Functional Linear Models Extend linear model ideas to
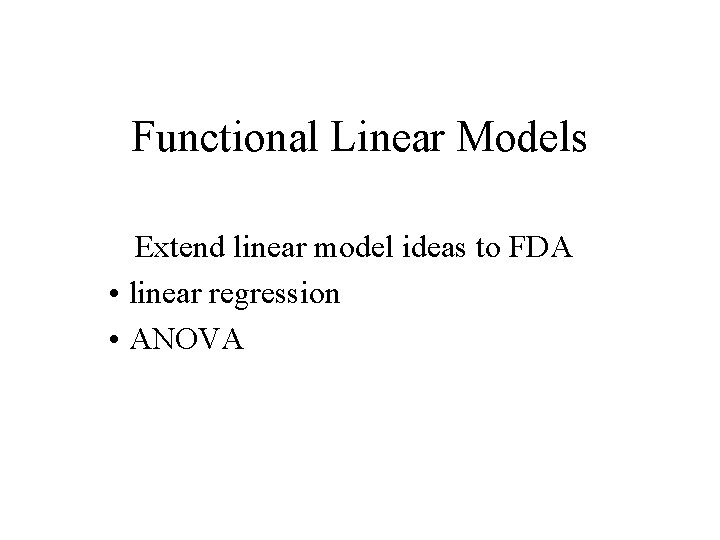
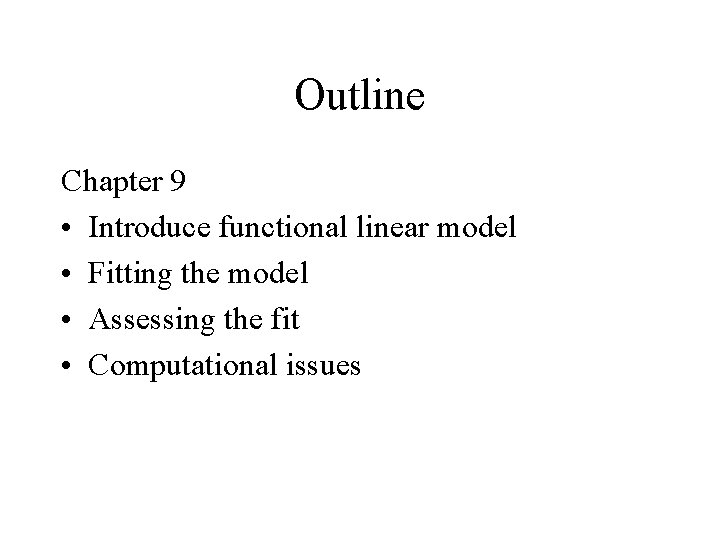
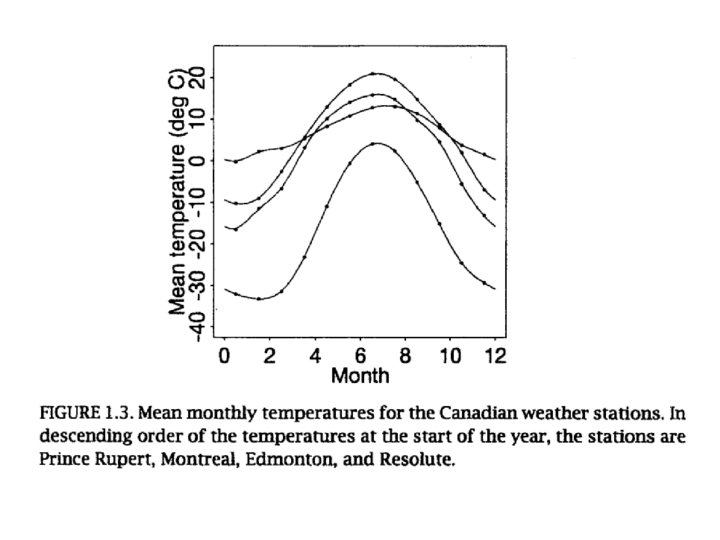
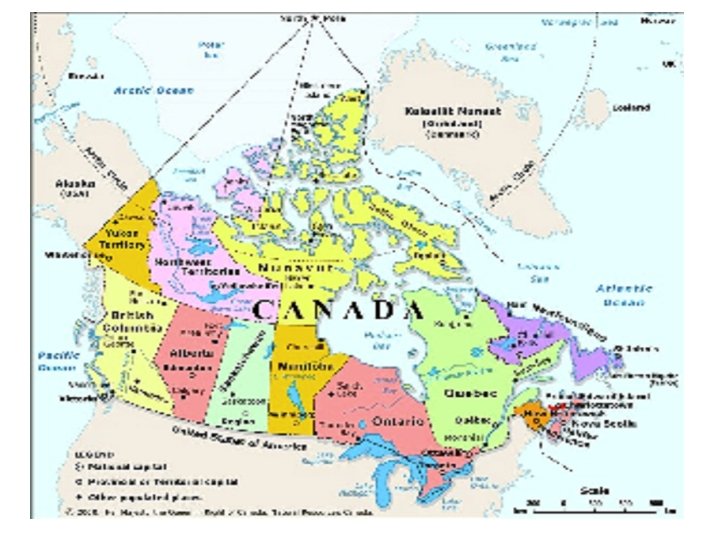
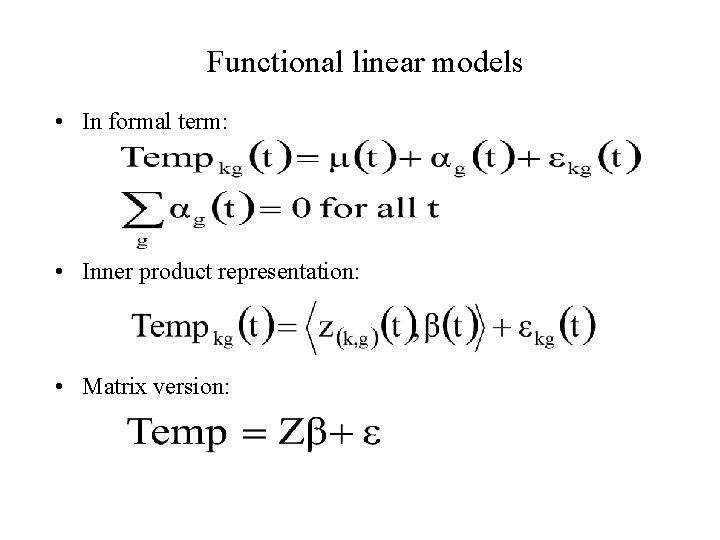
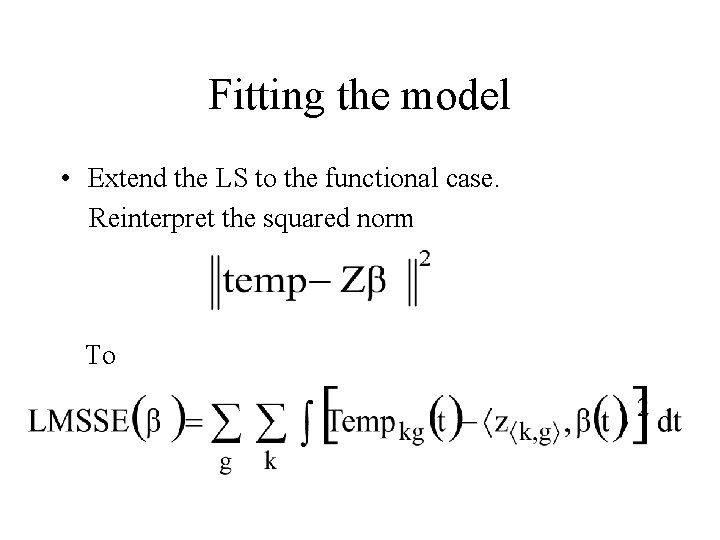
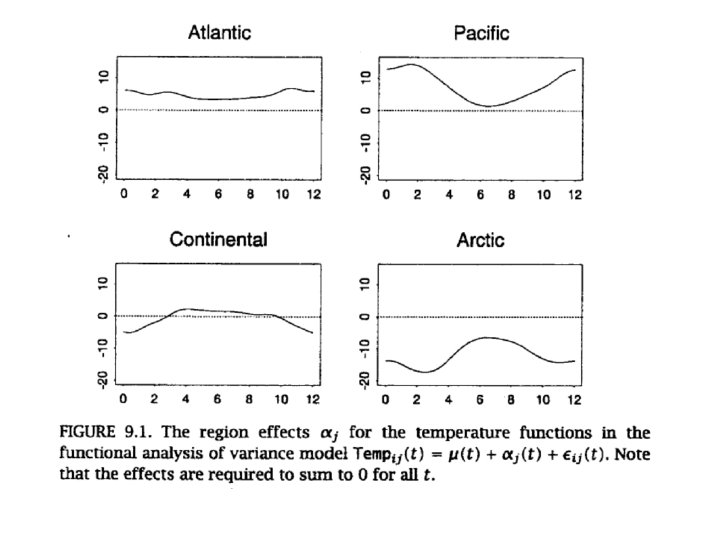
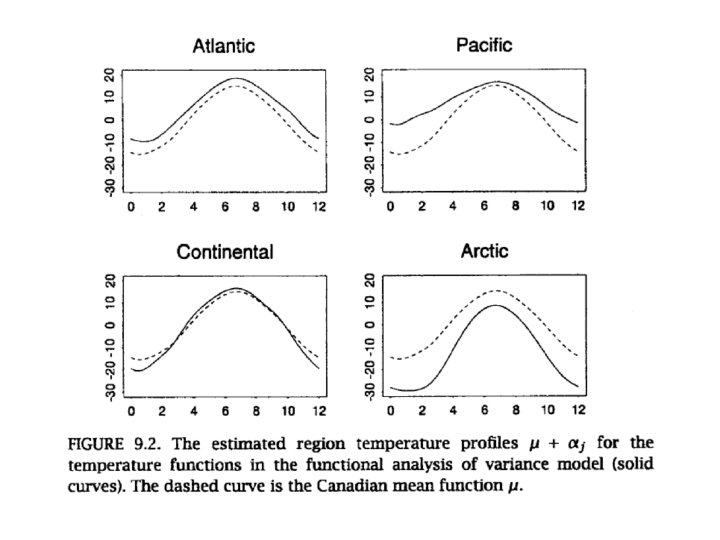
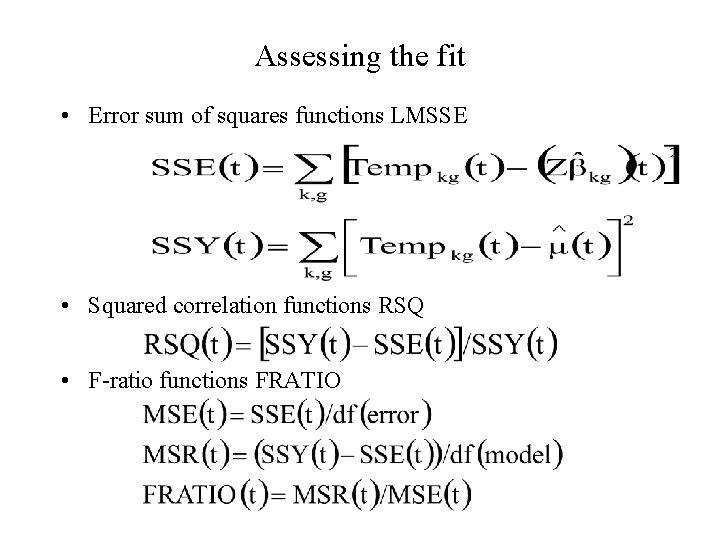
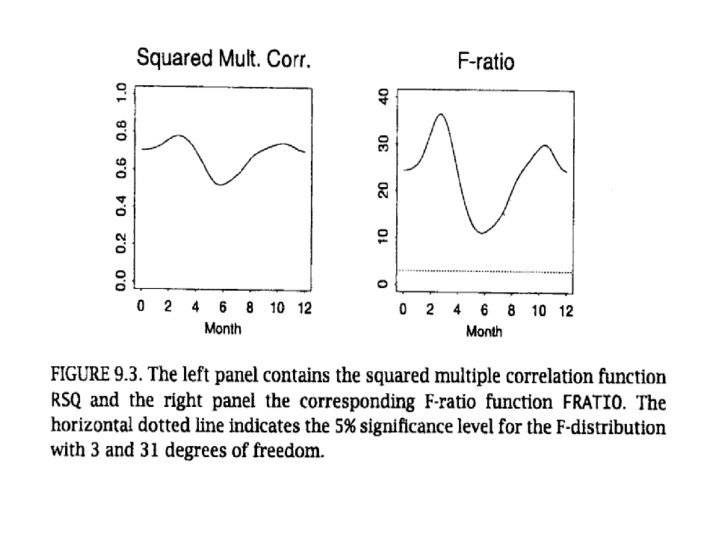
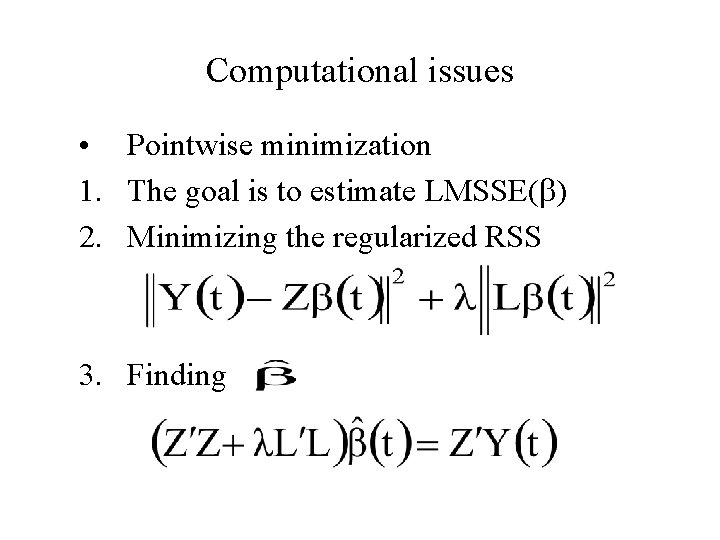
