Assimilation of GPS Radio Occultation refractivity with an
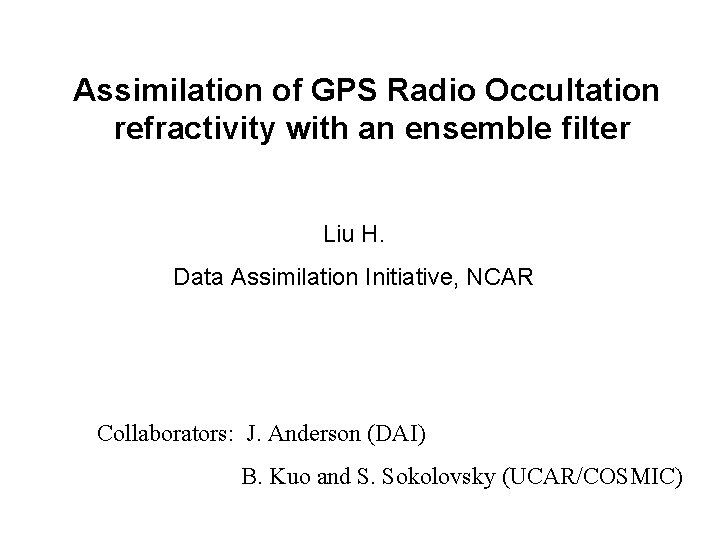
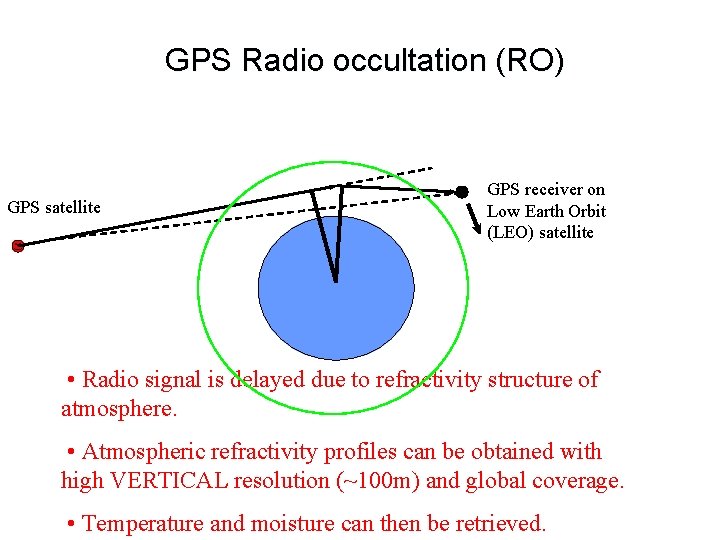
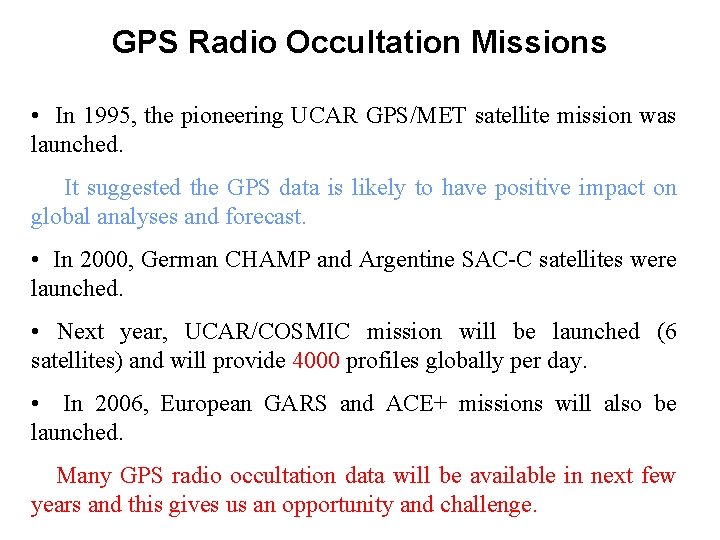
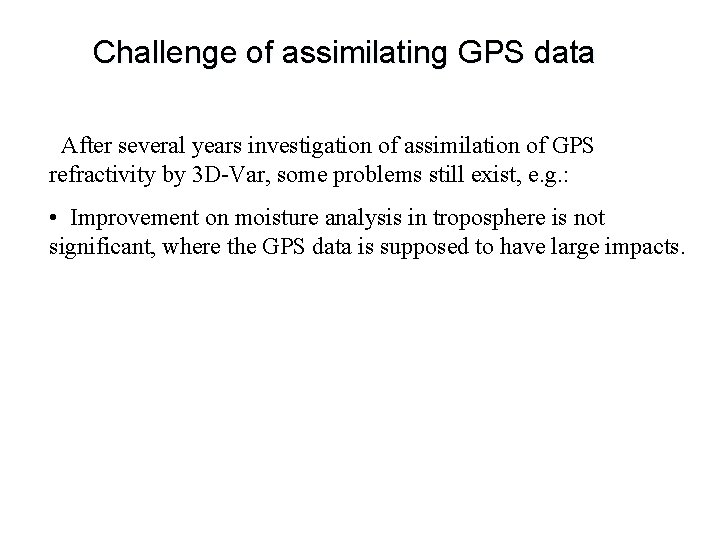
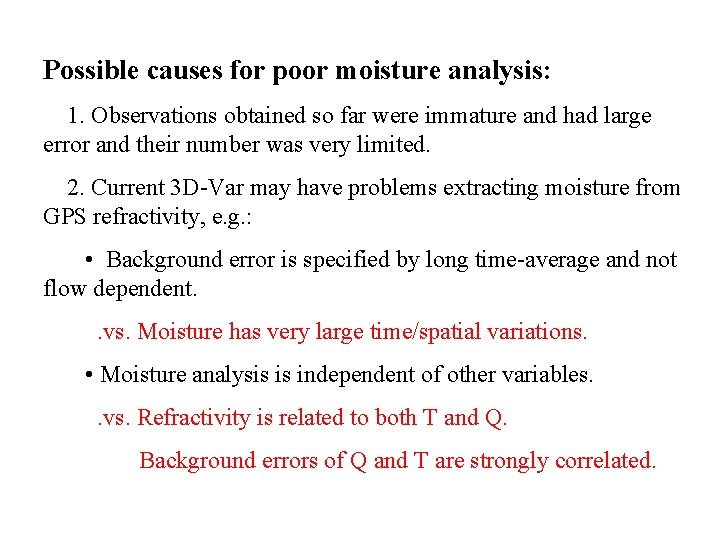
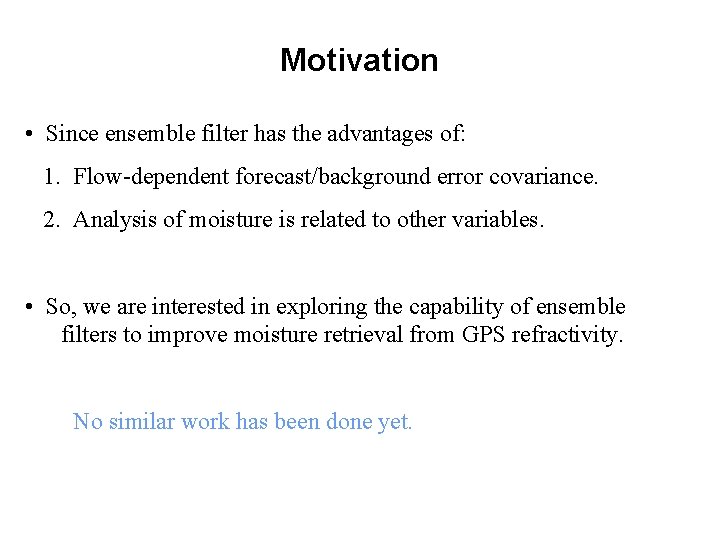
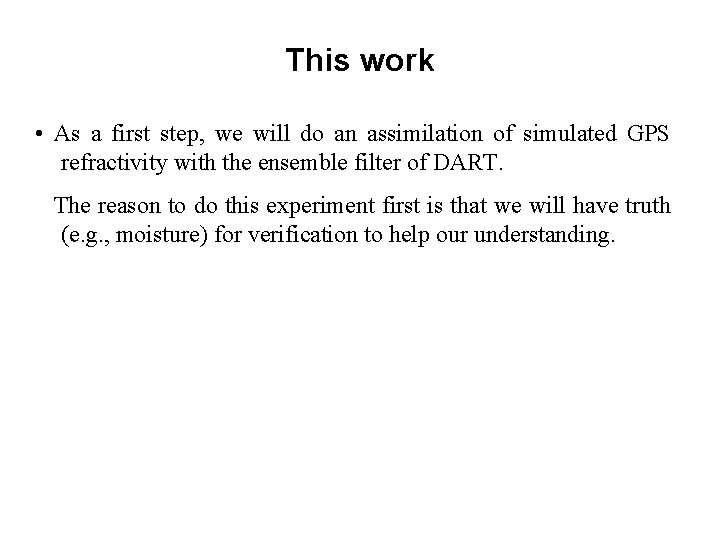
