What is fiducial inference and why Gunnar Taraldsen
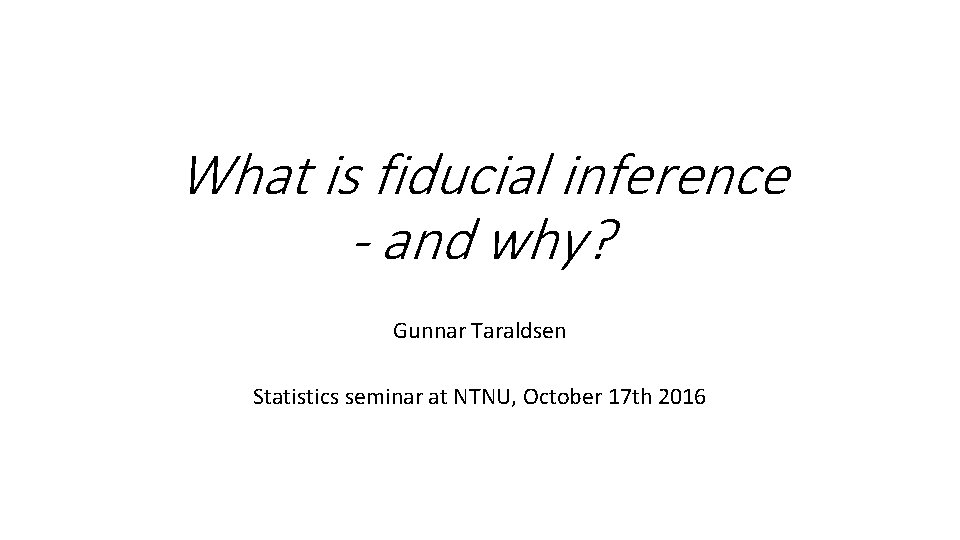
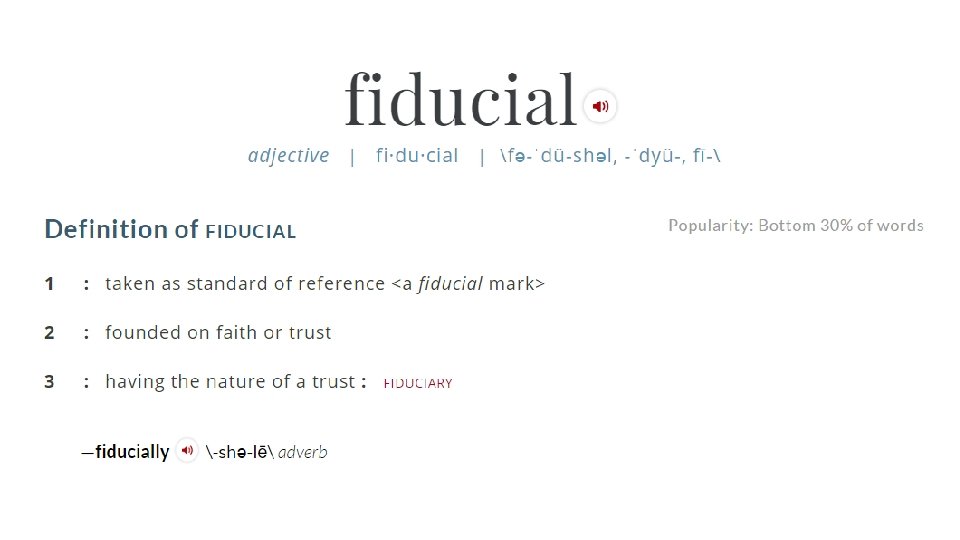
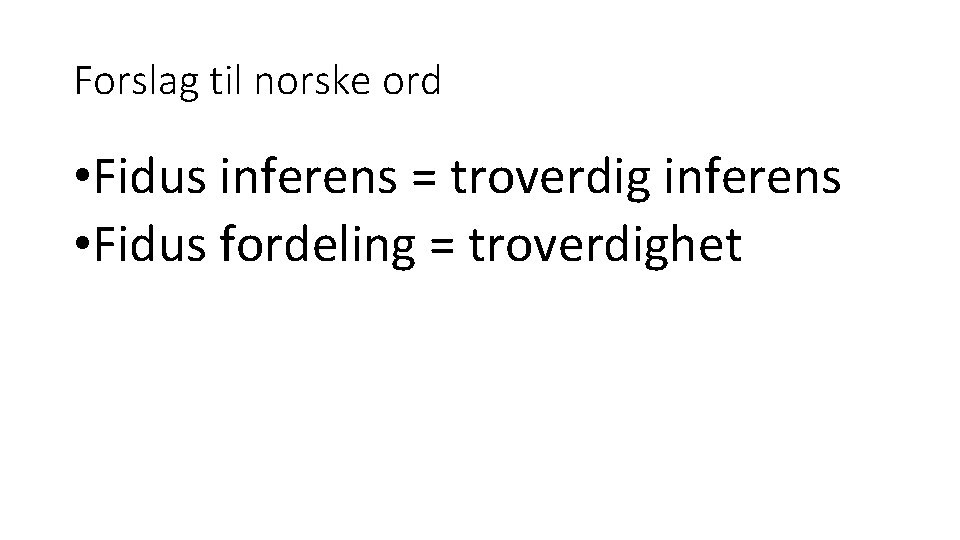
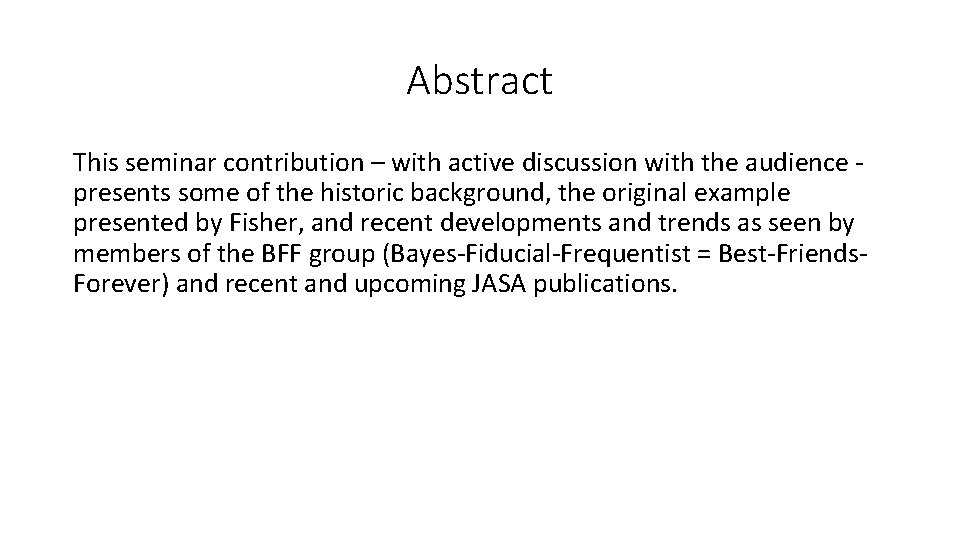
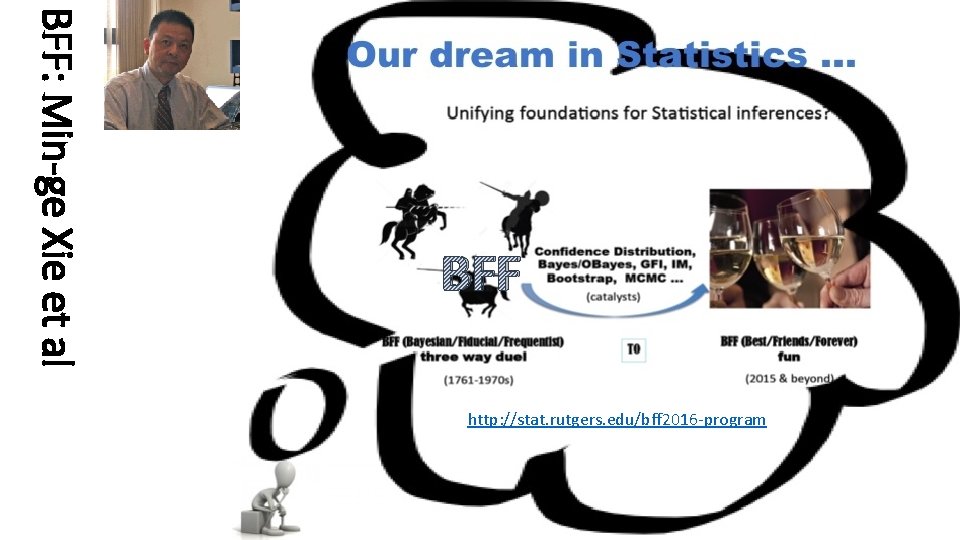
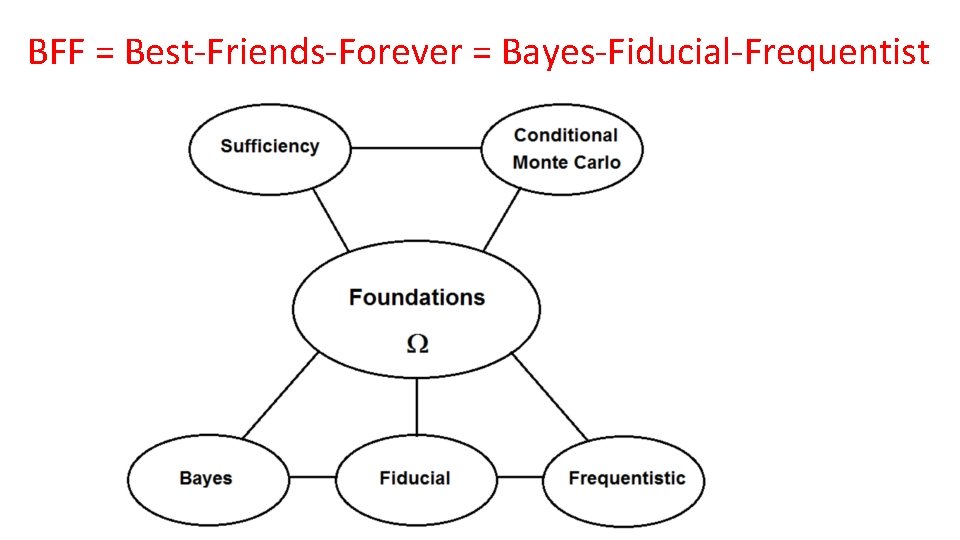
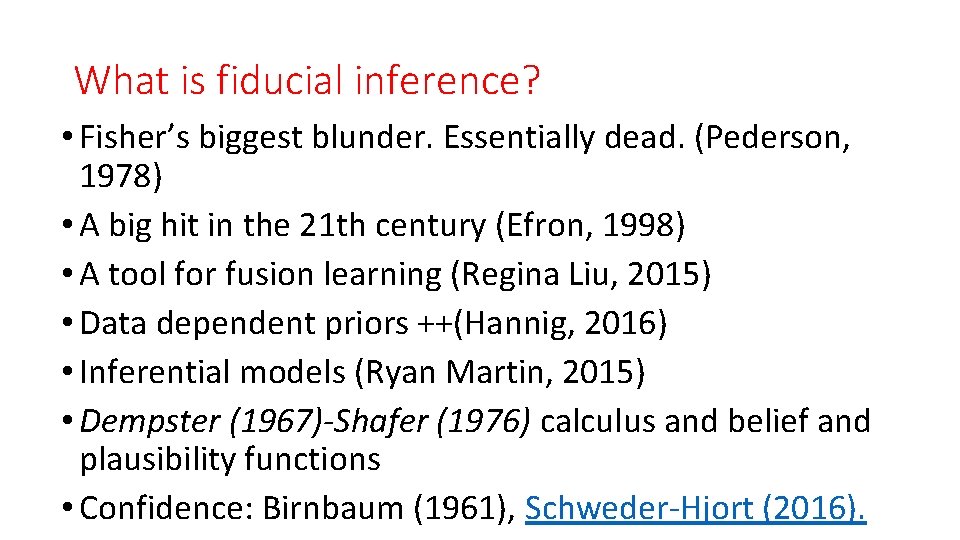
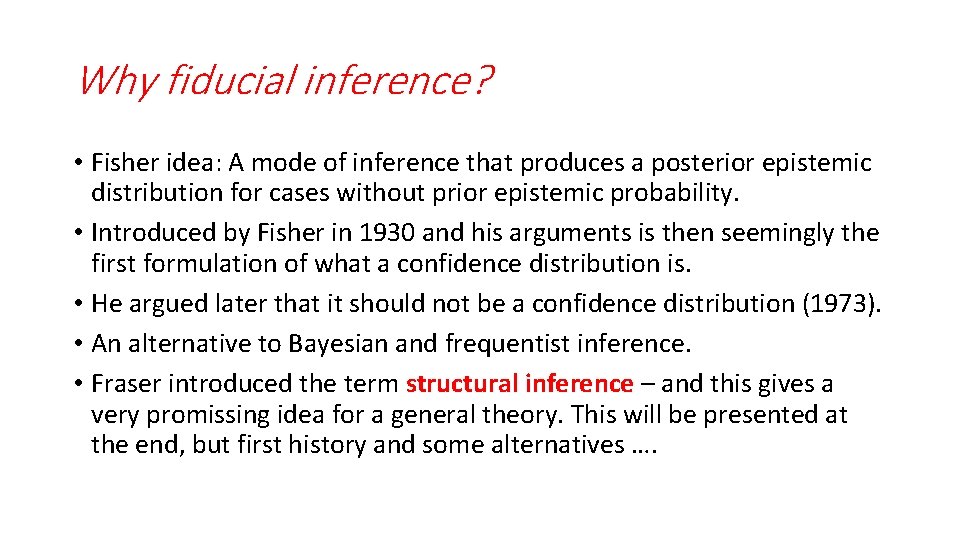
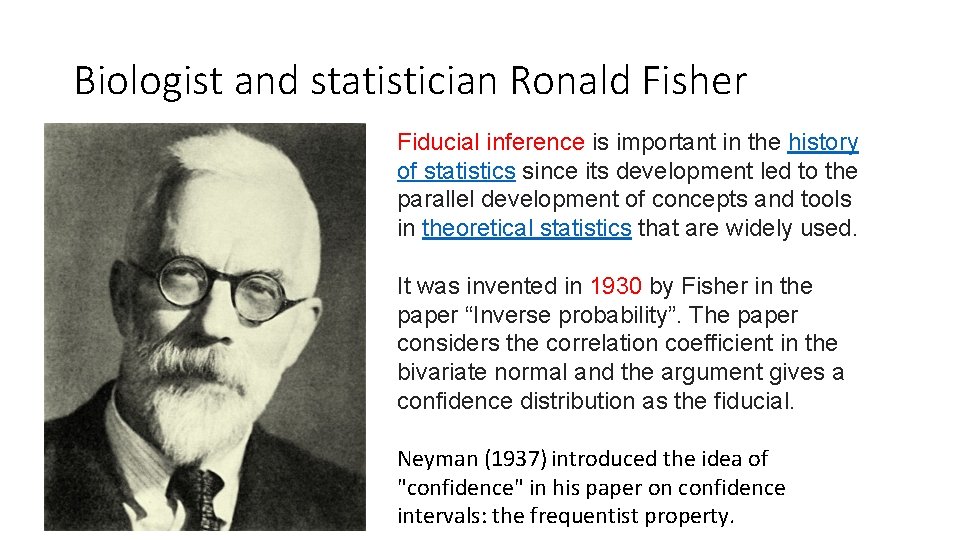
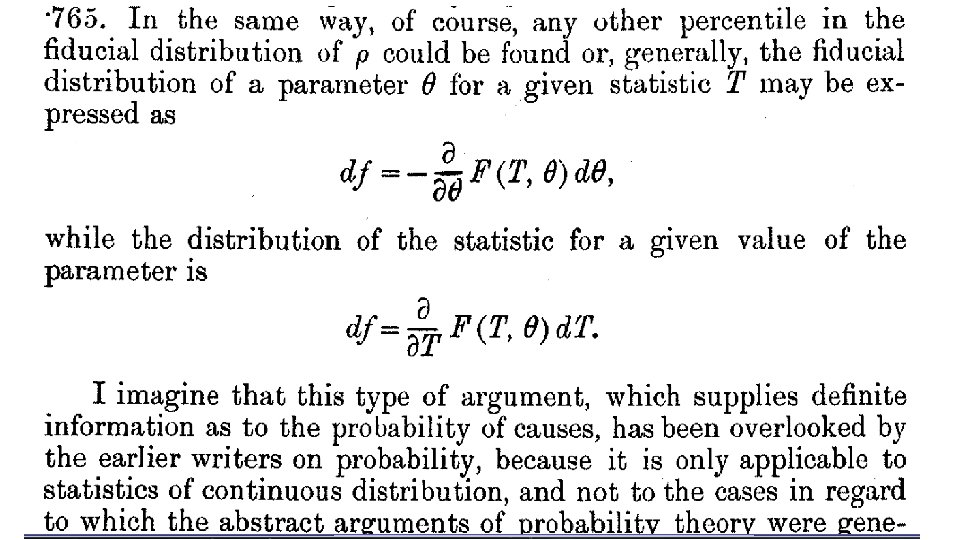
