Agent Gatekeeper Drug Dealer How Content Creators Craft
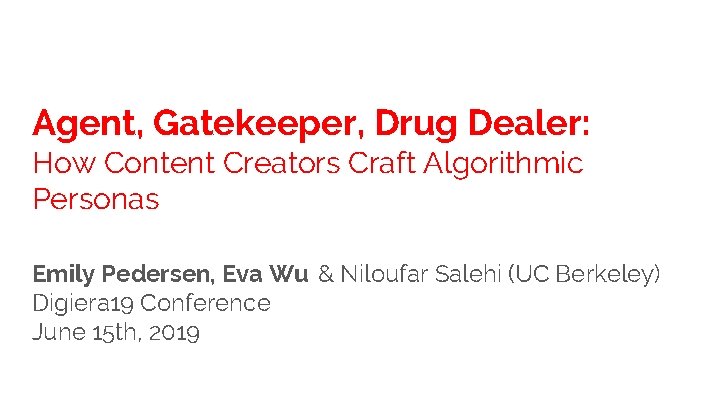
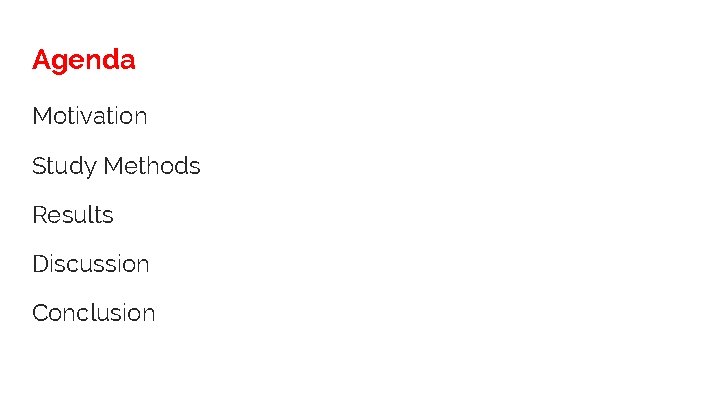
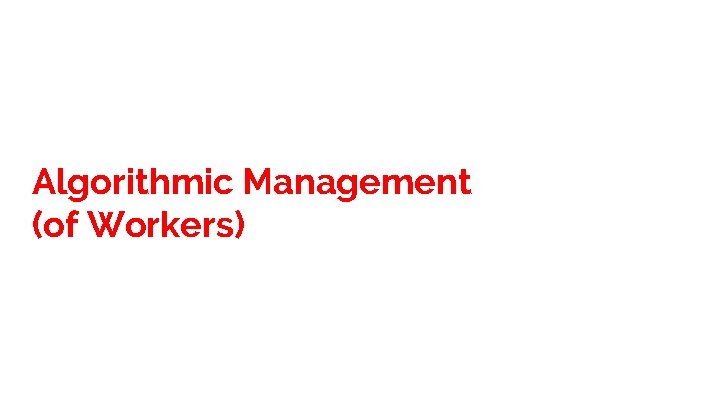
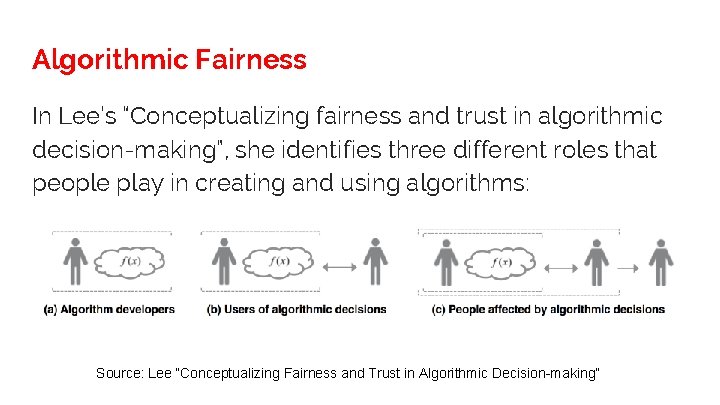
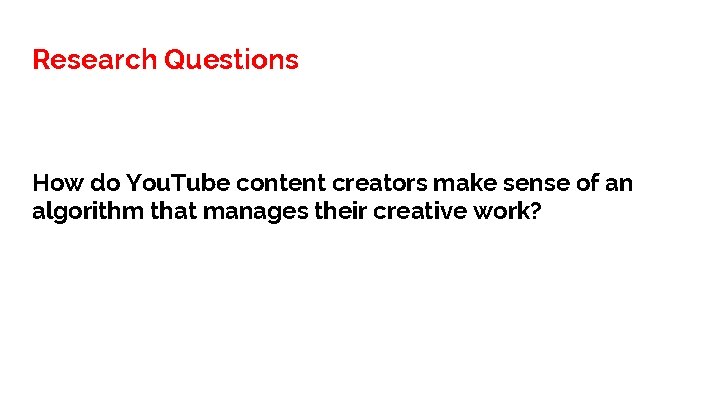
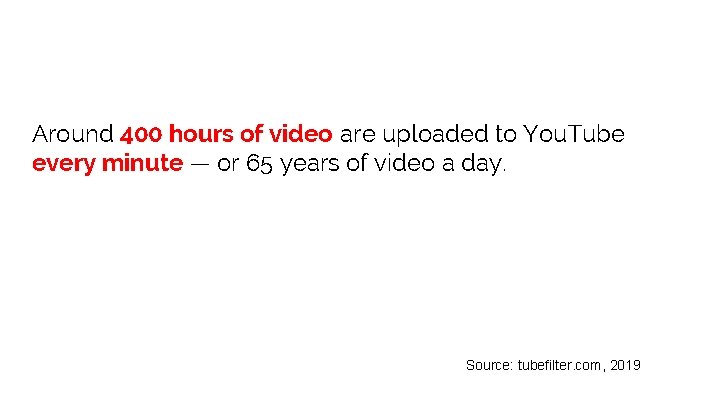
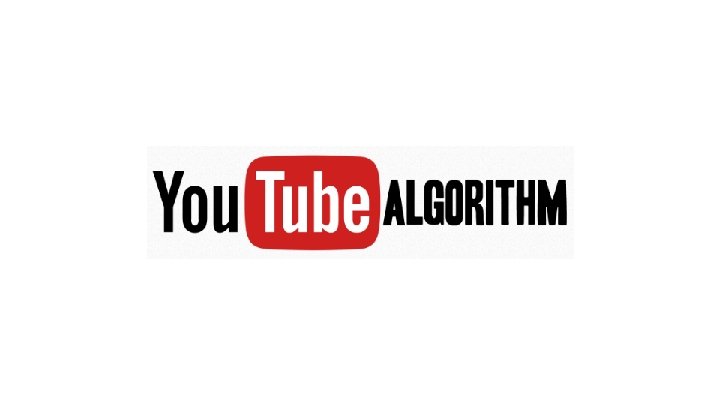
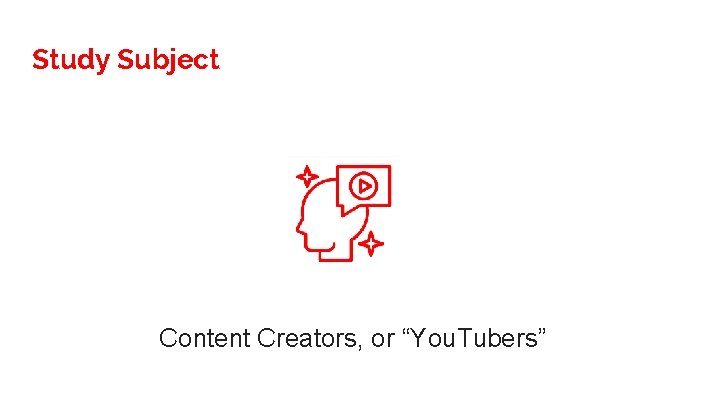
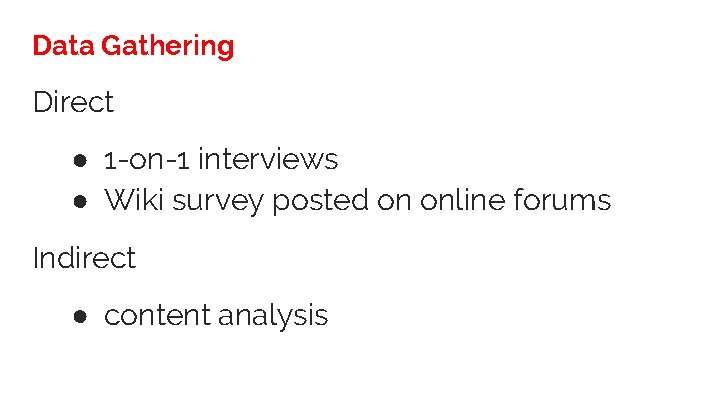
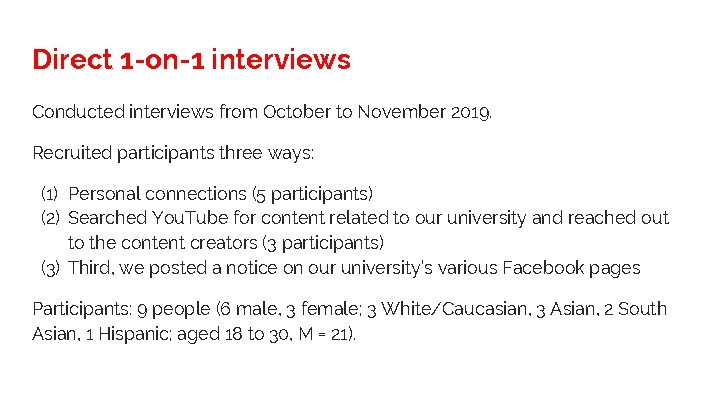
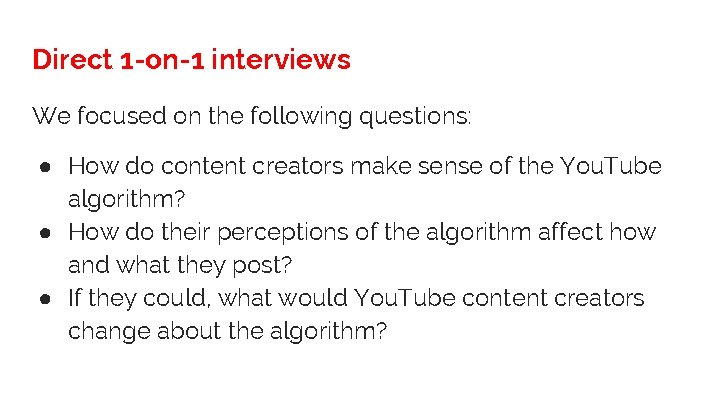
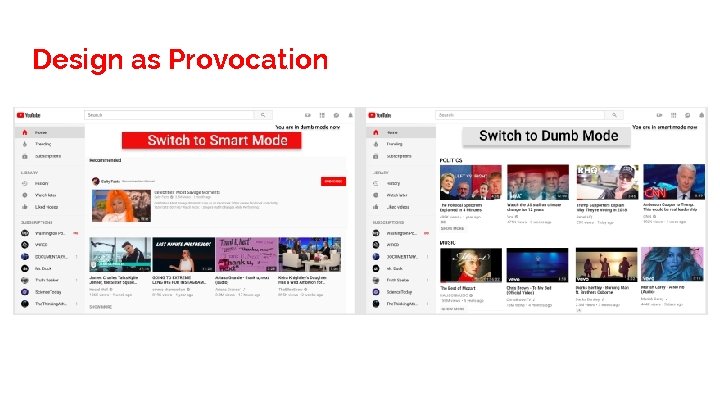
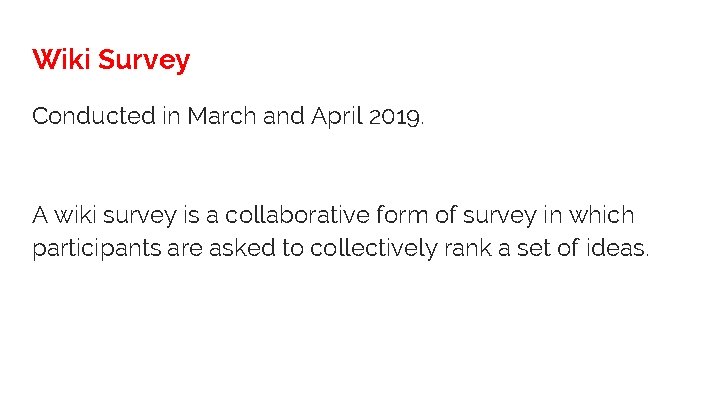
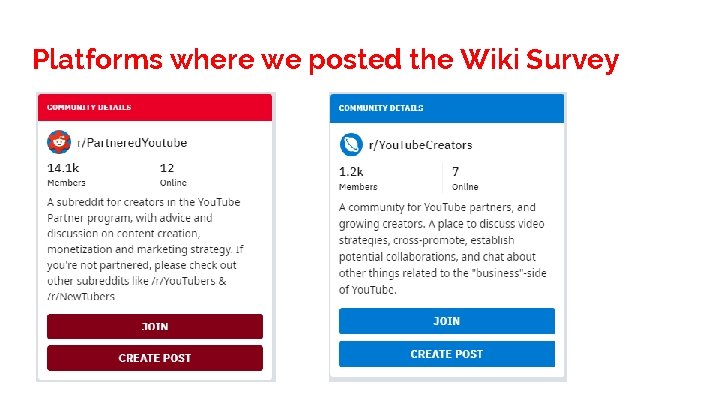
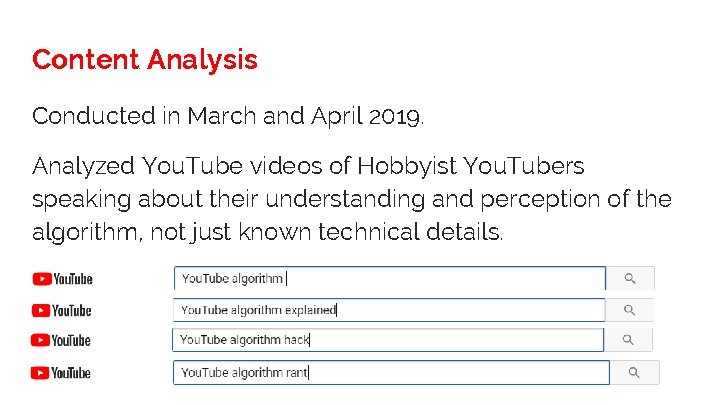
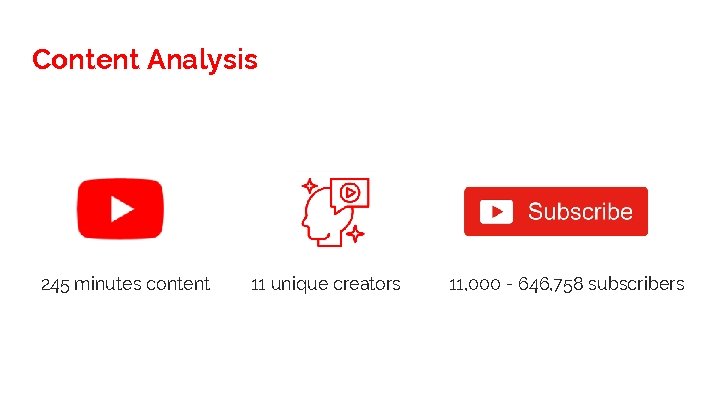
