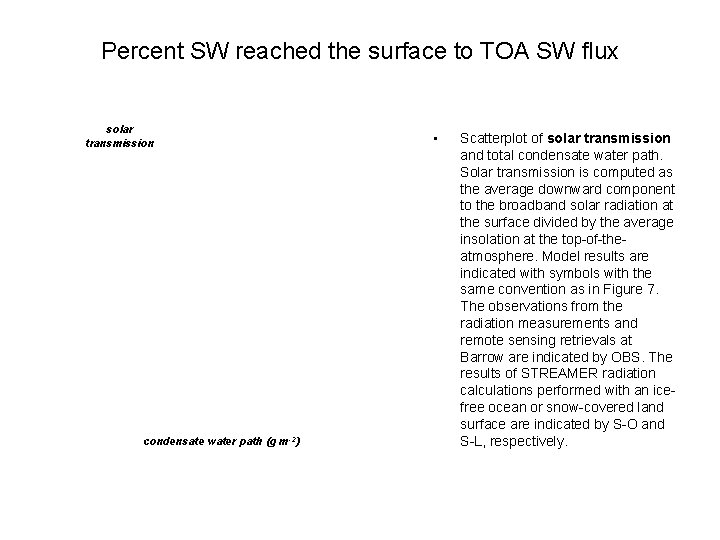
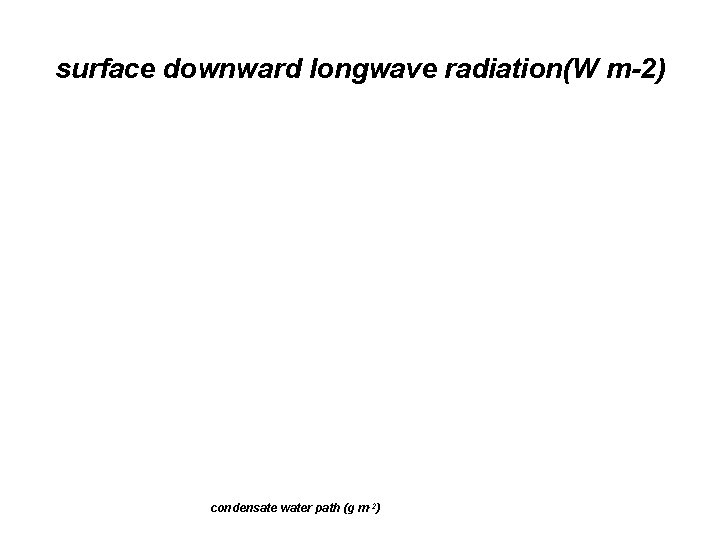
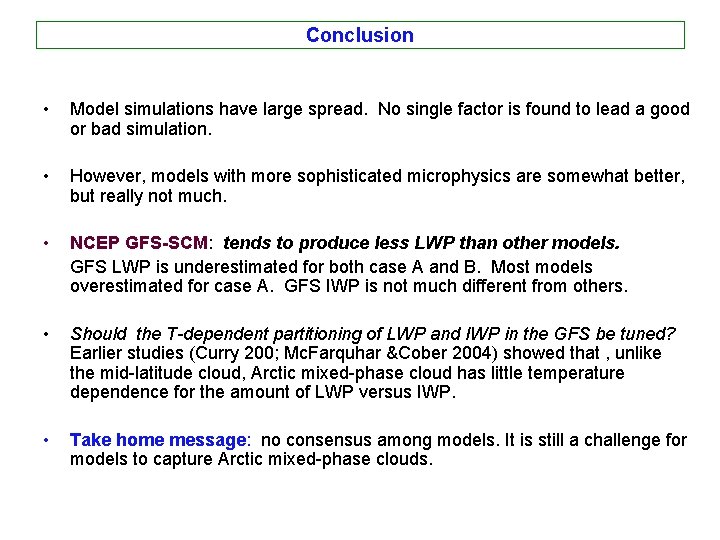
- Slides: 18
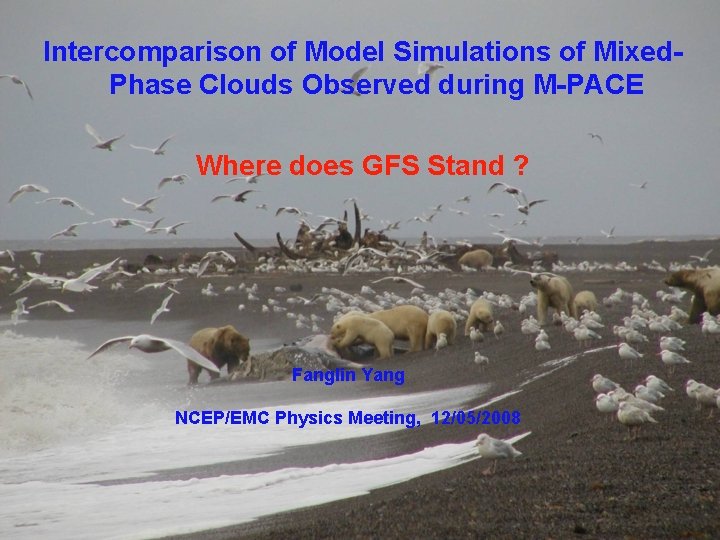
Intercomparison of Model Simulations of Mixed. Phase Clouds Observed during M-PACE Where does GFS Stand ? Fanglin Yang NCEP/EMC Physics Meeting, 12/05/2008
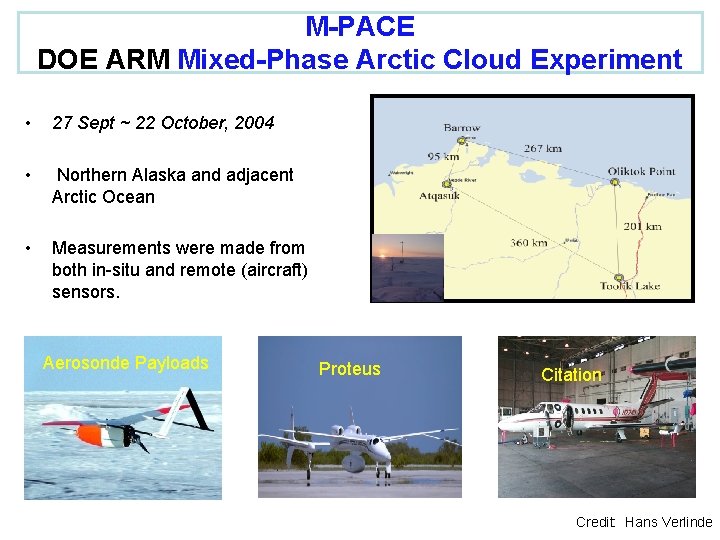
M-PACE DOE ARM Mixed-Phase Arctic Cloud Experiment • 27 Sept ~ 22 October, 2004 • Northern Alaska and adjacent Arctic Ocean • Measurements were made from both in-situ and remote (aircraft) sensors. Aerosonde Payloads Proteus Citation Credit: Hans Verlinde
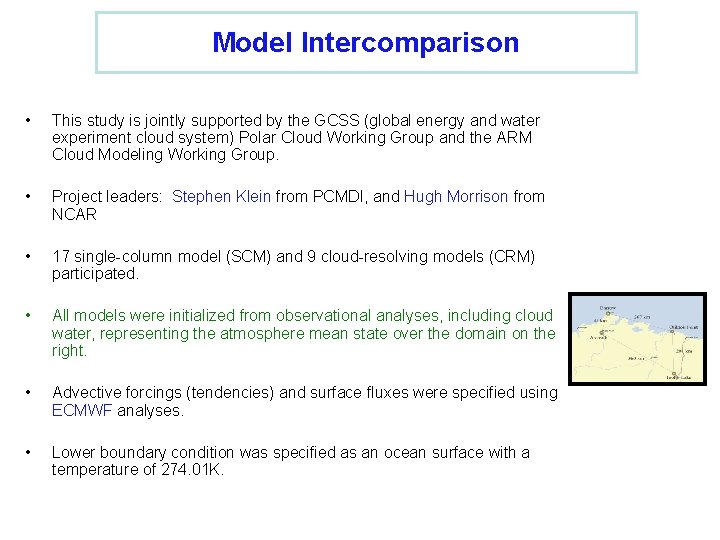
Model Intercomparison • This study is jointly supported by the GCSS (global energy and water experiment cloud system) Polar Cloud Working Group and the ARM Cloud Modeling Working Group. • Project leaders: Stephen Klein from PCMDI, and Hugh Morrison from NCAR • 17 single-column model (SCM) and 9 cloud-resolving models (CRM) participated. • All models were initialized from observational analyses, including cloud water, representing the atmosphere mean state over the domain on the right. • Advective forcings (tendencies) and surface fluxes were specified using ECMWF analyses. • Lower boundary condition was specified as an ocean surface with a temperature of 274. 01 K.