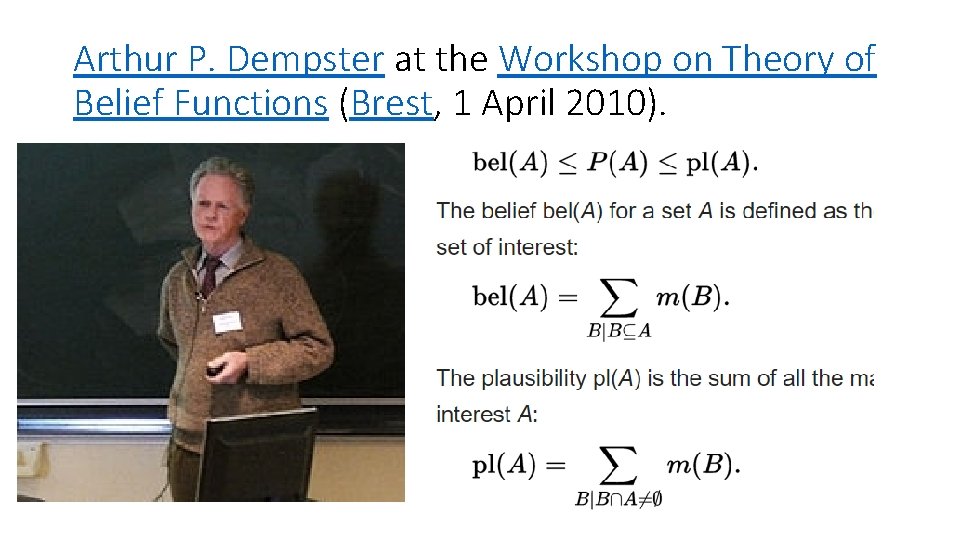
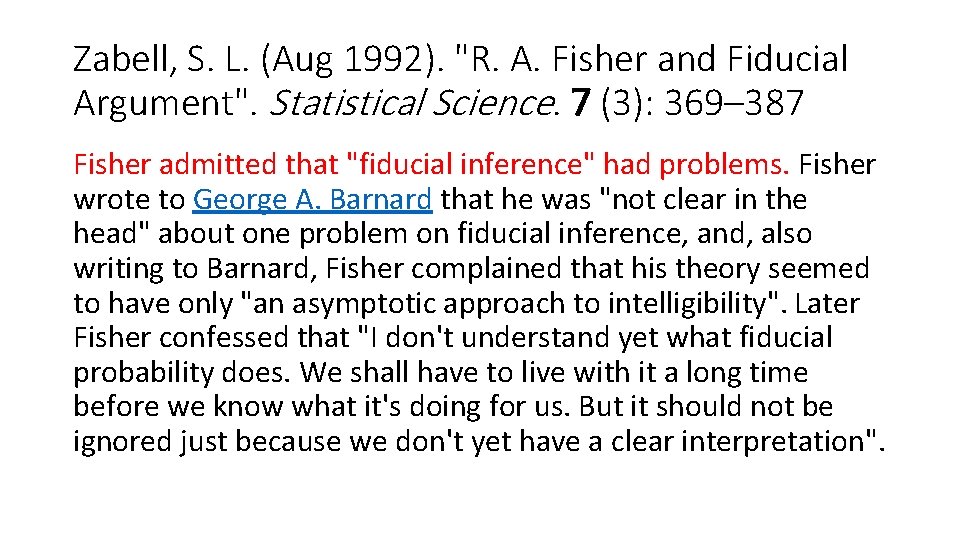
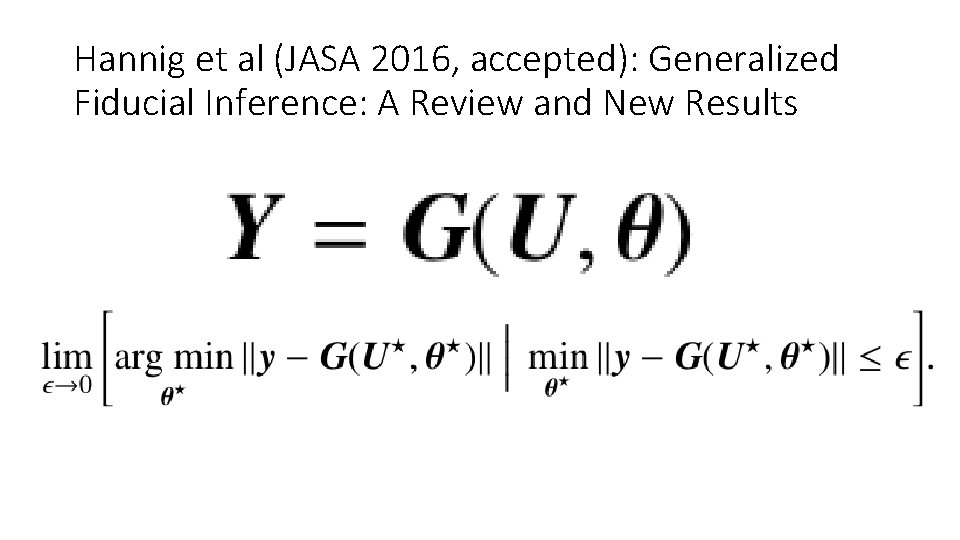
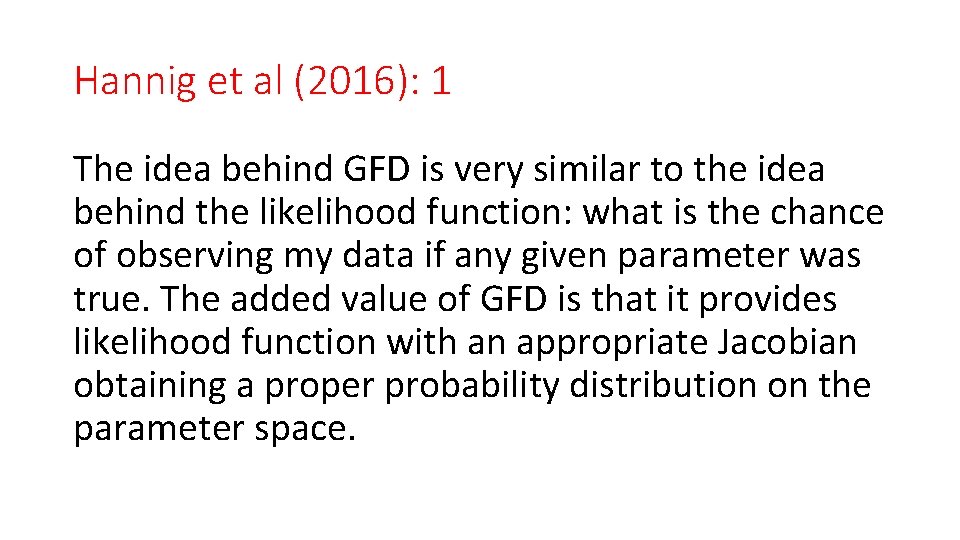
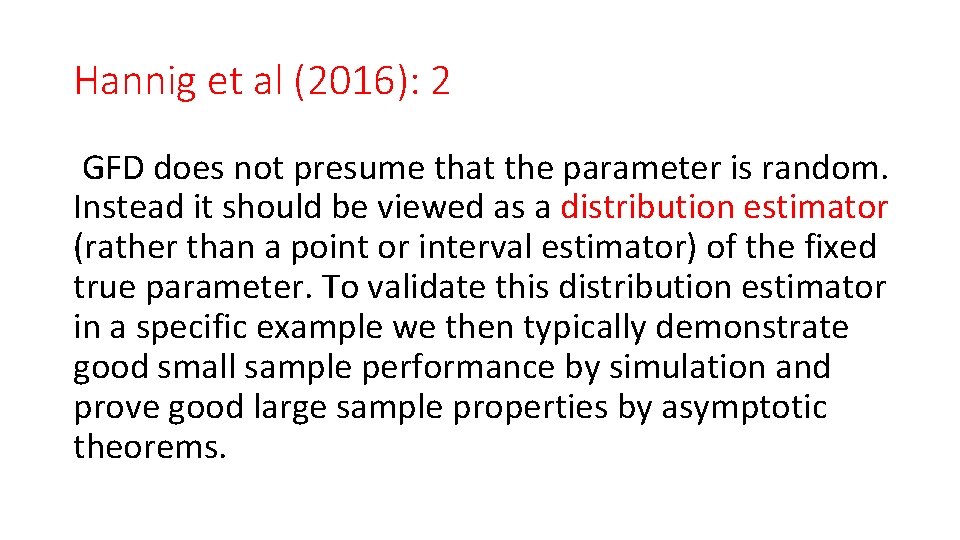
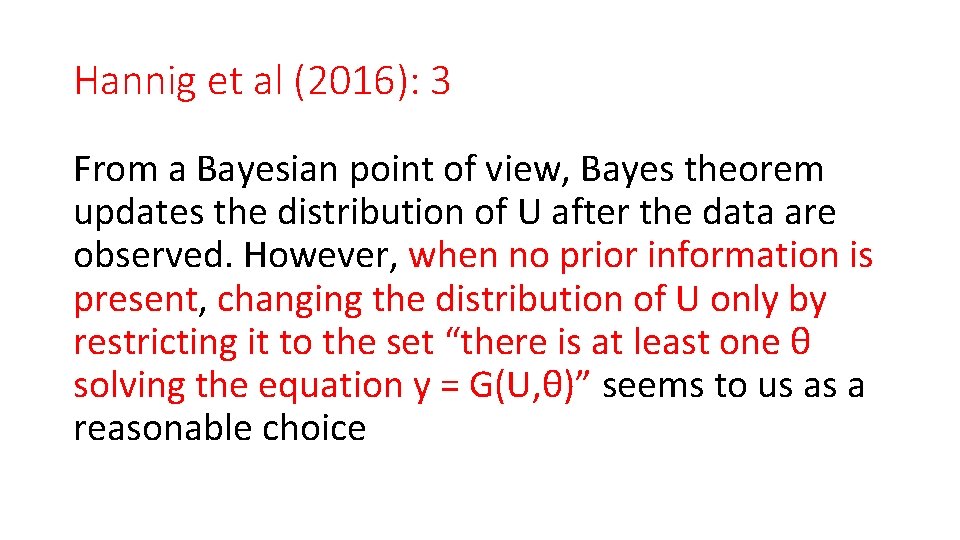
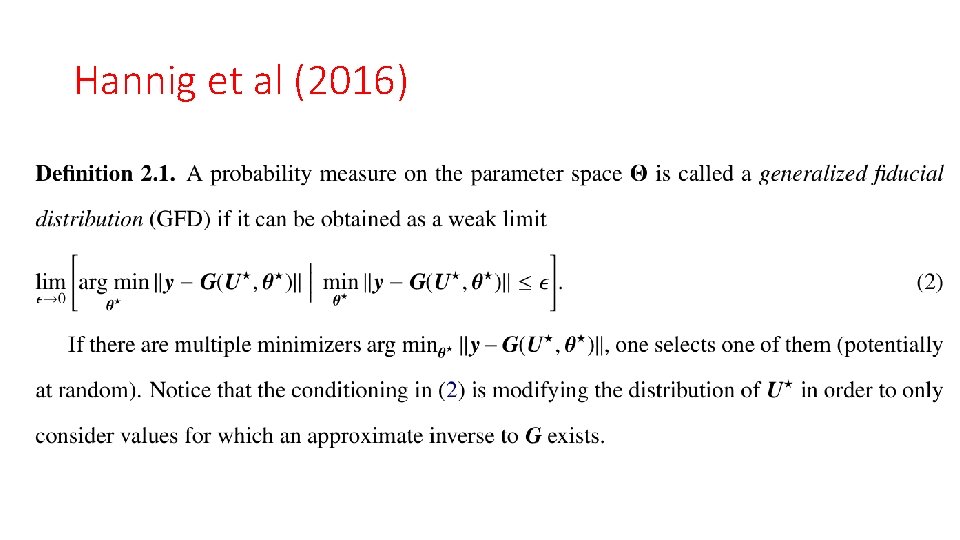
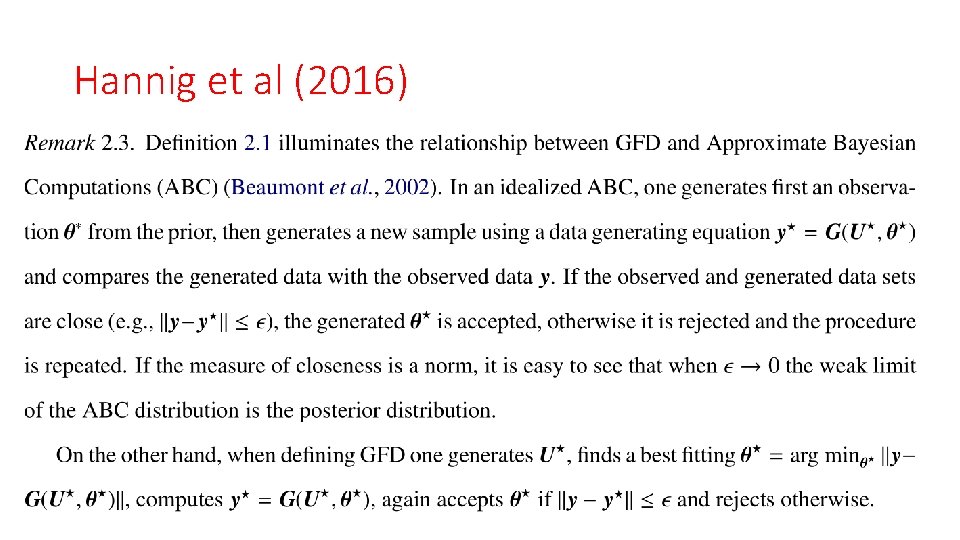
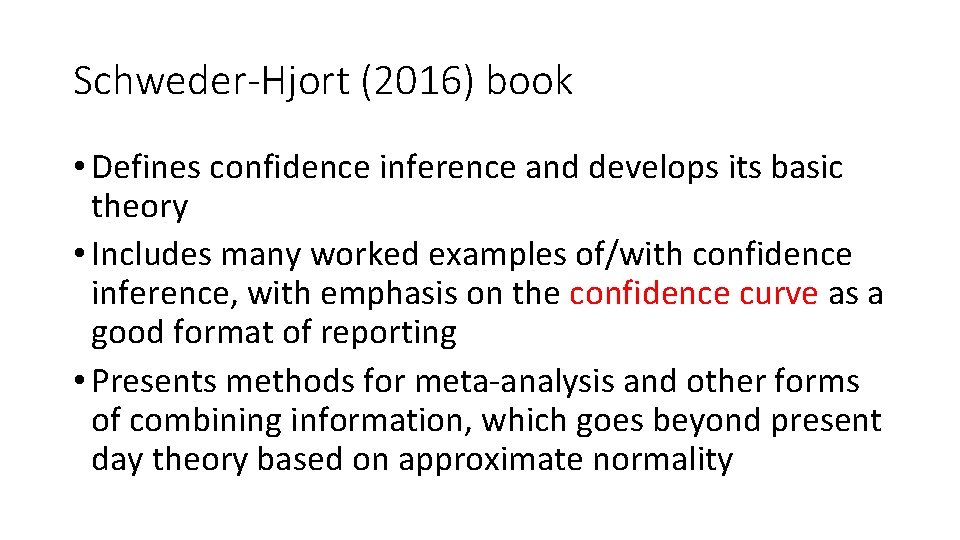
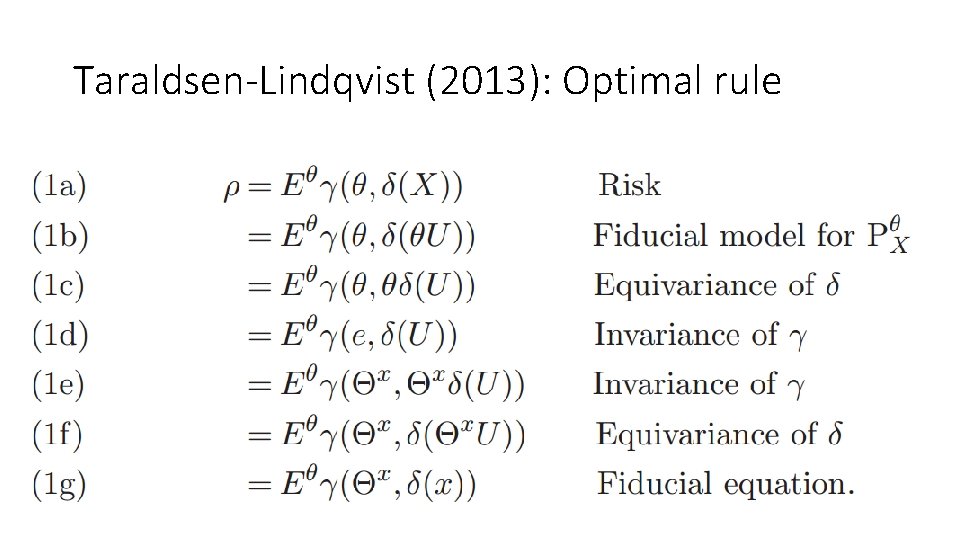
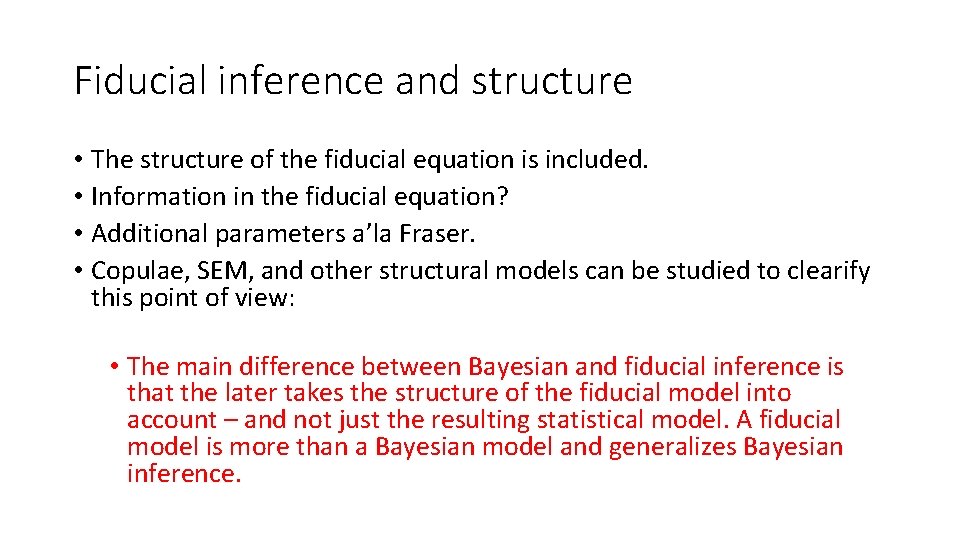
