Challenges in Computational Functional Genomics Igor Ulitsky Genomics
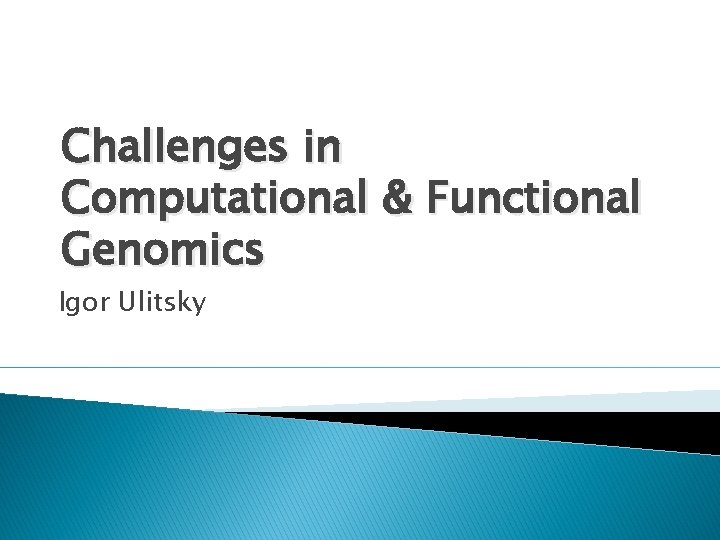
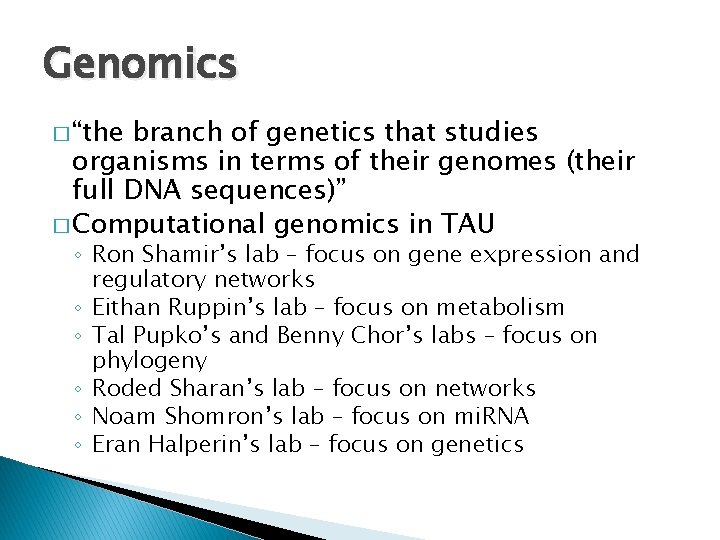
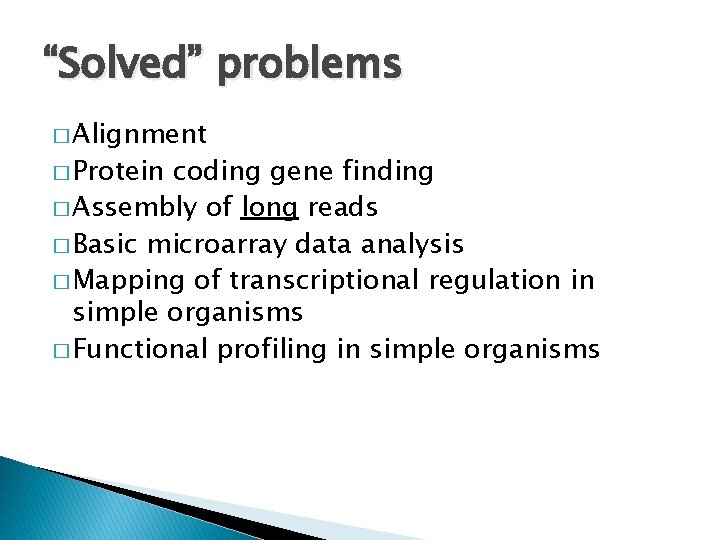
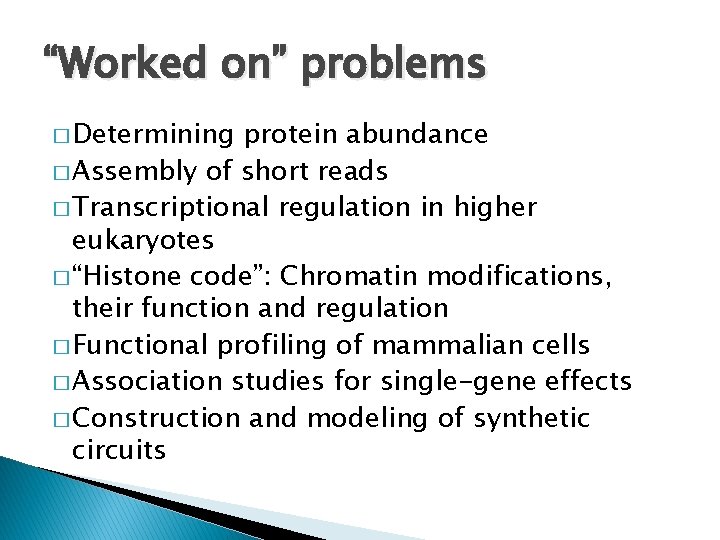
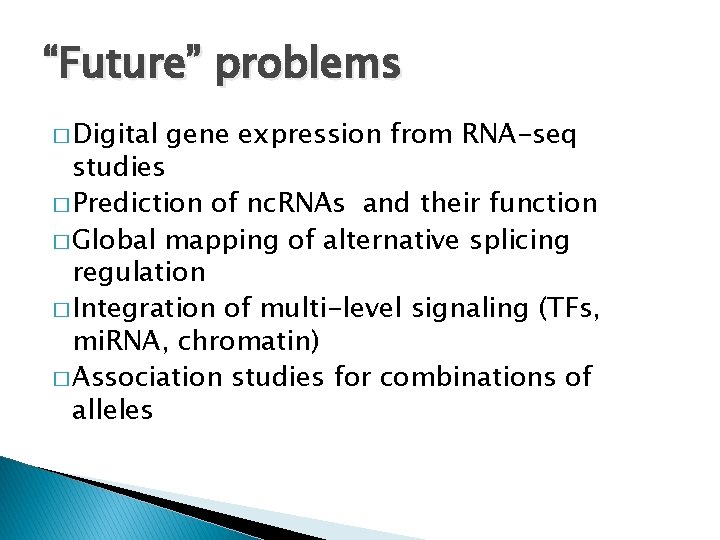
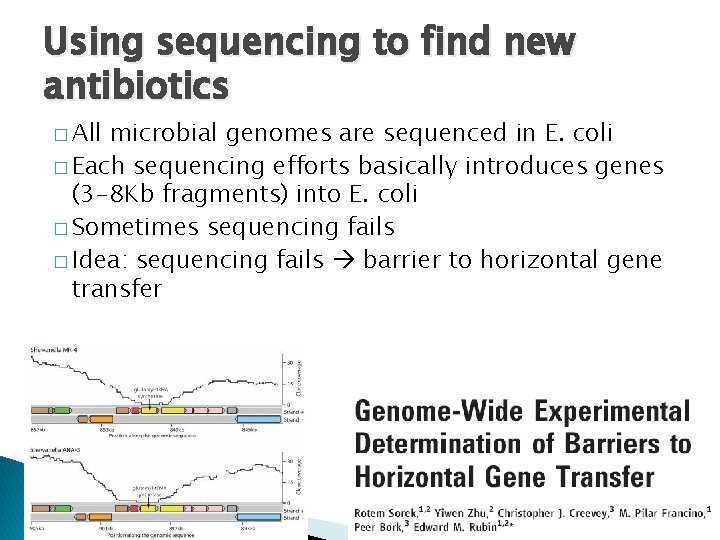
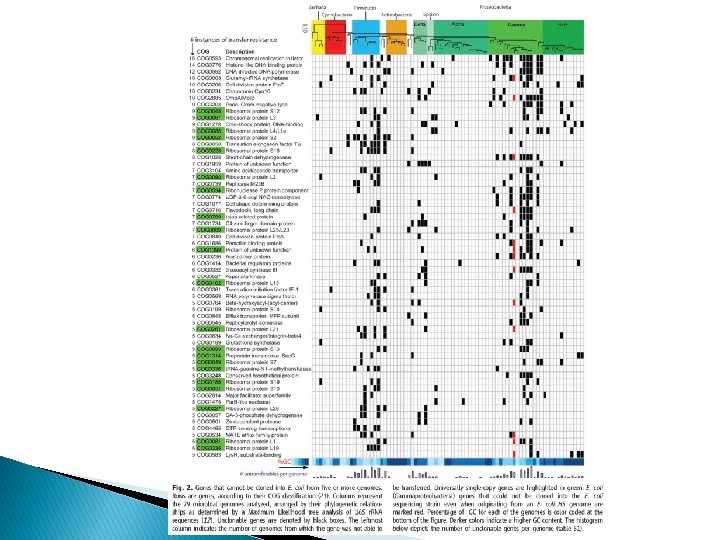
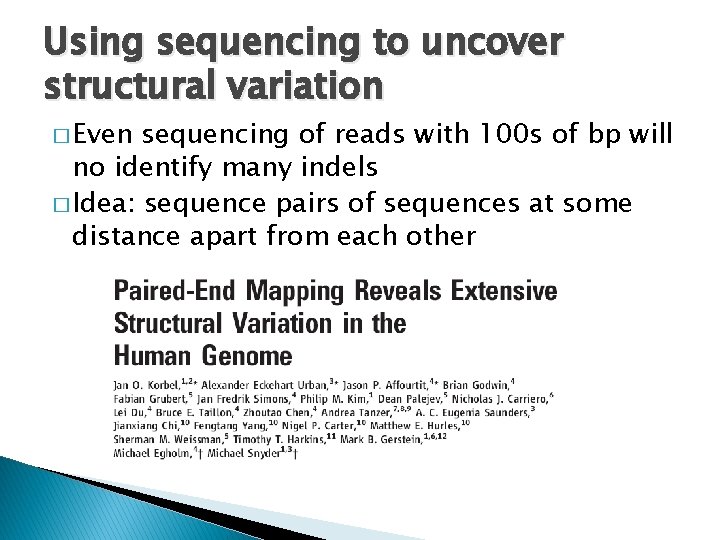
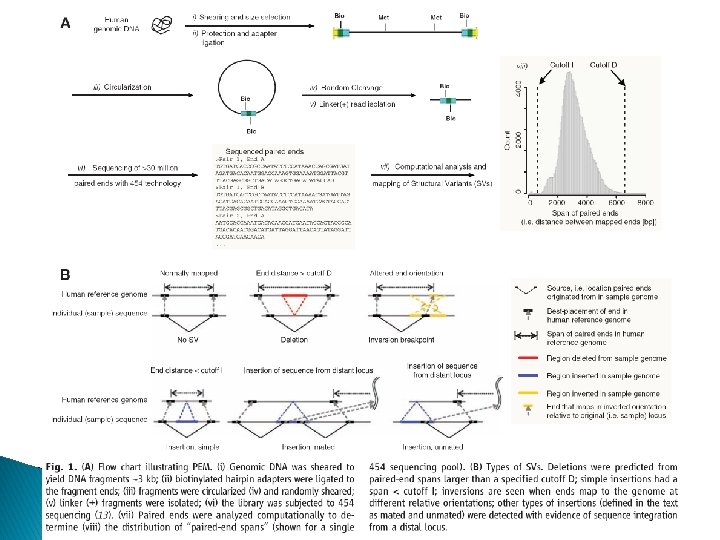
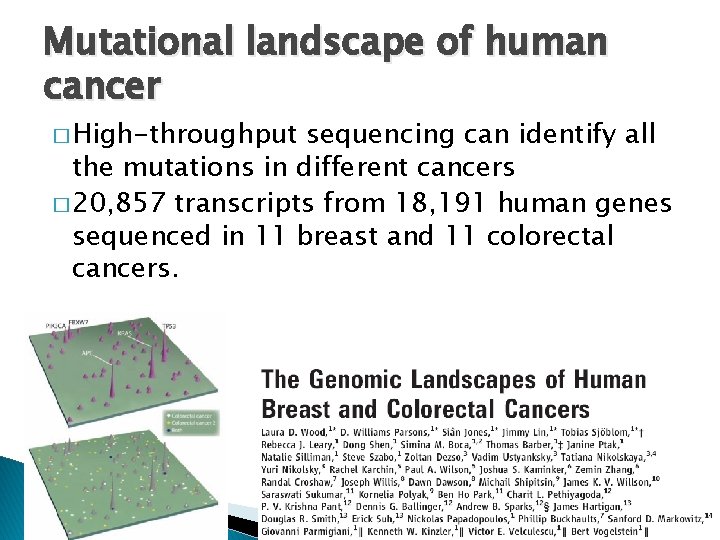
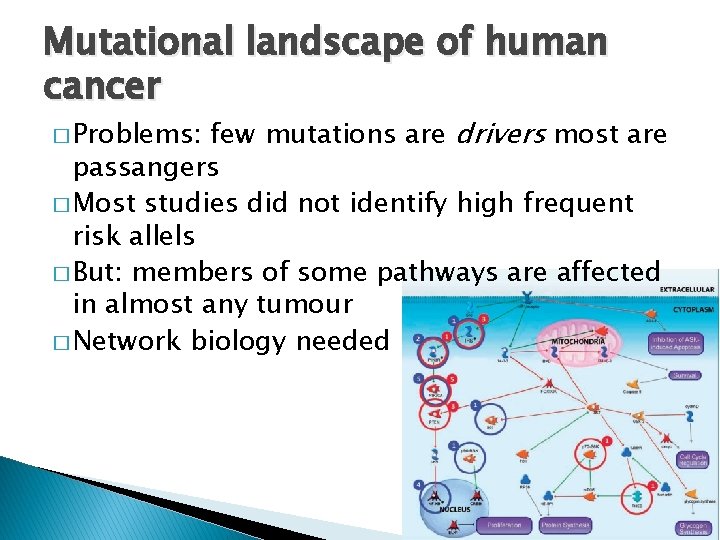
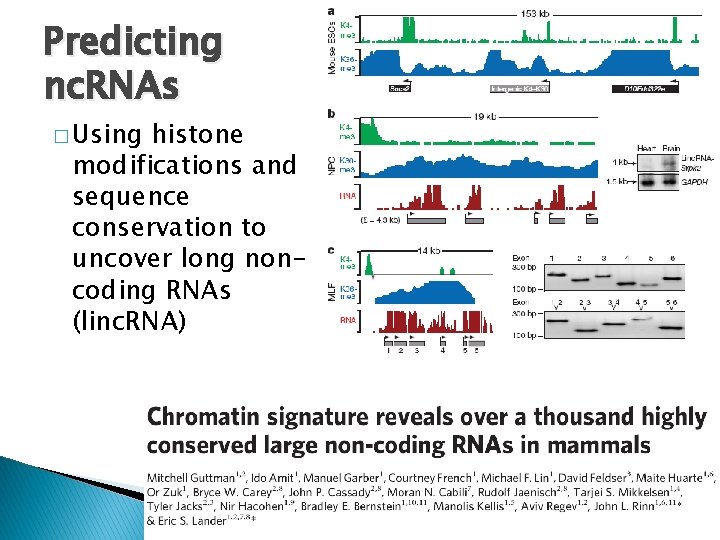