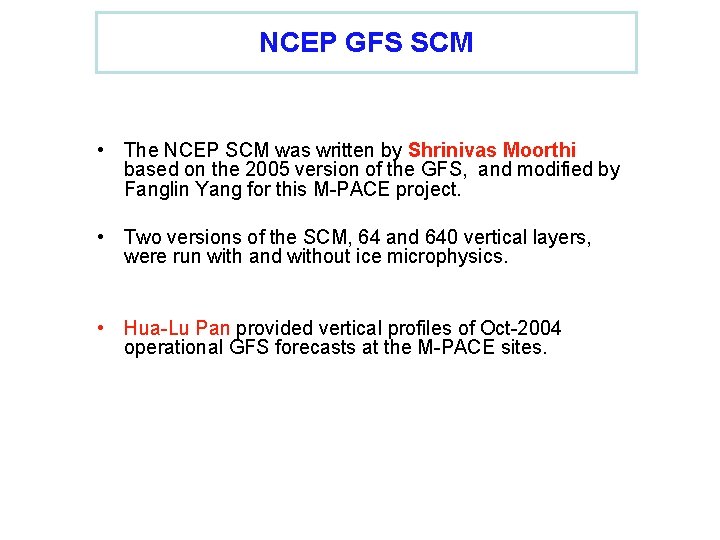
NCEP GFS SCM • The NCEP SCM was written by Shrinivas Moorthi based on the 2005 version of the GFS, and modified by Fanglin Yang for this M-PACE project. • Two versions of the SCM, 64 and 640 vertical layers, were run with and without ice microphysics. • Hua-Lu Pan provided vertical profiles of Oct-2004 operational GFS forecasts at the M-PACE sites.
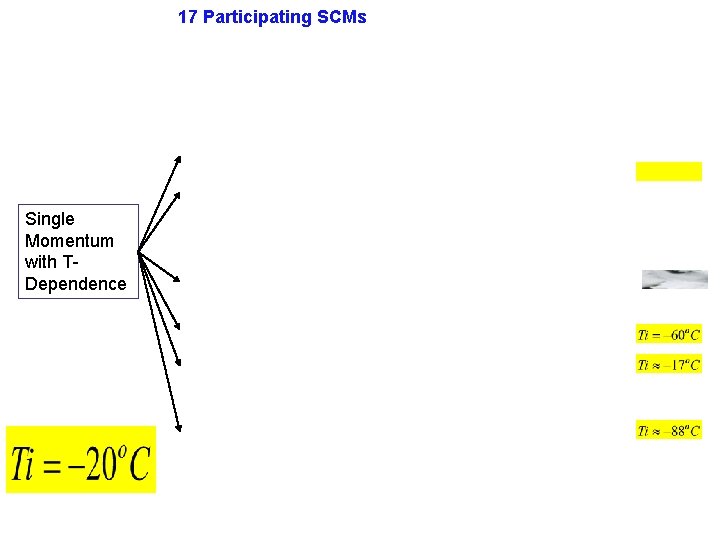
17 Participating SCMs Single Momentum with TDependence
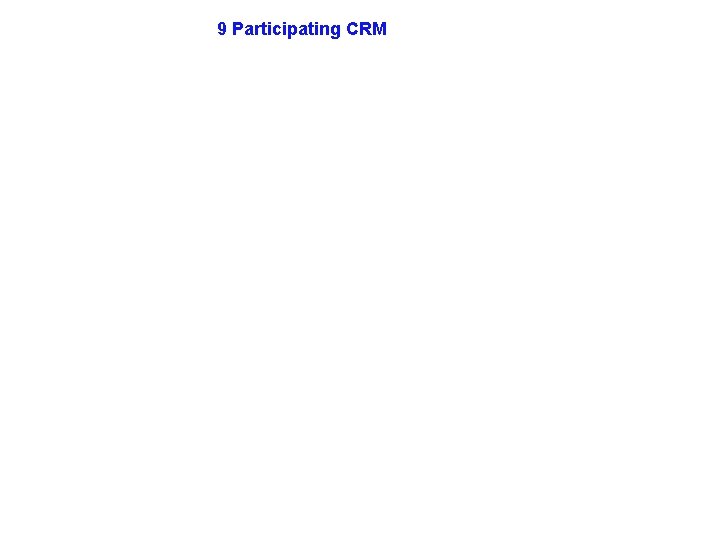
9 Participating CRM
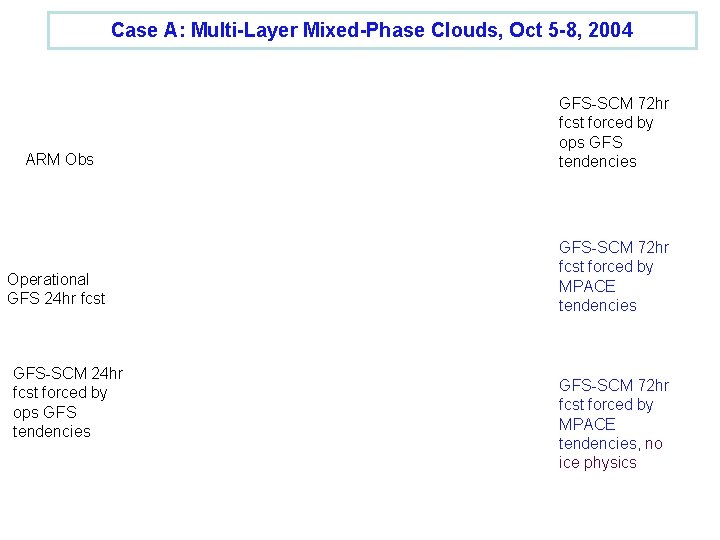
Case A: Multi-Layer Mixed-Phase Clouds, Oct 5 -8, 2004 ARM Obs GFS-SCM 72 hr fcst forced by ops GFS tendencies Operational GFS 24 hr fcst GFS-SCM 72 hr fcst forced by MPACE tendencies GFS-SCM 24 hr fcst forced by ops GFS tendencies GFS-SCM 72 hr fcst forced by MPACE tendencies, no ice physics
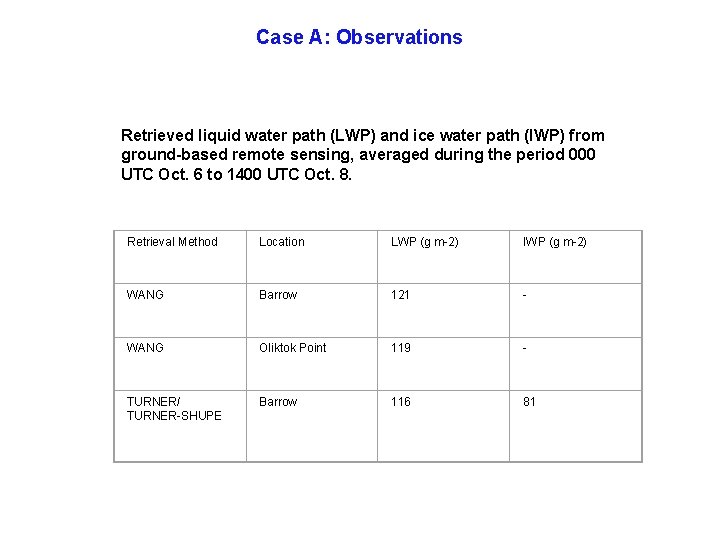
Case A: Observations Retrieved liquid water path (LWP) and ice water path (IWP) from ground-based remote sensing, averaged during the period 000 UTC Oct. 6 to 1400 UTC Oct. 8. Retrieval Method Location LWP (g m-2) IWP (g m-2) WANG Barrow 121 - WANG Oliktok Point 119 - TURNER/ TURNER-SHUPE Barrow 116 81