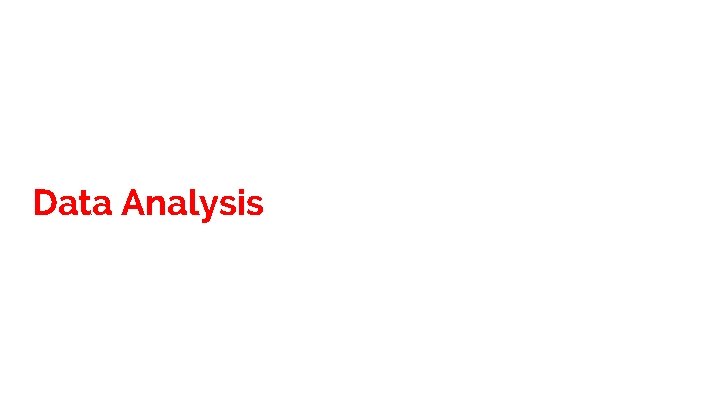
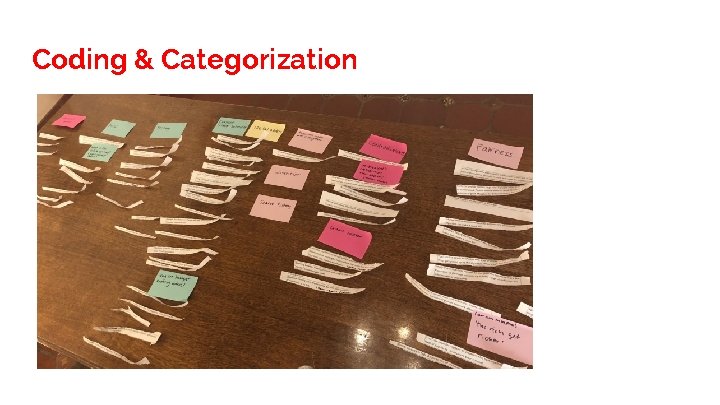
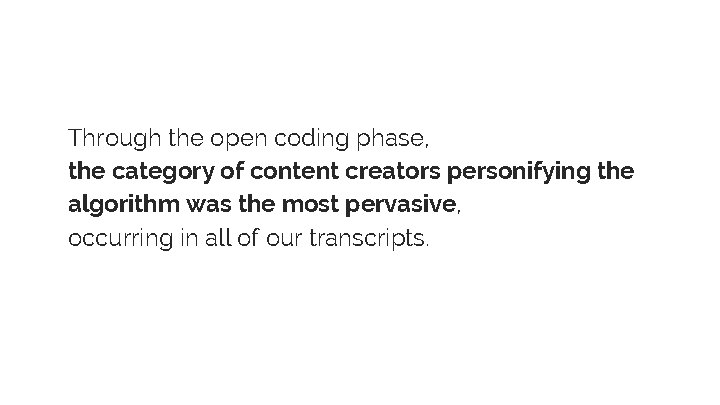
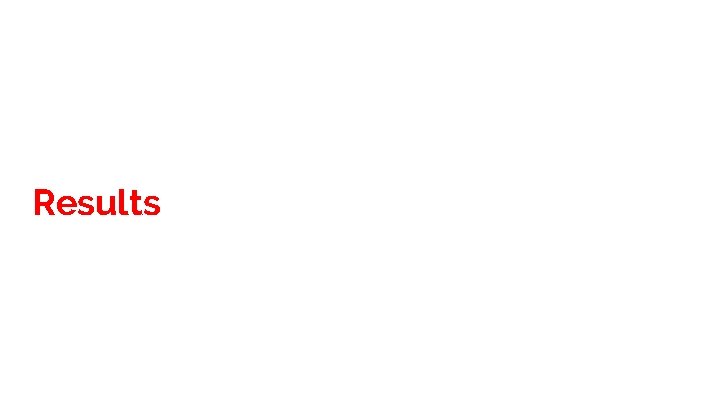
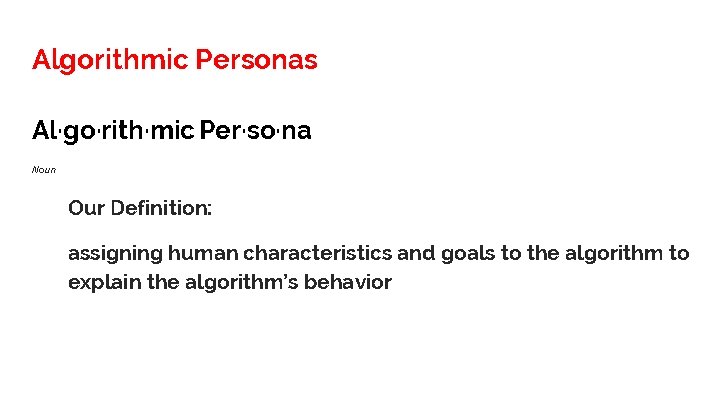
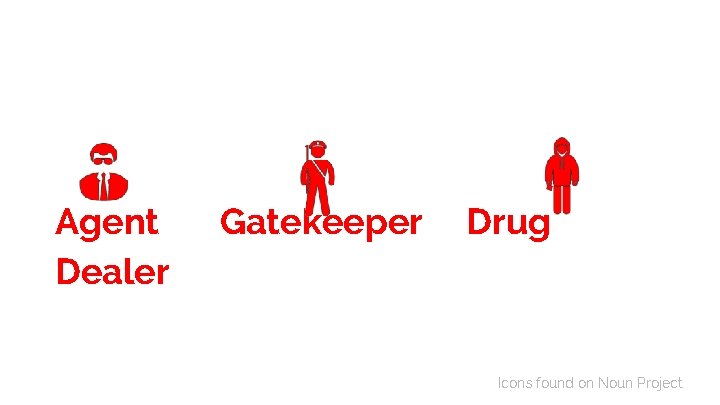
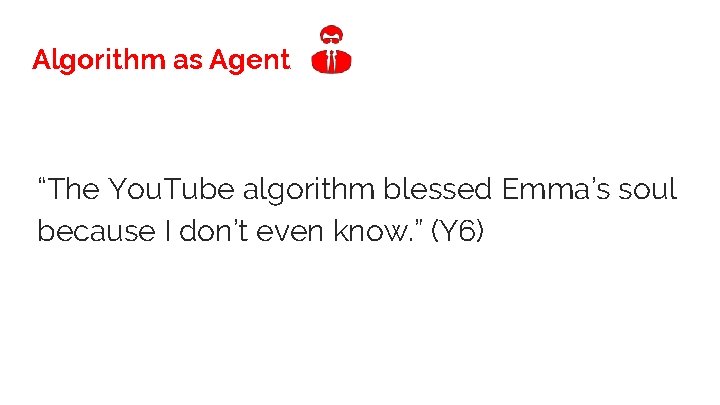
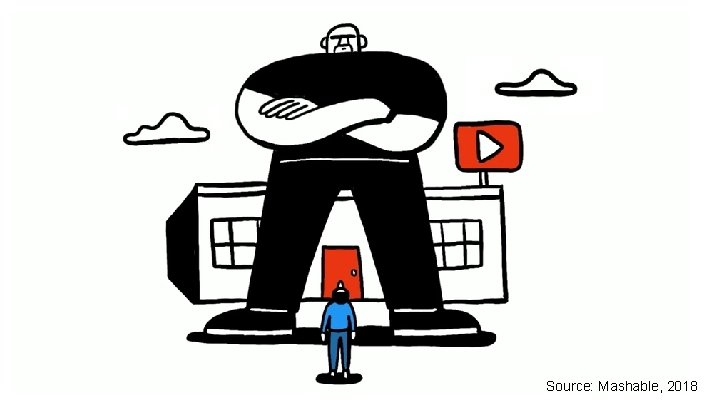
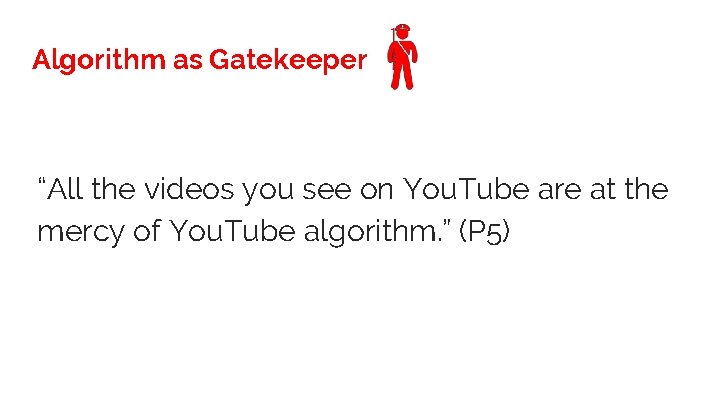
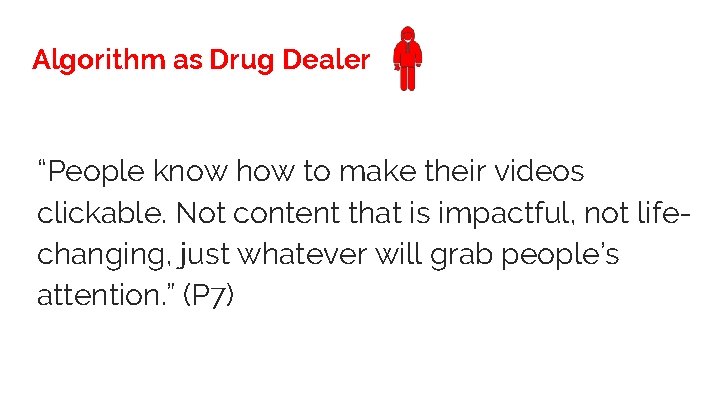
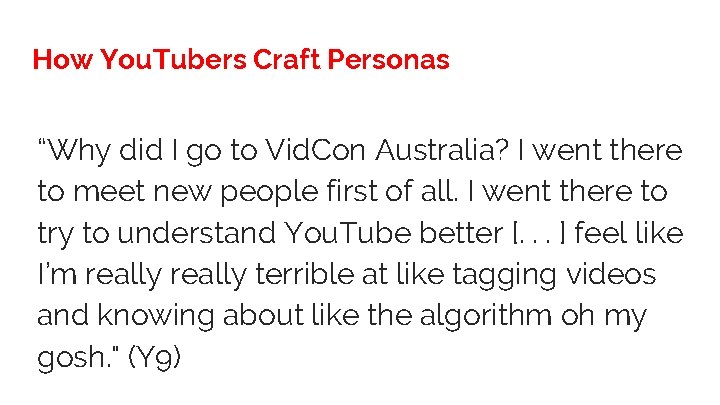
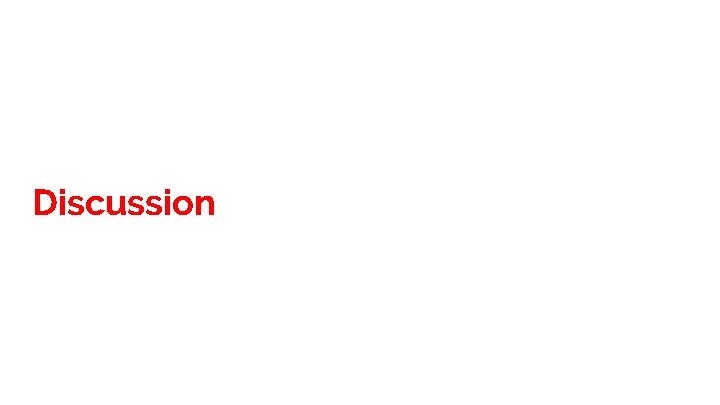
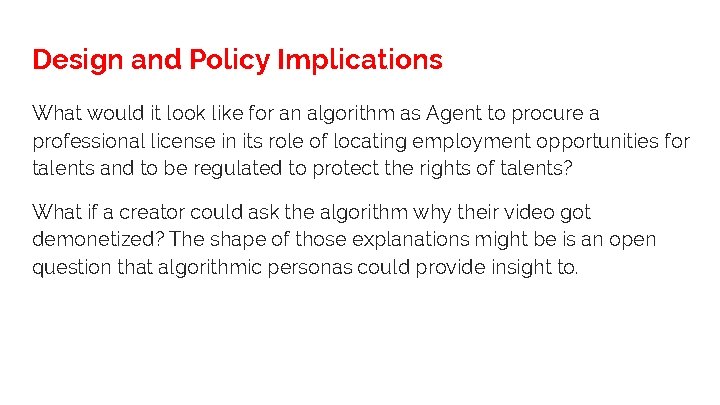
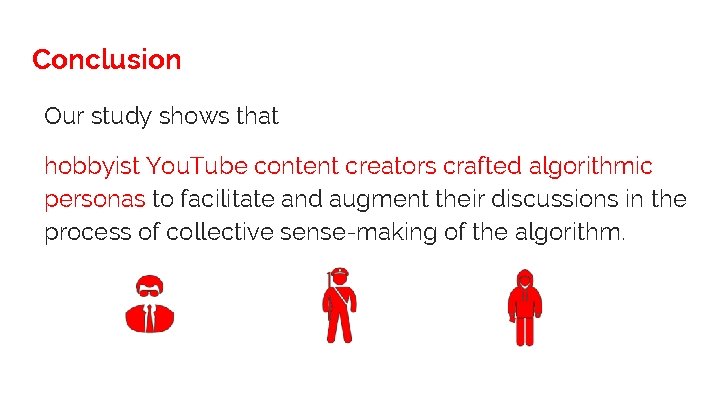
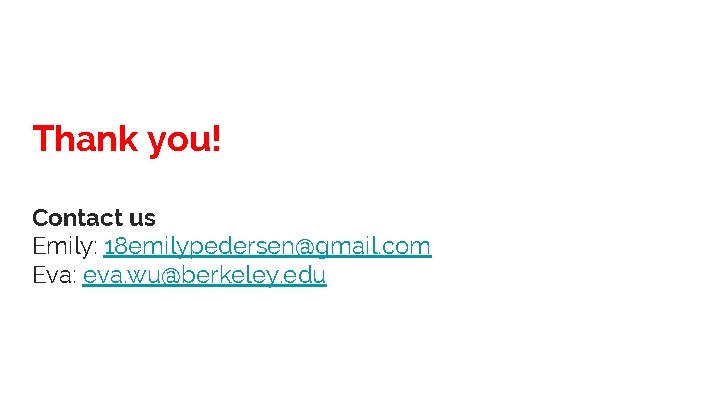
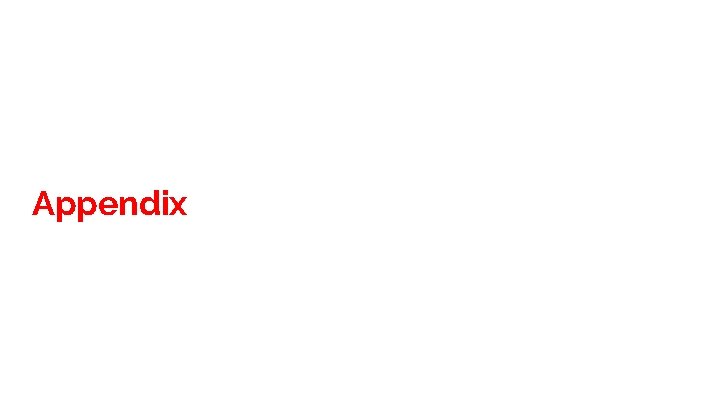
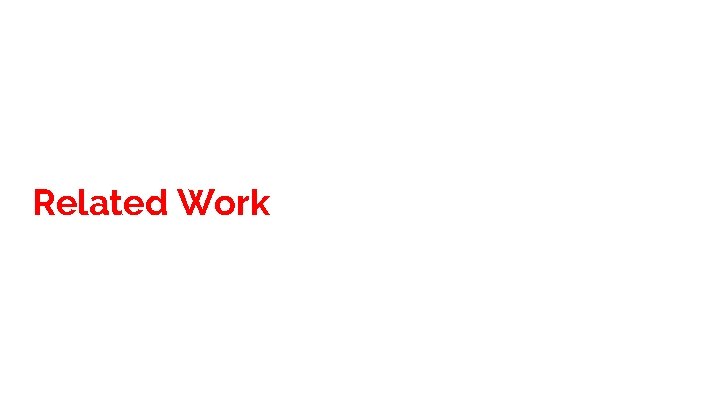
