Seasonality of influenza Analysis of seasonality l To
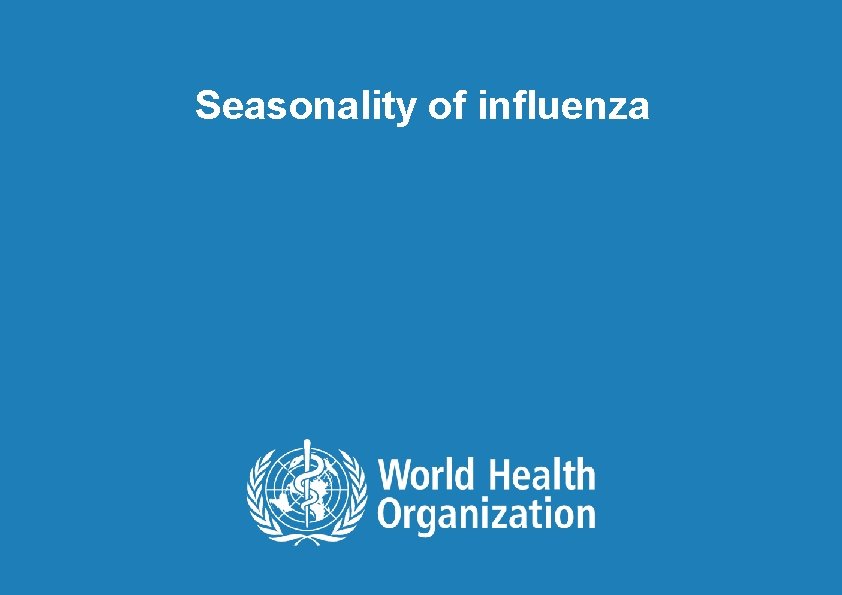
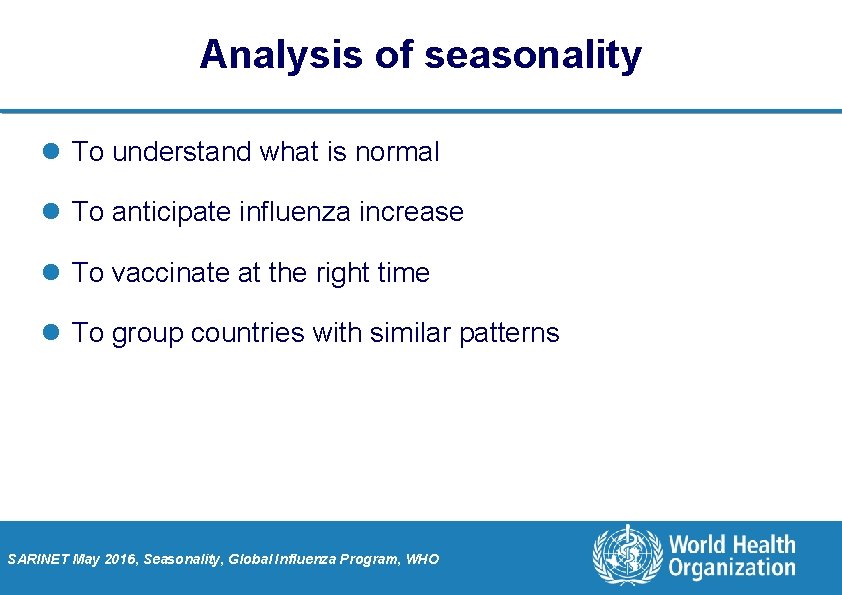
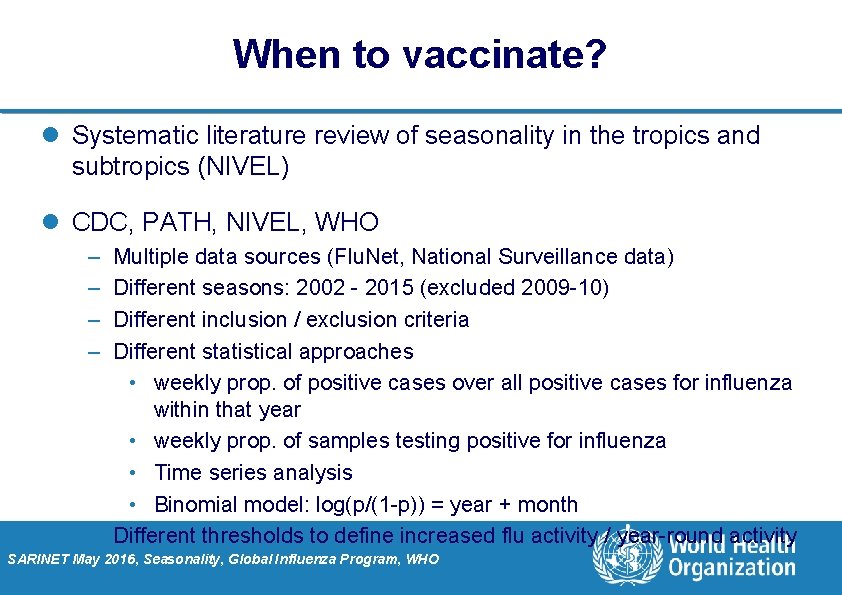
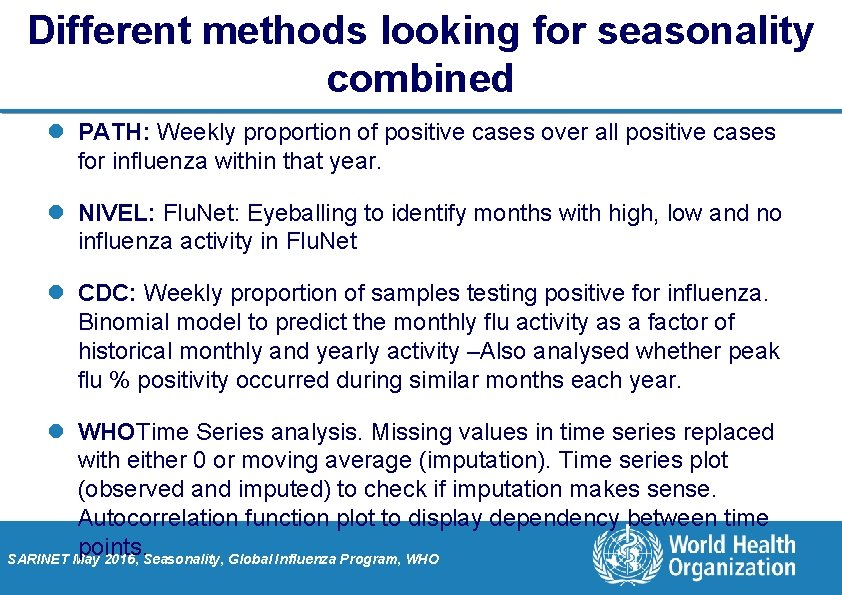
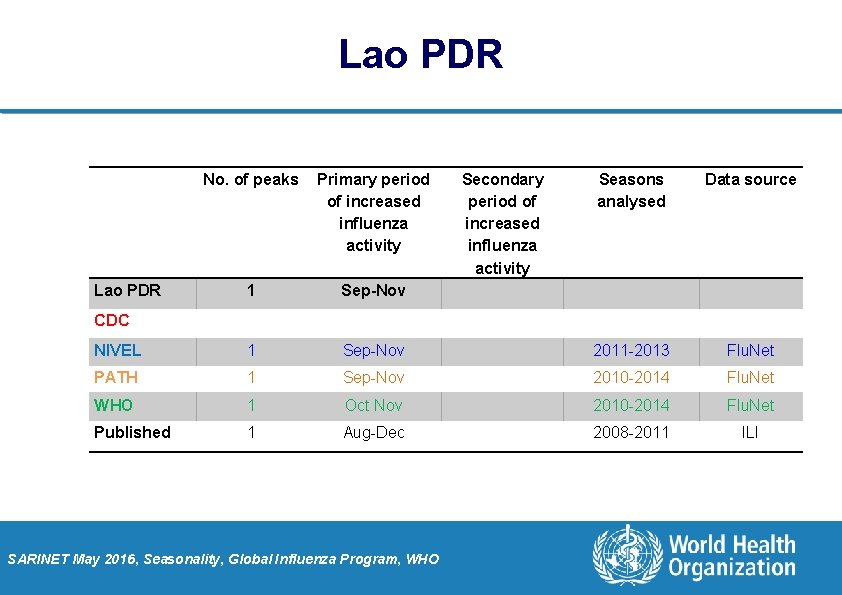
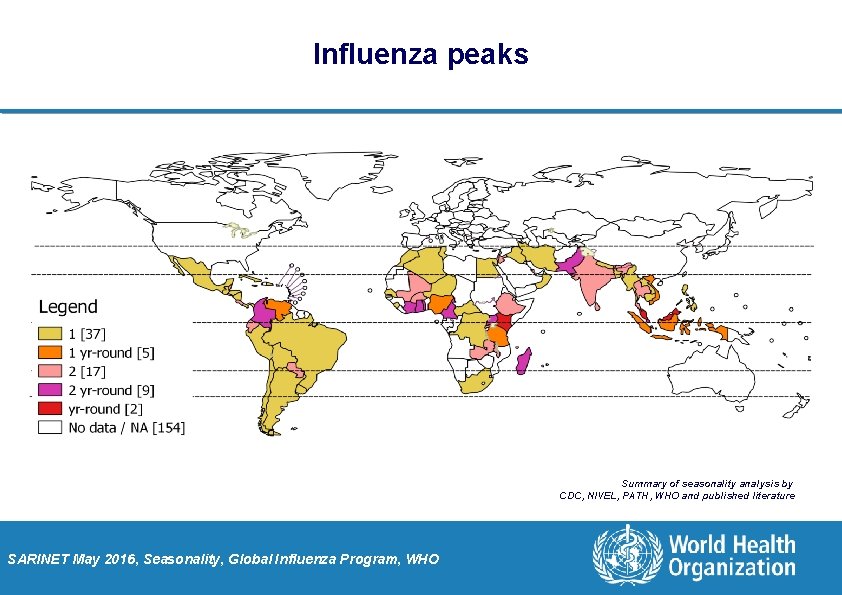
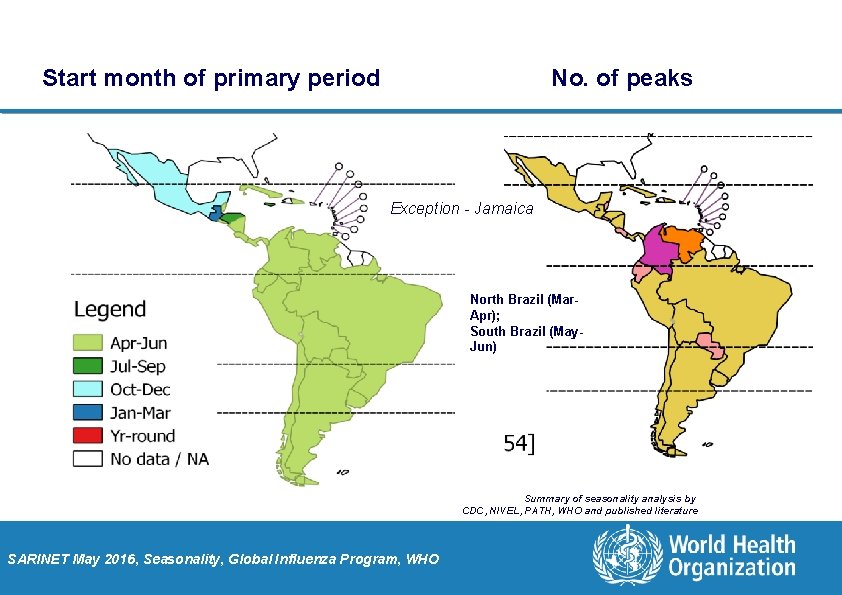
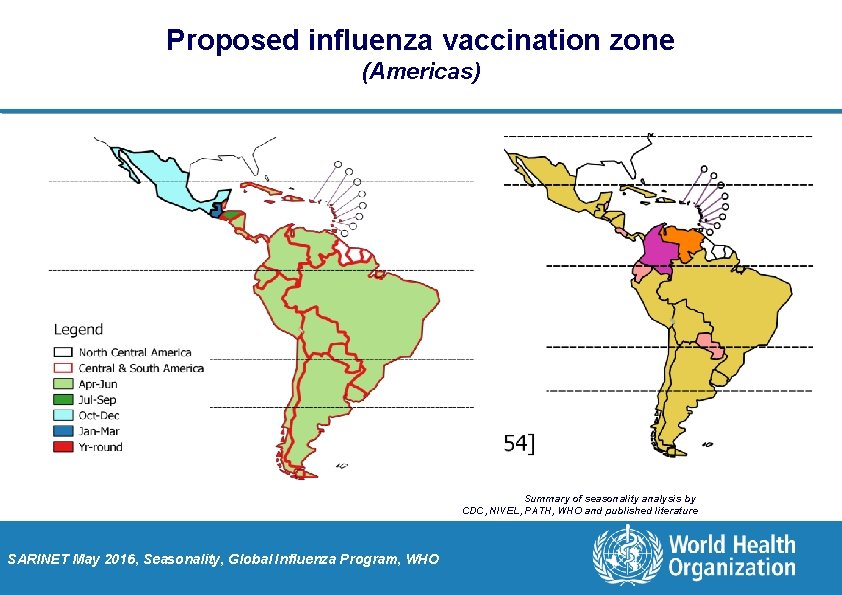
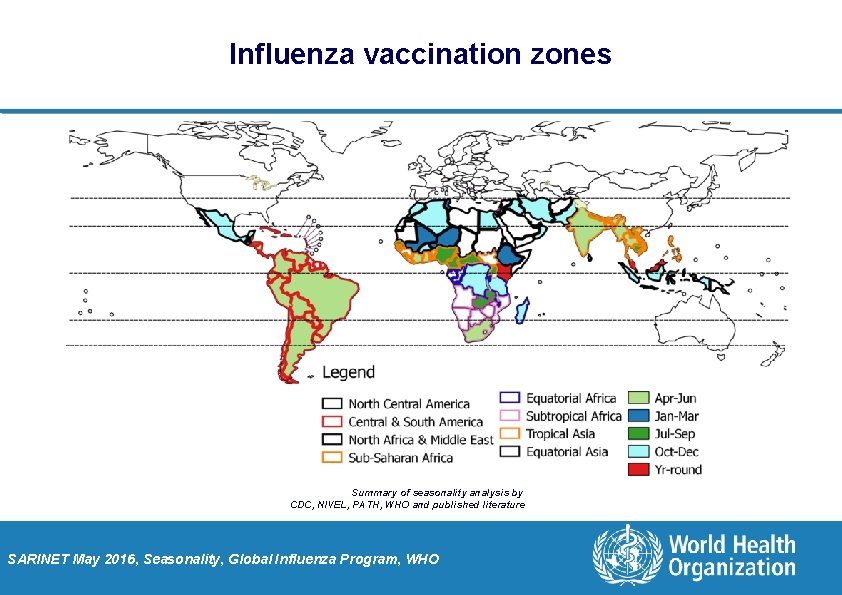
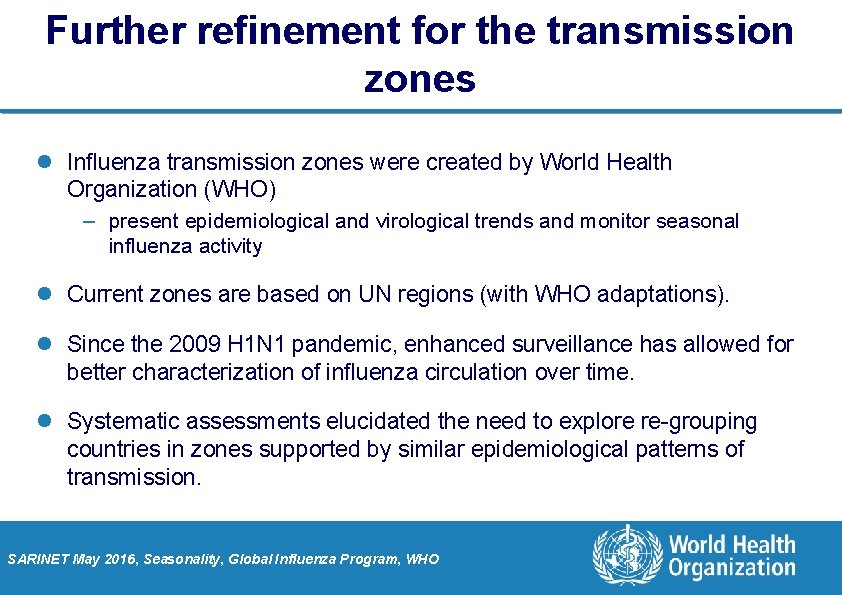
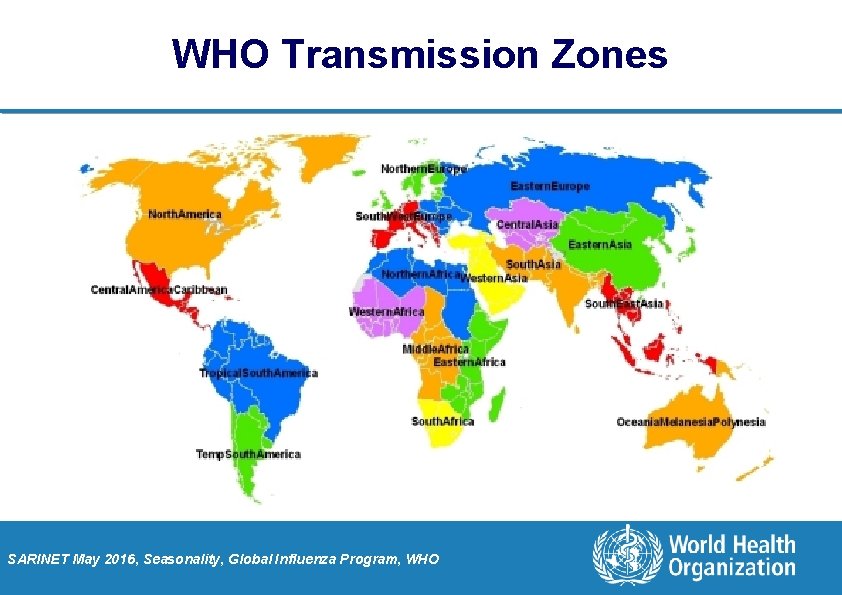
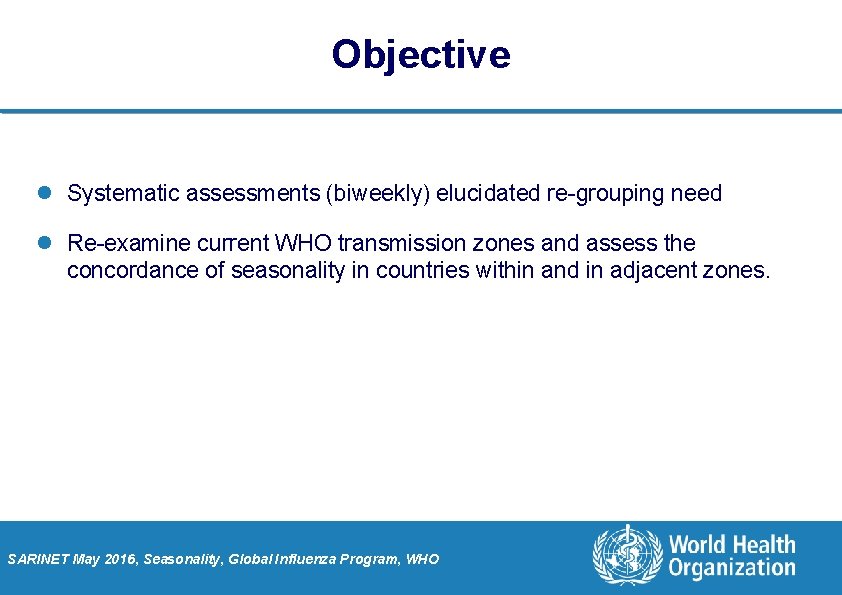
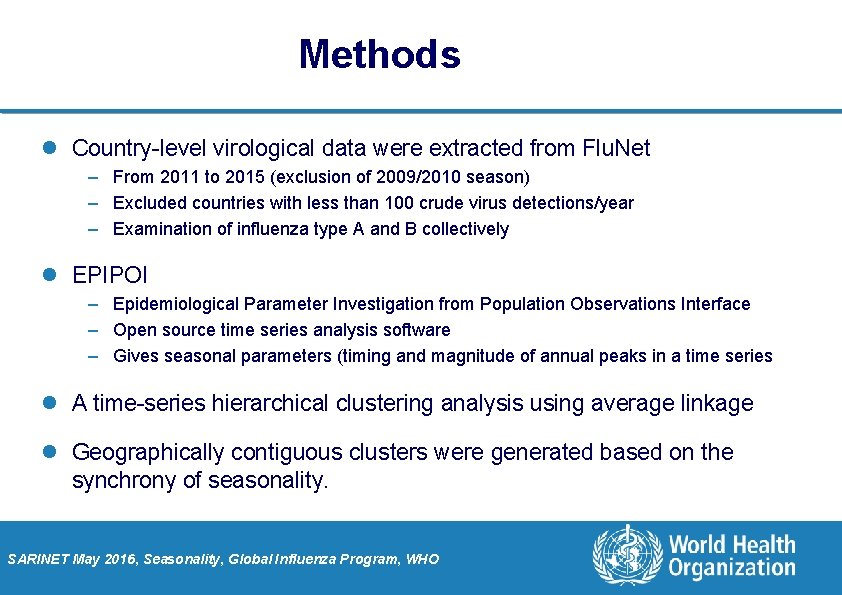
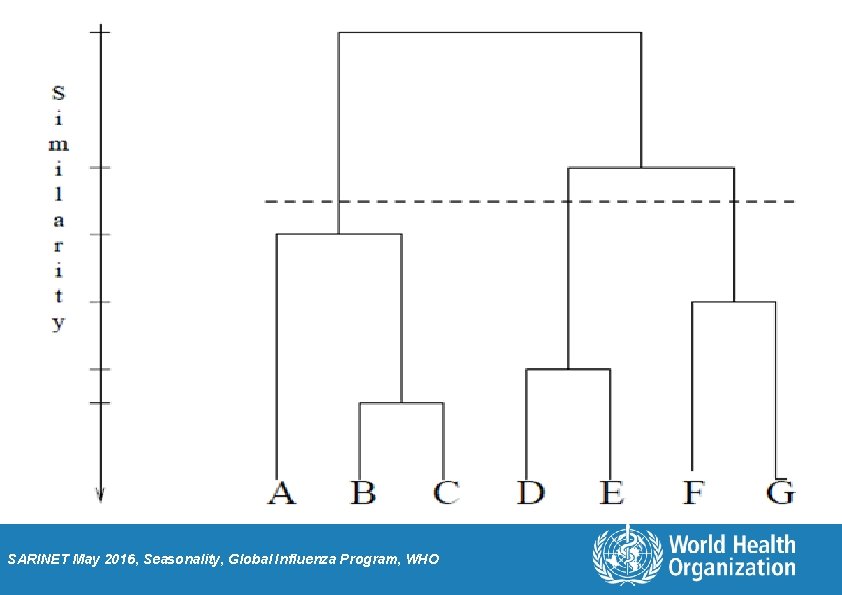
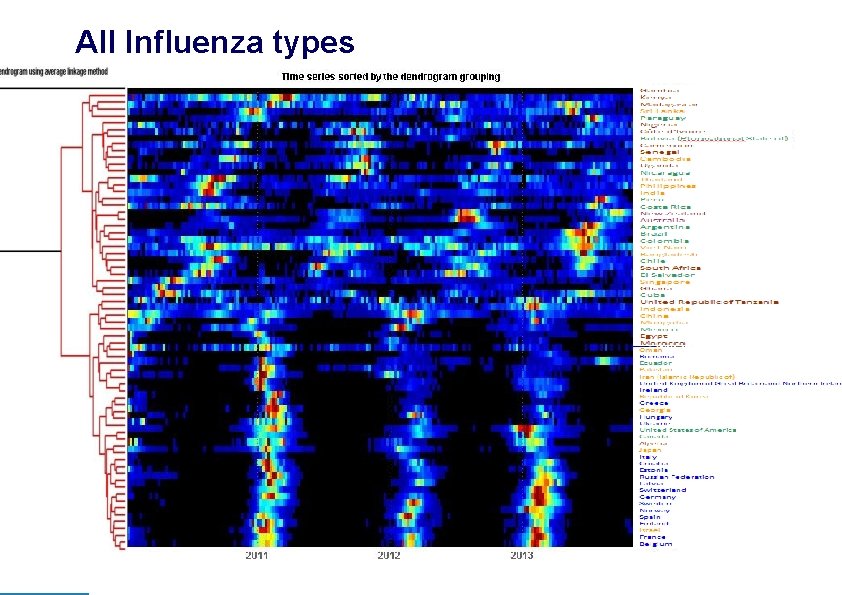
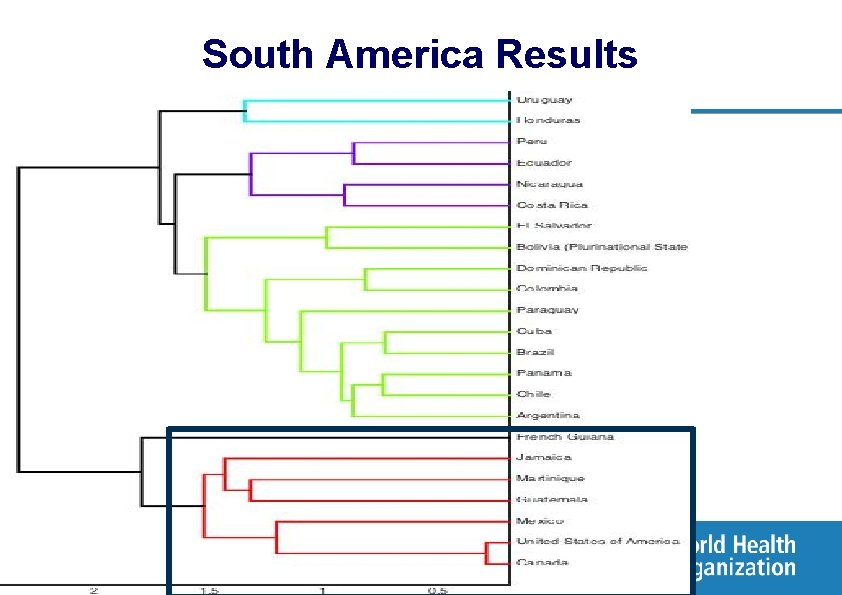
![Results l Southern Asia/South-East Asia – [People’s Republic of Lao, Nepal, India, Bhutan], [Sri Results l Southern Asia/South-East Asia – [People’s Republic of Lao, Nepal, India, Bhutan], [Sri](https://slidetodoc.com/presentation_image_h2/6f23c1ed20119e0607c5469800f87723/image-17.jpg)
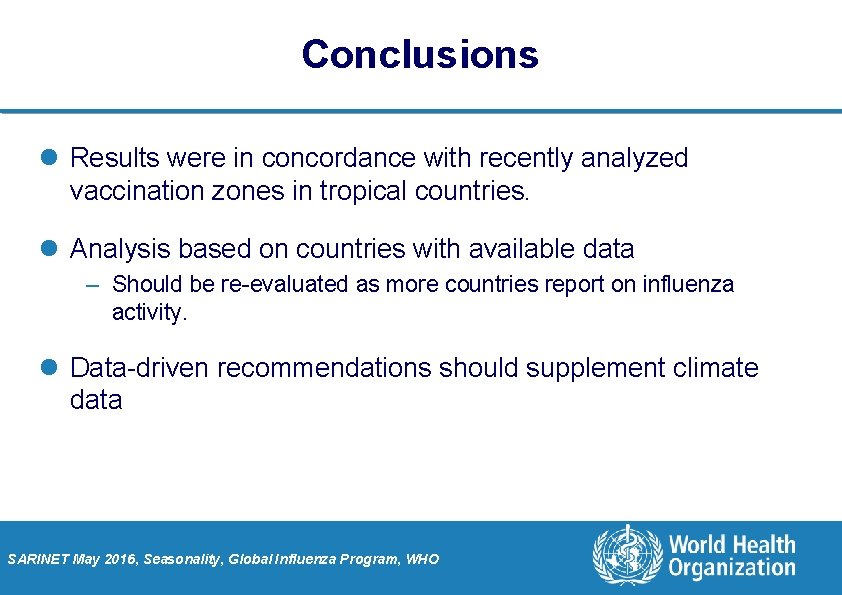
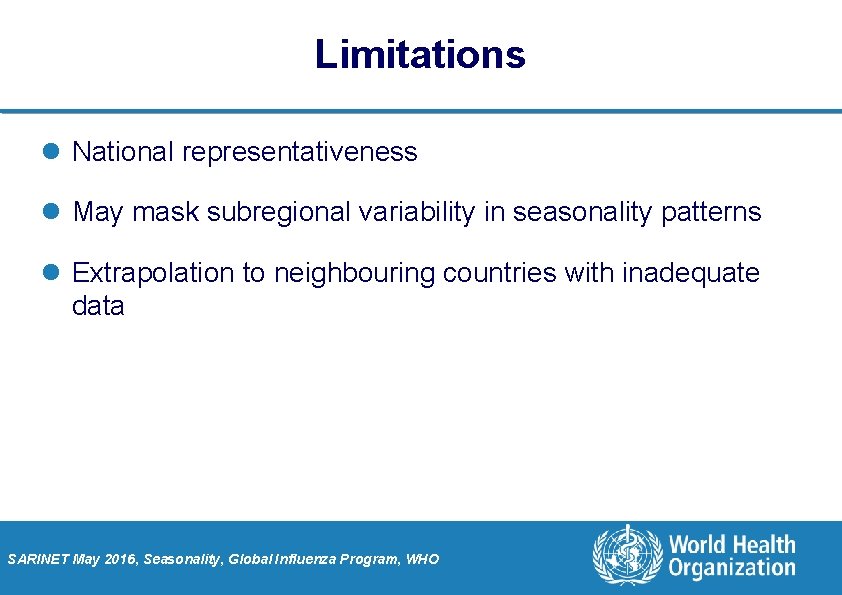
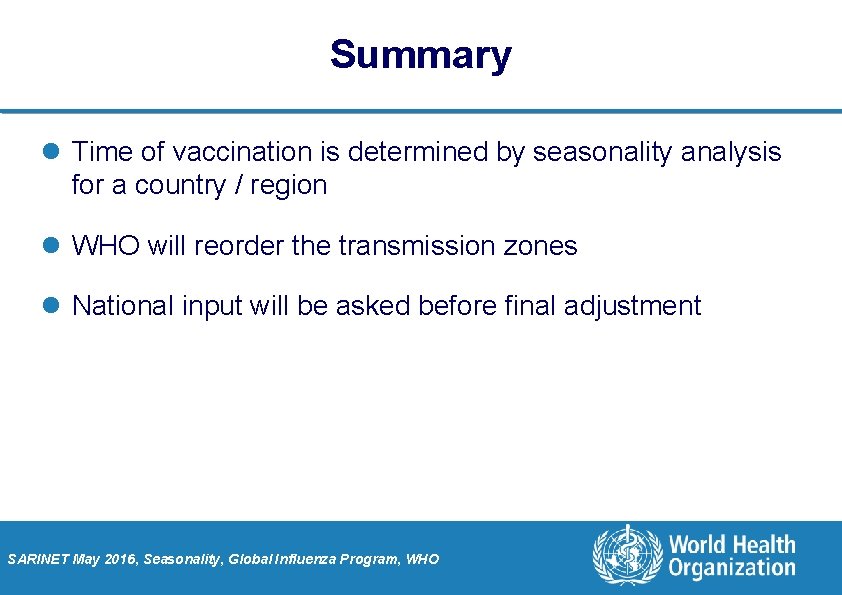
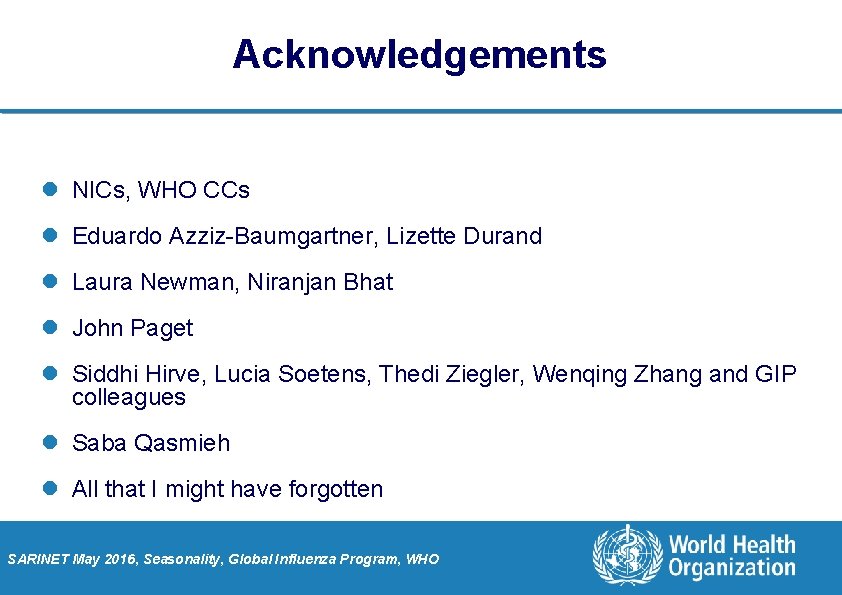
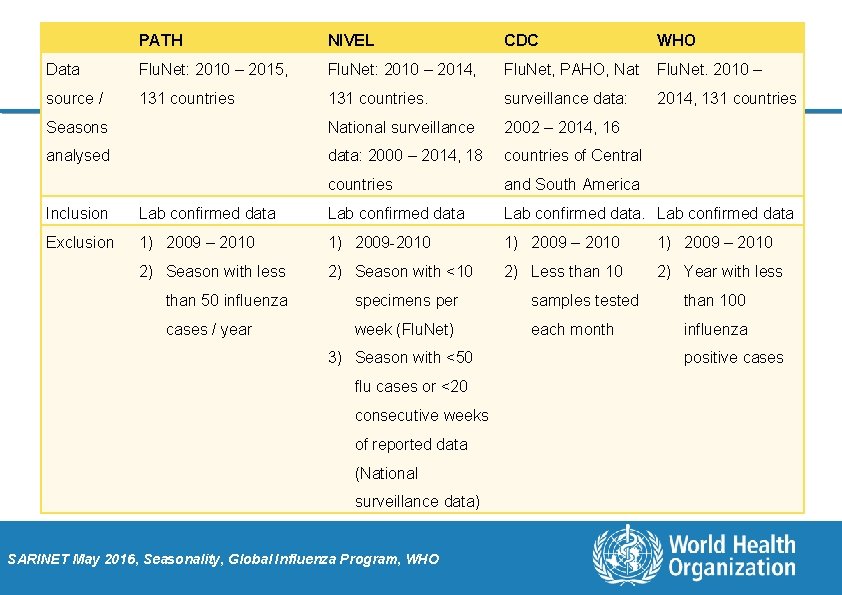
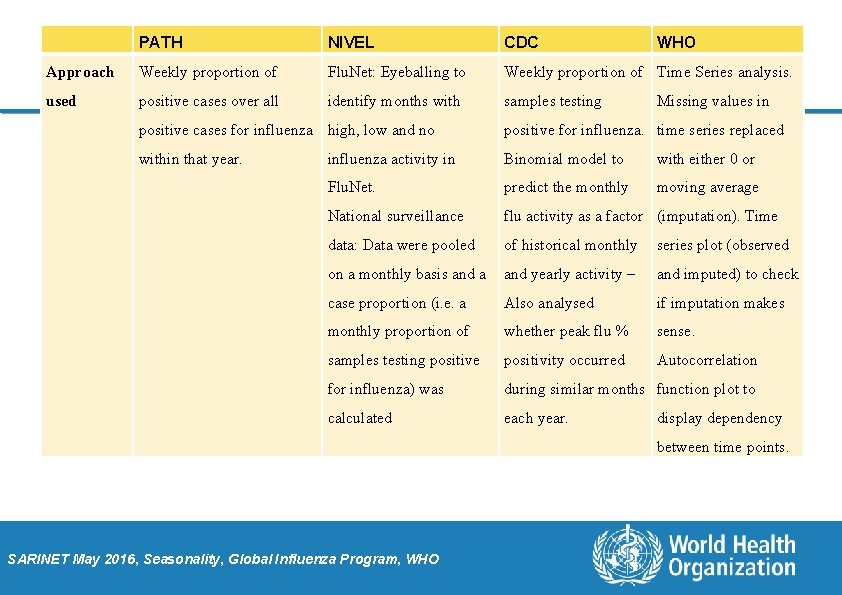
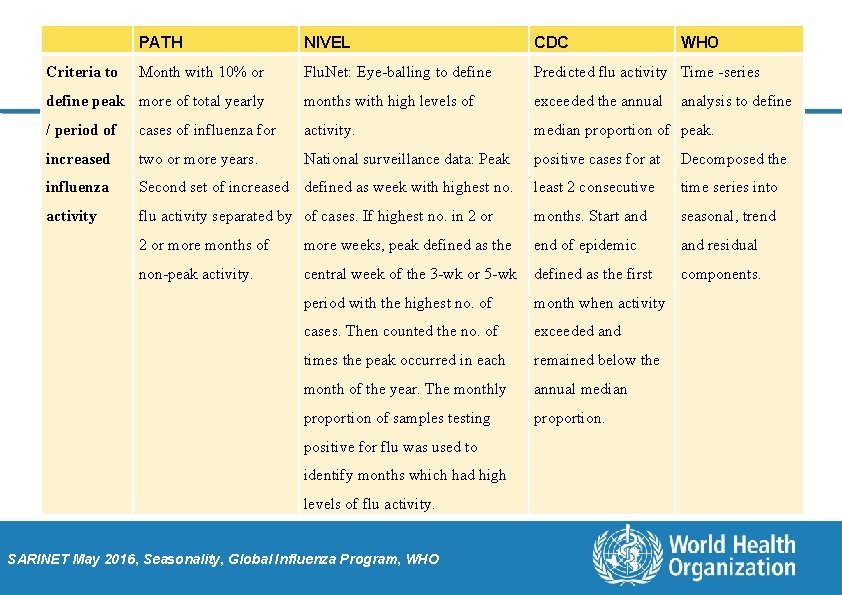
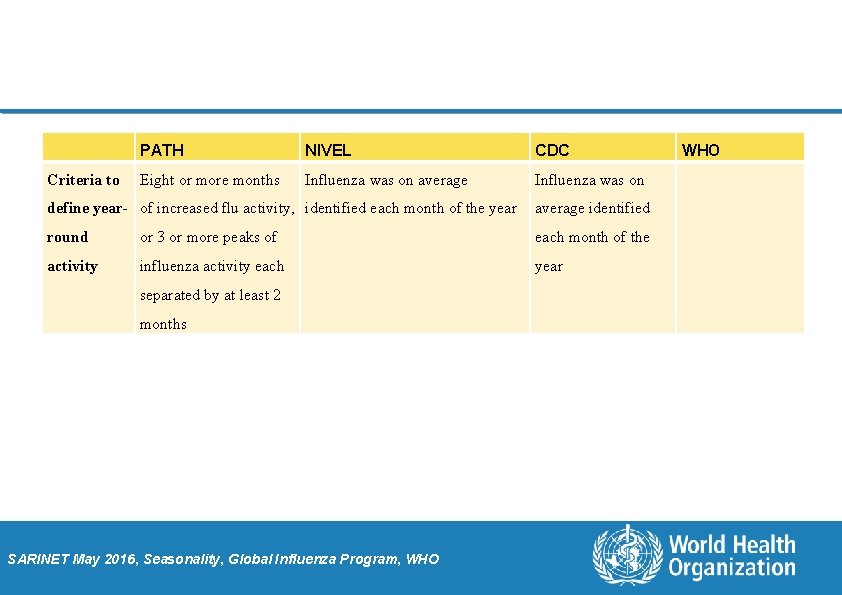
- Slides: 25
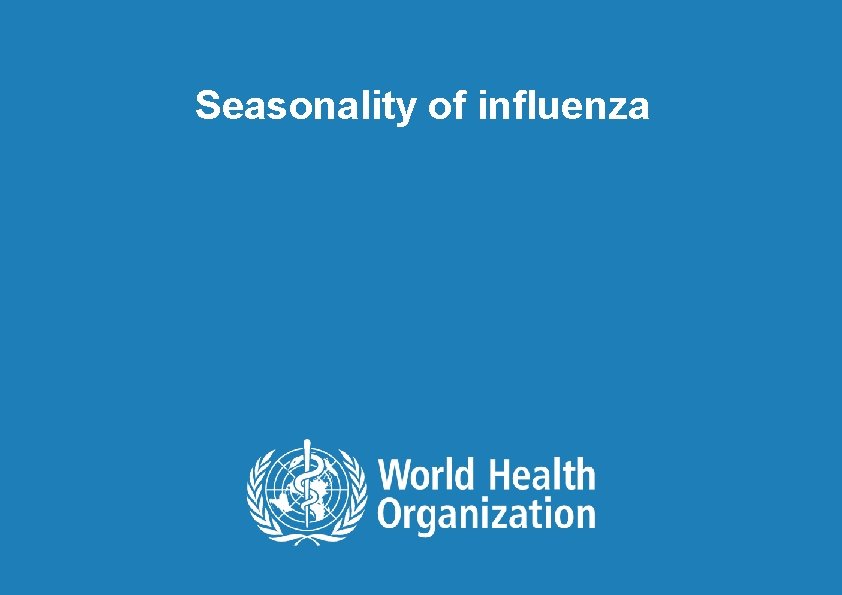
Seasonality of influenza
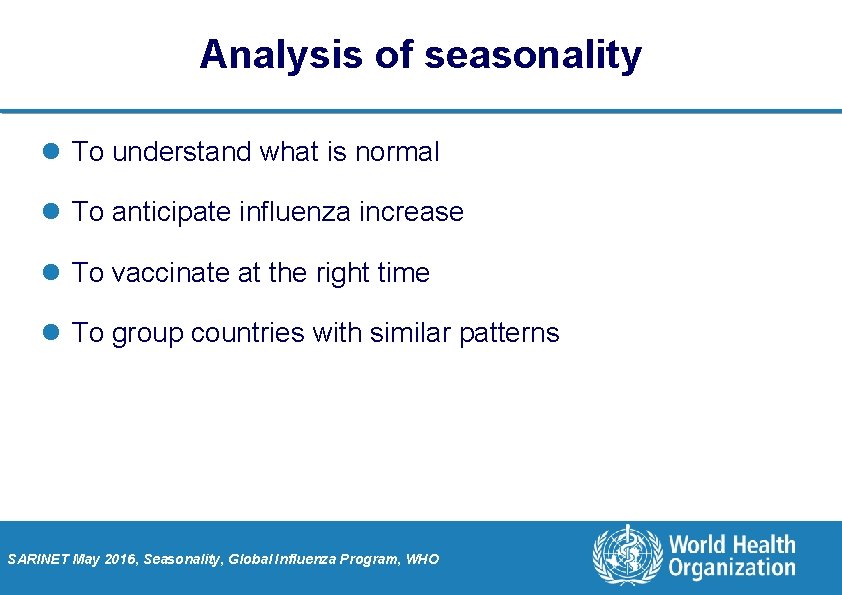
Analysis of seasonality l To understand what is normal l To anticipate influenza increase l To vaccinate at the right time l To group countries with similar patterns SARINET May 2016, Seasonality, Global Influenza Program, WHO