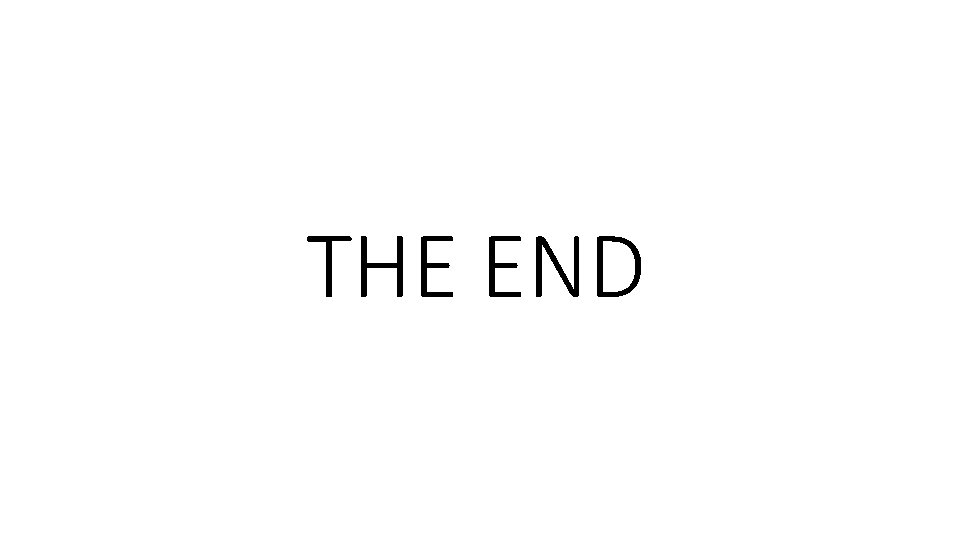
- Slides: 22
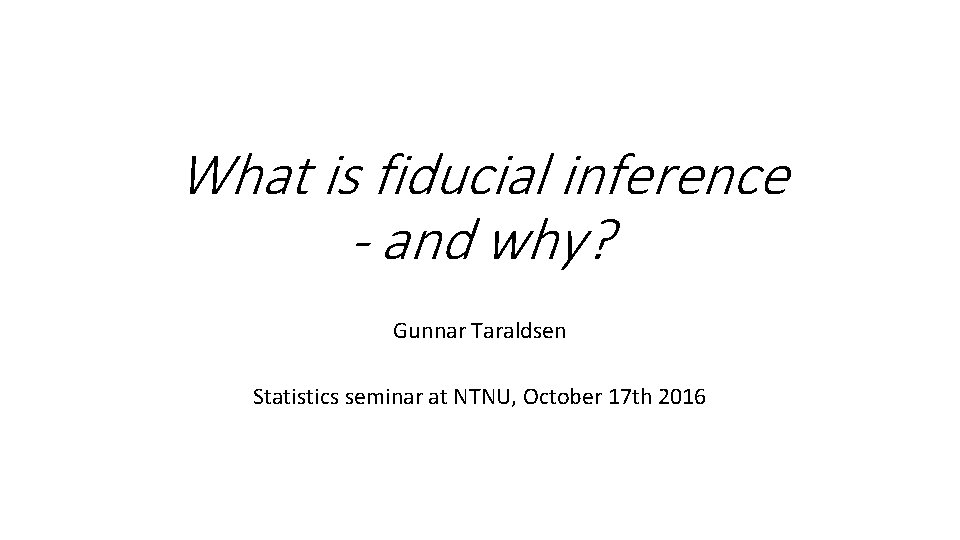
What is fiducial inference - and why? Gunnar Taraldsen Statistics seminar at NTNU, October 17 th 2016
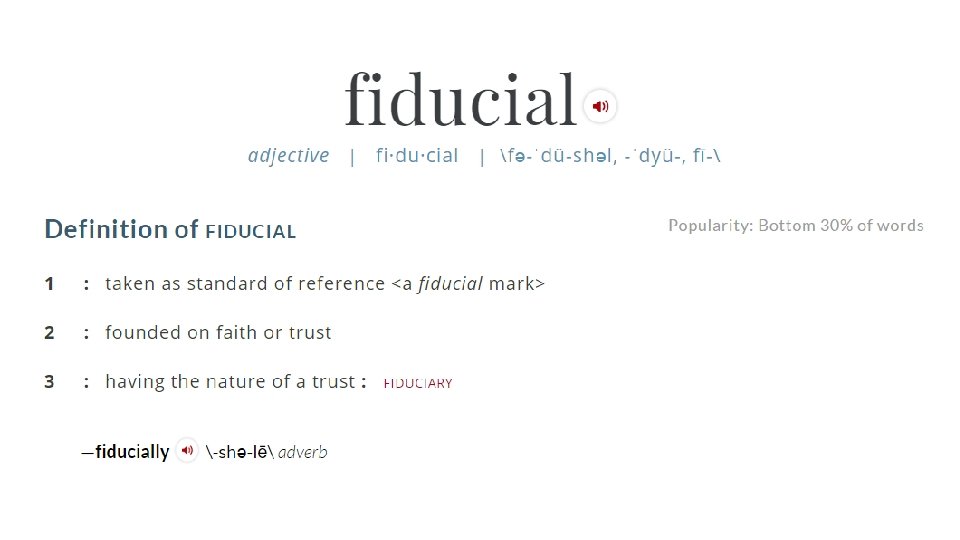
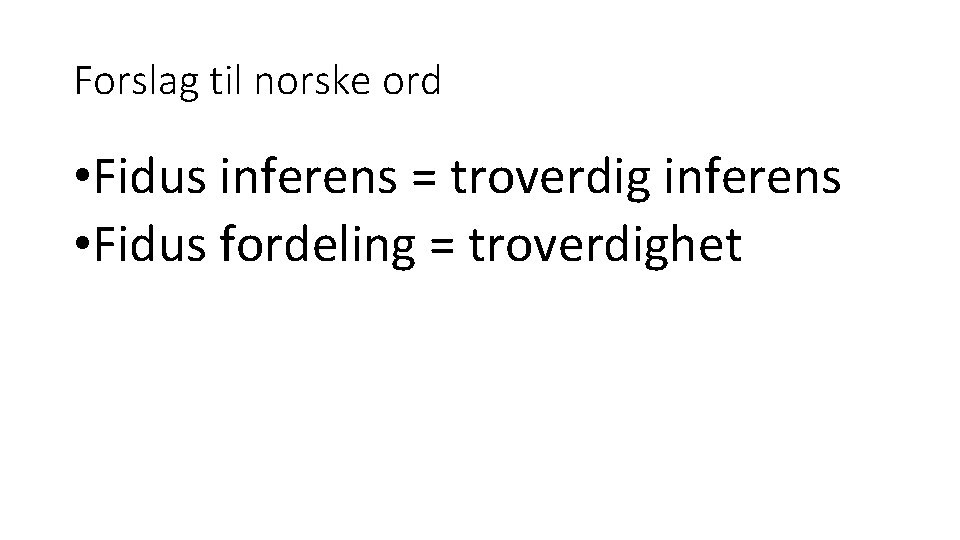
Forslag til norske ord • Fidus inferens = troverdig inferens • Fidus fordeling = troverdighet
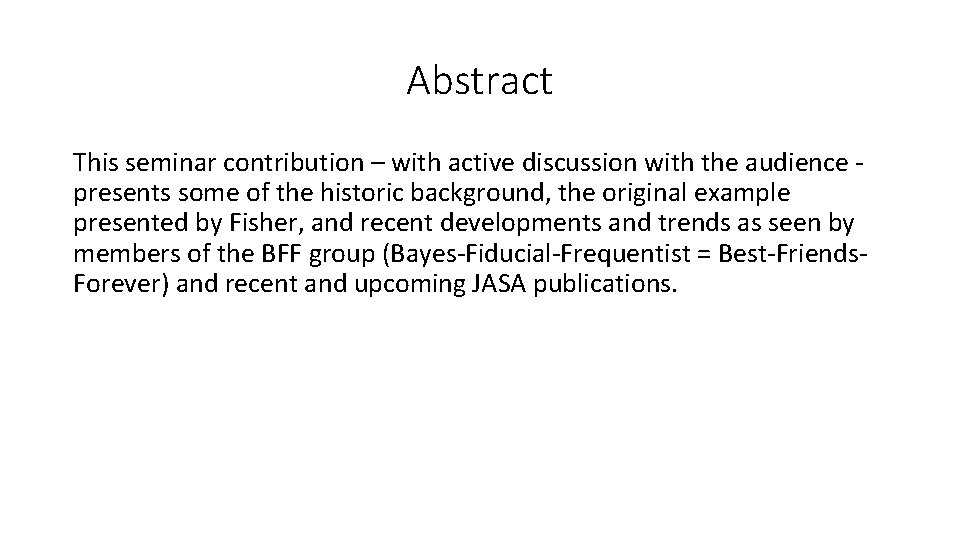