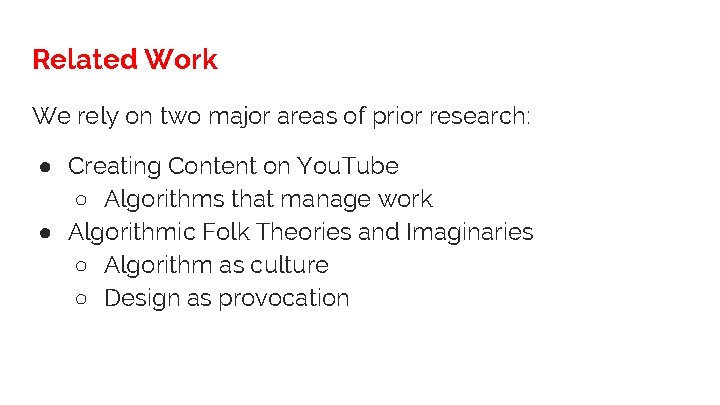
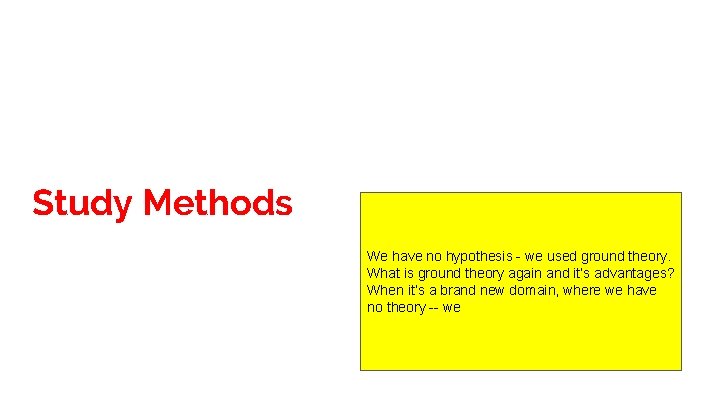
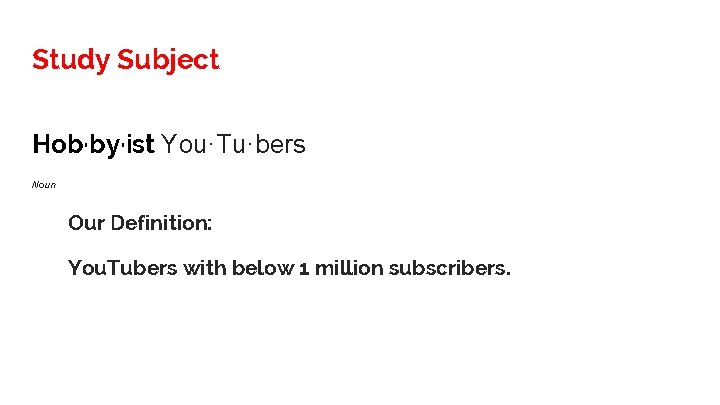
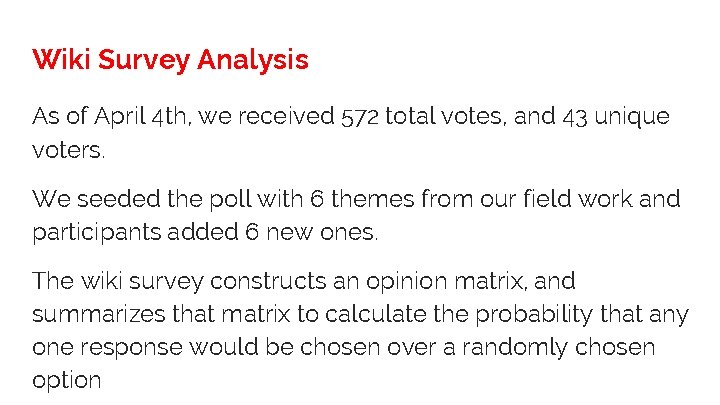
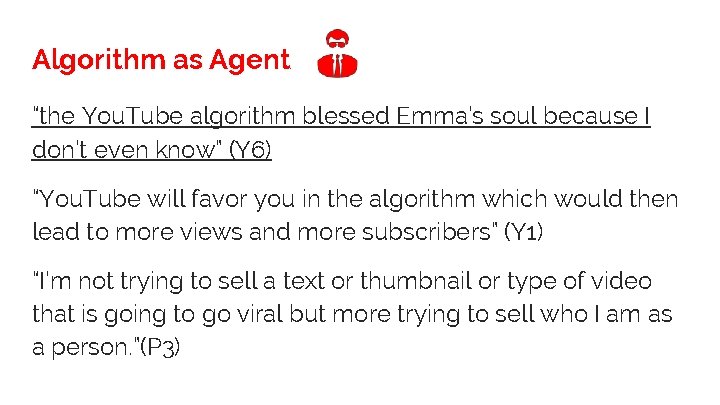
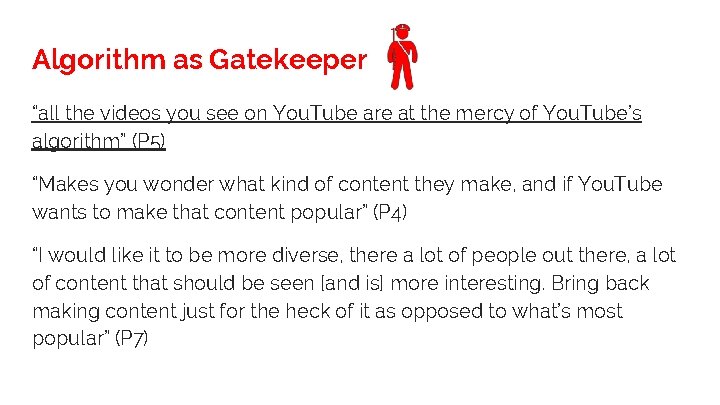
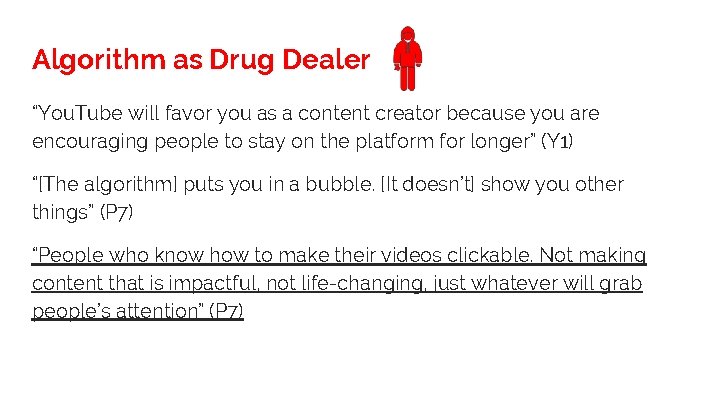
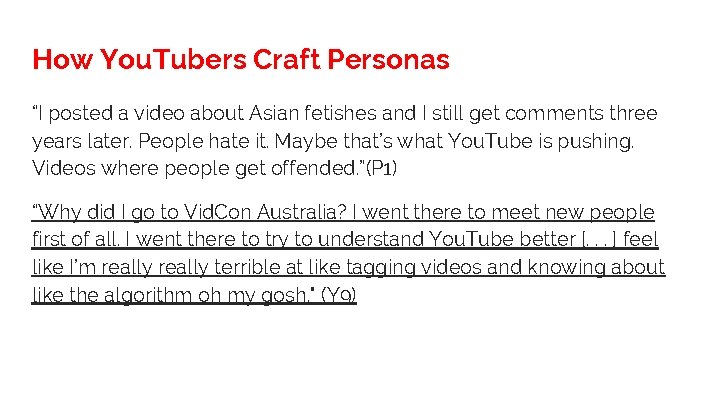
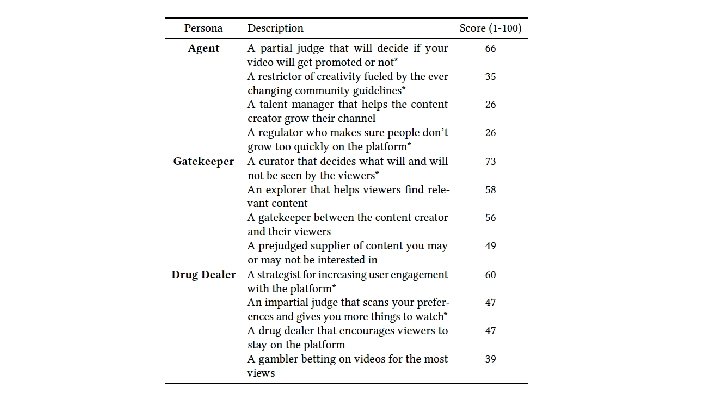
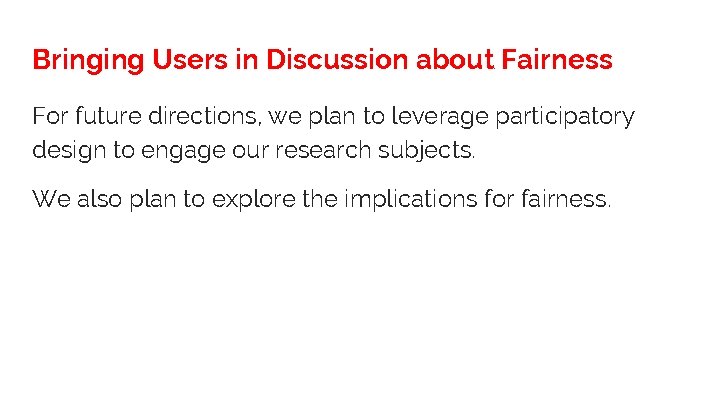
- Slides: 43
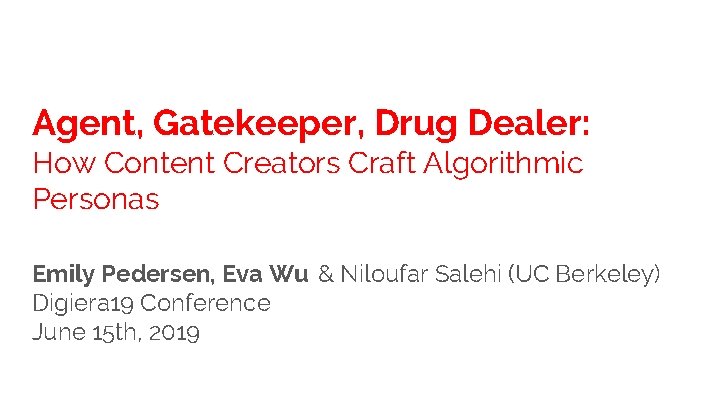
Agent, Gatekeeper, Drug Dealer: How Content Creators Craft Algorithmic Personas Emily Pedersen, Eva Wu & Niloufar Salehi (UC Berkeley) Digiera 19 Conference June 15 th, 2019
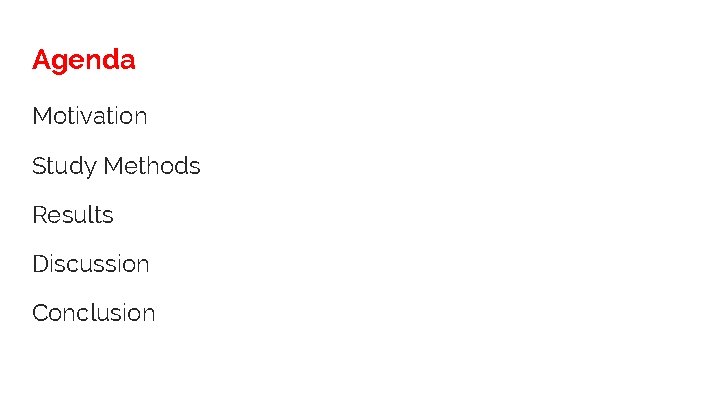