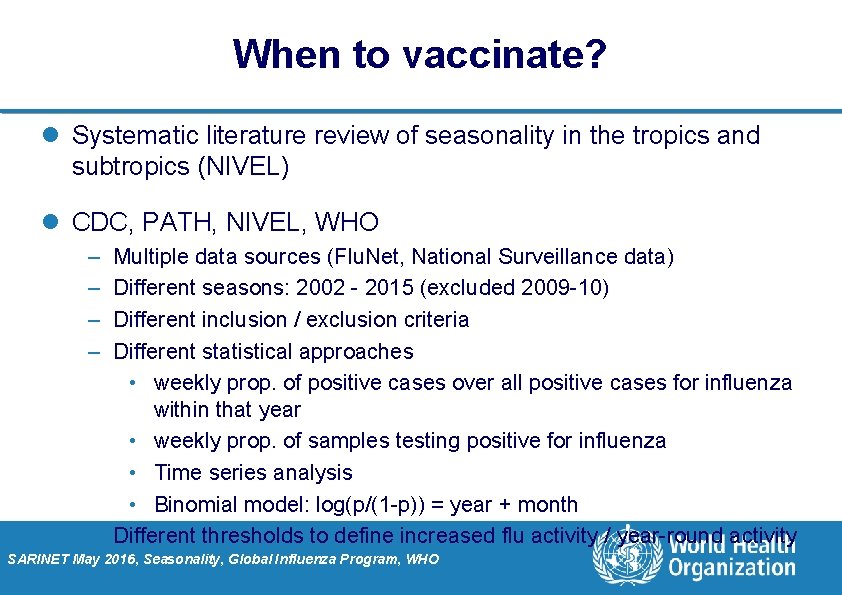
When to vaccinate? l Systematic literature review of seasonality in the tropics and subtropics (NIVEL) l CDC, PATH, NIVEL, WHO – – Multiple data sources (Flu. Net, National Surveillance data) Different seasons: 2002 - 2015 (excluded 2009 -10) Different inclusion / exclusion criteria Different statistical approaches • weekly prop. of positive cases over all positive cases for influenza within that year • weekly prop. of samples testing positive for influenza • Time series analysis • Binomial model: log(p/(1 -p)) = year + month – Different thresholds to define increased flu activity / year-round activity SARINET May 2016, Seasonality, Global Influenza Program, WHO
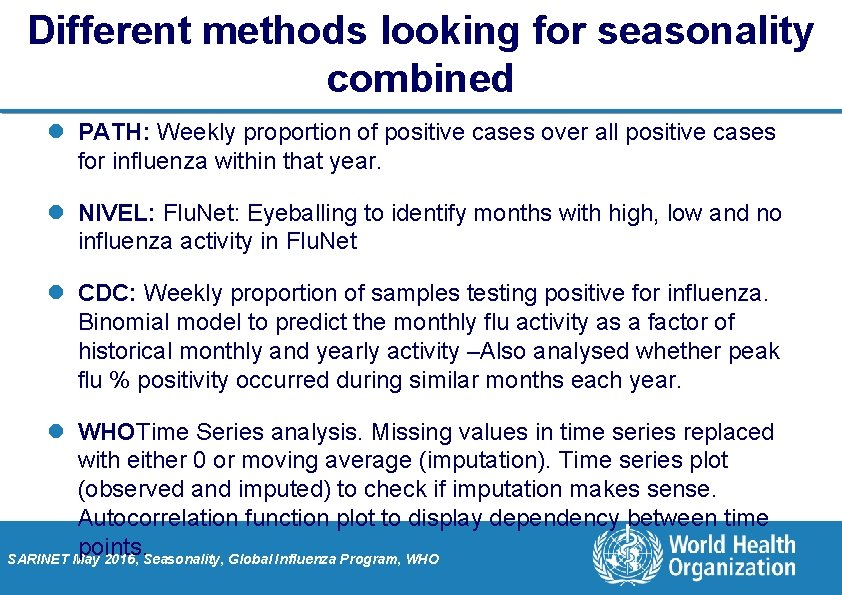
Different methods looking for seasonality combined l PATH: Weekly proportion of positive cases over all positive cases for influenza within that year. l NIVEL: Flu. Net: Eyeballing to identify months with high, low and no influenza activity in Flu. Net l CDC: Weekly proportion of samples testing positive for influenza. Binomial model to predict the monthly flu activity as a factor of historical monthly and yearly activity –Also analysed whether peak flu % positivity occurred during similar months each year. l WHOTime Series analysis. Missing values in time series replaced with either 0 or moving average (imputation). Time series plot (observed and imputed) to check if imputation makes sense. Autocorrelation function plot to display dependency between time points. SARINET May 2016, Seasonality, Global Influenza Program, WHO