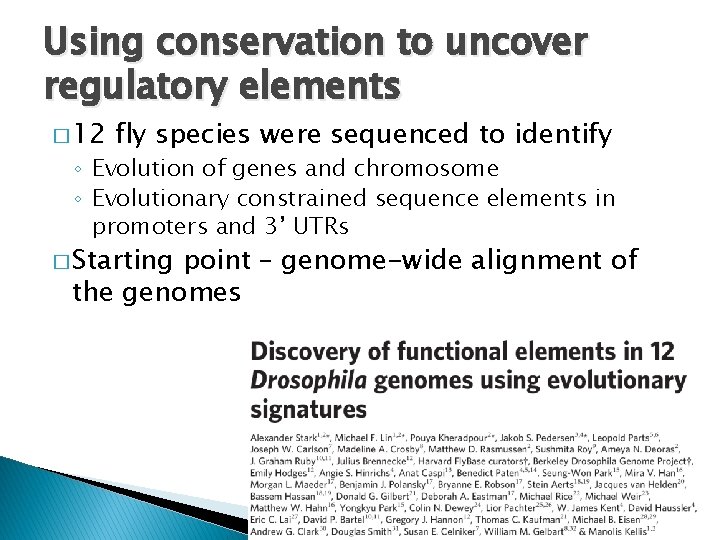
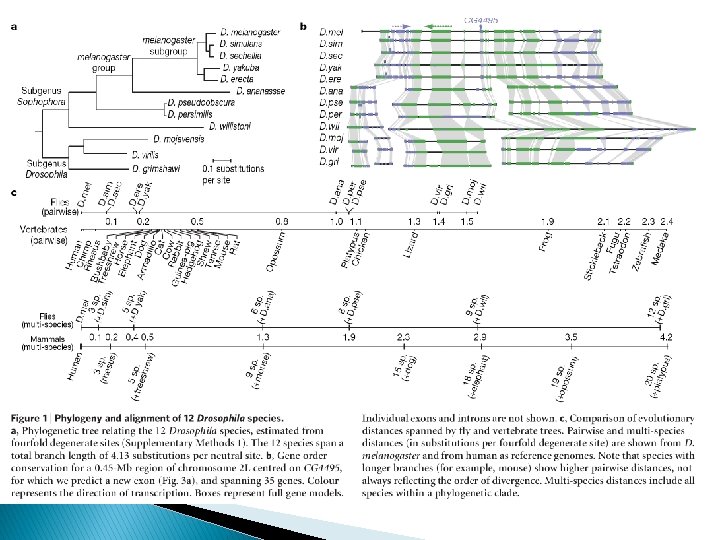
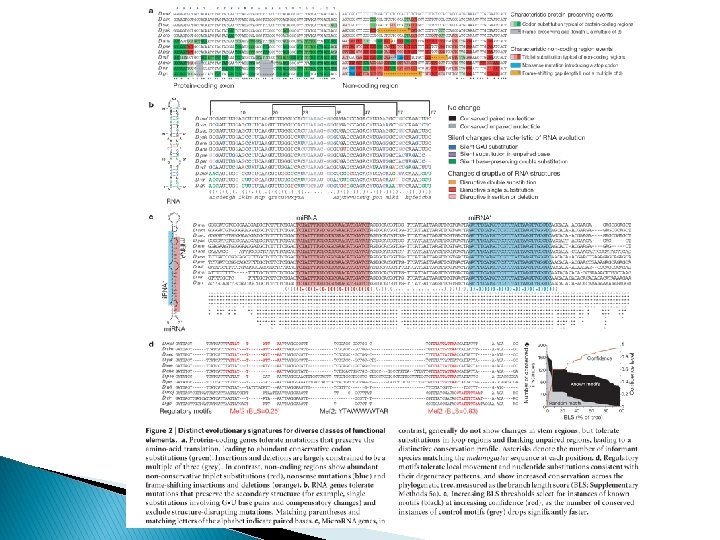
- Slides: 15
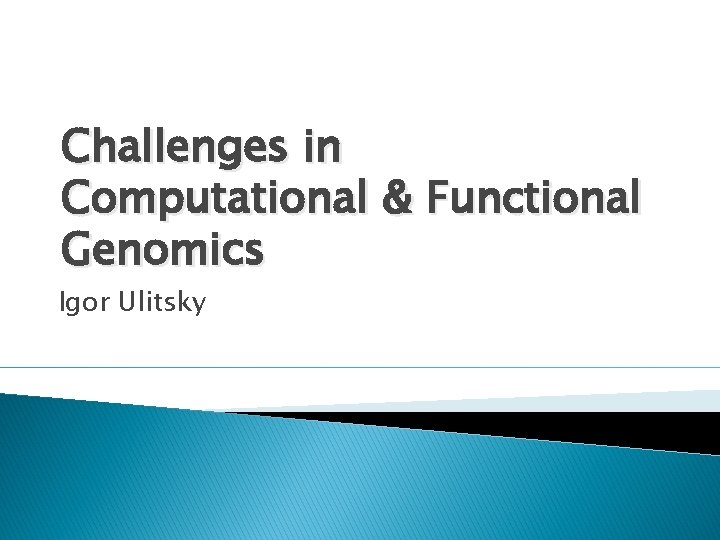
Challenges in Computational & Functional Genomics Igor Ulitsky
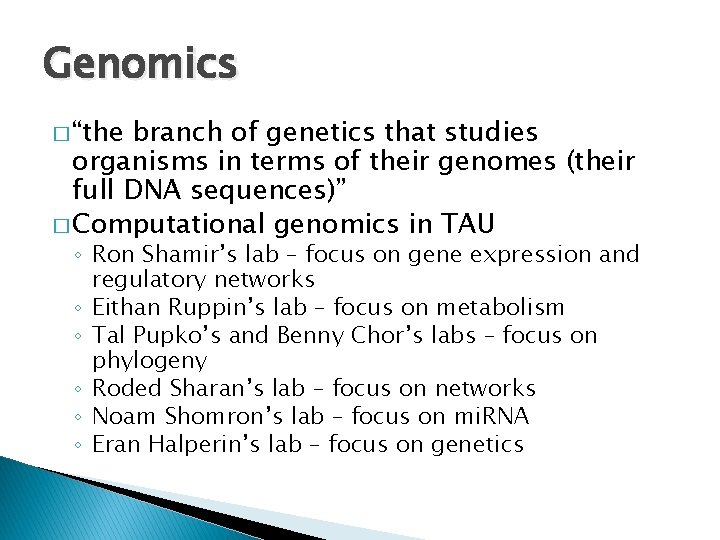
Genomics � “the branch of genetics that studies organisms in terms of their genomes (their full DNA sequences)” � Computational genomics in TAU ◦ Ron Shamir’s lab – focus on gene expression and regulatory networks ◦ Eithan Ruppin’s lab – focus on metabolism ◦ Tal Pupko’s and Benny Chor’s labs – focus on phylogeny ◦ Roded Sharan’s lab – focus on networks ◦ Noam Shomron’s lab – focus on mi. RNA ◦ Eran Halperin’s lab – focus on genetics
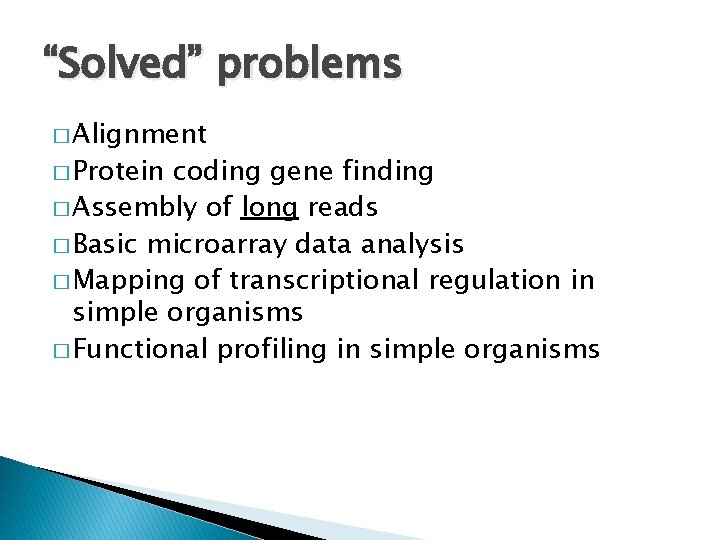
“Solved” problems � Alignment � Protein coding gene finding � Assembly of long reads � Basic microarray data analysis � Mapping of transcriptional regulation in simple organisms � Functional profiling in simple organisms
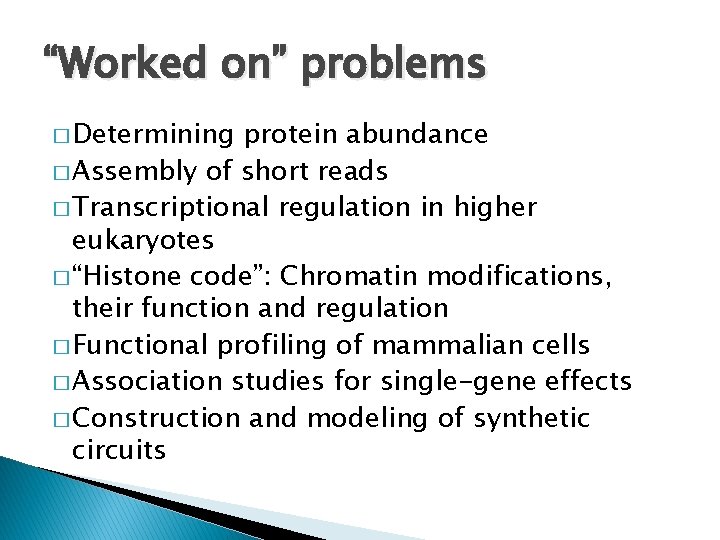