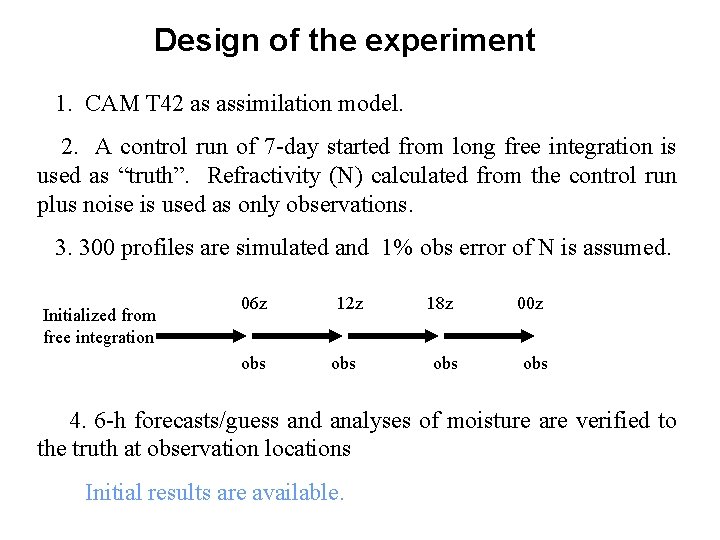
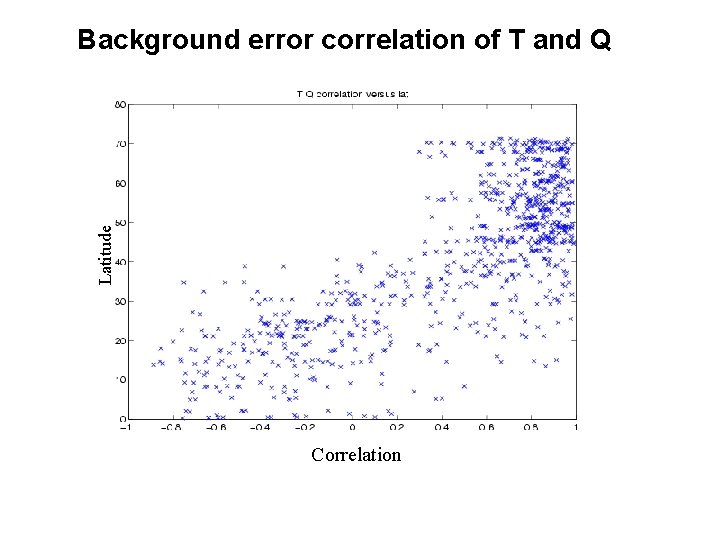
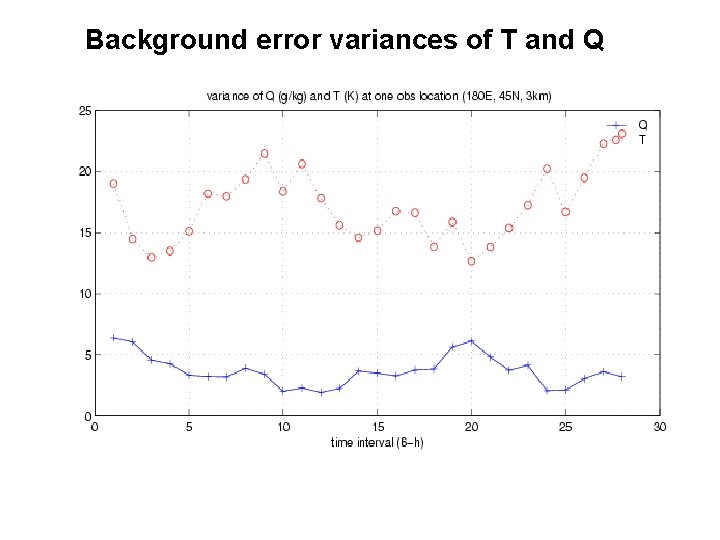
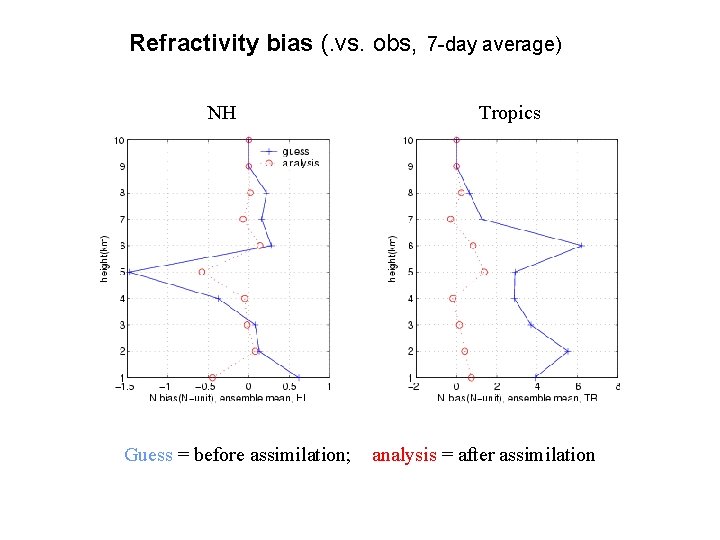
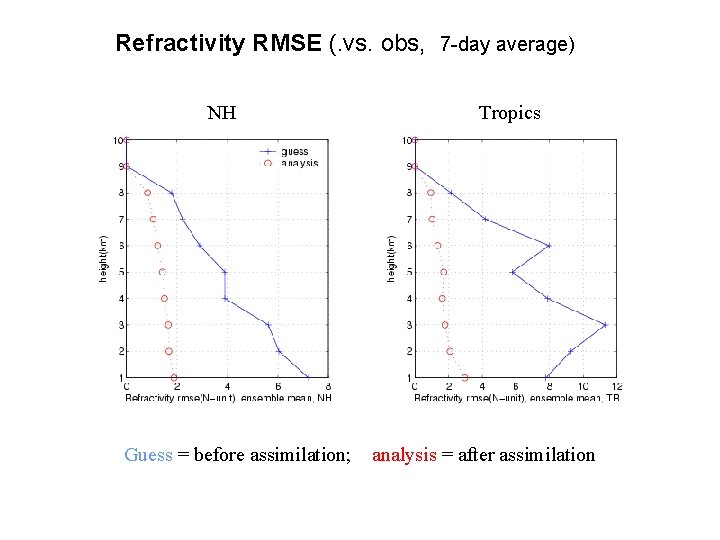
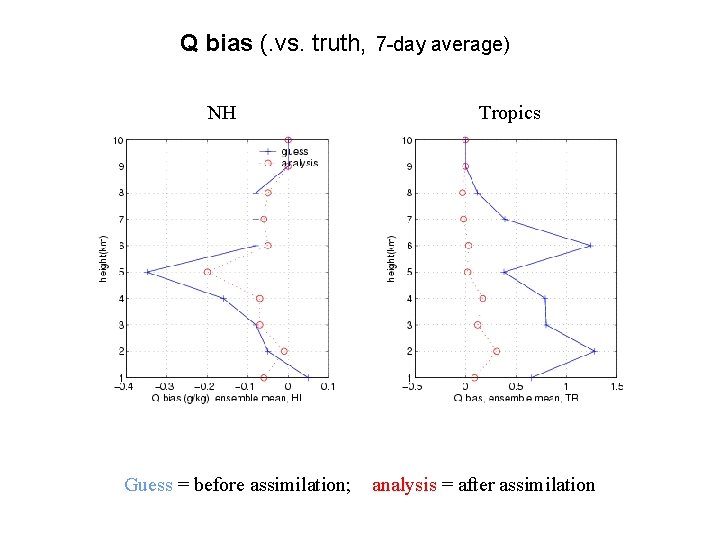
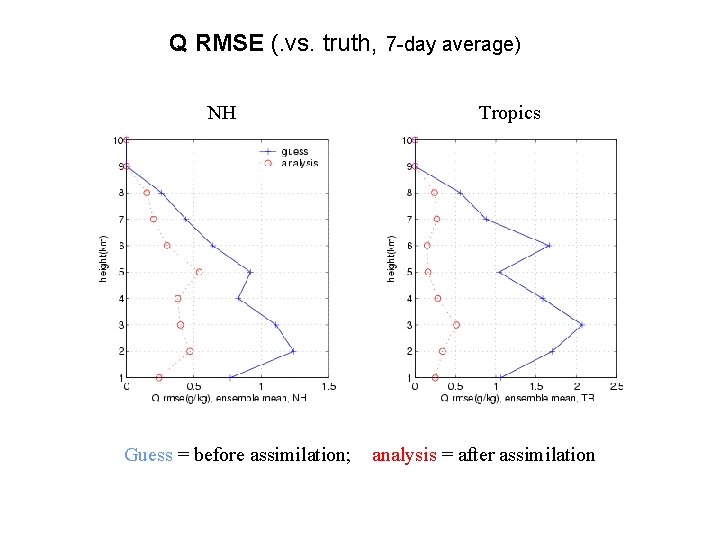
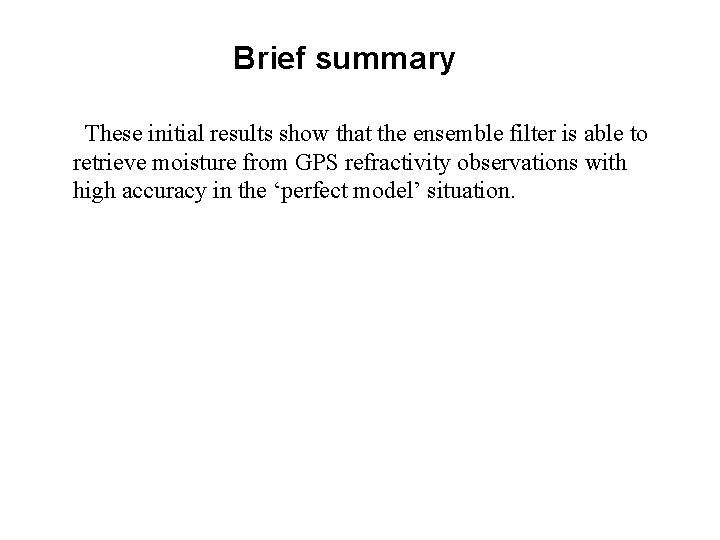