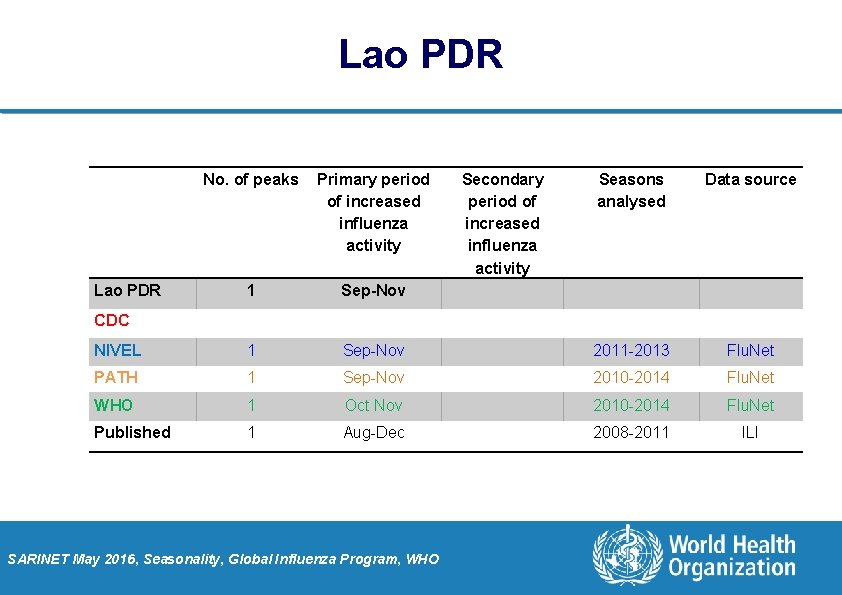
Lao PDR No. of peaks Primary period of increased influenza activity Seasons analysed Data source 1 Sep-Nov NIVEL 1 Sep-Nov 2011 -2013 Flu. Net PATH 1 Sep-Nov 2010 -2014 Flu. Net WHO 1 Oct Nov 2010 -2014 Flu. Net Published 1 Aug-Dec 2008 -2011 ILI Lao PDR Secondary period of increased influenza activity CDC SARINET May 2016, Seasonality, Global Influenza Program, WHO
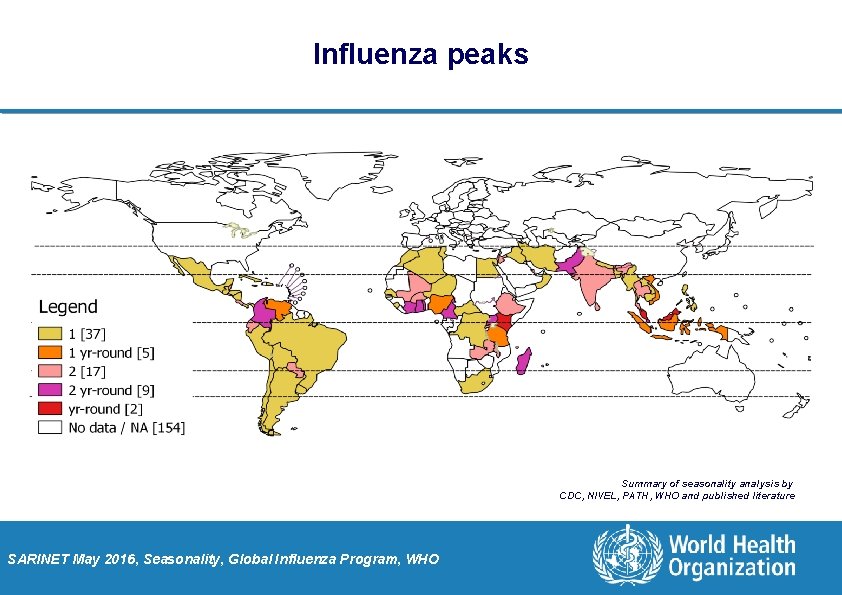
Influenza peaks Summary of seasonality analysis by CDC, NIVEL, PATH, WHO and published literature SARINET May 2016, Seasonality, Global Influenza Program, WHO
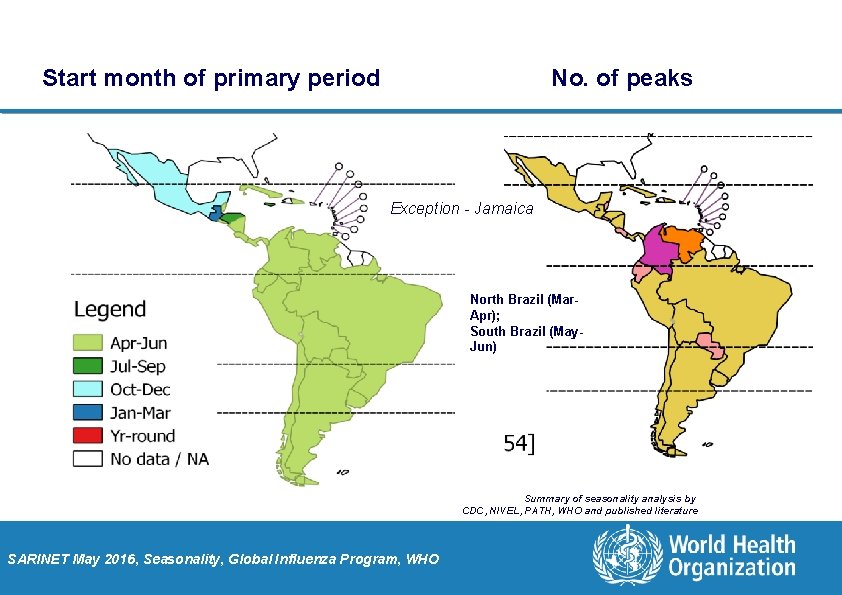
Start month of primary period No. of peaks Exception - Jamaica North Brazil (Mar. Apr); South Brazil (May. Jun) Summary of seasonality analysis by CDC, NIVEL, PATH, WHO and published literature SARINET May 2016, Seasonality, Global Influenza Program, WHO
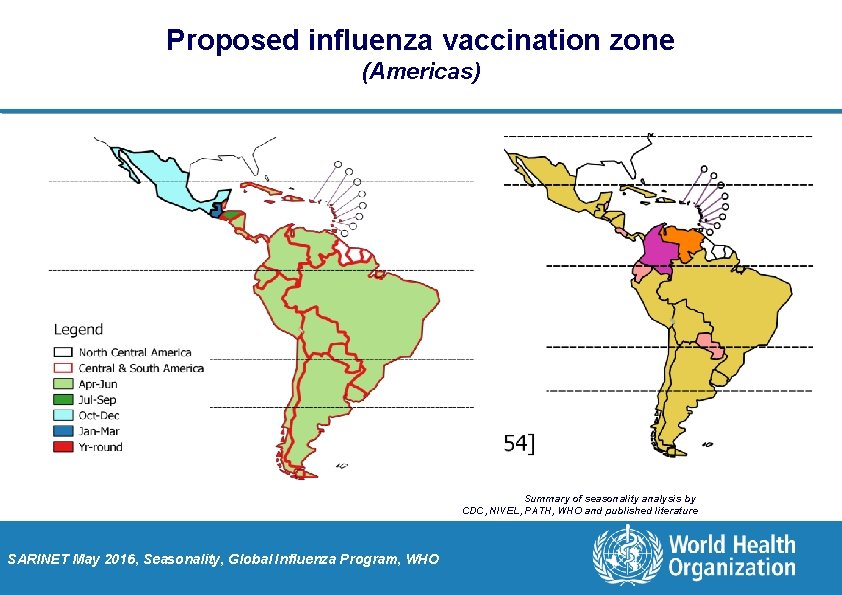
Proposed influenza vaccination zone (Americas) Summary of seasonality analysis by CDC, NIVEL, PATH, WHO and published literature SARINET May 2016, Seasonality, Global Influenza Program, WHO
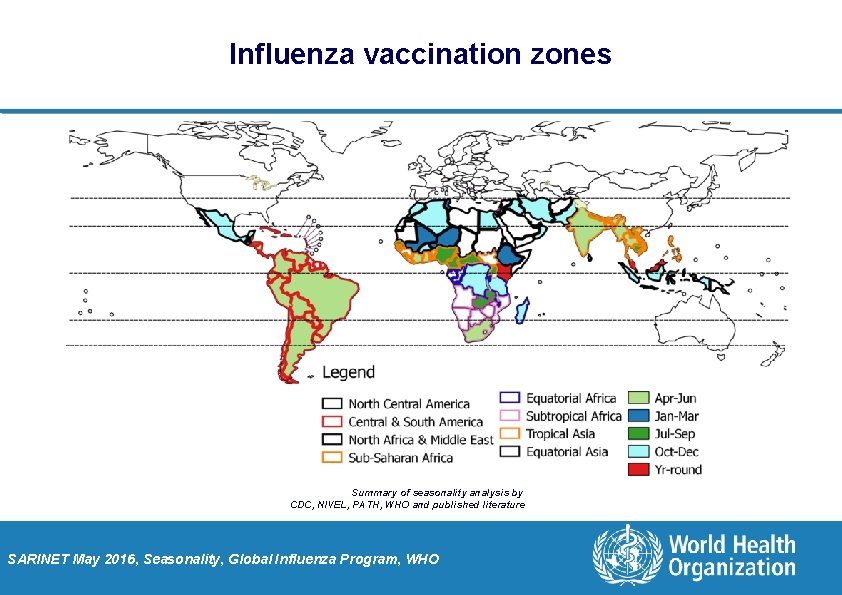
Influenza vaccination zones Summary of seasonality analysis by CDC, NIVEL, PATH, WHO and published literature SARINET May 2016, Seasonality, Global Influenza Program, WHO
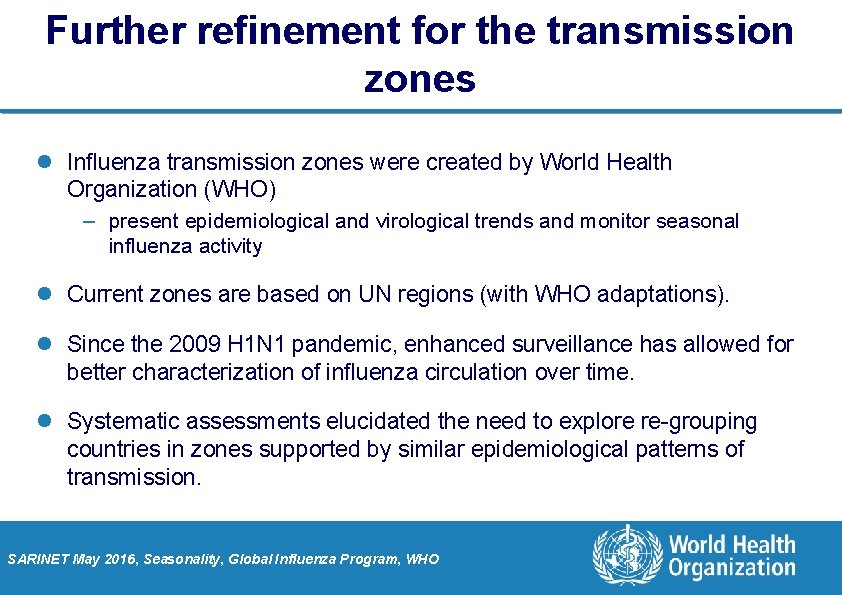
Further refinement for the transmission zones l Influenza transmission zones were created by World Health Organization (WHO) – present epidemiological and virological trends and monitor seasonal influenza activity l Current zones are based on UN