Does Russian have full paradigms Laura A Janda
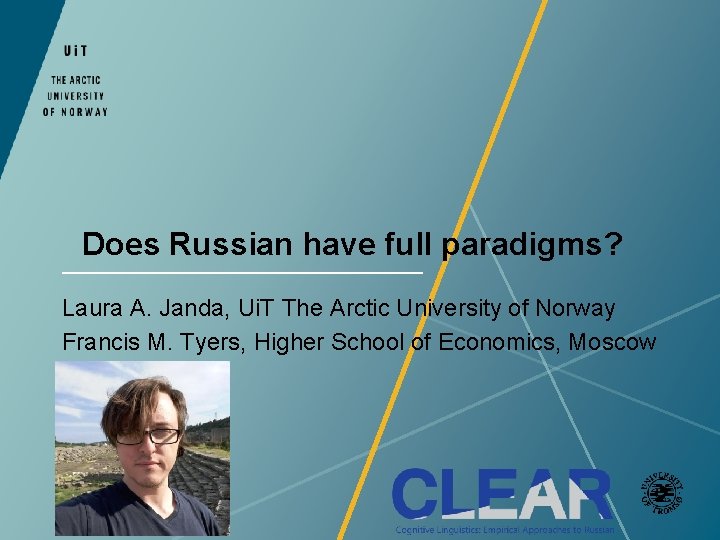
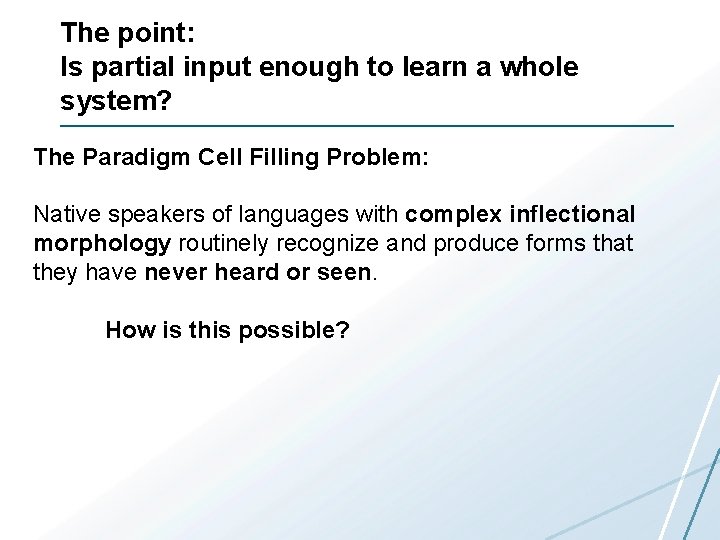
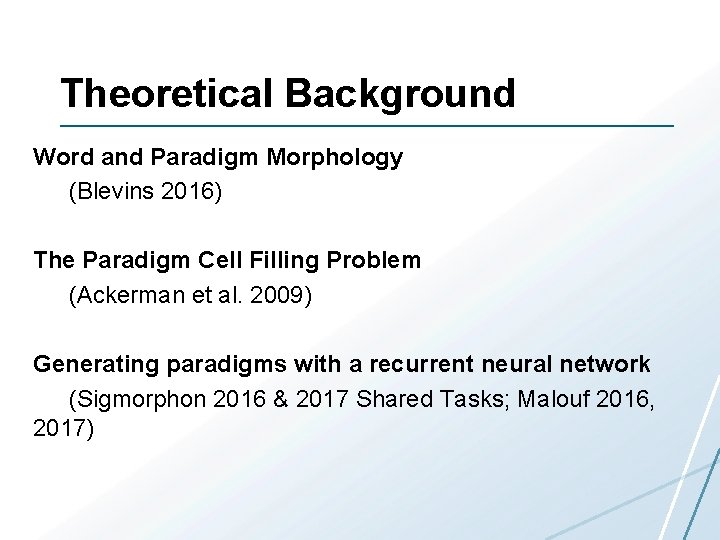
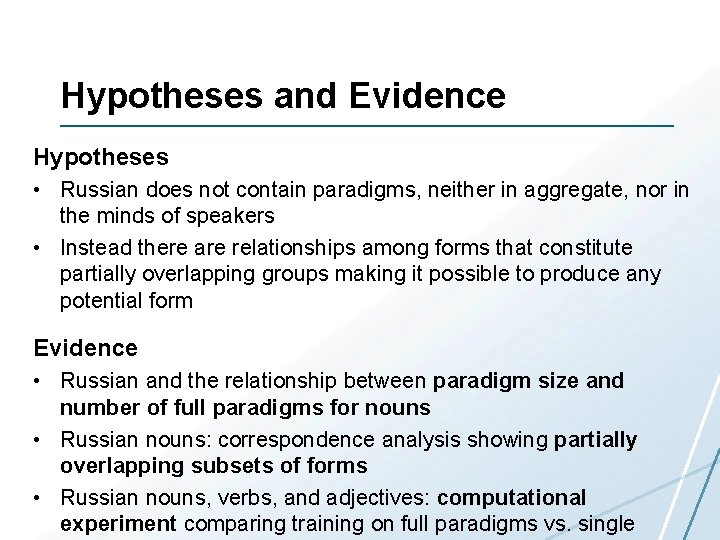
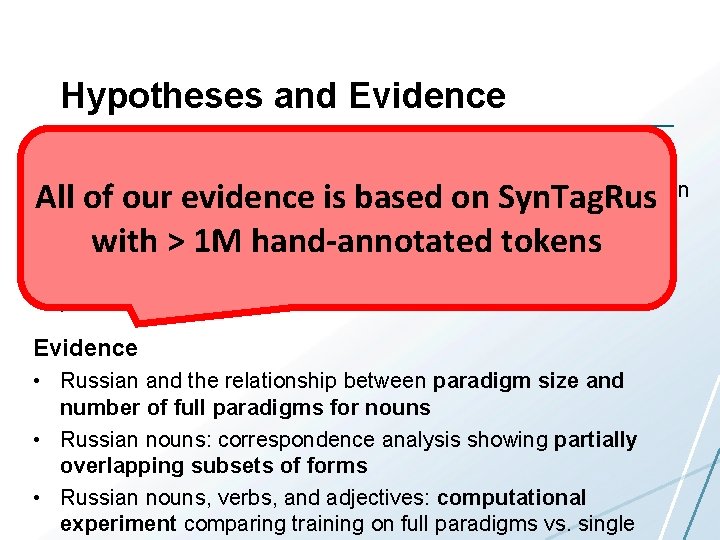
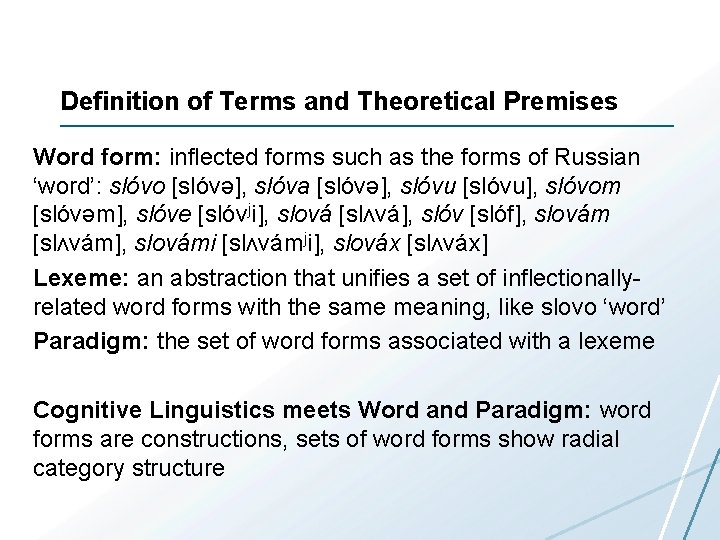
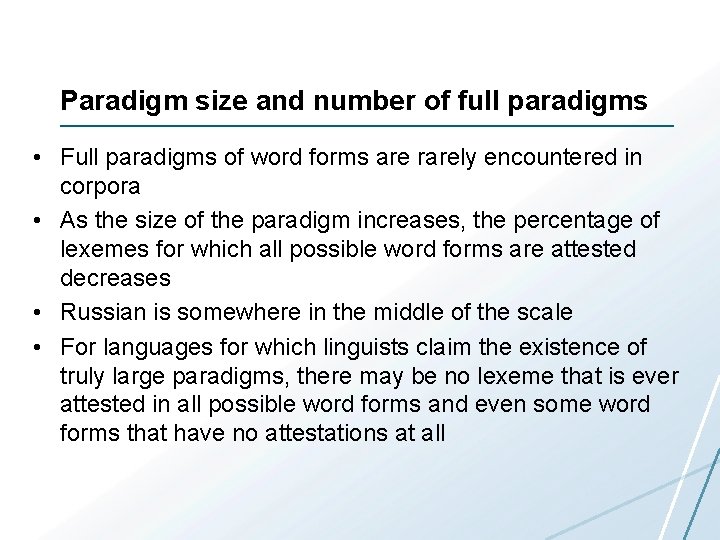
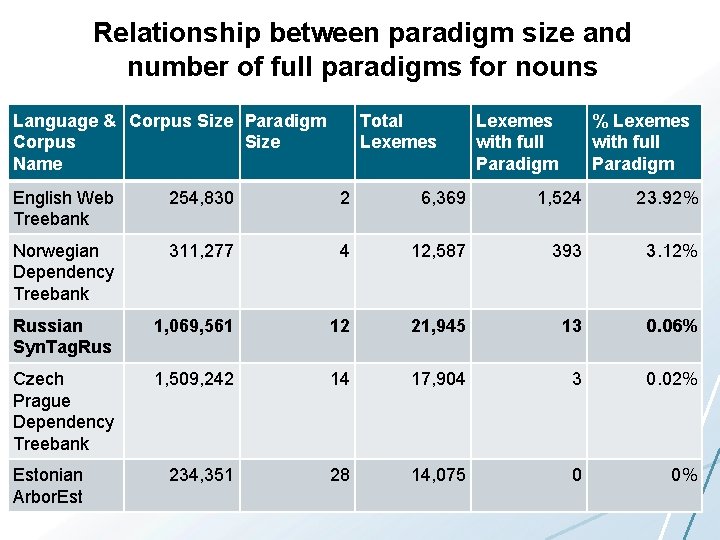
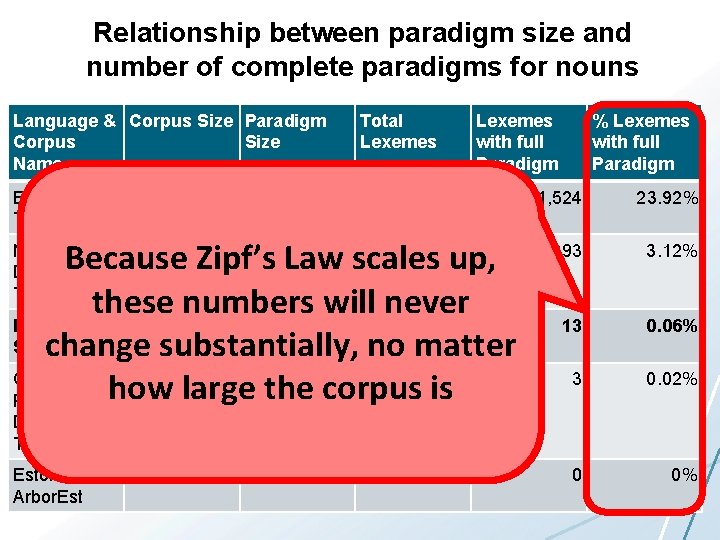
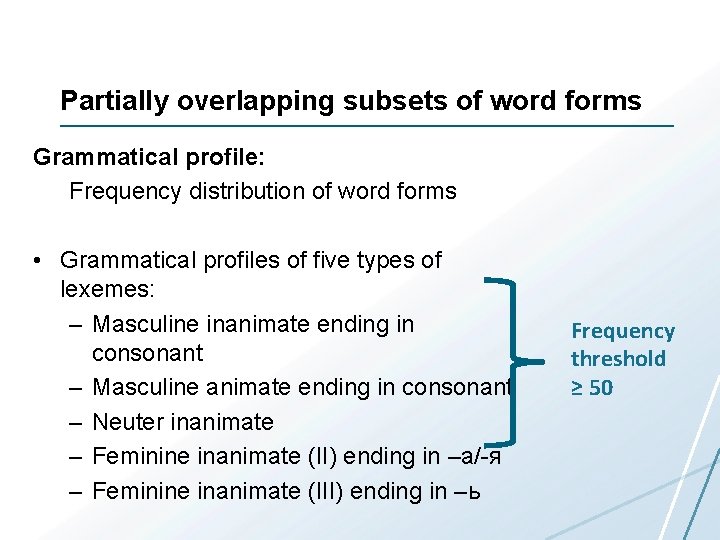
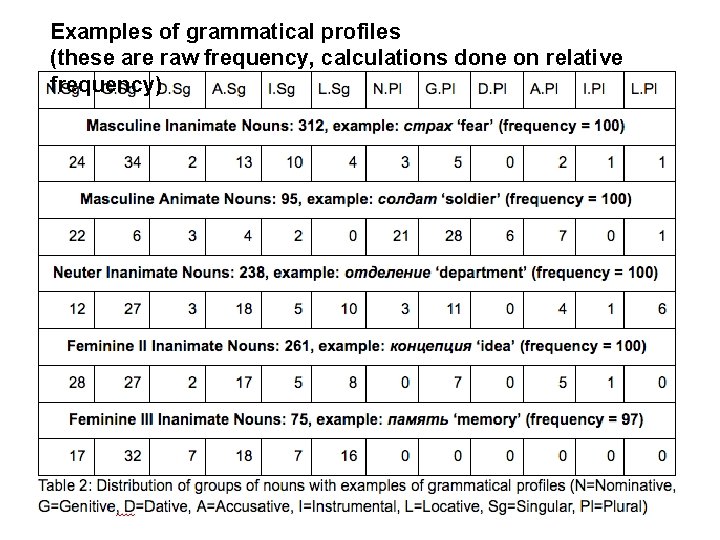
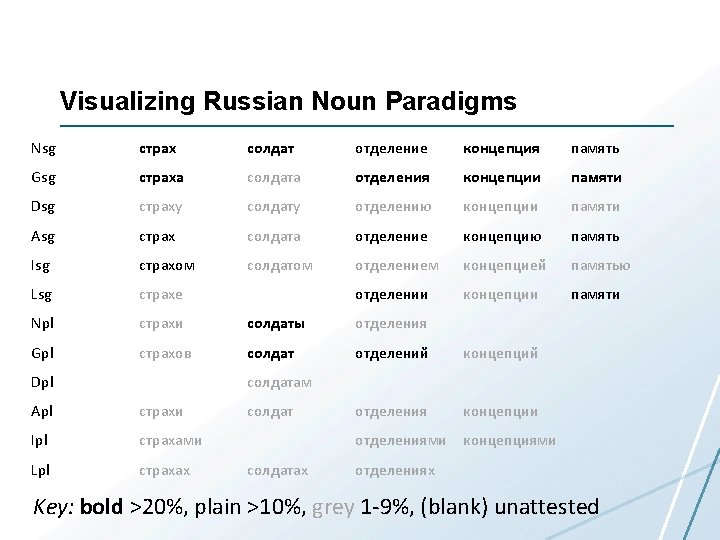
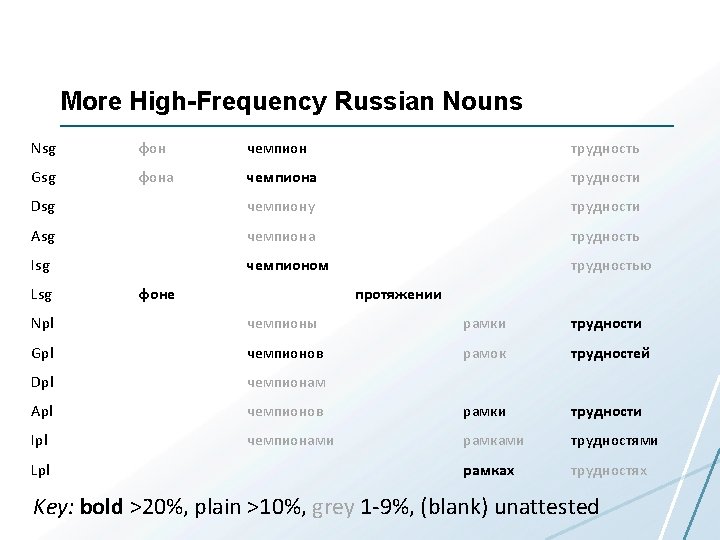