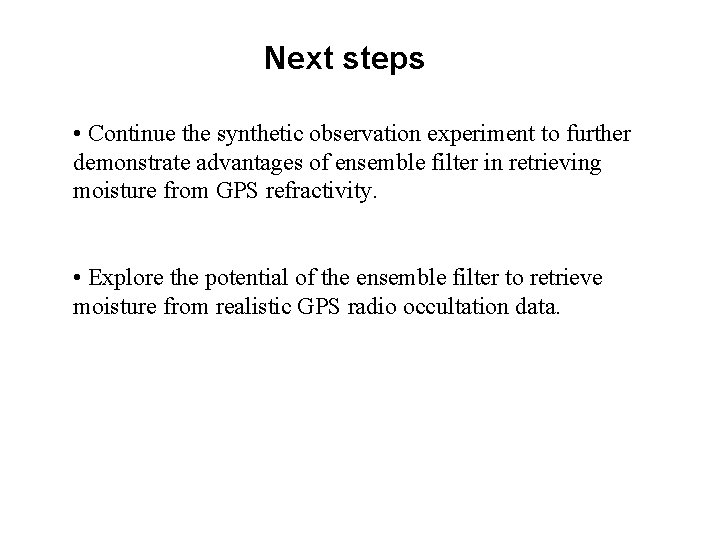
- Slides: 16
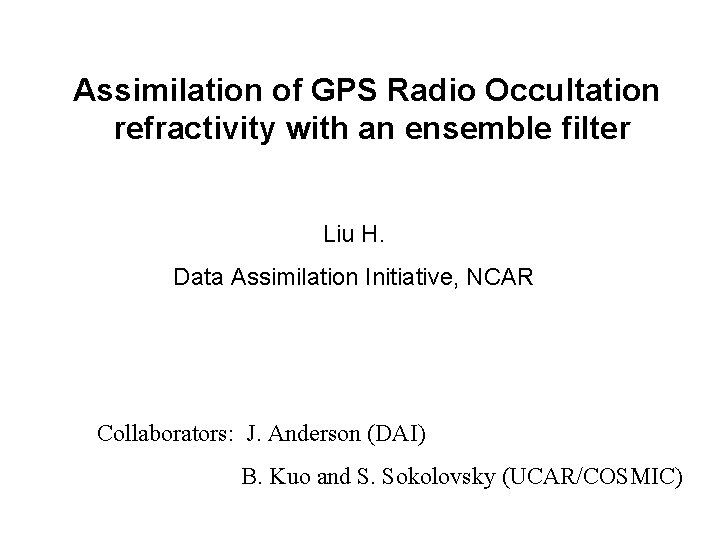
Assimilation of GPS Radio Occultation refractivity with an ensemble filter Liu H. Data Assimilation Initiative, NCAR Collaborators: J. Anderson (DAI) B. Kuo and S. Sokolovsky (UCAR/COSMIC)
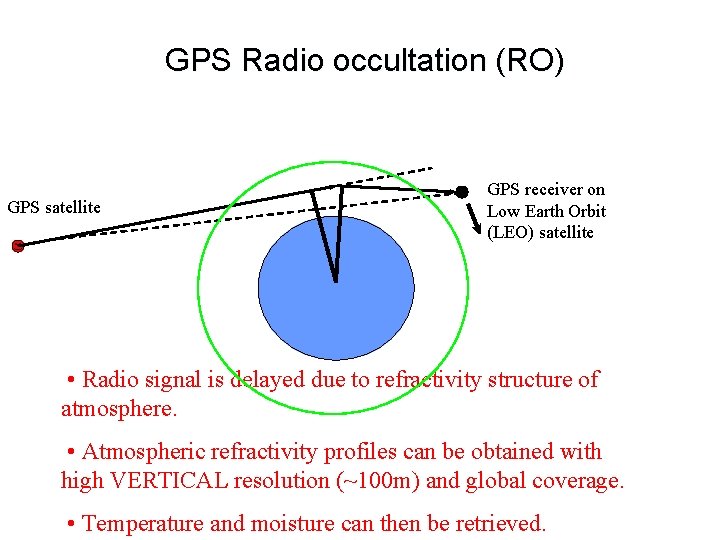
GPS Radio occultation (RO) GPS satellite GPS receiver on Low Earth Orbit (LEO) satellite • Radio signal is delayed due to refractivity structure of atmosphere. • Atmospheric refractivity profiles can be obtained with high VERTICAL resolution (~100 m) and global coverage. • Temperature and moisture can then be retrieved.