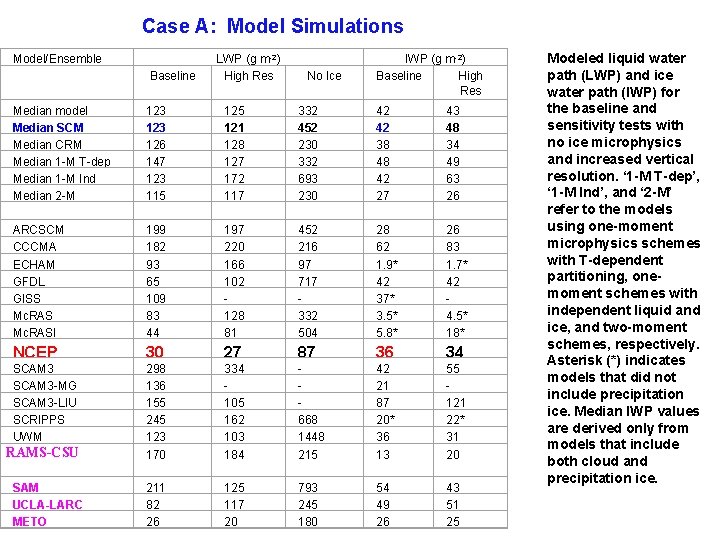
Case A: Model Simulations Model/Ensemble Baseline LWP (g m-2) High Res No Ice IWP (g m-2) Baseline High Res Median model Median SCM Median CRM Median 1 -M T-dep Median 1 -M Ind Median 2 -M 123 126 147 123 115 121 128 127 172 117 332 452 230 332 693 230 42 42 38 48 42 27 43 48 34 49 63 26 ARCSCM CCCMA ECHAM GFDL GISS Mc. RASI 199 182 93 65 109 83 44 197 220 166 102 128 81 452 216 97 717 332 504 28 62 1. 9* 42 37* 3. 5* 5. 8* 26 83 1. 7* 42 4. 5* 18* NCEP 30 27 87 36 34 SCAM 3 -MG SCAM 3 -LIU SCRIPPS UWM 298 136 155 245 123 170 334 105 162 103 184 668 1448 215 42 21 87 20* 36 13 55 121 22* 31 20 211 82 26 125 117 20 793 245 180 54 49 26 43 51 25 RAMS-CSU SAM UCLA-LARC METO Modeled liquid water path (LWP) and ice water path (IWP) for the baseline and sensitivity tests with no ice microphysics and increased vertical resolution. ‘ 1 -M T-dep’, ‘ 1 -M Ind’, and ‘ 2 -M’ refer to the models using one-moment microphysics schemes with T-dependent partitioning, onemoment schemes with independent liquid and ice, and two-moment schemes, respectively. Asterisk (*) indicates models that did not include precipitation ice. Median IWP values are derived only from models that include both cloud and precipitation ice.
