Seize The Data IDOL Under the Hood Techniques
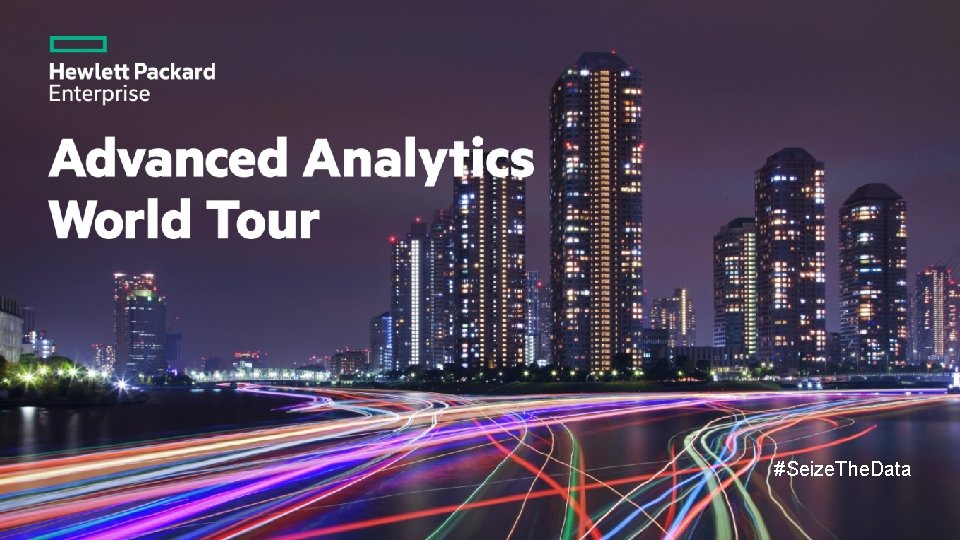
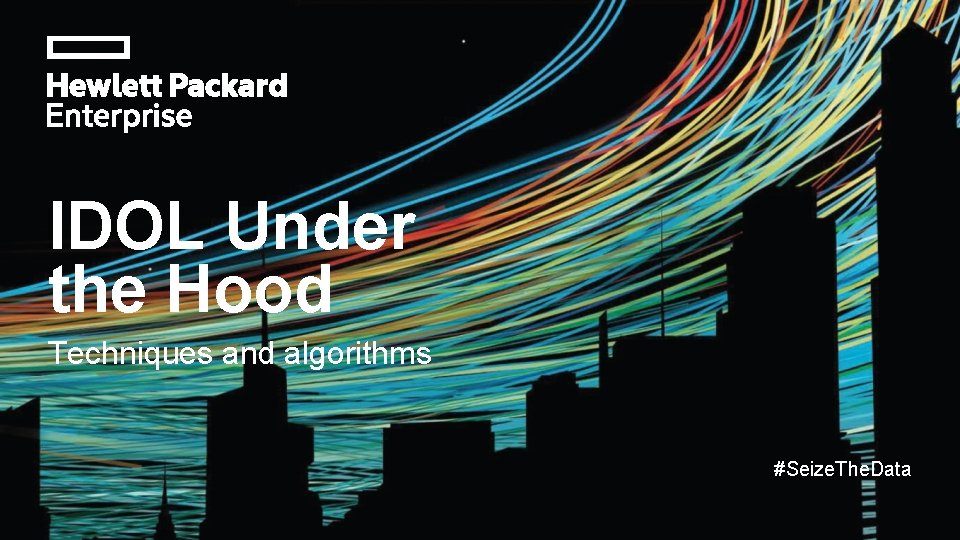
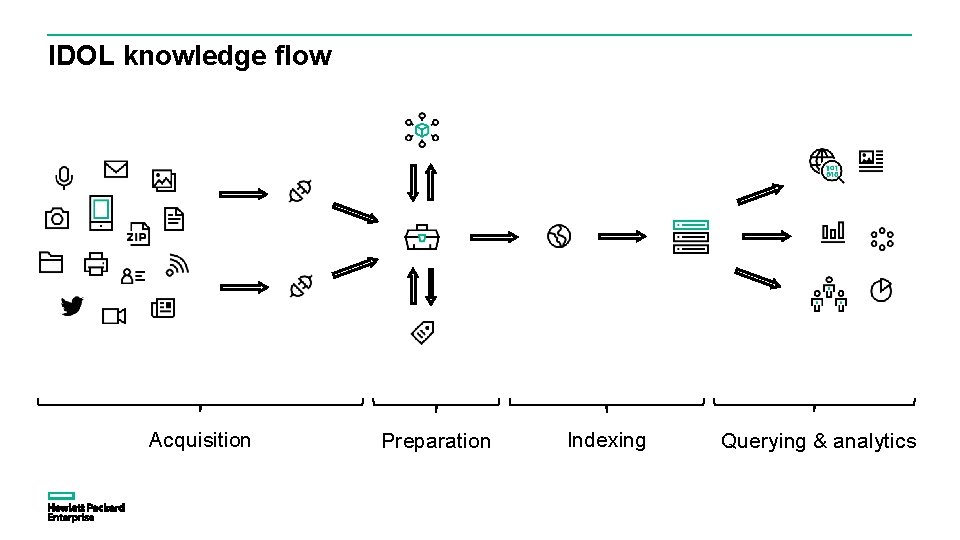
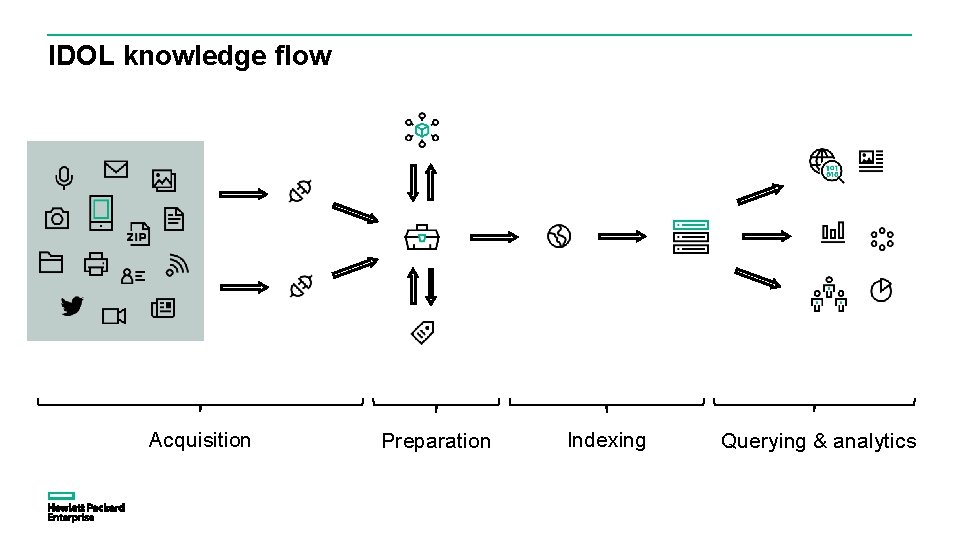
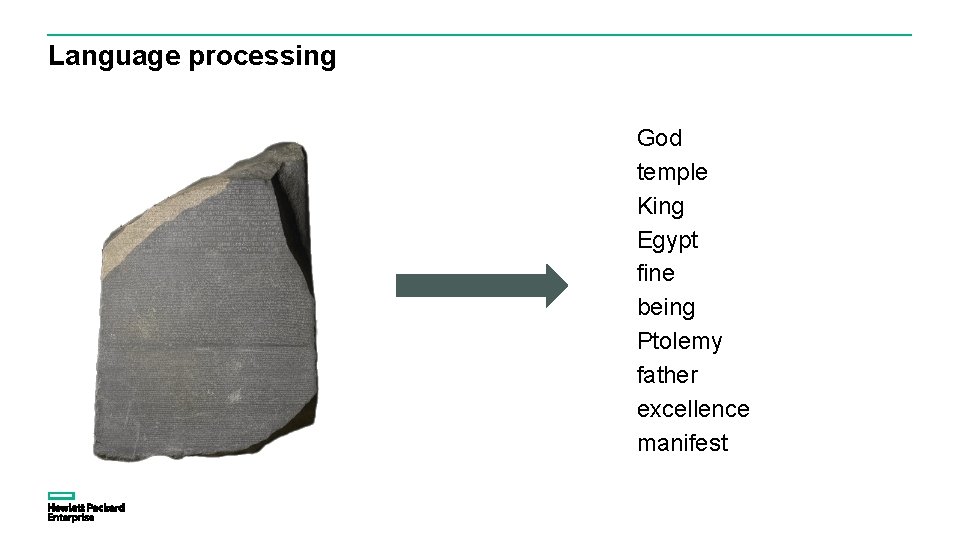
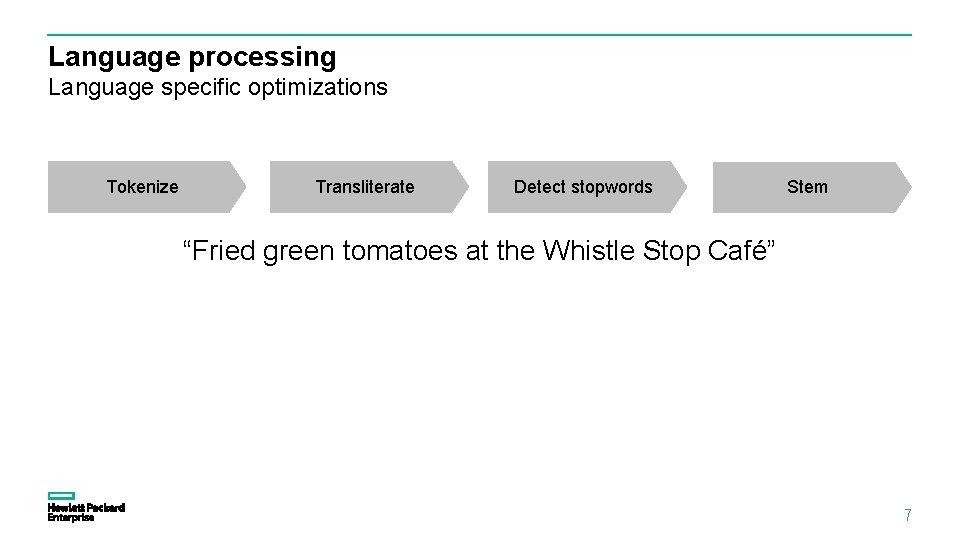
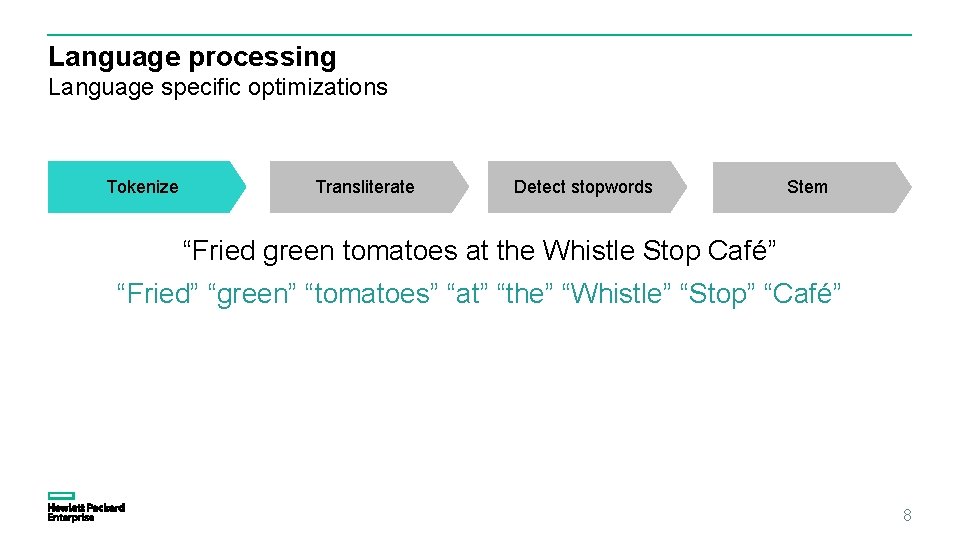
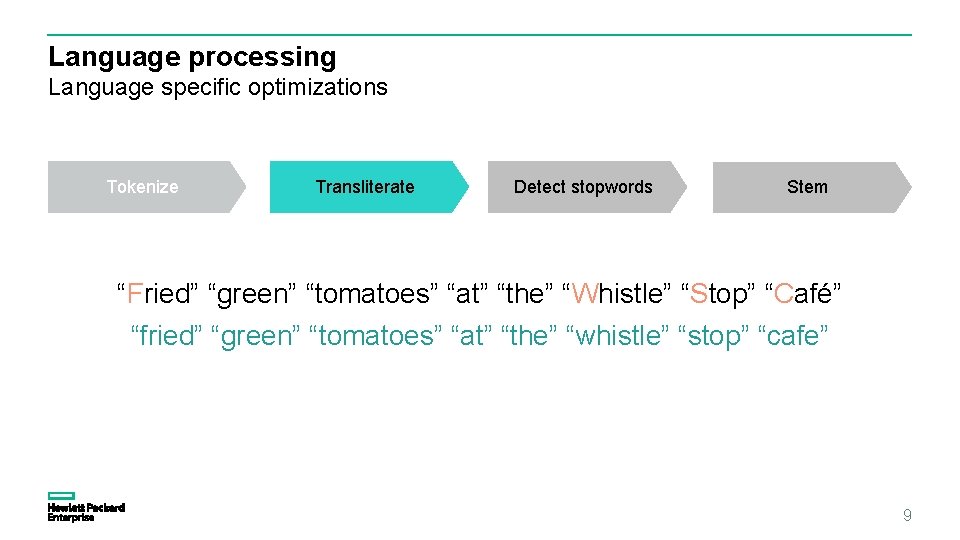
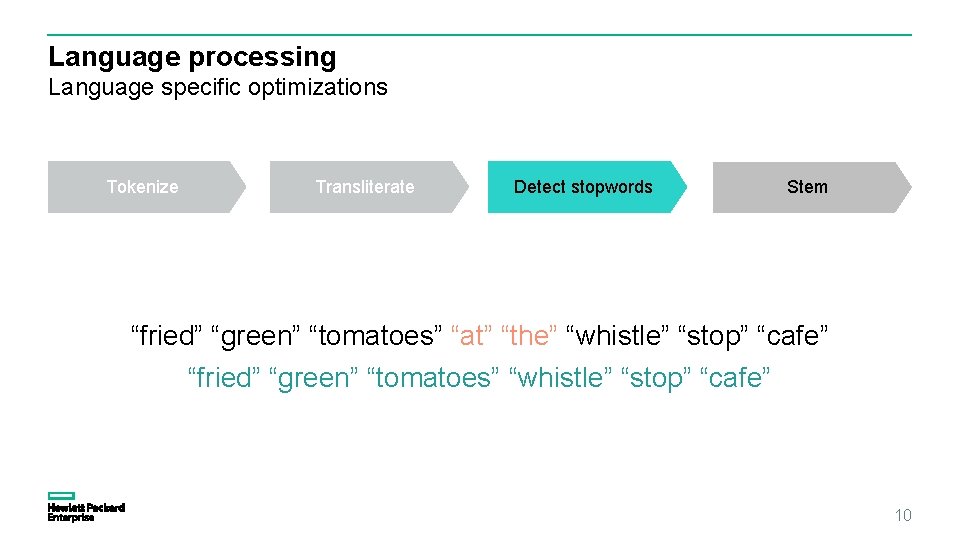
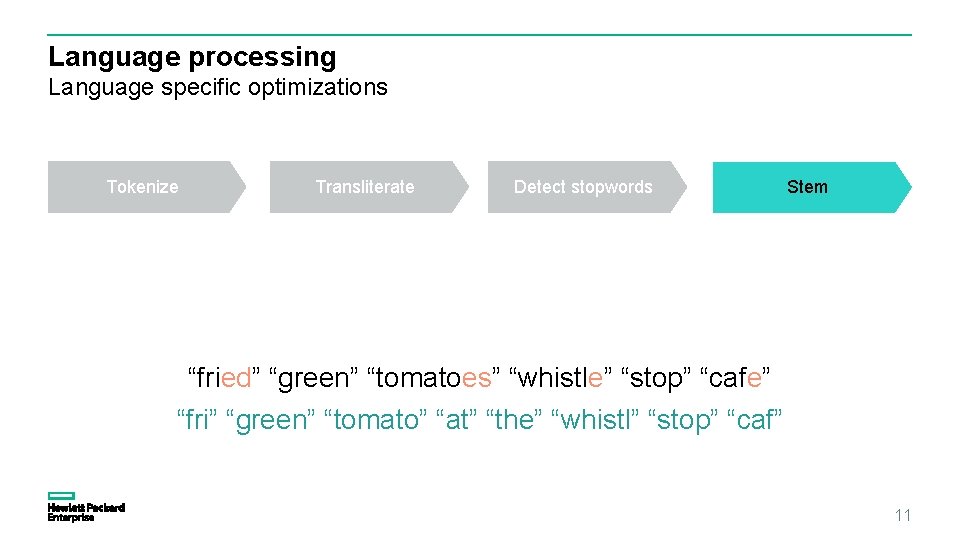
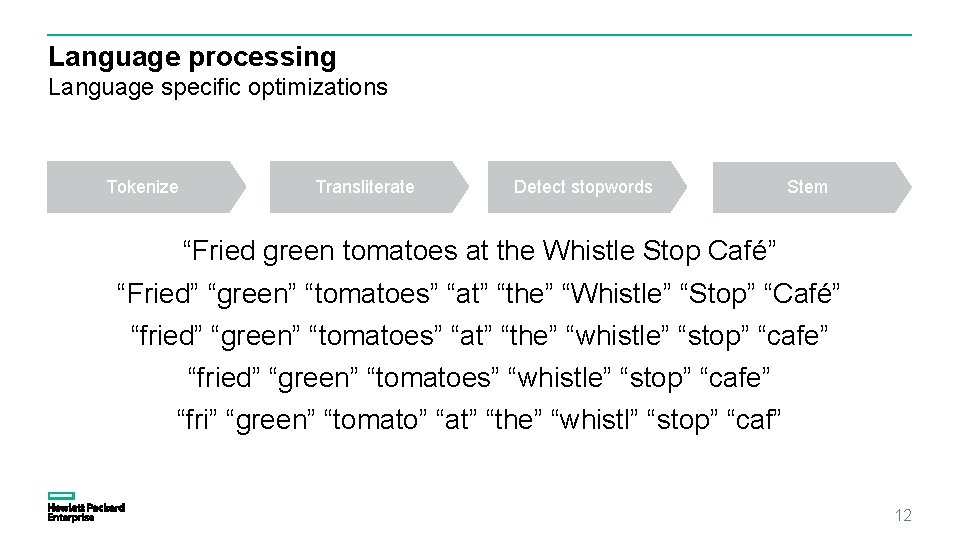
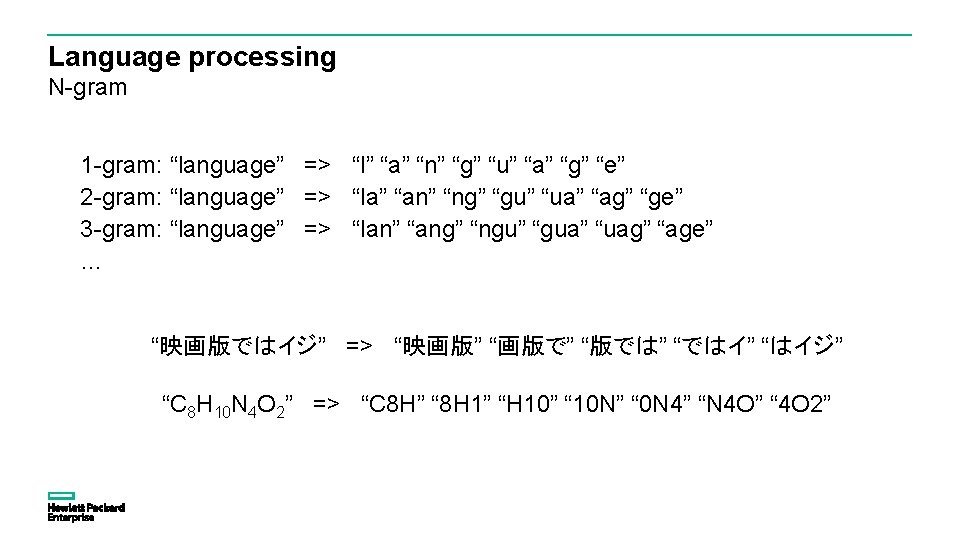
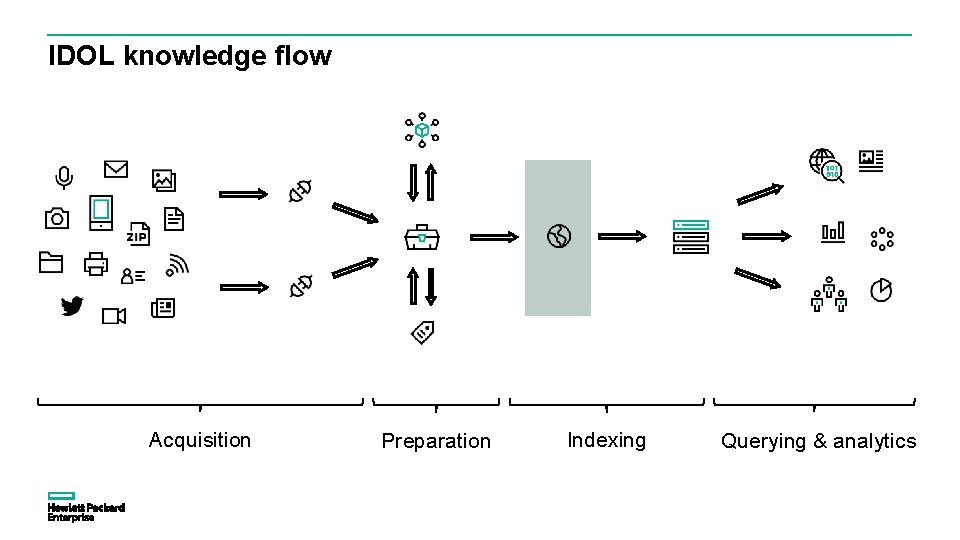
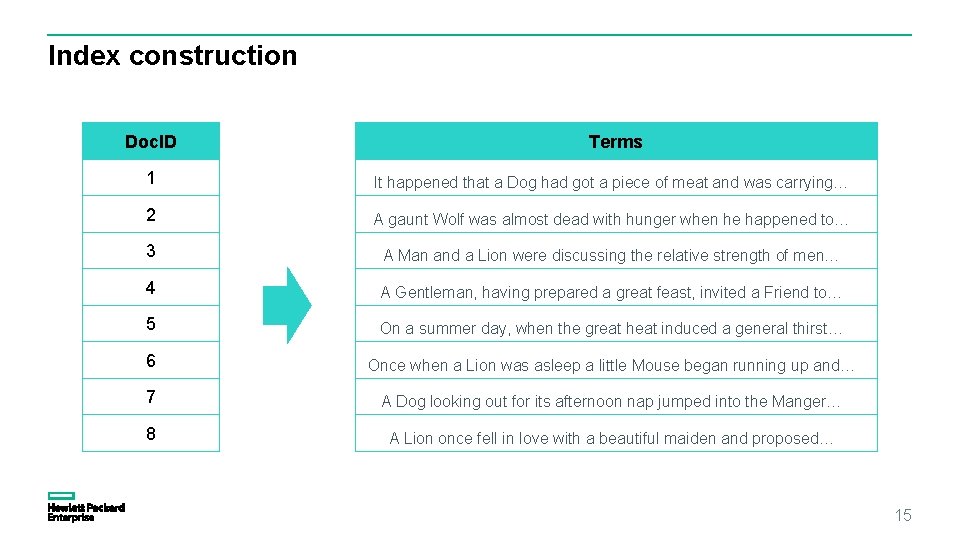
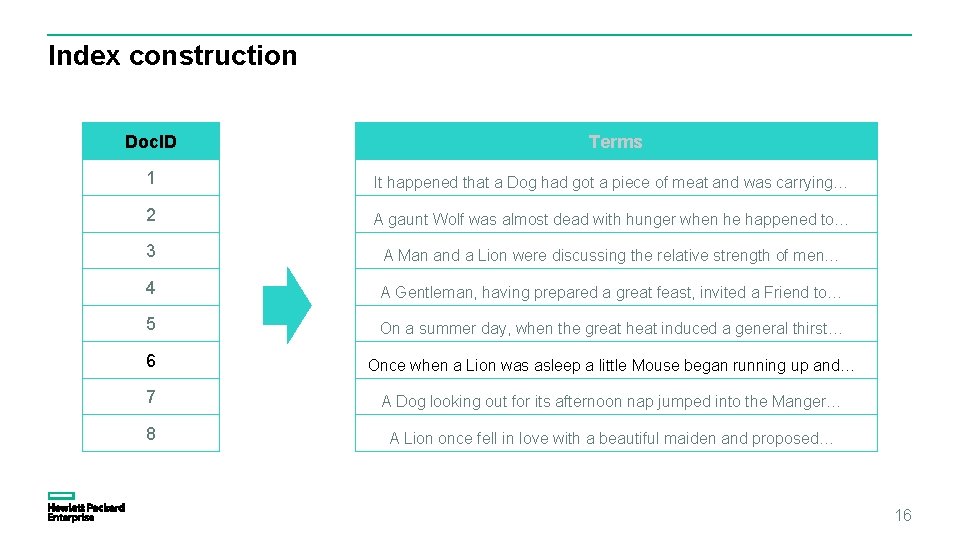
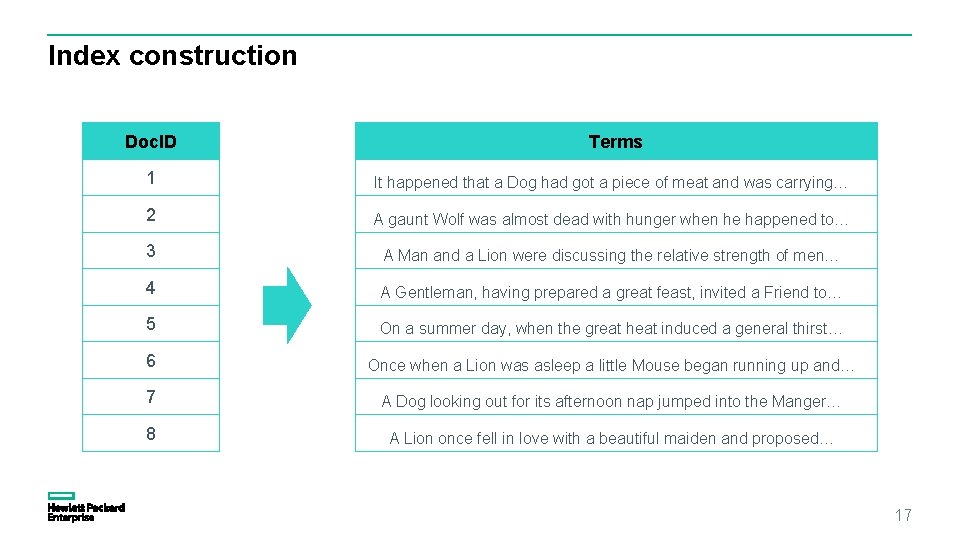
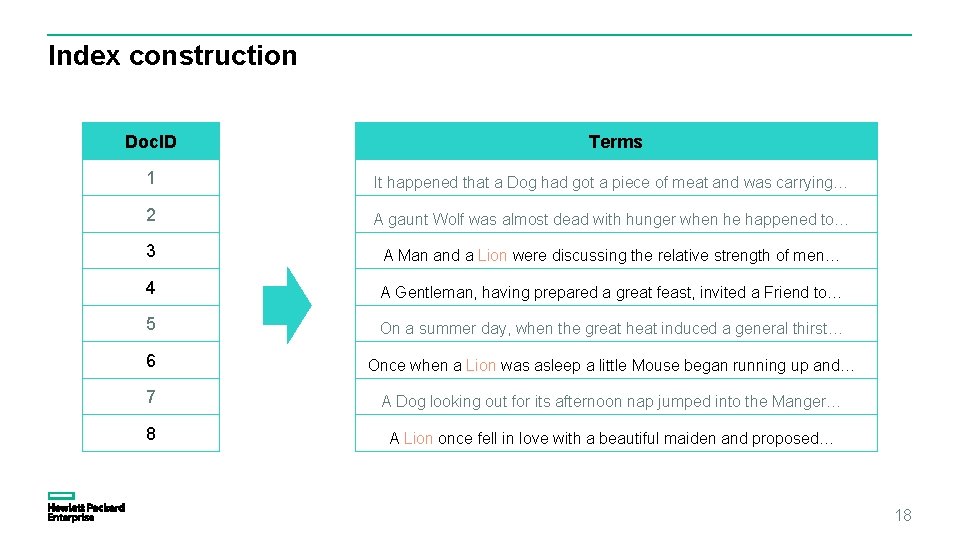
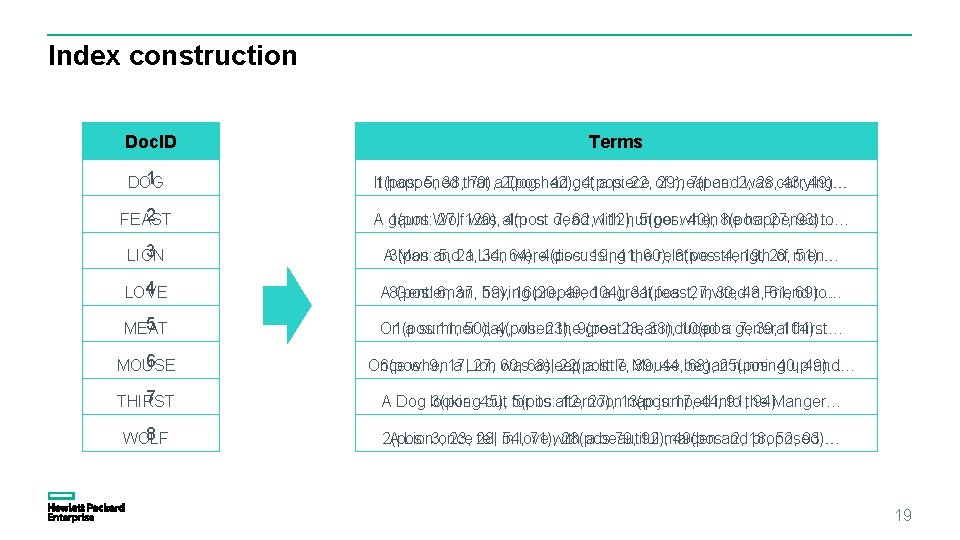
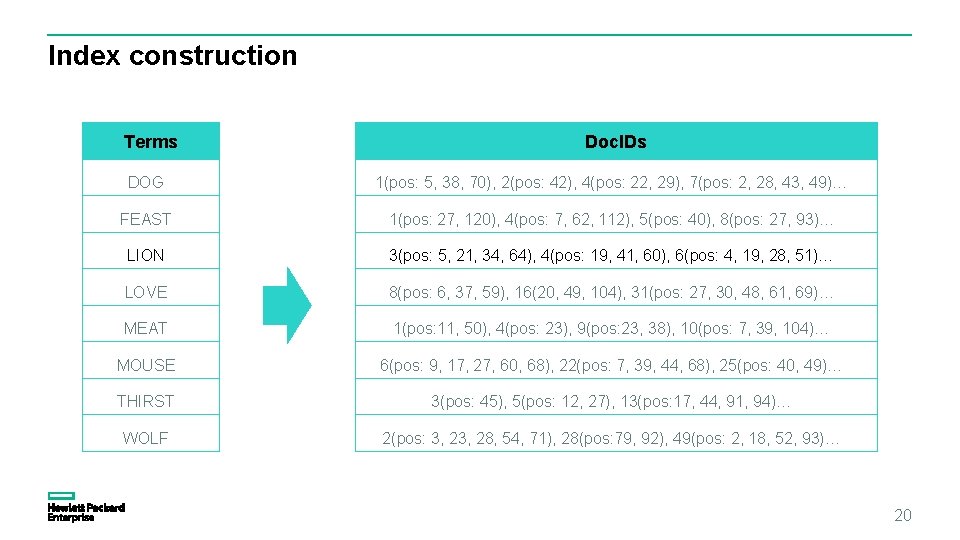
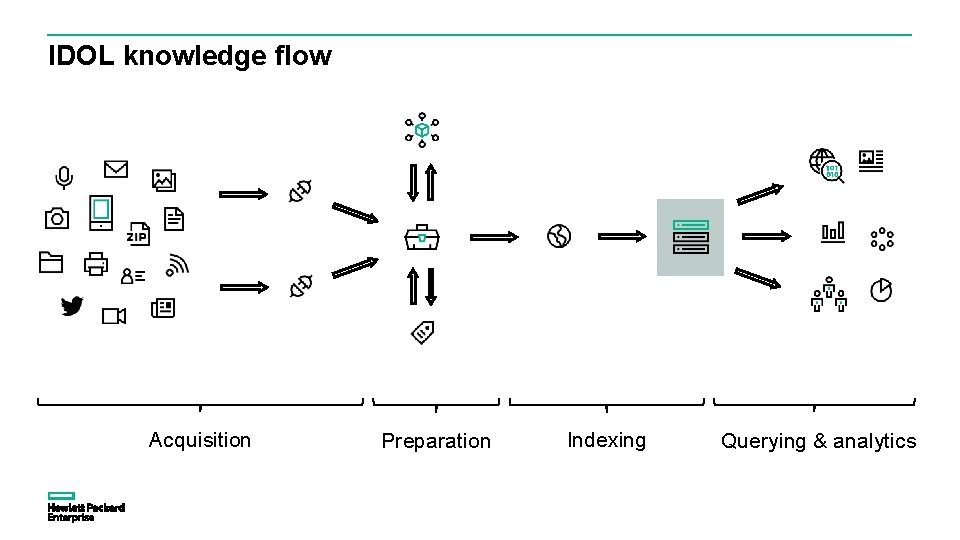
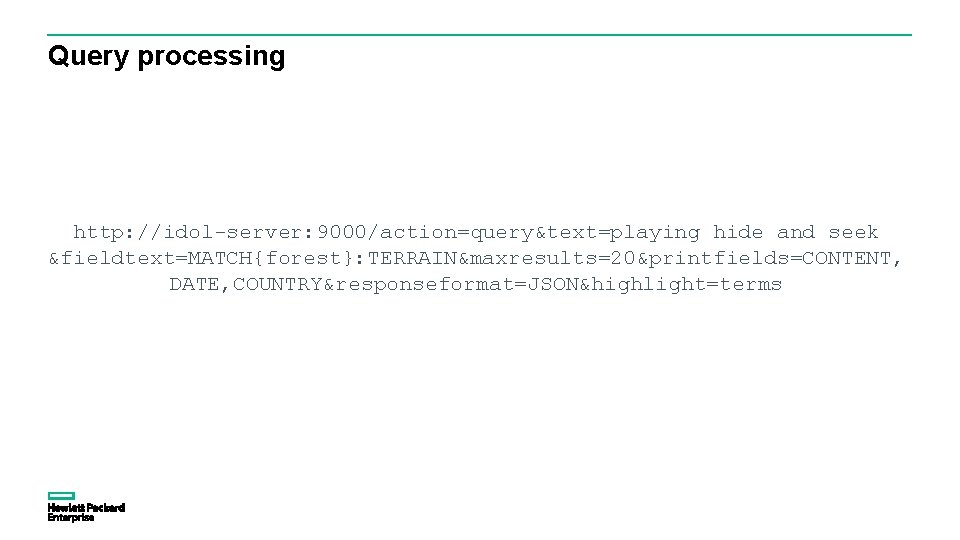
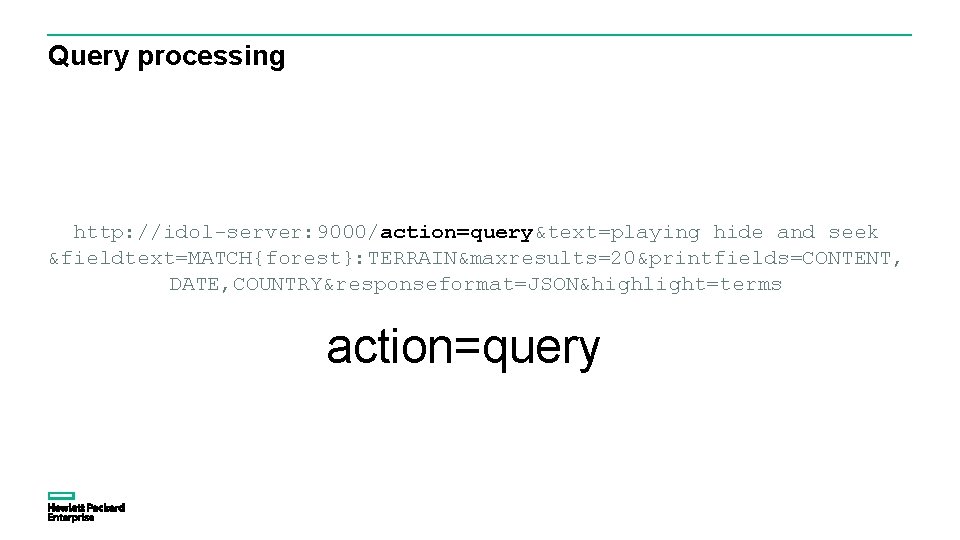
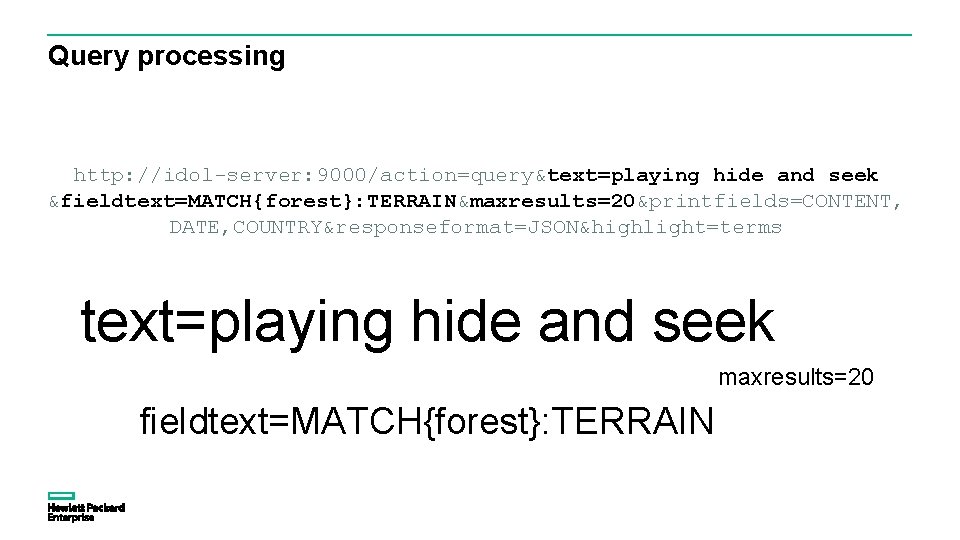
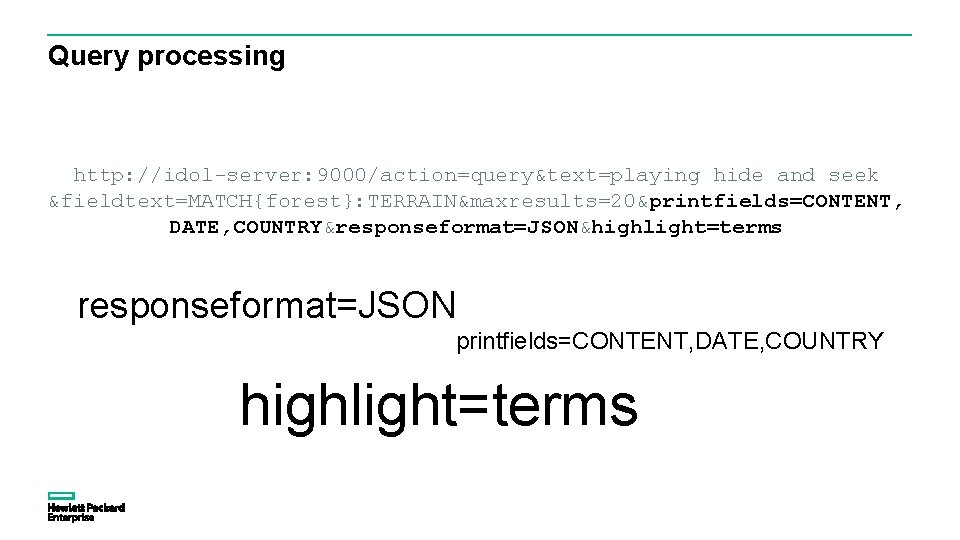
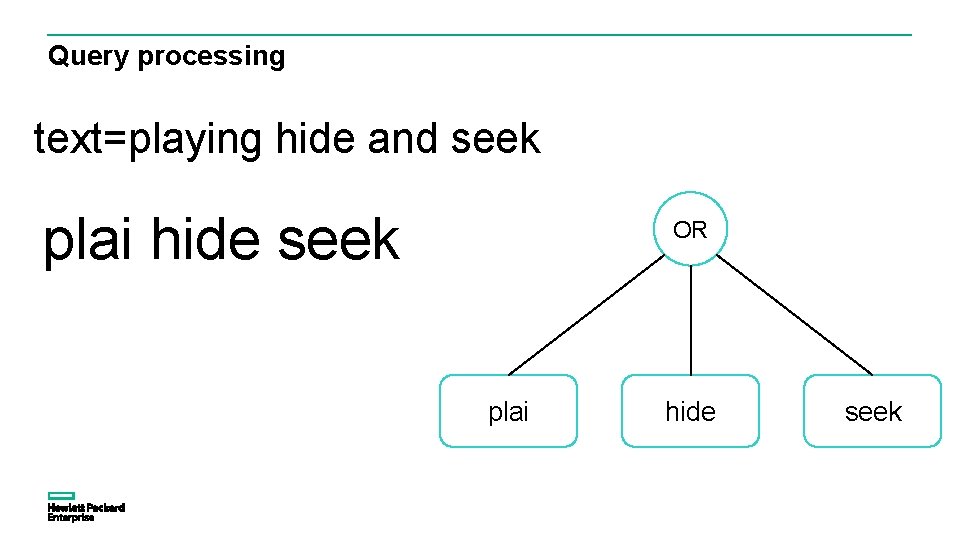
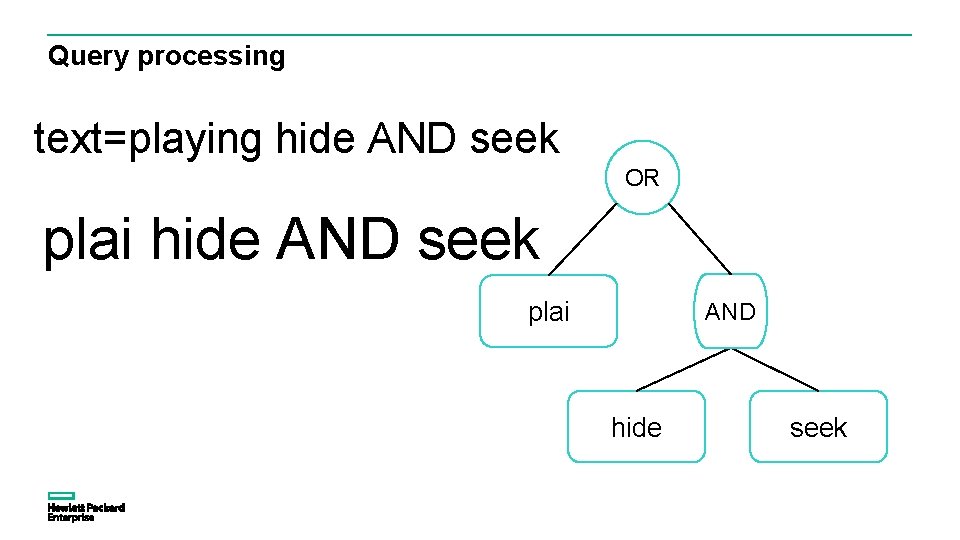
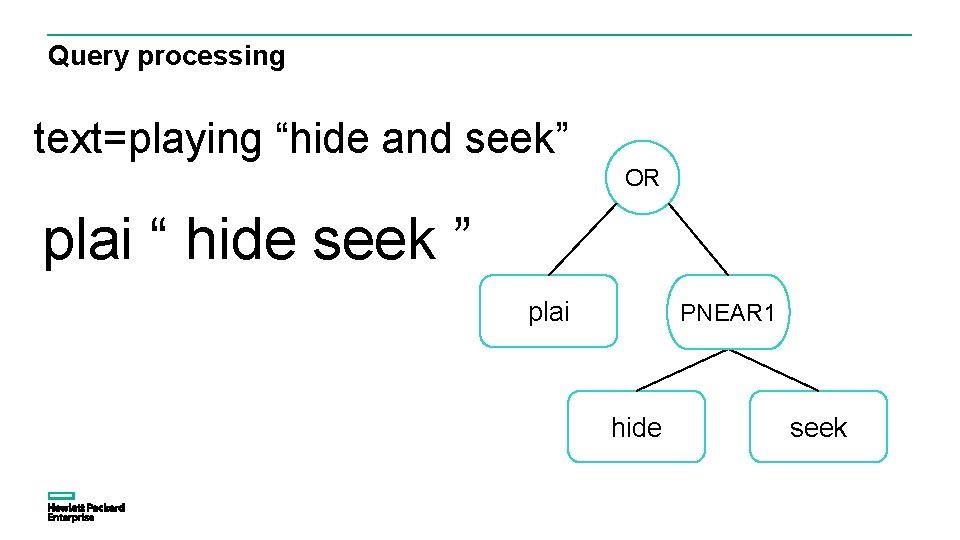
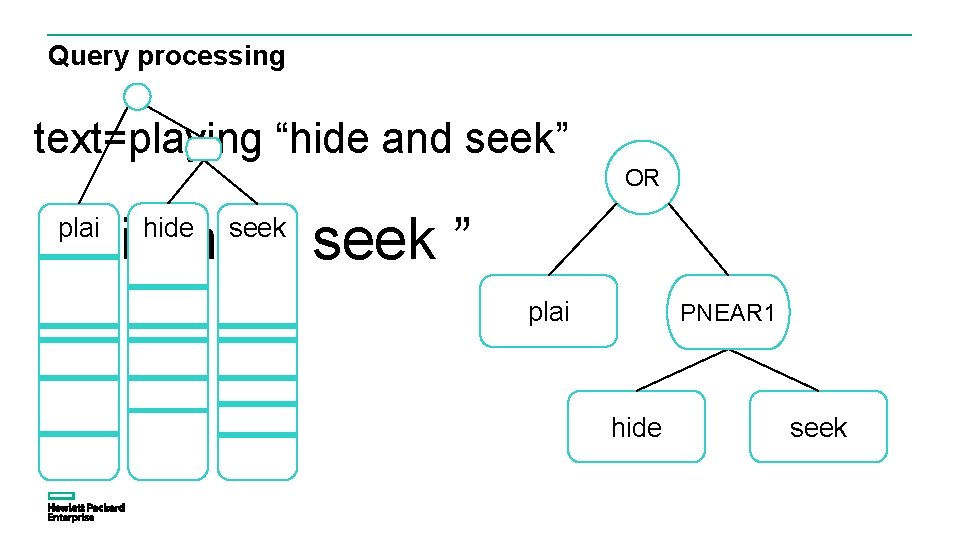
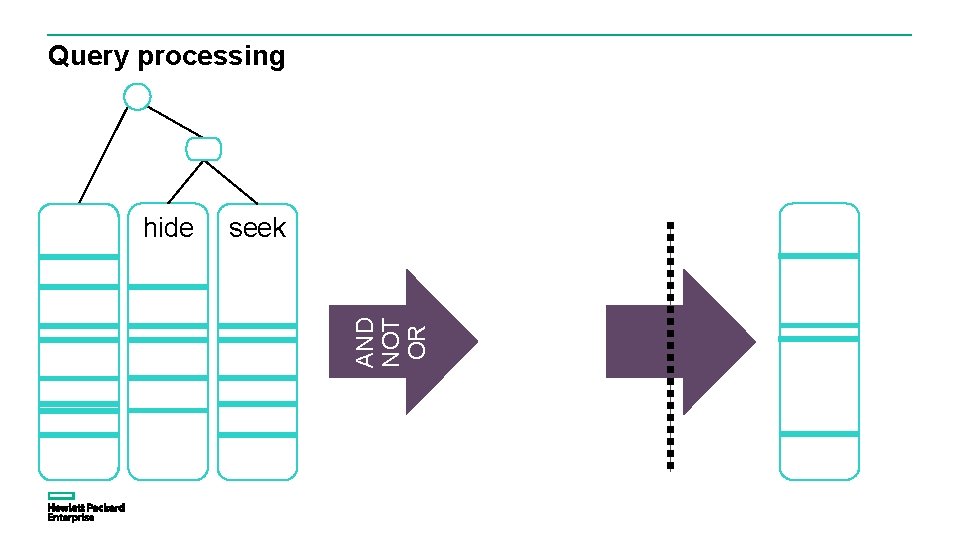
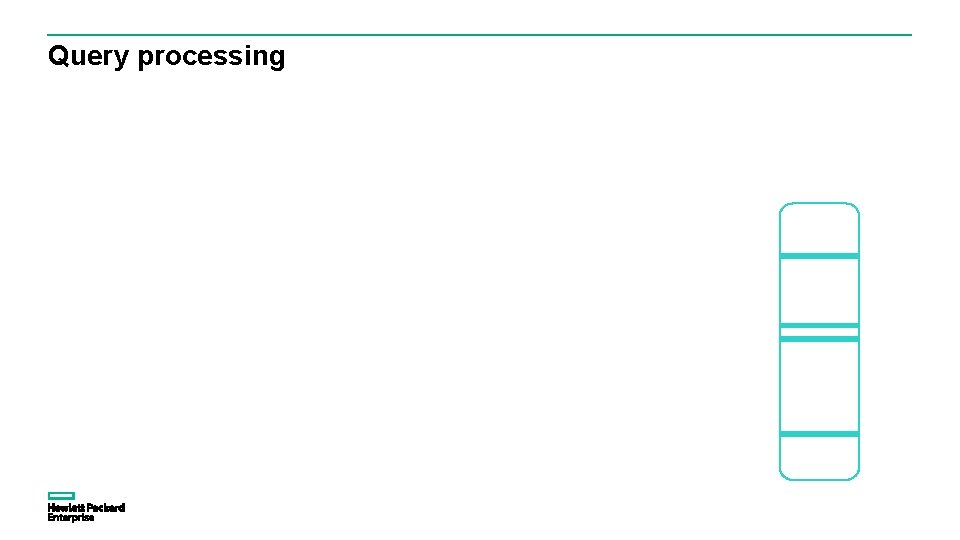
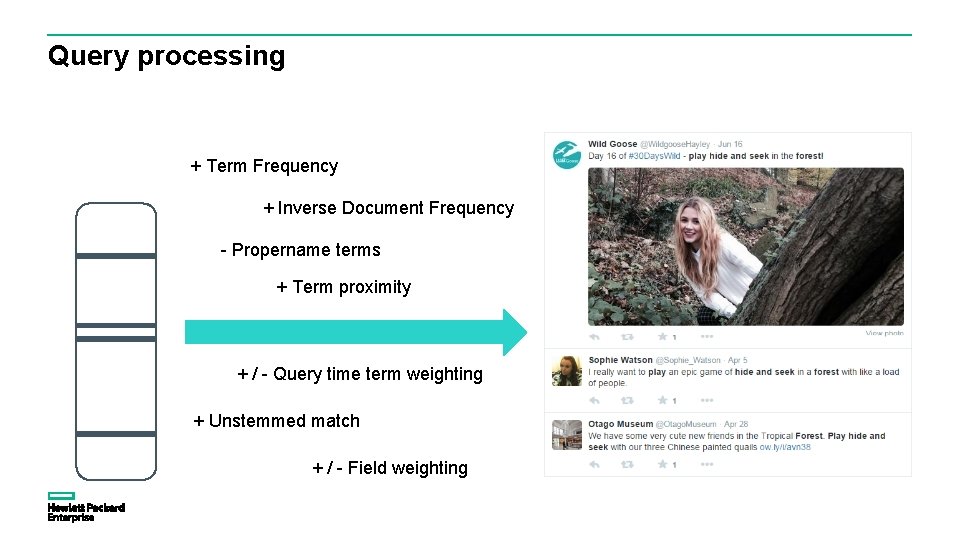
![Renaissance[80] Santa[66] Maria[66] Painter[70] Leonardo[100] 1582243 30 12 22 1 1 4 17 77. Renaissance[80] Santa[66] Maria[66] Painter[70] Leonardo[100] 1582243 30 12 22 1 1 4 17 77.](https://slidetodoc.com/presentation_image/49256a3a63e5e41eb3eb4a6333facbfa/image-32.jpg)
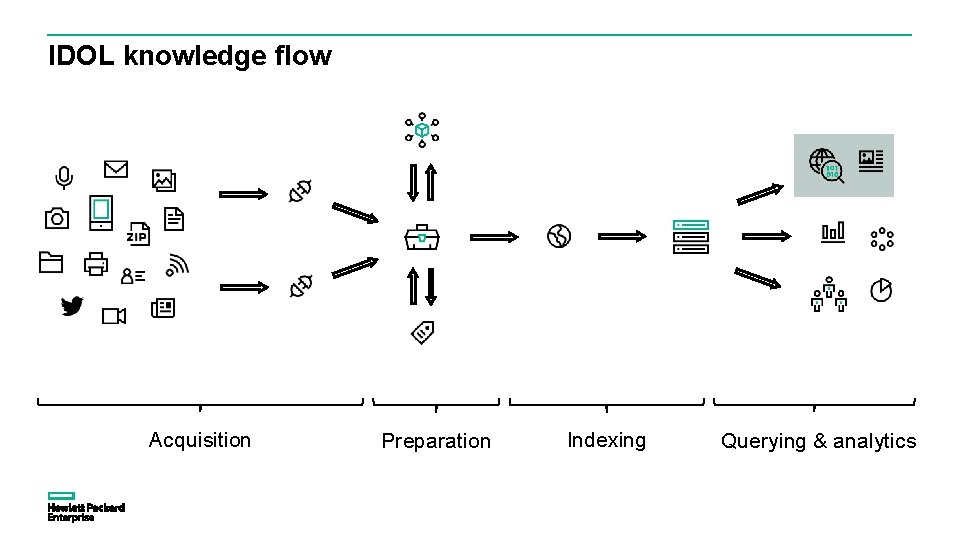