regions (with WHO adaptations). l Since the 2009 H 1 N 1 pandemic, enhanced surveillance has allowed for better characterization of influenza circulation over time. l Systematic assessments elucidated the need to explore re-grouping countries in zones supported by similar epidemiological patterns of transmission. SARINET May 2016, Seasonality, Global Influenza Program, WHO
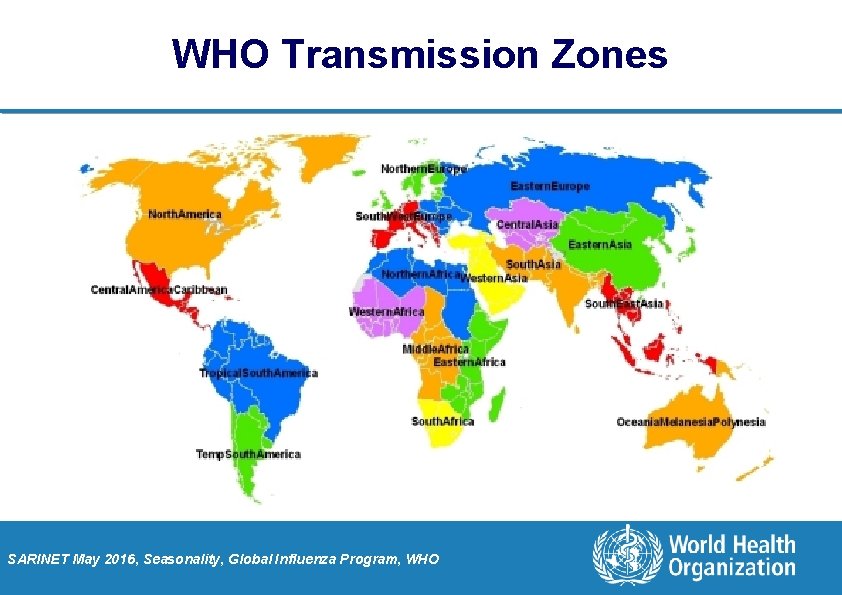
WHO Transmission Zones SARINET May 2016, Seasonality, Global Influenza Program, WHO
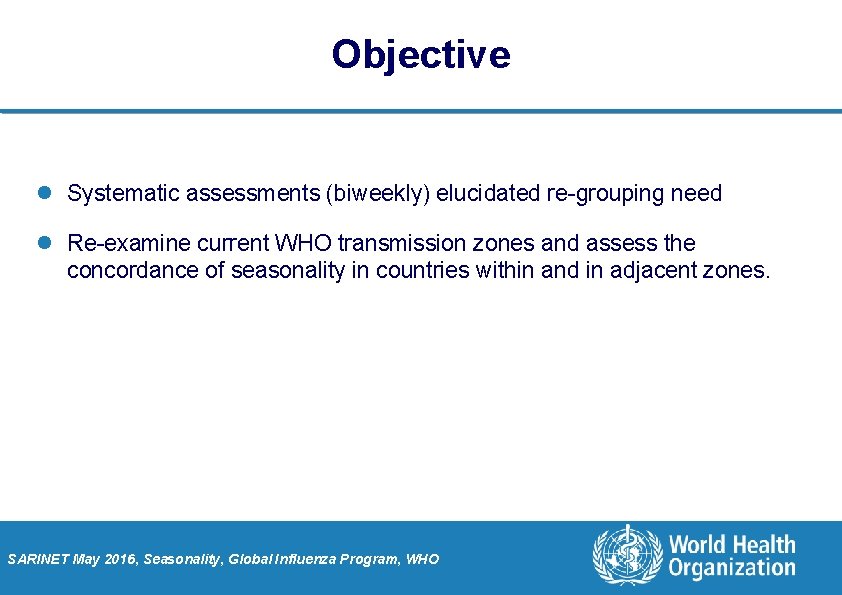
Objective l Systematic assessments (biweekly) elucidated re-grouping need l Re-examine current WHO transmission zones and assess the concordance of seasonality in countries within and in adjacent zones. SARINET May 2016, Seasonality, Global Influenza Program, WHO
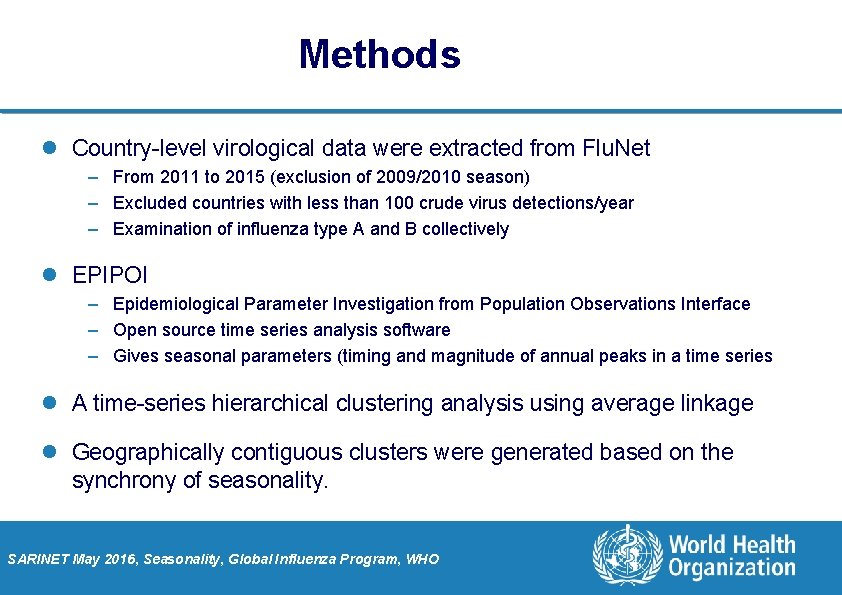
Methods l Country-level virological data were extracted from Flu. Net – From 2011 to 2015 (exclusion of 2009/2010 season) – Excluded countries with less than 100 crude virus detections/year – Examination of influenza type A and B collectively l EPIPOI – Epidemiological Parameter Investigation from Population Observations Interface – Open source time series analysis software – Gives seasonal parameters (timing and magnitude of annual peaks in a time series l A time-series hierarchical clustering analysis using average linkage l Geographically contiguous clusters were generated based on the synchrony of seasonality. SARINET May 2016, Seasonality, Global Influenza Program, WHO
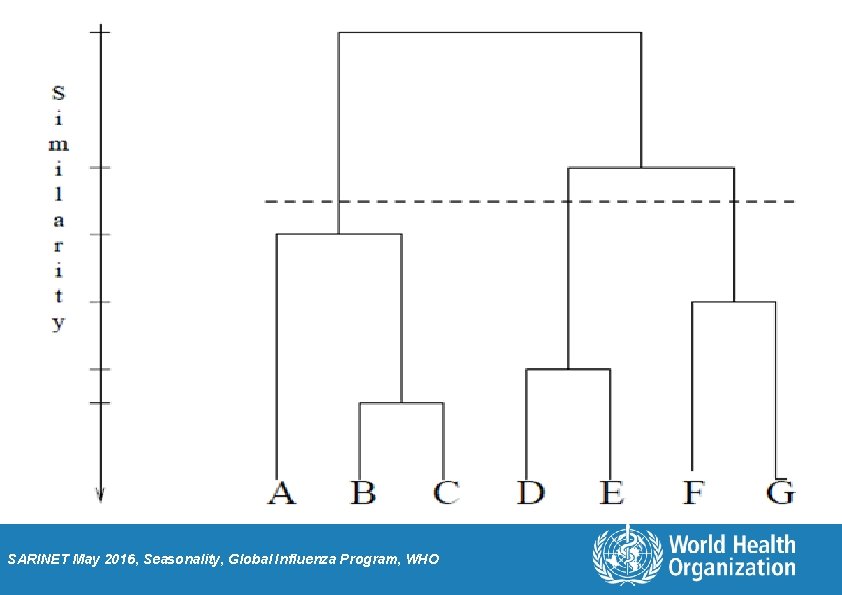
SARINET May 2016, Seasonality, Global Influenza Program, WHO
