Characterizing Photospheric Flows David Hathaway NASAMSFC with John
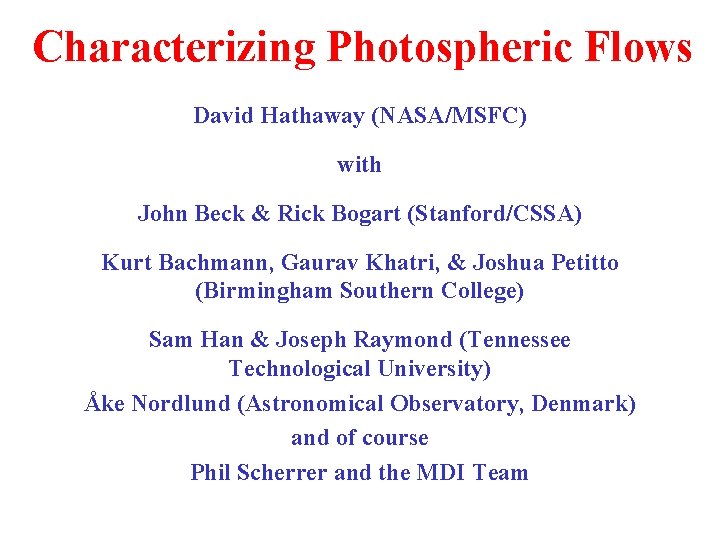
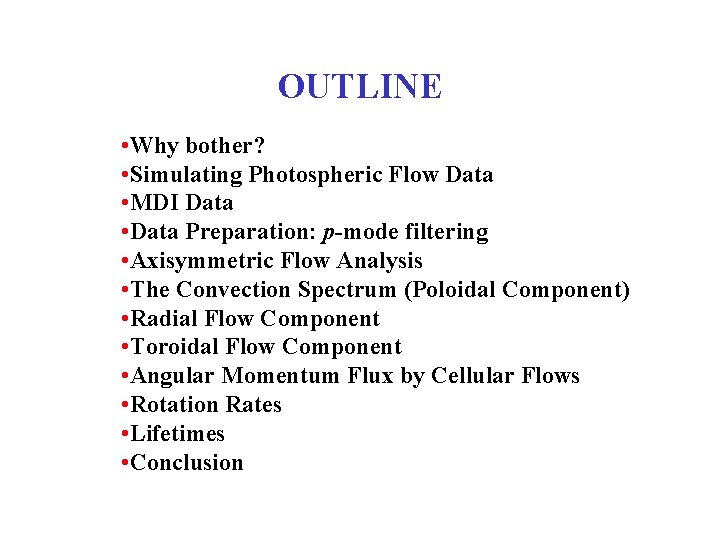
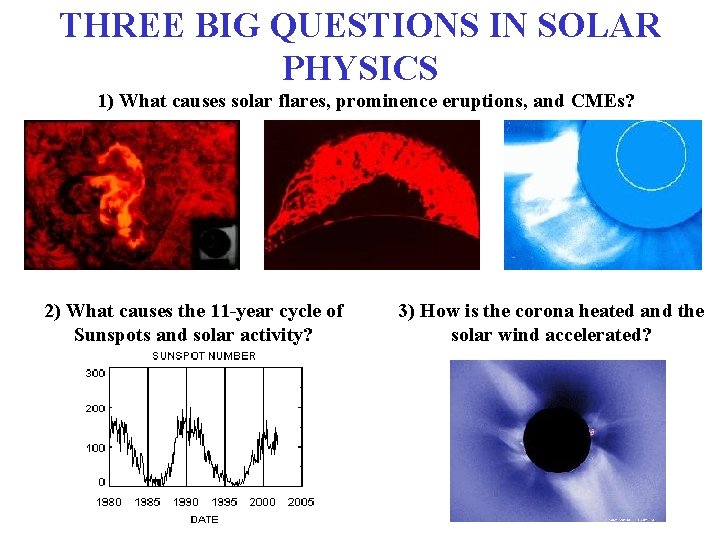
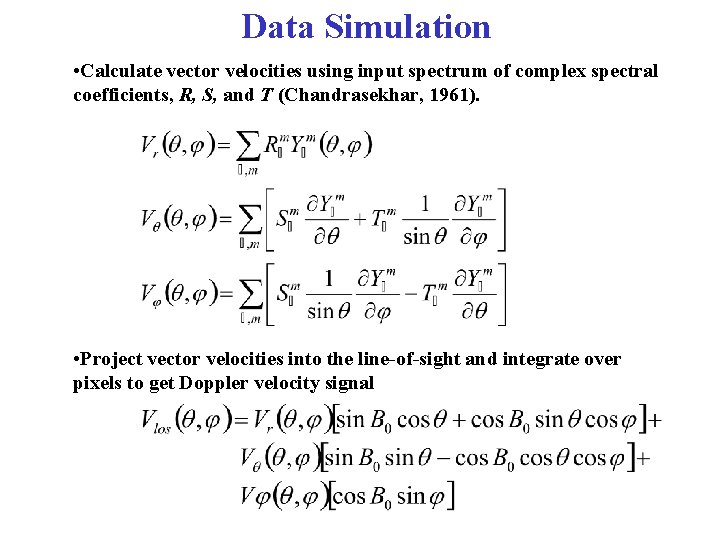
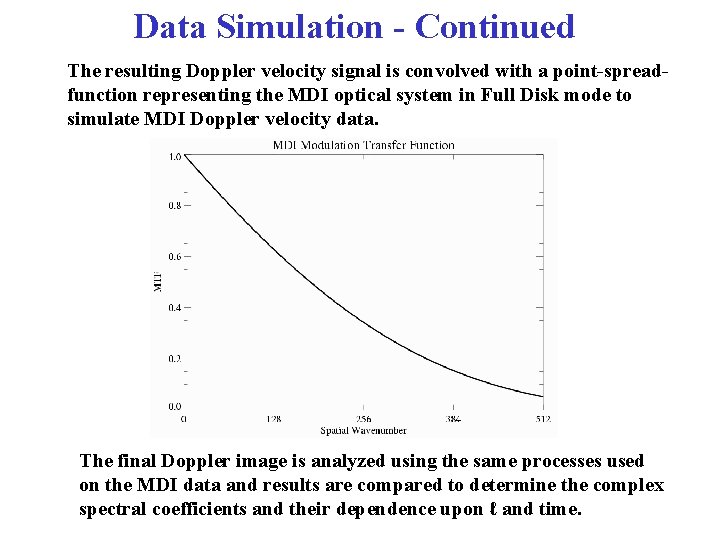
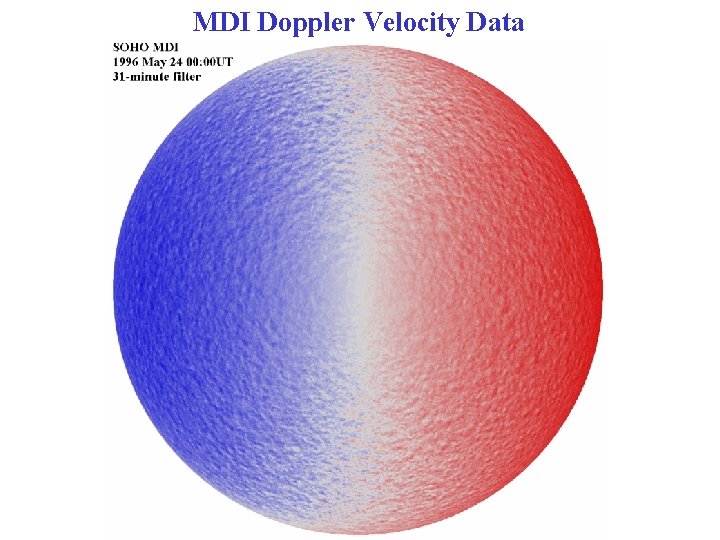
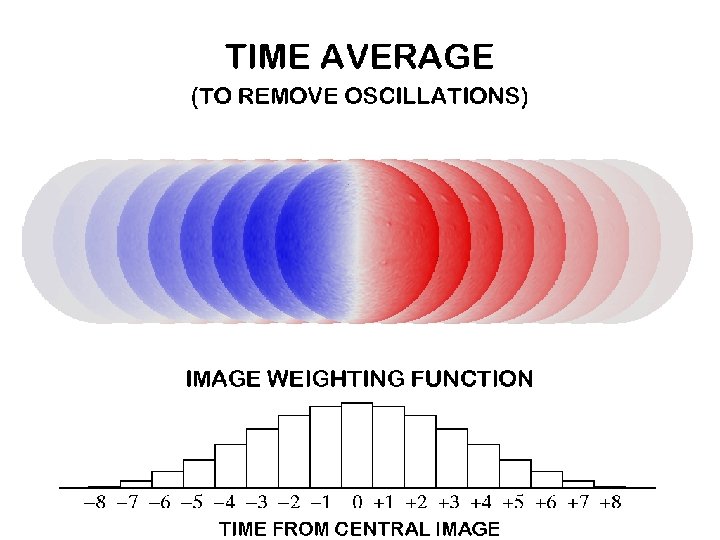
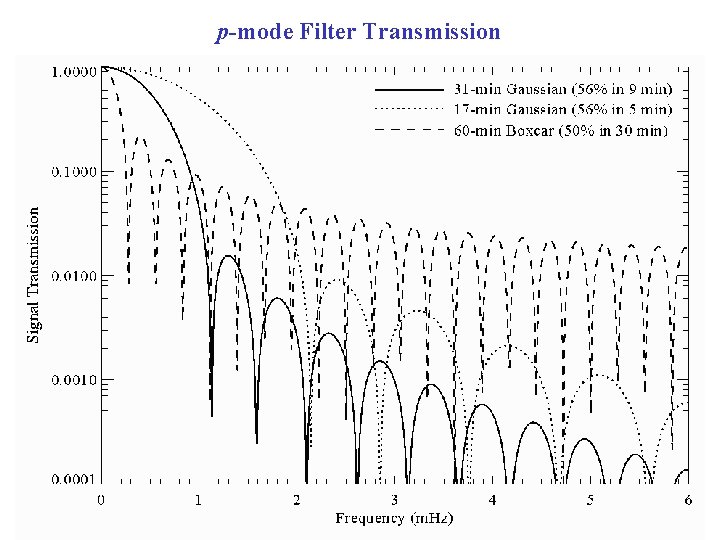
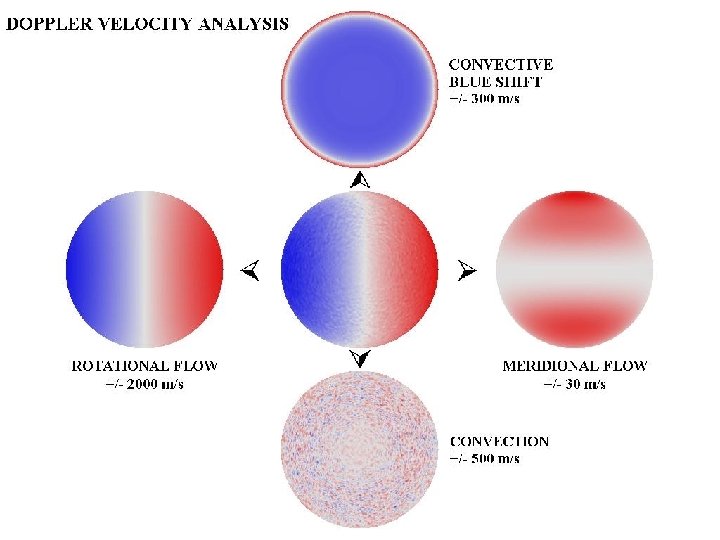
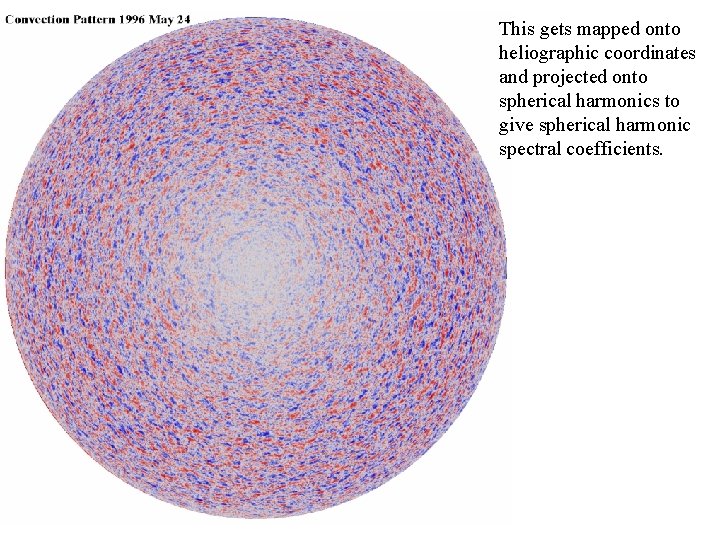
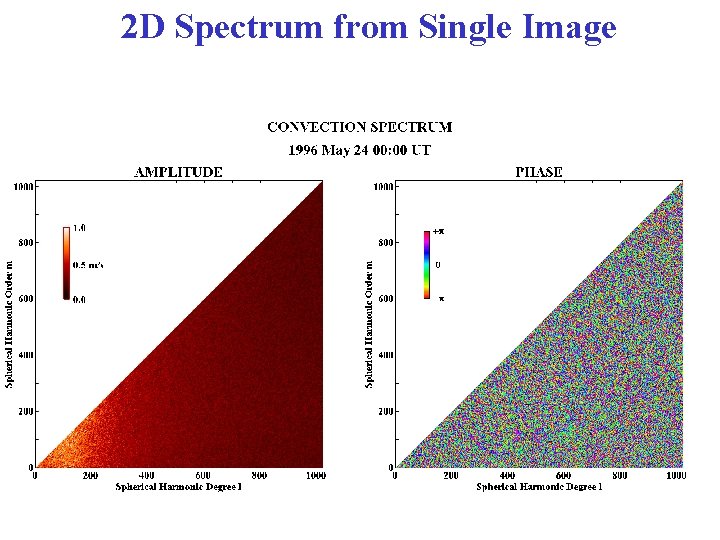
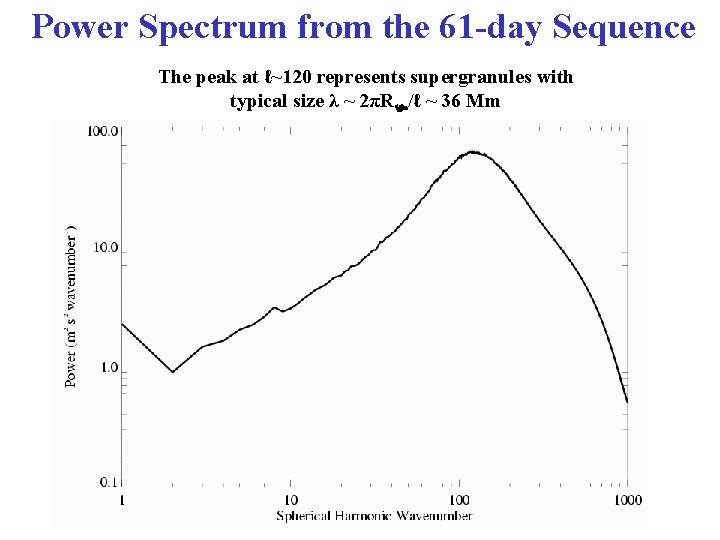
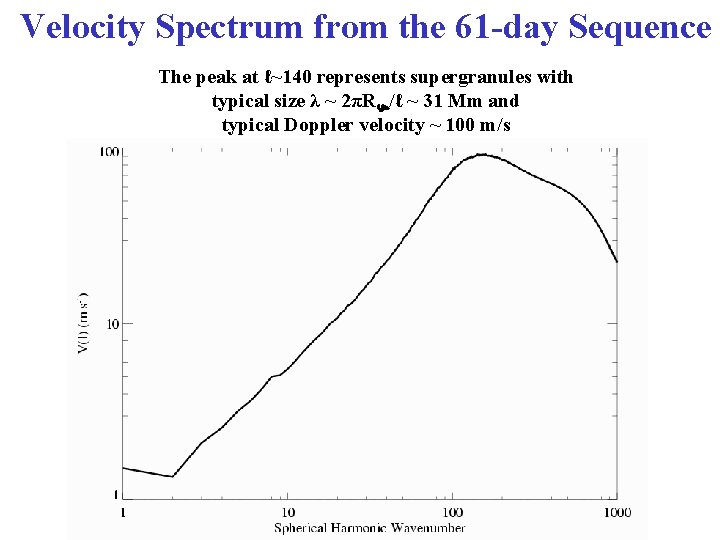
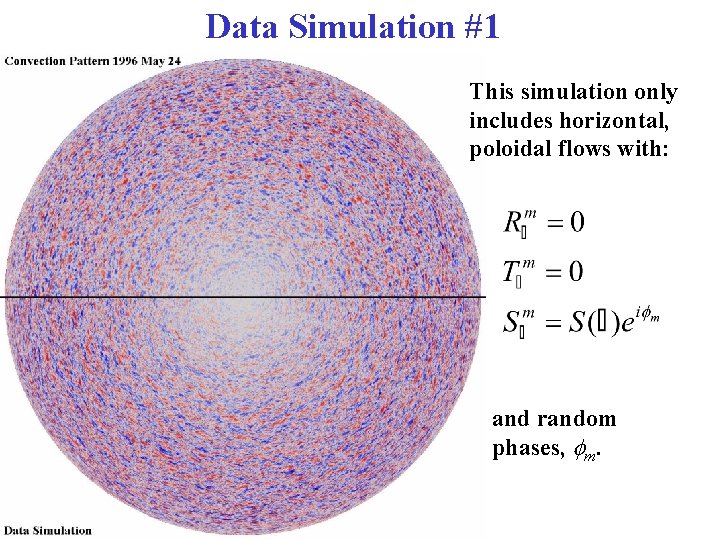
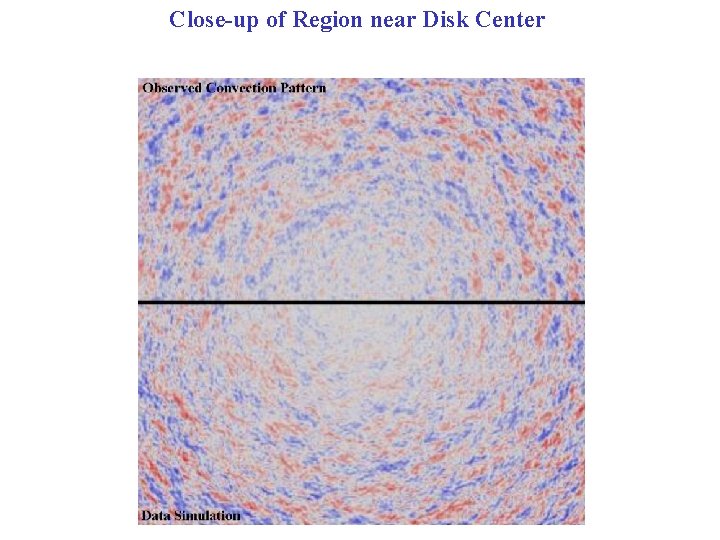
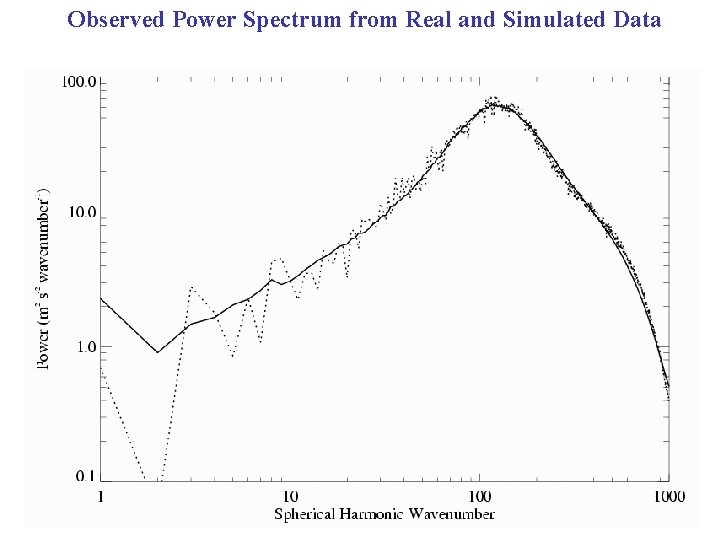
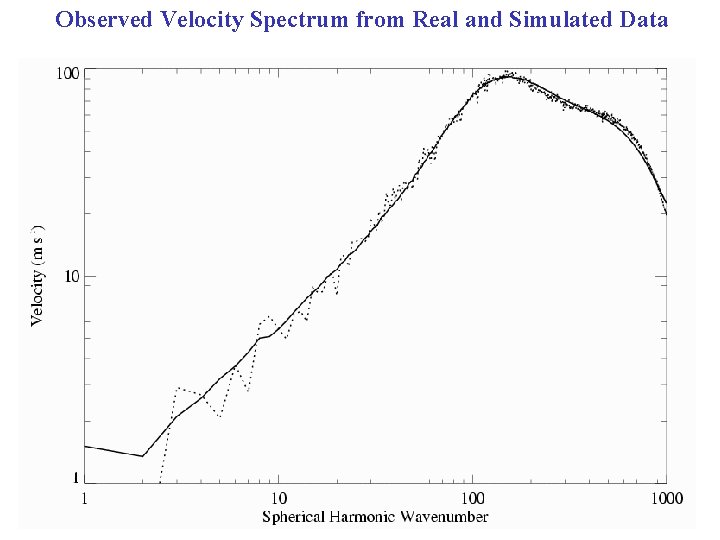
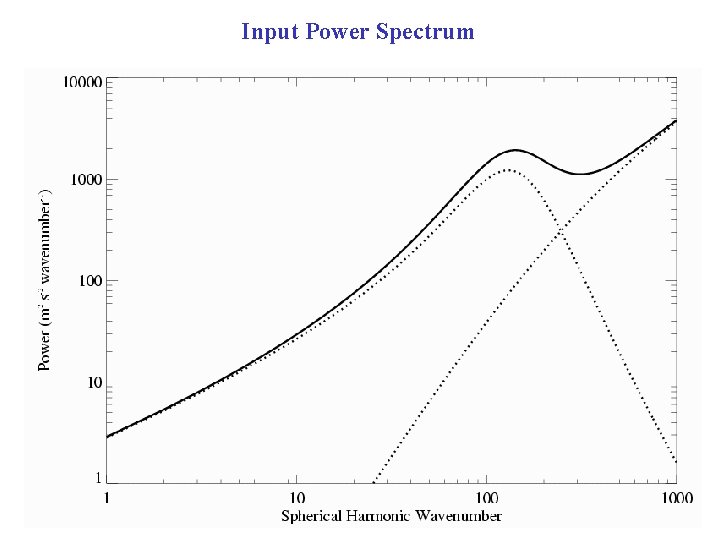
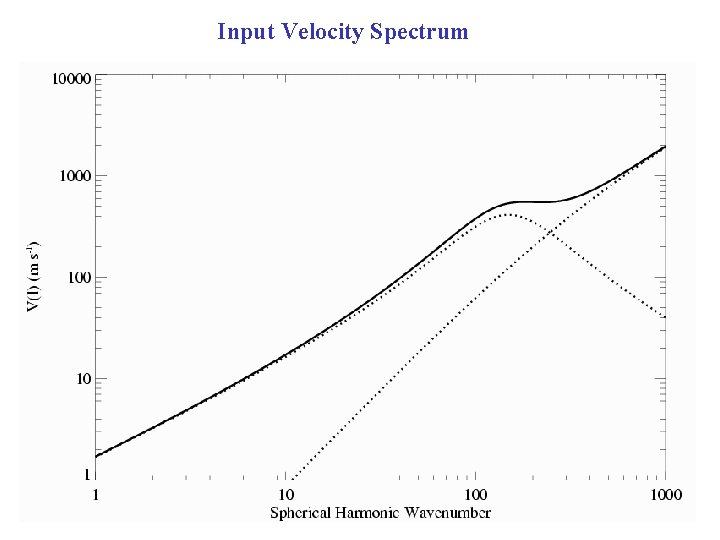