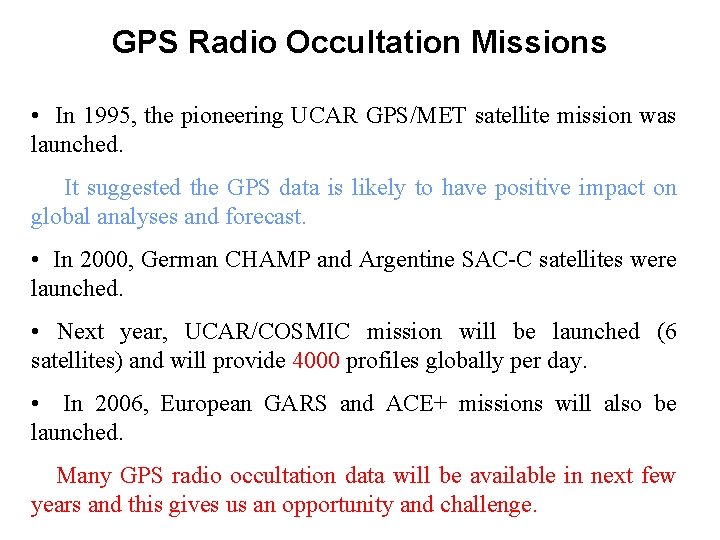
GPS Radio Occultation Missions • In 1995, the pioneering UCAR GPS/MET satellite mission was launched. It suggested the GPS data is likely to have positive impact on global analyses and forecast. • In 2000, German CHAMP and Argentine SAC-C satellites were launched. • Next year, UCAR/COSMIC mission will be launched (6 satellites) and will provide 4000 profiles globally per day. • In 2006, European GARS and ACE+ missions will also be launched. Many GPS radio occultation data will be available in next few years and this gives us an opportunity and challenge.
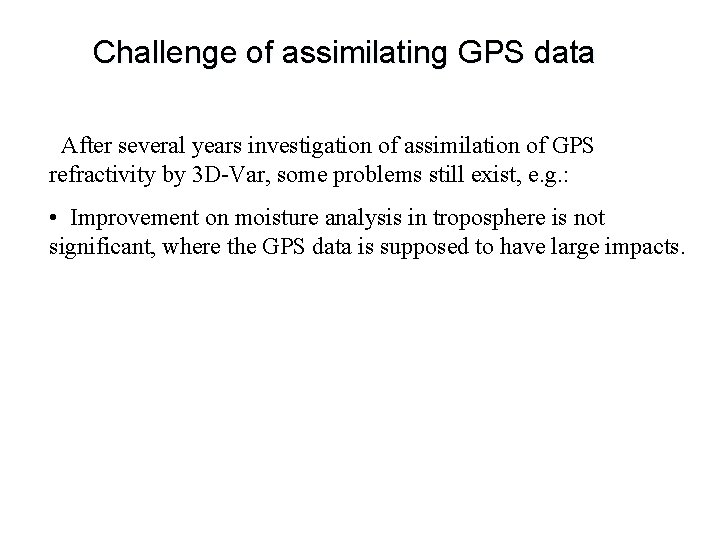
Challenge of assimilating GPS data After several years investigation of assimilation of GPS refractivity by 3 D-Var, some problems still exist, e. g. : • Improvement on moisture analysis in troposphere is not significant, where the GPS data is supposed to have large impacts.
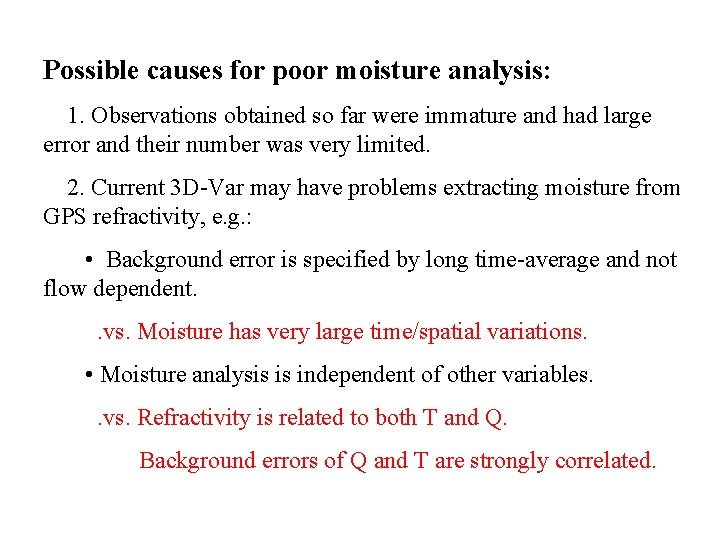
Possible causes for poor moisture analysis: 1. Observations obtained so far were immature and had large error and their number was very limited. 2. Current 3 D-Var may have problems extracting moisture from GPS refractivity, e. g. : • Background error is specified by long time-average and not flow dependent. . vs. Moisture has very large time/spatial variations. • Moisture analysis is independent of other variables. . vs. Refractivity is related to both T and Q. Background errors of Q and T are strongly correlated.