“Worked on” problems � Determining protein abundance � Assembly of short reads � Transcriptional regulation in higher eukaryotes � “Histone code”: Chromatin modifications, their function and regulation � Functional profiling of mammalian cells � Association studies for single-gene effects � Construction and modeling of synthetic circuits
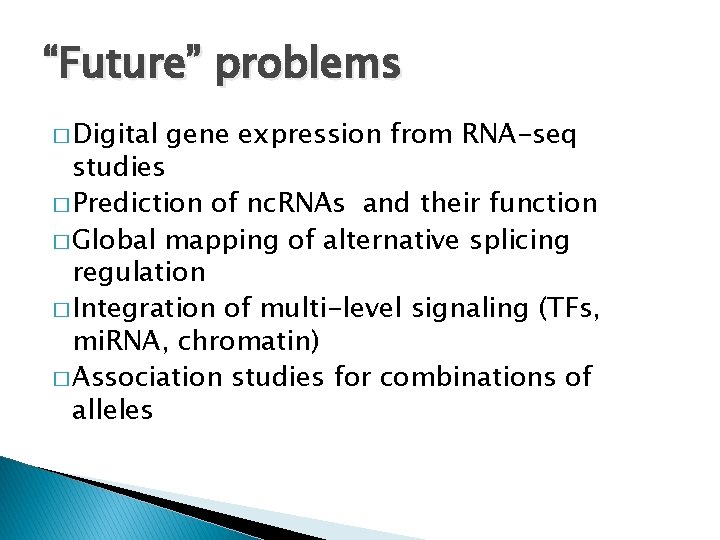
“Future” problems � Digital gene expression from RNA-seq studies � Prediction of nc. RNAs and their function � Global mapping of alternative splicing regulation � Integration of multi-level signaling (TFs, mi. RNA, chromatin) � Association studies for combinations of alleles