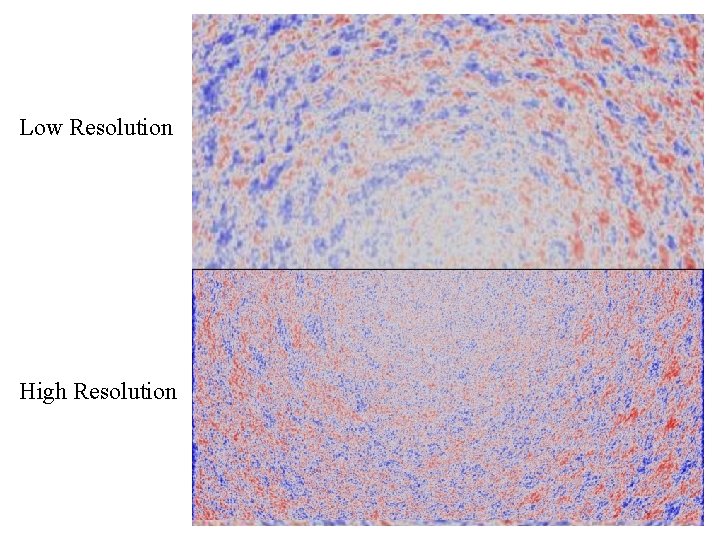
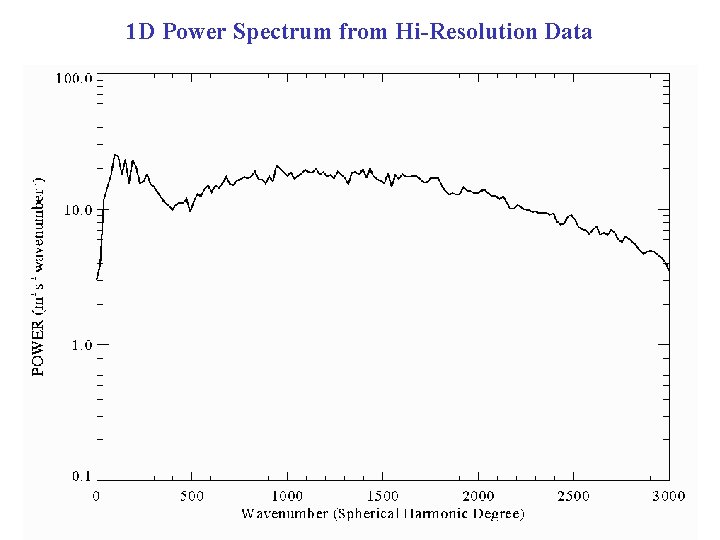
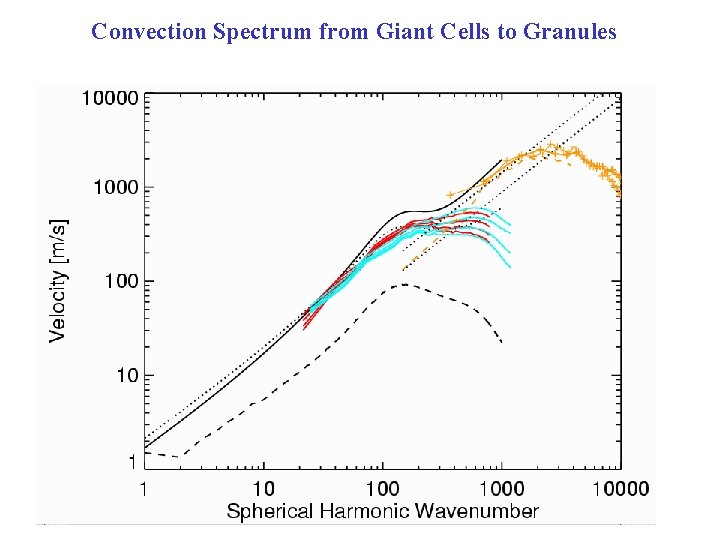
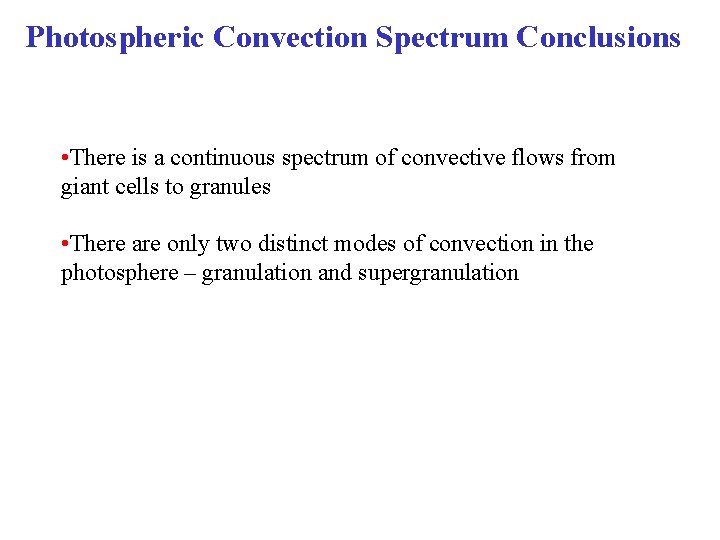
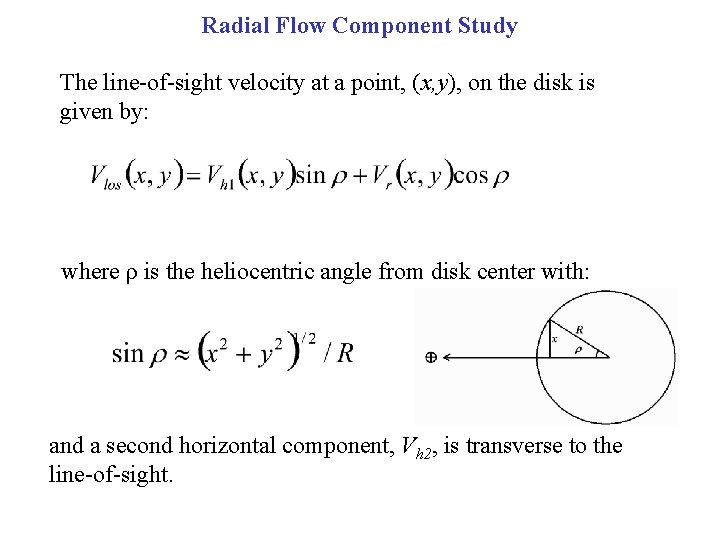
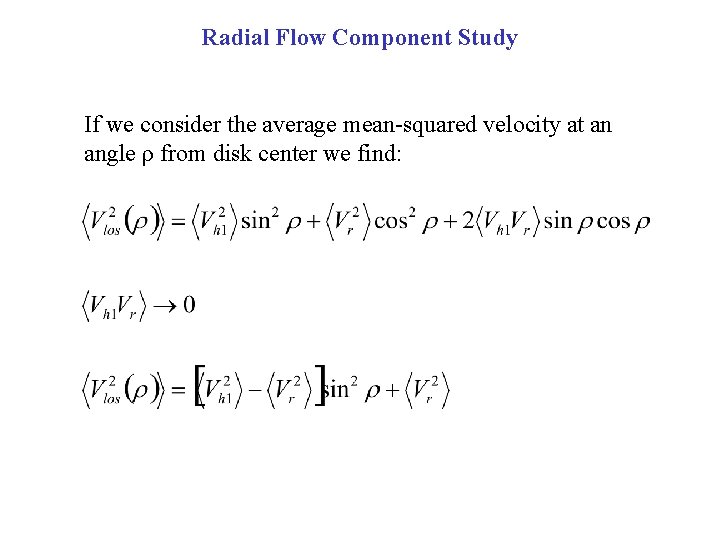
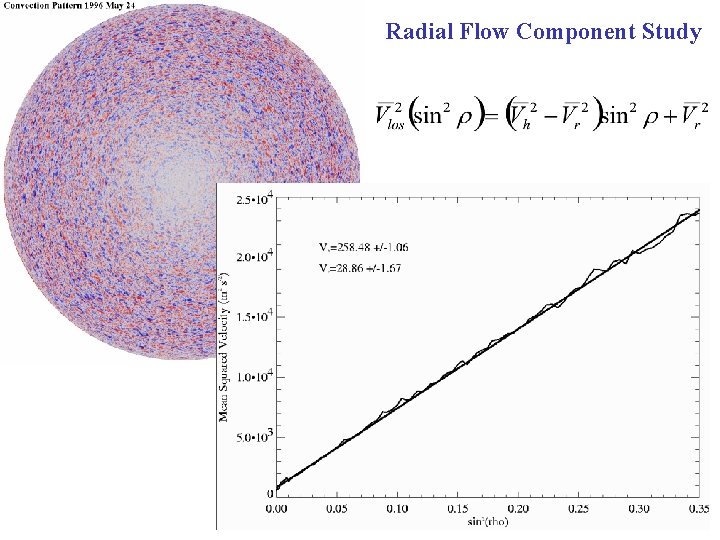
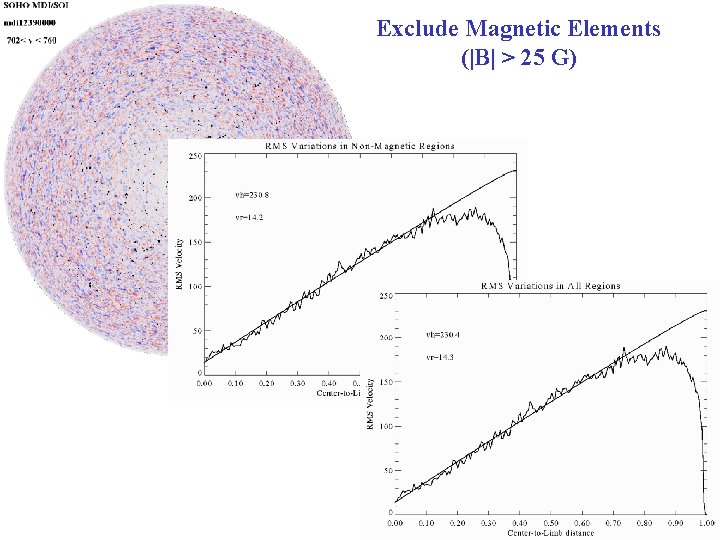
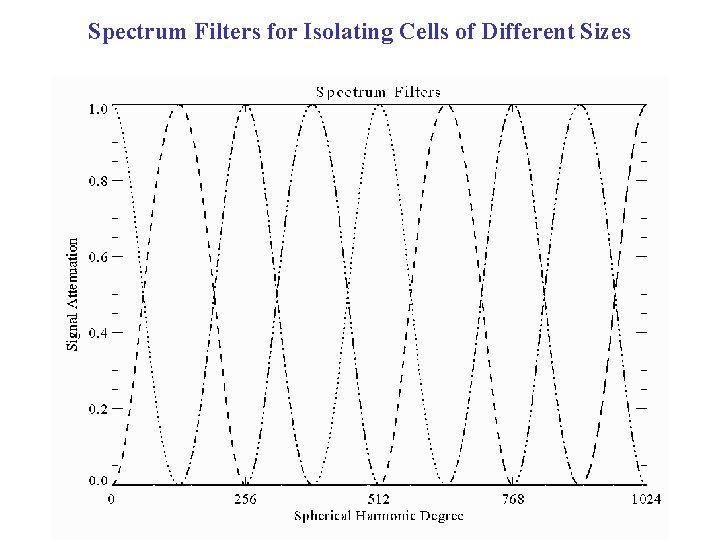
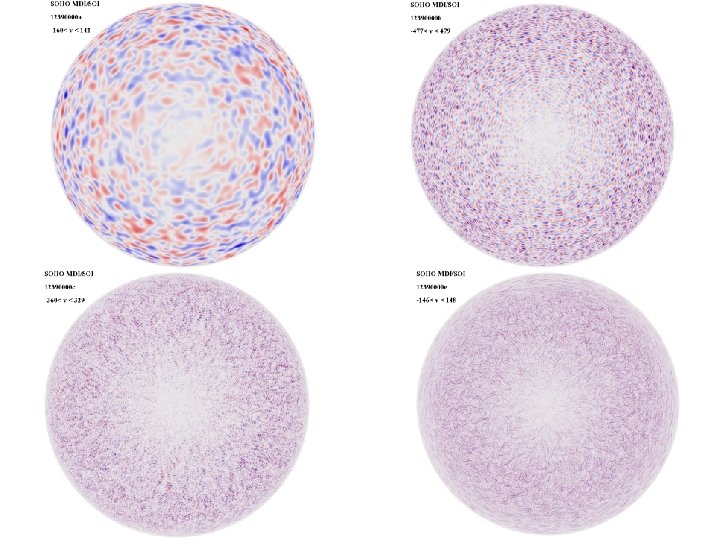
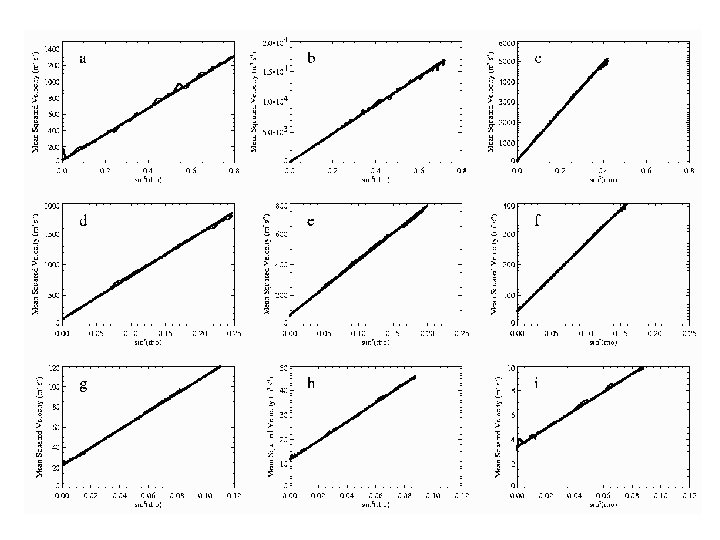
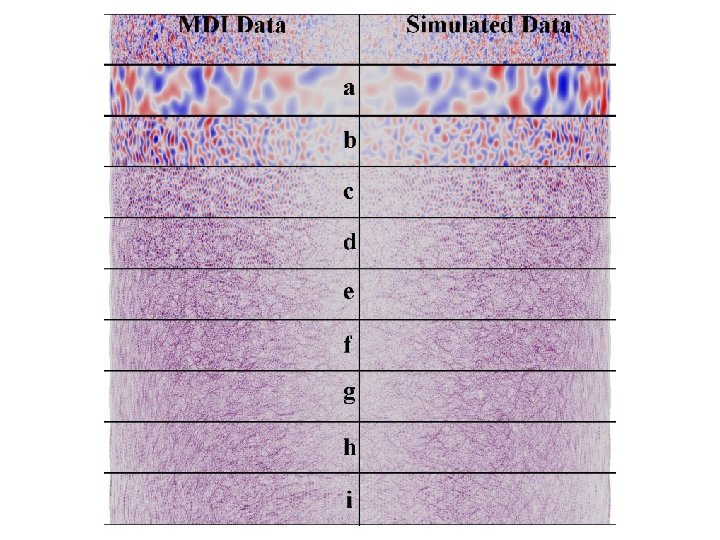
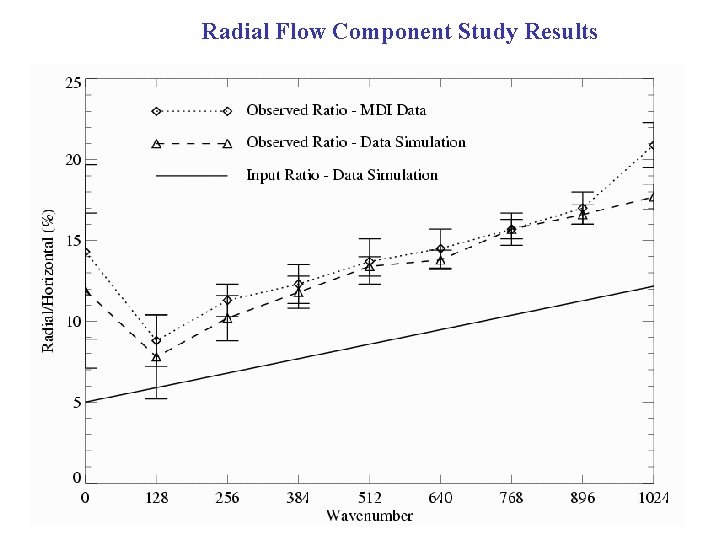
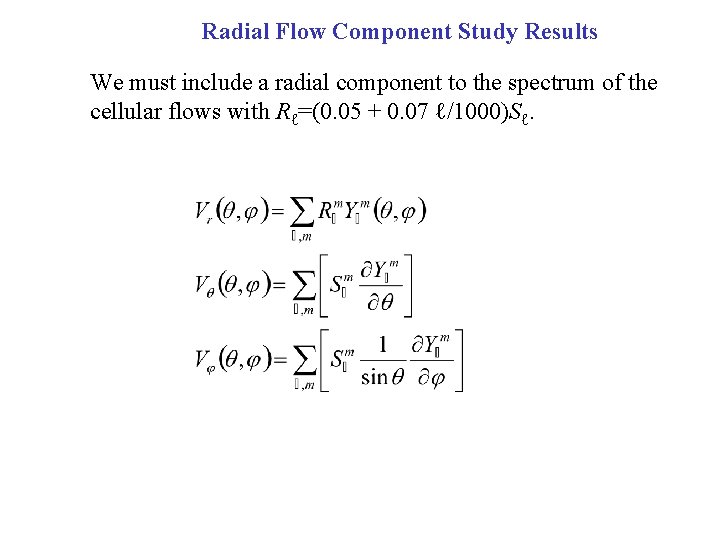
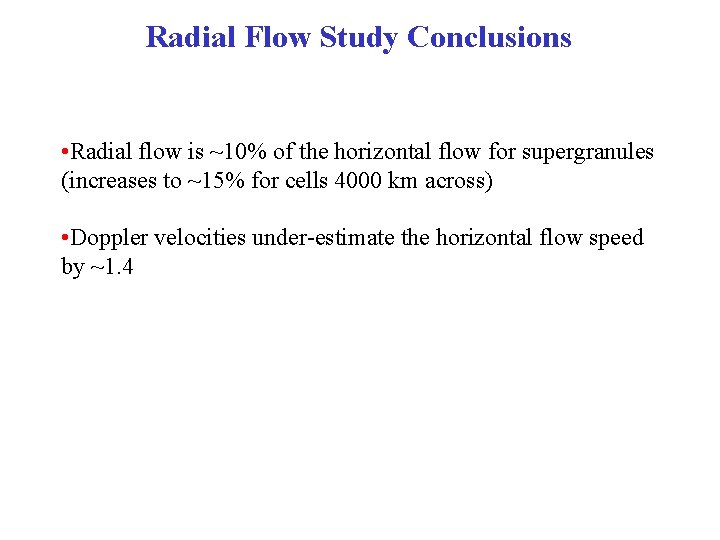
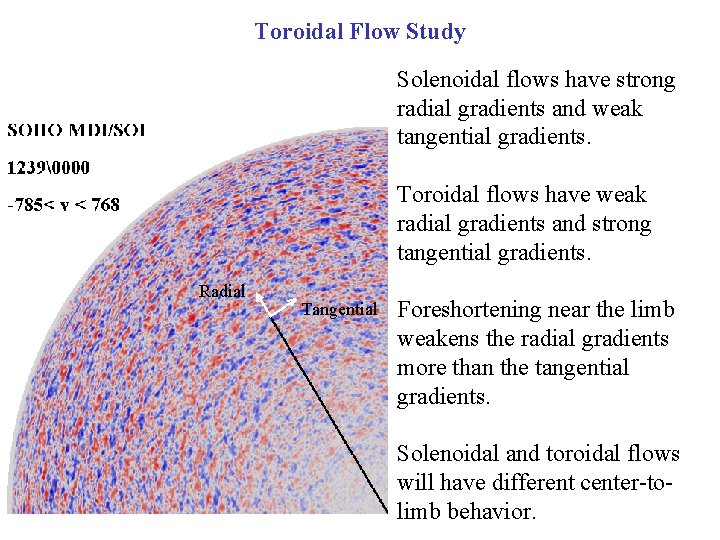
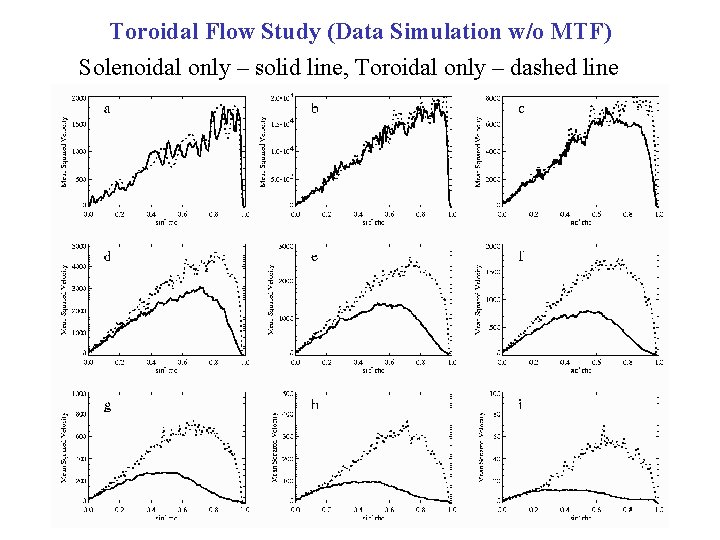
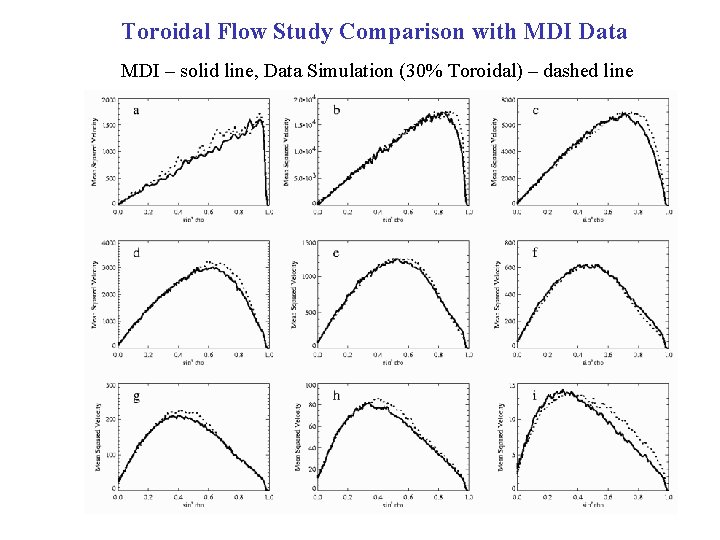
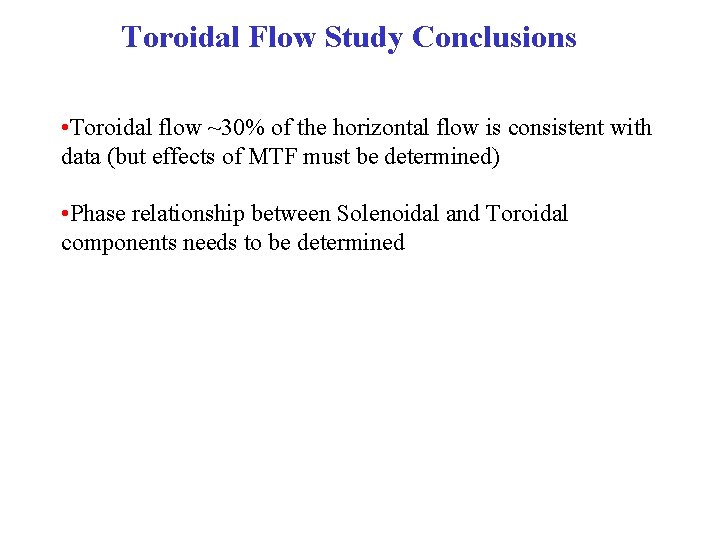
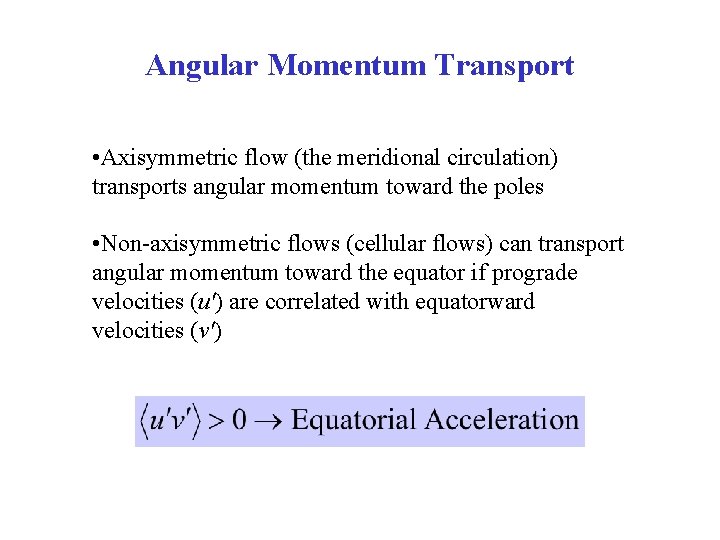
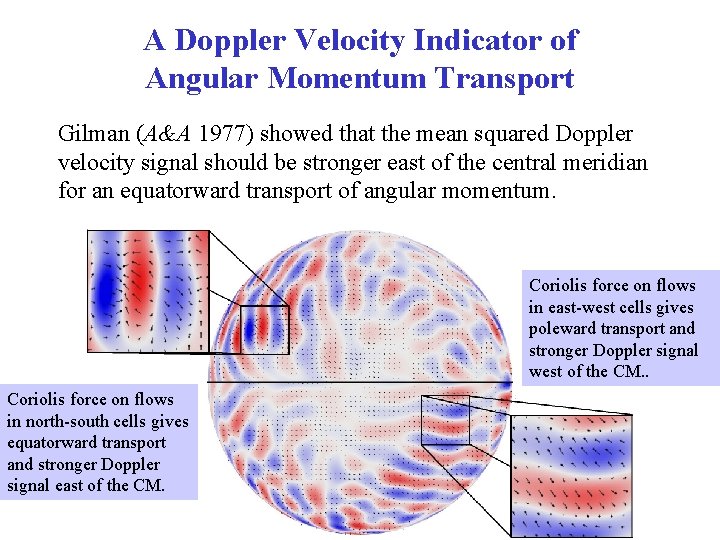
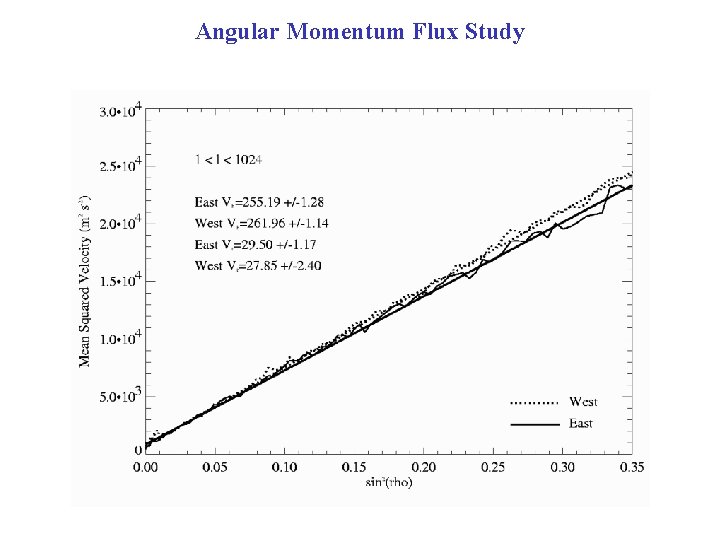