Agenda Motivation Study Methods Results Discussion Conclusion
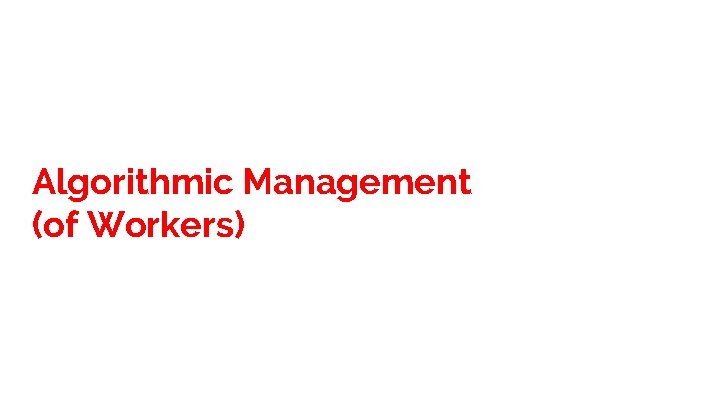
Algorithmic Management (of Workers)
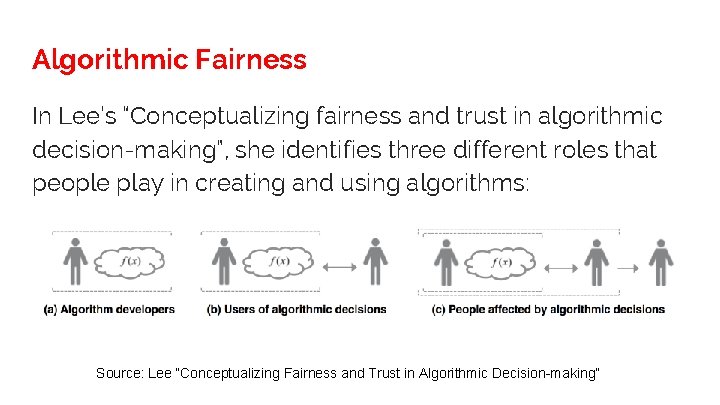
Algorithmic Fairness In Lee’s “Conceptualizing fairness and trust in algorithmic decision-making”, she identifies three different roles that people play in creating and using algorithms: 1. Algorithm Developers 2. Consumers of Algorithmic Decisions 3. People affected by Algorithmic Decisions Source: Lee “Conceptualizing Fairness and Trust in Algorithmic Decision-making”
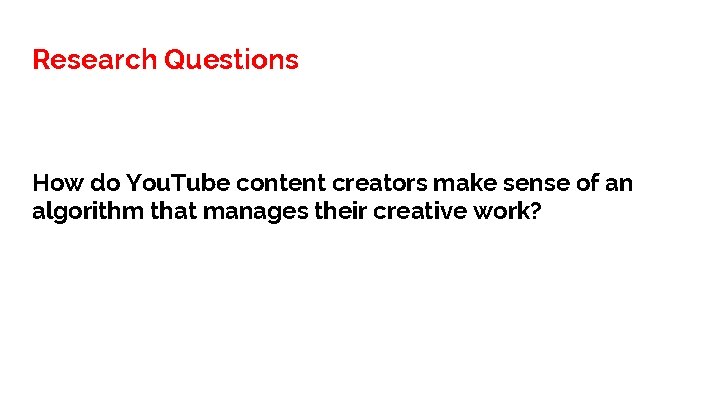
Research Questions How do You. Tube content creators make sense of an algorithm that manages their creative work?