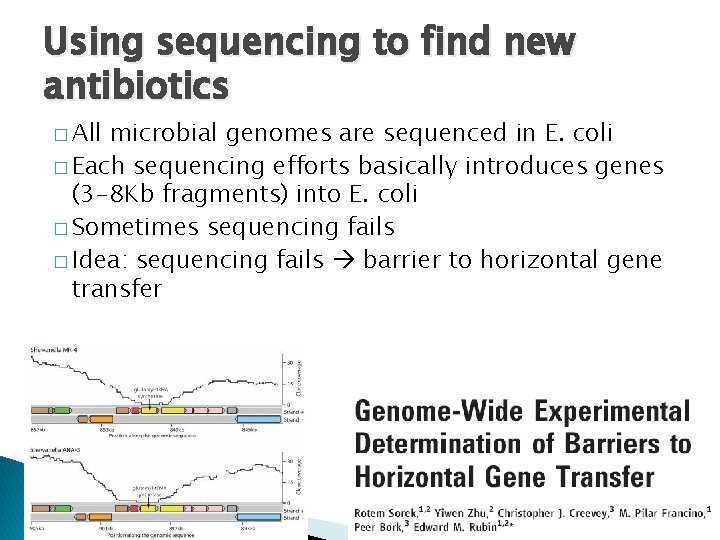
Using sequencing to find new antibiotics � All microbial genomes are sequenced in E. coli � Each sequencing efforts basically introduces genes (3 -8 Kb fragments) into E. coli � Sometimes sequencing fails � Idea: sequencing fails barrier to horizontal gene transfer
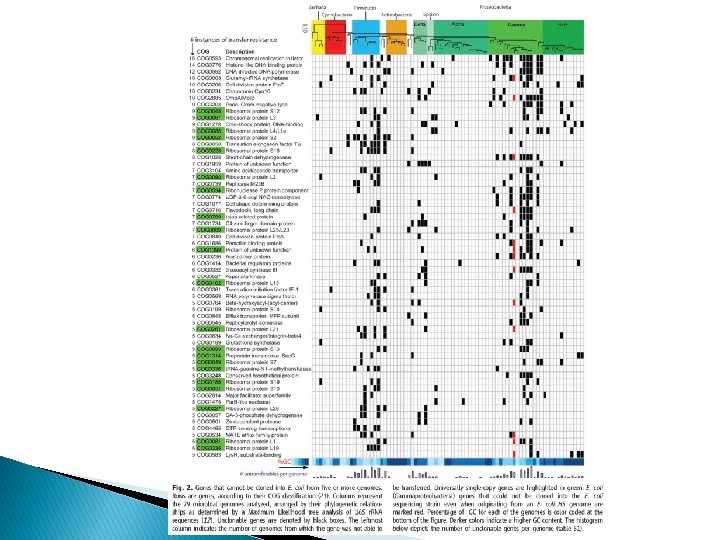
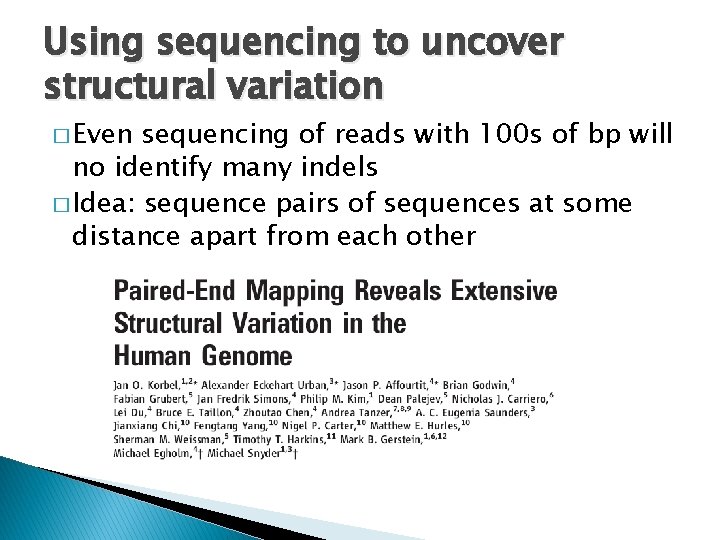
Using sequencing to uncover structural variation � Even sequencing of reads with 100 s of bp will no identify many indels � Idea: sequence pairs of sequences at some distance apart from each other