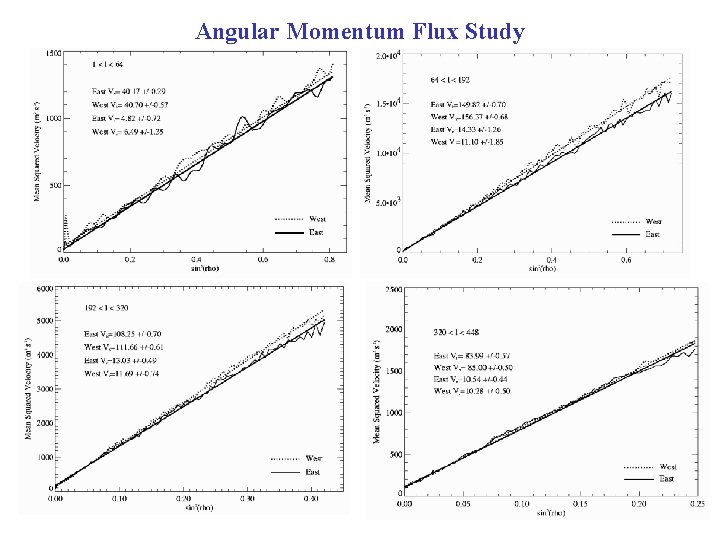
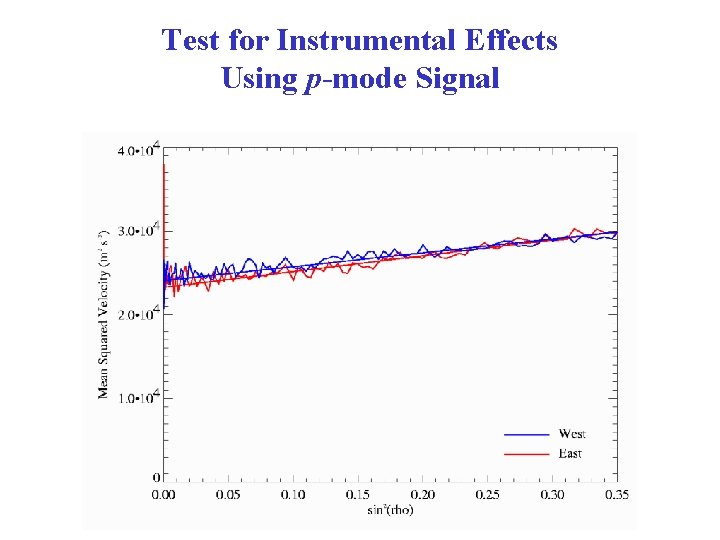
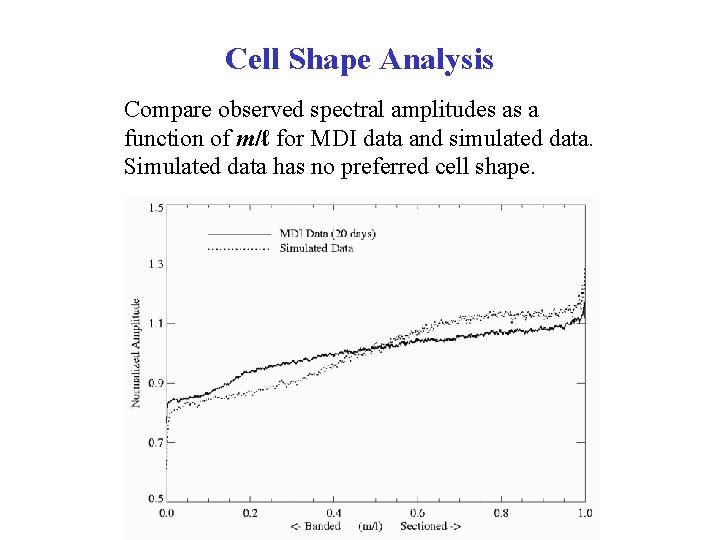
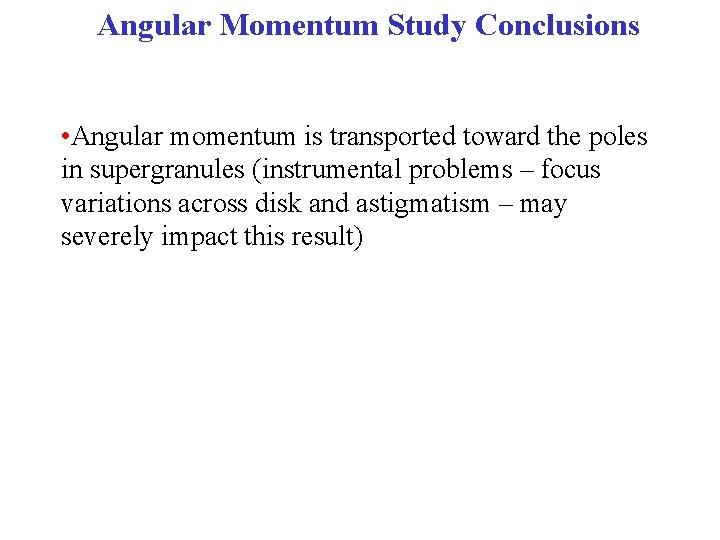
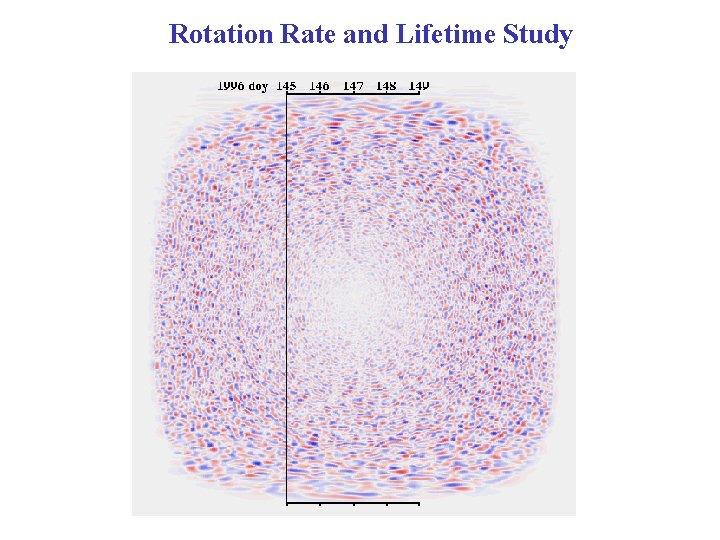
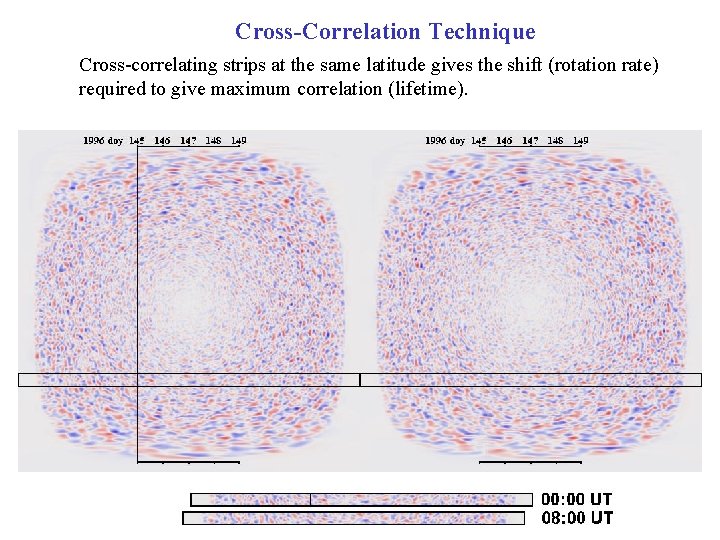
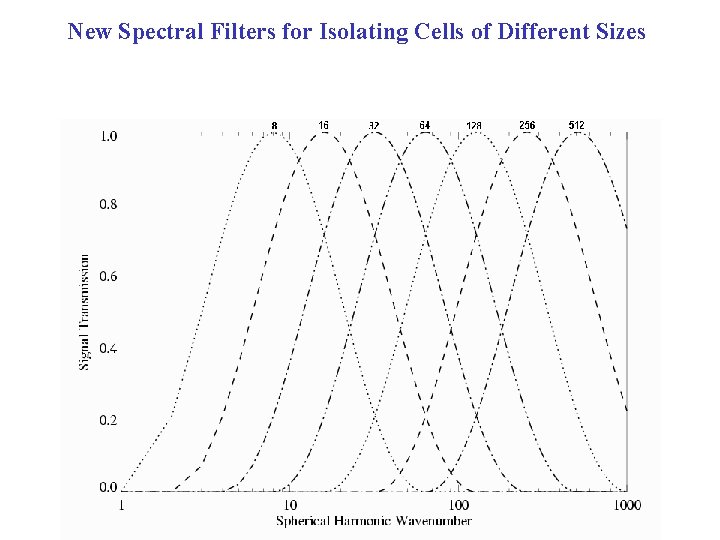
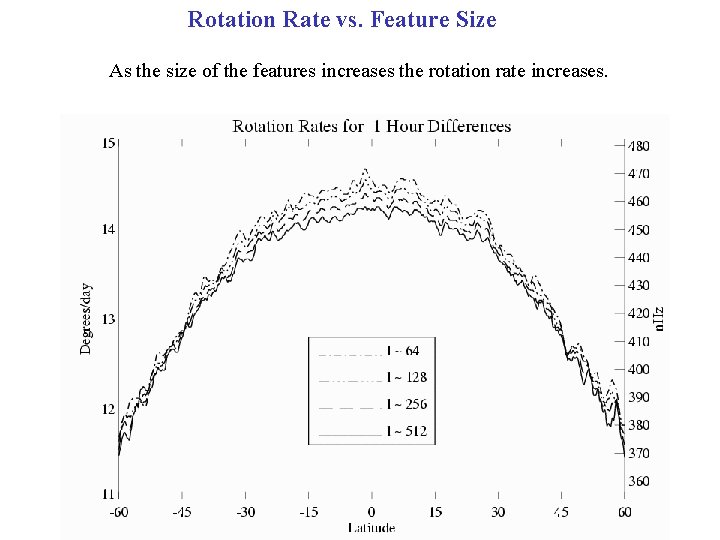
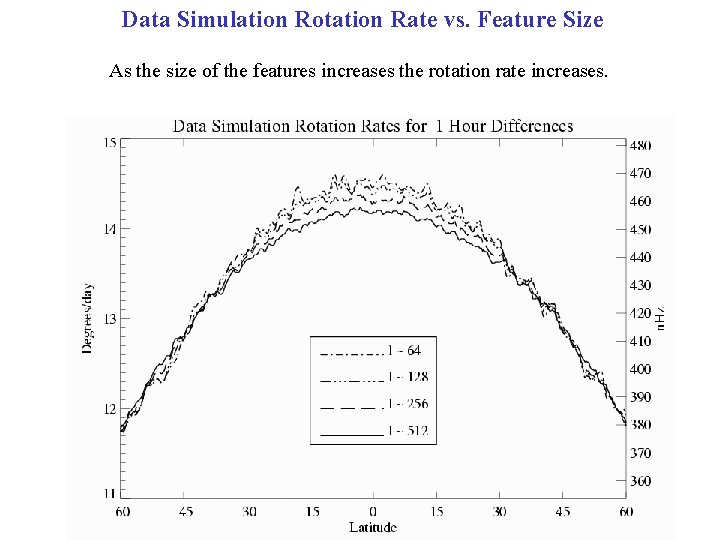
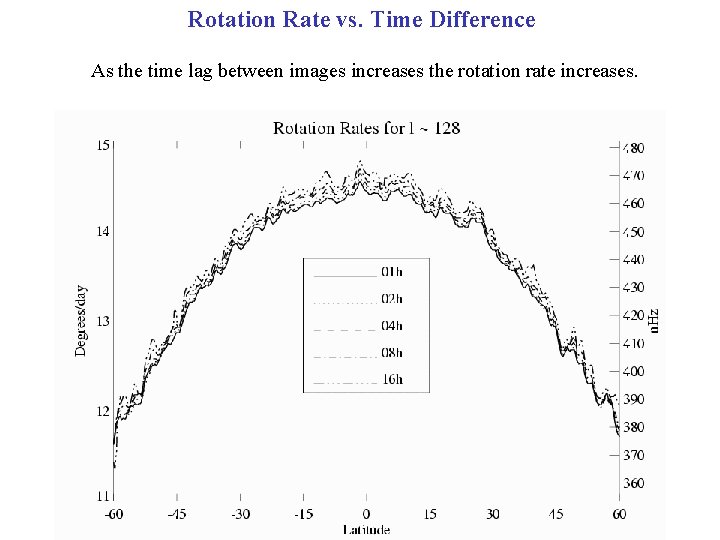
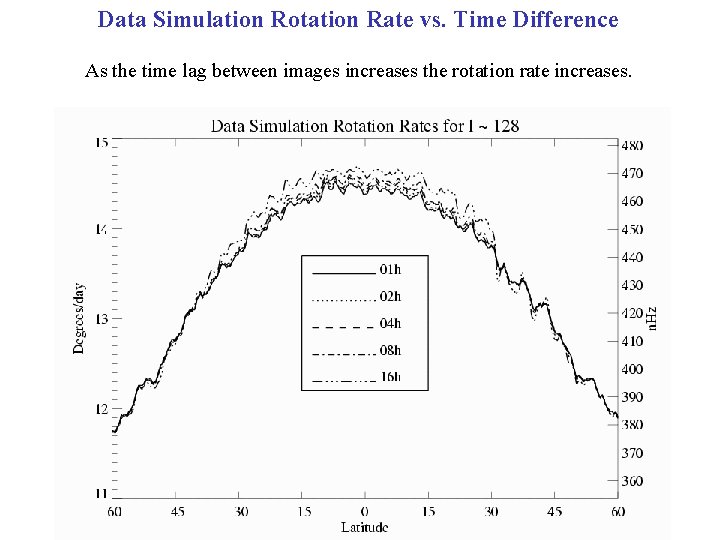
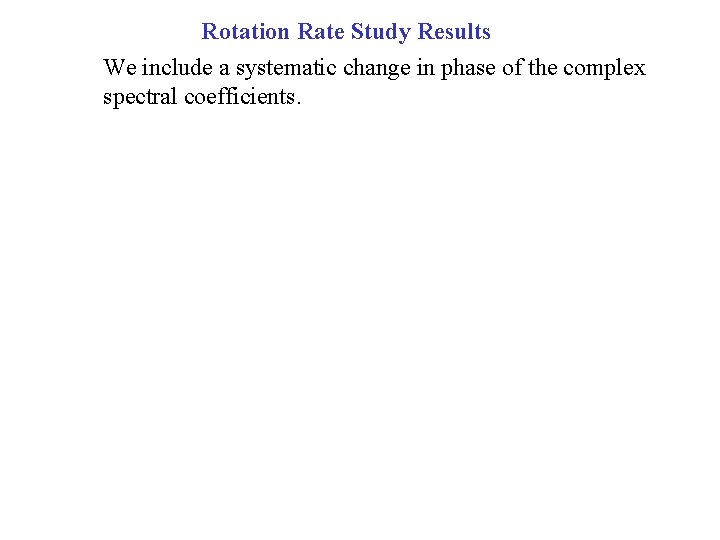
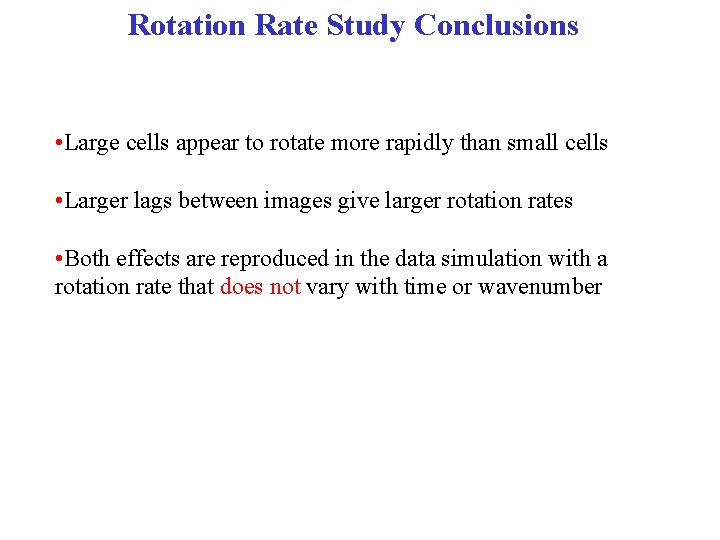
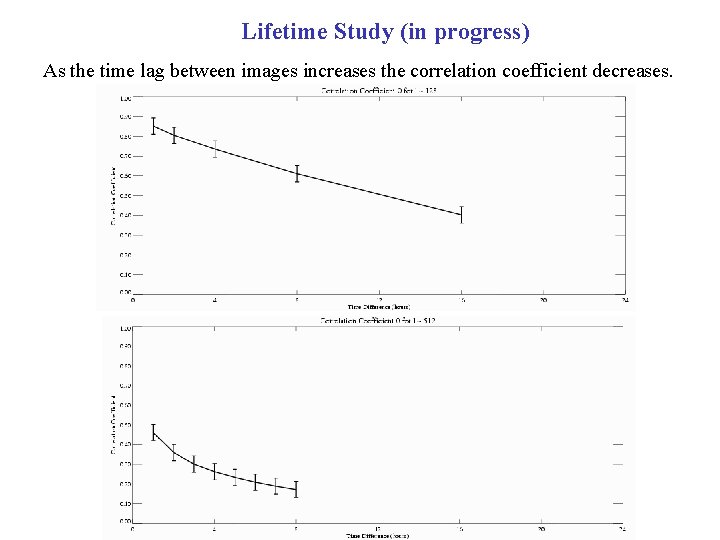
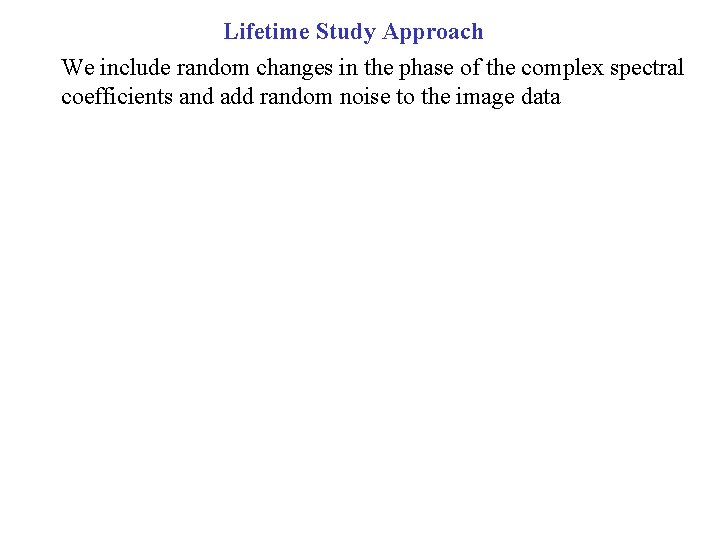
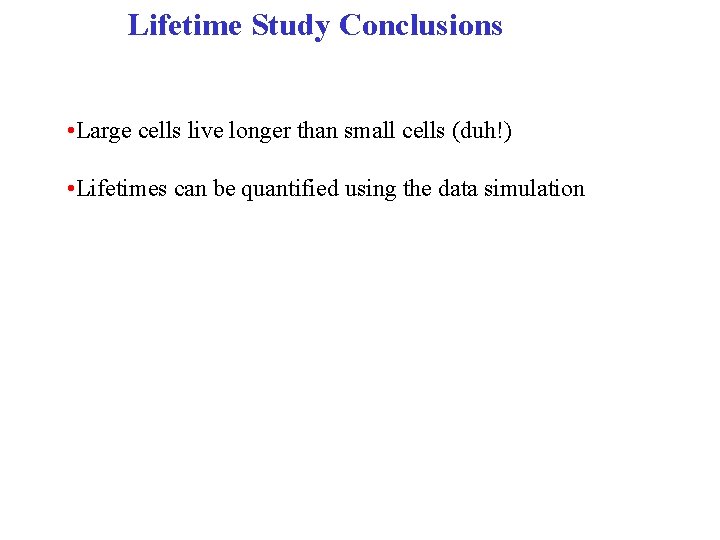
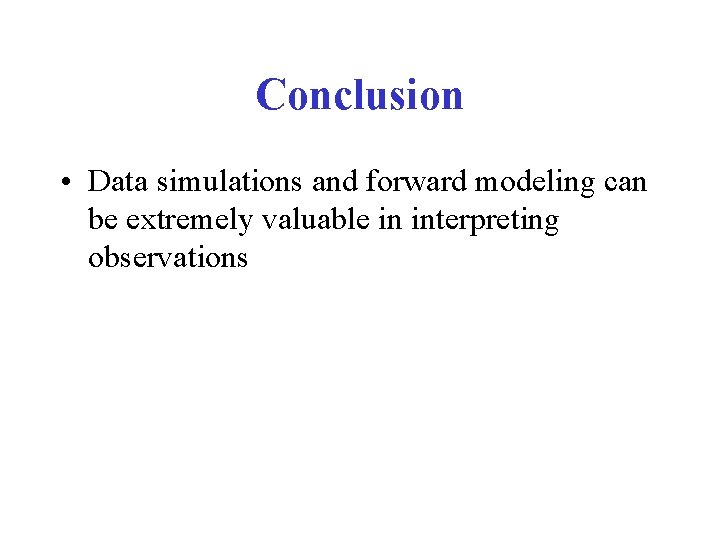
- Slides: 58
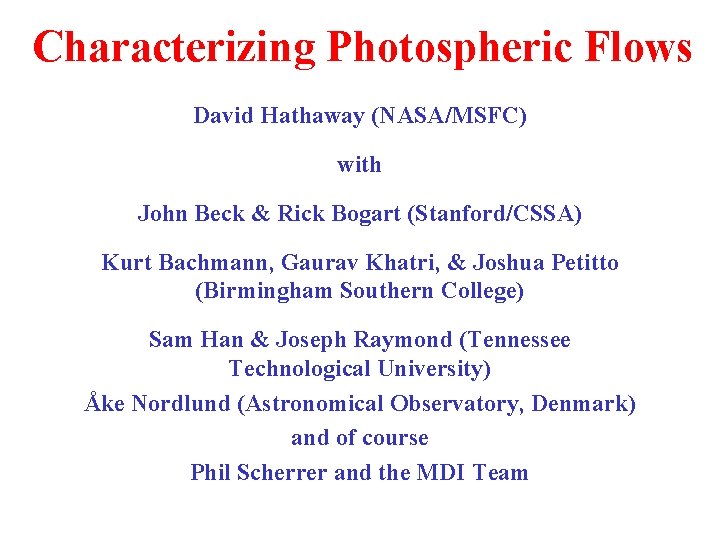