Abstract This seminar contribution – with active discussion with the audience - presents some of the historic background, the original example presented by Fisher, and recent developments and trends as seen by members of the BFF group (Bayes-Fiducial-Frequentist = Best-Friends. Forever) and recent and upcoming JASA publications.
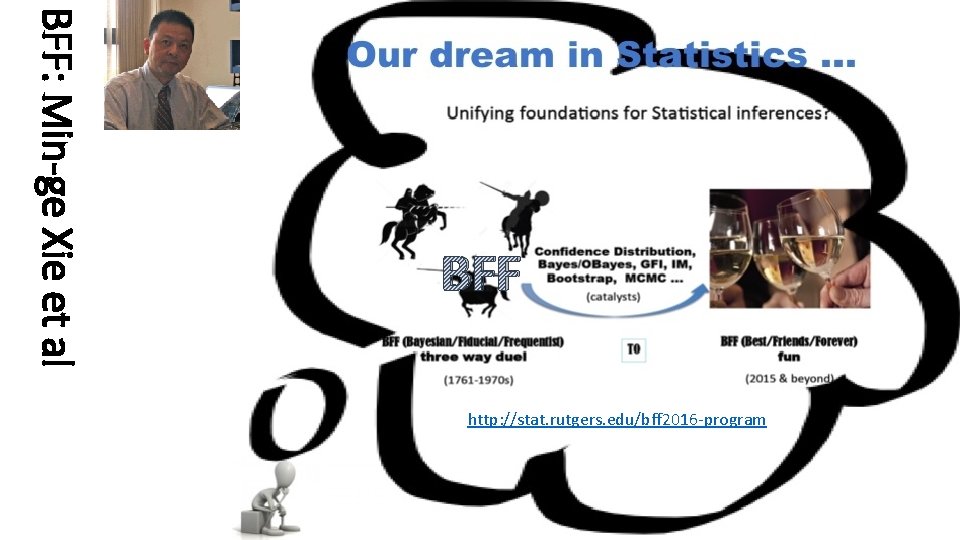
BFF: Min-ge Xie et al BFF http: //stat. rutgers. edu/bff 2016 -program
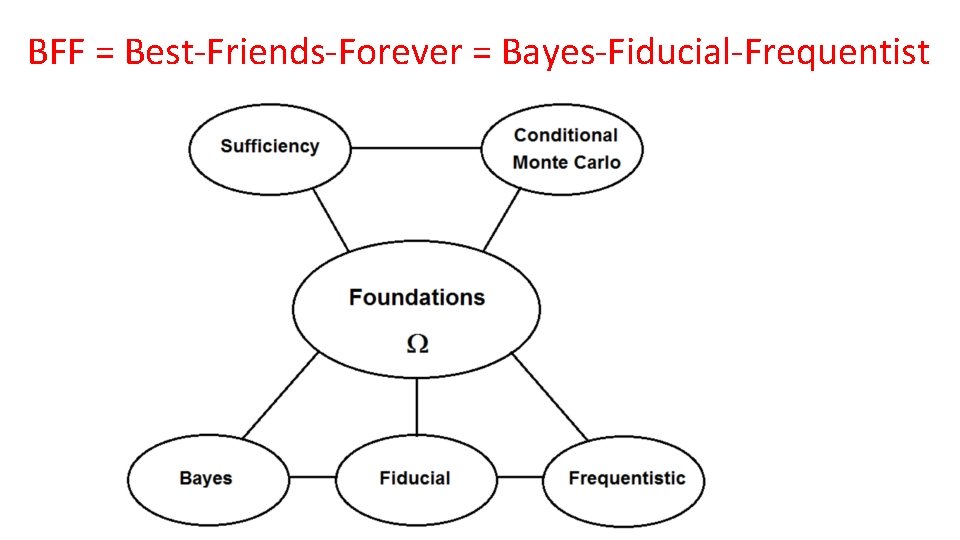
BFF = Best-Friends-Forever = Bayes-Fiducial-Frequentist