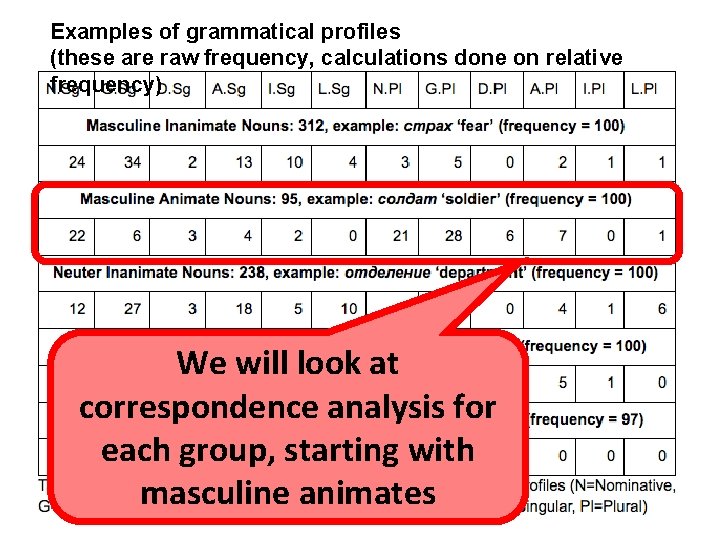
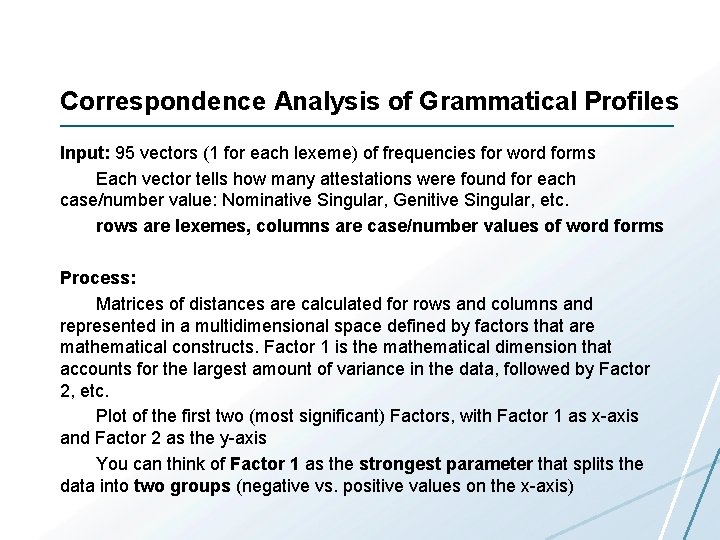
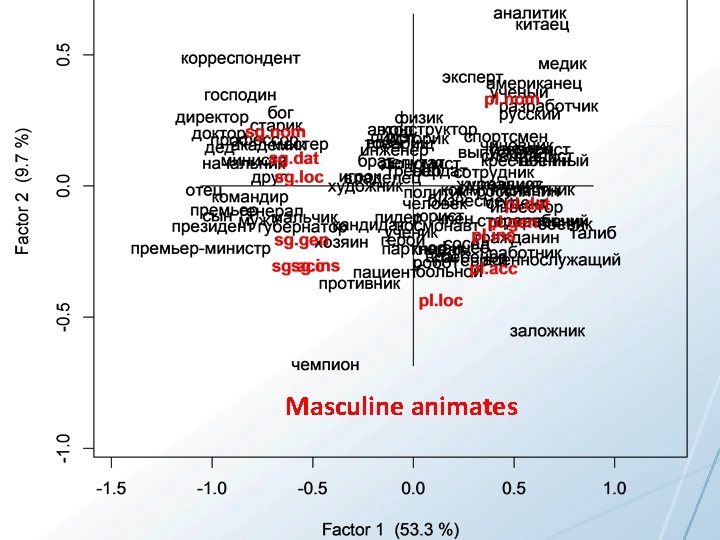
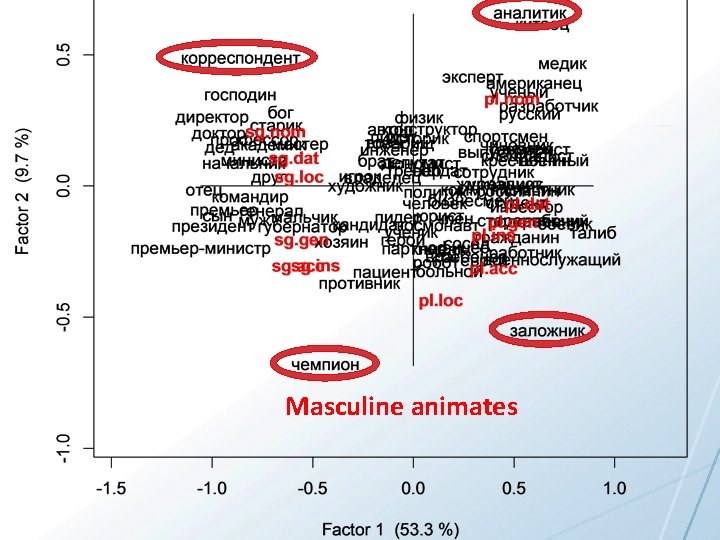
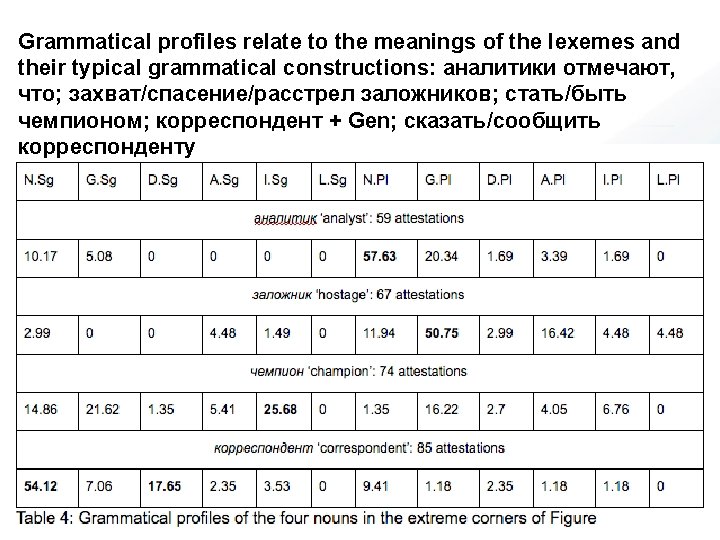
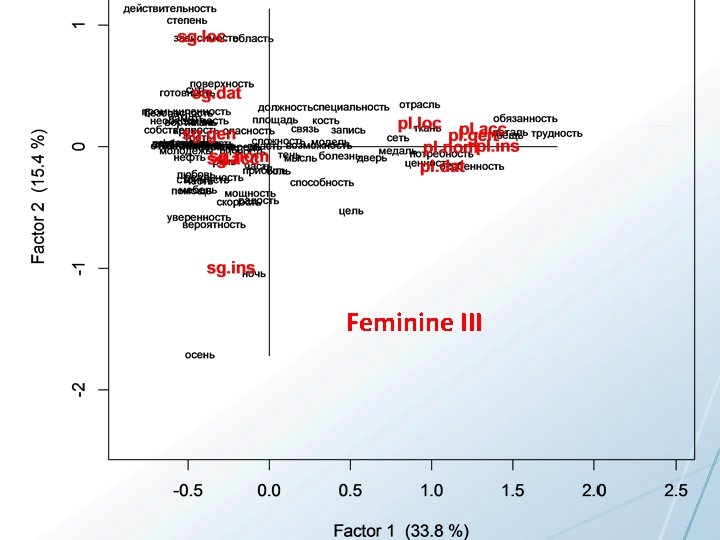
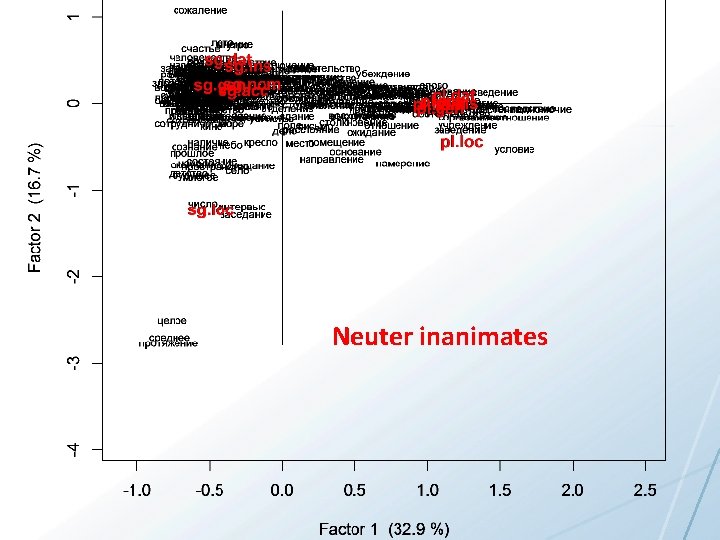
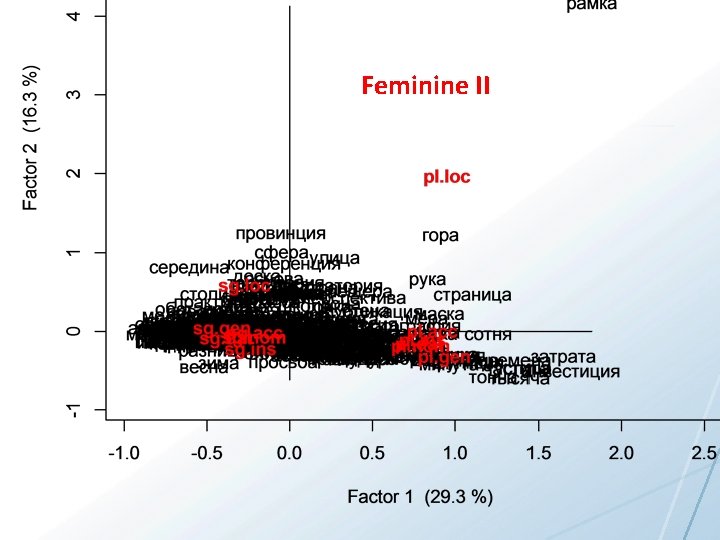
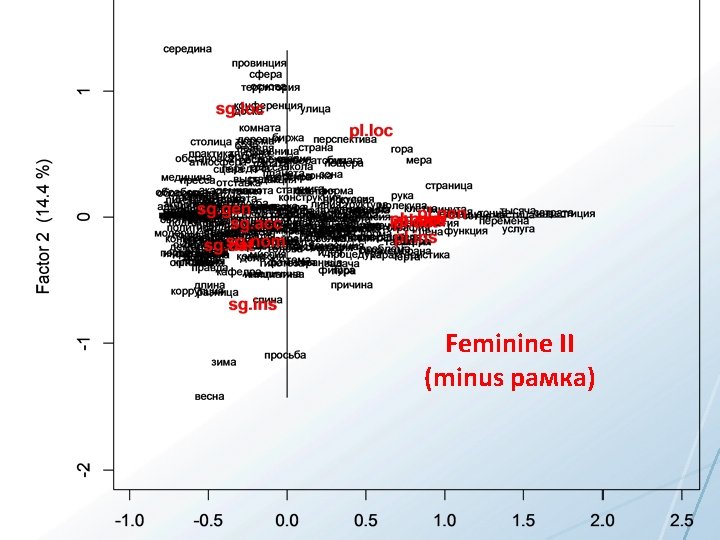
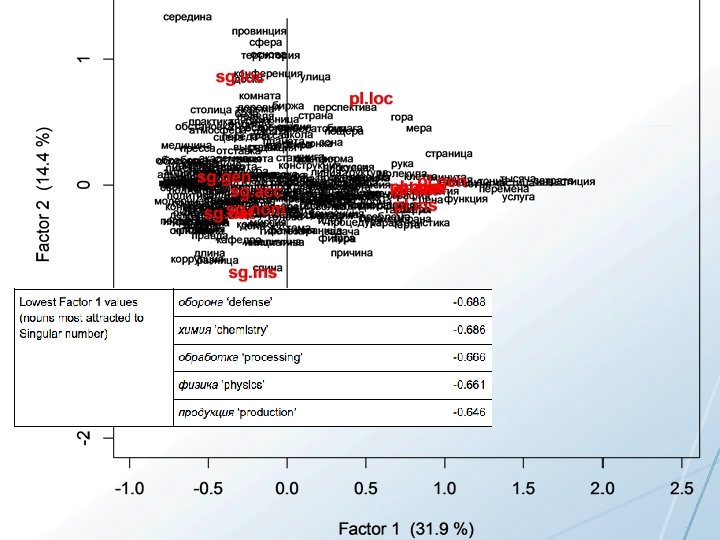
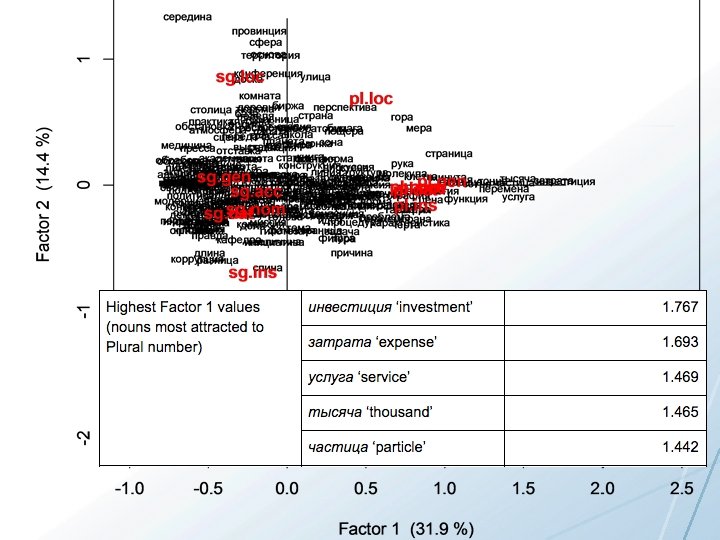
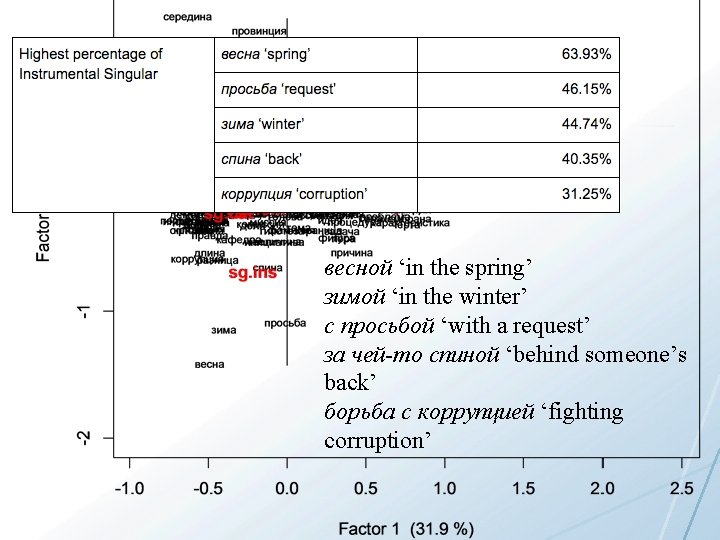
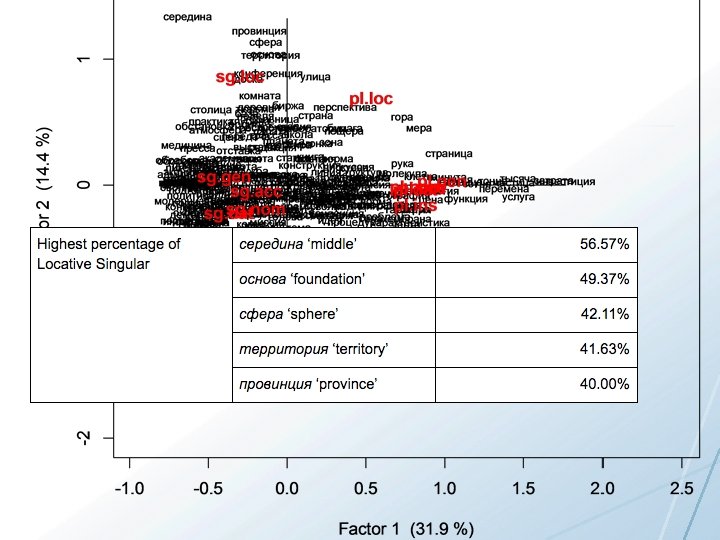
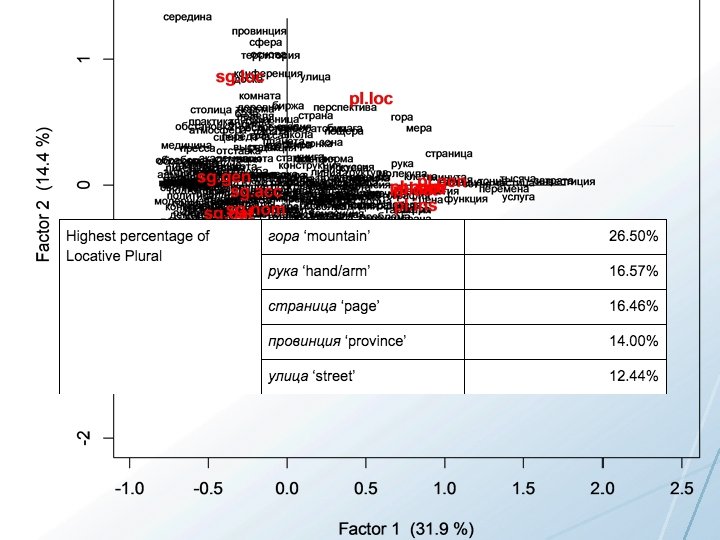