Characterizing Photospheric Flows David Hathaway (NASA/MSFC) with John Beck & Rick Bogart (Stanford/CSSA) Kurt Bachmann, Gaurav Khatri, & Joshua Petitto (Birmingham Southern College) Sam Han & Joseph Raymond (Tennessee Technological University) Åke Nordlund (Astronomical Observatory, Denmark) and of course Phil Scherrer and the MDI Team
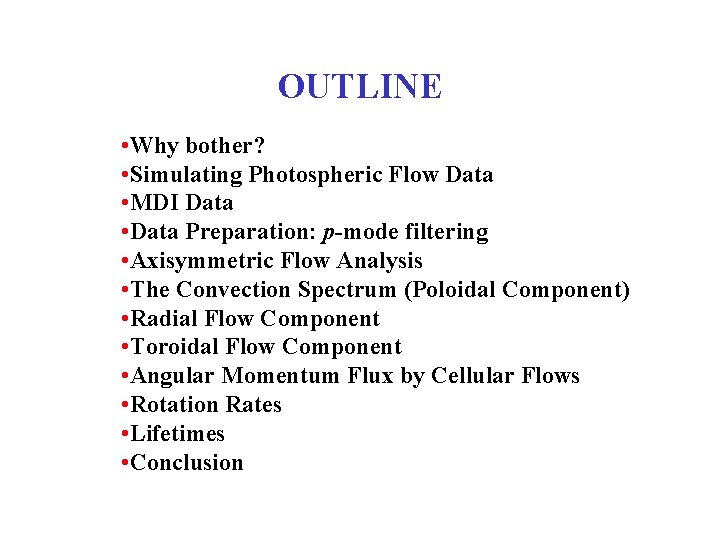
OUTLINE • Why bother? • Simulating Photospheric Flow Data • MDI Data • Data Preparation: p-mode filtering • Axisymmetric Flow Analysis • The Convection Spectrum (Poloidal Component) • Radial Flow Component • Toroidal Flow Component • Angular Momentum Flux by Cellular Flows • Rotation Rates • Lifetimes • Conclusion
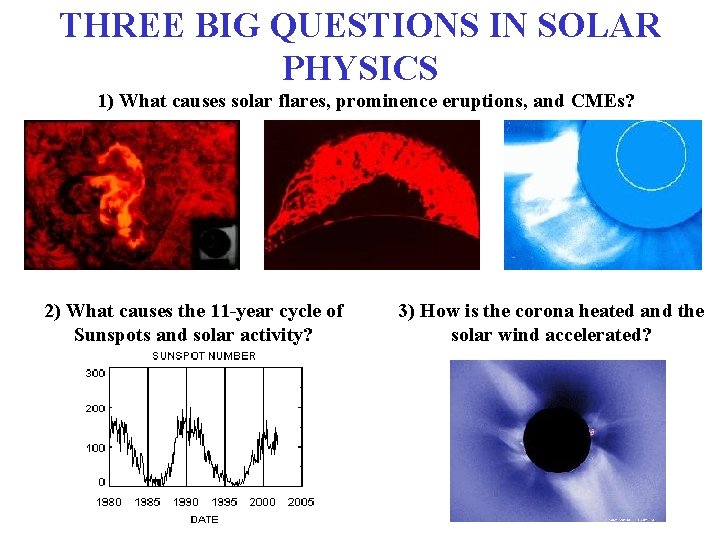
THREE BIG QUESTIONS IN SOLAR PHYSICS 1) What causes solar flares, prominence eruptions, and CMEs? 2) What causes the 11 -year cycle of Sunspots and solar activity? 3) How is the corona heated and the solar wind accelerated?