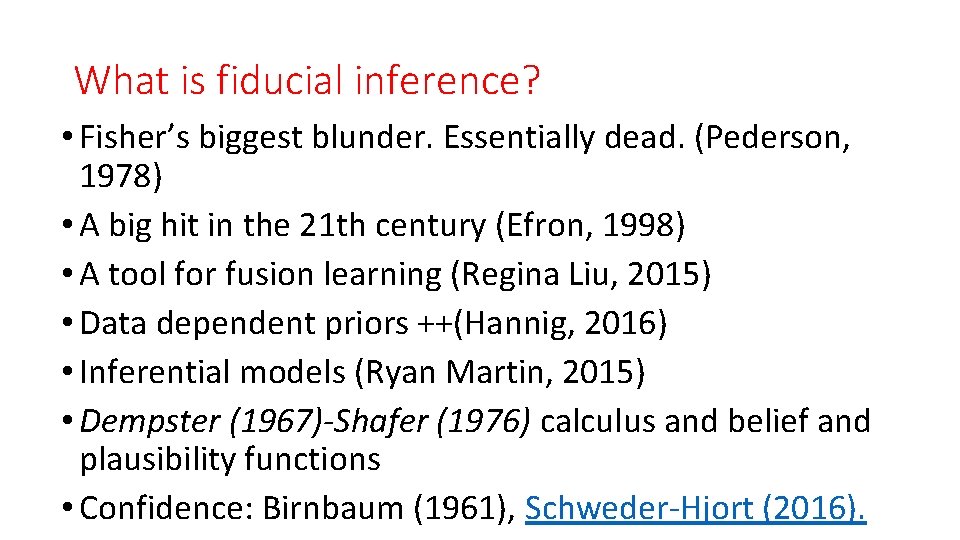
What is fiducial inference? • Fisher’s biggest blunder. Essentially dead. (Pederson, 1978) • A big hit in the 21 th century (Efron, 1998) • A tool for fusion learning (Regina Liu, 2015) • Data dependent priors ++(Hannig, 2016) • Inferential models (Ryan Martin, 2015) • Dempster (1967)-Shafer (1976) calculus and belief and plausibility functions • Confidence: Birnbaum (1961), Schweder-Hjort (2016).