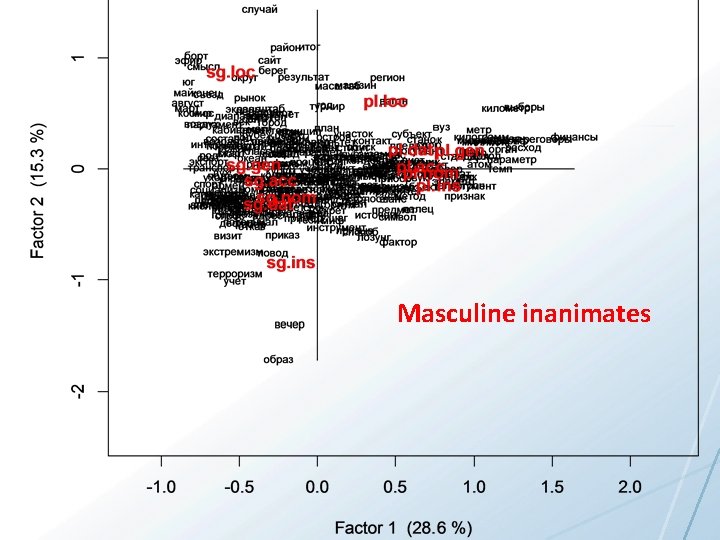
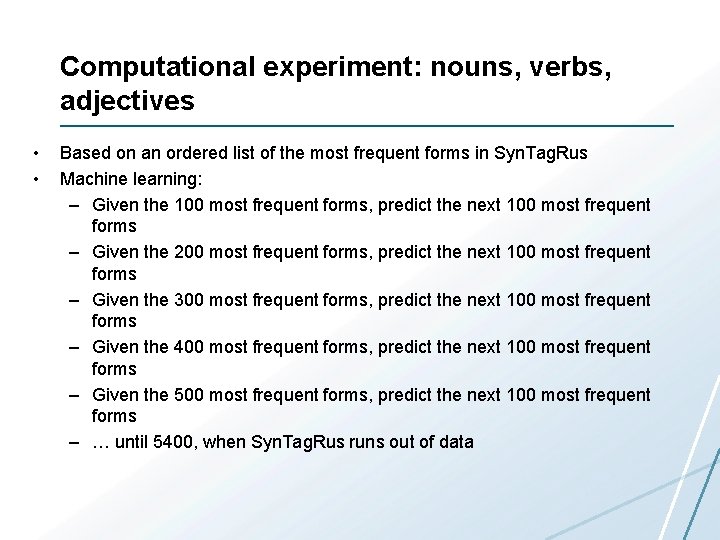
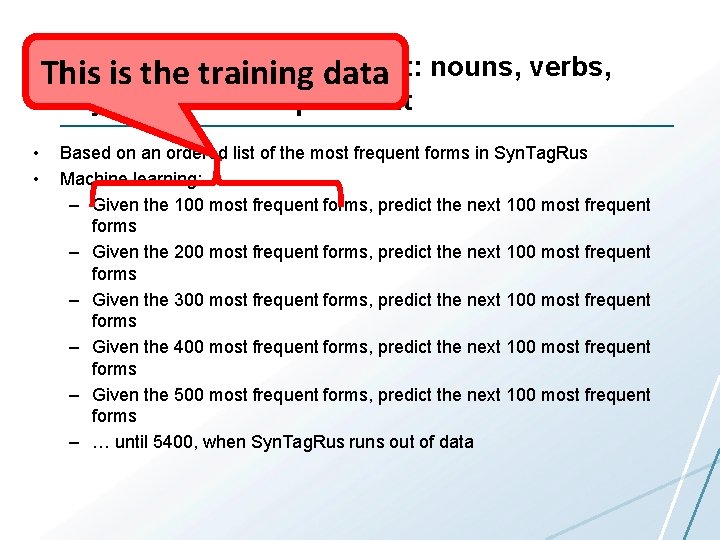
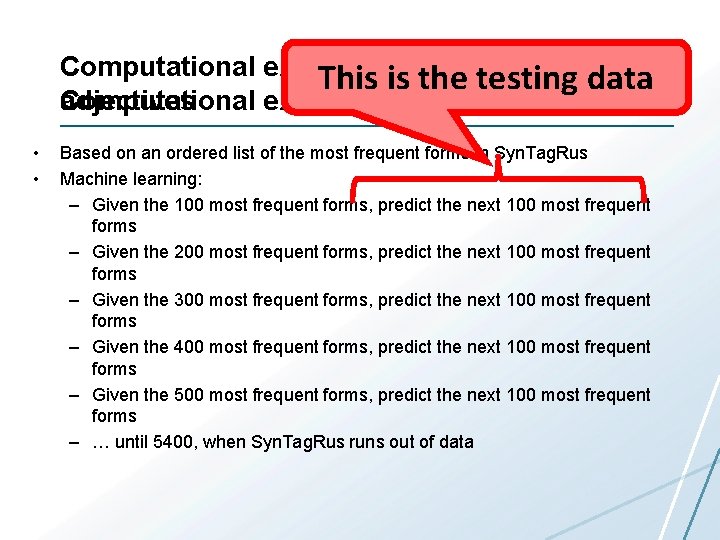
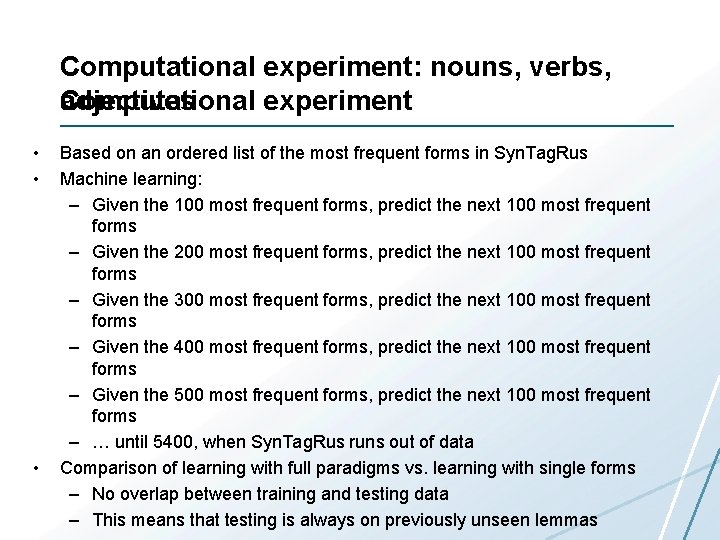
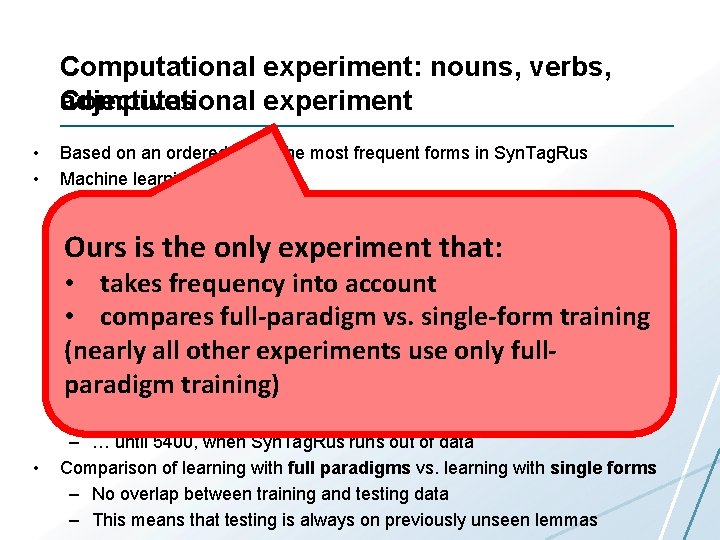
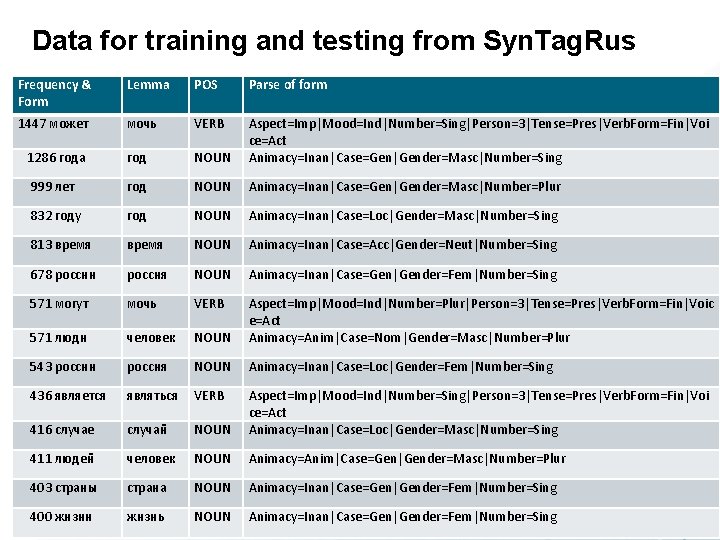
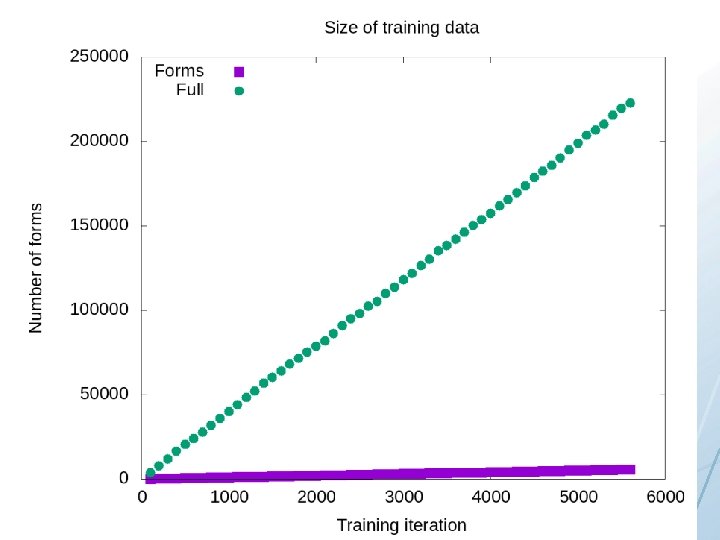
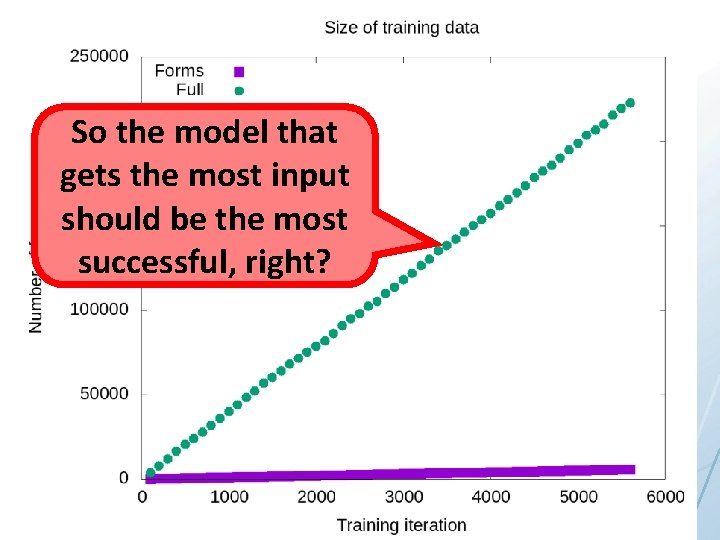
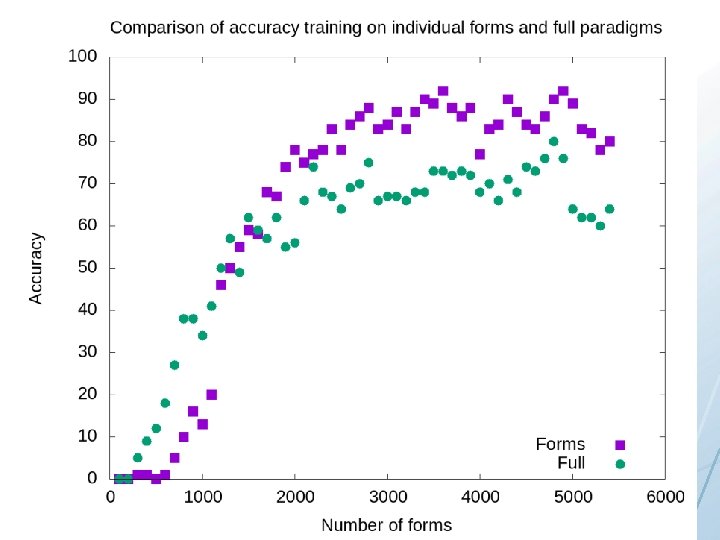
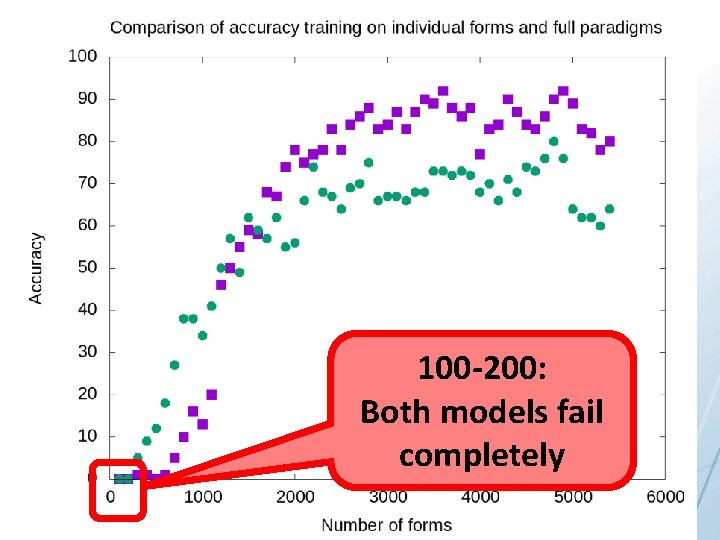
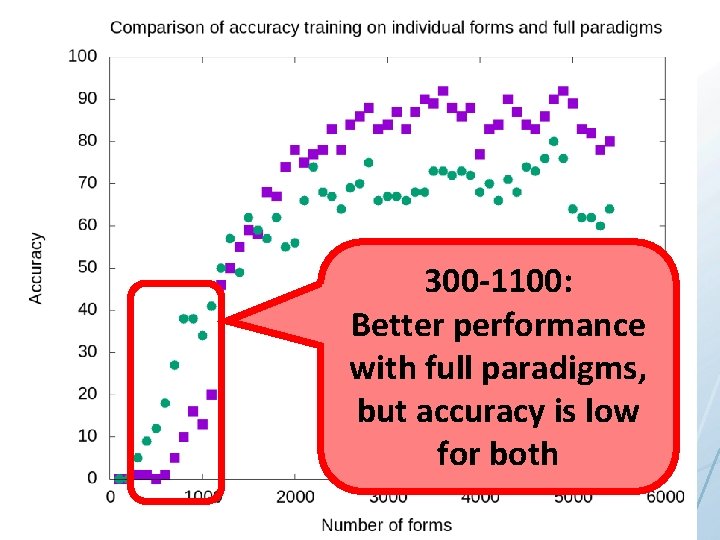
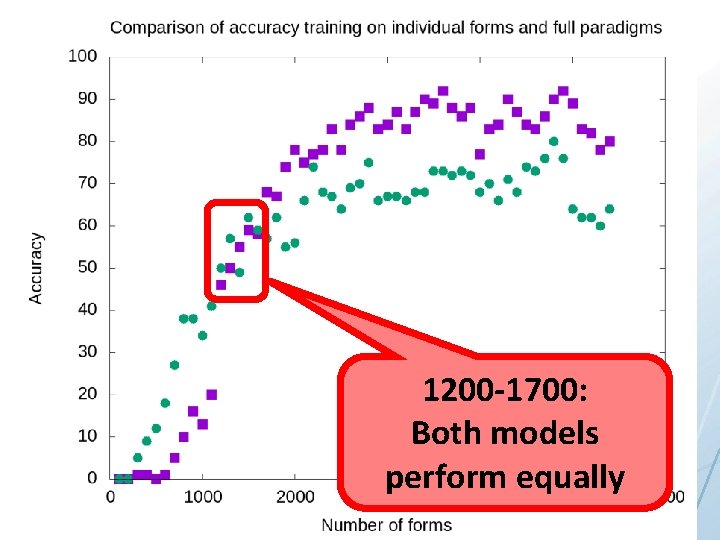
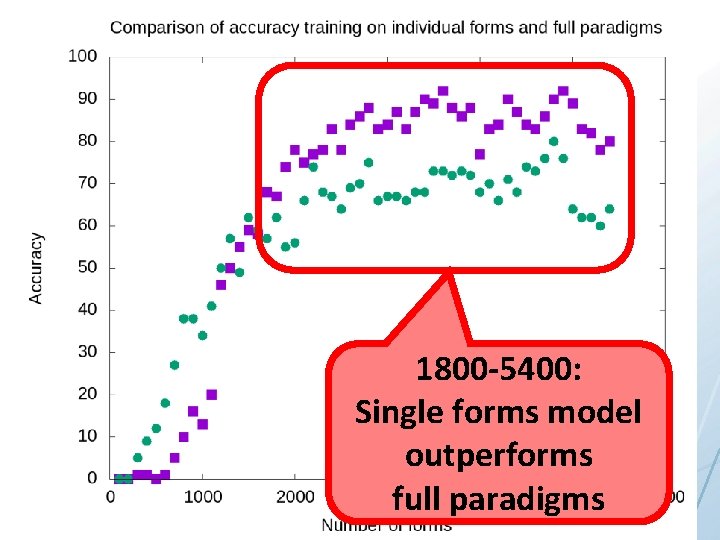