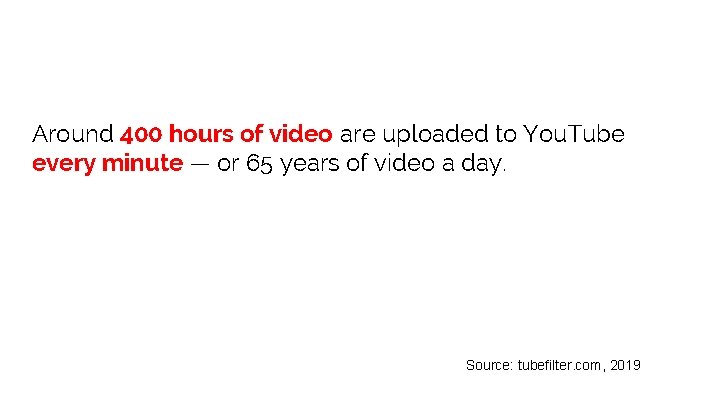
Around 400 hours of video are uploaded to You. Tube every minute — or 65 years of video a day. Source: tubefilter. com, 2019
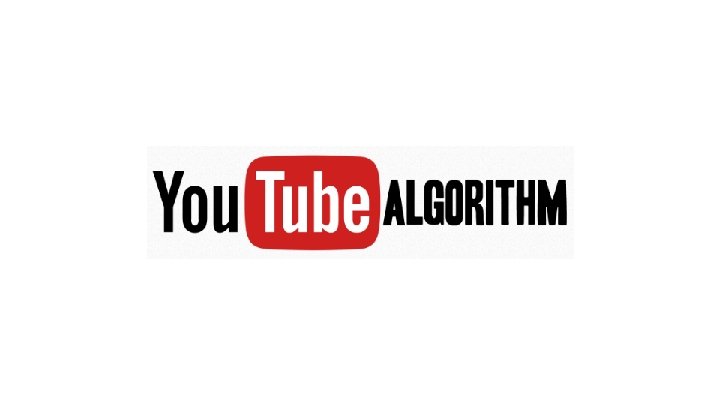
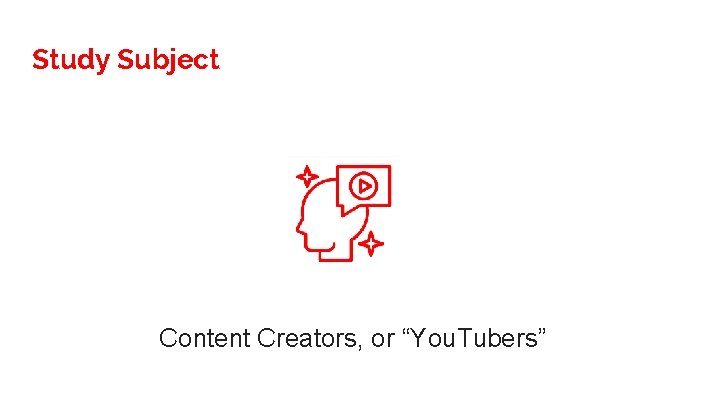
Study Subject Content Creators, or “You. Tubers”
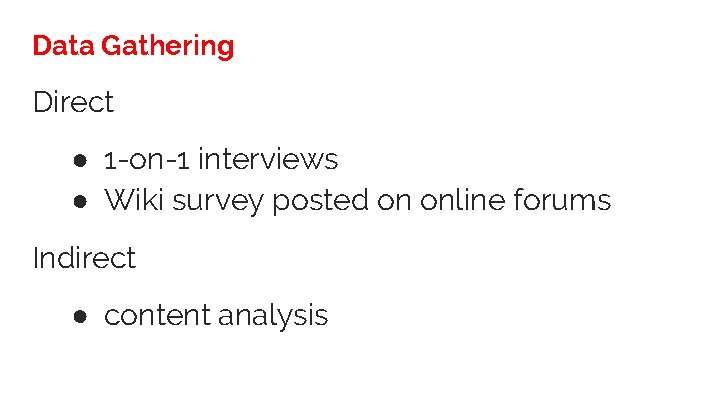
Data Gathering Direct ● 1 -on-1 interviews ● Wiki survey posted on online forums Indirect ● content analysis
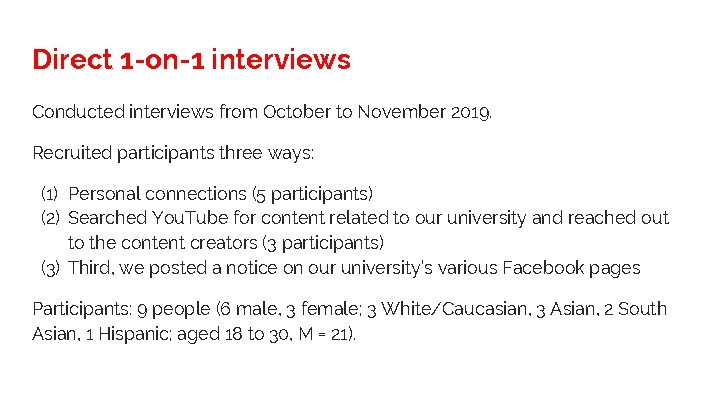
Direct 1 -on-1 interviews Conducted interviews from October to November 2019. Recruited participants three ways: (1) Personal connections (5 participants) (2) Searched You. Tube for content related to our university and reached out to the content creators (3 participants) (3) Third, we posted a notice on our university’s various Facebook pages Participants: 9 people (6 male, 3 female; 3 White/Caucasian, 3 Asian, 2 South Asian, 1 Hispanic; aged 18 to 30, M = 21).
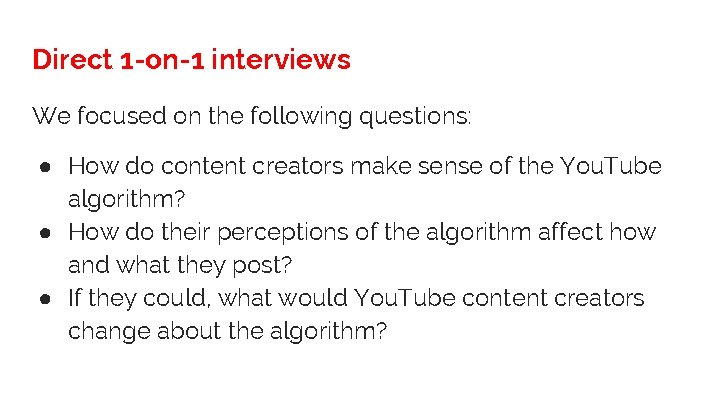
Direct 1 -on-1 interviews We focused on the following questions: ● How do content creators make sense of the You. Tube algorithm? ● How do their perceptions of the algorithm affect how and what they post? ● If they could, what would You. Tube content creators change about the algorithm?
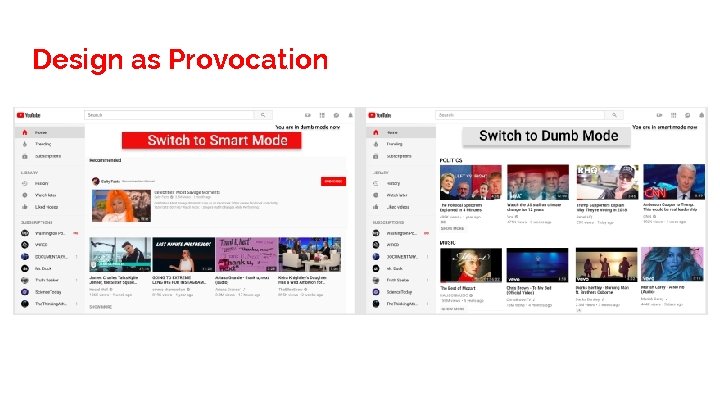
Design as Provocation
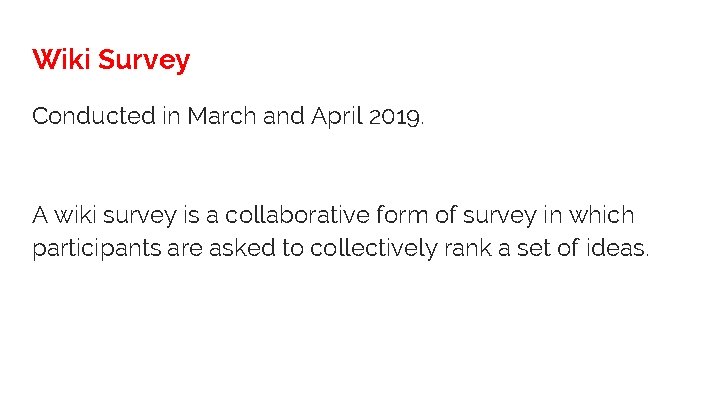
Wiki Survey Conducted in March and April 2019. A wiki survey is a collaborative form of survey in which participants are asked to collectively rank a set of ideas.