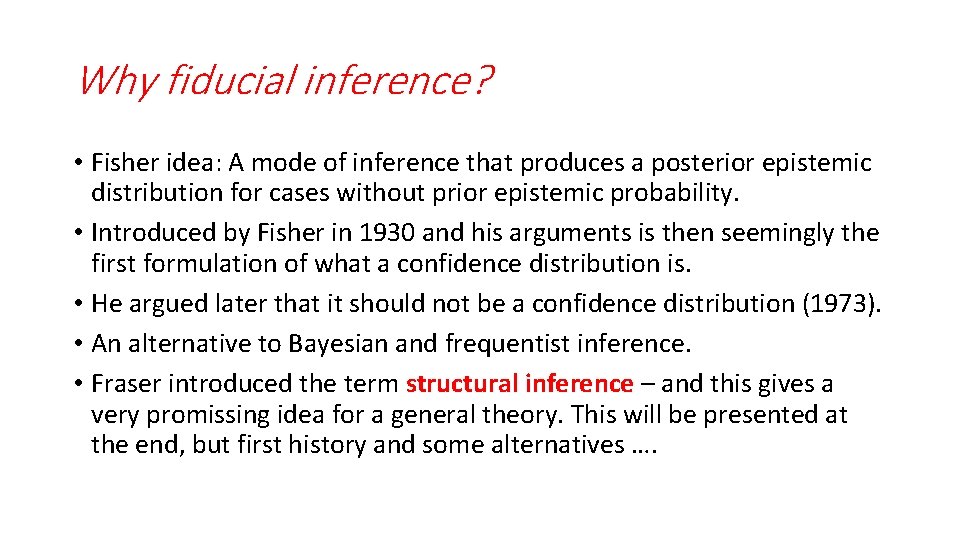
Why fiducial inference? • Fisher idea: A mode of inference that produces a posterior epistemic distribution for cases without prior epistemic probability. • Introduced by Fisher in 1930 and his arguments is then seemingly the first formulation of what a confidence distribution is. • He argued later that it should not be a confidence distribution (1973). • An alternative to Bayesian and frequentist inference. • Fraser introduced the term structural inference – and this gives a very promissing idea for a general theory. This will be presented at the end, but first history and some alternatives ….
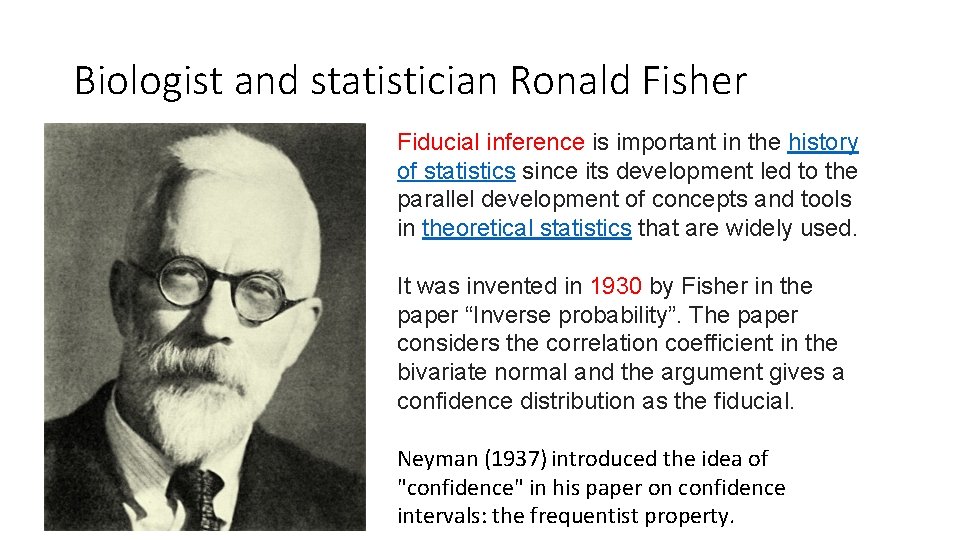
Biologist and statistician Ronald Fisher Fiducial inference is important in the history of statistics since its development led to the parallel development of concepts and tools in theoretical statistics that are widely used. It was invented in 1930 by Fisher in the paper “Inverse probability”. The paper considers the correlation coefficient in the bivariate normal and the argument gives a confidence distribution as the fiducial. Neyman (1937) introduced the idea of "confidence" in his paper on confidence intervals: the frequentist property.
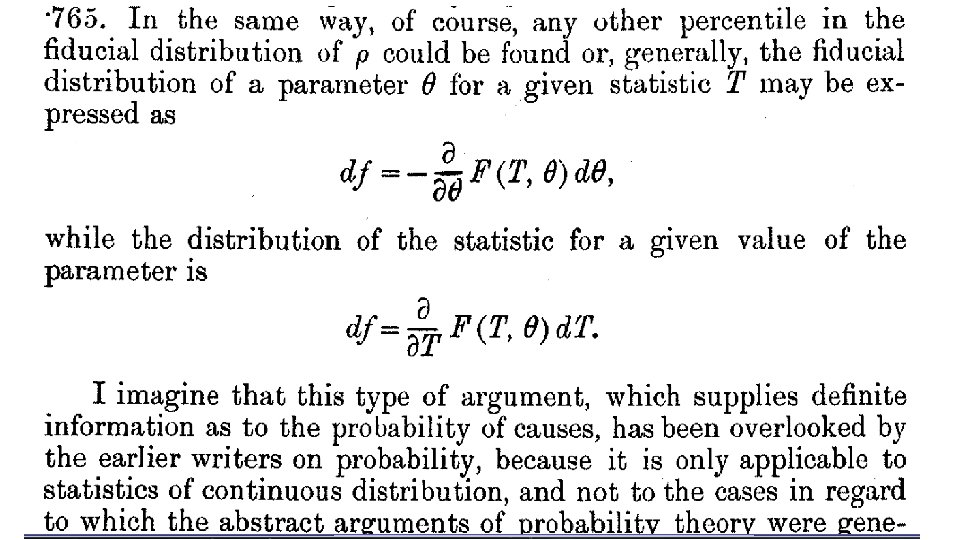
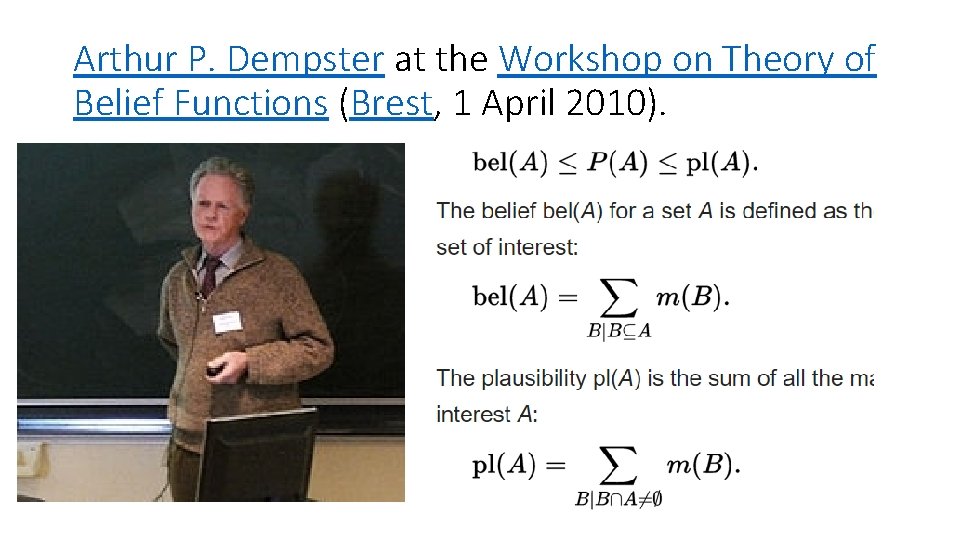
Arthur P. Dempster at the Workshop on Theory of Belief Functions (Brest, 1 April 2010).
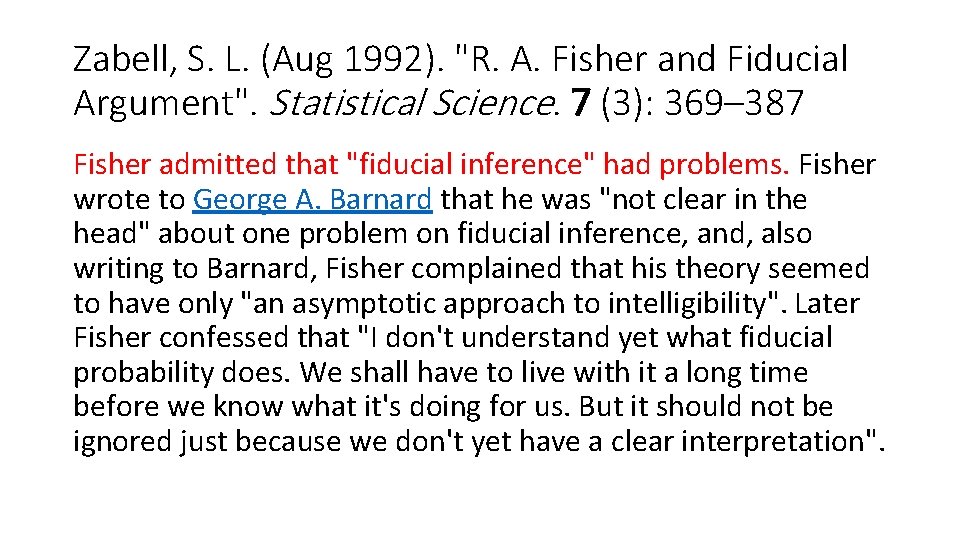
Zabell, S. L. (Aug 1992). "R. A. Fisher and Fiducial Argument". Statistical Science. 7 (3): 369– 387 Fisher admitted that "fiducial inference" had problems. Fisher wrote to George A. Barnard that he was "not clear in the head" about one problem on fiducial inference, and, also writing to Barnard, Fisher complained that his theory seemed to have only "an asymptotic approach to intelligibility". Later Fisher confessed that "I don't understand yet what fiducial probability does. We shall have to live with it a long time before we know what it's doing for us. But it should not be ignored just because we don't yet have a clear interpretation".