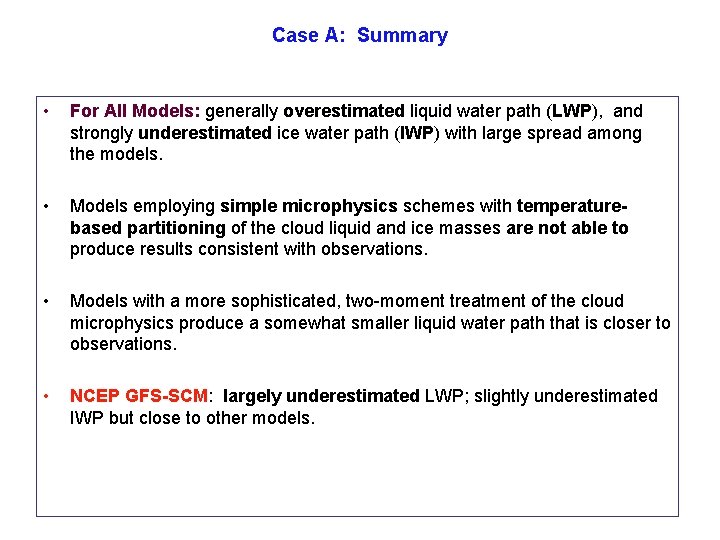
Case A: Summary • For All Models: generally overestimated liquid water path (LWP), and strongly underestimated ice water path (IWP) with large spread among the models. • Models employing simple microphysics schemes with temperaturebased partitioning of the cloud liquid and ice masses are not able to produce results consistent with observations. • Models with a more sophisticated, two-moment treatment of the cloud microphysics produce a somewhat smaller liquid water path that is closer to observations. • NCEP GFS-SCM: largely underestimated LWP; slightly underestimated IWP but close to other models.
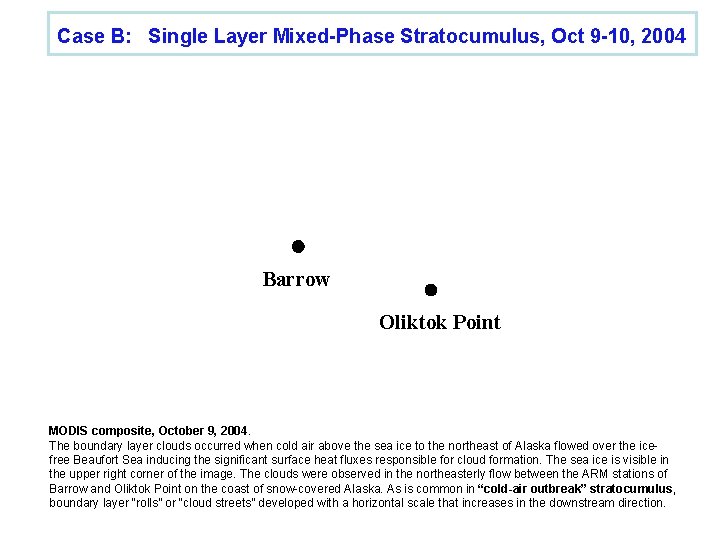
Case B: Single Layer Mixed-Phase Stratocumulus, Oct 9 -10, 2004 Barrow Oliktok Point MODIS composite, October 9, 2004. The boundary layer clouds occurred when cold air above the sea ice to the northeast of Alaska flowed over the icefree Beaufort Sea inducing the significant surface heat fluxes responsible for cloud formation. The sea ice is visible in the upper right corner of the image. The clouds were observed in the northeasterly flow between the ARM stations of Barrow and Oliktok Point on the coast of snow-covered Alaska. As is common in “cold-air outbreak” stratocumulus, boundary layer “rolls” or “cloud streets” developed with a horizontal scale that increases in the downstream direction.
