WIGOS and Data Sharing WIGOS Surface 2040 Geneva
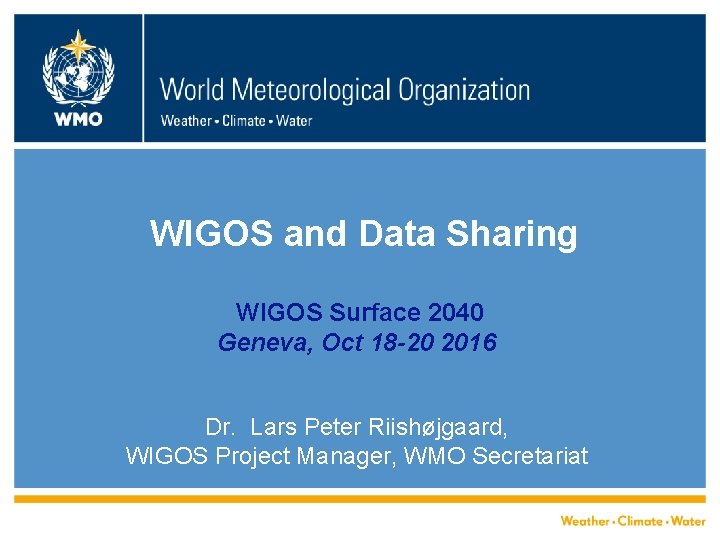
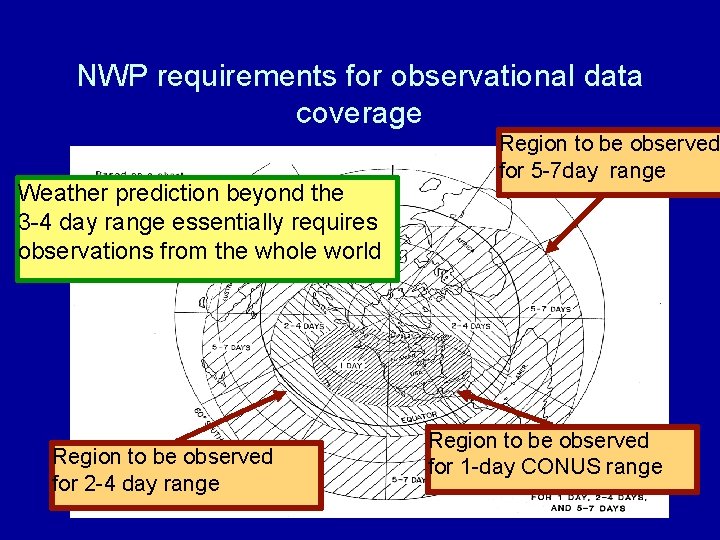
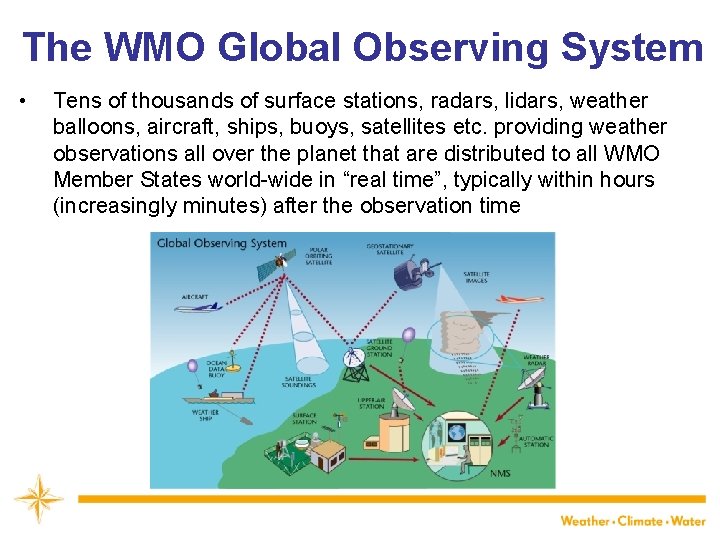
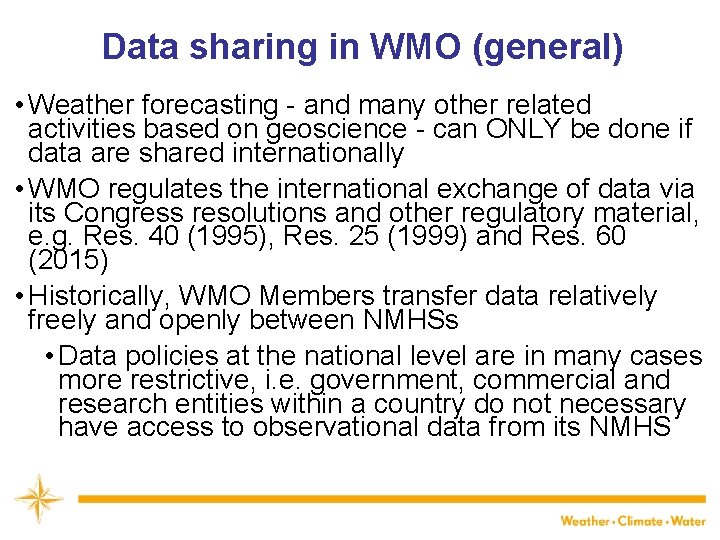
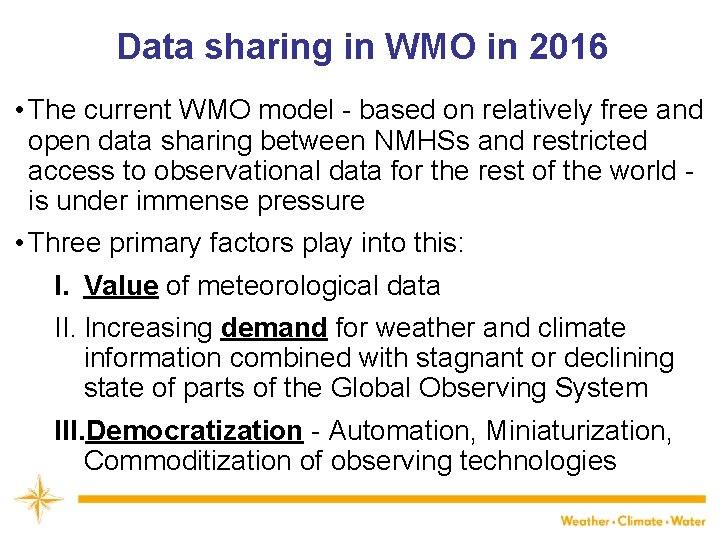
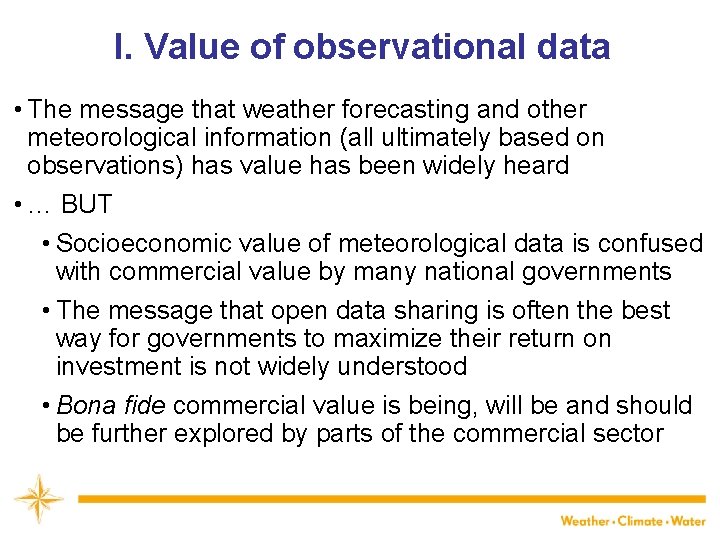
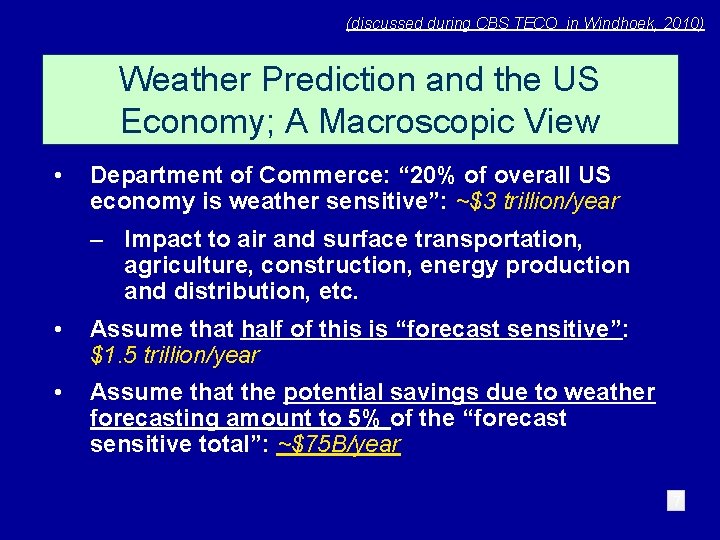
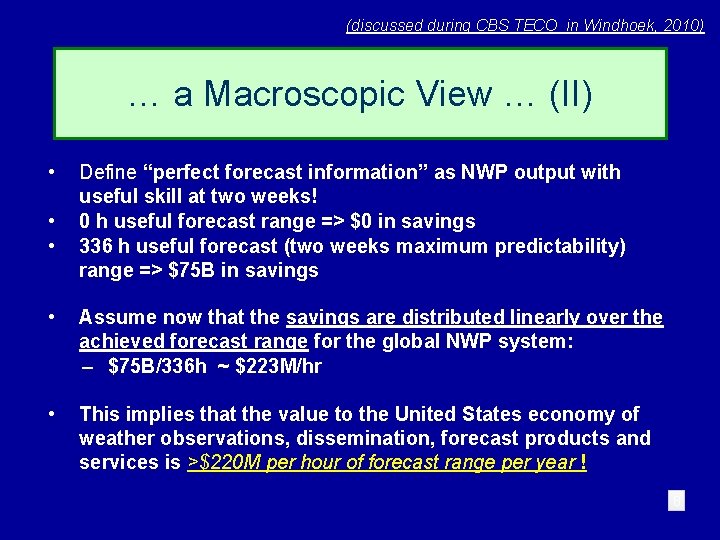
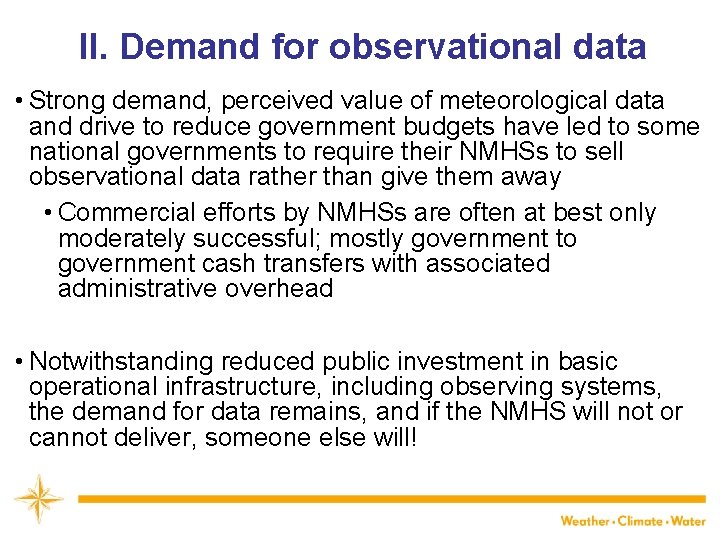
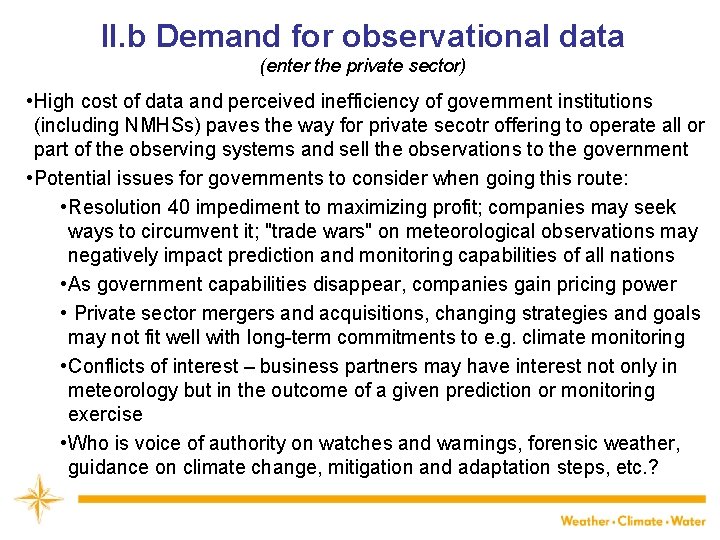
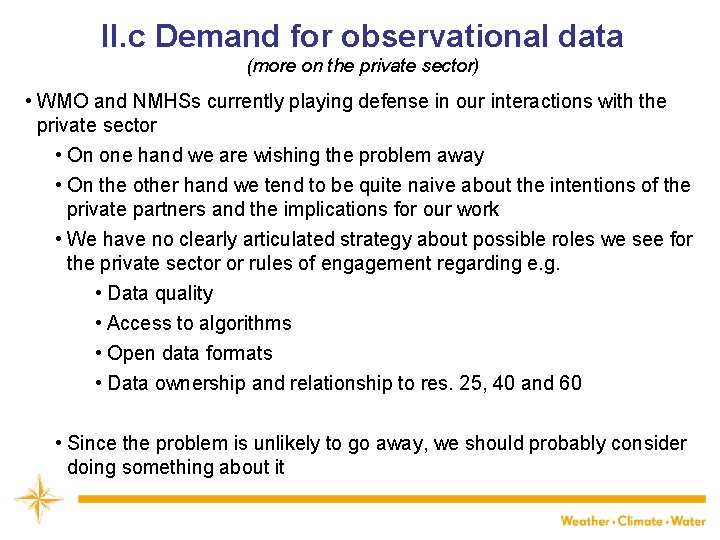
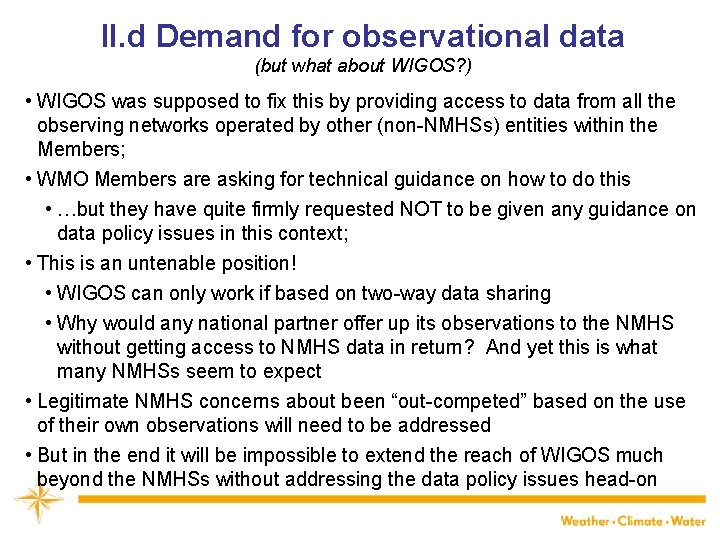
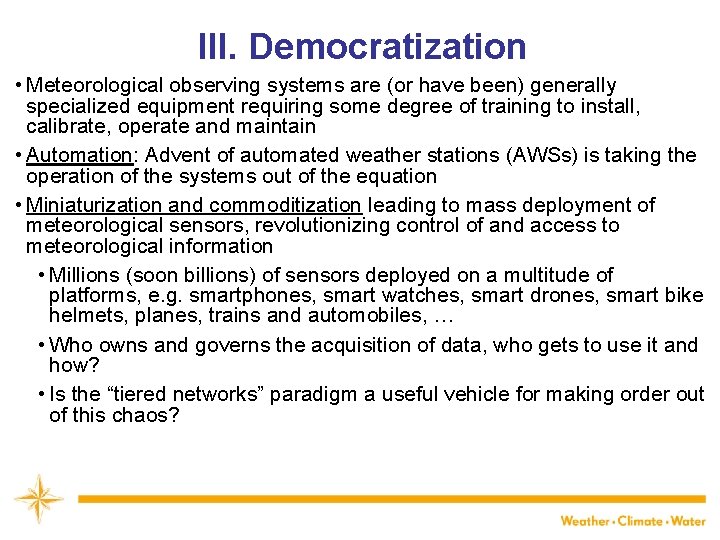
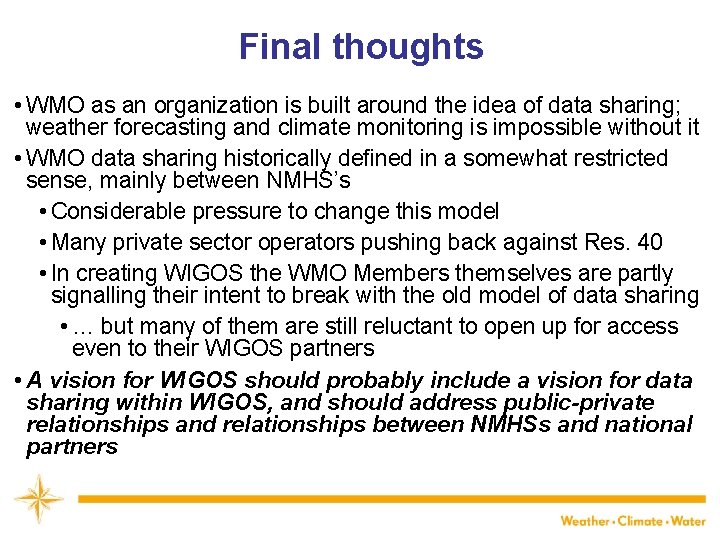
- Slides: 14
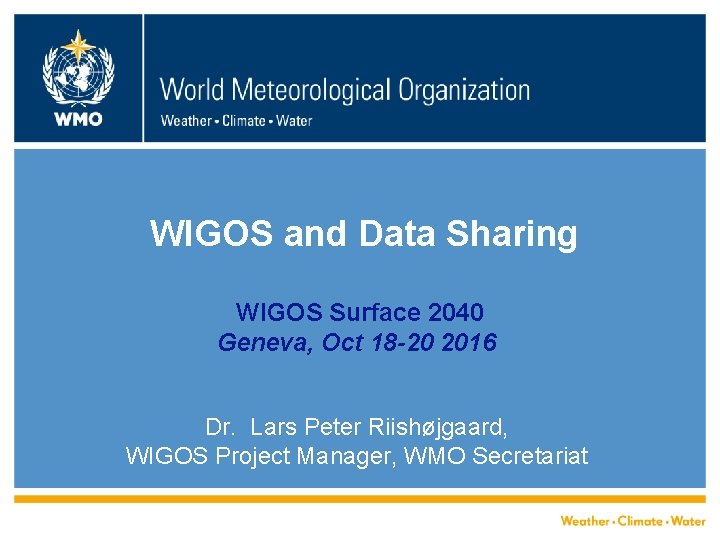
WIGOS and Data Sharing WIGOS Surface 2040 Geneva, Oct 18 -20 2016 Dr. Lars Peter Riishøjgaard, WIGOS Project Manager, WMO Secretariat
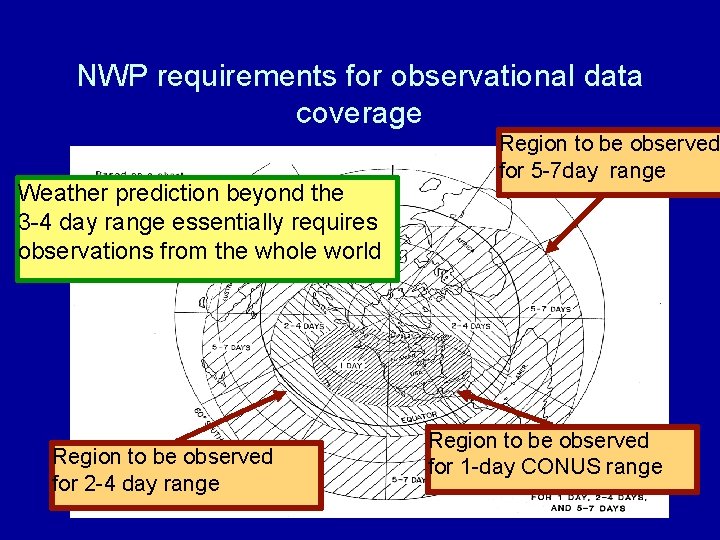