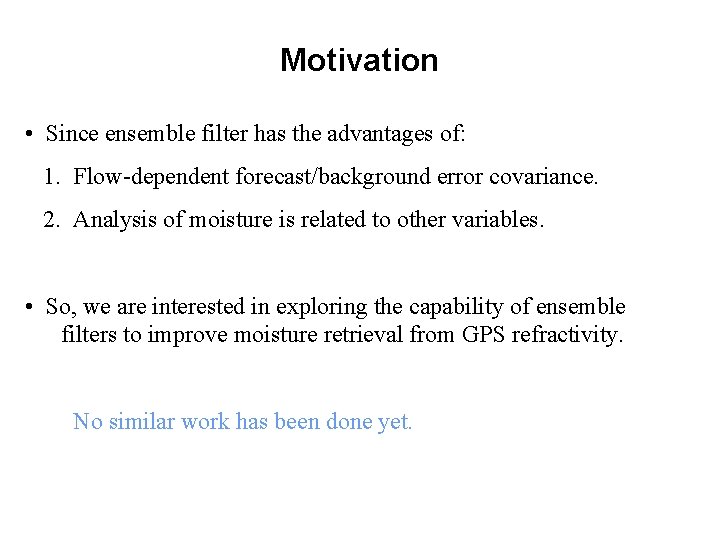
Motivation • Since ensemble filter has the advantages of: 1. Flow-dependent forecast/background error covariance. 2. Analysis of moisture is related to other variables. • So, we are interested in exploring the capability of ensemble filters to improve moisture retrieval from GPS refractivity. No similar work has been done yet.
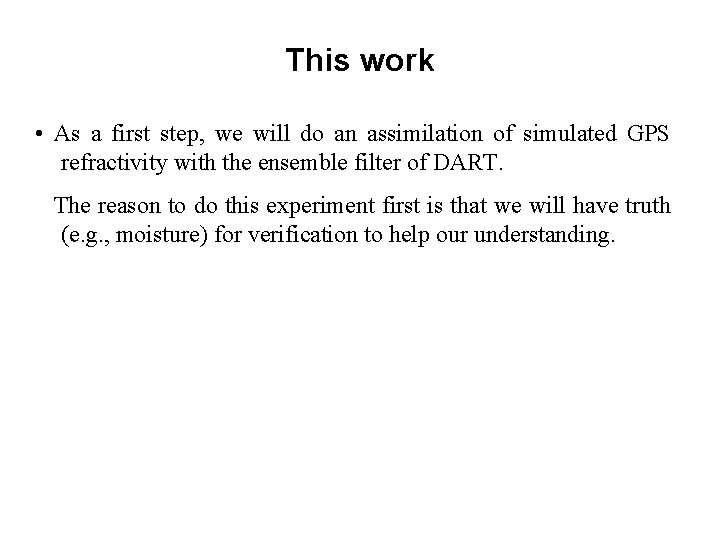
This work • As a first step, we will do an assimilation of simulated GPS refractivity with the ensemble filter of DART. The reason to do this experiment first is that we will have truth (e. g. , moisture) for verification to help our understanding.
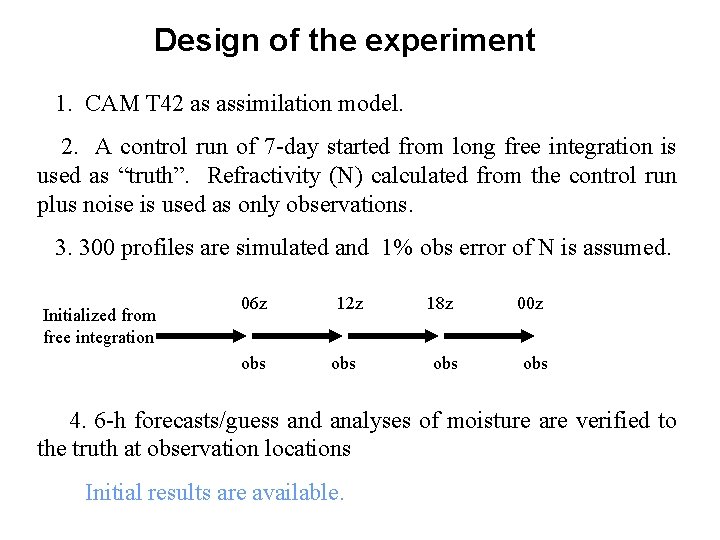
Design of the experiment 1. CAM T 42 as assimilation model. 2. A control run of 7 -day started from long free integration is used as “truth”. Refractivity (N) calculated from the control run plus noise is used as only observations. 3. 300 profiles are simulated and 1% obs error of N is assumed. Initialized from free integration 06 z obs 12 z obs 18 z 00 z obs 4. 6 -h forecasts/guess and analyses of moisture are verified to the truth at observation locations Initial results are available.