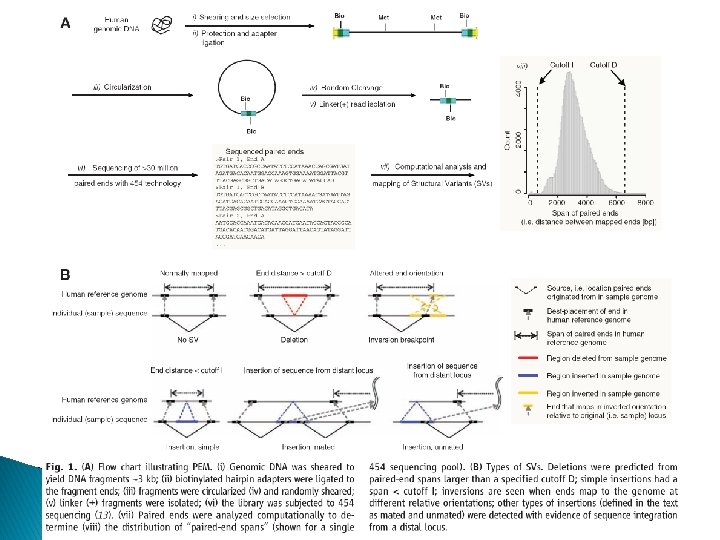
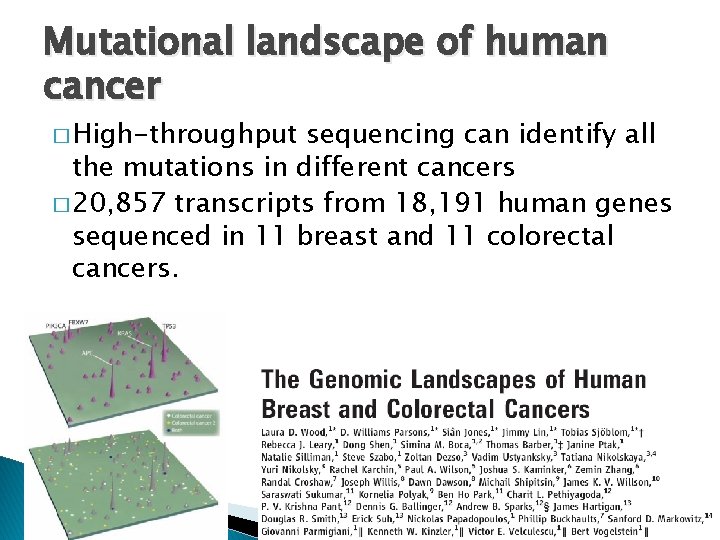
Mutational landscape of human cancer � High-throughput sequencing can identify all the mutations in different cancers � 20, 857 transcripts from 18, 191 human genes sequenced in 11 breast and 11 colorectal cancers.
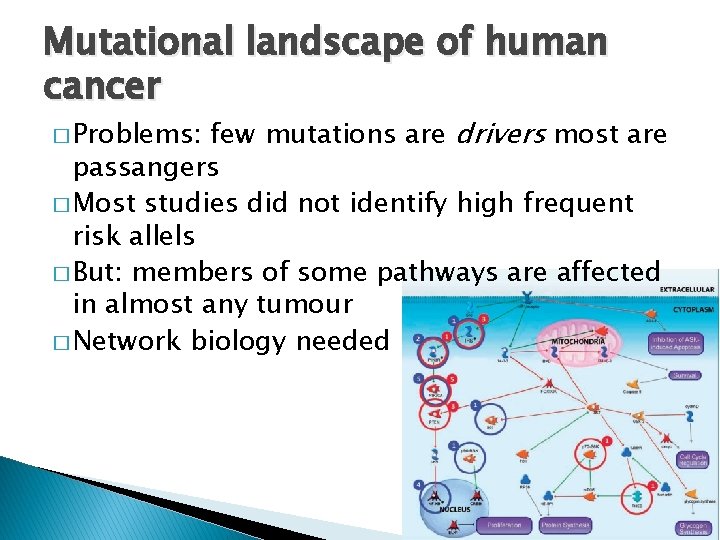
Mutational landscape of human cancer few mutations are drivers most are passangers � Most studies did not identify high frequent risk allels � But: members of some pathways are affected in almost any tumour � Network biology needed � Problems:
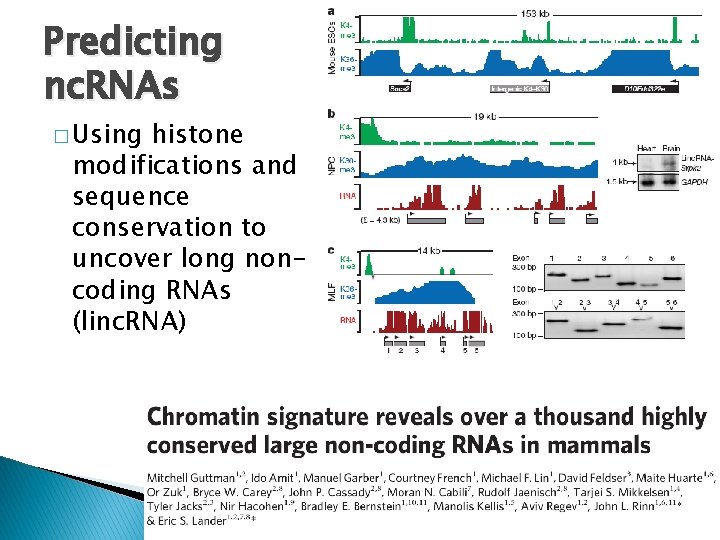
Predicting nc. RNAs � Using histone modifications and sequence conservation to uncover long noncoding RNAs (linc. RNA)
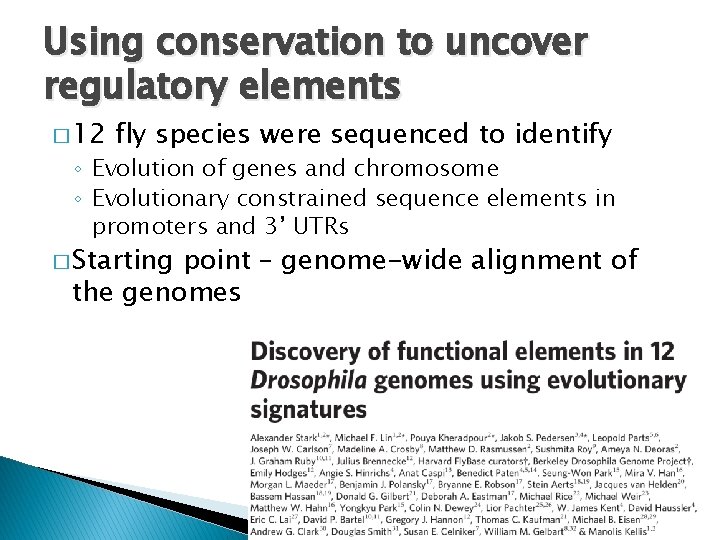
Using conservation to uncover regulatory elements � 12 fly species were sequenced to identify ◦ Evolution of genes and chromosome ◦ Evolutionary constrained sequence elements in promoters and 3’ UTRs � Starting point – genome-wide alignment of the genomes