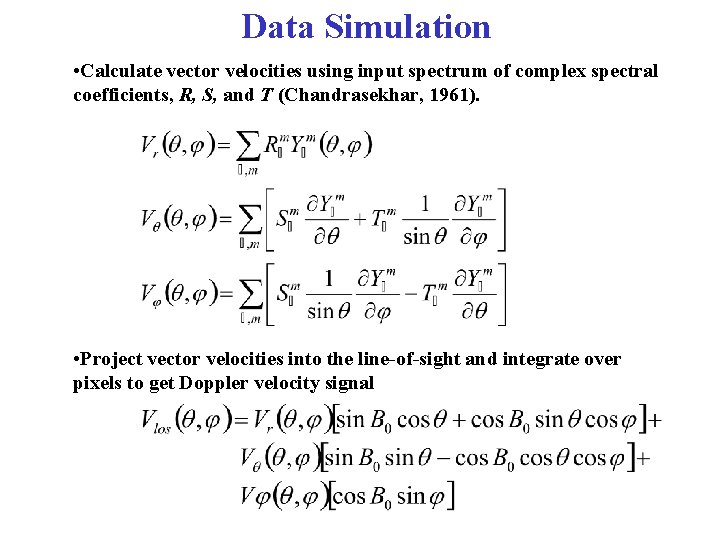
Data Simulation • Calculate vector velocities using input spectrum of complex spectral coefficients, R, S, and T (Chandrasekhar, 1961). • Project vector velocities into the line-of-sight and integrate over pixels to get Doppler velocity signal
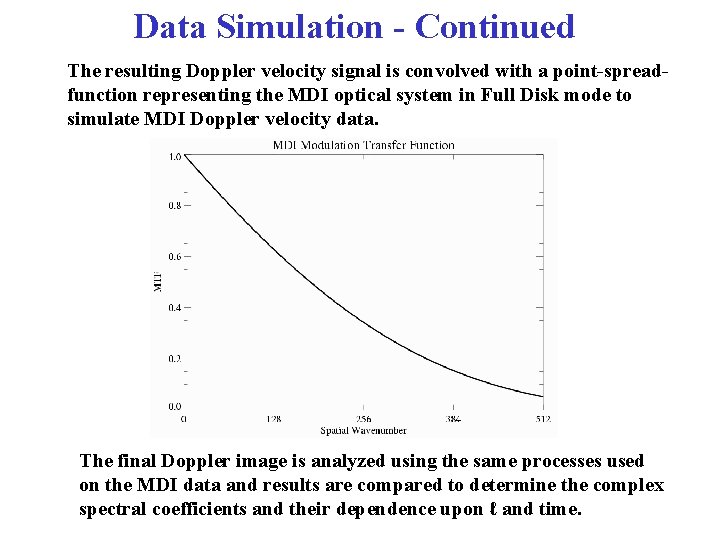
Data Simulation - Continued The resulting Doppler velocity signal is convolved with a point-spreadfunction representing the MDI optical system in Full Disk mode to simulate MDI Doppler velocity data. The final Doppler image is analyzed using the same processes used on the MDI data and results are compared to determine the complex spectral coefficients and their dependence upon ℓ and time.
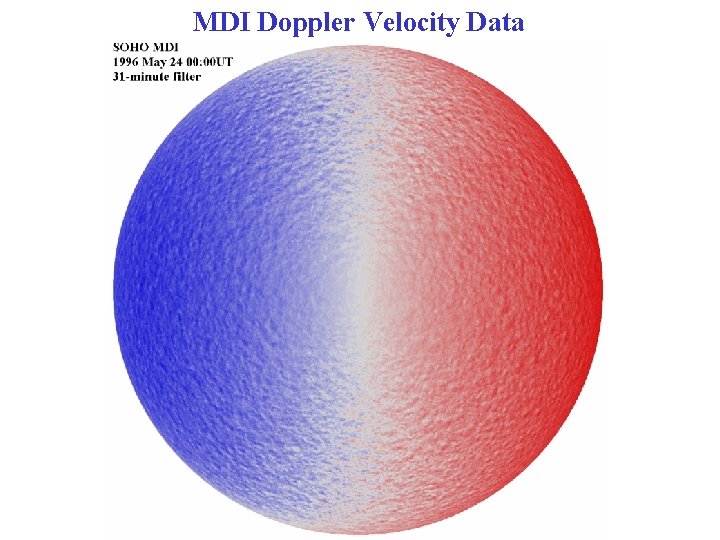
MDI Doppler Velocity Data
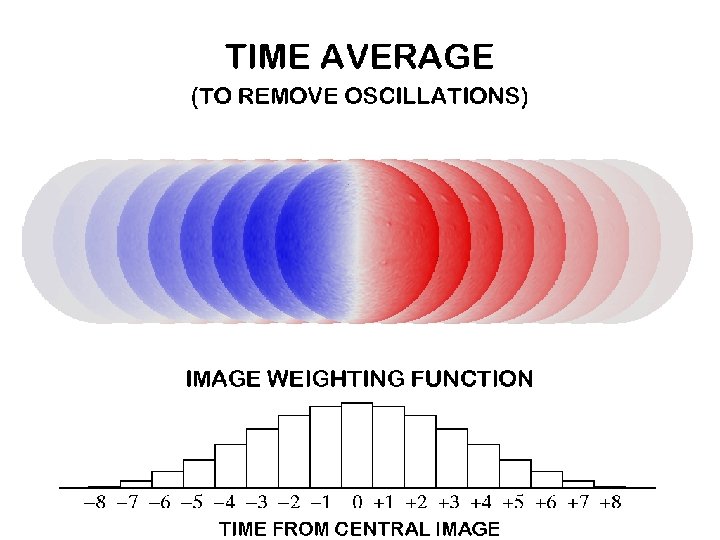
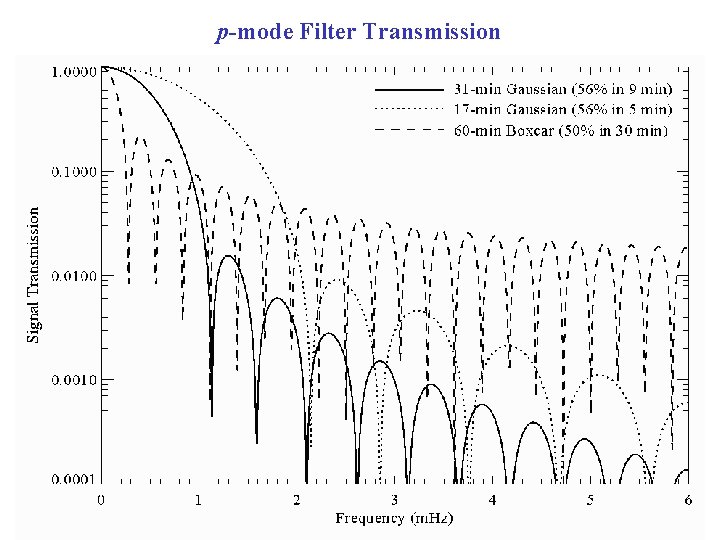
p-mode Filter Transmission
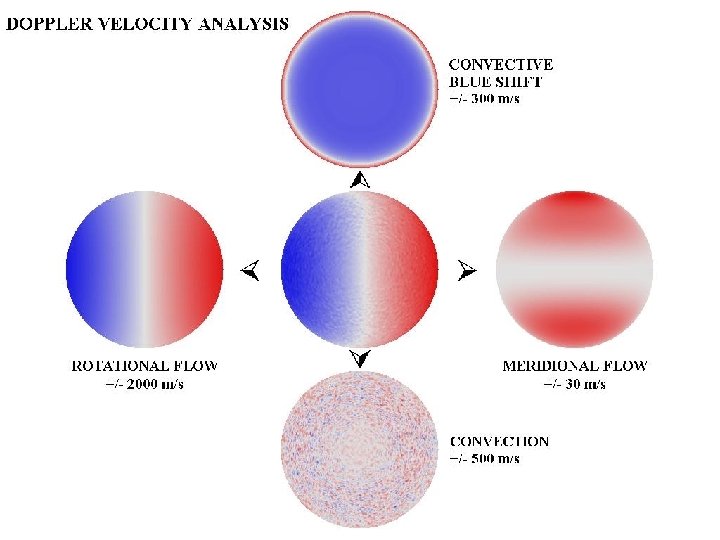
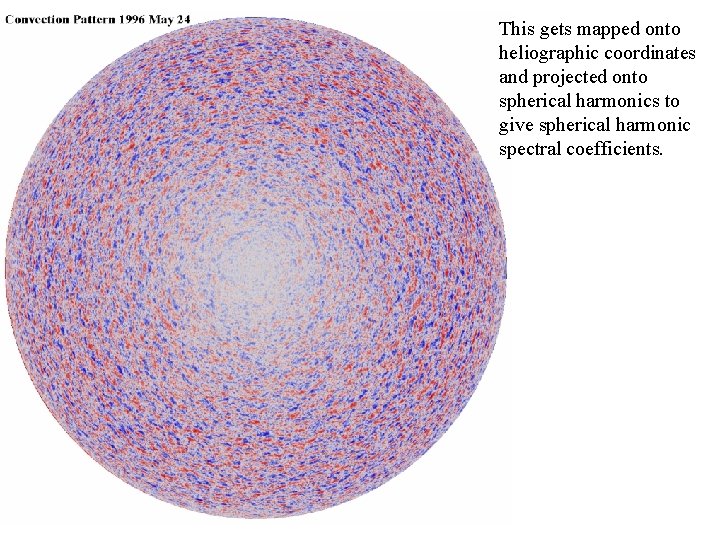
This gets mapped onto heliographic coordinates and projected onto spherical harmonics to give spherical harmonic spectral coefficients.
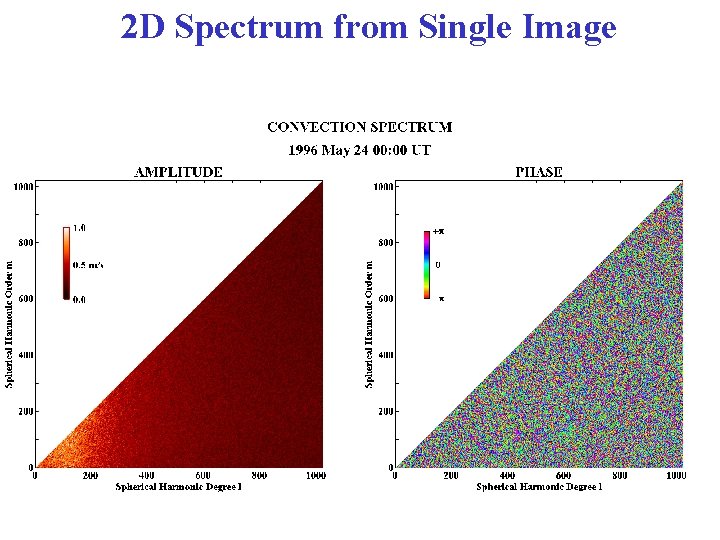
2 D Spectrum from Single Image
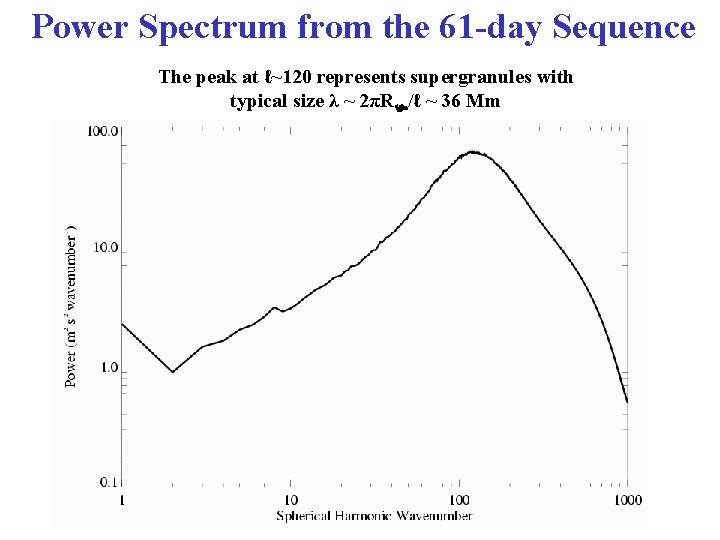
Power Spectrum from the 61 -day Sequence The peak at ℓ~120 represents supergranules with typical size λ ~ 2πR /ℓ ~ 36 Mm
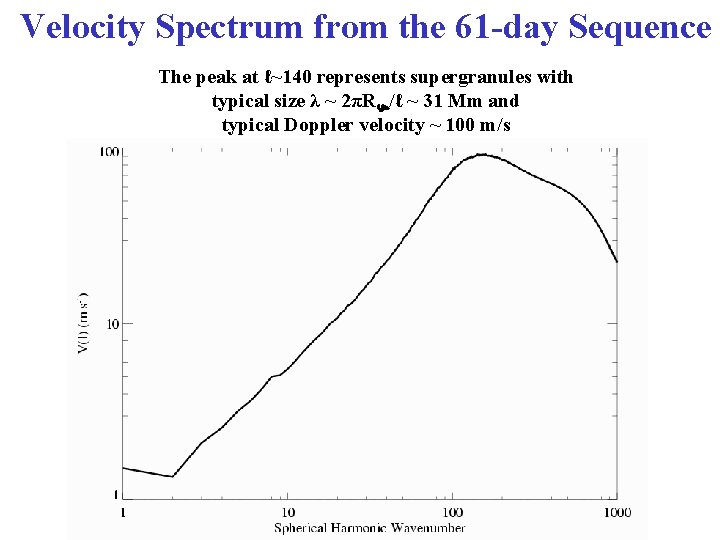
Velocity Spectrum from the 61 -day Sequence The peak at ℓ~140 represents supergranules with typical size λ ~ 2πR /ℓ ~ 31 Mm and typical Doppler velocity ~ 100 m/s
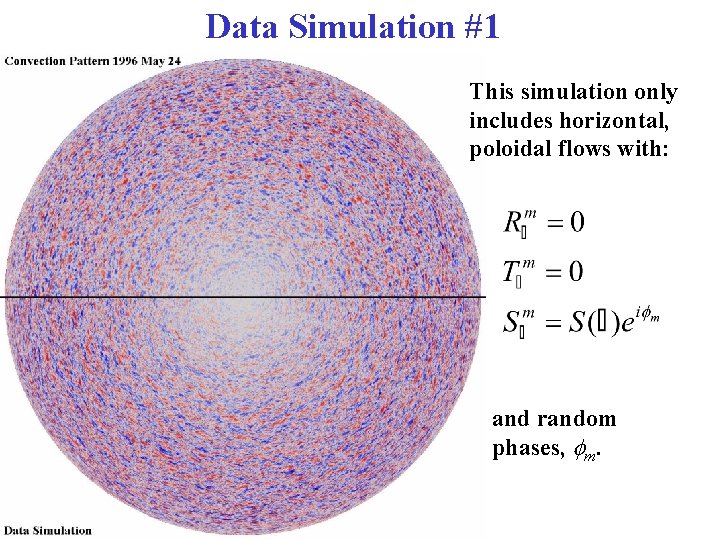
Data Simulation #1 This simulation only includes horizontal, poloidal flows with: and random phases, m.