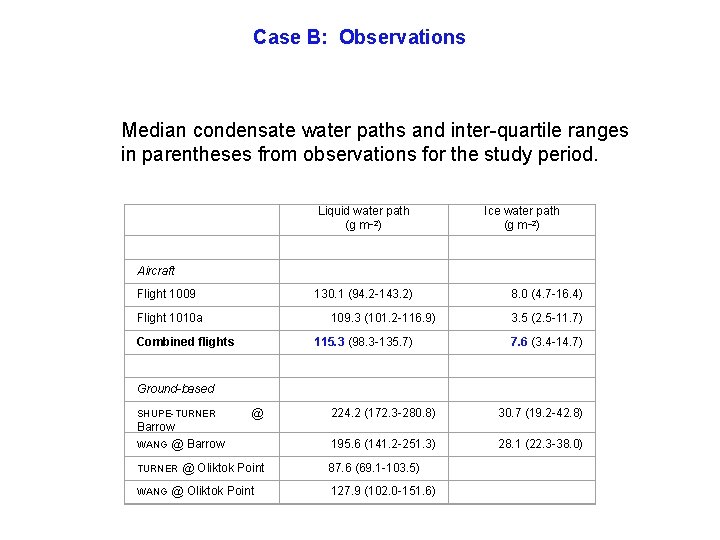
Case B: Observations Median condensate water paths and inter-quartile ranges in parentheses from observations for the study period. Liquid water path (g m– 2) Ice water path (g m– 2) Aircraft Flight 1009 130. 1 (94. 2 -143. 2) Flight 1010 a 109. 3 (101. 2 -116. 9) Combined flights 115. 3 (98. 3 -135. 7) 8. 0 (4. 7 -16. 4) 3. 5 (2. 5 -11. 7) 7. 6 (3. 4 -14. 7) Ground-based SHUPE-TURNER @ Barrow WANG @ Barrow TURNER WANG @ Oliktok Point 224. 2 (172. 3 -280. 8) 30. 7 (19. 2 -42. 8) 195. 6 (141. 2 -251. 3) 28. 1 (22. 3 -38. 0) 87. 6 (69. 1 -103. 5) 127. 9 (102. 0 -151. 6)
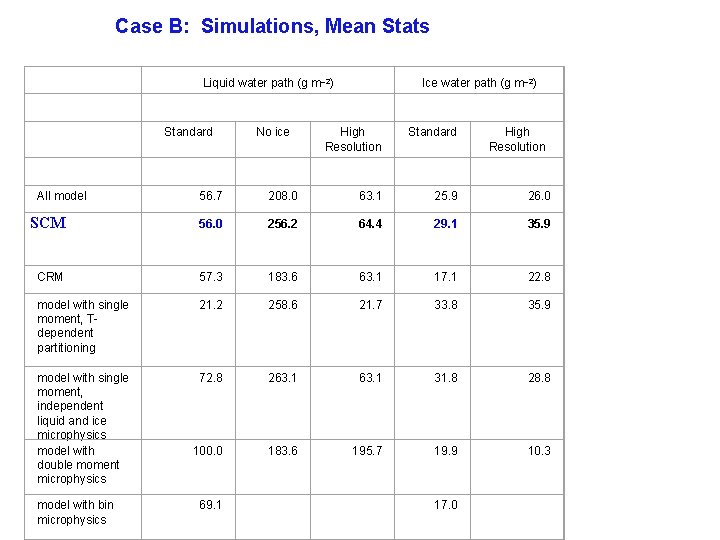
Case B: Simulations, Mean Stats Liquid water path (g m– 2) Standard All model No ice Ice water path (g m– 2) High Resolution Standard High Resolution 56. 7 208. 0 63. 1 25. 9 26. 0 SCM 56. 0 256. 2 64. 4 29. 1 35. 9 CRM 57. 3 183. 6 63. 1 17. 1 22. 8 model with single moment, Tdependent partitioning 21. 2 258. 6 21. 7 33. 8 35. 9 model with single moment, independent liquid and ice microphysics model with double moment microphysics 72. 8 263. 1 31. 8 28. 8 100. 0 183. 6 195. 7 19. 9 10. 3 model with bin microphysics 69. 1 17. 0
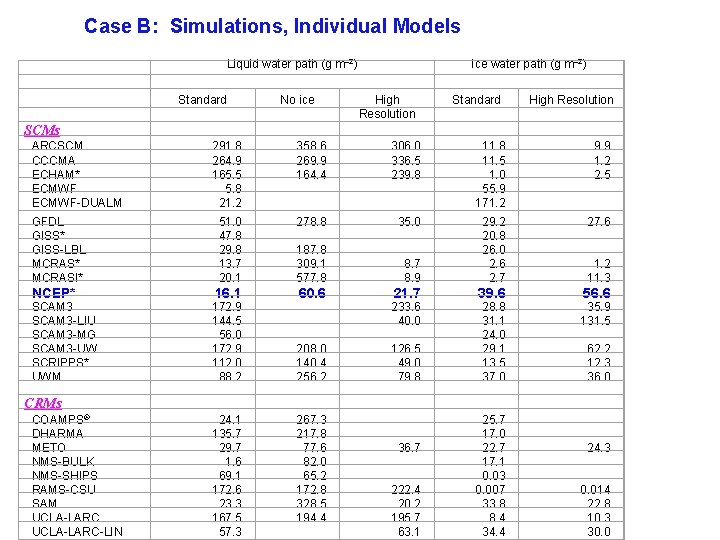
Case B: Simulations, Individual Models Liquid water path (g m– 2) Standard No ice Ice water path (g m– 2) High Resolution Standard High Resolution SCMs ARCSCM CCCMA ECHAM* ECMWF-DUALM GFDL GISS* GISS-LBL MCRAS* MCRASI* NCEP* SCAM 3 -LIU SCAM 3 -MG SCAM 3 -UW SCRIPPS* UWM 291. 8 264. 9 165. 5 5. 8 21. 2 358. 6 269. 9 164. 4 306. 0 336. 5 239. 8 11. 5 1. 0 55. 9 171. 2 9. 9 1. 2 2. 5 51. 0 47. 8 29. 8 13. 7 20. 