NWP requirements for observational data coverage Weather prediction beyond the 3 -4 day range essentially requires observations from the whole world Region to be observed for 2 -4 day range Region to be observed for 5 -7 day range Region to be observed for 1 -day CONUS range
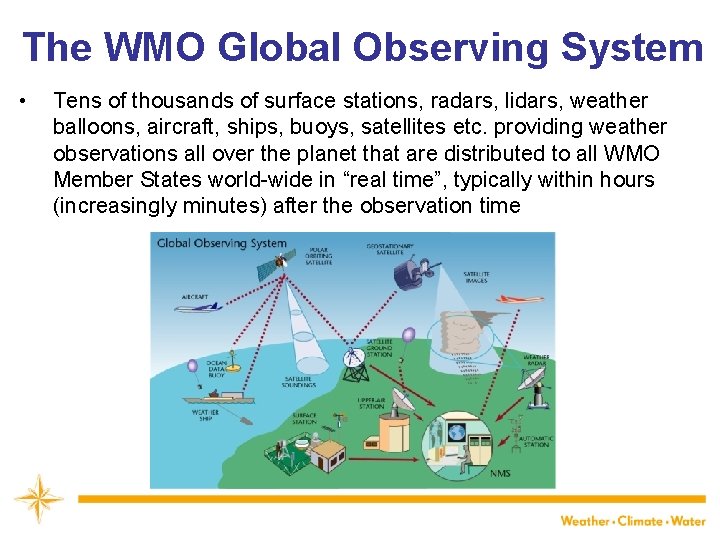
The WMO Global Observing System • Tens of thousands of surface stations, radars, lidars, weather balloons, aircraft, ships, buoys, satellites etc. providing weather observations all over the planet that are distributed to all WMO Member States world-wide in “real time”, typically within hours (increasingly minutes) after the observation time
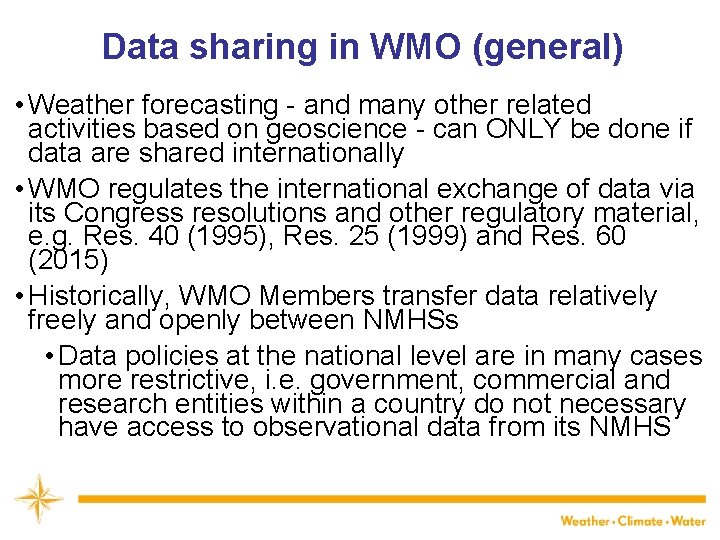
Data sharing in WMO (general) • Weather forecasting - and many other related activities based on geoscience - can ONLY be done if data are shared internationally • WMO