![Agents SHAKESPEAR~[500] ROMEO~[400] JULIET~[400] OTHELLO~[350] HAMLET~[350] LEAR~[300] STRATFORD~[250] RSC~[200] CORIOLANU~[200] MACBETH~[150] WILLIAMSHAKESPEAR~[100]. . . Agents SHAKESPEAR~[500] ROMEO~[400] JULIET~[400] OTHELLO~[350] HAMLET~[350] LEAR~[300] STRATFORD~[250] RSC~[200] CORIOLANU~[200] MACBETH~[150] WILLIAMSHAKESPEAR~[100]. . .](https://slidetodoc.com/presentation_image/49256a3a63e5e41eb3eb4a6333facbfa/image-34.jpg)
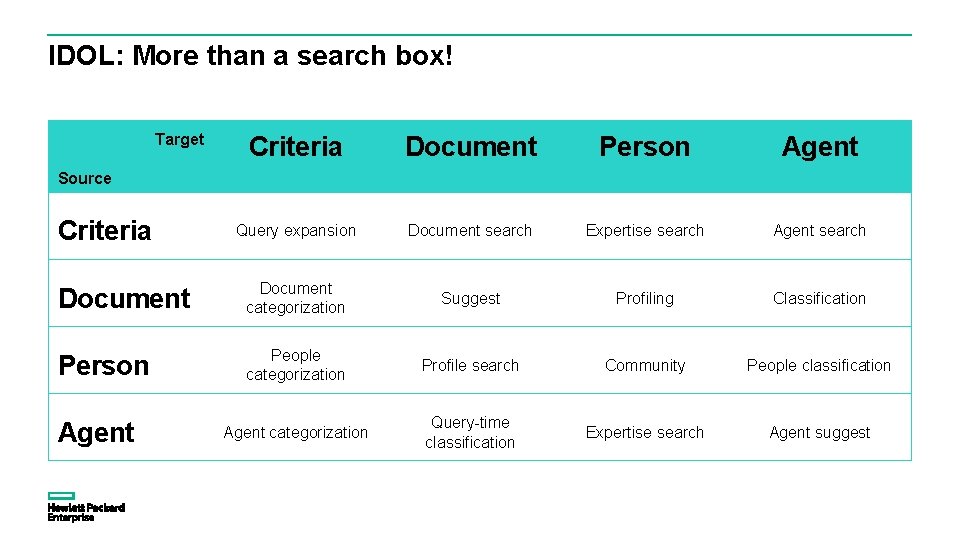
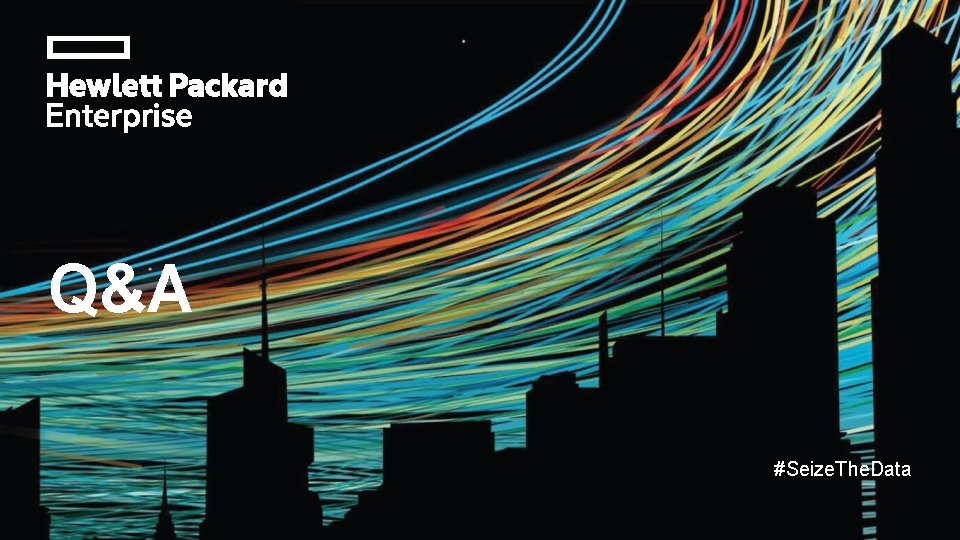
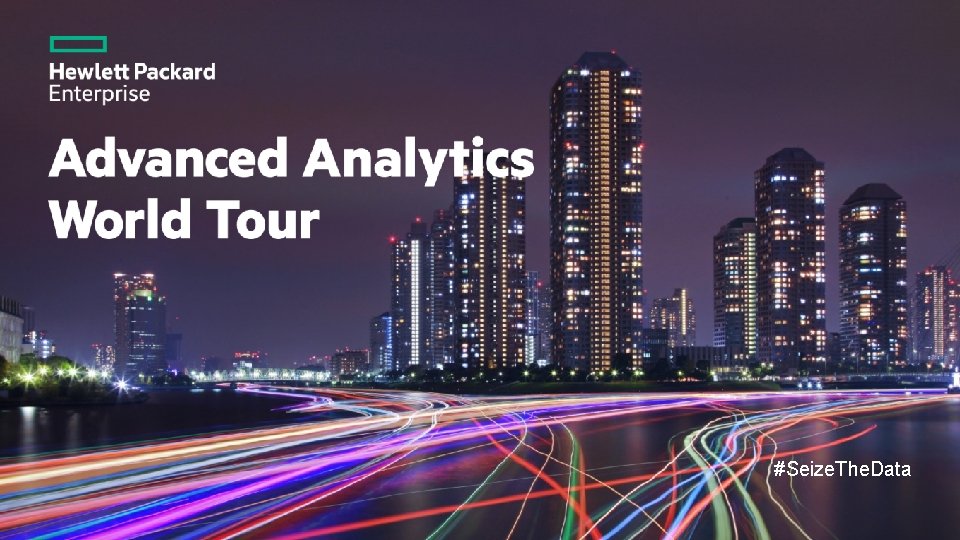
- Slides: 37
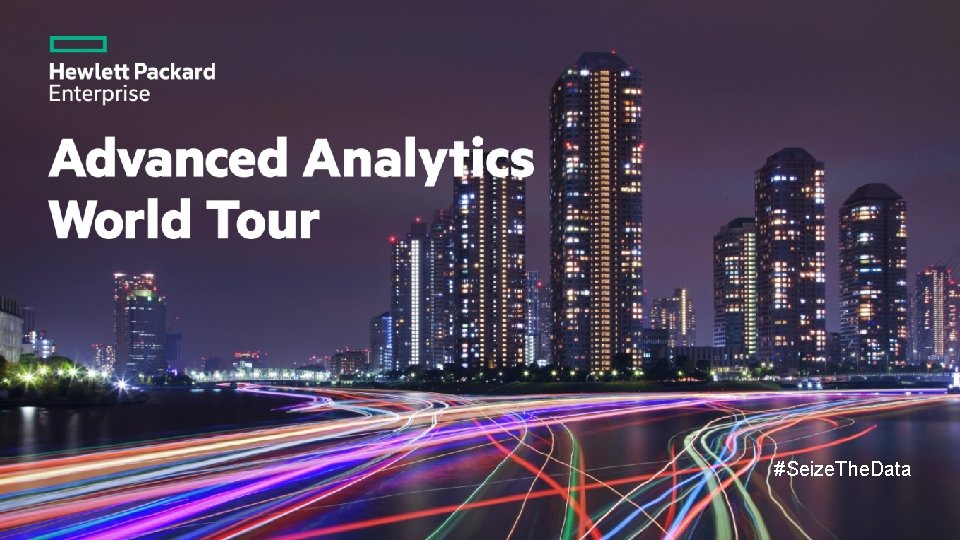
#Seize. The. Data
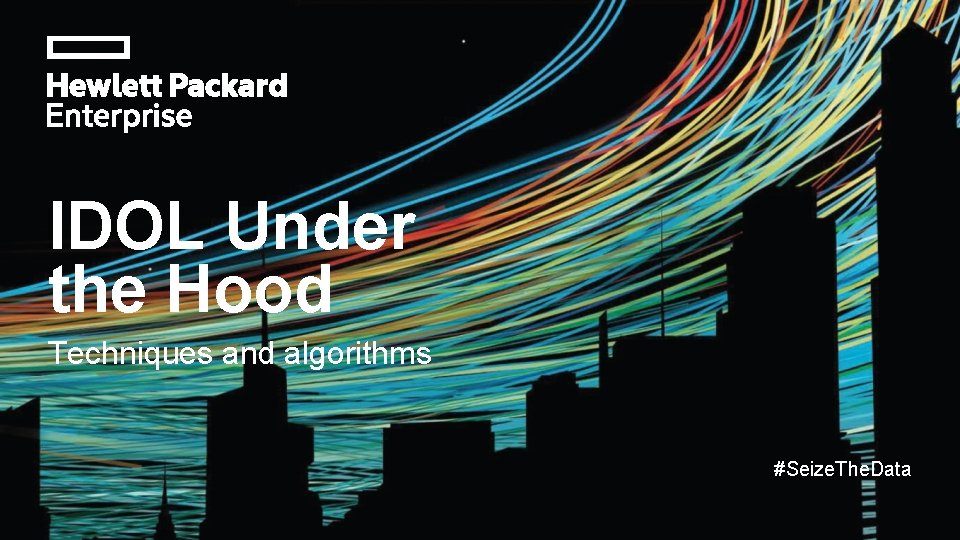
IDOL Under the Hood Techniques and algorithms #Seize. The. Data
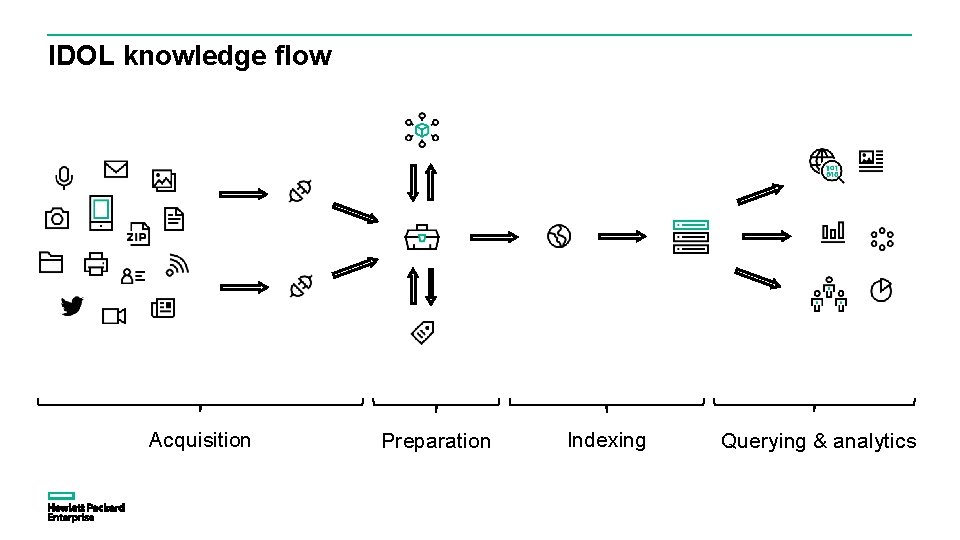
IDOL knowledge flow Acquisition Preparation Indexing Querying & analytics
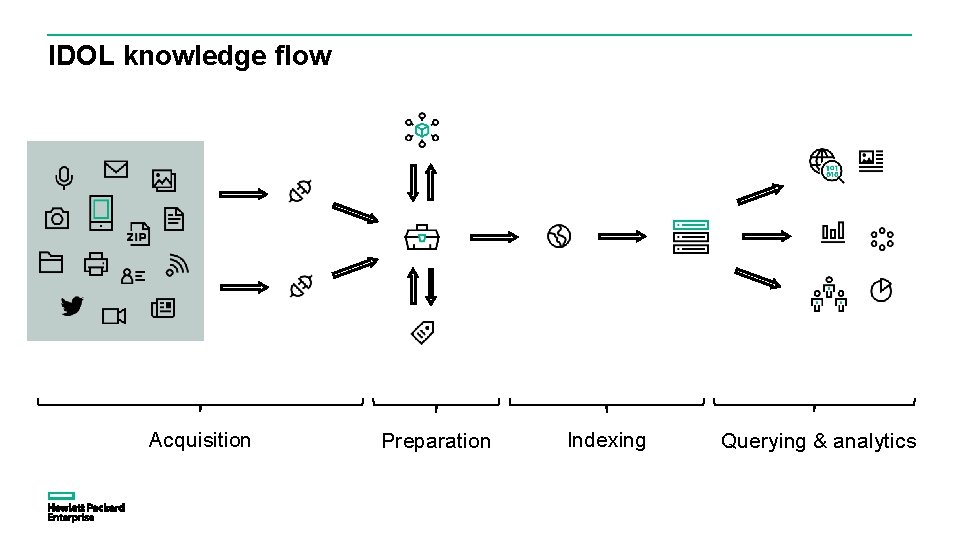
IDOL knowledge flow Acquisition Preparation Indexing Querying & analytics
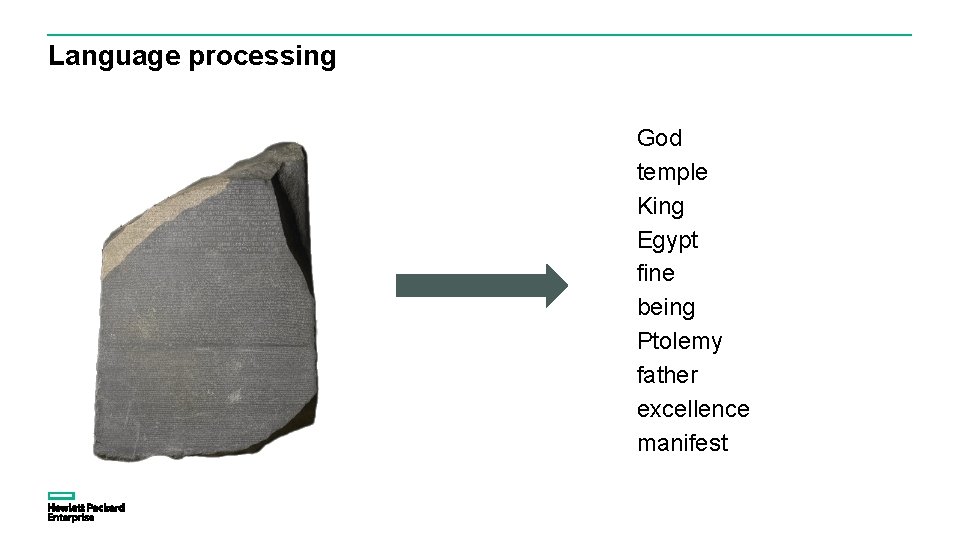
Language processing God temple King Egypt fine being Ptolemy father excellence manifest
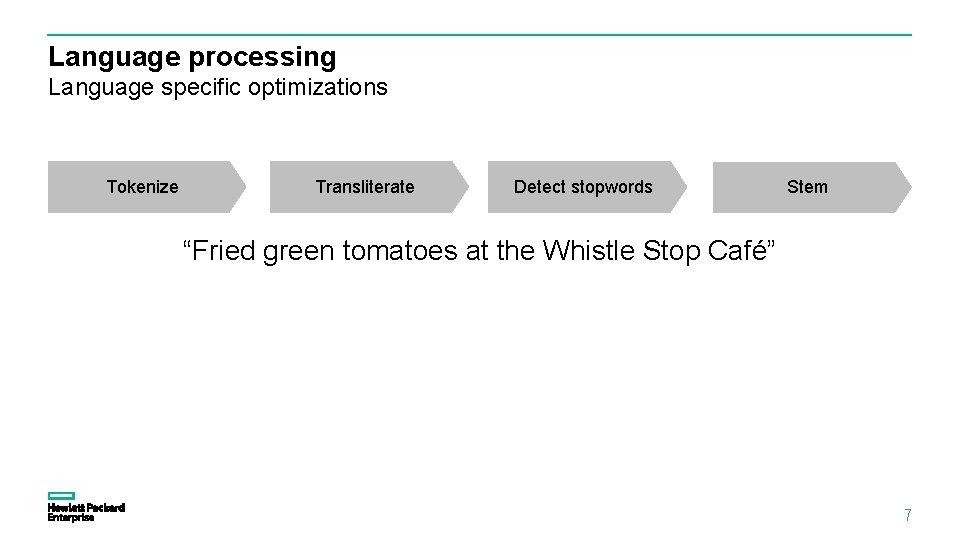
Language processing Language specific optimizations Tokenize Transliterate Detect stopwords Stem “Fried green tomatoes at the Whistle Stop Café” 7
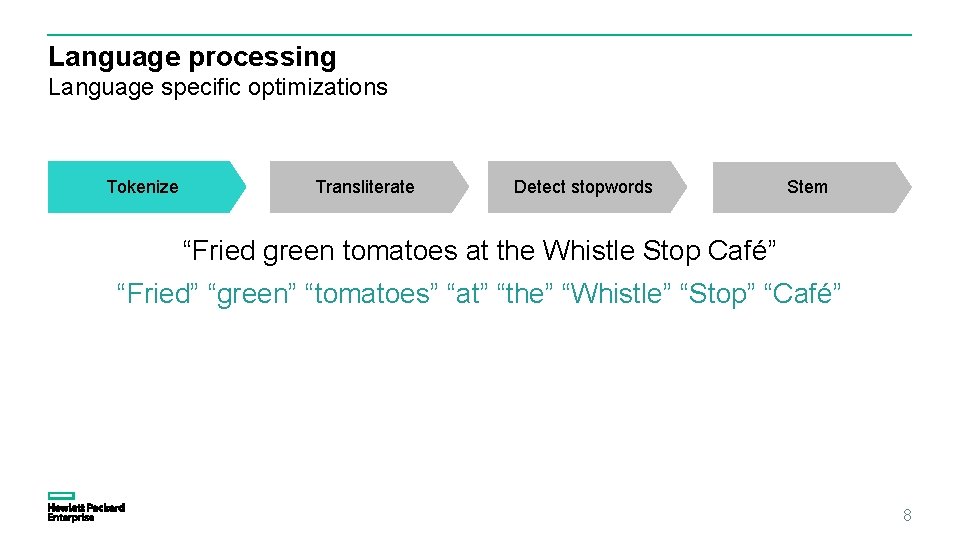
Language processing Language specific optimizations Tokenize Transliterate Detect stopwords Stem “Fried green tomatoes at the Whistle Stop Café” “Fried” “green” “tomatoes” “at” “the” “Whistle” “Stop” “Café” 8
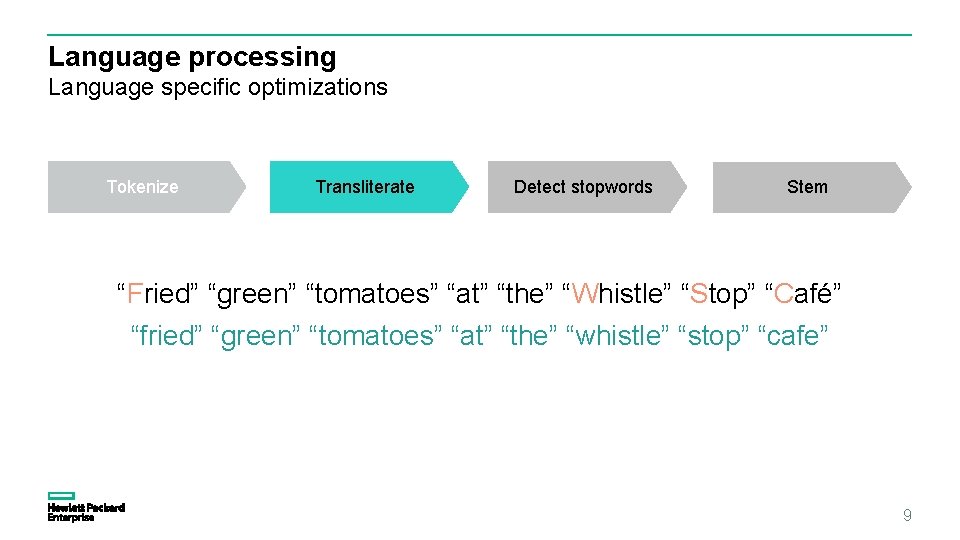
Language processing Language specific optimizations Tokenize Transliterate Detect stopwords Stem “Fried” “green” “tomatoes” “at” “the” “Whistle” “Stop” “Café” “fried” “green” “tomatoes” “at” “the” “whistle” “stop” “cafe” 9