1 278. 8 35. 0 27. 6 187. 8 309. 1 577. 8 8. 7 8. 9 29. 2 20. 8 26. 0 2. 6 2. 7 16. 1 60. 6 21. 7 39. 6 56. 6 172. 9 144. 5 56. 0 172. 9 112. 0 88. 2 208. 0 140. 4 256. 2 233. 6 40. 0 126. 5 49. 0 79. 8 28. 8 31. 1 24. 0 29. 1 13. 5 37. 0 1. 2 11. 3 35. 9 131. 5 62. 2 12. 3 36. 0 CRMs COAMPS® DHARMA METO NMS-BULK NMS-SHIPS RAMS-CSU SAM UCLA-LARC-LIN 24. 1 135. 7 29. 7 1. 6 69. 1 172. 6 23. 3 167. 5 57. 3 267. 3 217. 8 77. 6 82. 0 65. 2 172. 8 328. 5 194. 4 36. 7 222. 4 20. 2 195. 7 63. 1 25. 7 17. 0 22. 7 17. 1 0. 03 0. 007 33. 8 8. 4 34. 4 24. 3 0. 014 22. 8 10. 3 30. 0
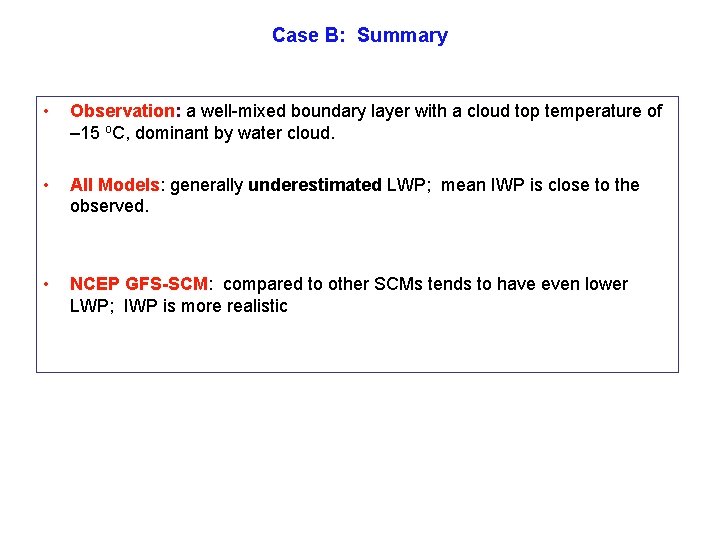
Case B: Summary • Observation: a well-mixed boundary layer with a cloud top temperature of – 15 o. C, dominant by water cloud. • All Models: generally underestimated LWP; mean IWP is close to the observed. • NCEP GFS-SCM: compared to other SCMs tends to have even lower LWP; IWP is more realistic
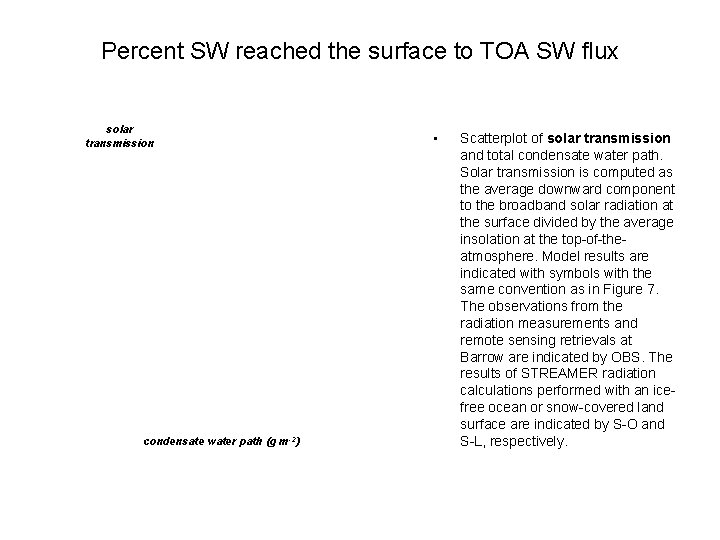
Percent SW reached the surface to TOA SW flux solar transmission condensate water path (g m-2) • Scatterplot of solar transmission and total condensate water path. Solar transmission is computed as the average downward component to the broadband solar radiation at the surface divided by the average insolation at the top-of-theatmosphere. Model results are indicated with symbols with the same convention as in Figure 7. The observations from the radiation measurements and remote sensing retrievals at Barrow are indicated by OBS. The results of STREAMER radiation calculations performed with an icefree ocean or snow-covered land surface are indicated by S-O and S-L, respectively.