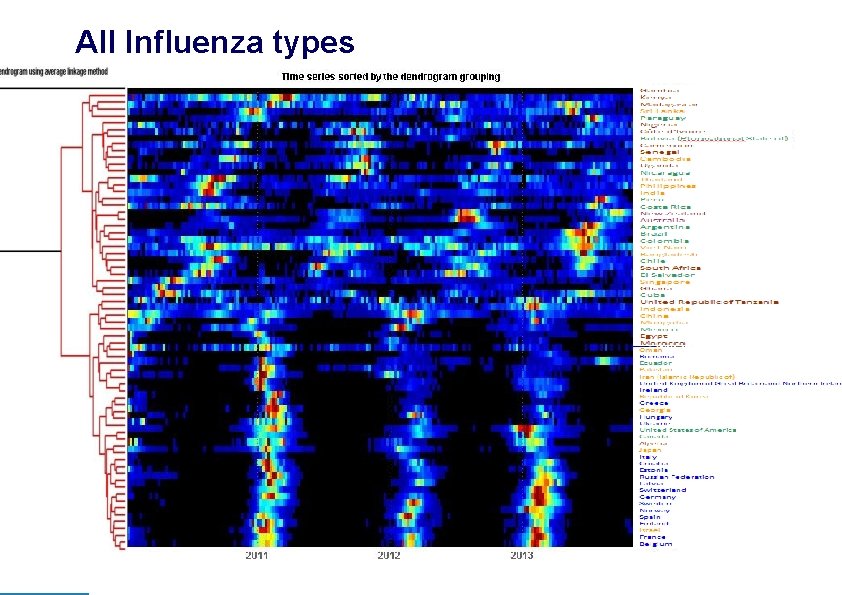
All Influenza types SARINET May 2016, Seasonality, Global Influenza Program, WHO
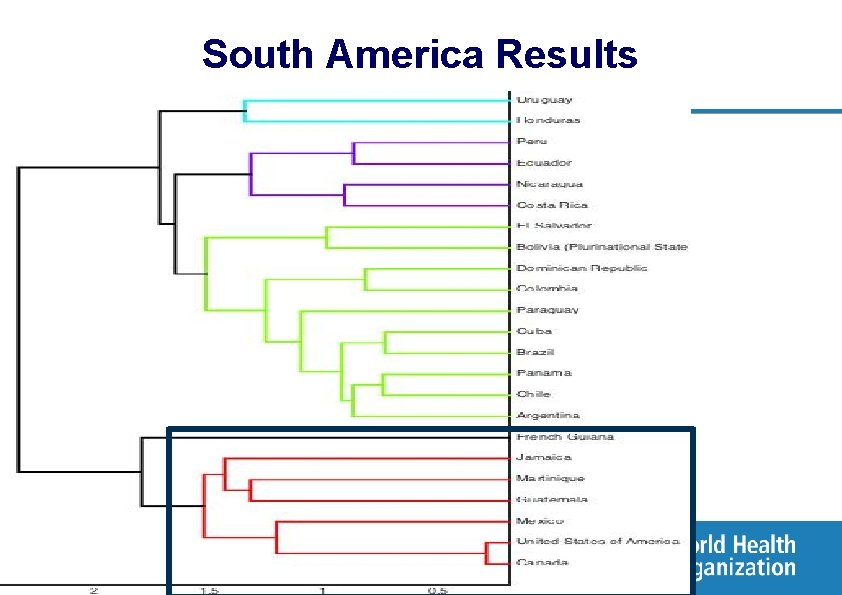
South America Results SARINET May 2016, Seasonality, Global Influenza Program, WHO
![Results l Southern AsiaSouthEast Asia Peoples Republic of Lao Nepal India Bhutan Sri Results l Southern Asia/South-East Asia – [People’s Republic of Lao, Nepal, India, Bhutan], [Sri](https://slidetodoc.com/presentation_image_h2/6f23c1ed20119e0607c5469800f87723/image-17.jpg)
Results l Southern Asia/South-East Asia – [People’s Republic of Lao, Nepal, India, Bhutan], [Sri Lanka, Singapore, Malaysia], [Thailand, Philippines, Cambodia, Viet Nam] l Eastern Asia – [China, Japan, Republic of Korea, Mongolia] l Western Asia – – [Egypt, Pakistan, Iran (Islamic Republic of)] Georgia currently in Western Asia clustered closely with Eastern Europe countries l South America – [Mexico, Jamaica, Guatemala] clustered with countries in North America SARINET May 2016, Seasonality, Global Influenza Program, WHO
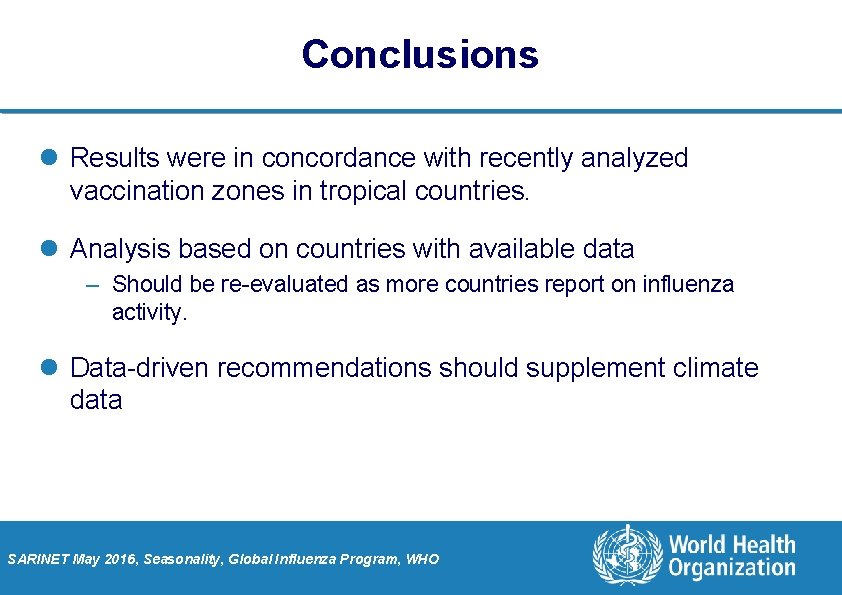
Conclusions l Results were in concordance with recently analyzed vaccination zones in tropical countries. l Analysis based on countries with available data – Should be re-evaluated as more countries report on influenza activity. l Data-driven recommendations should supplement climate data SARINET May 2016, Seasonality, Global Influenza Program, WHO
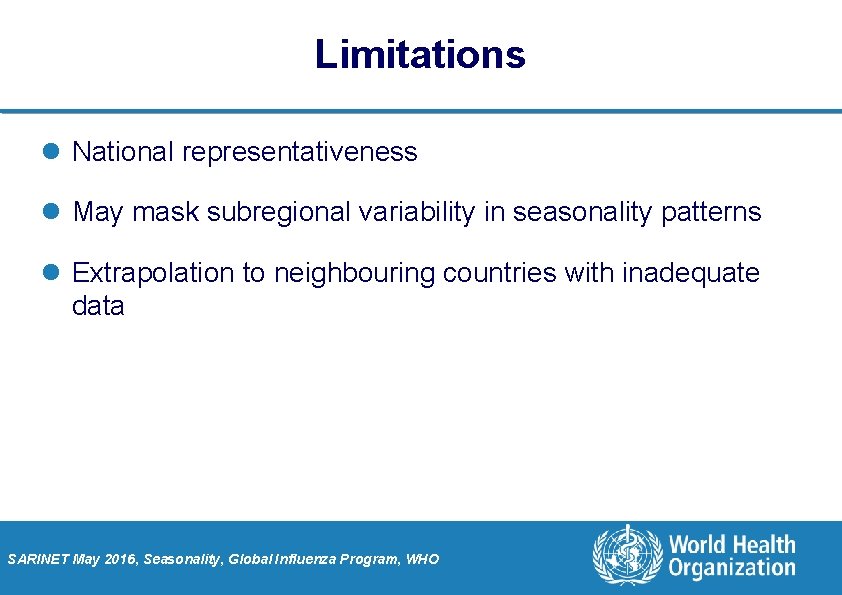
Limitations l National representativeness l May mask subregional variability in seasonality patterns l Extrapolation to neighbouring countries with inadequate data SARINET May 2016, Seasonality, Global Influenza Program, WHO