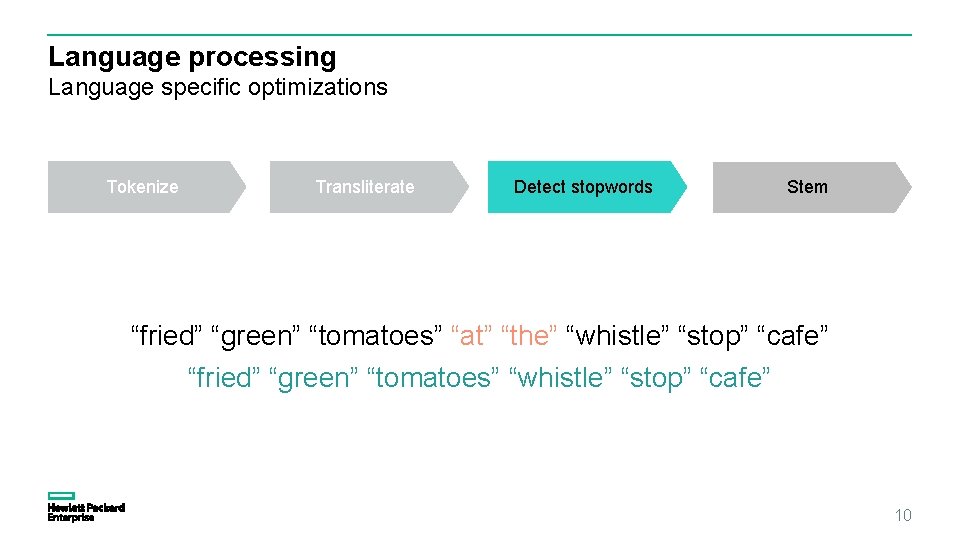
Language processing Language specific optimizations Tokenize Transliterate Detect stopwords Stem “fried” “green” “tomatoes” “at” “the” “whistle” “stop” “cafe” “fried” “green” “tomatoes” “whistle” “stop” “cafe” 10
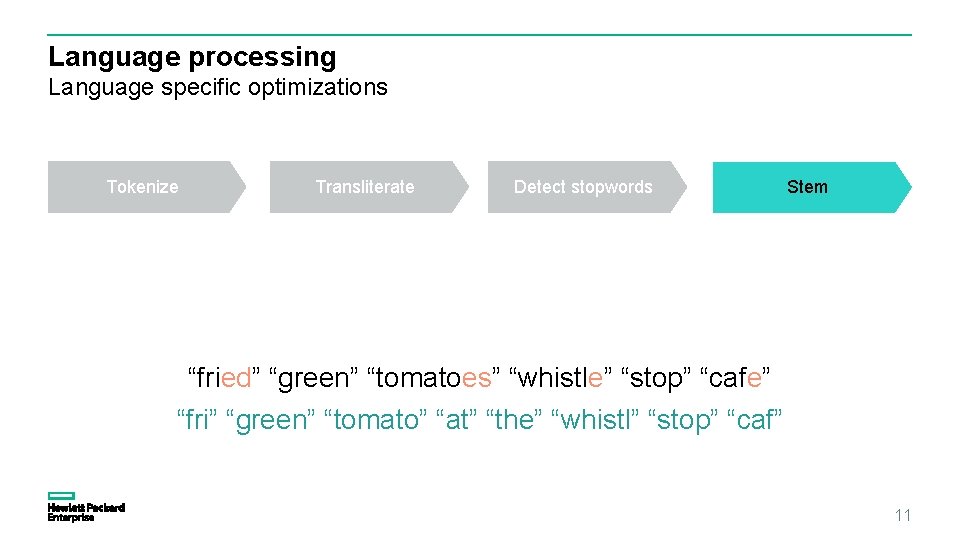
Language processing Language specific optimizations Tokenize Transliterate Detect stopwords Stem “fried” “green” “tomatoes” “whistle” “stop” “cafe” “fri” “green” “tomato” “at” “the” “whistl” “stop” “caf” 11
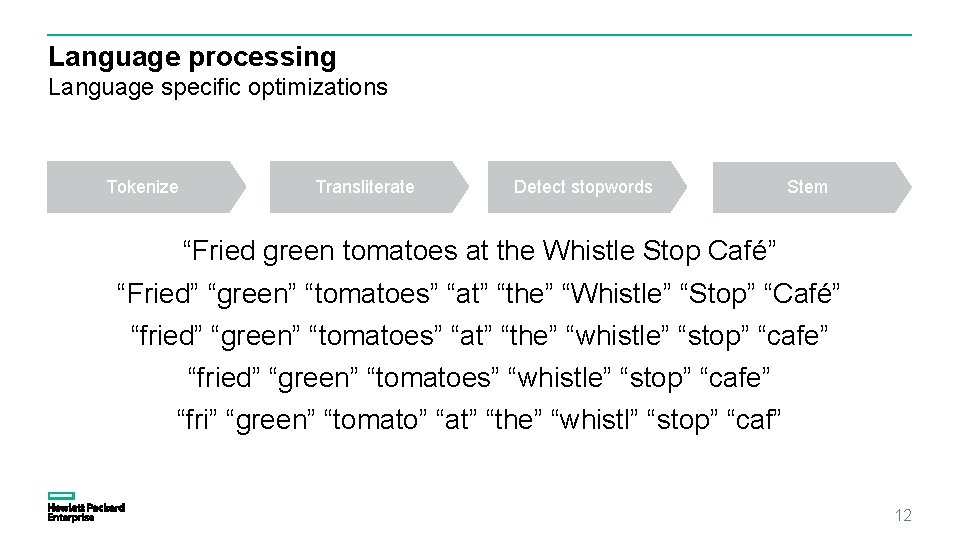
Language processing Language specific optimizations Tokenize Transliterate Detect stopwords Stem “Fried green tomatoes at the Whistle Stop Café” “Fried” “green” “tomatoes” “at” “the” “Whistle” “Stop” “Café” “fried” “green” “tomatoes” “at” “the” “whistle” “stop” “cafe” “fried” “green” “tomatoes” “whistle” “stop” “cafe” “fri” “green” “tomato” “at” “the” “whistl” “stop” “caf” 12
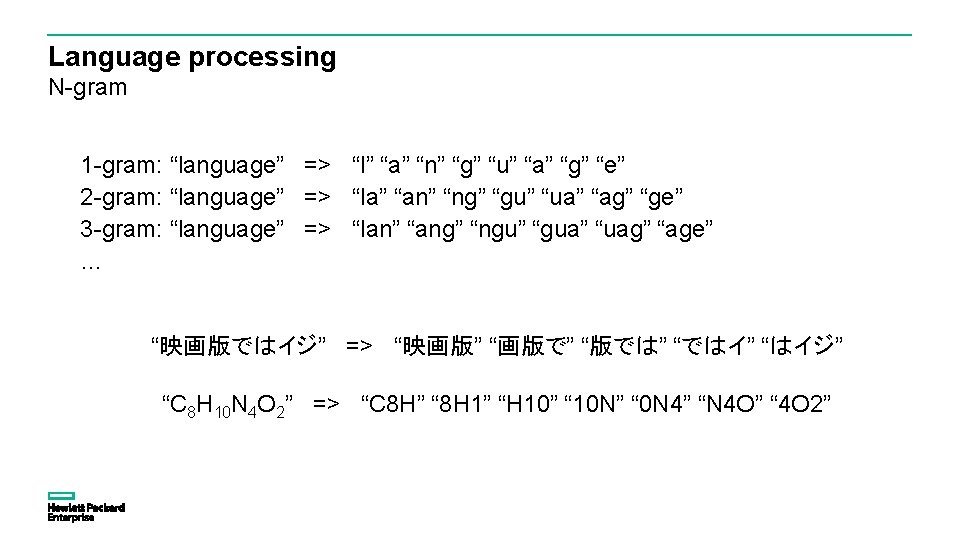
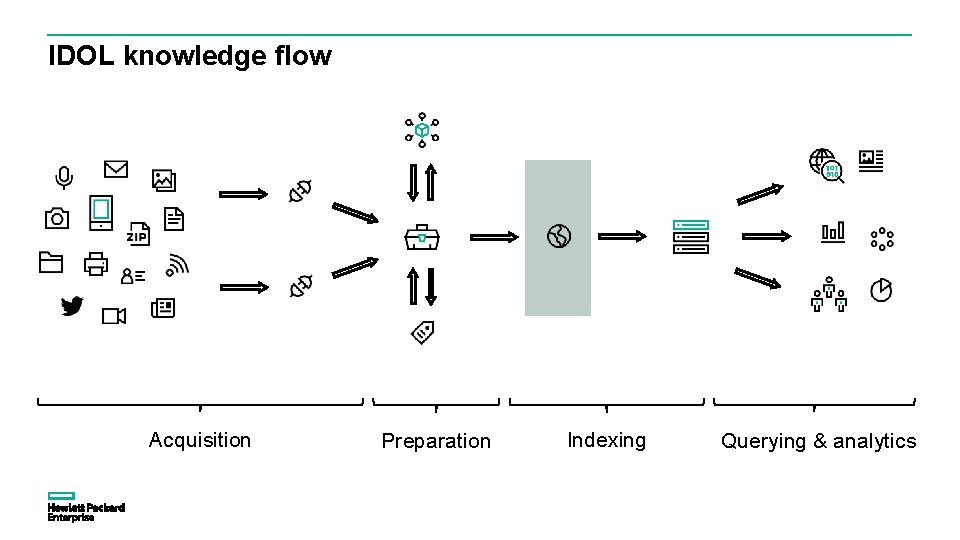
IDOL knowledge flow Acquisition Preparation Indexing Querying & analytics
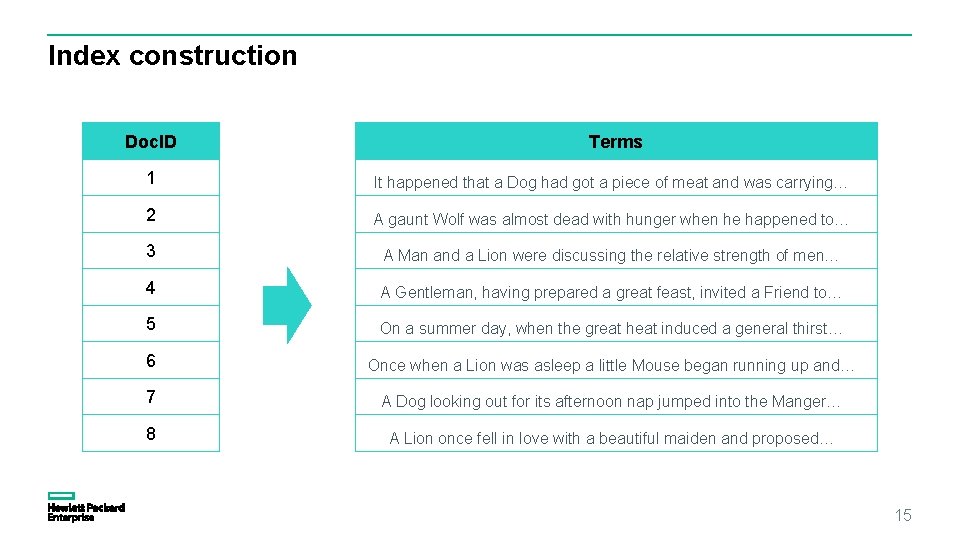
Index construction Doc. ID Terms 1 It happened that a Dog had got a piece of meat and was carrying… 2 A gaunt Wolf was almost dead with hunger when he happened to… 3 A Man and a Lion were discussing the relative strength of men… 4 A Gentleman, having prepared a great feast, invited a Friend to… 5 On a summer day, when the great heat induced a general thirst… 6 Once when a Lion was asleep a little Mouse began running up and… 7 A Dog looking out for its afternoon nap jumped into the Manger… 8 A Lion once fell in love with a beautiful maiden and proposed… 15