regulates the international exchange of data via its Congress resolutions and other regulatory material, e. g. Res. 40 (1995), Res. 25 (1999) and Res. 60 (2015) • Historically, WMO Members transfer data relatively freely and openly between NMHSs • Data policies at the national level are in many cases more restrictive, i. e. government, commercial and research entities within a country do not necessary have access to observational data from its NMHS
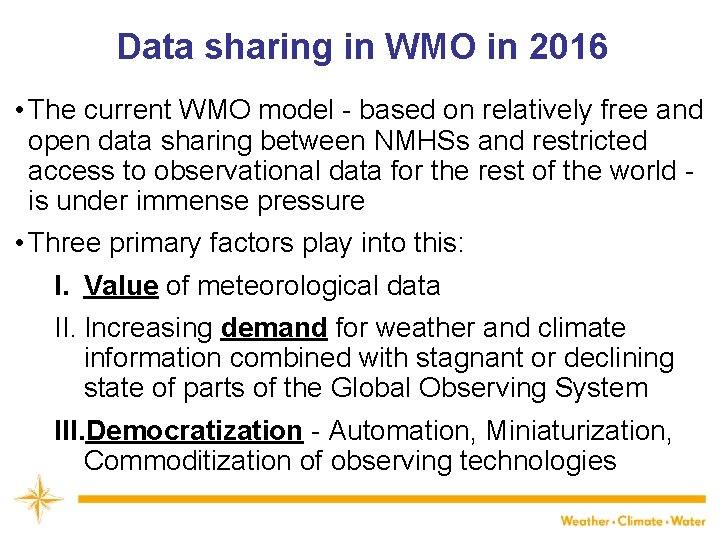
Data sharing in WMO in 2016 • The current WMO model - based on relatively free and open data sharing between NMHSs and restricted access to observational data for the rest of the world is under immense pressure • Three primary factors play into this: I. Value of meteorological data II. Increasing demand for weather and climate information combined with stagnant or