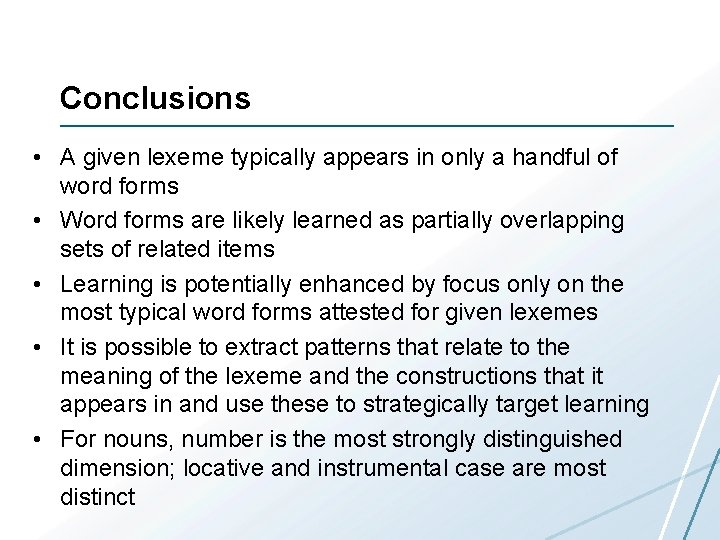
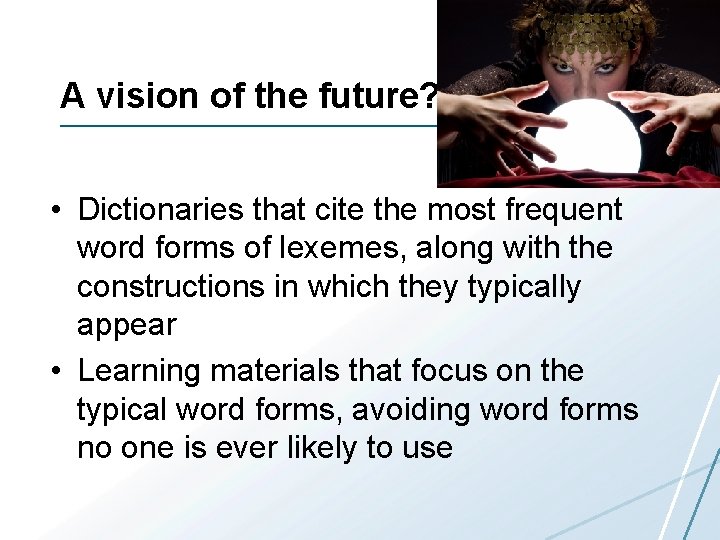
- Slides: 43
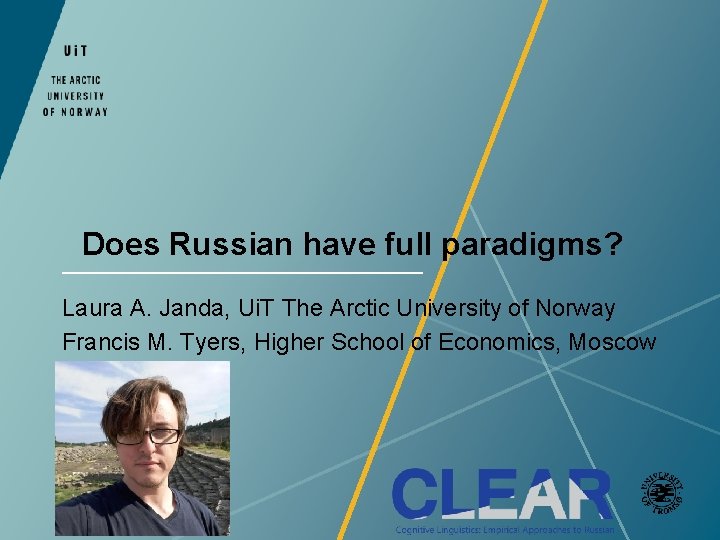
Does Russian have full paradigms? Laura A. Janda, Ui. T The Arctic University of Norway Francis M. Tyers, Higher School of Economics, Moscow
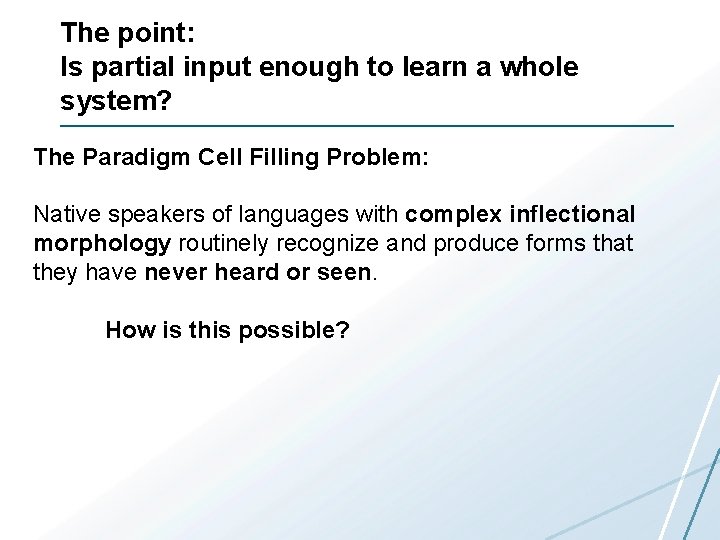
The point: Is partial input enough to learn a whole system? The Paradigm Cell Filling Problem: Native speakers of languages with complex inflectional morphology routinely recognize and produce forms that they have never heard or seen. How is this possible?