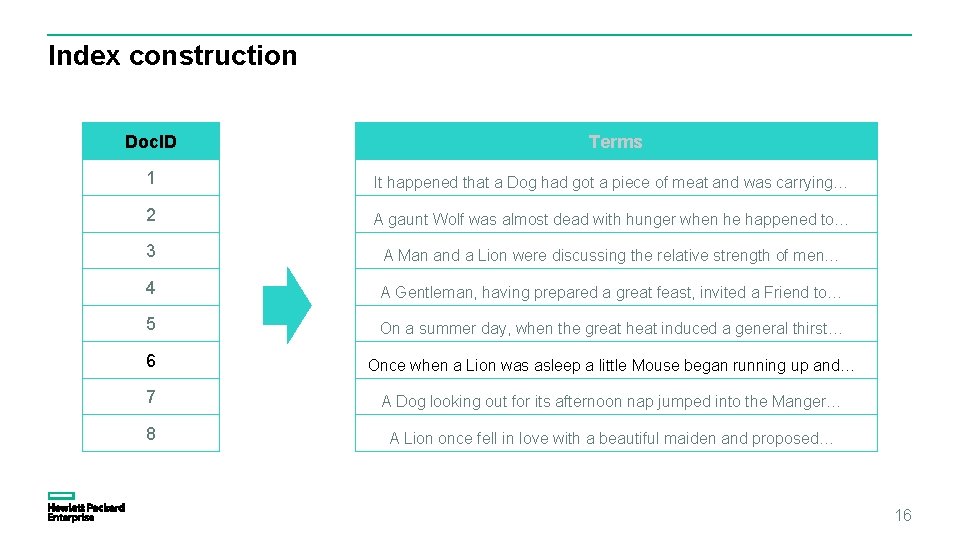
Index construction Doc. ID Terms 1 It happened that a Dog had got a piece of meat and was carrying… 2 A gaunt Wolf was almost dead with hunger when he happened to… 3 A Man and a Lion were discussing the relative strength of men… 4 A Gentleman, having prepared a great feast, invited a Friend to… 5 On a summer day, when the great heat induced a general thirst… 6 Once when a Lion was asleep a little Mouse began running up and… 7 A Dog looking out for its afternoon nap jumped into the Manger… 8 A Lion once fell in love with a beautiful maiden and proposed… 16
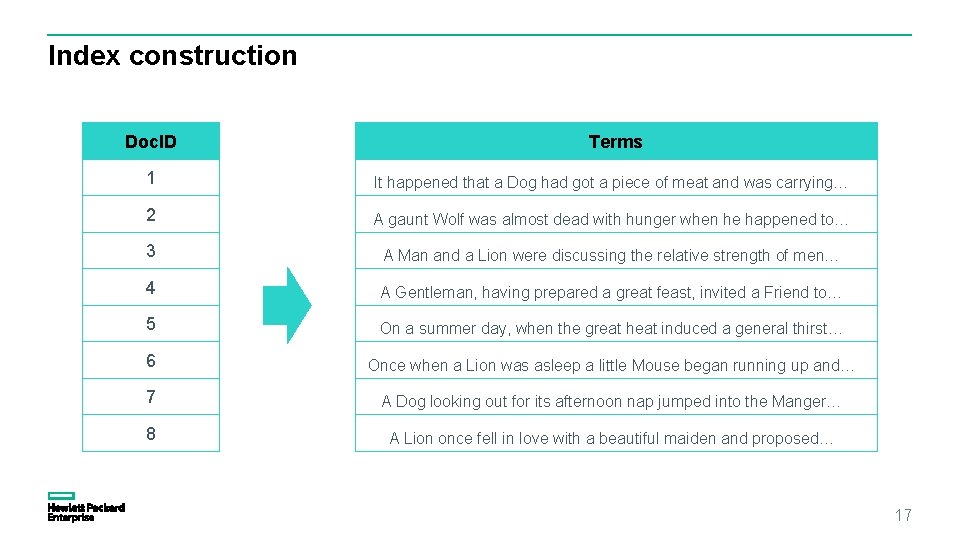
Index construction Doc. ID Terms 1 It happened that a Dog had got a piece of meat and was carrying… 2 A gaunt Wolf was almost dead with hunger when he happened to… 3 A Man and a Lion were discussing the relative strength of men… 4 A Gentleman, having prepared a great feast, invited a Friend to… 5 On a summer day, when the great heat induced a general thirst… 6 Once when a Lion was asleep a little Mouse began running up and… 7 A Dog looking out for its afternoon nap jumped into the Manger… 8 A Lion once fell in love with a beautiful maiden and proposed… 17
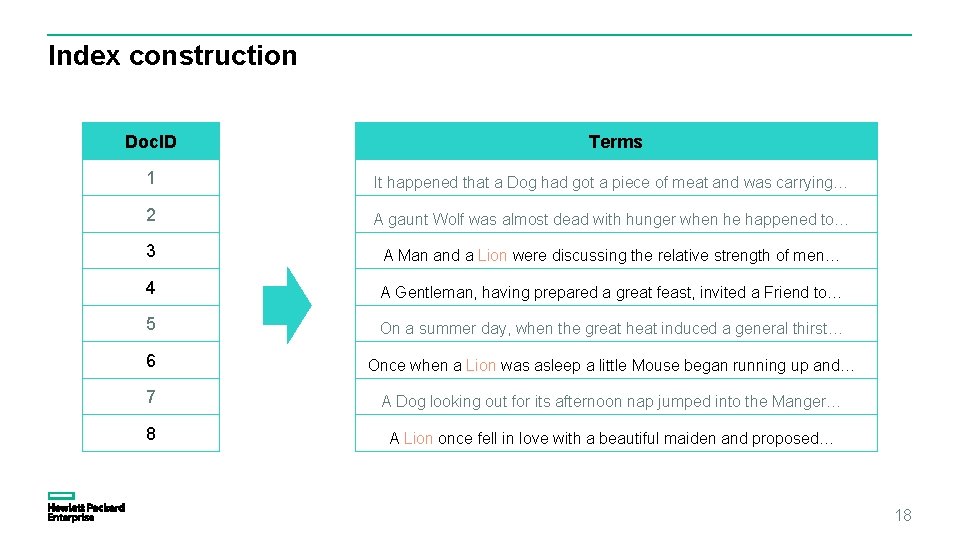
Index construction Doc. ID Terms 1 It happened that a Dog had got a piece of meat and was carrying… 2 A gaunt Wolf was almost dead with hunger when he happened to… 3 A Man and a Lion were discussing the relative strength of men… 4 A Gentleman, having prepared a great feast, invited a Friend to… 5 On a summer day, when the great heat induced a general thirst… 6 Once when a Lion was asleep a little Mouse began running up and… 7 A Dog looking out for its afternoon nap jumped into the Manger… 8 A Lion once fell in love with a beautiful maiden and proposed… 18