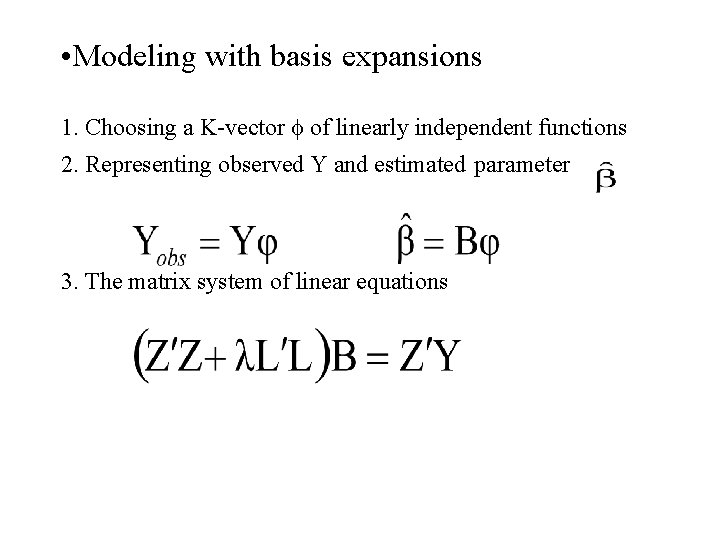
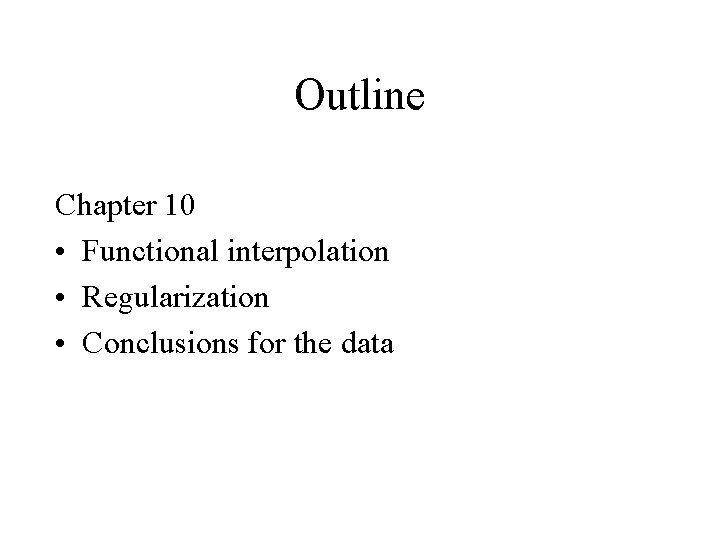
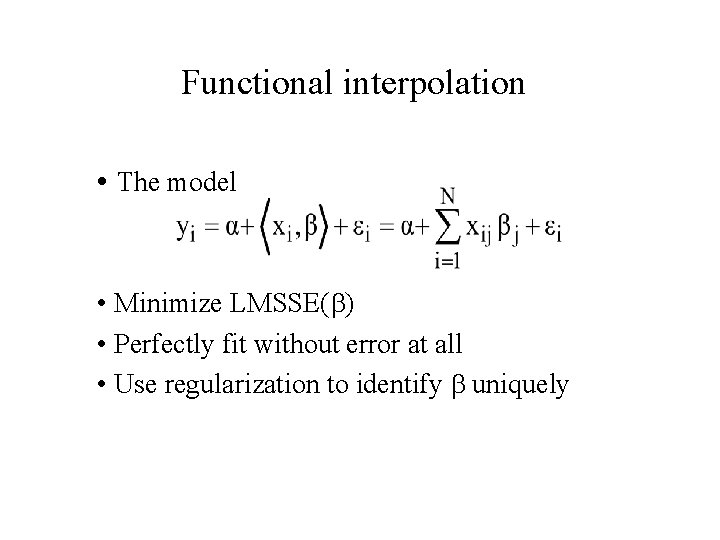
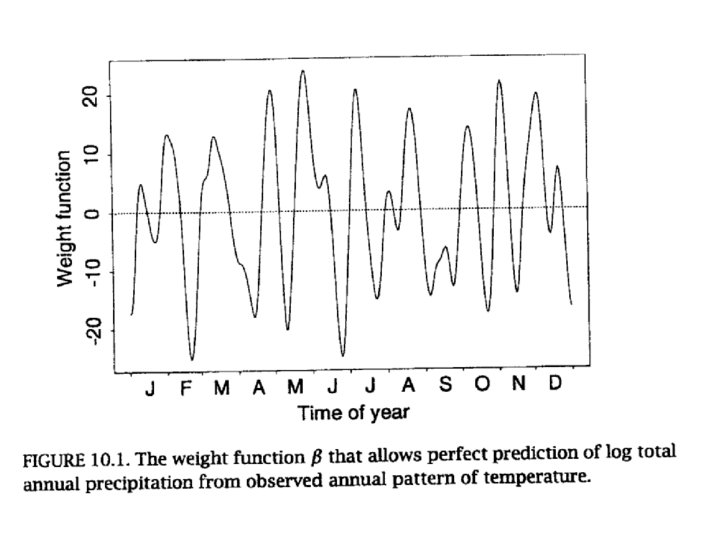
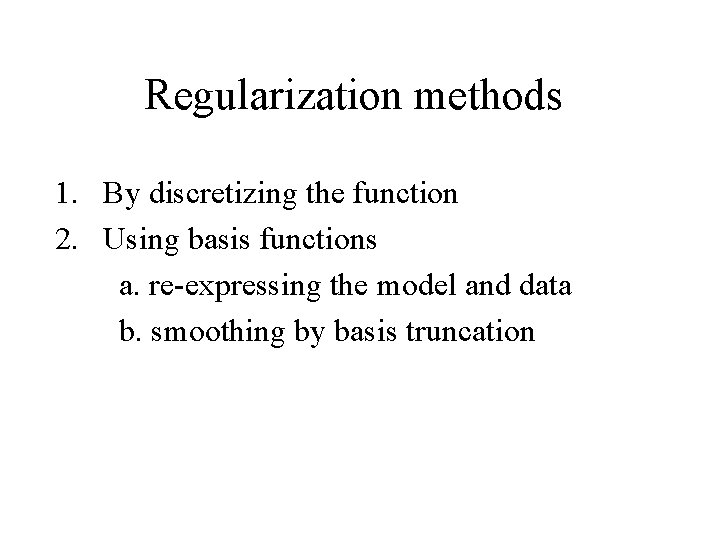
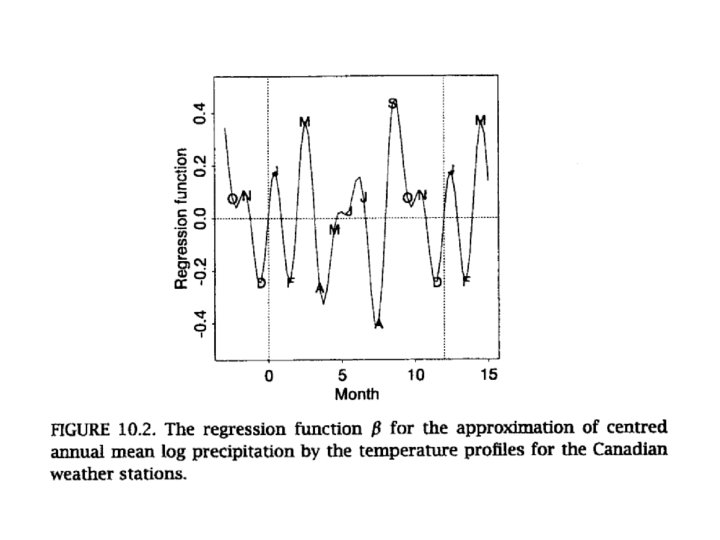
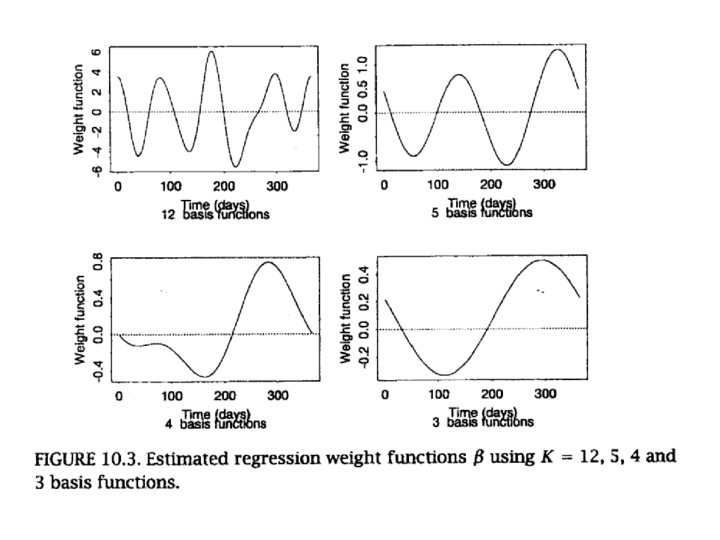
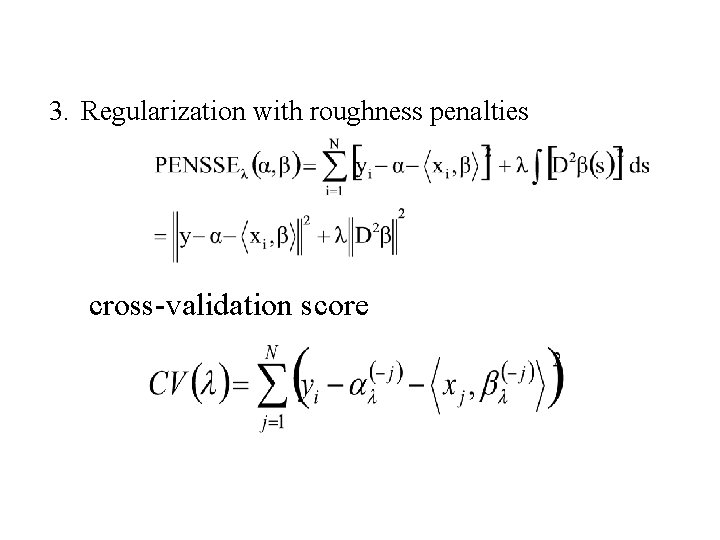
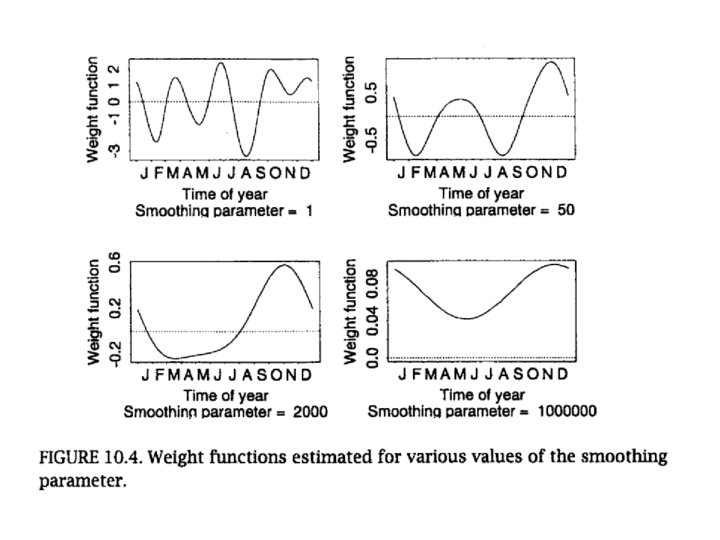
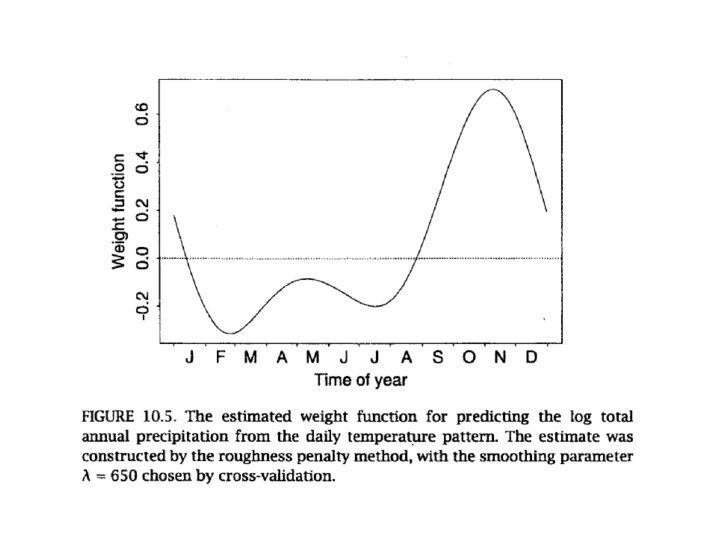
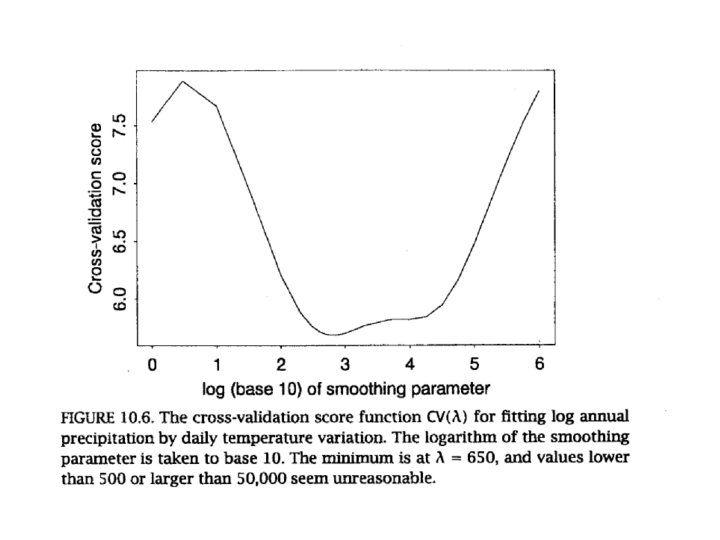
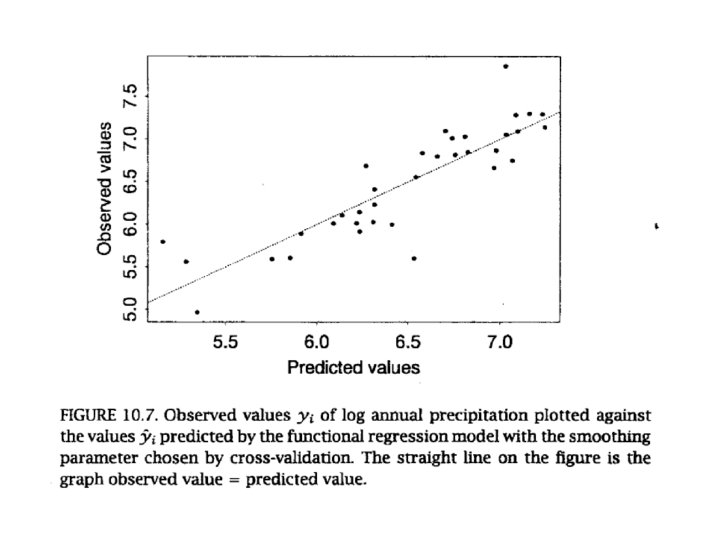
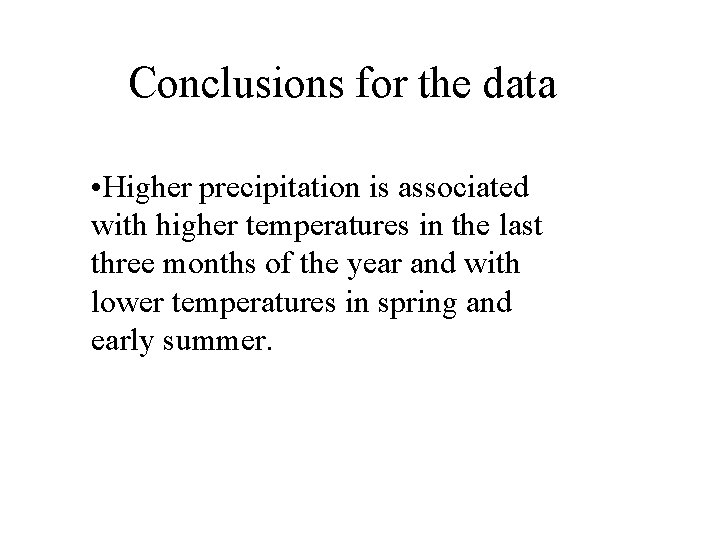