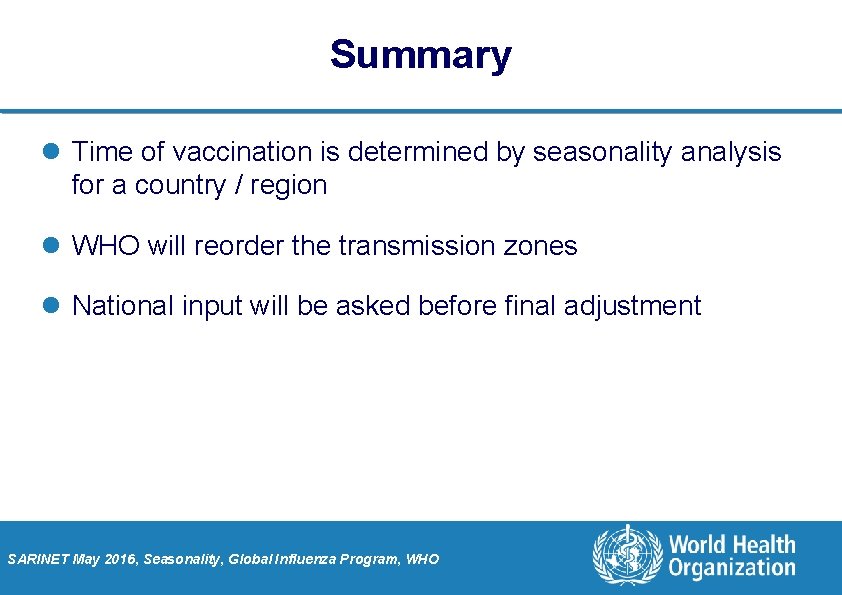
Summary l Time of vaccination is determined by seasonality analysis for a country / region l WHO will reorder the transmission zones l National input will be asked before final adjustment SARINET May 2016, Seasonality, Global Influenza Program, WHO
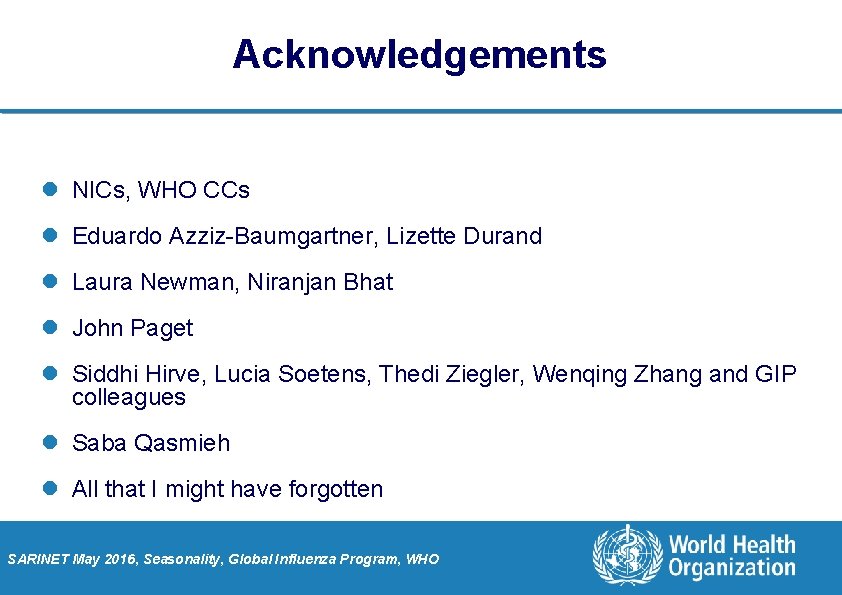
Acknowledgements l NICs, WHO CCs l Eduardo Azziz-Baumgartner, Lizette Durand l Laura Newman, Niranjan Bhat l John Paget l Siddhi Hirve, Lucia Soetens, Thedi Ziegler, Wenqing Zhang and GIP colleagues l Saba Qasmieh l All that I might have forgotten SARINET May 2016, Seasonality, Global Influenza Program, WHO
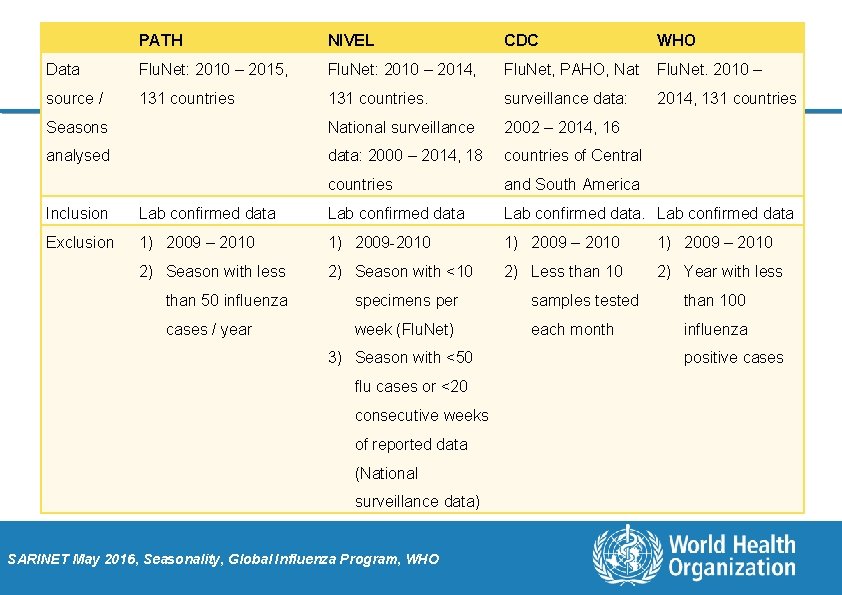
PATH NIVEL CDC WHO Data Flu. Net: 2010 – 2015, Flu. Net: 2010 – 2014, Flu. Net, PAHO, Nat Flu. Net. 2010 – source / 131 countries. surveillance data: 2014, 131 countries Seasons National surveillance 2002 – 2014, 16 analysed data: 2000 – 2014, 18 countries of Central countries and South America Inclusion Lab confirmed data Exclusion 1) 2009 – 2010 1) 2009 -2010 1) 2009 – 2010 2) Season with less 2) Season with <10 2) Less than 10 2) Year with less than 50 influenza specimens per samples tested than 100 cases / year week (Flu. Net) each month influenza 3) Season with <50 flu cases or <20 consecutive weeks of reported data (National surveillance data) SARINET May 2016, Seasonality, Global Influenza Program, WHO positive cases
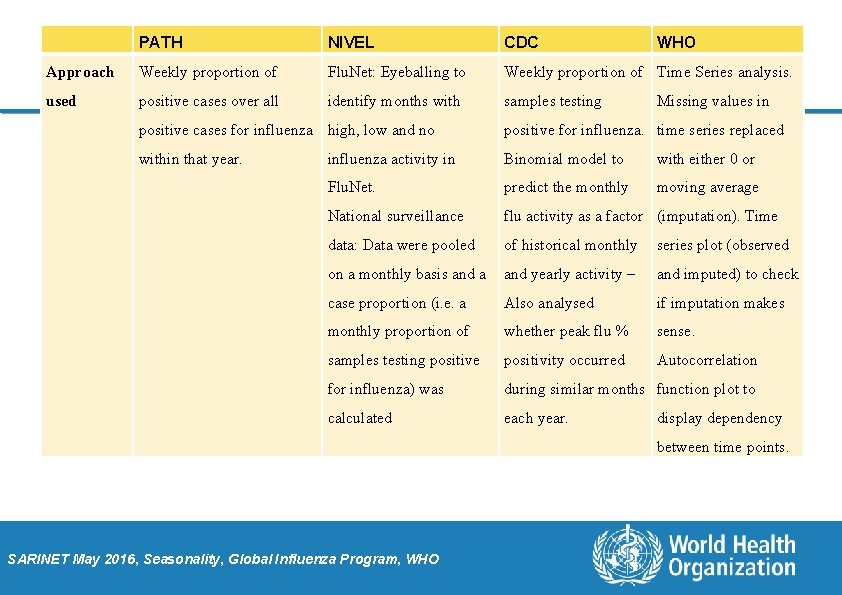