declining state of parts of the Global Observing System III. Democratization - Automation, Miniaturization, Commoditization of observing technologies
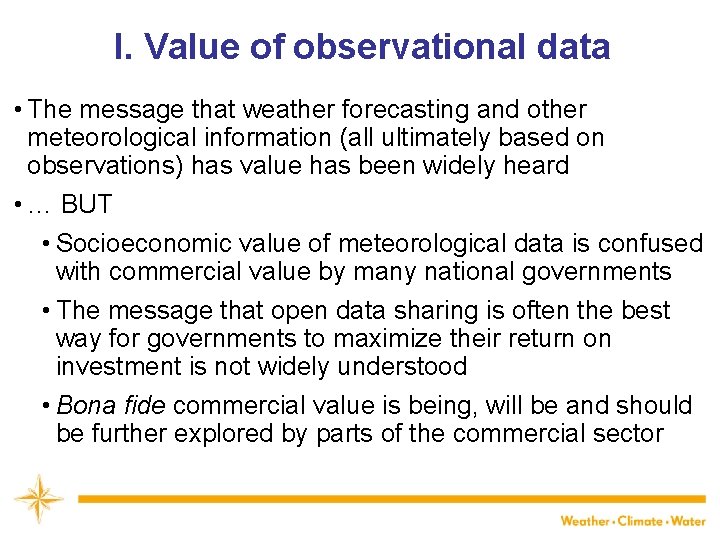
I. Value of observational data • The message that weather forecasting and other meteorological information (all ultimately based on observations) has value has been widely heard • … BUT • Socioeconomic value of meteorological data is confused with commercial value by many national governments • The message that open data sharing is often the best way for governments to maximize their return on investment is not widely understood • Bona fide commercial value is being, will be and should be further explored by parts of the commercial sector
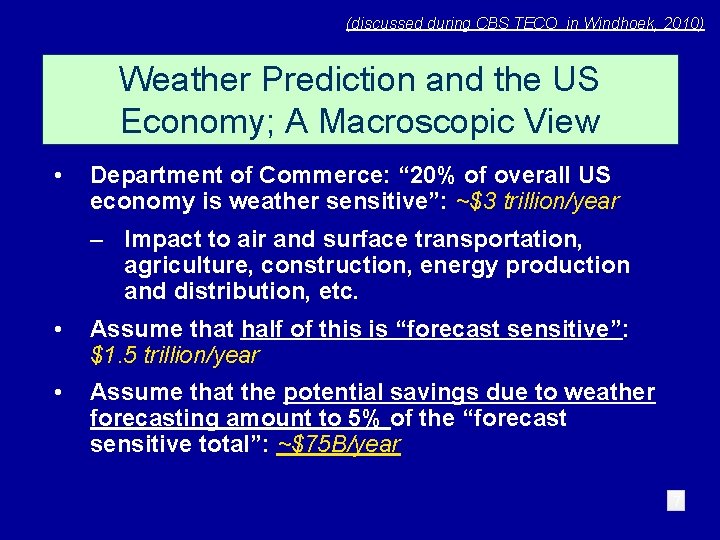