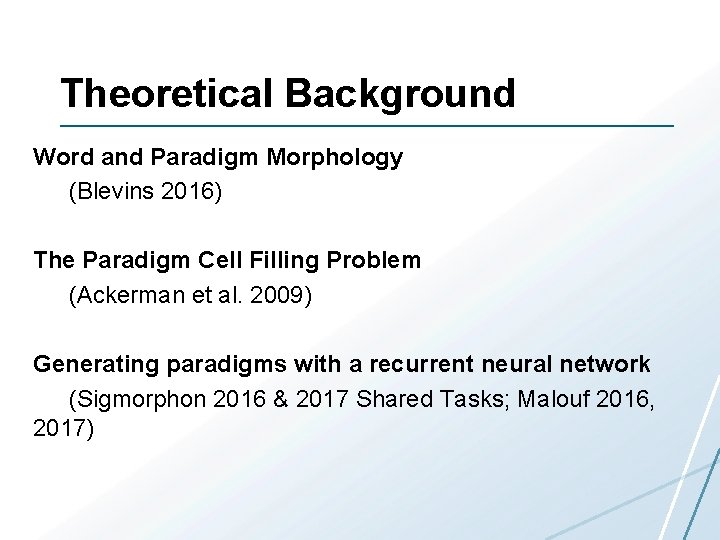
Theoretical Background Word and Paradigm Morphology (Blevins 2016) The Paradigm Cell Filling Problem (Ackerman et al. 2009) Generating paradigms with a recurrent neural network (Sigmorphon 2016 & 2017 Shared Tasks; Malouf 2016, 2017)
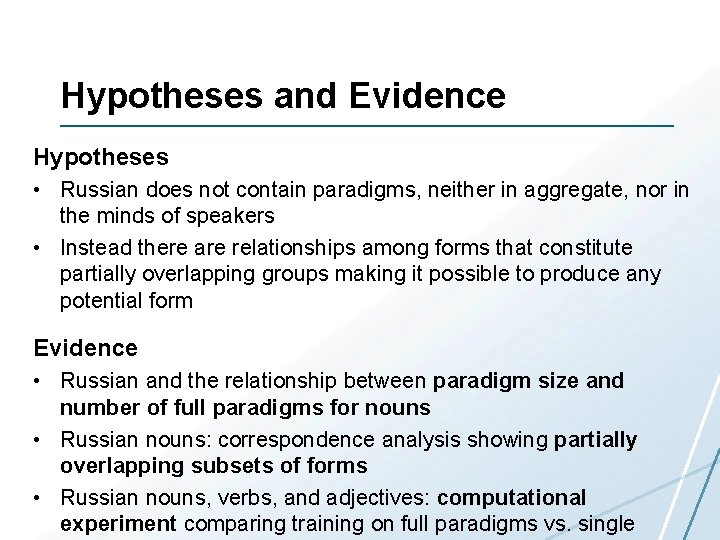
Hypotheses and Evidence Hypotheses • Russian does not contain paradigms, neither in aggregate, nor in the minds of speakers • Instead there are relationships among forms that constitute partially overlapping groups making it possible to produce any potential form Evidence • Russian and the relationship between paradigm size and number of full paradigms for nouns • Russian nouns: correspondence analysis showing partially overlapping subsets of forms • Russian nouns, verbs, and adjectives: computational experiment comparing training on full paradigms vs. single
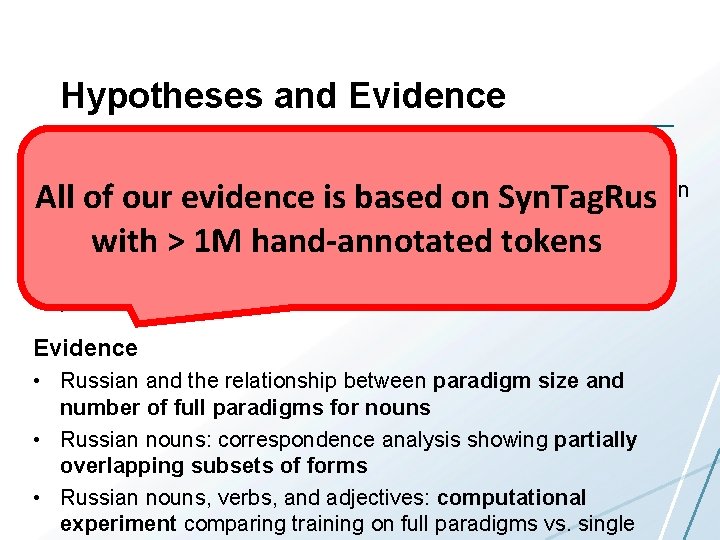
Hypotheses and Evidence Hypotheses All of our evidence is based on Syn. Tag. Rus with > 1 M hand-annotated tokens • Russian does not contain paradigms, neither in aggregate, nor in the minds of speakers • Instead there are relationships among forms that constitute partially overlapping groups making it possible to guess any potential form Evidence • Russian and the relationship between paradigm size and number of full paradigms for nouns • Russian nouns: correspondence analysis showing partially overlapping subsets of forms • Russian nouns, verbs, and adjectives: computational experiment comparing training on full paradigms vs. single
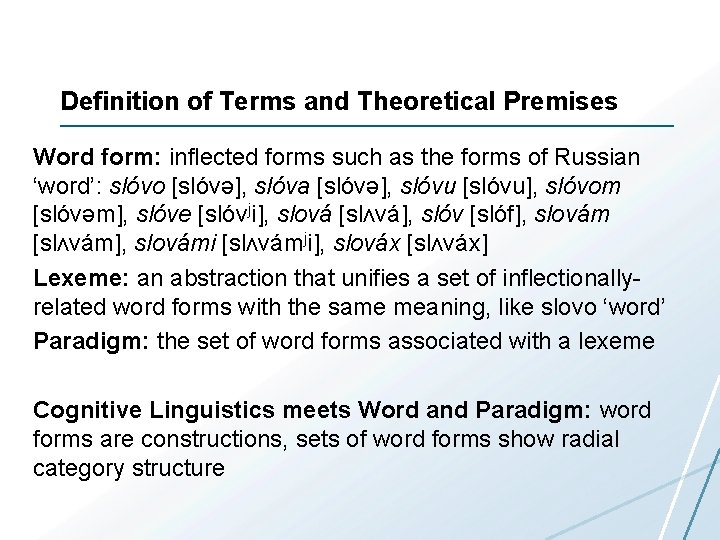
Definition of Terms and Theoretical Premises Word form: inflected forms such as the forms of Russian ‘word’: slóvo [slóvǝ], slóva [slóvǝ], slóvu [slóvu], slóvom [slóvǝm], slóve [slóvji], slová [slʌvá], slóv [slóf], slovám [slʌvám], slovámi [slʌvámji], slováx [slʌváx] Lexeme: an abstraction that unifies a set of inflectionallyrelated word forms with the same meaning, like slovo ‘word’ Paradigm: the set of word forms associated with a lexeme Cognitive Linguistics meets Word and Paradigm: word forms are constructions, sets of word forms show radial category structure
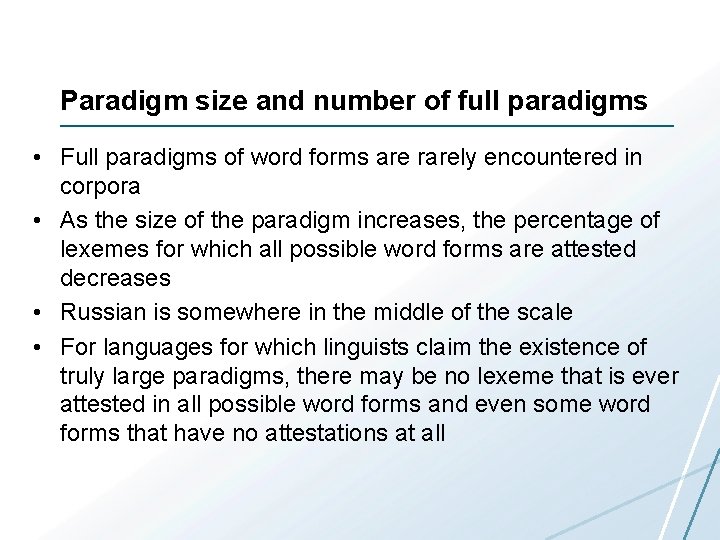
Paradigm size and number of full paradigms • Full paradigms of word forms are rarely encountered in corpora • As the size of the paradigm increases, the percentage of lexemes for which all possible word forms are attested decreases • Russian is somewhere in the middle of the scale • For languages for which linguists claim the existence of truly large paradigms, there may be no lexeme that is ever attested in all possible word forms and even some word forms that have no attestations at all
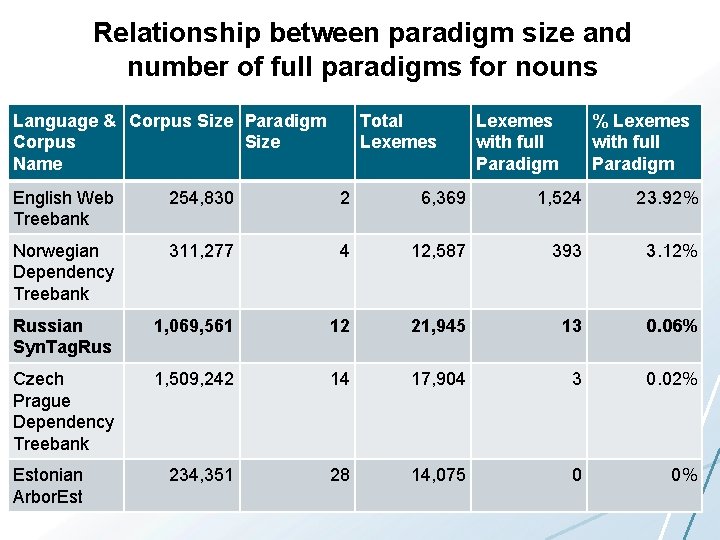
Relationship between paradigm size and number of full paradigms for nouns Language & Corpus Size Paradigm Corpus Size Name Total Lexemes with full Paradigm % Lexemes with full Paradigm English Web Treebank 254, 830 2 6, 369 1, 524 23. 92% Norwegian Dependency Treebank 311, 277 4 12, 587 393 3. 12% Russian Syn. Tag. Rus 1, 069, 561 12 21, 945 13 0. 06% Czech Prague Dependency Treebank 1, 509, 242 14 17, 904 3 0. 02% 234, 351 28 14, 075 0 0% Estonian Arbor. Est
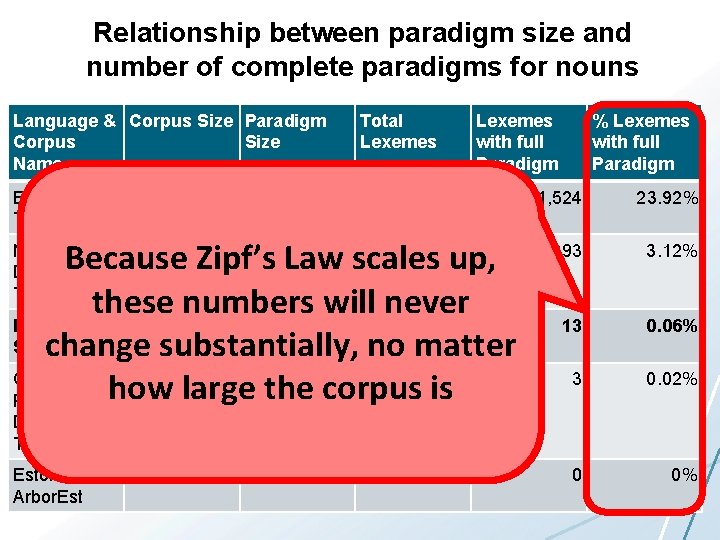
Relationship between paradigm size and number of complete paradigms for nouns Language & Corpus Size Paradigm Corpus Size Name English Web Treebank 254, 830 Total Lexemes 2 Lexemes with full Paradigm 6, 369 311, 277 12, 587 Because Zipf’s Law 4 scales up, these numbers will never Russian 1, 032, 644 12 21, 945 Syn. Tag. Rus change substantially, no matter Czech 1, 509, 242 14 17, 904 how large the corpus is Prague Norwegian Dependency Treebank % Lexemes with full Paradigm 1, 524 23. 92% 393 3. 12% 13 0. 06% 3 0. 02% 0 0% Dependency Treebank Estonian Arbor. Est 234, 351 28 14, 075