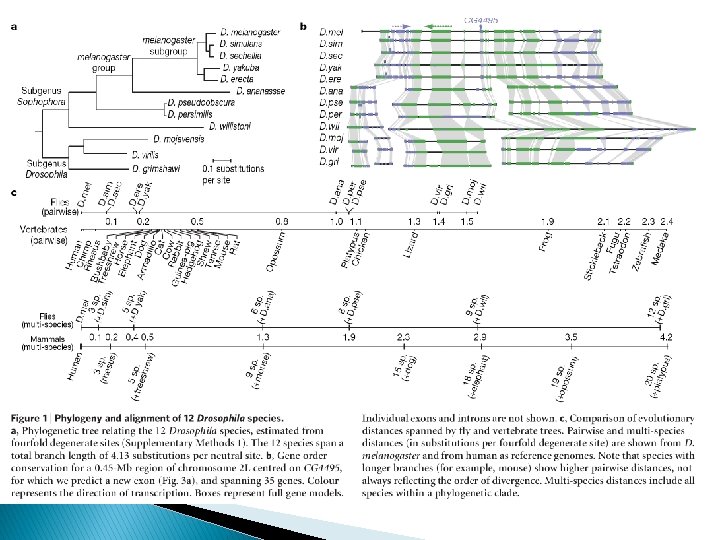
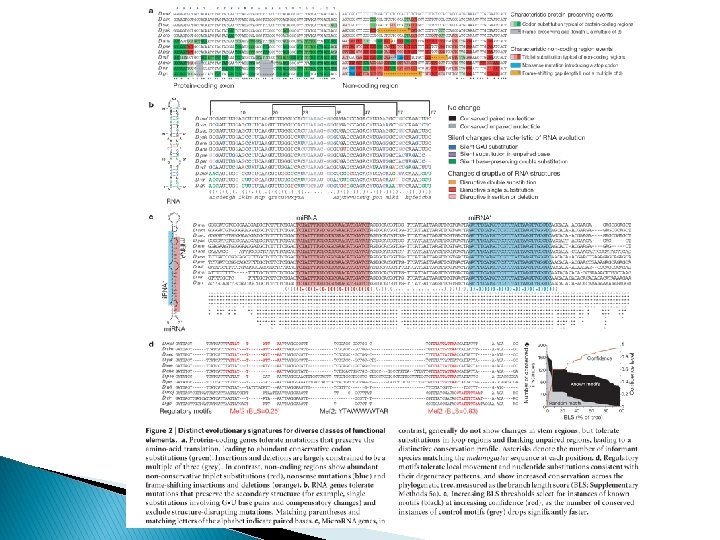
Difference between structural and functional genomics
Functional genomics
Difference between structural and functional genomics
Functional and non functional plasma enzymes
Enzymes of blood plasma
Functional and non functional
Band and loop space maintainer indications
Types of genomics
Vcf viewer
Harvest genomics
"encoded genomics" -job
A vision for the future of genomics research
Genome is
"encoded genomics"
Integrative genomics viewer
Application of genomics