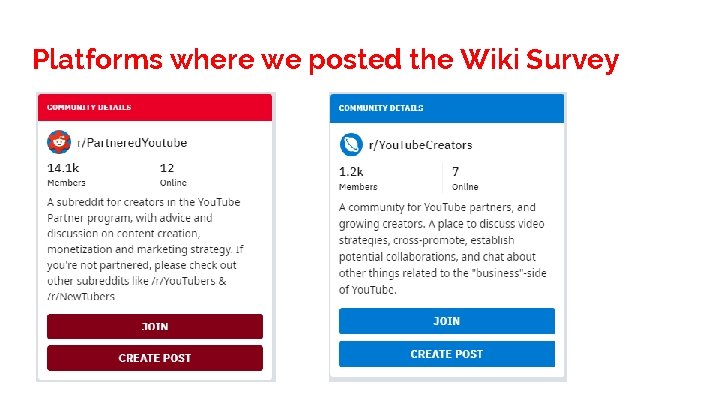
Platforms where we posted the Wiki Survey
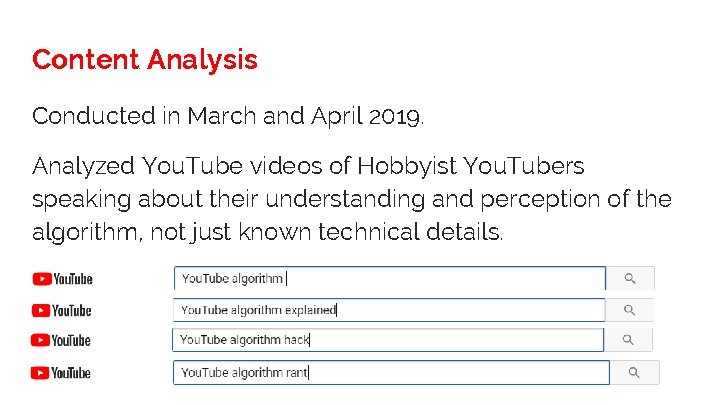
Content Analysis Conducted in March and April 2019. Analyzed You. Tube videos of Hobbyist You. Tubers speaking about their understanding and perception of the algorithm, not just known technical details. of the algorithm.
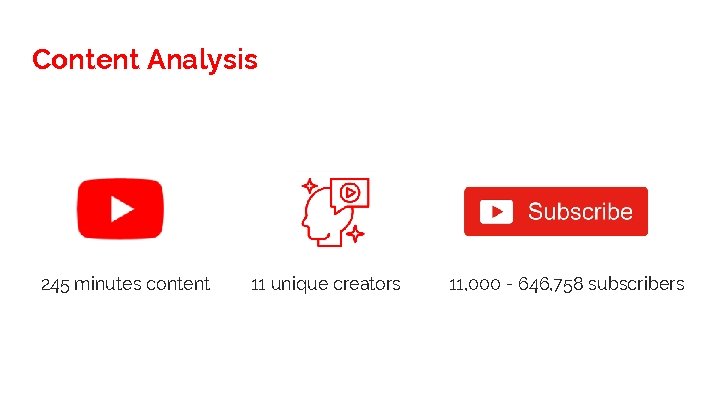
Content Analysis 245 minutes content 11 unique creators 11, 000 - 646, 758 subscribers
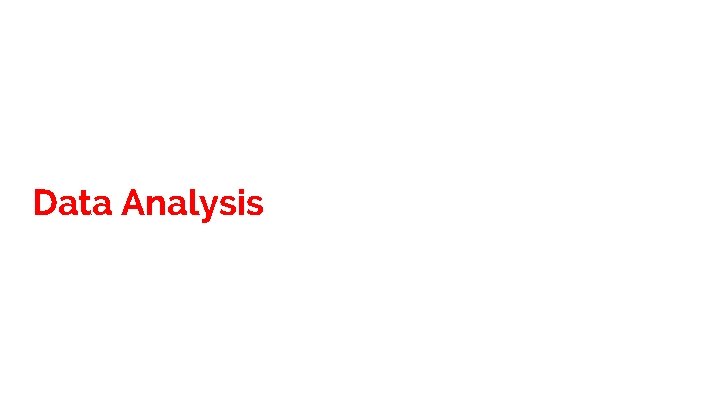
Data Analysis
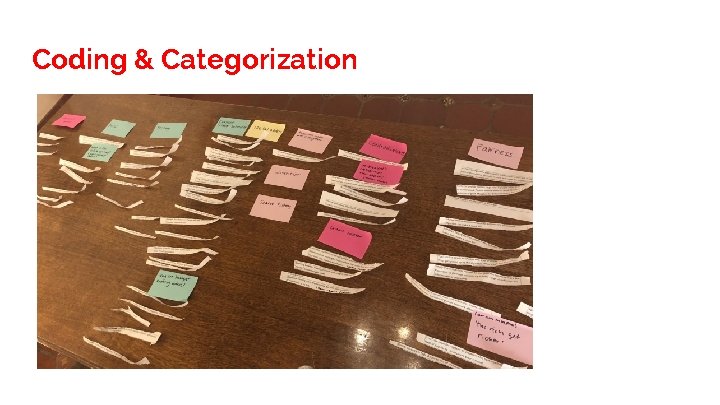
Coding & Categorization
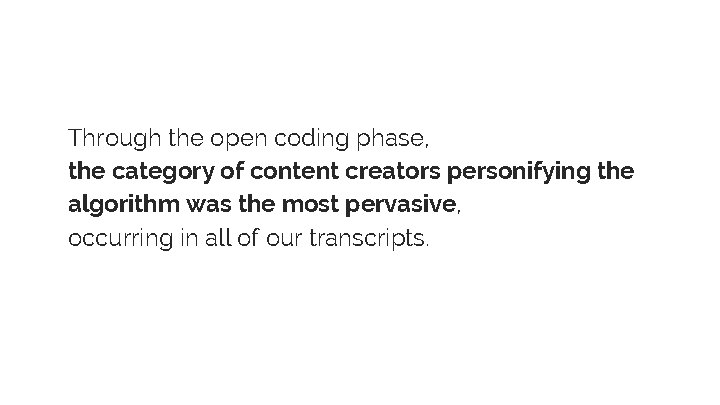
Through the open coding phase, the category of content creators personifying the algorithm was the most pervasive, occurring in all of our transcripts.
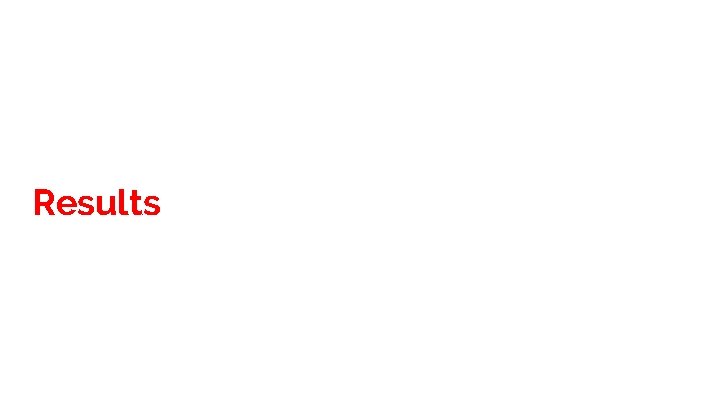
Results
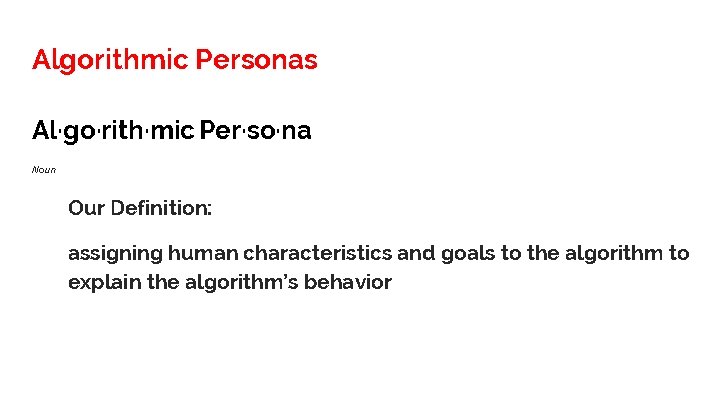
Algorithmic Personas Al·go·rith·mic Per·so·na Noun Our Definition: assigning human characteristics and goals to the algorithm to explain the algorithm’s behavior
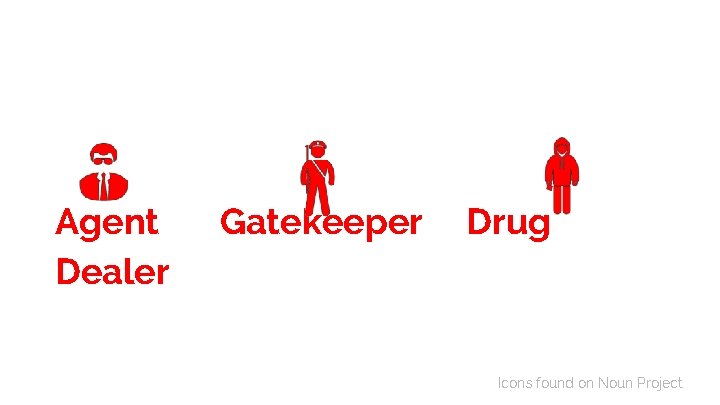
Agent Dealer Gatekeeper Drug Icons found on Noun Project
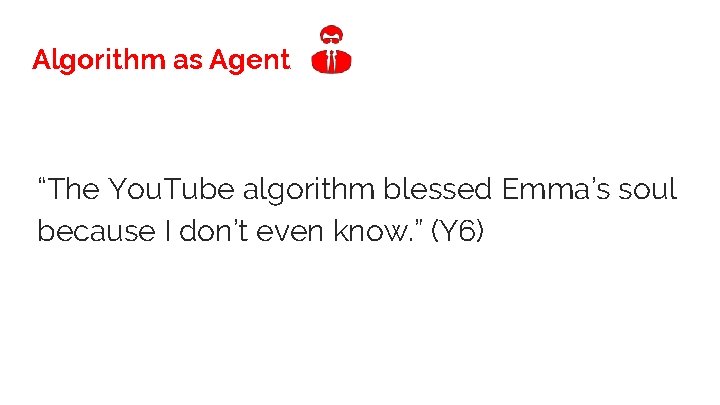
Algorithm as Agent “The You. Tube algorithm blessed Emma’s soul because I don’t even know. ” (Y 6)
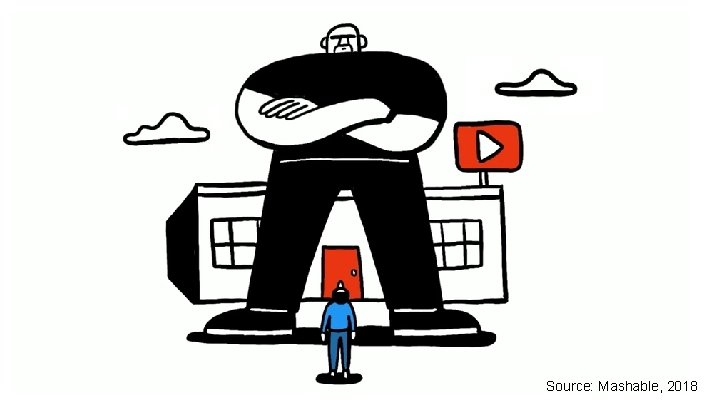
Source: Mashable, 2018
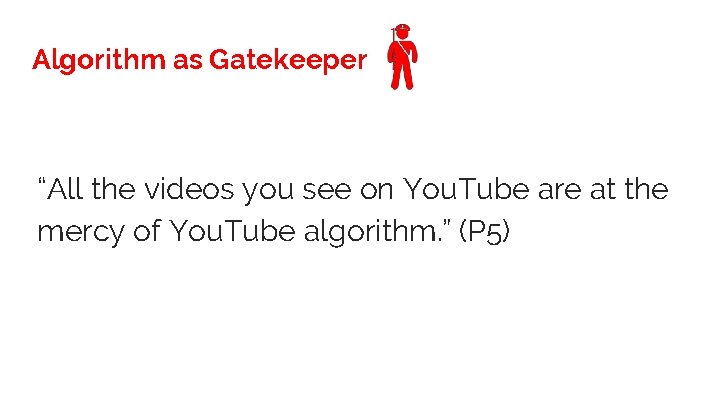
Algorithm as Gatekeeper “All the videos you see on You. Tube are at the mercy of You. Tube algorithm. ” (P 5)
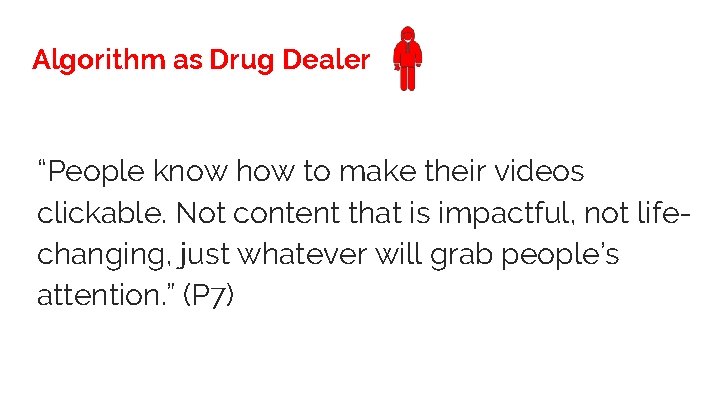
Algorithm as Drug Dealer “People know how to make their videos clickable. Not content that is impactful, not lifechanging, just whatever will grab people’s attention. ” (P 7)
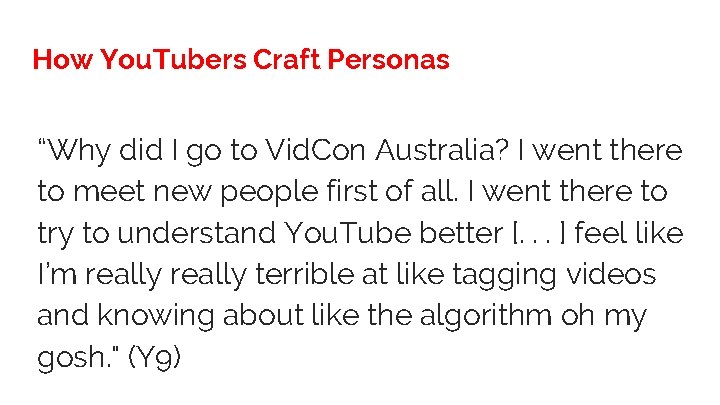