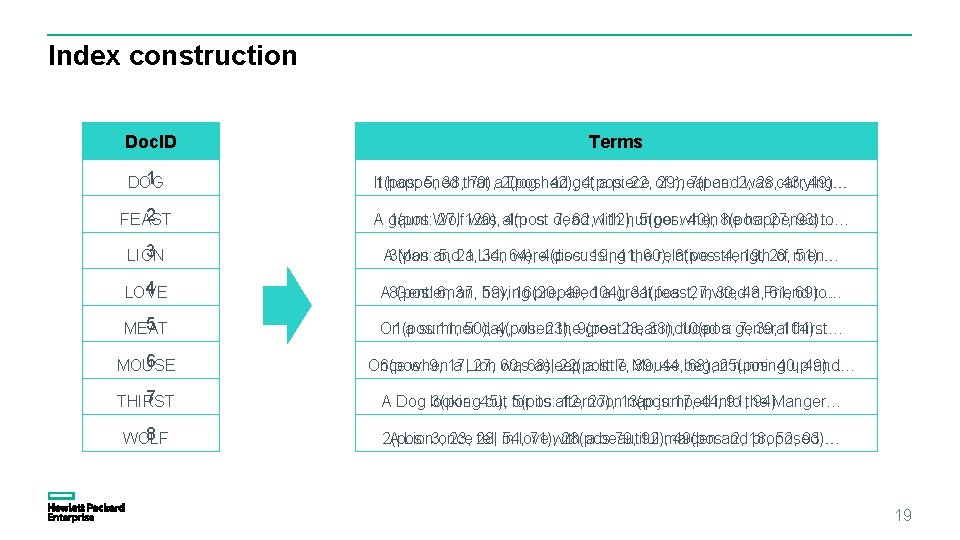
Index construction Terms Doc. IDs Terms 1 DOG It 1(pos: happened 5, 38, that 70), a 2(pos: Dog had 42), got 4(pos: a piece 22, of 29), meat 7(pos: and 2, was 28, carrying… 43, 49)… 2 FEAST A gaunt 1(pos: Wolf 27, 120), was almost 4(pos: dead 7, 62, with 112), hunger 5(pos: when 40), 8(pos: he happened 27, 93)… to… 3 LION A 3(pos: Man and 5, 21, a Lion 34, 64), were 4(pos: discussing 19, 41, the 60), relative 6(pos: strength 4, 19, 28, of men… 51)… 4 LOVE A 8(pos: Gentleman, 6, 37, 59), having 16(20, prepared 49, 104), a great 31(pos: feast, 27, invited 30, 48, a Friend 61, 69)… to… 5 MEAT On 1(pos: 11, a summer 50), day, 4(pos: when 23), the 9(pos: 23, great heat 38), induced 10(pos: a general 7, 39, 104)… thirst… 6 MOUSE Once 6(pos: when 9, 17, a Lion 27, 60, was 68), asleep 22(pos: a little 7, Mouse 39, 44, began 68), 25(pos: running 40, up 49)… and… 7 THIRST A Dog looking 3(pos: 45), out for 5(pos: its afternoon 12, 27), 13(pos: 17, nap jumped 44, into 91, the 94)… Manger… 8 WOLF 2(pos: A Lion 3, once 23, 28, fell 54, in love 71), with 28(pos: 79, a beautiful 92), maiden 49(pos: and 2, 18, proposed… 52, 93)… 19
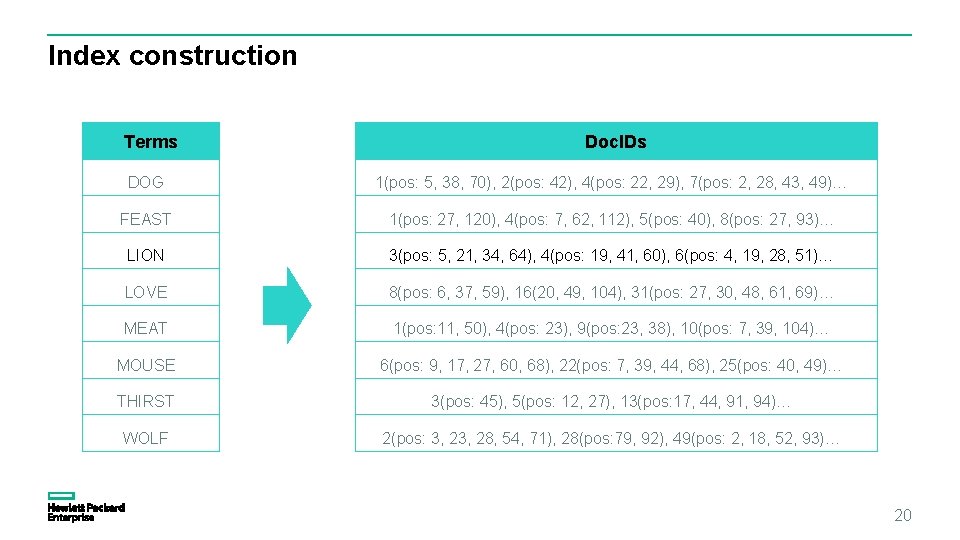
Index construction Terms Doc. IDs DOG 1(pos: 5, 38, 70), 2(pos: 42), 4(pos: 22, 29), 7(pos: 2, 28, 43, 49)… FEAST 1(pos: 27, 120), 4(pos: 7, 62, 112), 5(pos: 40), 8(pos: 27, 93)… LION 3(pos: 5, 21, 34, 64), 4(pos: 19, 41, 60), 6(pos: 4, 19, 28, 51)… LOVE 8(pos: 6, 37, 59), 16(20, 49, 104), 31(pos: 27, 30, 48, 61, 69)… MEAT 1(pos: 11, 50), 4(pos: 23), 9(pos: 23, 38), 10(pos: 7, 39, 104)… MOUSE 6(pos: 9, 17, 27, 60, 68), 22(pos: 7, 39, 44, 68), 25(pos: 40, 49)… THIRST 3(pos: 45), 5(pos: 12, 27), 13(pos: 17, 44, 91, 94)… WOLF 2(pos: 3, 28, 54, 71), 28(pos: 79, 92), 49(pos: 2, 18, 52, 93)… 20