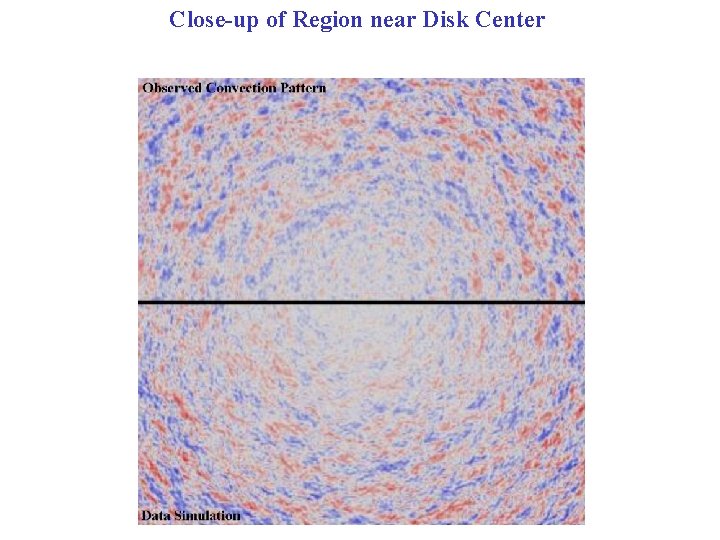
Close-up of Region near Disk Center
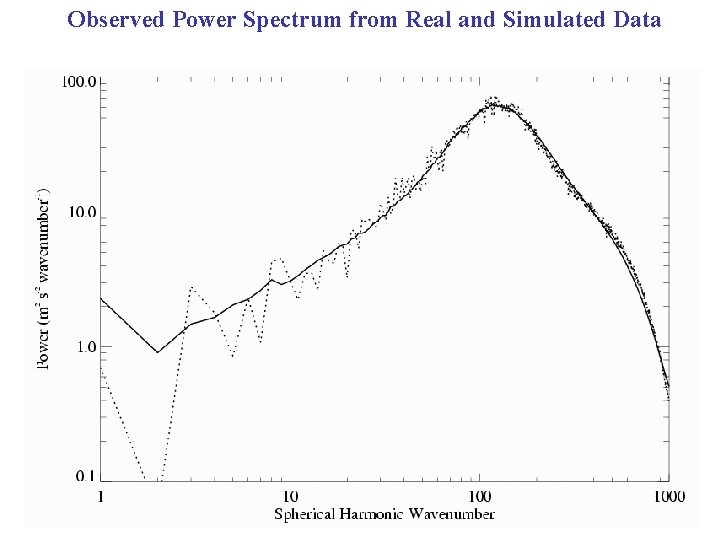
Observed Power Spectrum from Real and Simulated Data
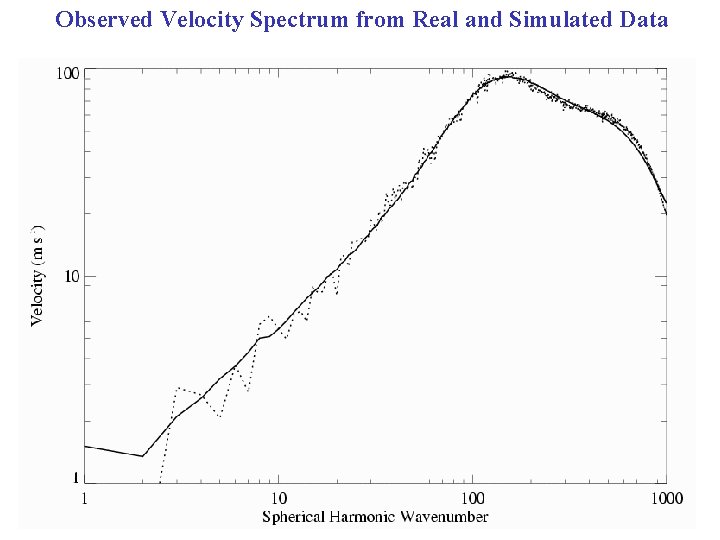
Observed Velocity Spectrum from Real and Simulated Data
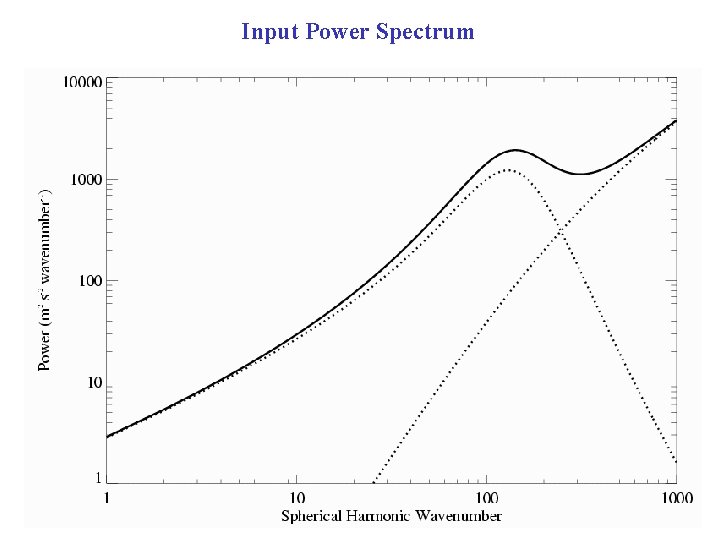
Input Power Spectrum
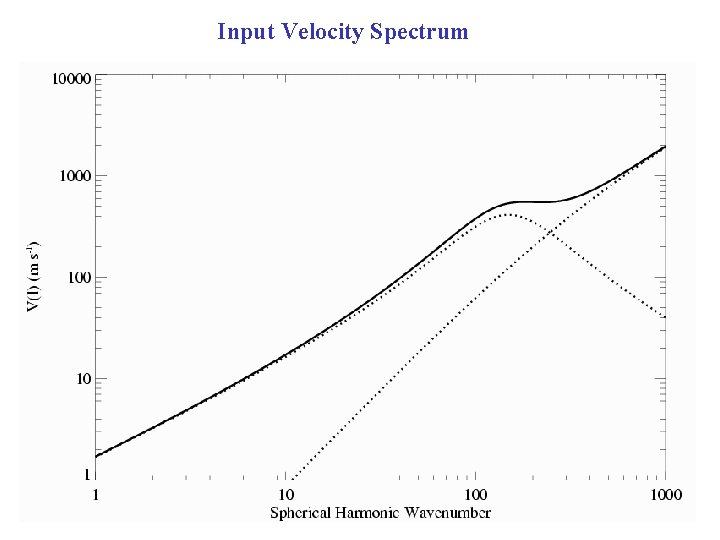
Input Velocity Spectrum
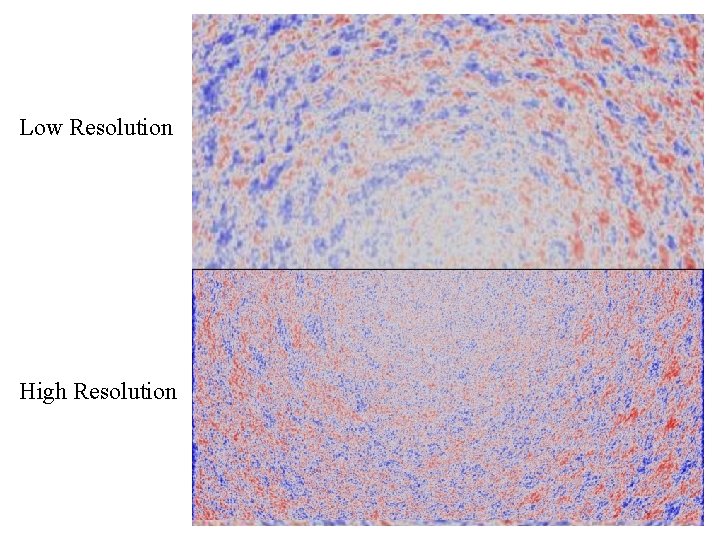
Low Resolution High Resolution
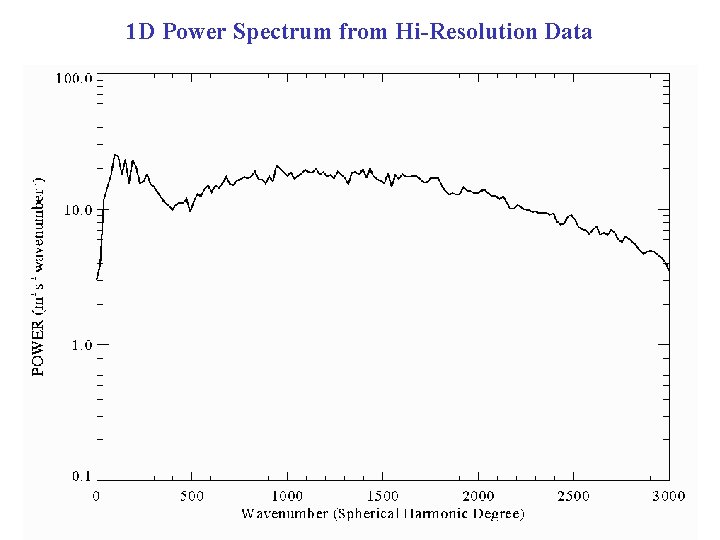
1 D Power Spectrum from Hi-Resolution Data
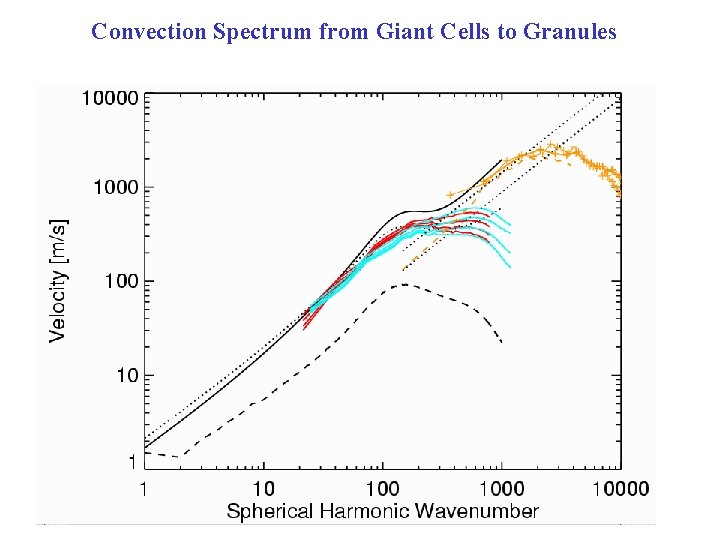
Convection Spectrum from Giant Cells to Granules
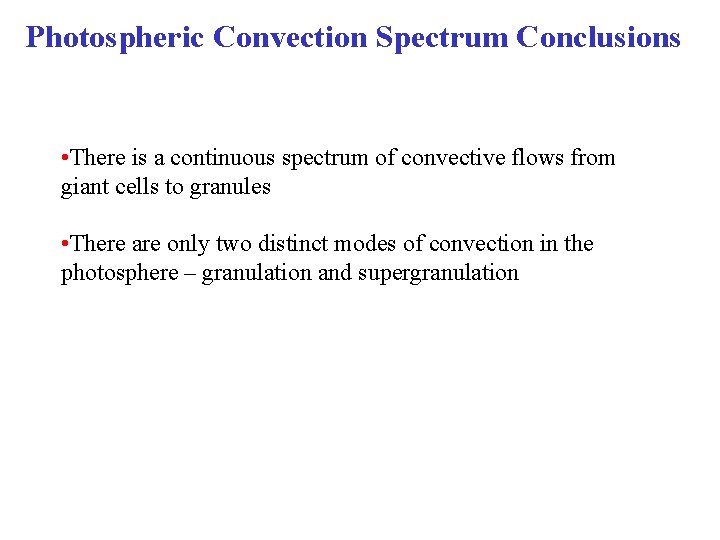
Photospheric Convection Spectrum Conclusions • There is a continuous spectrum of convective flows from giant cells to granules • There are only two distinct modes of convection in the photosphere – granulation and supergranulation
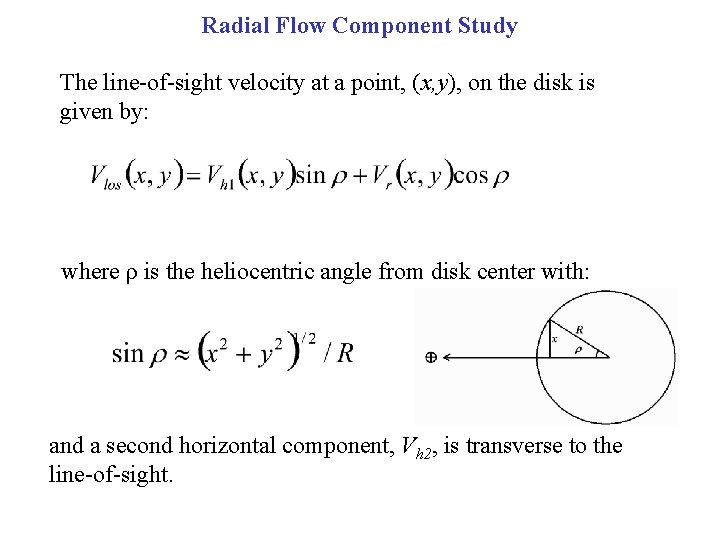
Radial Flow Component Study The line-of-sight velocity at a point, (x, y), on the disk is given by: where ρ is the heliocentric angle from disk center with: and a second horizontal component, Vh 2, is transverse to the line-of-sight.
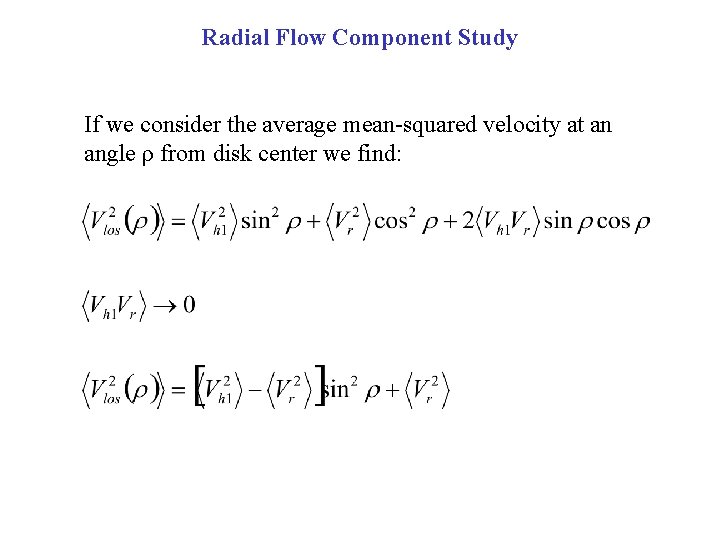
Radial Flow Component Study If we consider the average mean-squared velocity at an angle ρ from disk center we find:
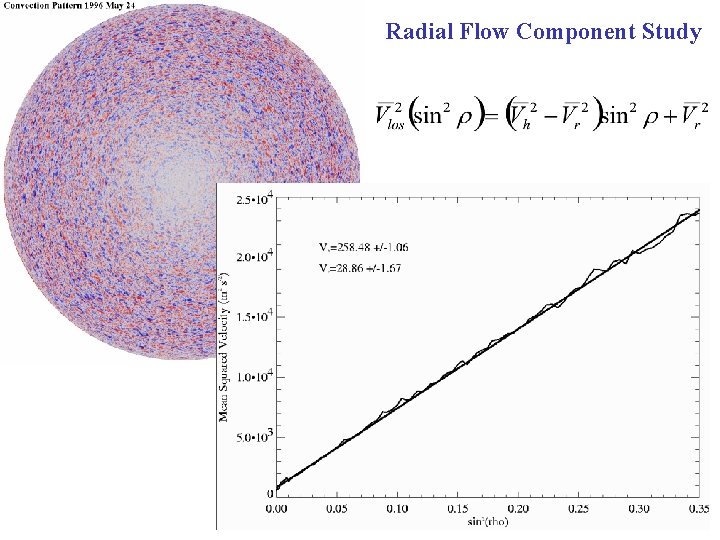
Radial Flow Component Study
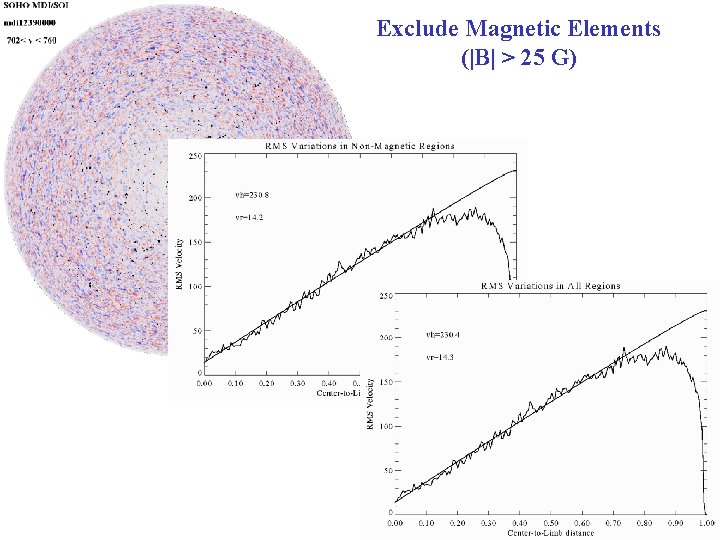
Exclude Magnetic Elements (|B| > 25 G)
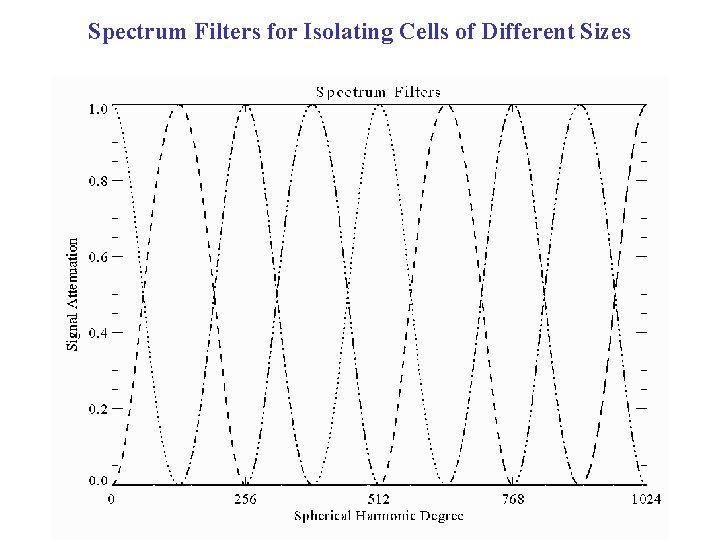
Spectrum Filters for Isolating Cells of Different Sizes
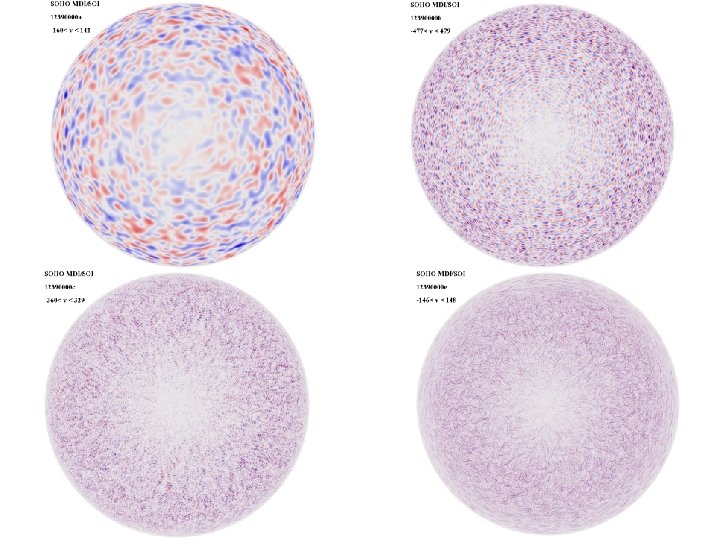
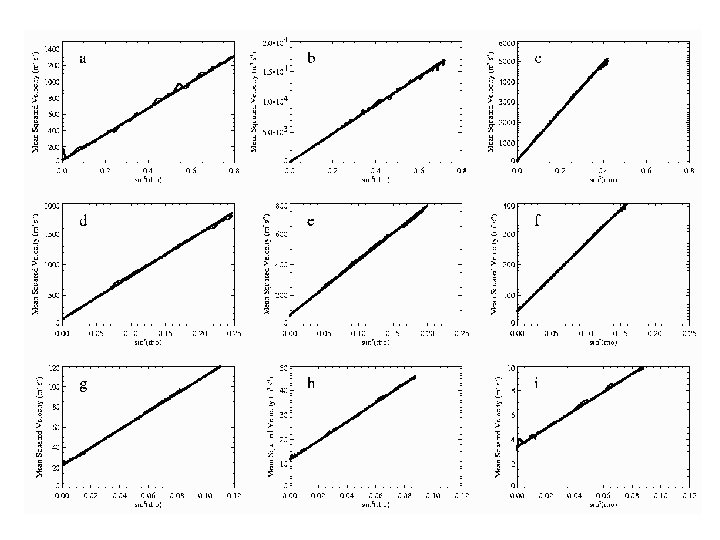
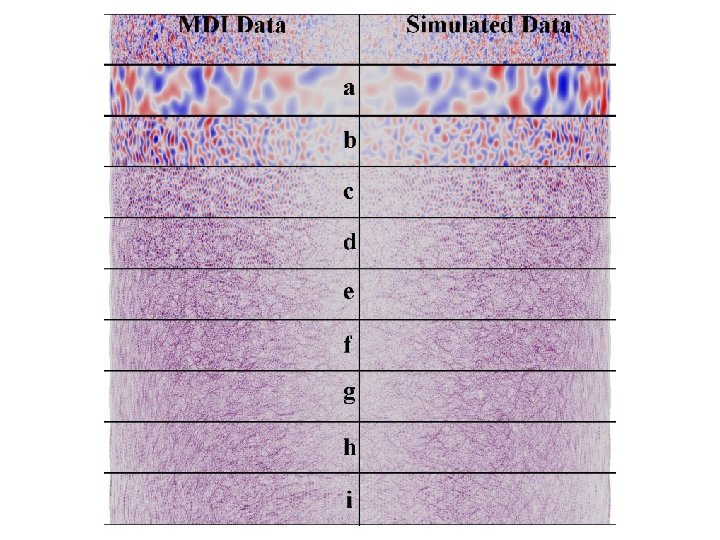
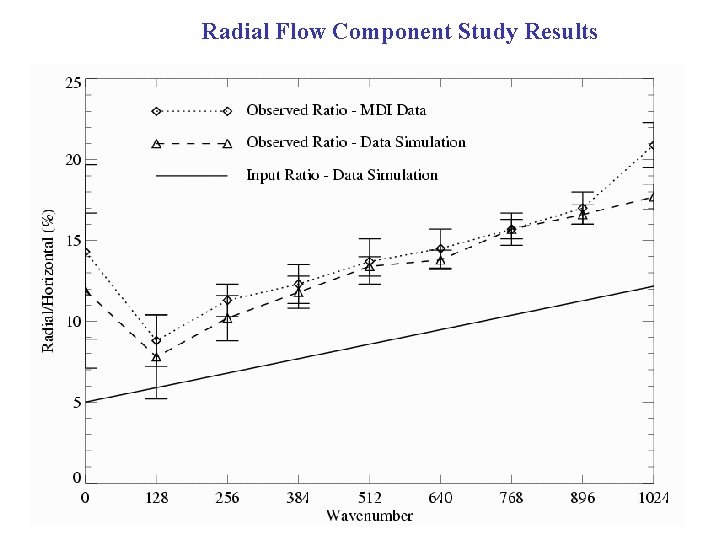
Radial Flow Component Study Results
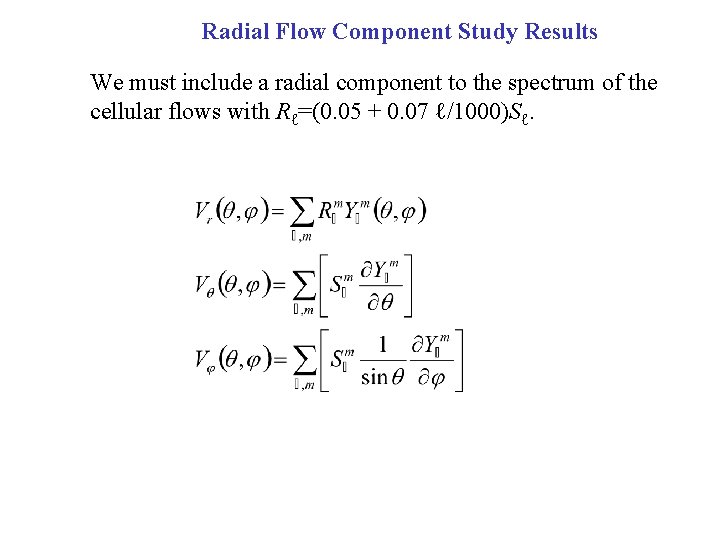
Radial Flow Component Study Results We must include a radial component to the spectrum of the cellular flows with Rℓ=(0. 05 + 0. 07 ℓ/1000)Sℓ.