PATH NIVEL CDC WHO Approach Weekly proportion of Flu. Net: Eyeballing to Weekly proportion of Time Series analysis. used positive cases over all identify months with samples testing Missing values in positive cases for influenza high, low and no positive for influenza. time series replaced within that year. influenza activity in Binomial model to with either 0 or Flu. Net. predict the monthly moving average National surveillance flu activity as a factor (imputation). Time data: Data were pooled of historical monthly series plot (observed on a monthly basis and a and yearly activity – and imputed) to check case proportion (i. e. a Also analysed if imputation makes monthly proportion of whether peak flu % sense. samples testing positive positivity occurred Autocorrelation for influenza) was during similar months function plot to calculated each year. display dependency between time points. SARINET May 2016, Seasonality, Global Influenza Program, WHO
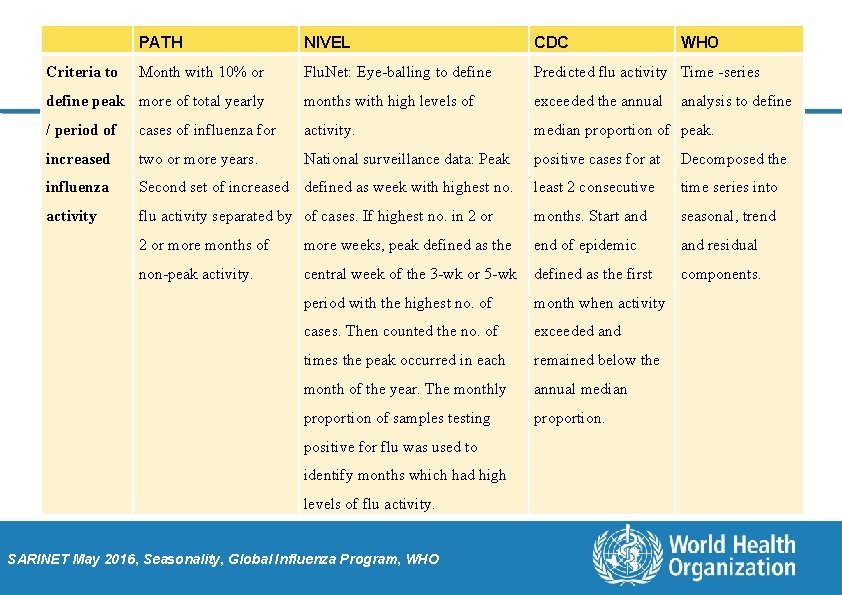
PATH NIVEL CDC Month with 10% or Flu. Net: Eye-balling to define Predicted flu activity Time -series define peak more of total yearly months with high levels of exceeded the annual / period of cases of influenza for activity. median proportion of peak. increased two or more years. National surveillance data: Peak positive cases for at Decomposed the influenza Second set of increased defined as week with highest no. least 2 consecutive time series into activity flu activity separated by of cases. If highest no. in 2 or months. Start and seasonal, trend 2 or more months of more weeks, peak defined as the end of epidemic and residual non-peak activity. central week of the 3 -wk or 5 -wk defined as the first components. period with