How You. Tubers Craft Personas “Why did I go to Vid. Con Australia? I went there to meet new people first of all. I went there to try to understand You. Tube better [. . . ] feel like I’m really terrible at like tagging videos and knowing about like the algorithm oh my gosh. " (Y 9)
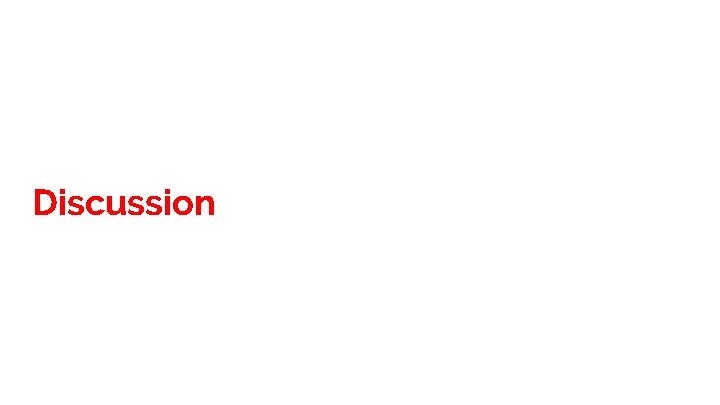
Discussion
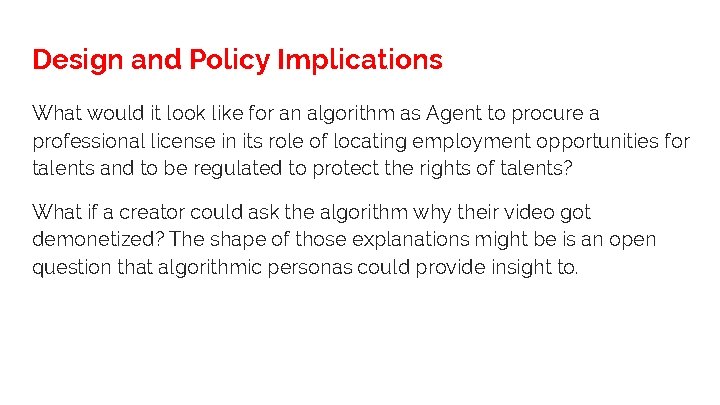
Design and Policy Implications What would it look like for an algorithm as Agent to procure a professional license in its role of locating employment opportunities for talents and to be regulated to protect the rights of talents? What if a creator could ask the algorithm why their video got demonetized? The shape of those explanations might be is an open question that algorithmic personas could provide insight to.
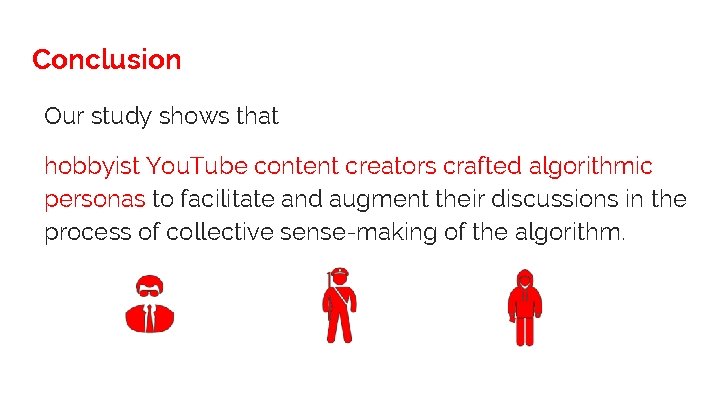
Conclusion Our study shows that hobbyist You. Tube content creators crafted algorithmic personas to facilitate and augment their discussions in the process of collective sense-making of the algorithm.
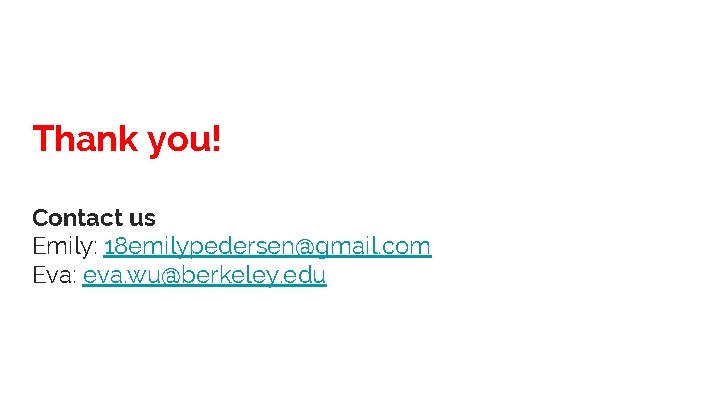
Thank you! Contact us Emily: 18 emilypedersen@gmail. com Eva: eva. wu@berkeley. edu
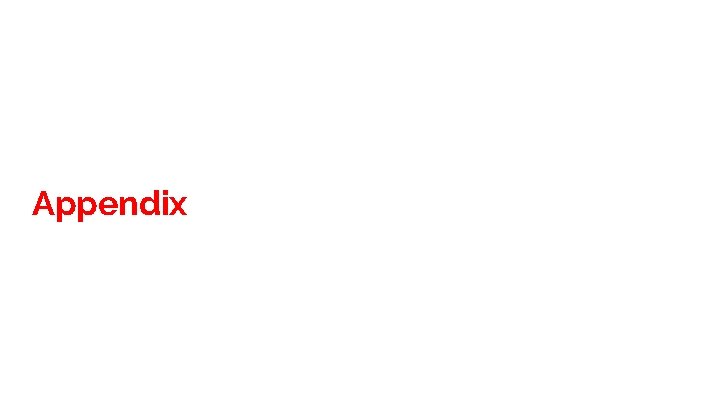
Appendix
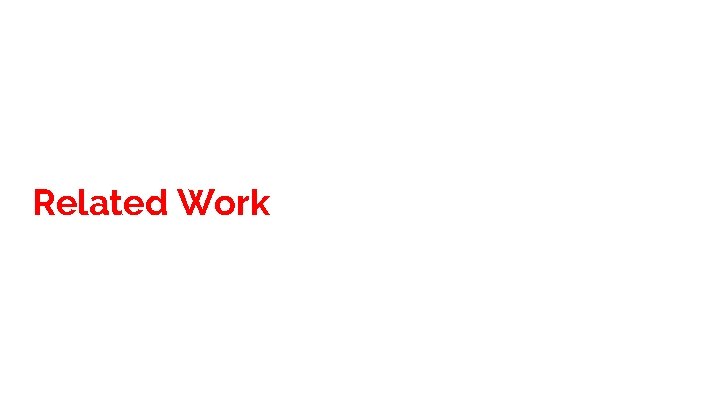
Related Work
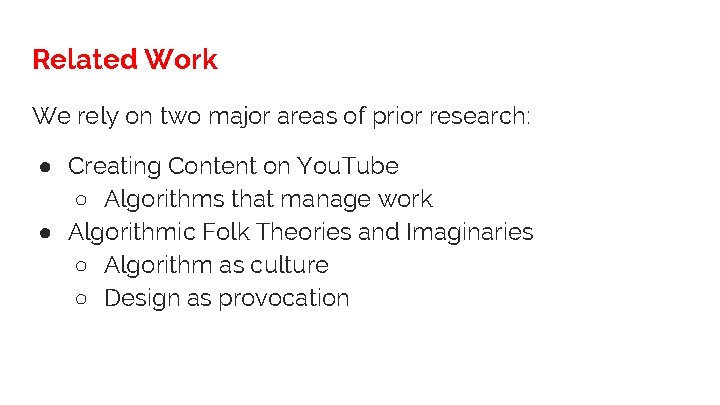
Related Work We rely on two major areas of prior research: ● Creating Content on You. Tube ○ Algorithms that manage work ● Algorithmic Folk Theories and Imaginaries ○ Algorithm as culture ○ Design as provocation
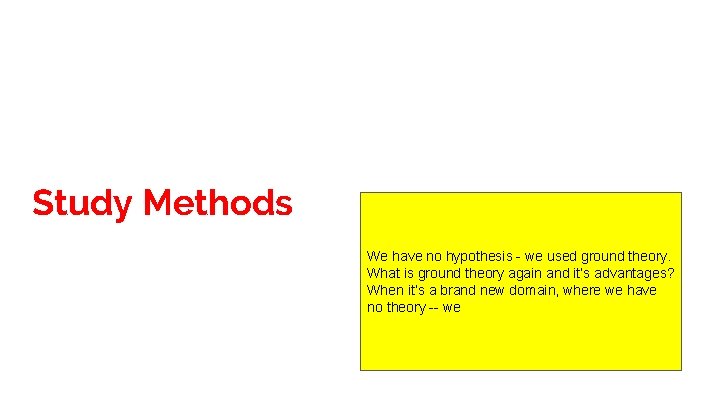
Study Methods We have no hypothesis - we used ground theory. What is ground theory again and it’s advantages? When it’s a brand new domain, where we have no theory -- we
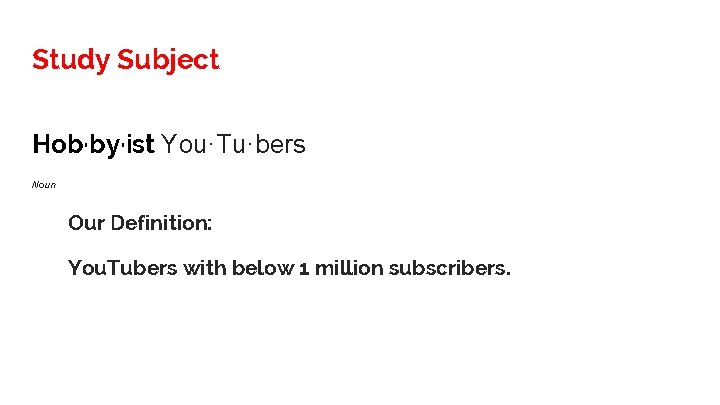
Study Subject Hob·by·ist You·Tu·bers Noun Our Definition: You. Tubers with below 1 million subscribers.