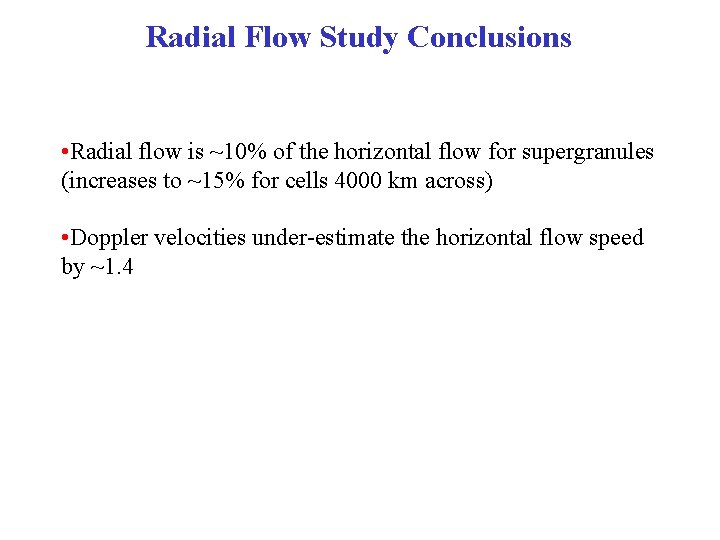
Radial Flow Study Conclusions • Radial flow is ~10% of the horizontal flow for supergranules (increases to ~15% for cells 4000 km across) • Doppler velocities under-estimate the horizontal flow speed by ~1. 4
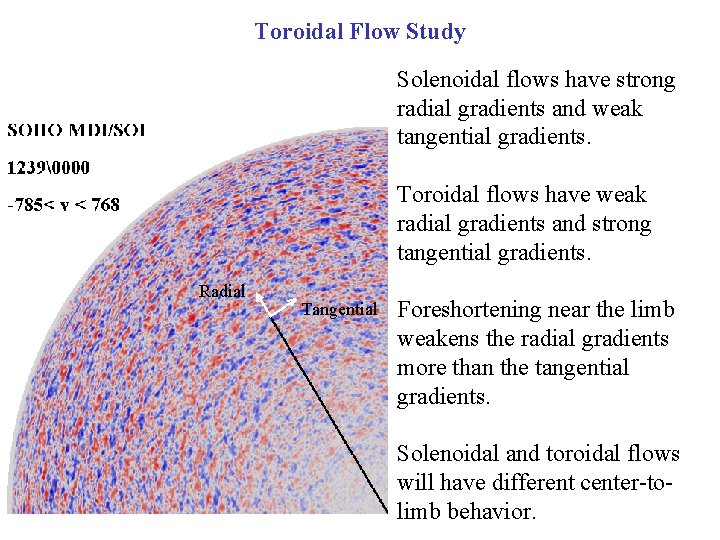
Toroidal Flow Study Solenoidal flows have strong radial gradients and weak tangential gradients. Toroidal flows have weak radial gradients and strong tangential gradients. Radial Tangential Foreshortening near the limb weakens the radial gradients more than the tangential gradients. Solenoidal and toroidal flows will have different center-tolimb behavior.
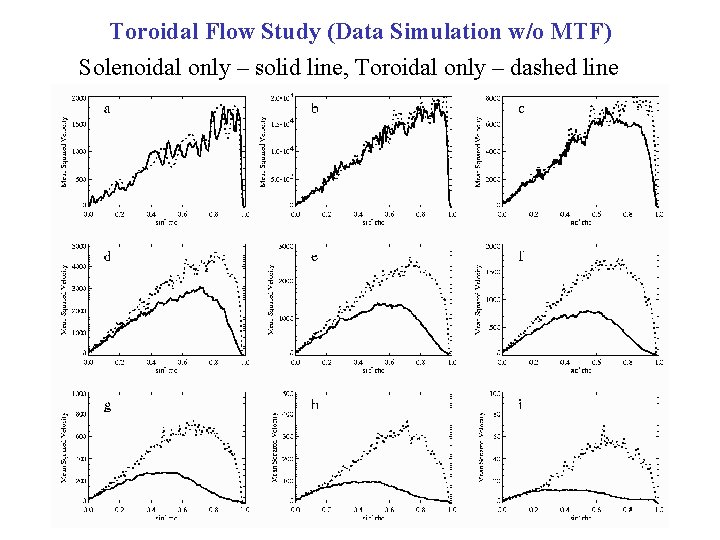
Toroidal Flow Study (Data Simulation w/o MTF) Solenoidal only – solid line, Toroidal only – dashed line
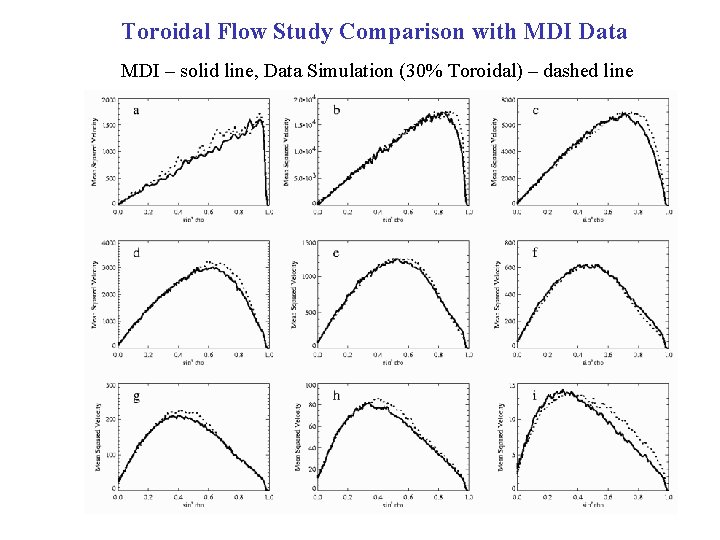
Toroidal Flow Study Comparison with MDI Data MDI – solid line, Data Simulation (30% Toroidal) – dashed line
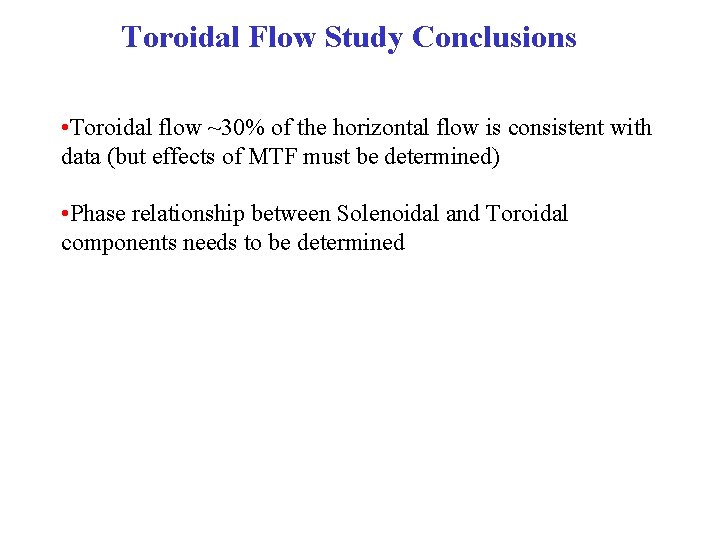
Toroidal Flow Study Conclusions • Toroidal flow ~30% of the horizontal flow is consistent with data (but effects of MTF must be determined) • Phase relationship between Solenoidal and Toroidal components needs to be determined
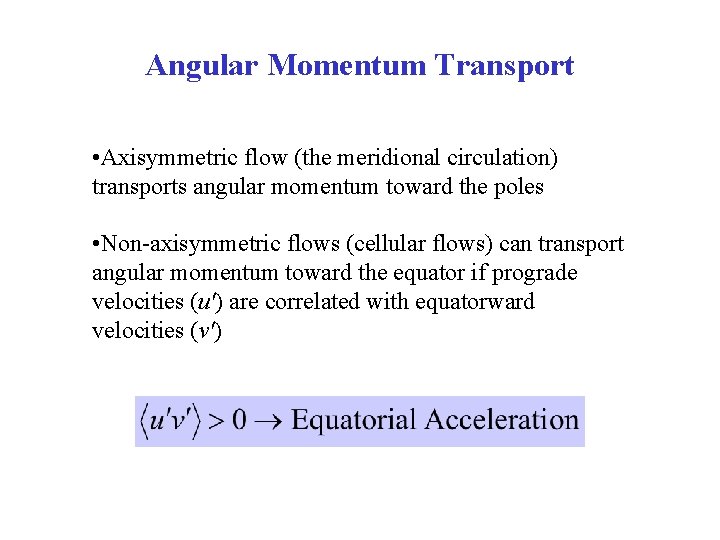
Angular Momentum Transport • Axisymmetric flow (the meridional circulation) transports angular momentum toward the poles • Non-axisymmetric flows (cellular flows) can transport angular momentum toward the equator if prograde velocities (u') are correlated with equatorward velocities (v')
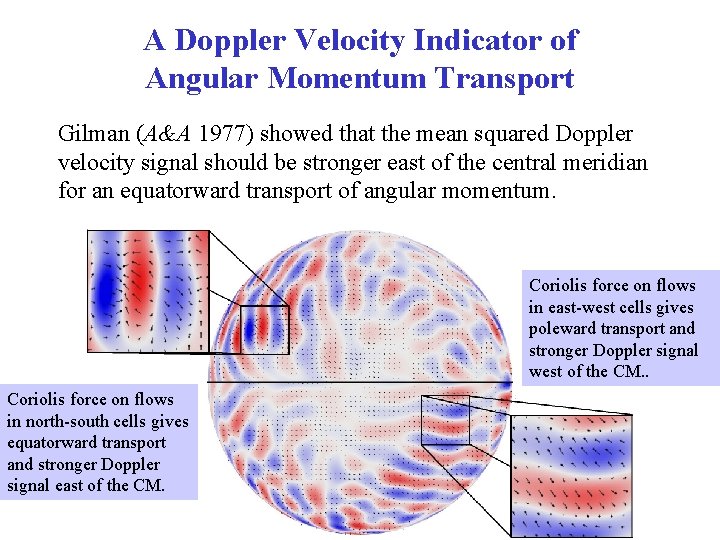
A Doppler Velocity Indicator of Angular Momentum Transport Gilman (A&A 1977) showed that the mean squared Doppler velocity signal should be stronger east of the central meridian for an equatorward transport of angular momentum. Coriolis force on flows in east-west cells gives poleward transport and stronger Doppler signal west of the CM. . Coriolis force on flows in north-south cells gives equatorward transport and stronger Doppler signal east of the CM.
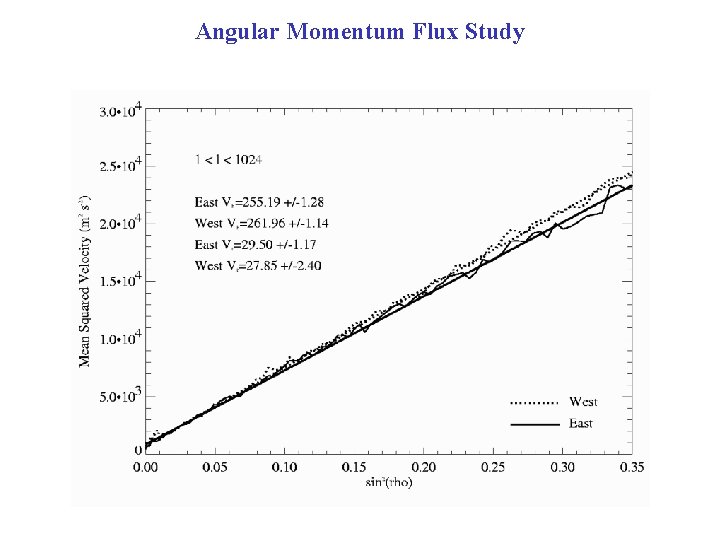
Angular Momentum Flux Study
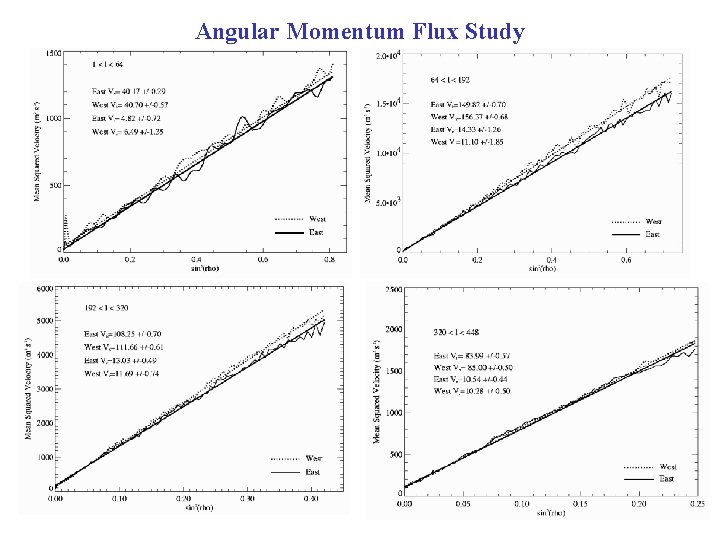
Angular Momentum Flux Study
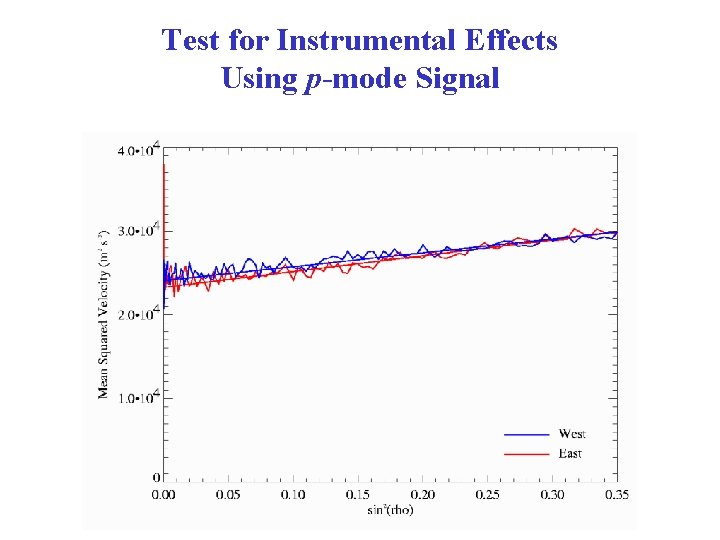
Test for Instrumental Effects Using p-mode Signal
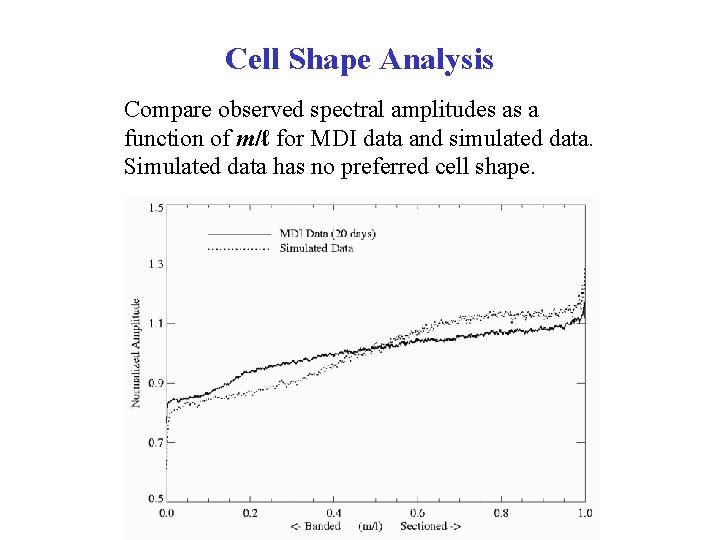
Cell Shape Analysis Compare observed spectral amplitudes as a function of m/ℓ for MDI data and simulated data. Simulated data has no preferred cell shape.