(discussed during CBS TECO in Windhoek, 2010) Weather Prediction and the US Economy; A Macroscopic View • Department of Commerce: “ 20% of overall US economy is weather sensitive”: ~$3 trillion/year – Impact to air and surface transportation, agriculture, construction, energy production and distribution, etc. • Assume that half of this is “forecast sensitive”: $1. 5 trillion/year • Assume that the potential savings due to weather forecasting amount to 5% of the “forecast sensitive total”: ~$75 B/year 7
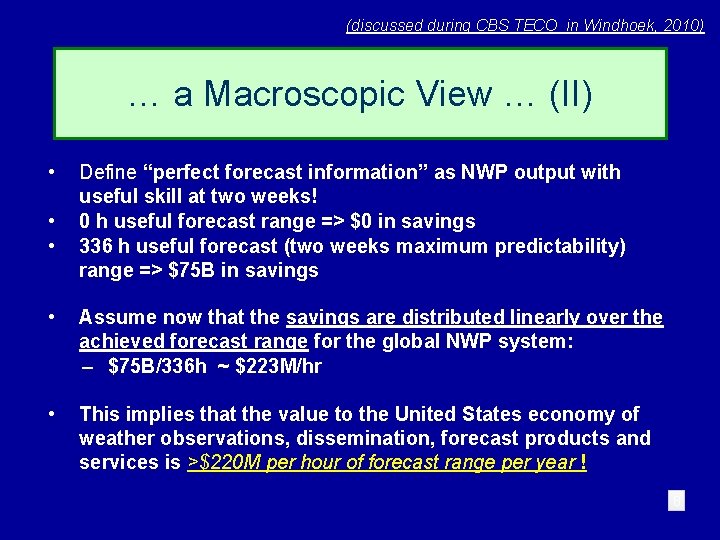
(discussed during CBS TECO in Windhoek, 2010) … a Macroscopic View … (II) • • • Define “perfect forecast information” as NWP output with useful skill at two weeks! 0 h useful forecast range => $0 in savings 336 h useful forecast (two weeks maximum predictability) range => $75 B in savings • Assume now that the savings are distributed linearly over the achieved forecast range for the global NWP