- Slides: 24
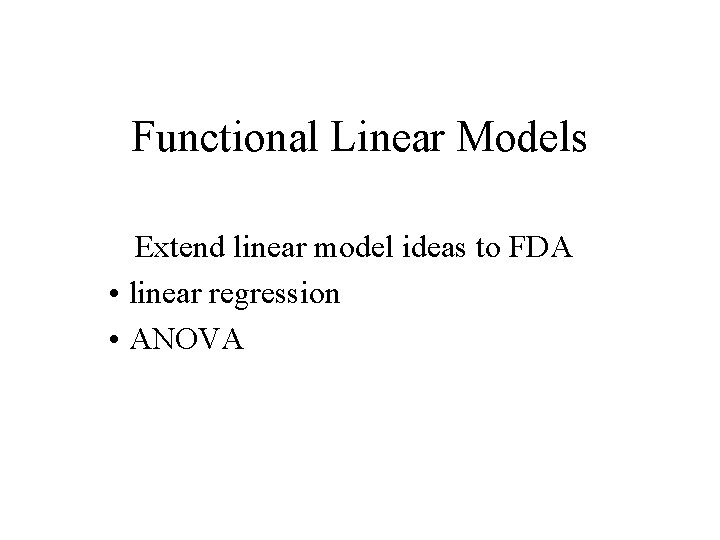
Functional Linear Models Extend linear model ideas to FDA • linear regression • ANOVA
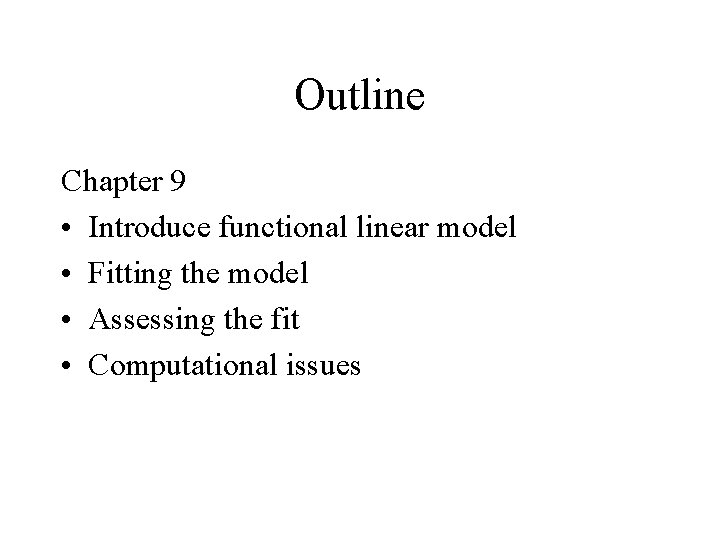
Outline Chapter 9 • Introduce functional linear model • Fitting the model • Assessing the fit • Computational issues
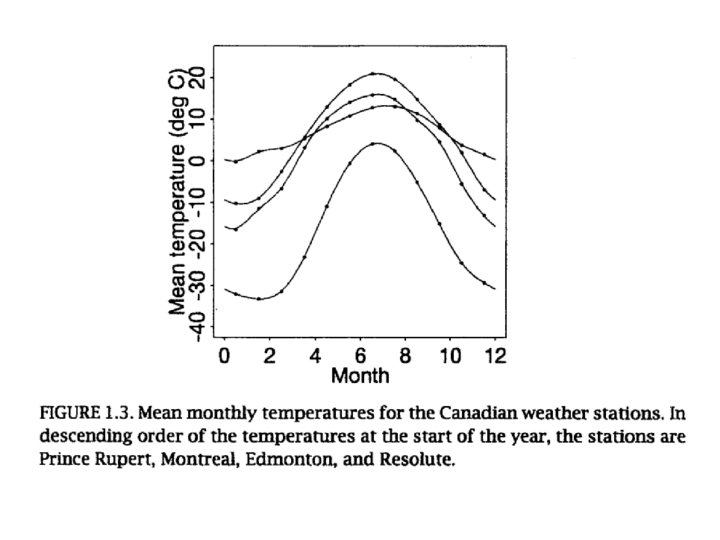
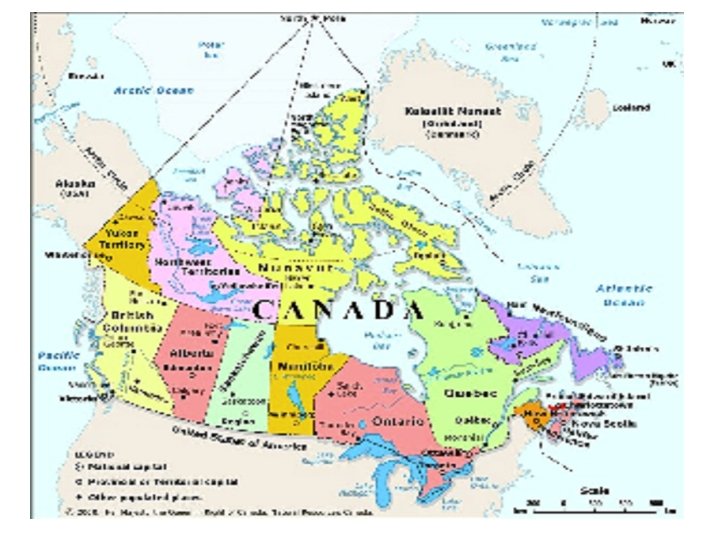
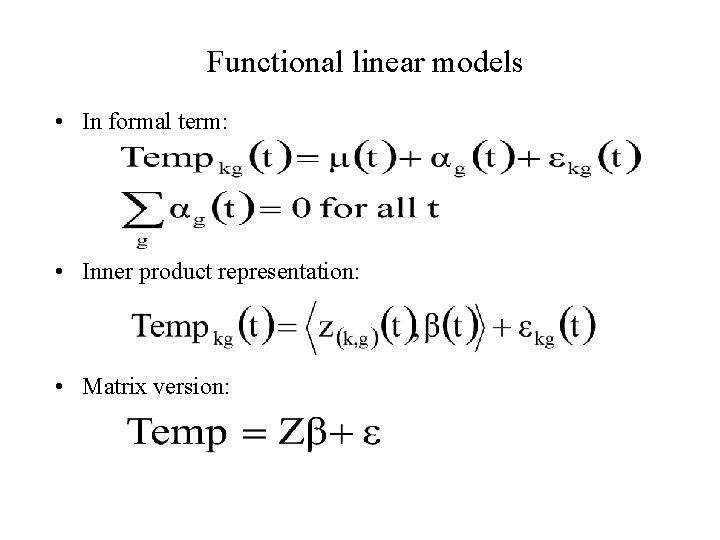
Functional linear models • In formal term: • Inner product representation: • Matrix version:
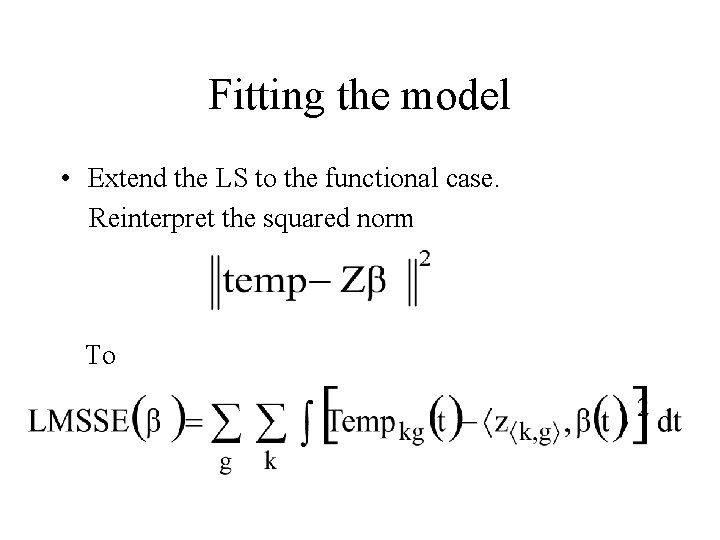
Fitting the model • Extend the LS to the functional case. Reinterpret the squared norm To
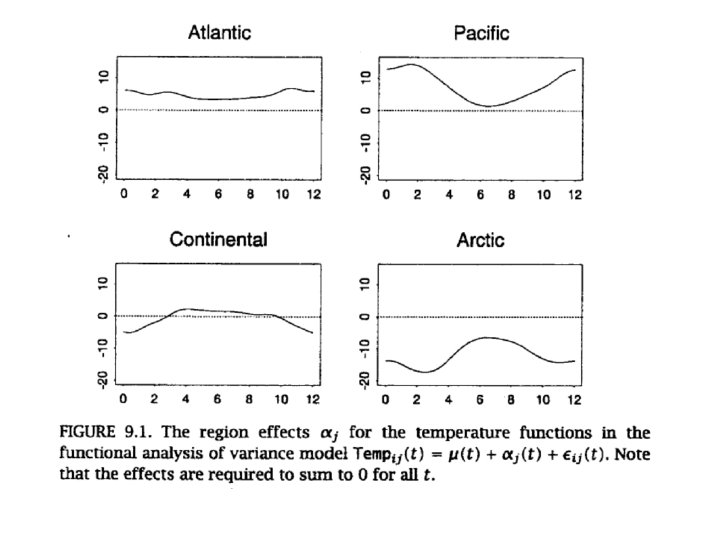
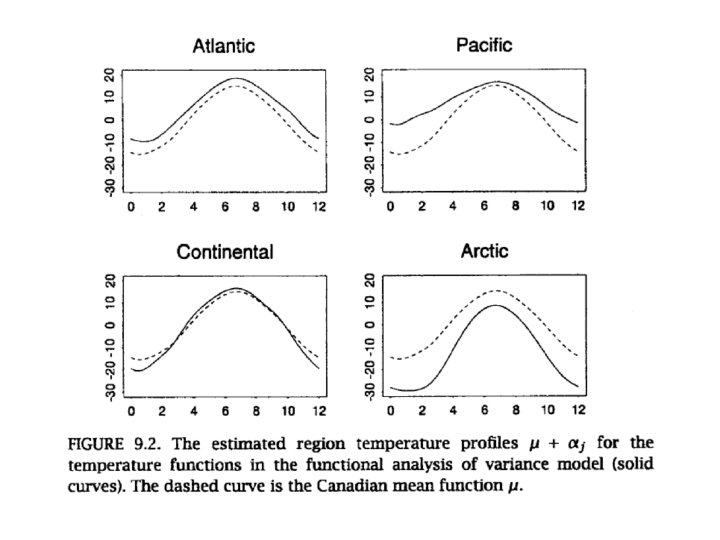
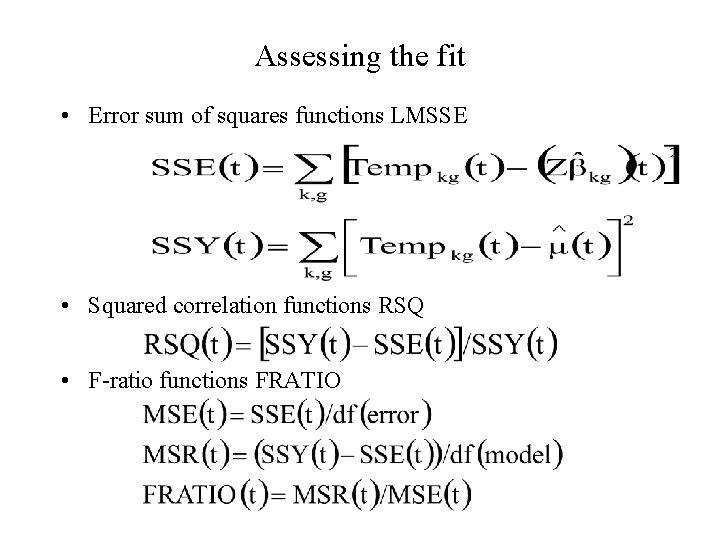
Assessing the fit • Error sum of squares functions LMSSE • Squared correlation functions RSQ • F-ratio functions FRATIO
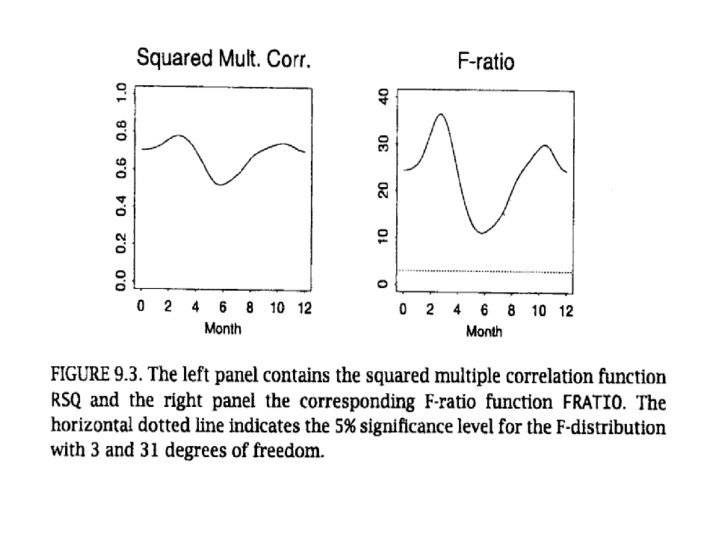
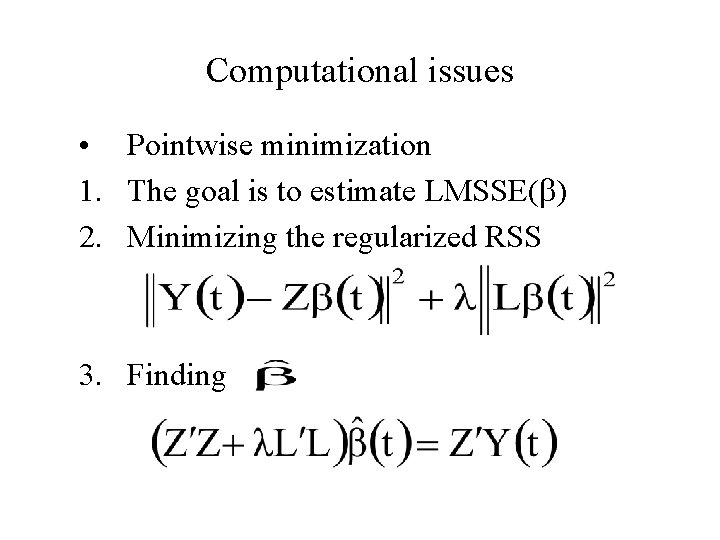
Computational issues • Pointwise minimization 1. The goal is to estimate LMSSE( ) 2. Minimizing the regularized RSS 3. Finding