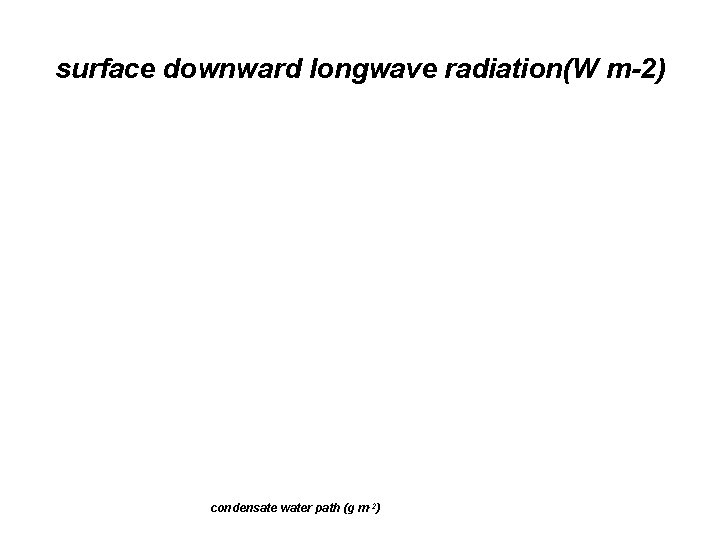
surface downward longwave radiation(W m-2) condensate water path (g m-2)
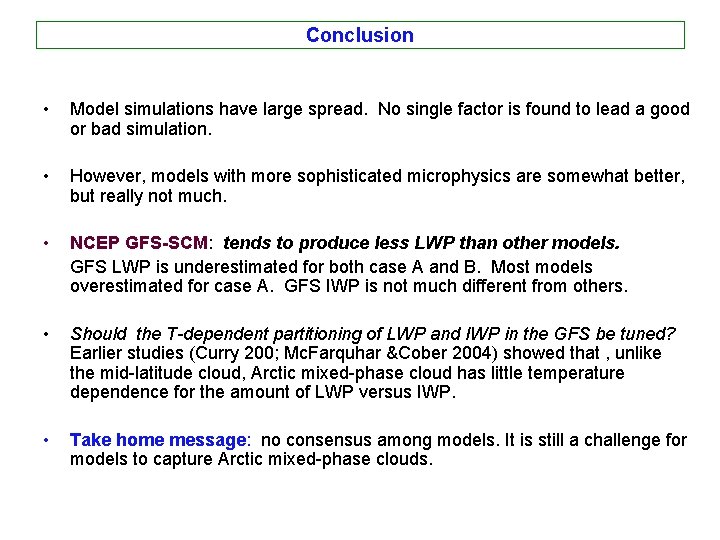
Conclusion • Model simulations have large spread. No single factor is found to lead a good or bad simulation. • However, models with more sophisticated microphysics are somewhat better, but really not much. • NCEP GFS-SCM: tends to produce less LWP than other models. GFS LWP is underestimated for both case A and B. Most models overestimated for case A. GFS IWP is not much different from others. • Should the T-dependent partitioning of LWP and IWP in the GFS be tuned? Earlier studies (Curry 200; Mc. Farquhar &Cober 2004) showed that , unlike the mid-latitude cloud, Arctic mixed-phase cloud has little temperature dependence for the amount of LWP versus IWP. • Take home message: no consensus among models. It is still a challenge for models to capture Arctic mixed-phase clouds.
Coupled model intercomparison project phase 5
Don't gamble with physical properties for simulations
Clinical simulations in nursing education
Chris harding simulations
Tcad simulations
World history simulations
Simulations for solid state physics
Www.irs.gov/app/understanding taxes/student/simulations.jsp
Ippd key tenets
Baton simulations
Normal phase vs reverse phase chromatography
Tswett pronunciation
Mobile phase and stationary phase
What is mobile and stationary phase
Normal phase vs reverse phase chromatography
Difference between phase voltage and line voltage
Adsorption chromatography
In a ∆-connected source feeding a y-connected load
Broad phase vs narrow phase