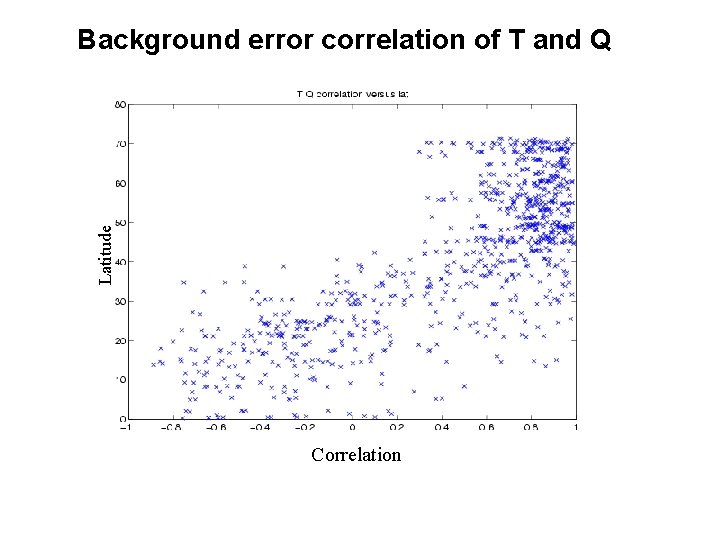
Latitude Background error correlation of T and Q Correlation
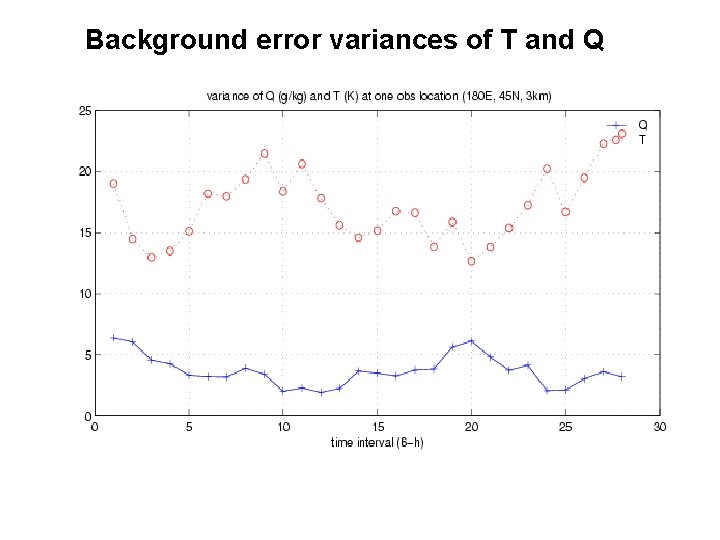
Background error variances of T and Q
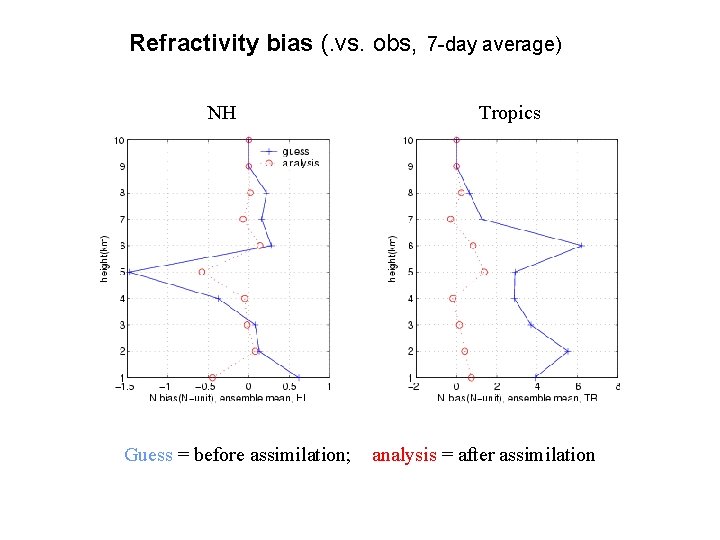
Refractivity bias (. vs. obs, 7 -day average) NH Guess = before assimilation; Tropics analysis = after assimilation
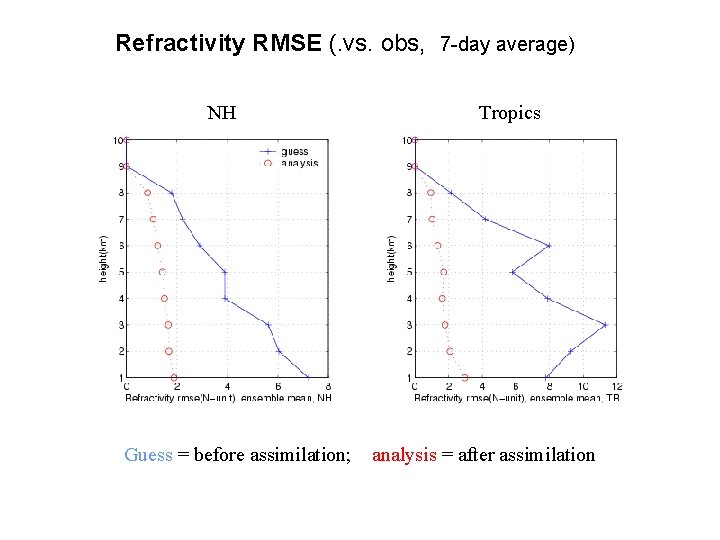
Refractivity RMSE (. vs. obs, 7 -day average) NH Guess = before assimilation; Tropics analysis = after assimilation
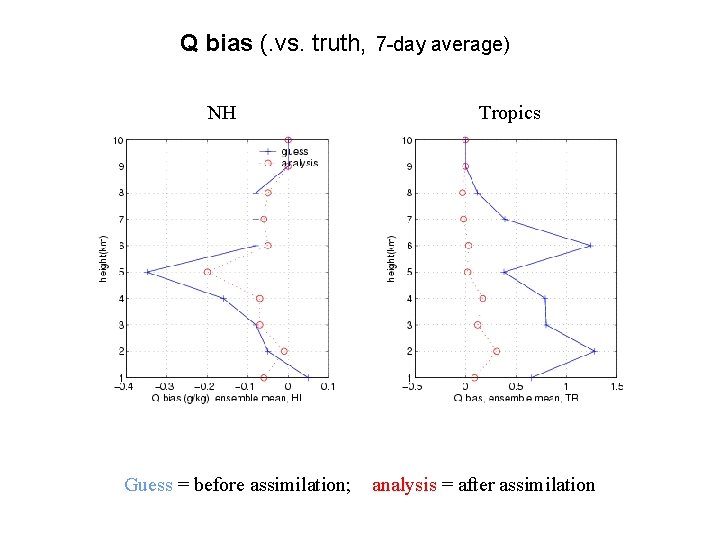
Q bias (. vs. truth, 7 -day average) NH Guess = before assimilation; Tropics analysis = after assimilation
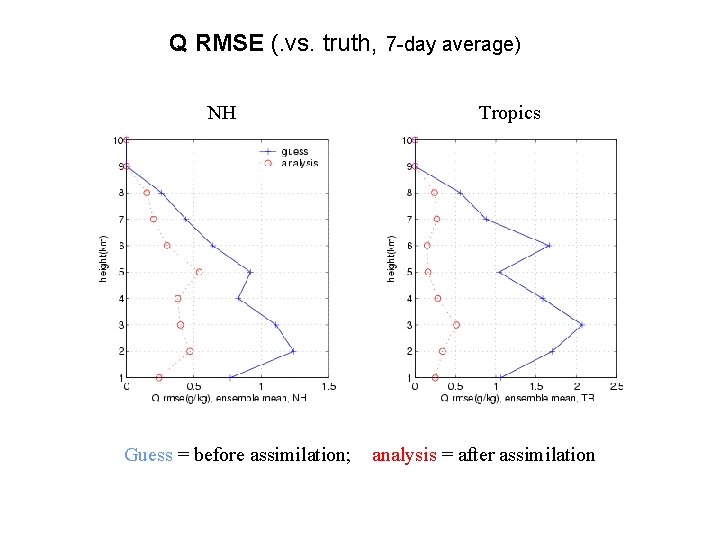
Q RMSE (. vs. truth, 7 -day average) NH Guess = before assimilation; Tropics analysis = after assimilation
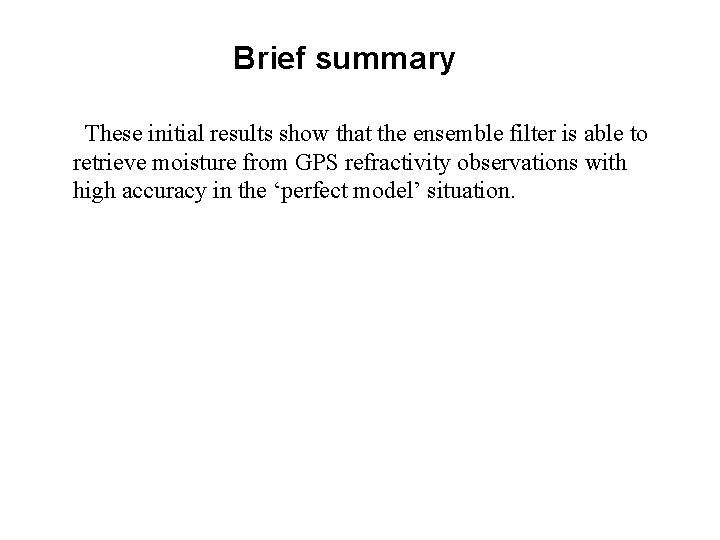