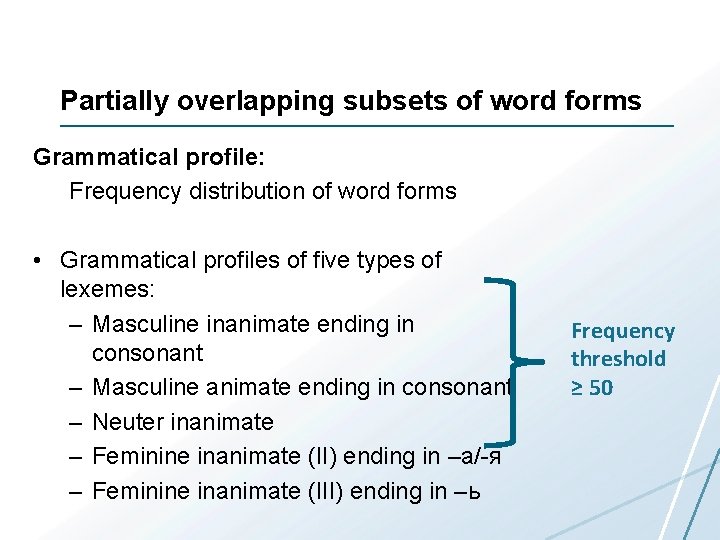
Partially overlapping subsets of word forms Grammatical profile: Frequency distribution of word forms • Grammatical profiles of five types of lexemes: – Masculine inanimate ending in consonant – Masculine animate ending in consonant – Neuter inanimate – Feminine inanimate (II) ending in –a/-я – Feminine inanimate (III) ending in –ь Frequency threshold ≥ 50
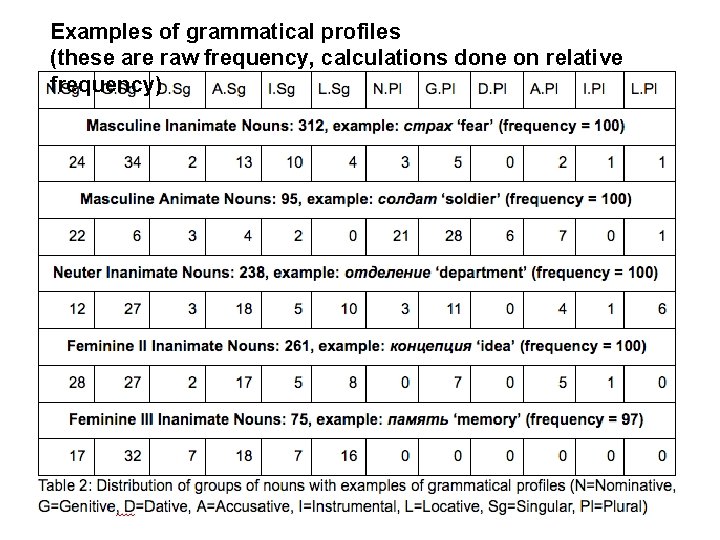
Examples of grammatical profiles (these are raw frequency, calculations done on relative frequency)
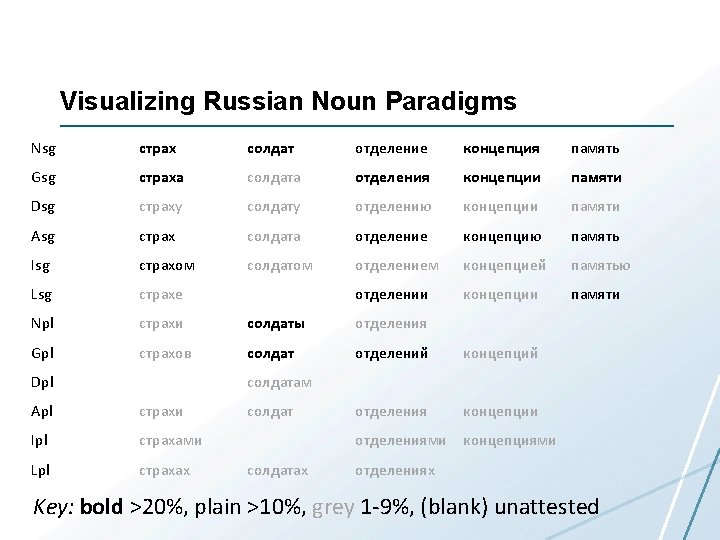
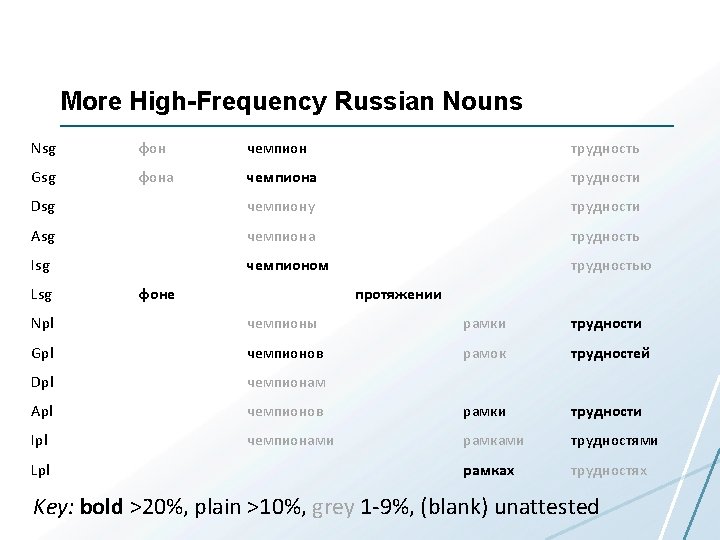
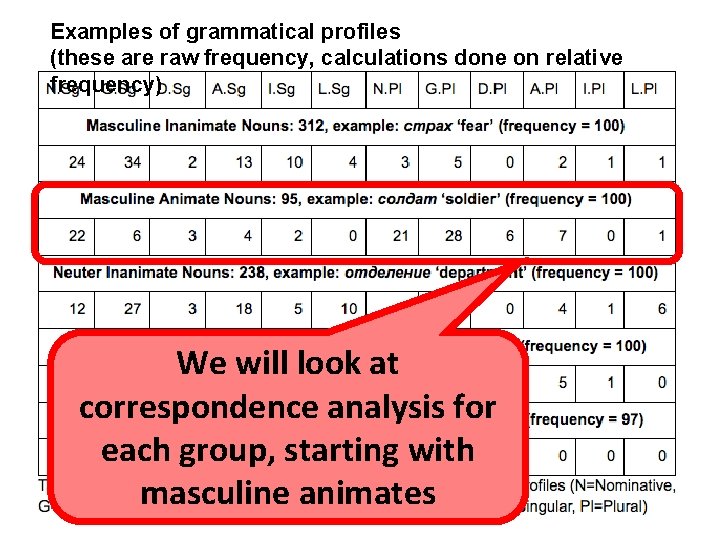
Examples of grammatical profiles (these are raw frequency, calculations done on relative frequency) We will look at correspondence analysis for each group, starting with masculine animates
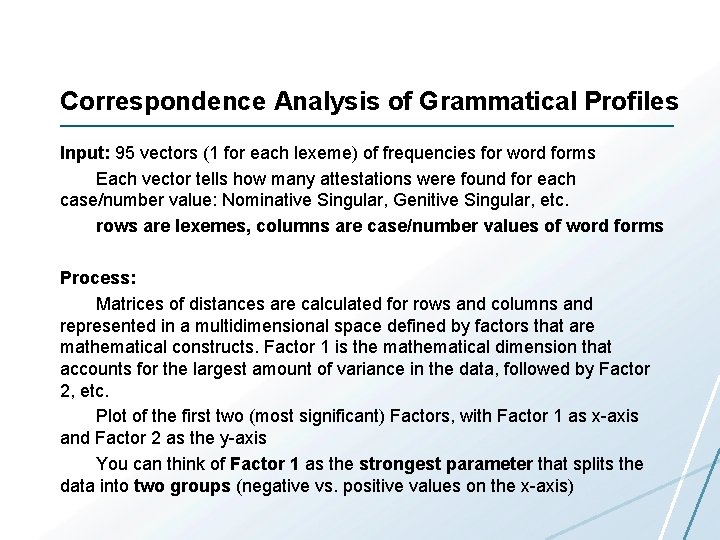
Correspondence Analysis of Grammatical Profiles Input: 95 vectors (1 for each lexeme) of frequencies for word forms Each vector tells how many attestations were found for each case/number value: Nominative Singular, Genitive Singular, etc. rows are lexemes, columns are case/number values of word forms Process: Matrices of distances are calculated for rows and columns and represented in a multidimensional space defined by factors that are mathematical constructs. Factor 1 is the mathematical dimension that accounts for the largest amount of variance in the data, followed by Factor 2, etc. Plot of the first two (most significant) Factors, with Factor 1 as x-axis and Factor 2 as the y-axis You can think of Factor 1 as the strongest parameter that splits the data into two groups (negative vs. positive values on the x-axis)
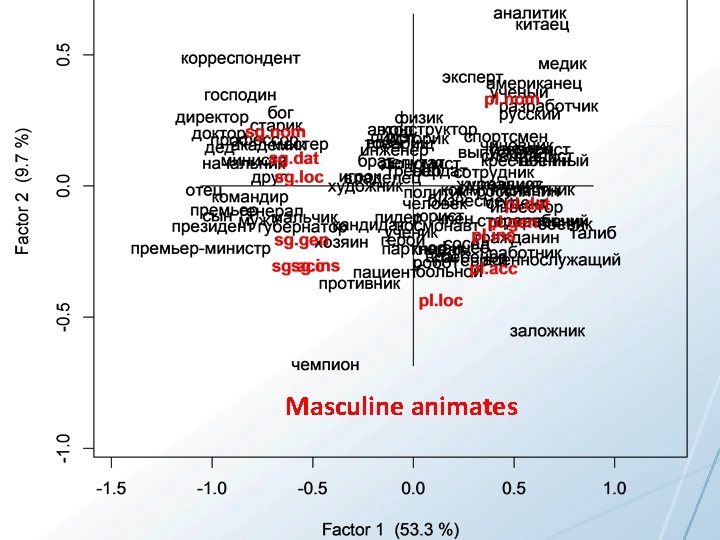
Masculine animates
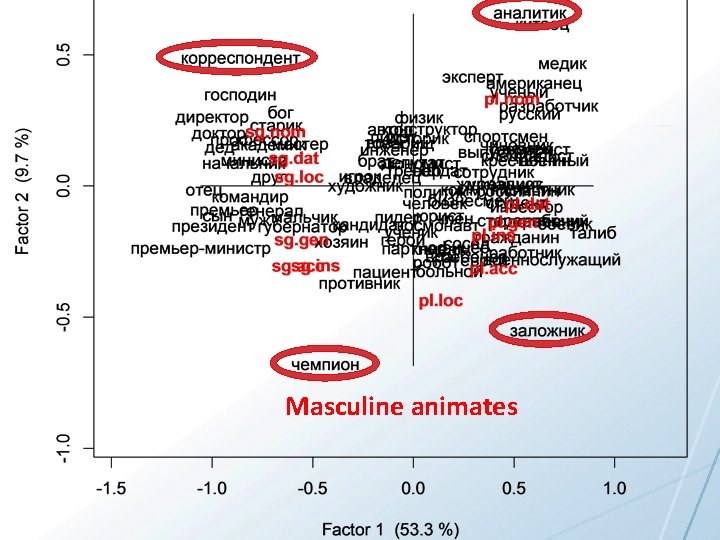
Masculine animates
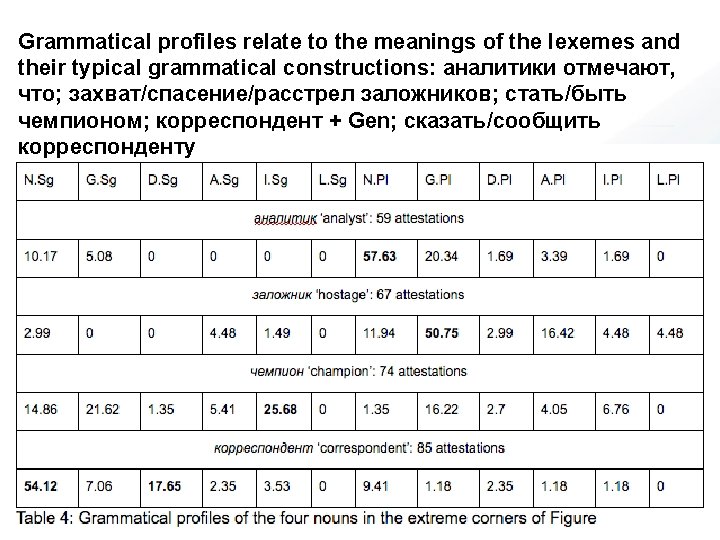
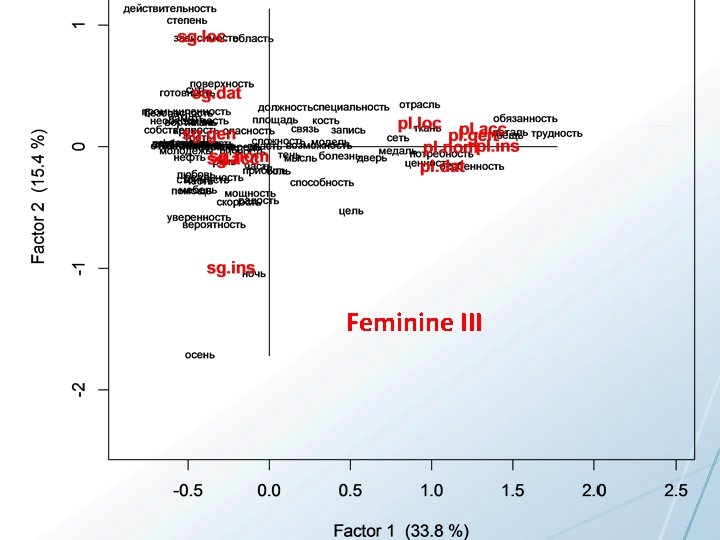
Feminine III
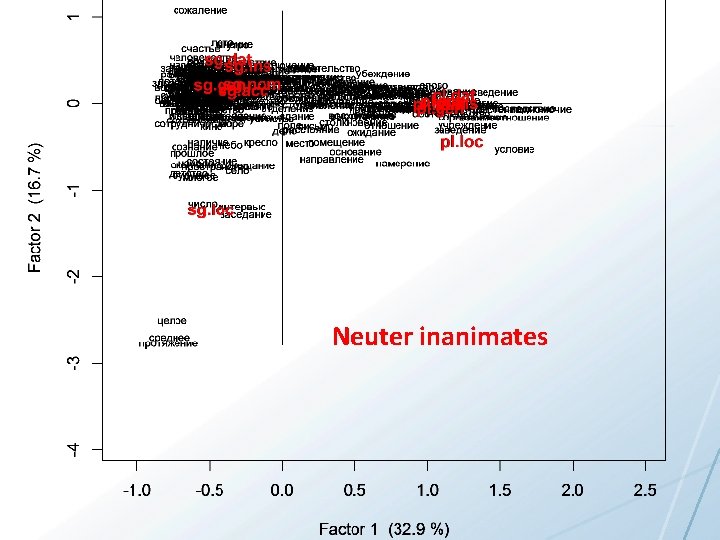
Neuter inanimates
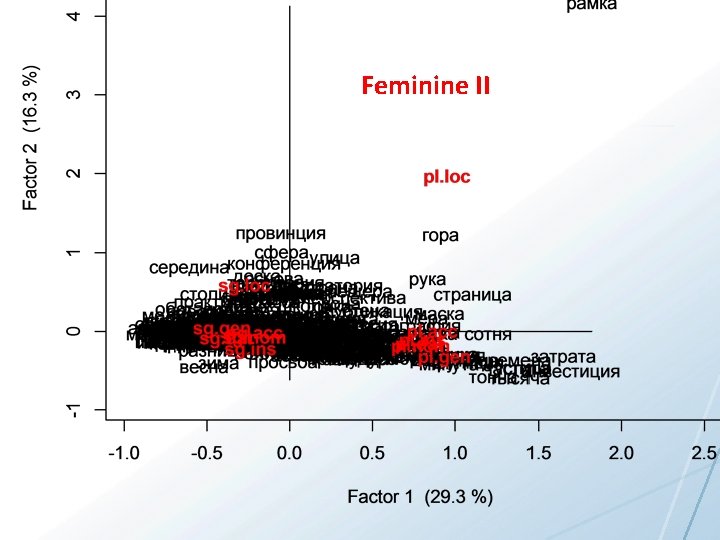
Feminine II
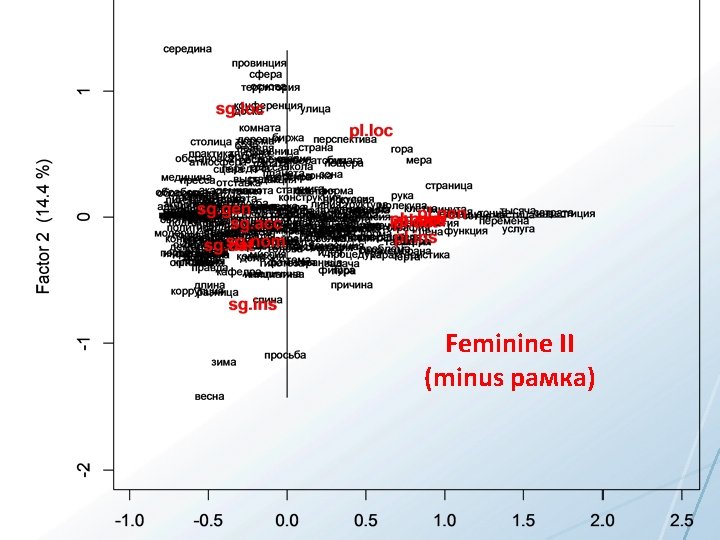
Feminine II (minus рамка)
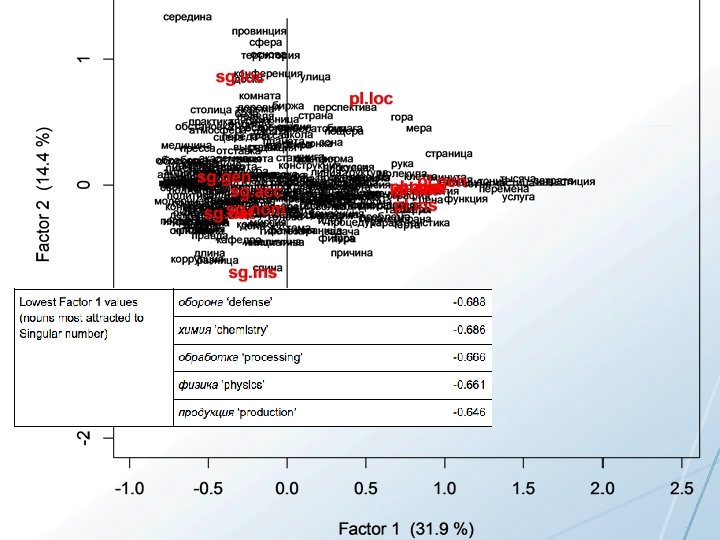
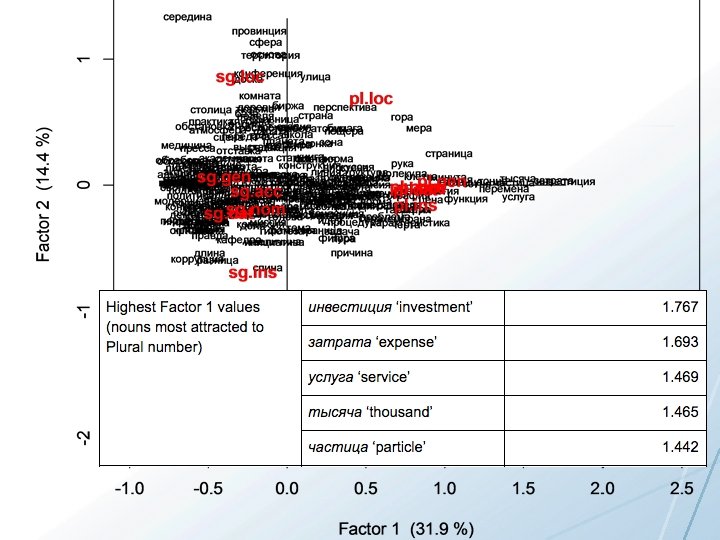
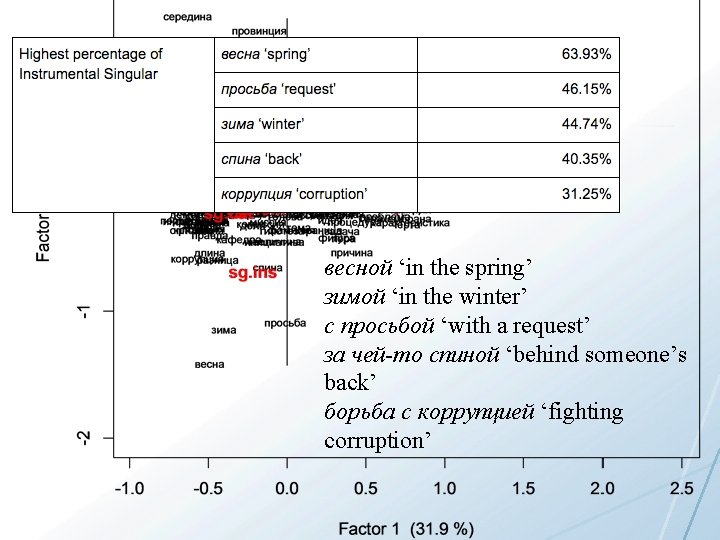
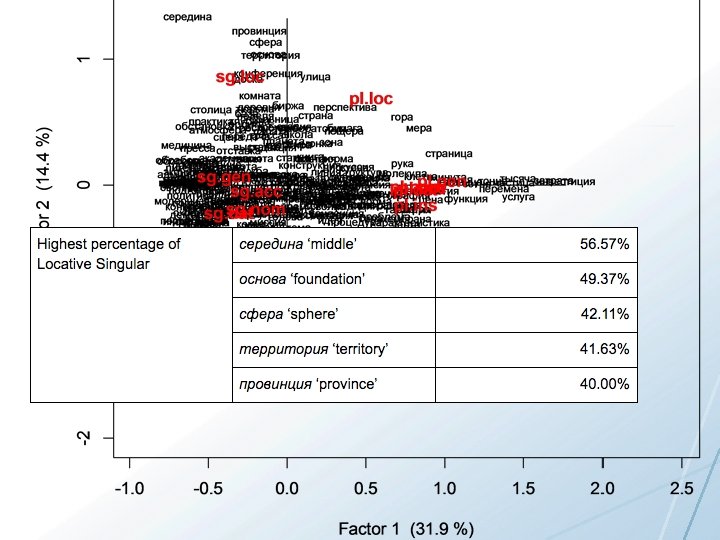
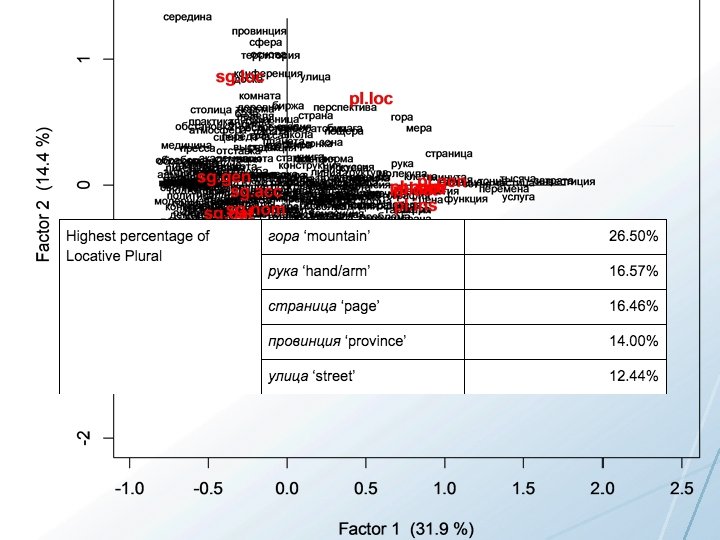
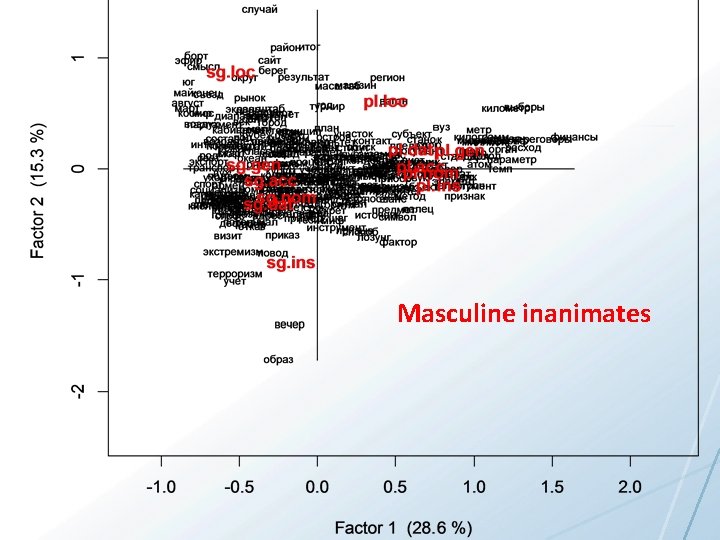
Masculine inanimates
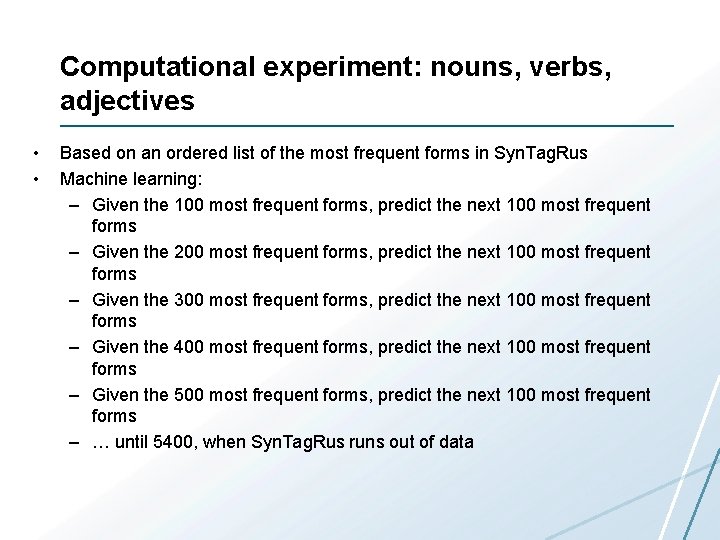
Computational experiment: nouns, verbs, adjectives • • Based on an ordered list of the most frequent forms in Syn. Tag. Rus Machine learning: – Given the 100 most frequent forms, predict the next 100 most frequent forms – Given the 200 most frequent forms, predict the next 100 most frequent forms – Given the 300 most frequent forms, predict the next 100 most frequent forms – Given the 400 most frequent forms, predict the next 100 most frequent forms – Given the 500 most frequent forms, predict the next 100 most frequent forms – … until 5400, when Syn. Tag. Rus runs out of data
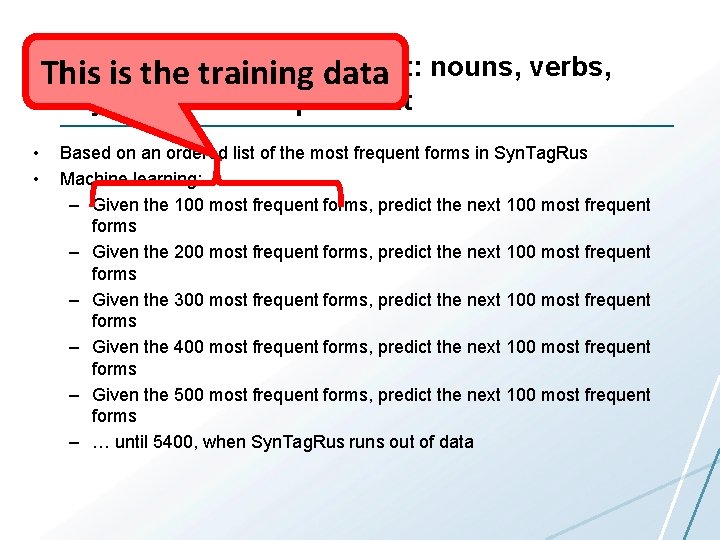
Computational experiment: This is the training data nouns, verbs, Computational experiment adjectives • • Based on an ordered list of the most frequent forms in Syn. Tag. Rus Machine learning: – Given the 100 most frequent forms, predict the next 100 most frequent forms – Given the 200 most frequent forms, predict the next 100 most frequent forms – Given the 300 most frequent forms, predict the next 100 most frequent forms – Given the 400 most frequent forms, predict the next 100 most frequent forms – Given the 500 most frequent forms, predict the next 100 most frequent forms – … until 5400, when Syn. Tag. Rus runs out of data
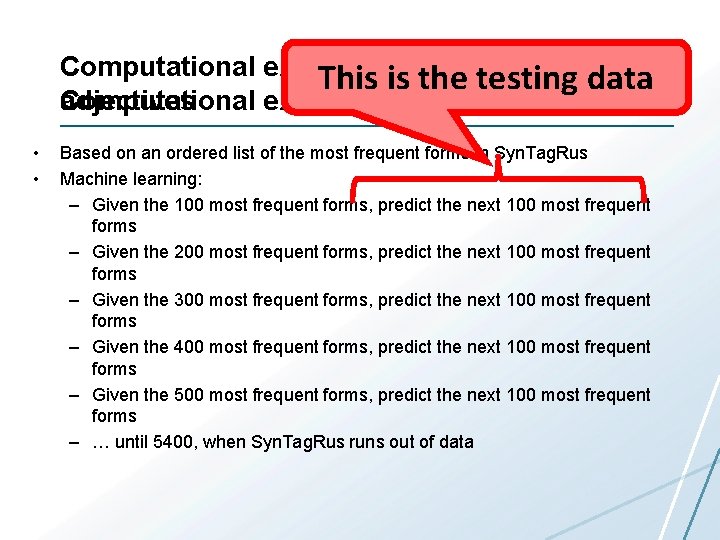