system: – $75 B/336 h ~ $223 M/hr • This implies that the value to the United States economy of weather observations, dissemination, forecast products and services is >$220 M per hour of forecast range per year ! 8
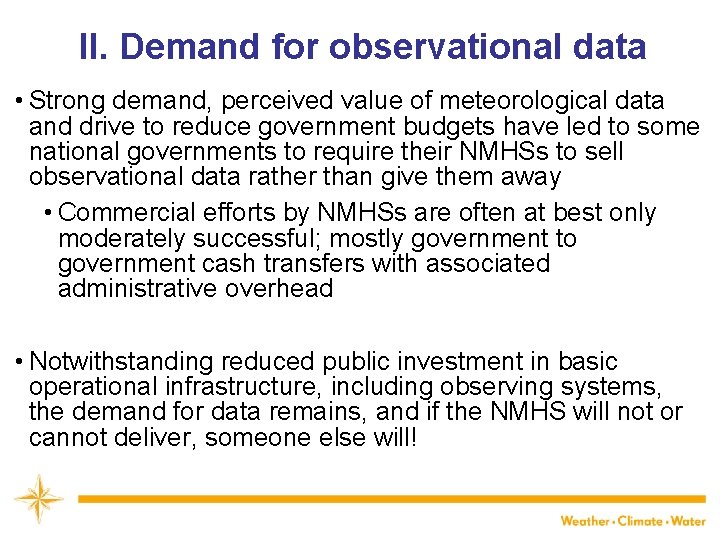
II. Demand for observational data • Strong demand, perceived value of meteorological data and drive to reduce government budgets have led to some national governments to require their NMHSs to sell observational data rather than give them away • Commercial efforts by NMHSs are often at best only moderately successful; mostly government to government cash transfers with associated administrative overhead • Notwithstanding reduced public investment in basic operational infrastructure, including observing systems, the demand for data remains, and if the NMHS will not or cannot deliver, someone else will!