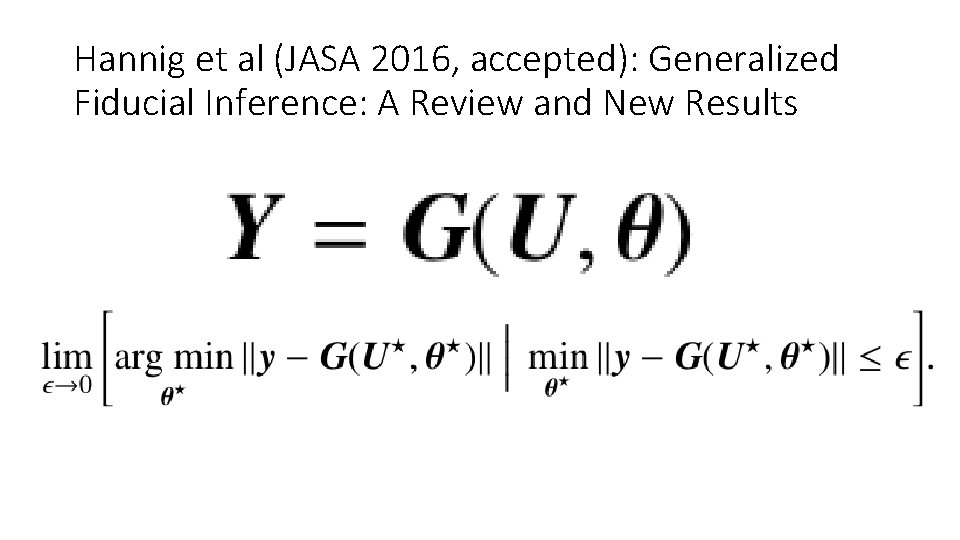
Hannig et al (JASA 2016, accepted): Generalized Fiducial Inference: A Review and New Results
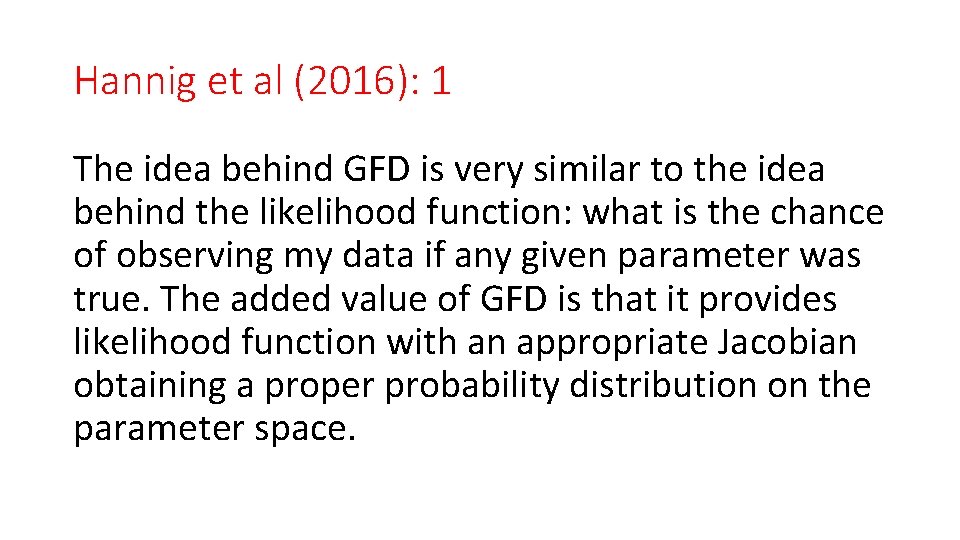
Hannig et al (2016): 1 The idea behind GFD is very similar to the idea behind the likelihood function: what is the chance of observing my data if any given parameter was true. The added value of GFD is that it provides likelihood function with an appropriate Jacobian obtaining a proper probability distribution on the parameter space.
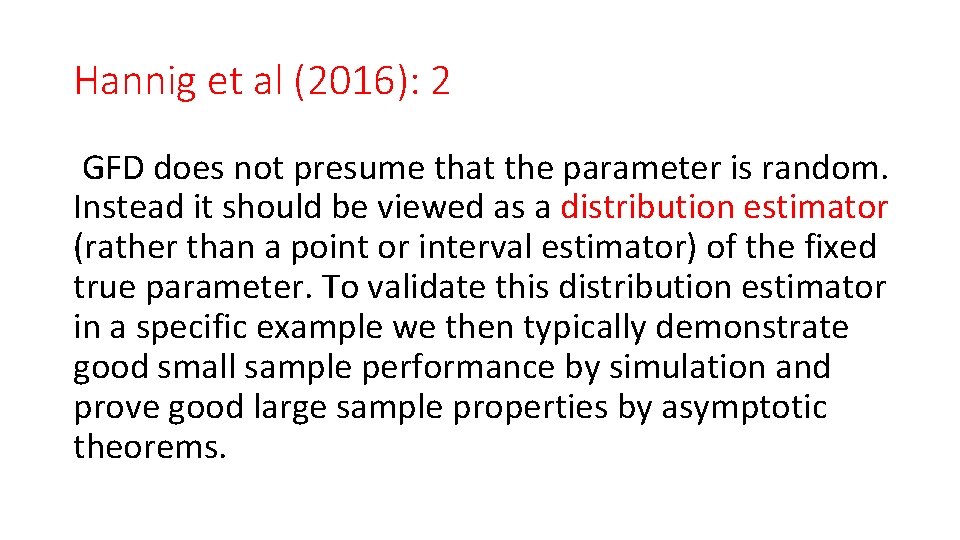
Hannig et al (2016): 2 GFD does not presume that the parameter is random. Instead it should be viewed as a distribution estimator (rather than a point or interval estimator) of the fixed true parameter. To validate this distribution estimator in a specific example we then typically demonstrate good small sample performance by simulation and prove good large sample properties by asymptotic theorems.
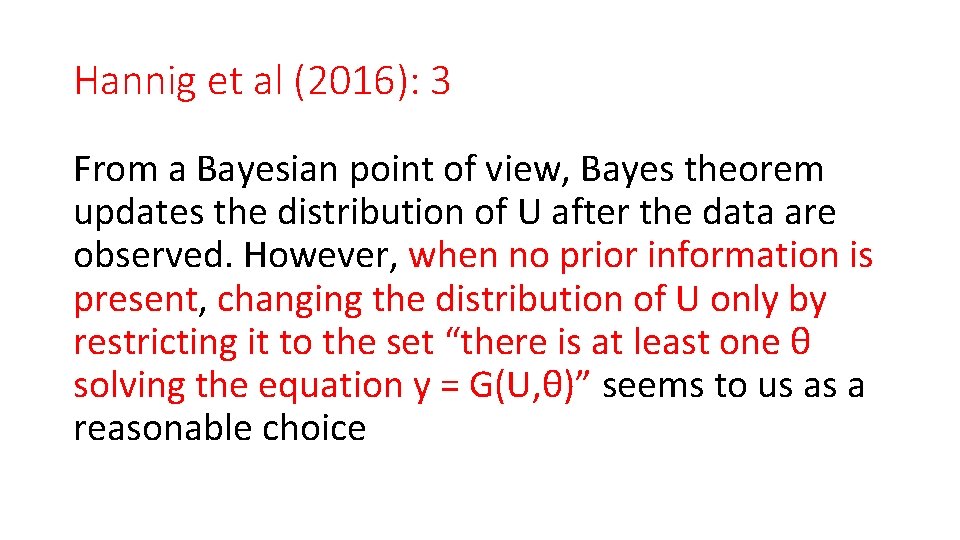
Hannig et al (2016): 3 From a Bayesian point of view, Bayes theorem updates the distribution of U after the data are observed. However, when no prior information is present, changing the distribution of U only by restricting it to the set “there is at least one θ solving the equation y = G(U, θ)” seems to us as a reasonable choice
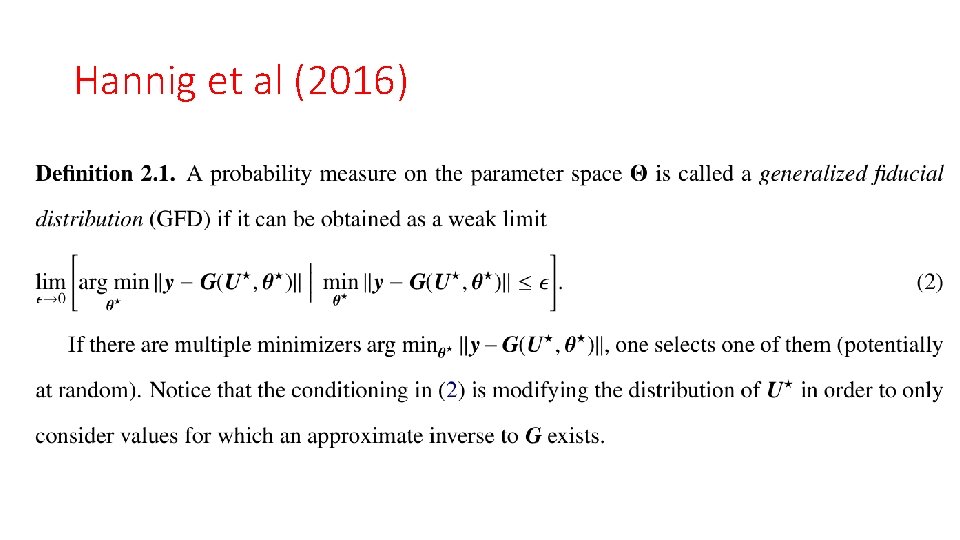
Hannig et al (2016)
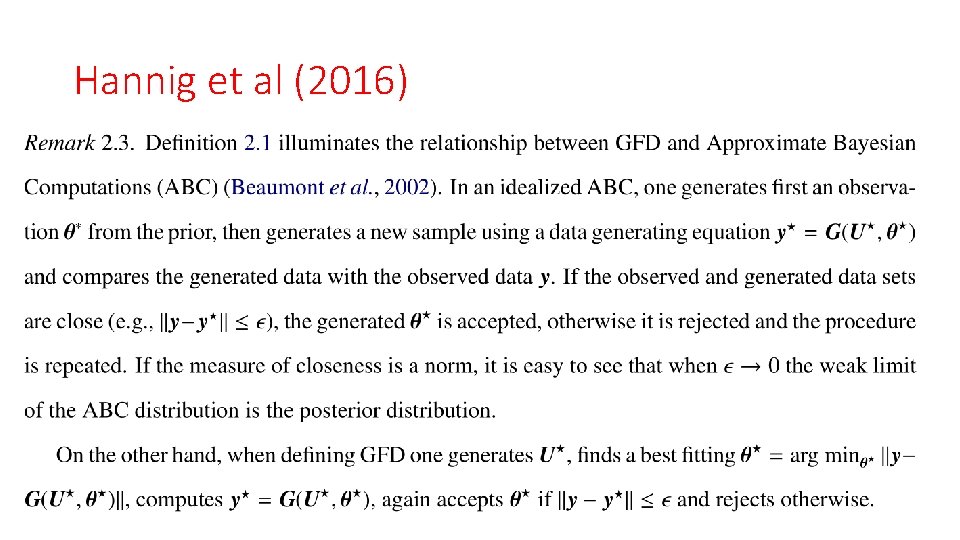
Hannig et al (2016)