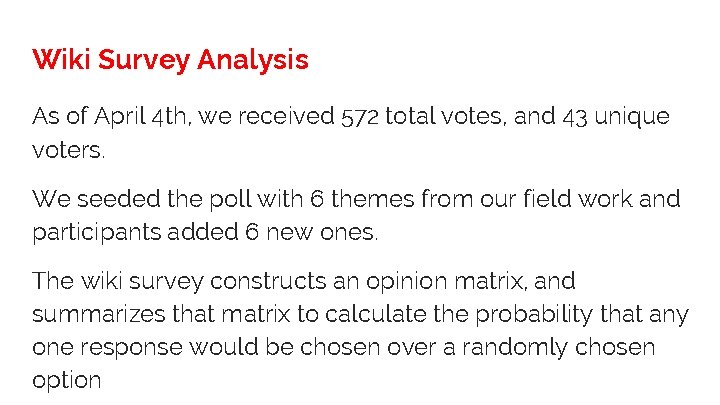
Wiki Survey Analysis As of April 4 th, we received 572 total votes, and 43 unique voters. We seeded the poll with 6 themes from our field work and participants added 6 new ones. The wiki survey constructs an opinion matrix, and summarizes that matrix to calculate the probability that any one response would be chosen over a randomly chosen option
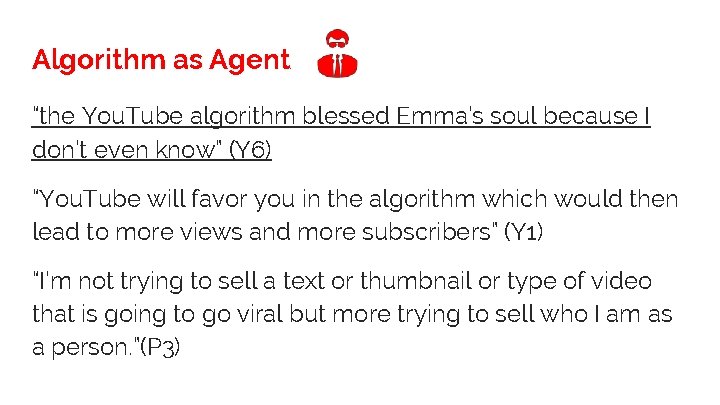
Algorithm as Agent “the You. Tube algorithm blessed Emma’s soul because I don’t even know” (Y 6) “You. Tube will favor you in the algorithm which would then lead to more views and more subscribers” (Y 1) “I’m not trying to sell a text or thumbnail or type of video that is going to go viral but more trying to sell who I am as a person. ”(P 3)
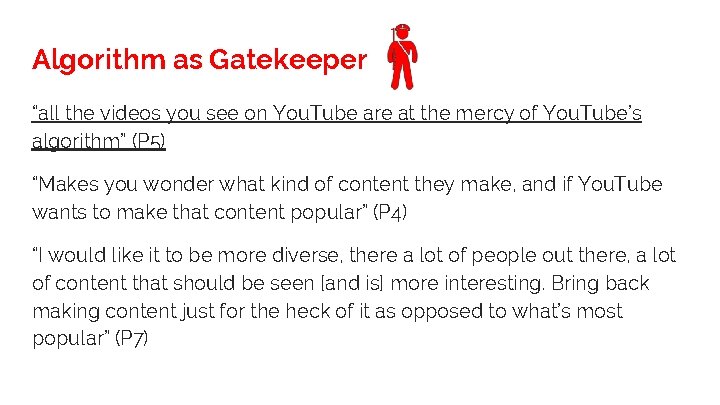
Algorithm as Gatekeeper “all the videos you see on You. Tube are at the mercy of You. Tube’s algorithm” (P 5) “Makes you wonder what kind of content they make, and if You. Tube wants to make that content popular” (P 4) “I would like it to be more diverse, there a lot of people out there, a lot of content that should be seen [and is] more interesting. Bring back making content just for the heck of it as opposed to what’s most popular” (P 7)
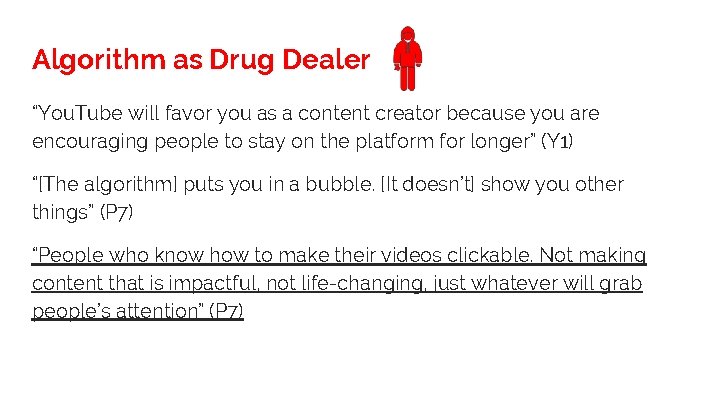
Algorithm as Drug Dealer “You. Tube will favor you as a content creator because you are encouraging people to stay on the platform for longer” (Y 1) “[The algorithm] puts you in a bubble. [It doesn’t] show you other things” (P 7) “People who know how to make their videos clickable. Not making content that is impactful, not life-changing, just whatever will grab people’s attention” (P 7)
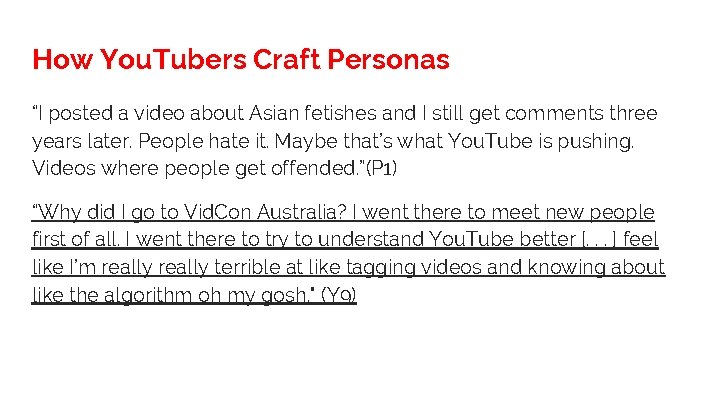
How You. Tubers Craft Personas “I posted a video about Asian fetishes and I still get comments three years later. People hate it. Maybe that’s what You. Tube is pushing. Videos where people get offended. ”(P 1) “Why did I go to Vid. Con Australia? I went there to meet new people first of all. I went there to try to understand You. Tube better [. . . ] feel like I’m really terrible at like tagging videos and knowing about like the algorithm oh my gosh. " (Y 9)
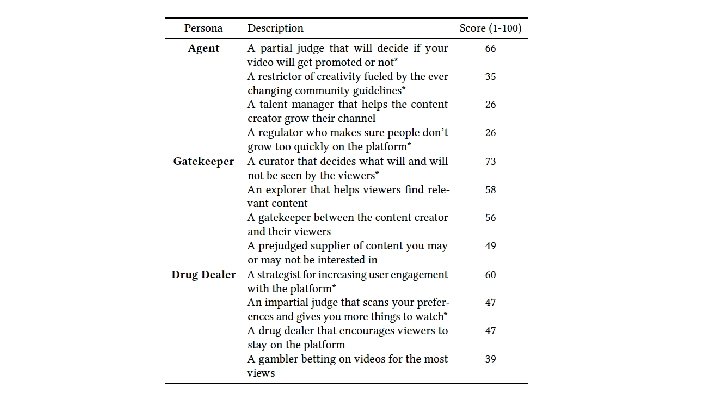
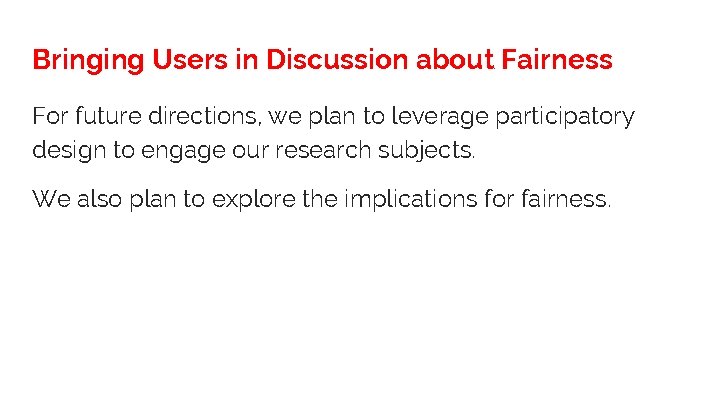
Bringing Users in Discussion about Fairness For future directions, we plan to leverage participatory design to engage our research subjects. We also plan to explore the implications for fairness.
Who are the three creators of money in the united states?
Creators spend as much time as possible in quadrant
Language windows 10
The creators of grammar answers
Uml creators
Deliberate adulteration definition
Agent function vs agent program
Reducing agent reduces
Peas description for part-picking robot
Standard potential table
Oil rig oxidation
Principles communication
Gatekeeper scorekeeper watchdog
Qpr training quiz answers
Decision making unit gatekeeper
Gatekeeperfunktion
Gatekeeper meaning
Meekaaeel angel
Contoh gatekeeper
Qpr gatekeeper quiz answers
Qpr gatekeeper quiz answers
Gatekeepermedia
Gatekeeper training
Subscription management oracle
Dynamic content vs static content
What is esp
Regina brett plain dealer
Michelin aad program
Dealer market
Auto dealership accounting guide
Ucfin dealer
Cattle dealer
Constant growth dividend discount model
Car dealer magazine
Zaz gps reviews
Derelict vehicle certificate
Kioti university
Bureau of dealer services
Galaxy control systems
For dealer use only
Boost sales portal
Aplikasi dealer motor
Pitbull real name
For dealer use only