Computational experiment: nouns, verbs, This is the testing data Computational experiment adjectives • • Based on an ordered list of the most frequent forms in Syn. Tag. Rus Machine learning: – Given the 100 most frequent forms, predict the next 100 most frequent forms – Given the 200 most frequent forms, predict the next 100 most frequent forms – Given the 300 most frequent forms, predict the next 100 most frequent forms – Given the 400 most frequent forms, predict the next 100 most frequent forms – Given the 500 most frequent forms, predict the next 100 most frequent forms – … until 5400, when Syn. Tag. Rus runs out of data
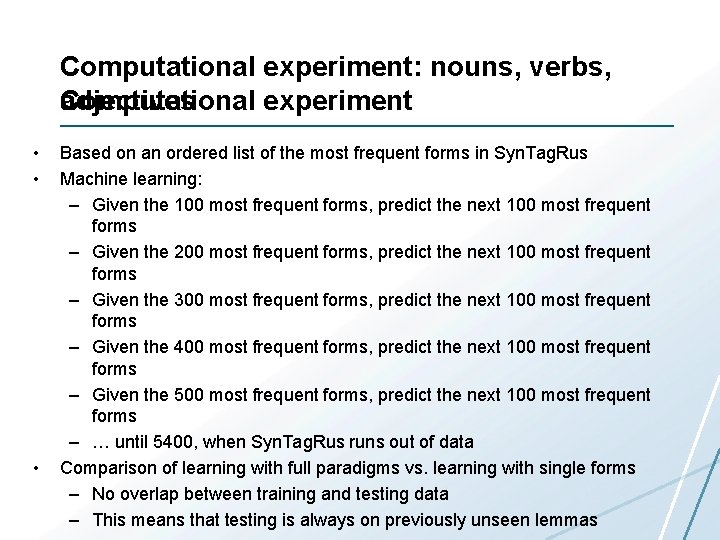
Computational experiment: nouns, verbs, Computational experiment adjectives • • • Based on an ordered list of the most frequent forms in Syn. Tag. Rus Machine learning: – Given the 100 most frequent forms, predict the next 100 most frequent forms – Given the 200 most frequent forms, predict the next 100 most frequent forms – Given the 300 most frequent forms, predict the next 100 most frequent forms – Given the 400 most frequent forms, predict the next 100 most frequent forms – Given the 500 most frequent forms, predict the next 100 most frequent forms – … until 5400, when Syn. Tag. Rus runs out of data Comparison of learning with full paradigms vs. learning with single forms – No overlap between training and testing data – This means that testing is always on previously unseen lemmas
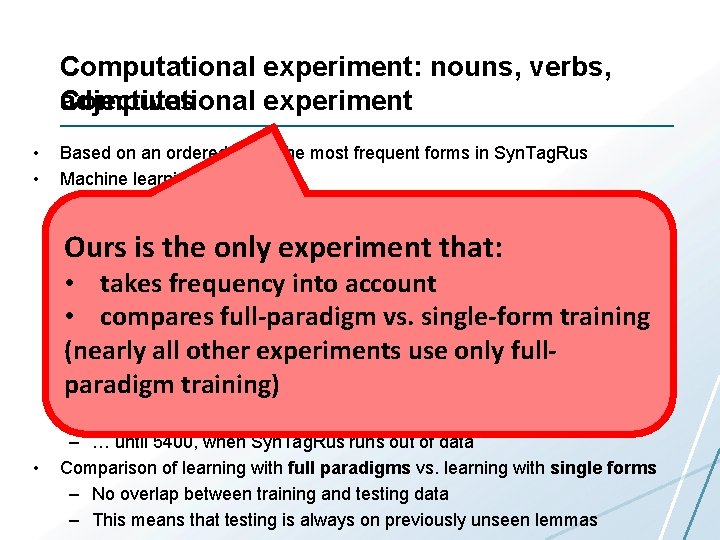
Computational experiment: nouns, verbs, Computational experiment adjectives • • Based on an ordered list of the most frequent forms in Syn. Tag. Rus Machine learning: – Given the 100 most frequent forms, predict the next 100 most frequent forms – Given the 200 most frequent forms, predict the next 100 most frequent • – forms takes frequency into account Given the 300 most frequent forms, predict the next 100 most frequent • forms compares full-paradigm vs. single-form training – Given the most frequent forms, predict next 100 most frequent (nearly all 400 other experiments usetheonly fullforms paradigm training) – Given the 500 most frequent forms, predict the next 100 most frequent forms – … until 5400, when Syn. Tag. Rus runs out of data Comparison of learning with full paradigms vs. learning with single forms – No overlap between training and testing data – This means that testing is always on previously unseen lemmas Ours is the only experiment that: •
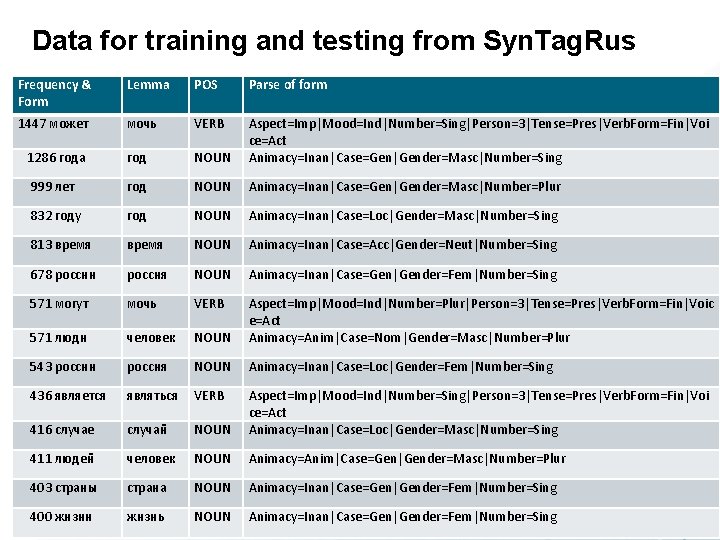
Data for training and testing from Syn. Tag. Rus Frequency & Form Lemma POS Parse of form 1447 может мочь VERB 1286 года год NOUN Aspect=Imp|Mood=Ind|Number=Sing|Person=3|Tense=Pres|Verb. Form=Fin|Voi ce=Act Animacy=Inan|Case=Gen|Gender=Masc|Number=Sing 999 лет год NOUN Animacy=Inan|Case=Gen|Gender=Masc|Number=Plur 832 году год NOUN Animacy=Inan|Case=Loc|Gender=Masc|Number=Sing 813 время NOUN Animacy=Inan|Case=Acc|Gender=Neut|Number=Sing 678 россии россия NOUN Animacy=Inan|Case=Gen|Gender=Fem|Number=Sing 571 могут мочь VERB 571 люди человек NOUN Aspect=Imp|Mood=Ind|Number=Plur|Person=3|Tense=Pres|Verb. Form=Fin|Voic e=Act Animacy=Anim|Case=Nom|Gender=Masc|Number=Plur 543 россии россия NOUN Animacy=Inan|Case=Loc|Gender=Fem|Number=Sing 436 является являться VERB 416 случае случай NOUN Aspect=Imp|Mood=Ind|Number=Sing|Person=3|Tense=Pres|Verb. Form=Fin|Voi ce=Act Animacy=Inan|Case=Loc|Gender=Masc|Number=Sing 411 людей человек NOUN Animacy=Anim|Case=Gen|Gender=Masc|Number=Plur 403 страны страна NOUN Animacy=Inan|Case=Gen|Gender=Fem|Number=Sing 400 жизни жизнь NOUN Animacy=Inan|Case=Gen|Gender=Fem|Number=Sing
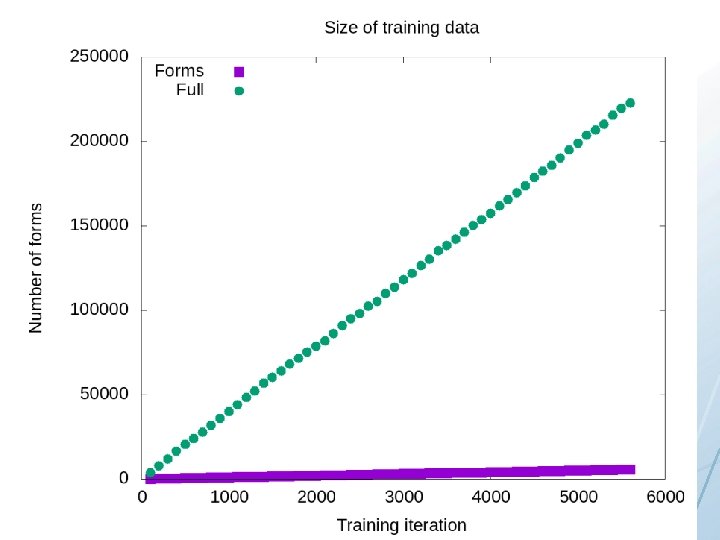
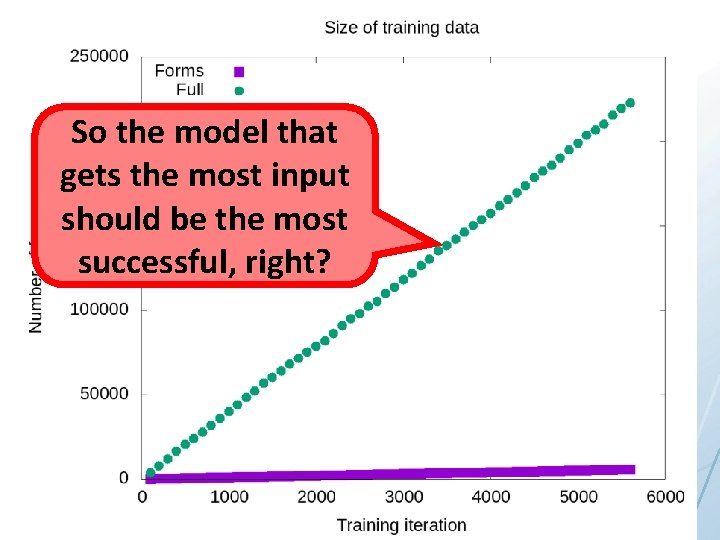
So the model that gets the most input should be the most successful, right?