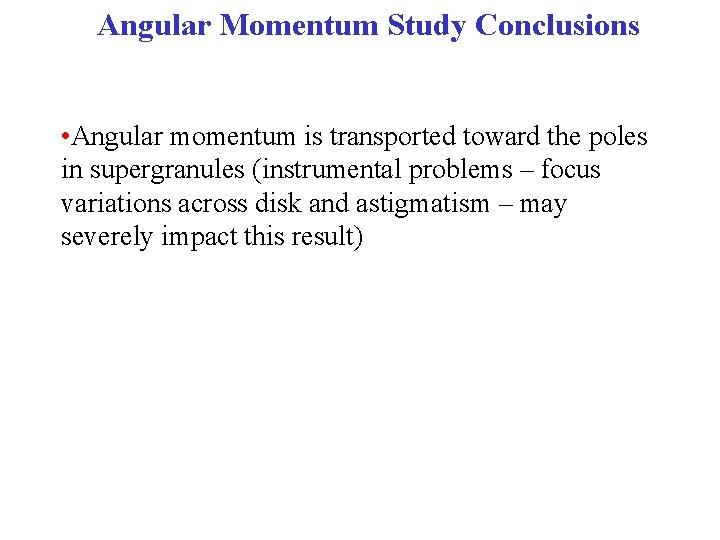
Angular Momentum Study Conclusions • Angular momentum is transported toward the poles in supergranules (instrumental problems – focus variations across disk and astigmatism – may severely impact this result)
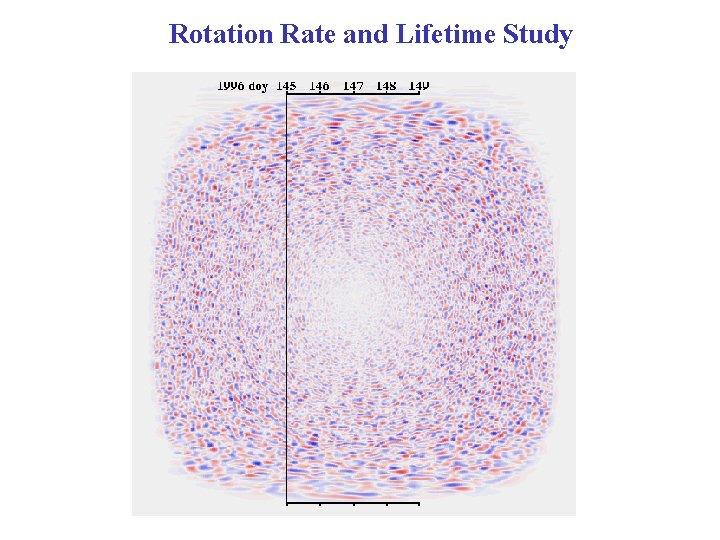
Rotation Rate and Lifetime Study
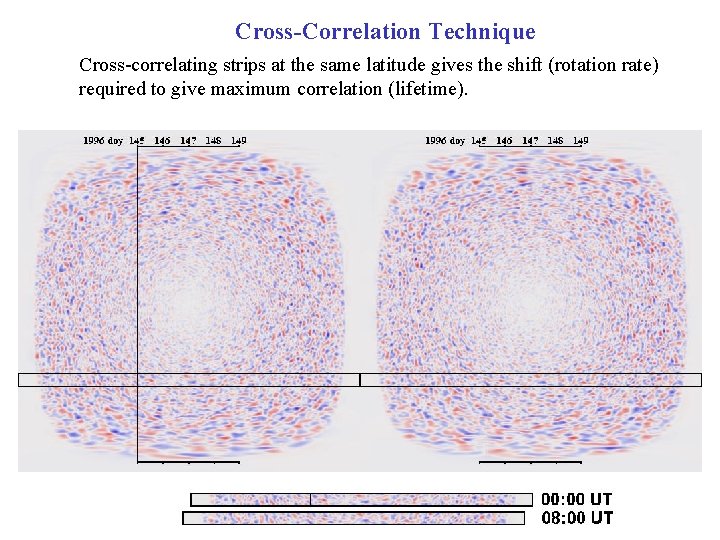
Cross-Correlation Technique Cross-correlating strips at the same latitude gives the shift (rotation rate) required to give maximum correlation (lifetime).
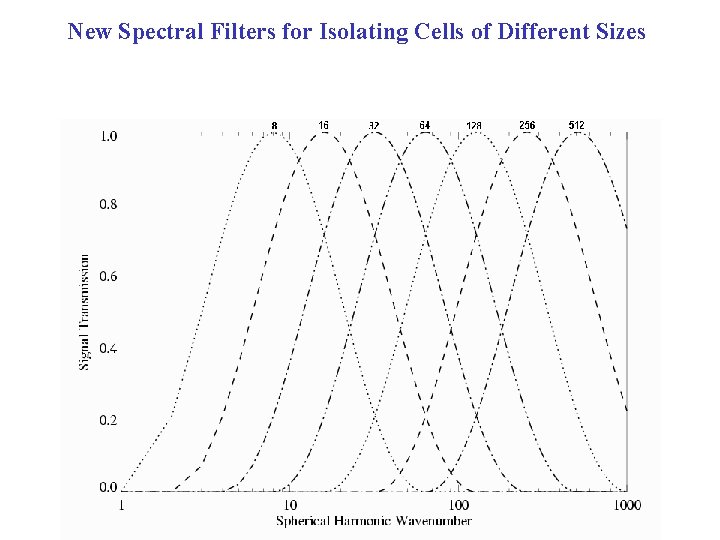
New Spectral Filters for Isolating Cells of Different Sizes
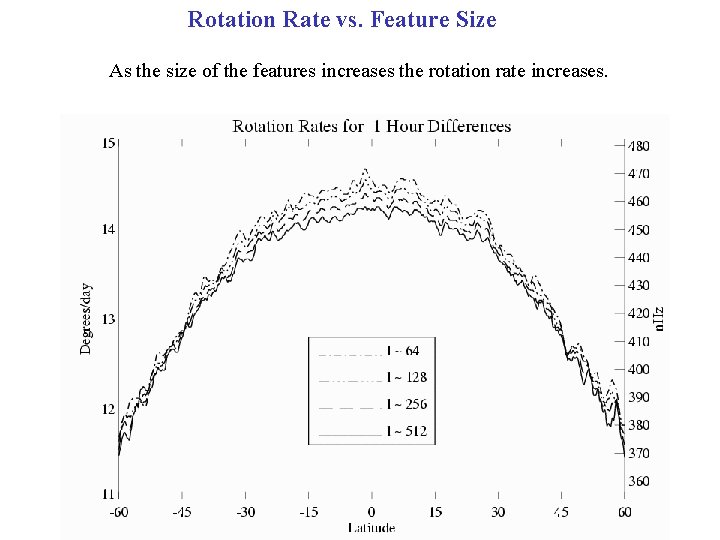
Rotation Rate vs. Feature Size As the size of the features increases the rotation rate increases.
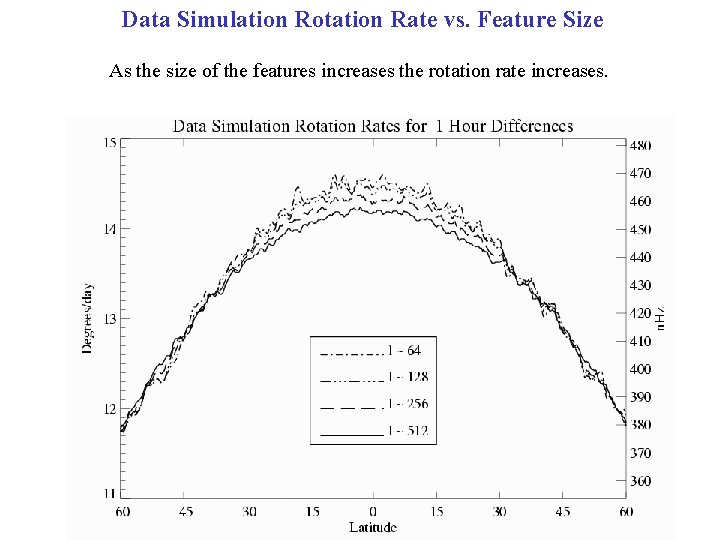
Data Simulation Rotation Rate vs. Feature Size As the size of the features increases the rotation rate increases.
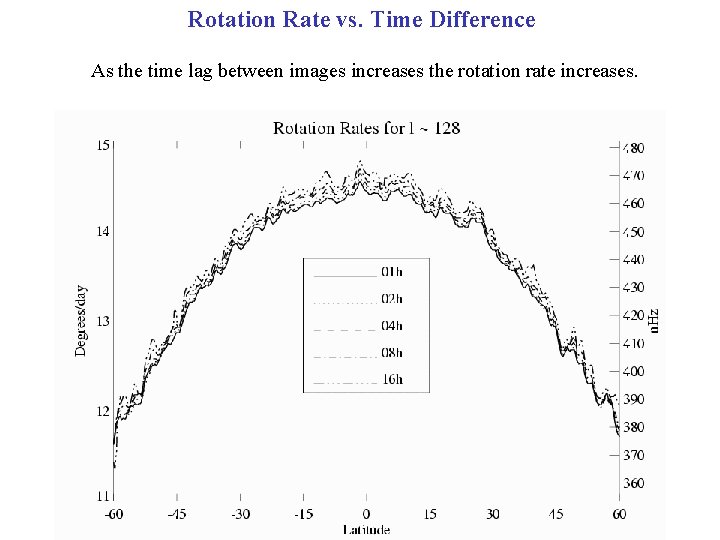
Rotation Rate vs. Time Difference As the time lag between images increases the rotation rate increases.
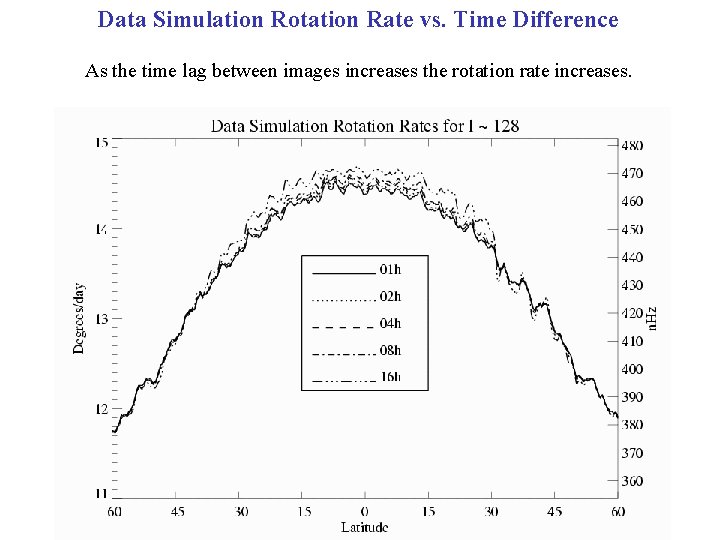
Data Simulation Rotation Rate vs. Time Difference As the time lag between images increases the rotation rate increases.
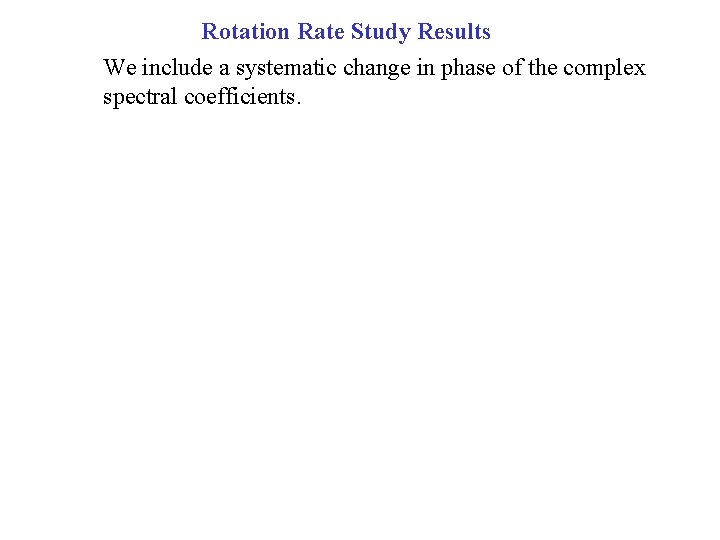
Rotation Rate Study Results We include a systematic change in phase of the complex spectral coefficients.
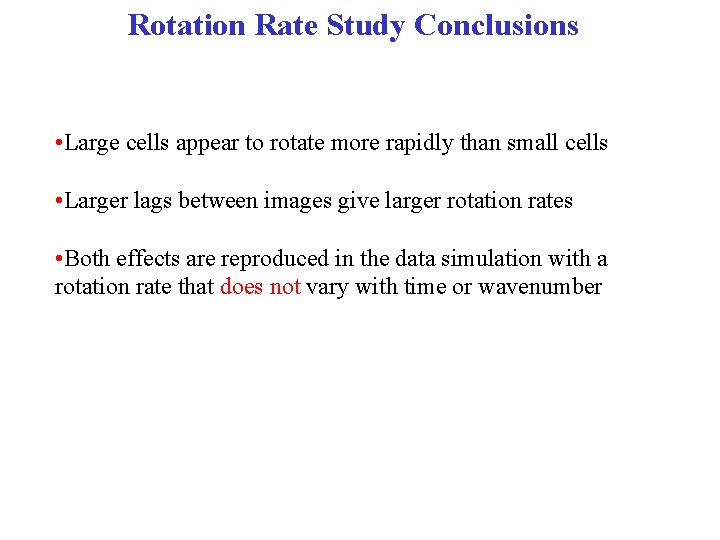
Rotation Rate Study Conclusions • Large cells appear to rotate more rapidly than small cells • Larger lags between images give larger rotation rates • Both effects are reproduced in the data simulation with a rotation rate that does not vary with time or wavenumber
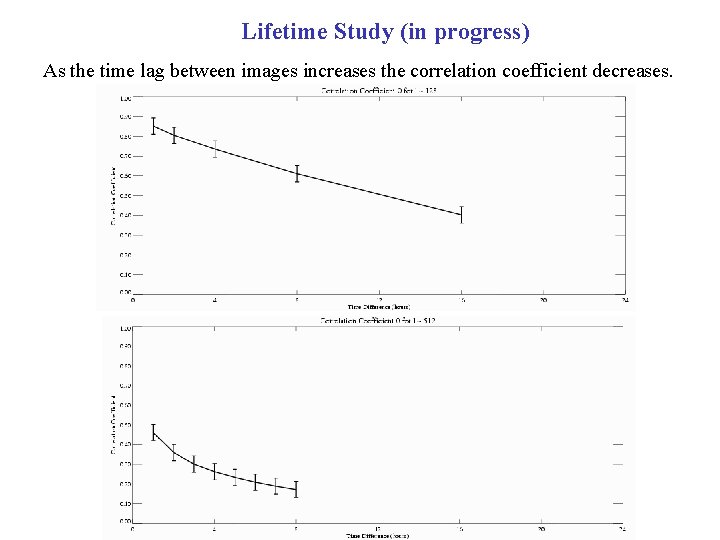
Lifetime Study (in progress) As the time lag between images increases the correlation coefficient decreases.
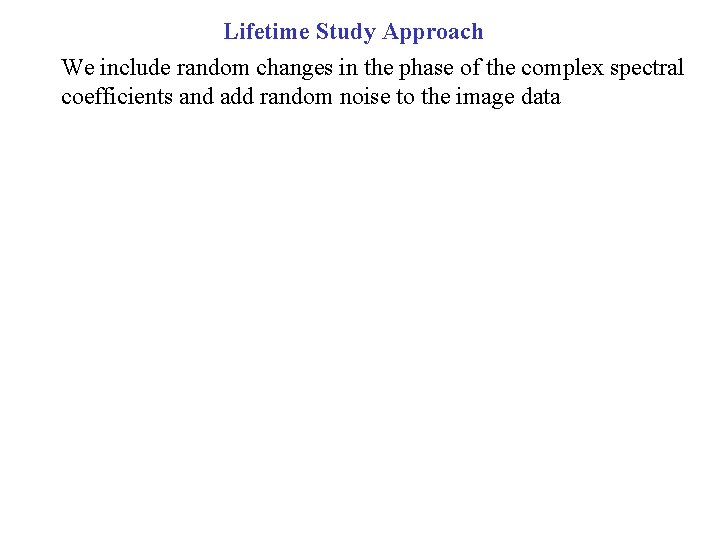
Lifetime Study Approach We include random changes in the phase of the complex spectral coefficients and add random noise to the image data
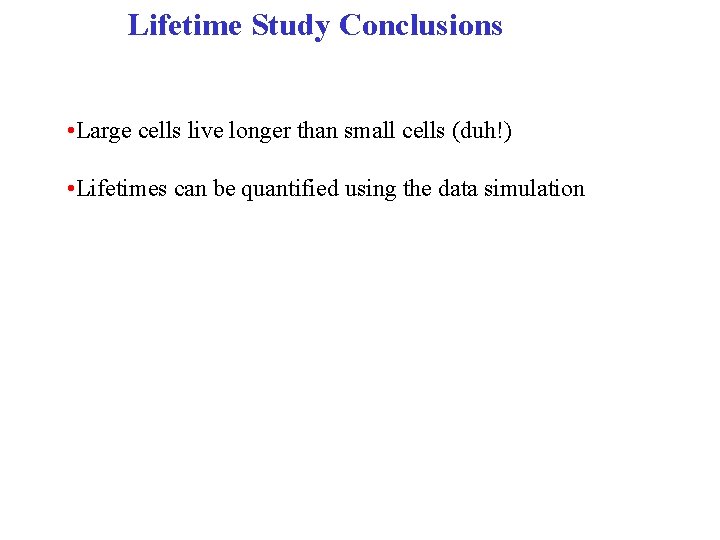
Lifetime Study Conclusions • Large cells live longer than small cells (duh!) • Lifetimes can be quantified using the data simulation
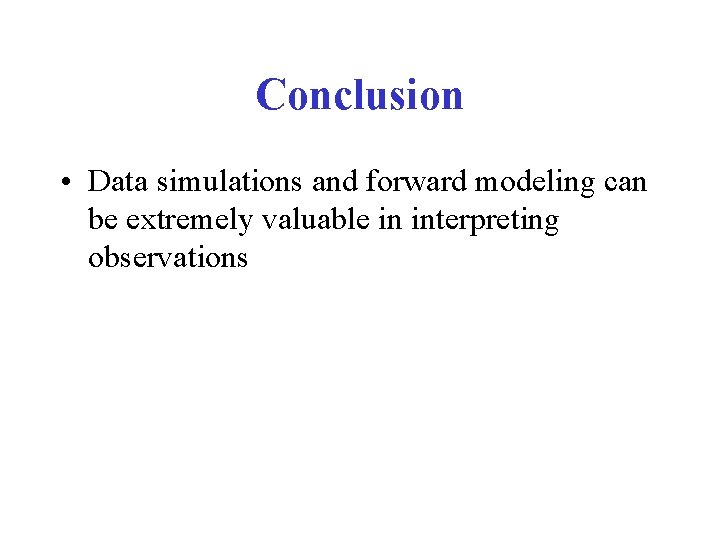
Conclusion • Data simulations and forward modeling can be extremely valuable in interpreting observations
Berkshire hathaway anne hathaway
Characterizing schedules based on recoverability
Characterizing schedules based on serializability
Characterizing schedules based on serializability
David hathaway nasa
David hathaway nasa
Berkshire hathaway products and services
William shakespeare anne hathaway poem
Anne hathaway duffy
Ann hathaway poem
Anne hathaway shakespeare biography
Anne hathaway memeleri
Anne hathaway background
In mrs tilscher's class genius
Ron hathaway
Poem the river summary
White queen anne hathaway
Palmar mandibular reflex
David john frank
John 13 1 17
John hennessy and david patterson
James mill asociacionismo
Yamuna river
Pv of cash flows formula
Great tao
Eight generic channel flows
Raw material in supply chain
Incremental cash flow
Mekong flows through which countries
Payback time formula
Outbound flows
Cmos process flows
Buys ballot law
4 flows of supply chain
Mass of ocean water that flows from one place to another
Cash flow indirect method
Incremental cash flows
Incremental cash flows
How to calculate incremental cash flows
Zero based channel
Contactual efficiency examples
The crest of ignorance
Iatcf
Partial statement of cash flows
What are plant assets
Schedule of cost of goods manufactured
Capital budgeting decisions emphasize flows.
Amg data services
Your mercy flows like a river wide
Census flows mapper
Mutual fund flows and performance in rational markets
Instantaneous gas flows
Fluid is a substance that
Cash flow pro
Cash flow statement prepaid expenses
The statement of cash flows reports
Risk adjusted npv
Juarez company acquired $1 200
My son, give me your heart meaning