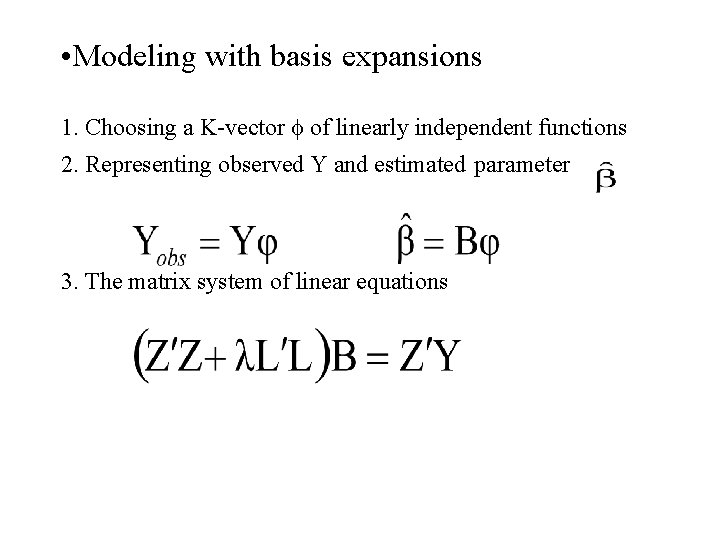
• Modeling with basis expansions 1. Choosing a K-vector of linearly independent functions 2. Representing observed Y and estimated parameter 3. The matrix system of linear equations
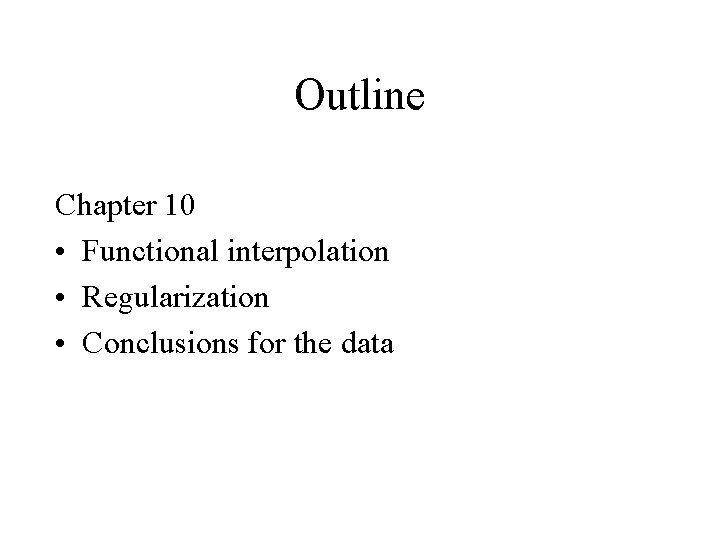
Outline Chapter 10 • Functional interpolation • Regularization • Conclusions for the data
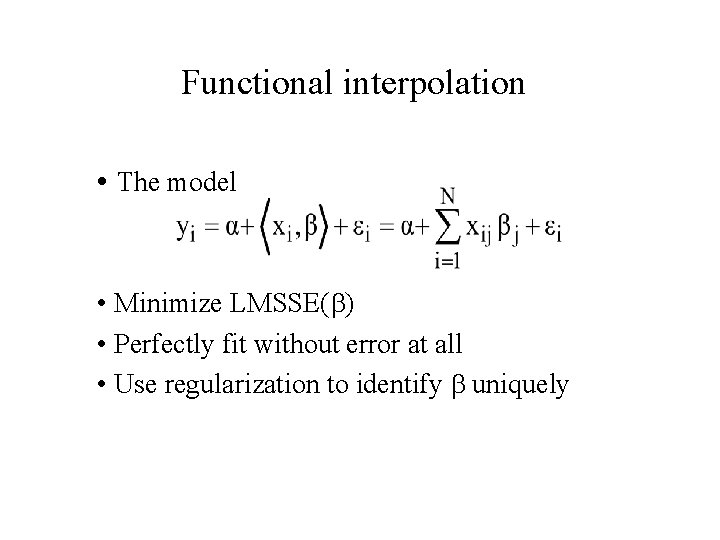
Functional interpolation • The model • Minimize LMSSE( ) • Perfectly fit without error at all • Use regularization to identify uniquely