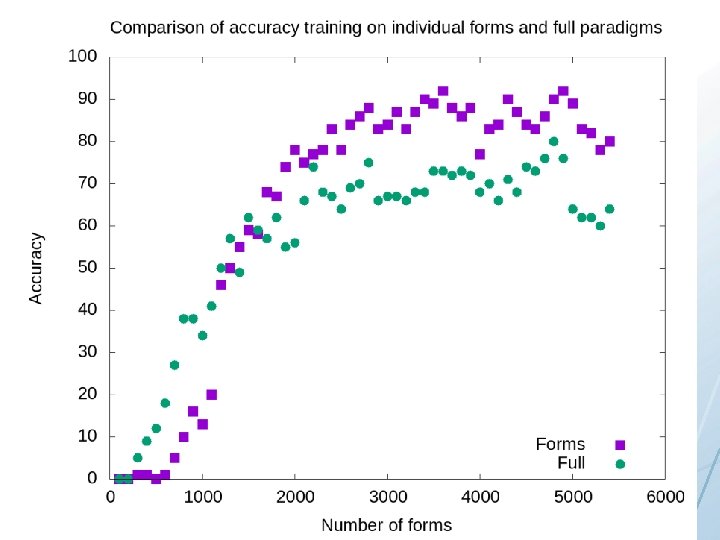
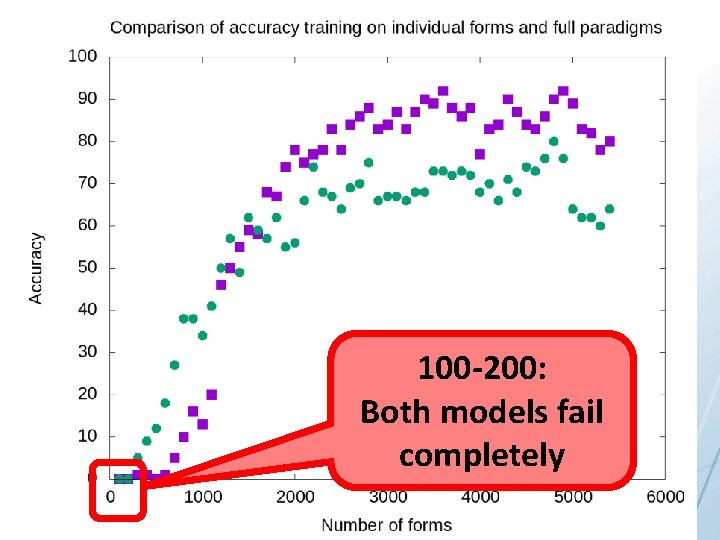
100 -200: Both models fail completely
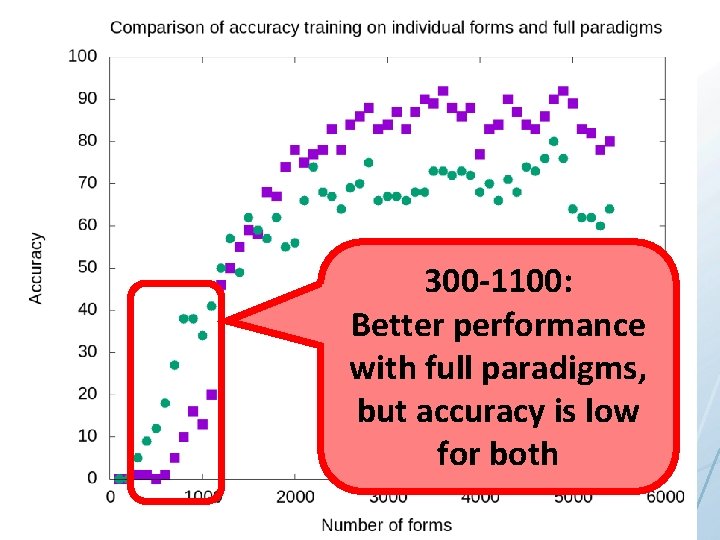
300 -1100: Better performance with full paradigms, but accuracy is low for both
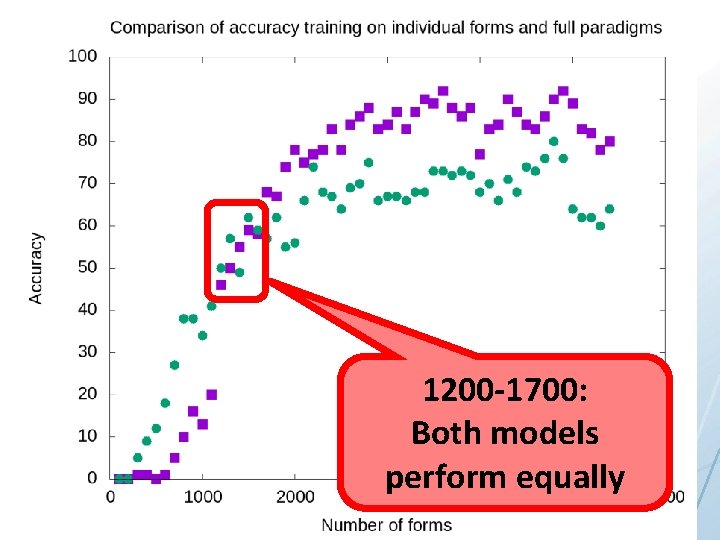
1200 -1700: Both models perform equally
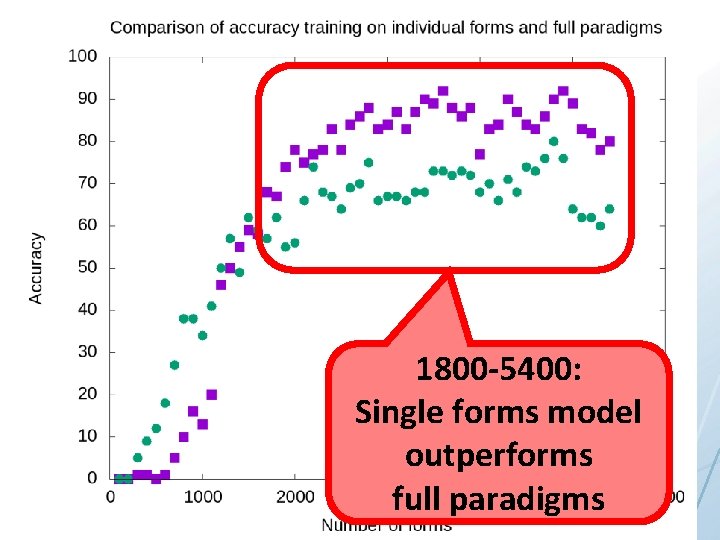
1800 -5400: Single forms model outperforms full paradigms
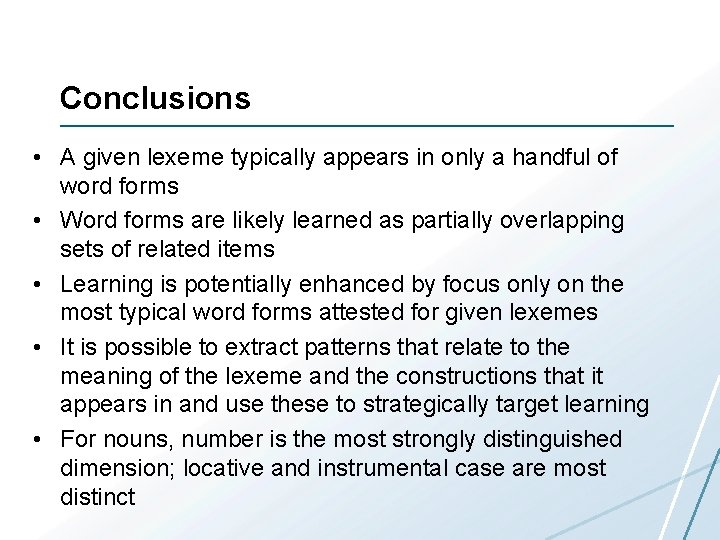
Conclusions • A given lexeme typically appears in only a handful of word forms • Word forms are likely learned as partially overlapping sets of related items • Learning is potentially enhanced by focus only on the most typical word forms attested for given lexemes • It is possible to extract patterns that relate to the meaning of the lexeme and the constructions that it appears in and use these to strategically target learning • For nouns, number is the most strongly distinguished dimension; locative and instrumental case are most distinct
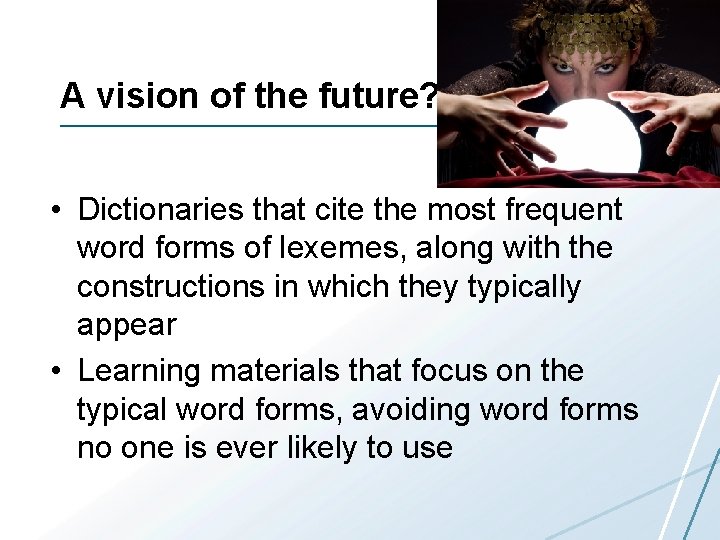
A vision of the future? • Dictionaries that cite the most frequent word forms of lexemes, along with the constructions in which they typically appear • Learning materials that focus on the typical word forms, avoiding word forms no one is ever likely to use
Edy suwarno
Janda
Slideshow sabbath school
Contoh pengisian sptb taspen 2021
Laura conocía bien a elián. elián conocía bien a laura.
It has 6 rectangular faces 8 corners and 12 edges
Does congress have the power to have no mail on saturdays
Peter hall policy paradigms
Careershodh
Paradigms and principles
Paradigmatic structure
Paradigms for interaction
Distributed systems principles and paradigms
Message ordering paradigms
Distributed systems principles and paradigms
Distributed paradigm
3 paradigms
Interpretivist approach to consumer behavior
Boyfriend/girlfriend centered paradigm
Binding in programming paradigms
Ktu programming paradigms notes
Self development and communication ppt
Designer released multimedia authoring system
Evaluation paradigms
Biological paradigm of psychopathology
R programming language paradigms
Php features and paradigms
Paradigms and principles
Distributed systems principles and paradigms
Development paradigms
Distributed systems principles and paradigms
Which russian leader does napoleon most resemble?
Words have meaning and names have power
Modal verbs for suggestion
Samuel they have rejected me
I have resolved
Ideas have consequences bad ideas have victims
Go7grow
Some animals are dangerous *
Is could past tense
Echinoderms have endoskeleton
Chapter 27 world war 1 and the russian revolution
What are the 5 vowels
Lesson 3 the russian revolution answer key