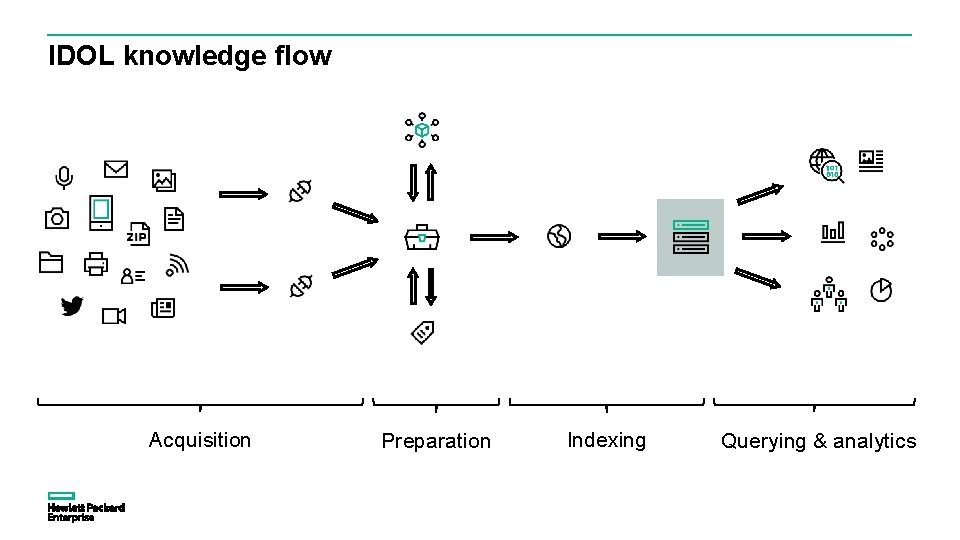
IDOL knowledge flow Acquisition Preparation Indexing Querying & analytics
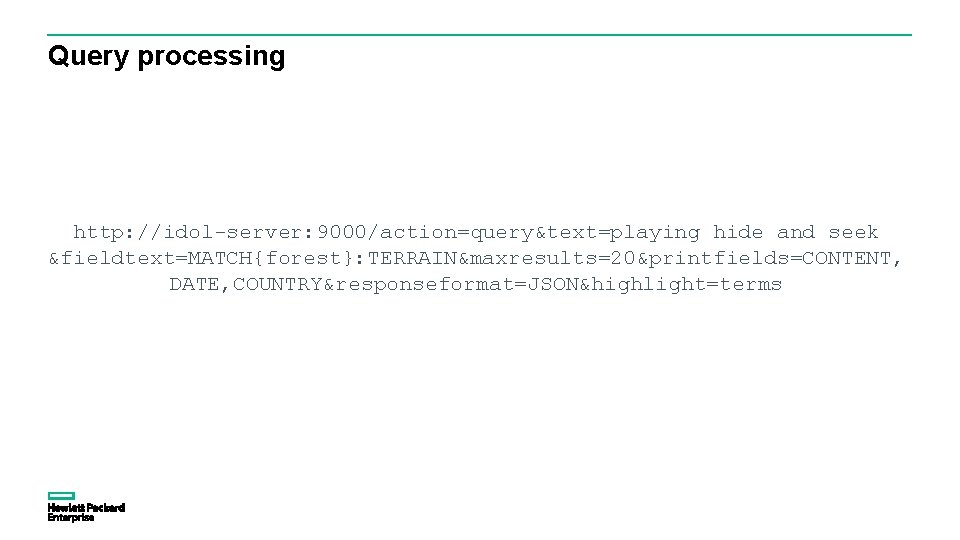
Query processing http: //idol-server: 9000/action=query&text=playing hide and seek &fieldtext=MATCH{forest}: TERRAIN&maxresults=20&printfields=CONTENT, DATE, COUNTRY&responseformat=JSON&highlight=terms
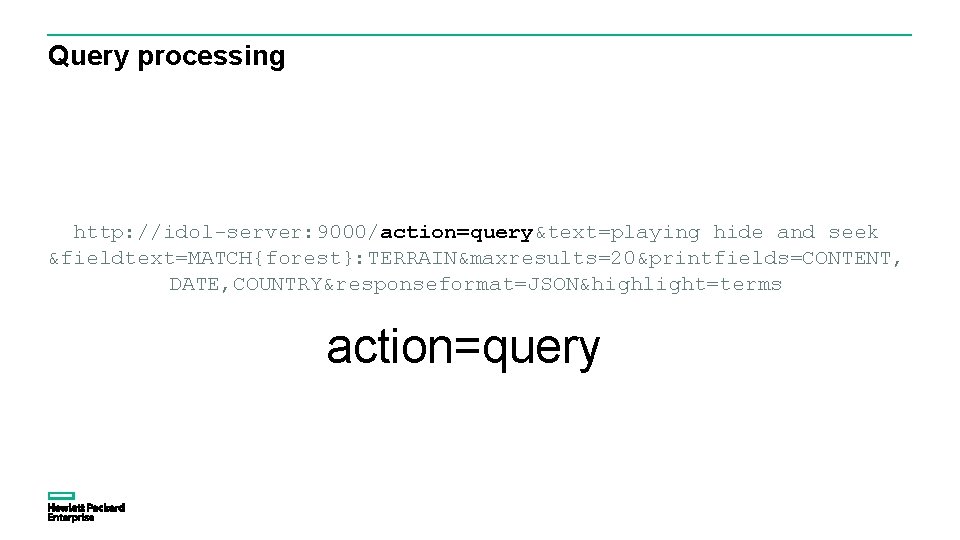
Query processing http: //idol-server: 9000/action=query&text=playing hide and seek &fieldtext=MATCH{forest}: TERRAIN&maxresults=20&printfields=CONTENT, DATE, COUNTRY&responseformat=JSON&highlight=terms action=query
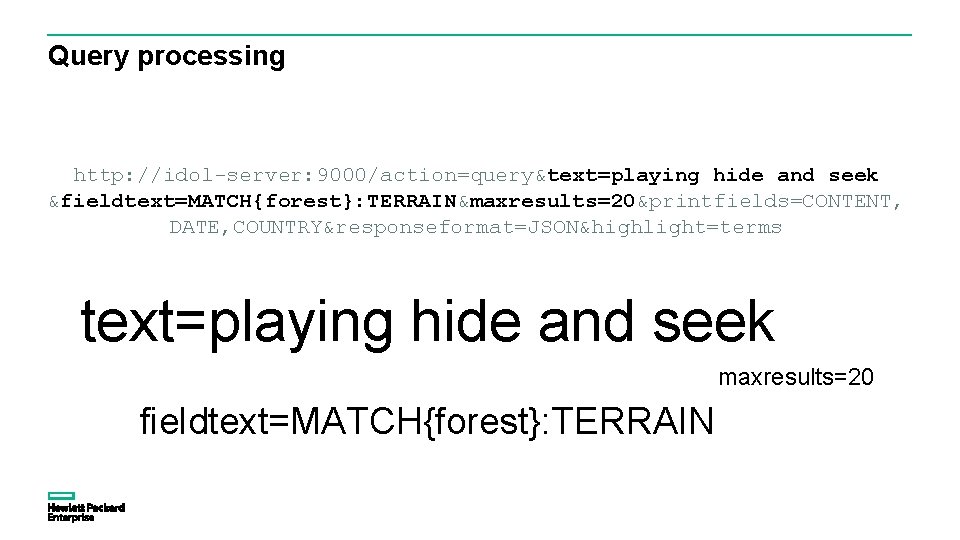
Query processing http: //idol-server: 9000/action=query&text=playing hide and seek &fieldtext=MATCH{forest}: TERRAIN&maxresults=20&printfields=CONTENT, DATE, COUNTRY&responseformat=JSON&highlight=terms text=playing hide and seek maxresults=20 fieldtext=MATCH{forest}: TERRAIN
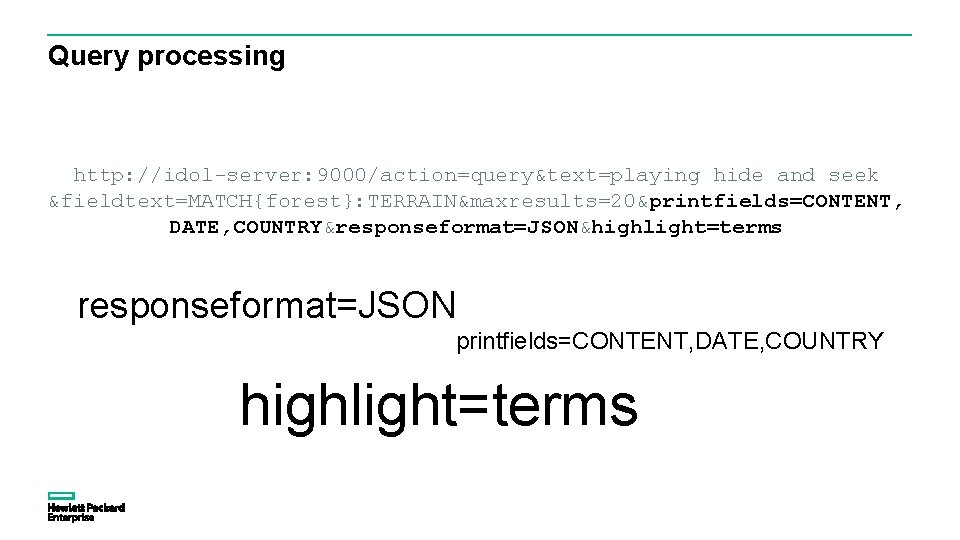
Query processing http: //idol-server: 9000/action=query&text=playing hide and seek &fieldtext=MATCH{forest}: TERRAIN&maxresults=20&printfields=CONTENT, DATE, COUNTRY&responseformat=JSON&highlight=terms responseformat=JSON printfields=CONTENT, DATE, COUNTRY highlight=terms
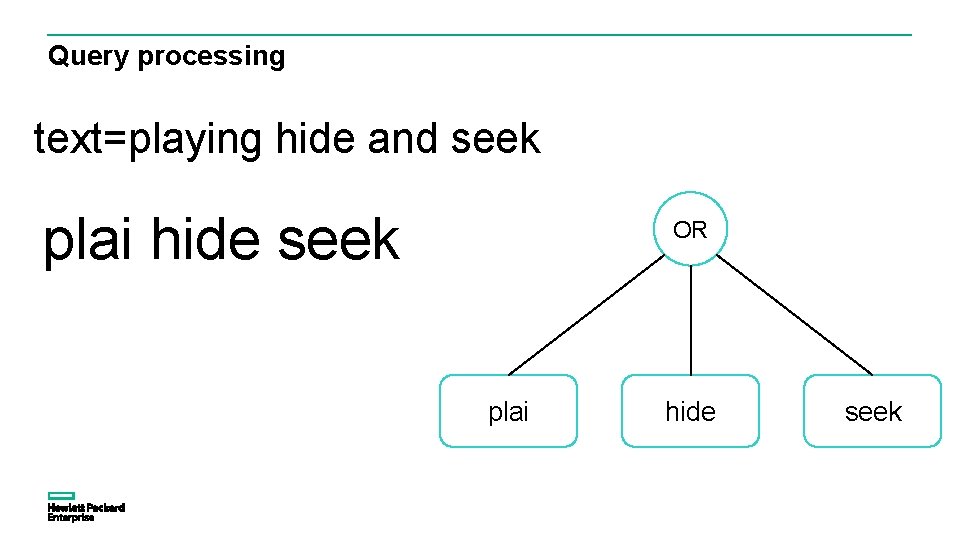
Query processing text=playing hide and seek plai hide seek OR plai hide seek
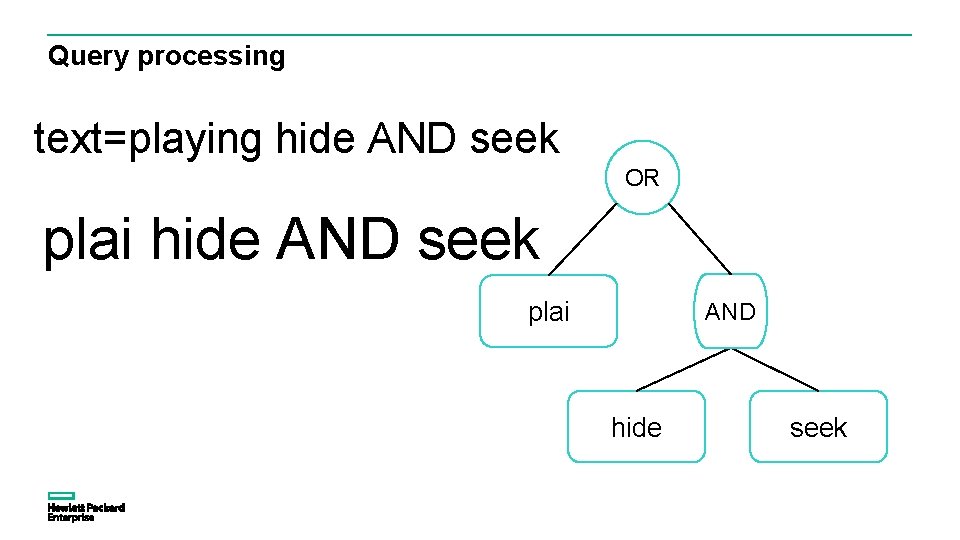
Query processing text=playing hide AND seek OR plai hide AND seek plai AND hide seek