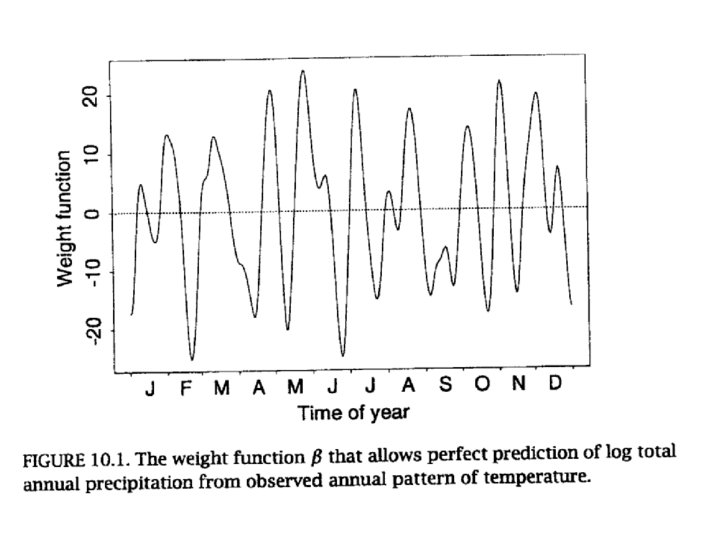
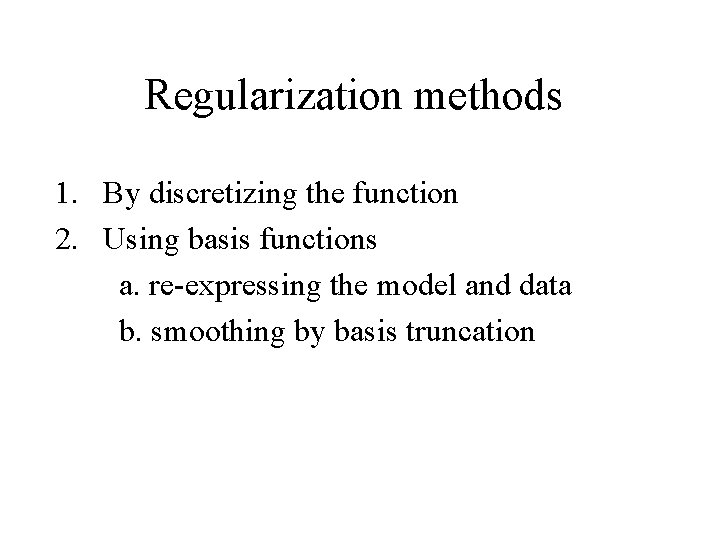
Regularization methods 1. By discretizing the function 2. Using basis functions a. re-expressing the model and data b. smoothing by basis truncation
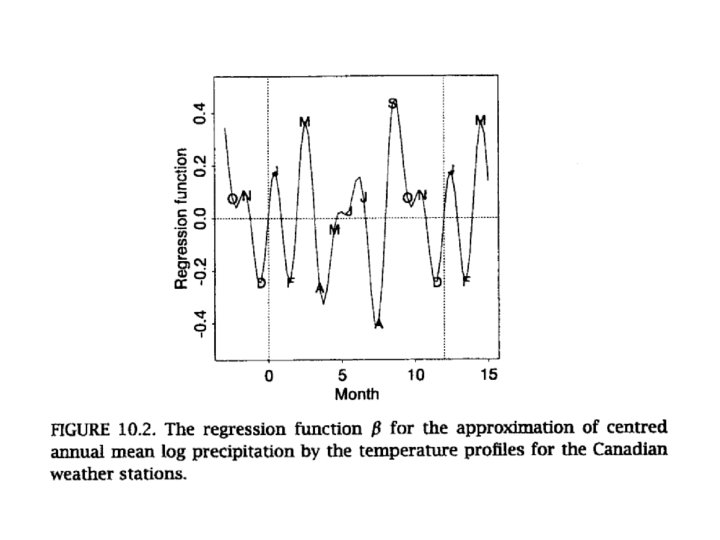
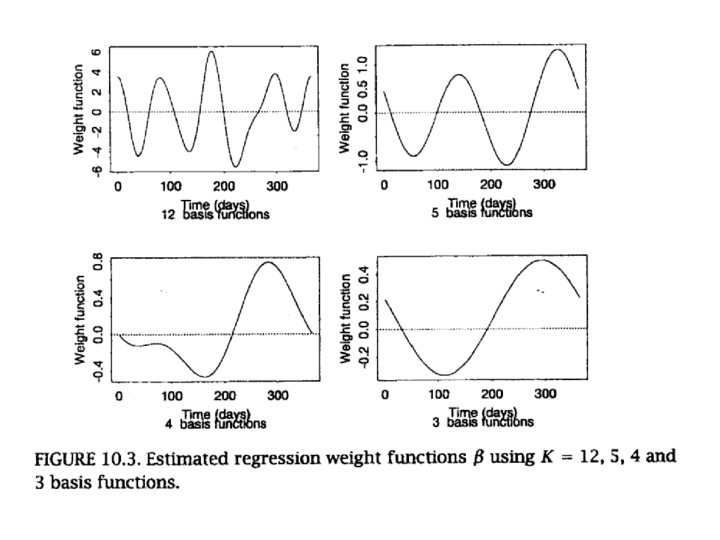
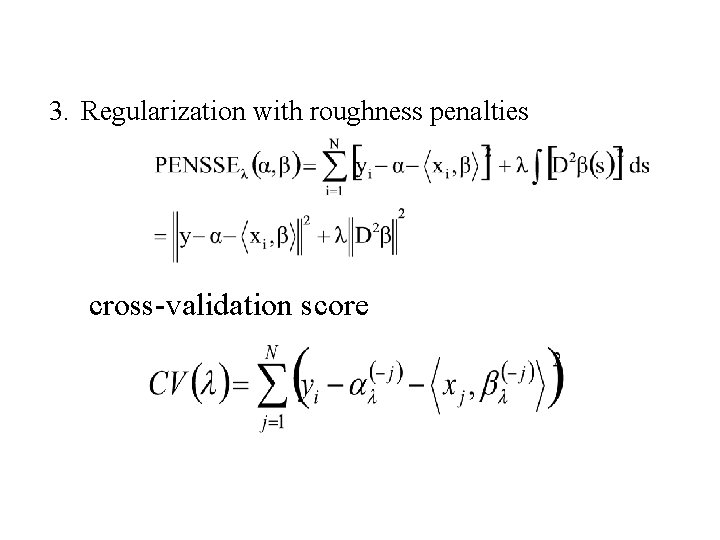
3. Regularization with roughness penalties cross-validation score
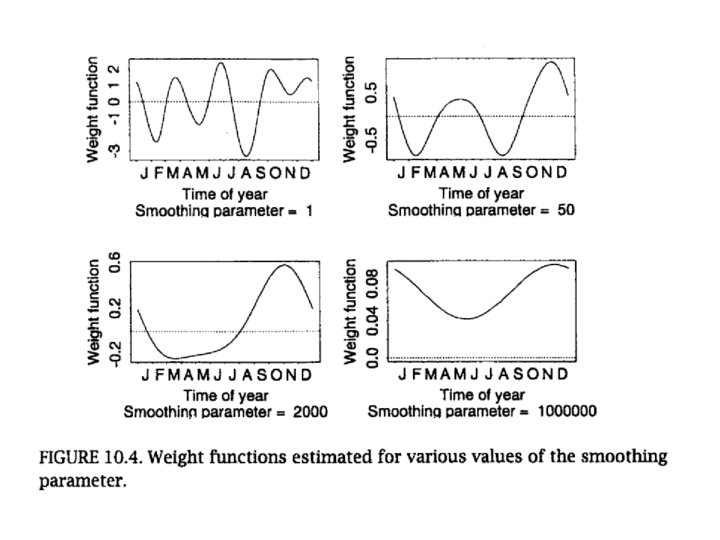