Brief summary These initial results show that the ensemble filter is able to retrieve moisture from GPS refractivity observations with high accuracy in the ‘perfect model’ situation.
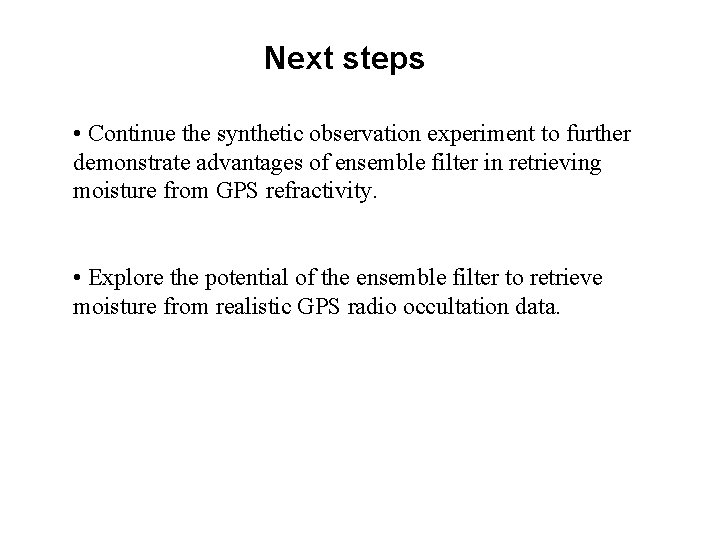
Next steps • Continue the synthetic observation experiment to further demonstrate advantages of ensemble filter in retrieving moisture from GPS refractivity. • Explore the potential of the ensemble filter to retrieve moisture from realistic GPS radio occultation data.
Gps gps gps
Modulo positioning
Double occultation
Trunking vs conventional radio system
What is assimilation
Data assimilation
Dissimilation rules
Data assimilation tutorial
Which type of nutrition
Nitrifying bacteria convert _____ to _____.
Phonetic transcription
ünsüz benzeşmesi
Assimilation in science
Phonological processes
Assimilation westward expansion
Weak forms examples
Regressive assimilation