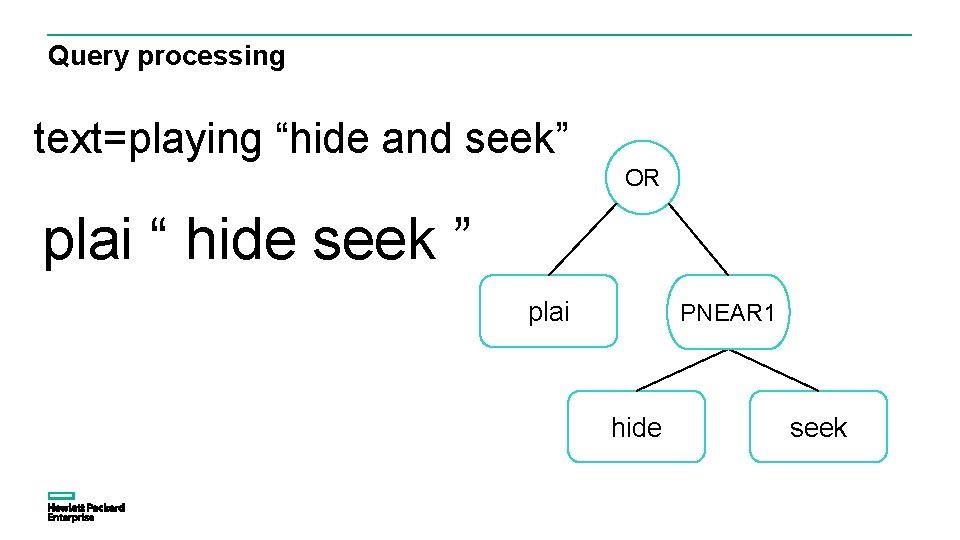
Query processing text=playing “hide and seek” OR plai “ hide seek ” plai PNEAR 1 hide seek
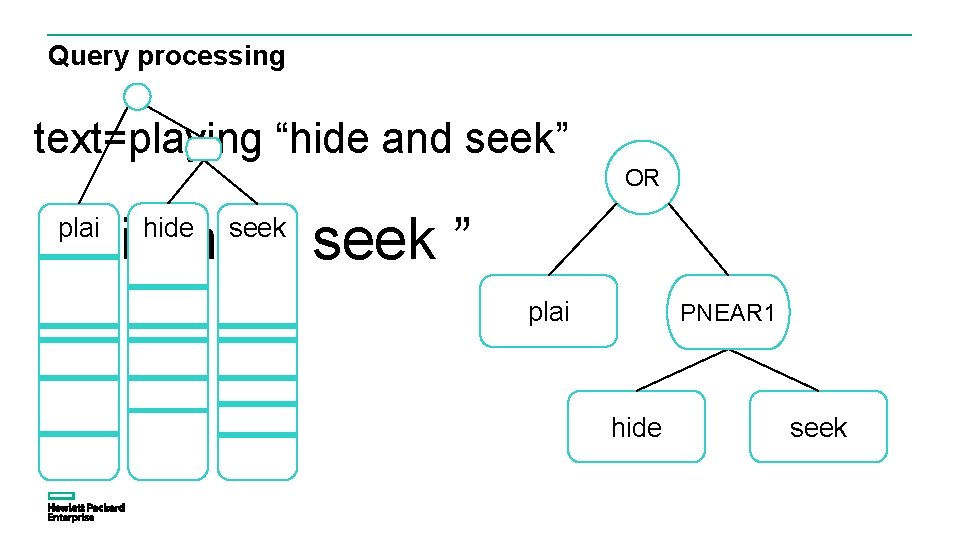
Query processing text=playing “hide and seek” OR plai “ hide seek ” plai hide seek plai PNEAR 1 hide seek
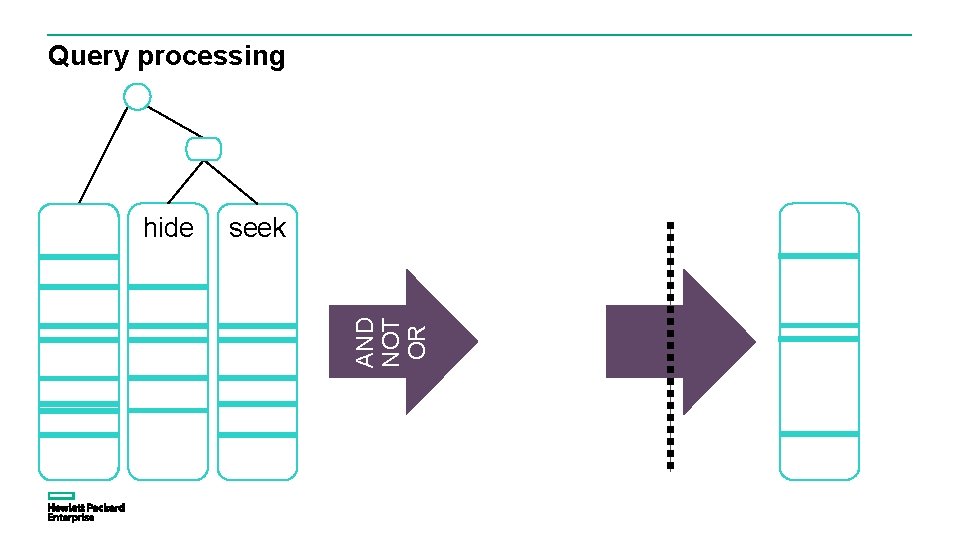
Query processing hide seek AND NOT OR plai
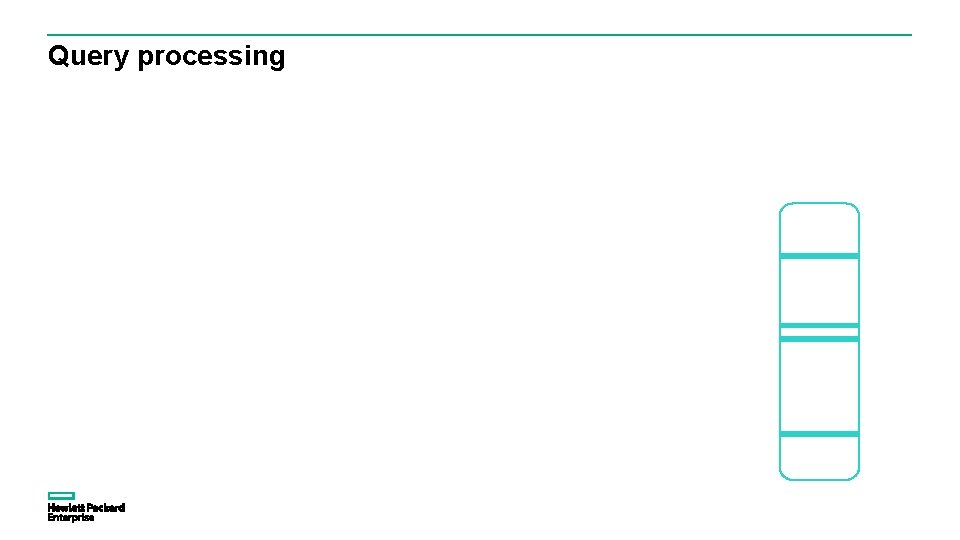
Query processing
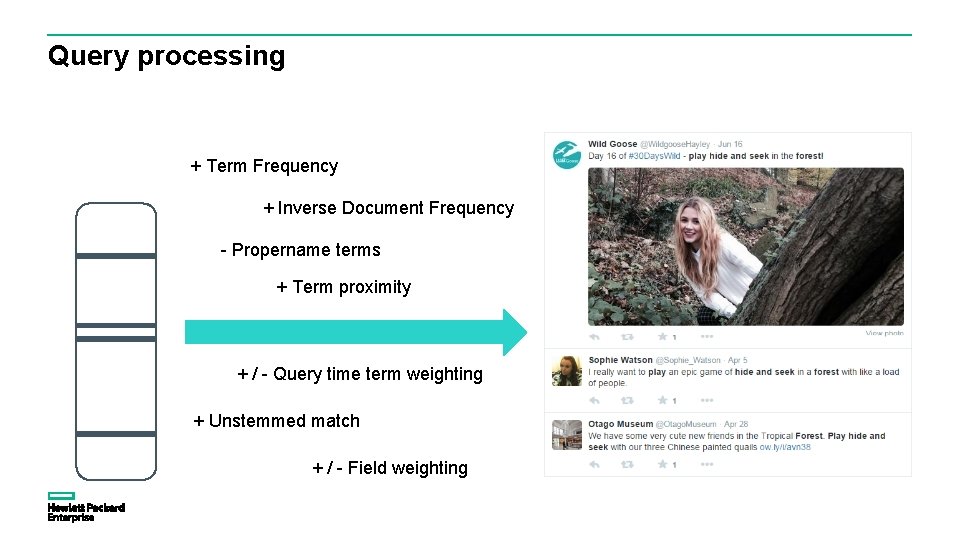
Query processing + Term Frequency + Inverse Document Frequency - Propername terms + Term proximity + / - Query time term weighting + Unstemmed match + / - Field weighting
![Renaissance80 Santa66 Maria66 Painter70 Leonardo100 1582243 30 12 22 1 1 4 17 77 Renaissance[80] Santa[66] Maria[66] Painter[70] Leonardo[100] 1582243 30 12 22 1 1 4 17 77.](https://slidetodoc.com/presentation_image/49256a3a63e5e41eb3eb4a6333facbfa/image-32.jpg)
Renaissance[80] Santa[66] Maria[66] Painter[70] Leonardo[100] 1582243 30 12 22 1 1 4 17 77. 75% Themes in Italian Renaissance painting 1565858 1 55 27 1 1 70 3 73. 73% 1480 s in art 23768 0 1 3 1 2 0 0 51. 04% Pope Paul II 2932666 0 0 2 1 1 0 0 40. 74% List of streets in Rome 41748 17 0 0 0 32. 67% Guy Ritchie Madonna[99] Italian[54] Query processing
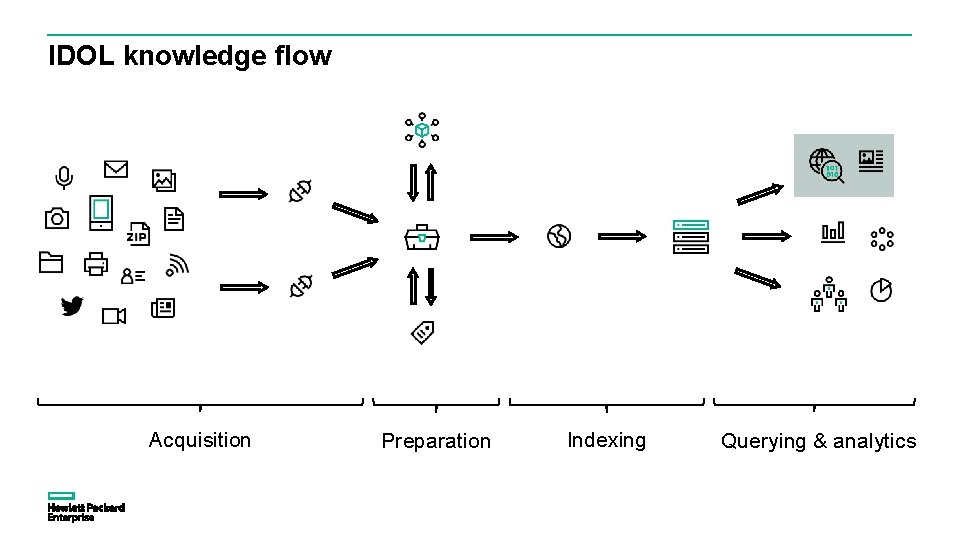
IDOL knowledge flow Acquisition Preparation Indexing Querying & analytics
![Agents SHAKESPEAR500 ROMEO400 JULIET400 OTHELLO350 HAMLET350 LEAR300 STRATFORD250 RSC200 CORIOLANU200 MACBETH150 WILLIAMSHAKESPEAR100 Agents SHAKESPEAR~[500] ROMEO~[400] JULIET~[400] OTHELLO~[350] HAMLET~[350] LEAR~[300] STRATFORD~[250] RSC~[200] CORIOLANU~[200] MACBETH~[150] WILLIAMSHAKESPEAR~[100]. . .](https://slidetodoc.com/presentation_image/49256a3a63e5e41eb3eb4a6333facbfa/image-34.jpg)