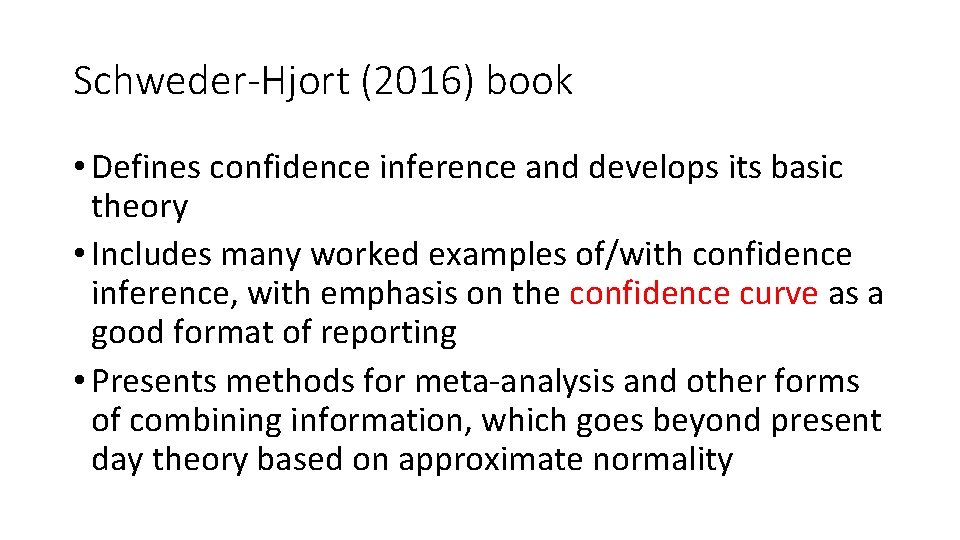
Schweder-Hjort (2016) book • Defines confidence inference and develops its basic theory • Includes many worked examples of/with confidence inference, with emphasis on the confidence curve as a good format of reporting • Presents methods for meta-analysis and other forms of combining information, which goes beyond present day theory based on approximate normality
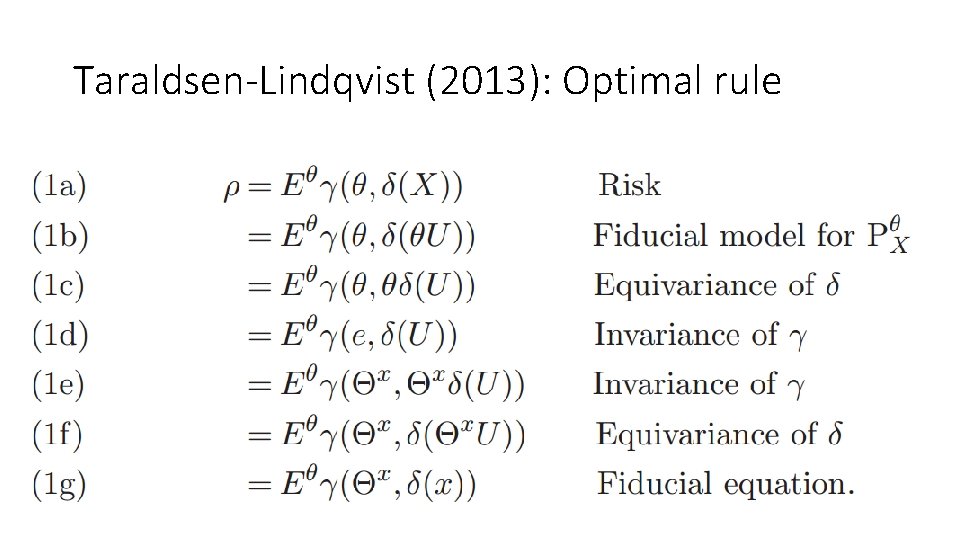
Taraldsen-Lindqvist (2013): Optimal rule
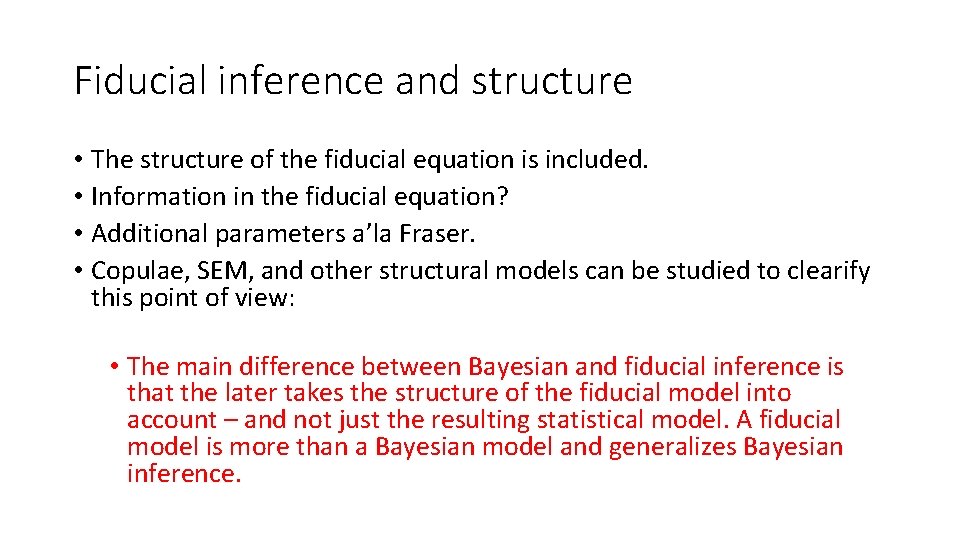
Fiducial inference and structure • The structure of the fiducial equation is included. • Information in the fiducial equation? • Additional parameters a’la Fraser. • Copulae, SEM, and other structural models can be studied to clearify this point of view: • The main difference between Bayesian and fiducial inference is that the later takes the structure of the fiducial model into account – and not just the resulting statistical model. A fiducial model is more than a Bayesian model and generalizes Bayesian inference.
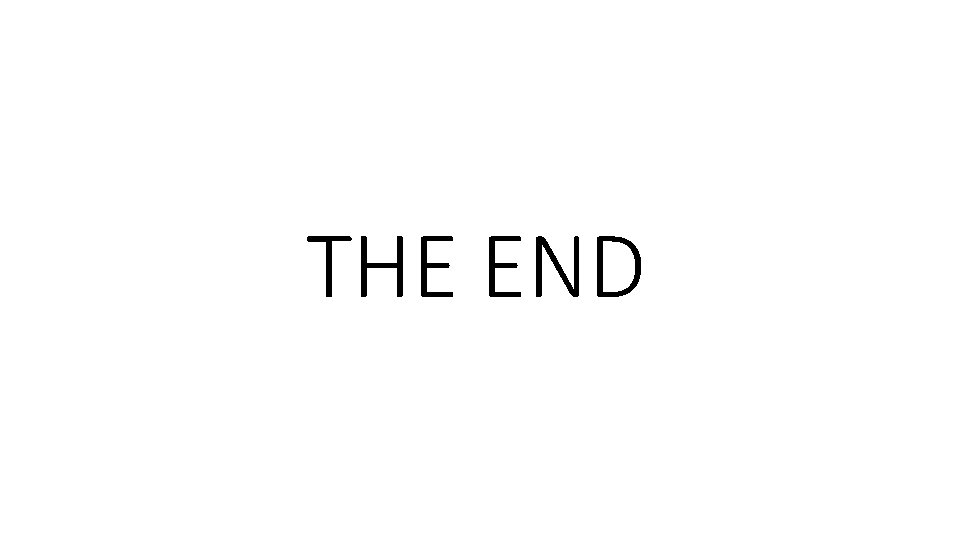
THE END
Fiducial inference
Pictures
Geometry of an aerial photograph
8 fiducial
Fiducial points
Dont ask why why why
Gunnar auðólfsson
Gunnar auðólfsson
Gunnar tobin
Gunnar mohr
Gunnar stetler
Gunnar sandberg
Gunnar nellis
Gunnar vaht
Gunnar kahlmeter
Gunnar bohman
Somatomotorisk
Gunnar reinapu
Gunnar selvik
Gunnar jeremias
Normativas de gunnar mendoza
Gunnar ingelman
Gunnar thorarensen