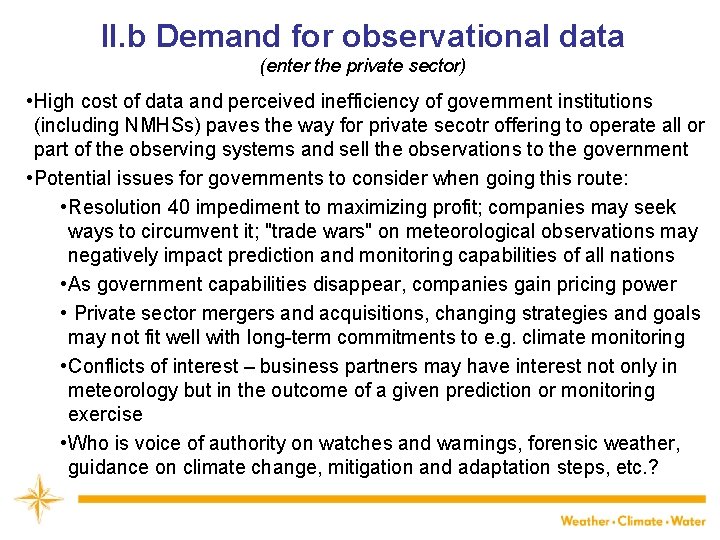
II. b Demand for observational data (enter the private sector) • High cost of data and perceived inefficiency of government institutions (including NMHSs) paves the way for private secotr offering to operate all or part of the observing systems and sell the observations to the government • Potential issues for governments to consider when going this route: • Resolution 40 impediment to maximizing profit; companies may seek ways to circumvent it; "trade wars" on meteorological observations may negatively impact prediction and monitoring capabilities of all nations • As government capabilities disappear, companies gain pricing power • Private sector mergers and acquisitions, changing strategies and goals may not fit well with long-term commitments to e. g. climate monitoring • Conflicts of interest – business partners may have interest not only in meteorology but in the outcome of a given prediction or monitoring exercise • Who is voice of authority on watches and warnings, forensic weather, guidance on climate change, mitigation and adaptation steps, etc. ?
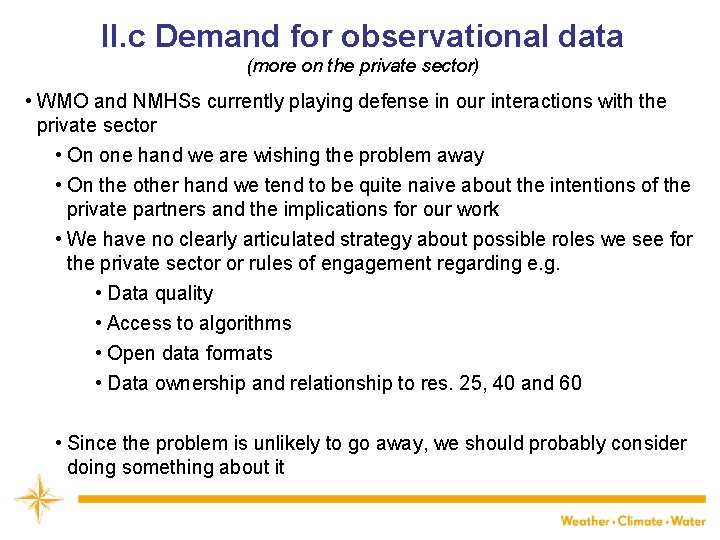
II. c Demand for observational data (more on the private sector) • WMO and NMHSs currently playing defense in our interactions with the private sector • On one hand we are wishing the problem away • On the other hand we tend to be quite naive about the intentions of the private partners and the implications for our work • We have no clearly articulated strategy about possible roles we see for the private sector or rules of engagement regarding e. g. • Data quality • Access to algorithms • Open data formats • Data ownership and relationship to res. 25, 40 and 60 • Since the problem is unlikely to go away, we should probably consider doing something about it
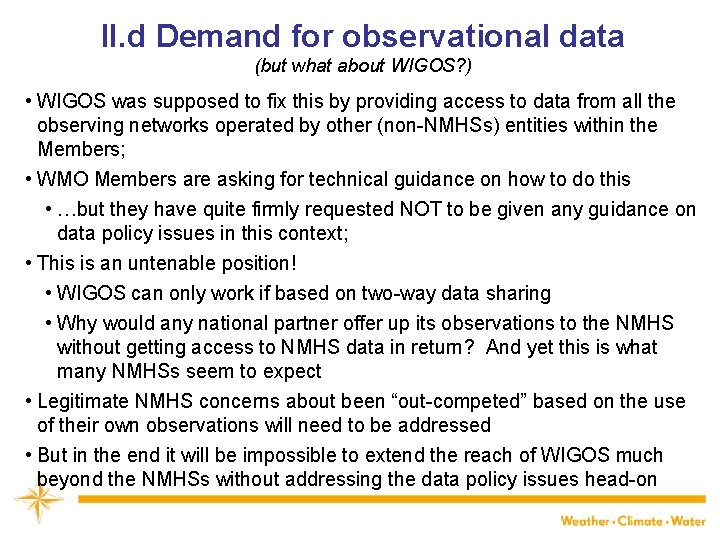
II. d Demand for observational data (but what about WIGOS? ) • WIGOS was supposed to fix this by providing access to data from all the observing networks operated by other (non-NMHSs) entities within the Members; • WMO Members are asking for technical guidance on how to do this • …but they have quite firmly requested NOT to be given any