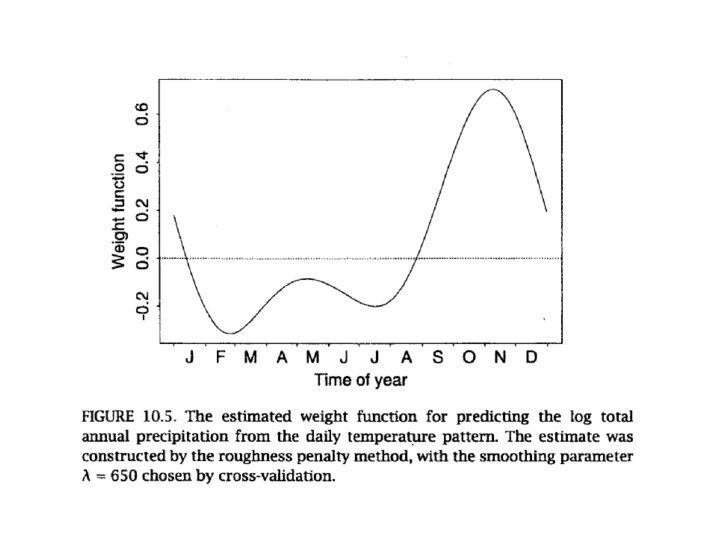
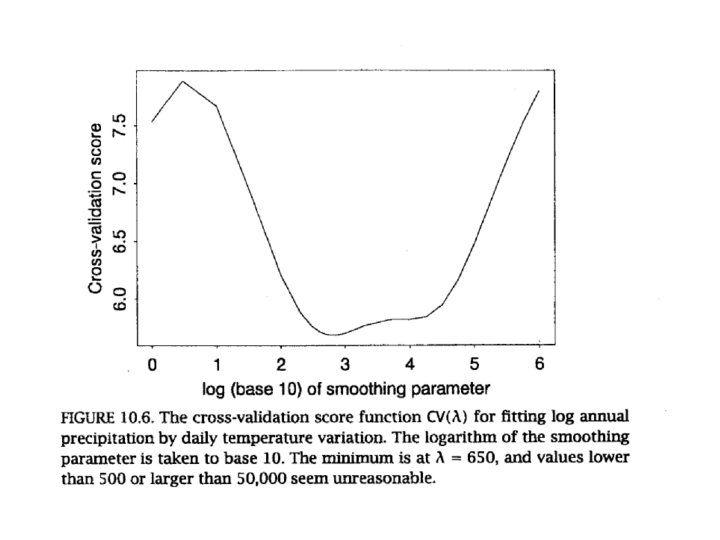
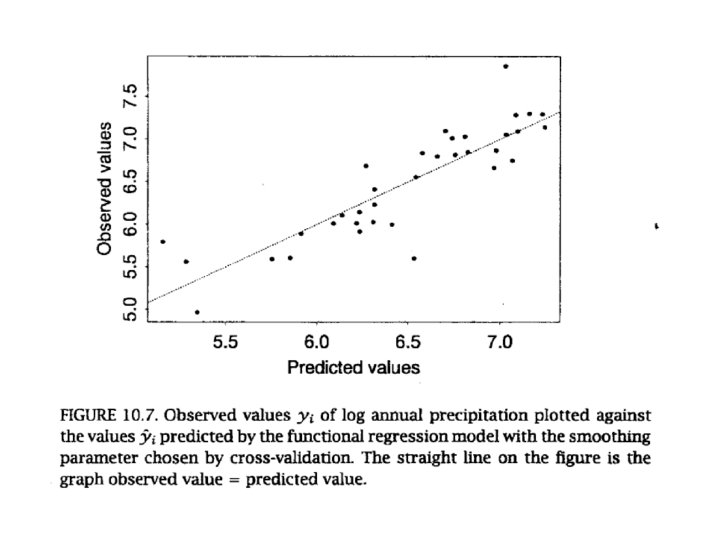
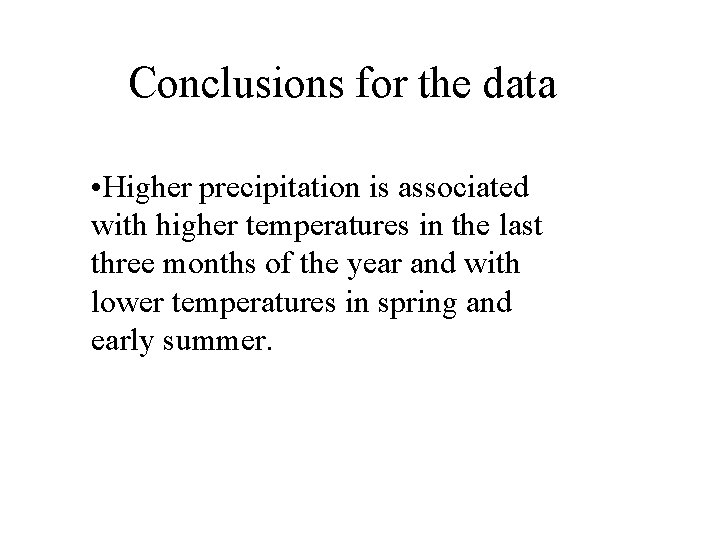
Conclusions for the data • Higher precipitation is associated with higher temperatures in the last three months of the year and with lower temperatures in spring and early summer.
Transpalatal arch space maintainer indications
Non functional plasma enzymes
Plasma enzyme
Functional and non functional
Functional forms of regression model
Semimodals
Nn models ideas
Ideas have consequences bad ideas have victims
Que es una idea principal
Use case primary and secondary actors
Use case extend
Jenis relasi yang bisa timbul pada use case diagram kecuali
Include extend use case
Leg rotation muscles
Which of these can be actors in a use case diagram
Java extend interface
Amphiarthroidal
Append and extend in python
Use case diagram
Caso de uso generalizacion
Retrocollis
Electric field lines about a point charge extend
Renpy games
Toolvoid
Diagrama de casos de uso include y extend