Agents SHAKESPEAR~[500] ROMEO~[400] JULIET~[400] OTHELLO~[350] HAMLET~[350] LEAR~[300] STRATFORD~[250] RSC~[200] CORIOLANU~[200] MACBETH~[150] WILLIAMSHAKESPEAR~[100]. . .
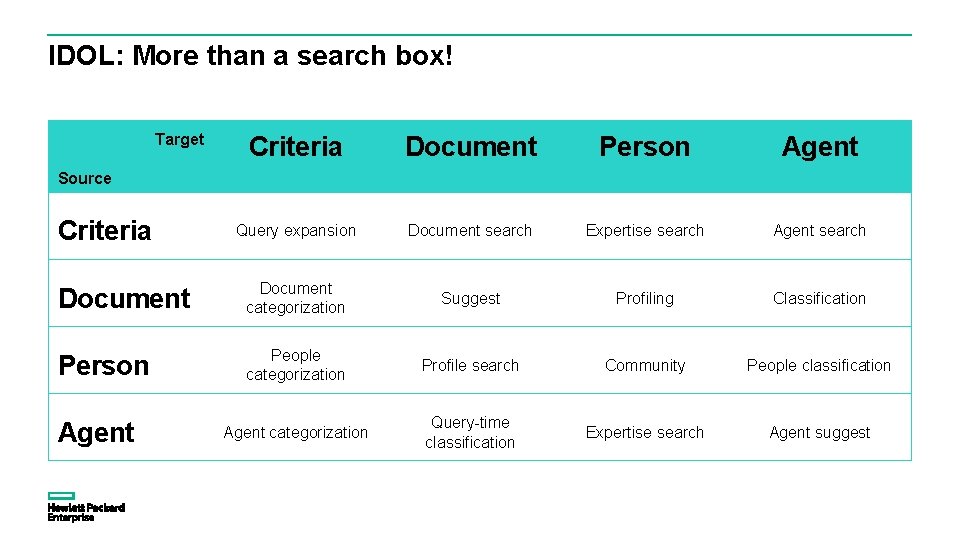
IDOL: More than a search box! Target Criteria Document Person Agent Query expansion Document search Expertise search Agent search Document categorization Suggest Profiling Classification Person People categorization Profile search Community People classification Agent categorization Query-time classification Expertise search Agent suggest Source Criteria Agent
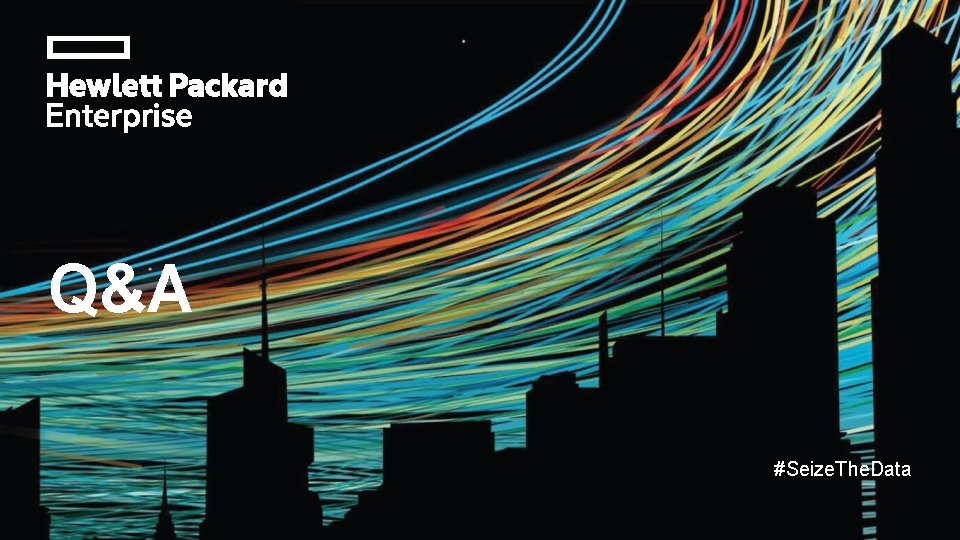
Q&A #Seize. The. Data
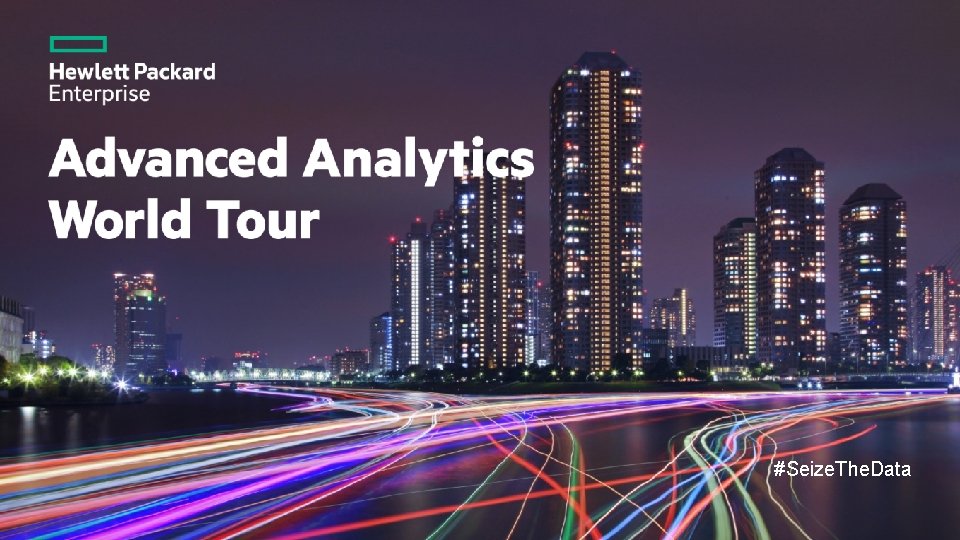
#Seize. The. Data
Under the hood of flyback smps designs
Seize the day poem
Carpe diem literary definition
아레나 시뮬레이션 예제
List of food laws in pakistan
Setting in the masque of the red death
Vendredi seize
Why were the tyrants able to seize control in athens
Les fonctions techniques et les solutions techniques
My idol sloh
Sanata in english
Baal idol worship
How to write a letter to your idol
Tandragee idol
Compliments for idols
American idol
Hamilton
The graph shown has a hamilton circuit which is
American idol
Lydia samuel american idol
American idol
American idol
Nebuchadnezzar golden idol
Unterschied idol vorbild
Idol text analytics
What do you think about vietnam idol shows
Ancient greek game
Idol certified payroll
American idol
American idol
Aleida march de la torre
Jack painted and garlanded sat there like an idol
On shows like american idol contestants often wonder
Micah's idol
How to check photo is real or fake
Hình ảnh bộ gõ cơ thể búng tay
Bổ thể