guidance on data policy issues in this context; • This is an untenable position! • WIGOS can only work if based on two-way data sharing • Why would any national partner offer up its observations to the NMHS without getting access to NMHS data in return? And yet this is what many NMHSs seem to expect • Legitimate NMHS concerns about been “out-competed” based on the use of their own observations will need to be addressed • But in the end it will be impossible to extend the reach of WIGOS much beyond the NMHSs without addressing the data policy issues head-on
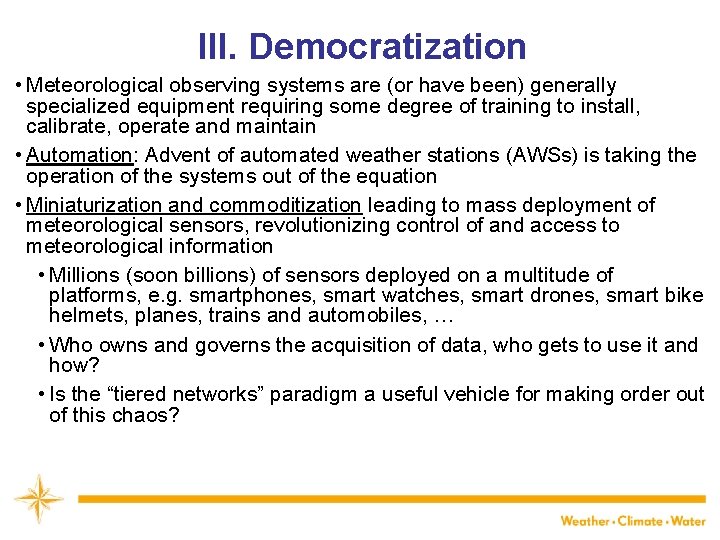
III. Democratization • Meteorological observing systems are (or have been) generally specialized equipment requiring some degree of training to install, calibrate, operate and maintain • Automation: Advent of automated weather stations (AWSs) is taking the operation of the systems out of the equation • Miniaturization and commoditization leading to mass deployment of meteorological sensors, revolutionizing control of and access to meteorological information • Millions (soon billions) of sensors deployed on a multitude of platforms, e. g. smartphones, smart watches, smart drones, smart bike helmets, planes, trains and automobiles, … • Who owns and governs the acquisition of data, who gets to use it and how? • Is the “tiered networks” paradigm a useful vehicle for making order out of this chaos?
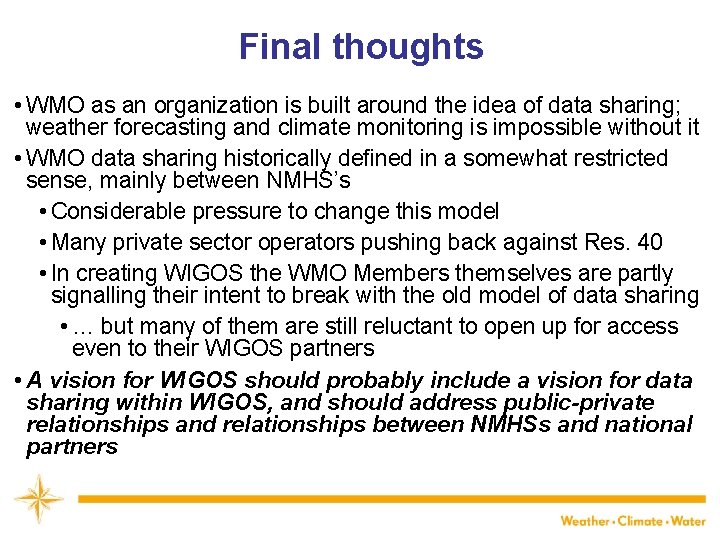
Final thoughts • WMO as an organization is built around the idea of data sharing; weather forecasting and climate monitoring is impossible without it • WMO data sharing historically defined in a somewhat restricted sense, mainly between NMHS’s • Considerable pressure to change this model • Many private sector operators pushing back against Res. 40 • In creating WIGOS the WMO Members themselves are partly signalling their intent to break with the old model of data sharing • … but many of them are still reluctant to open up for access even to their WIGOS partners • A vision for WIGOS should probably include a vision for data sharing within WIGOS, and should address public-private relationships and relationships between NMHSs and national partners