the highest no. of month when activity cases. Then counted the no. of exceeded and times the peak occurred in each remained below the month of the year. The monthly annual median proportion of samples testing proportion. Criteria to positive for flu was used to identify months which had high levels of flu activity. SARINET May 2016, Seasonality, Global Influenza Program, WHO analysis to define
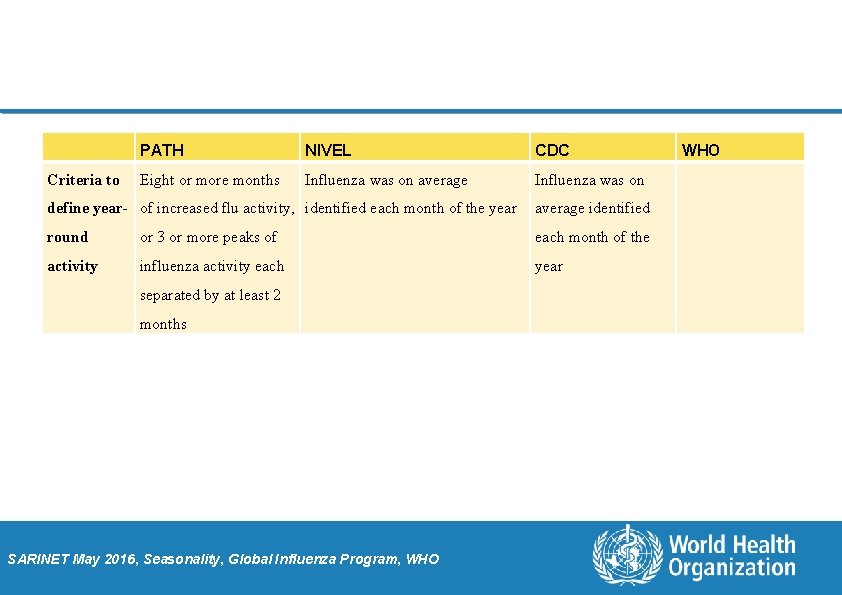
Criteria to PATH NIVEL CDC Eight or more months Influenza was on average Influenza was on define year- of increased flu activity, identified each month of the year average identified round or 3 or more peaks of each month of the activity influenza activity each year separated by at least 2 months SARINET May 2016, Seasonality, Global Influenza Program, WHO
The great influenza rhetorical analysis
Gold stock seasonality
Modulo termico materozza
Causative organism
Influenza
Influenza
Stomach flu vs influenza
Is influenza a airborne disease
Rimantidina
Influenza vaccine dosage chart 2019-2020
Influenza ww1
Influenza virus replication
Low pathogenic avian influenza
What is structured analysis?
Autohotkey obfuscator
Difference between content and discourse analysis
Differences between contrastive analysis and error analysis
Contrastive analysis error analysis and interlanguage
What is fact analysis
Difference between task analysis and content analysis
Transactions in transactional analysis
Structured vs object oriented approach
Syntax and lexical analysis
Content analysis secondary data
Feasibility in system analysis and design
Difference between factor analysis and cluster analysis