MSc Methods XX YY Dr Mathias Mat Disney
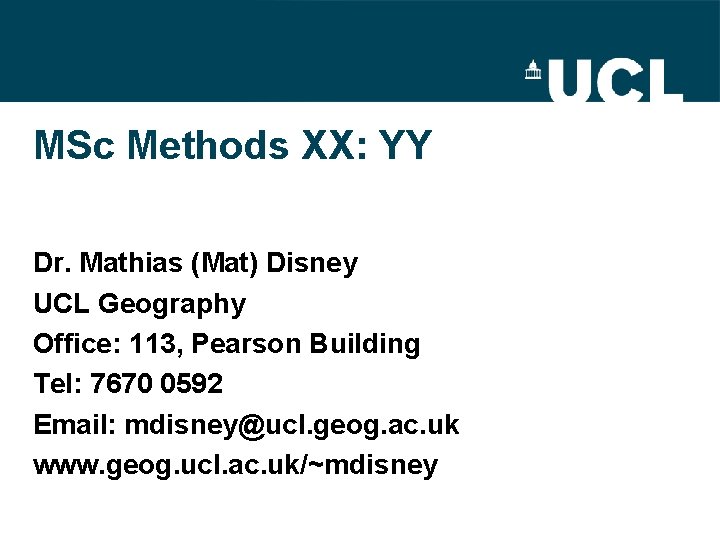
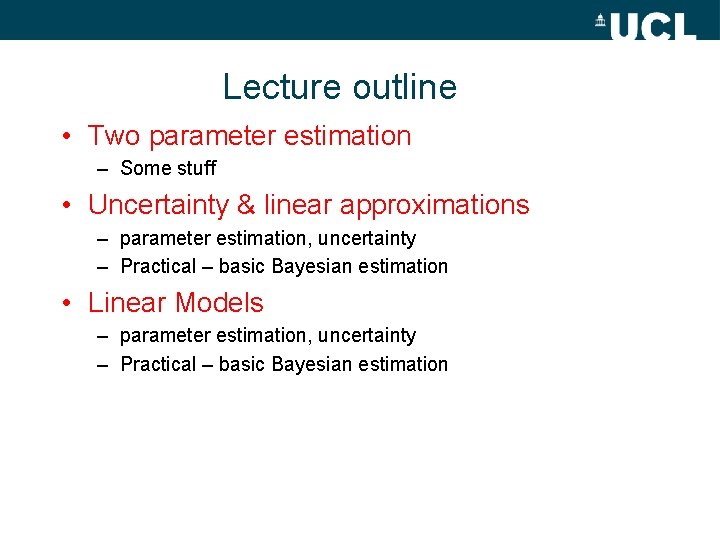
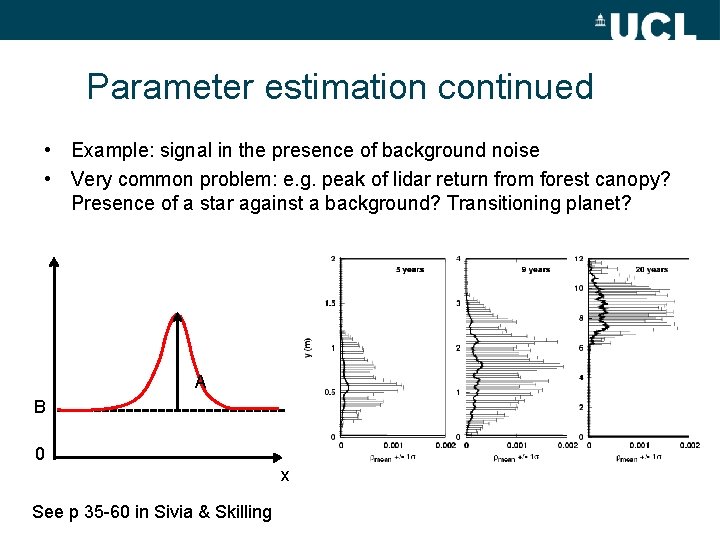
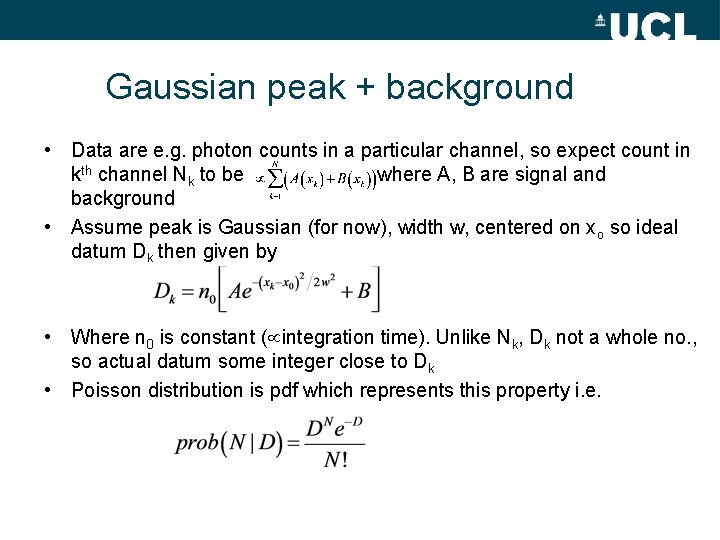
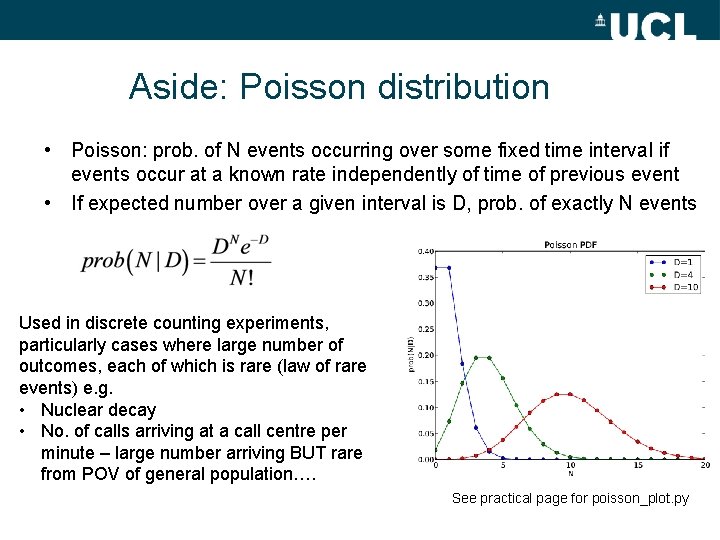
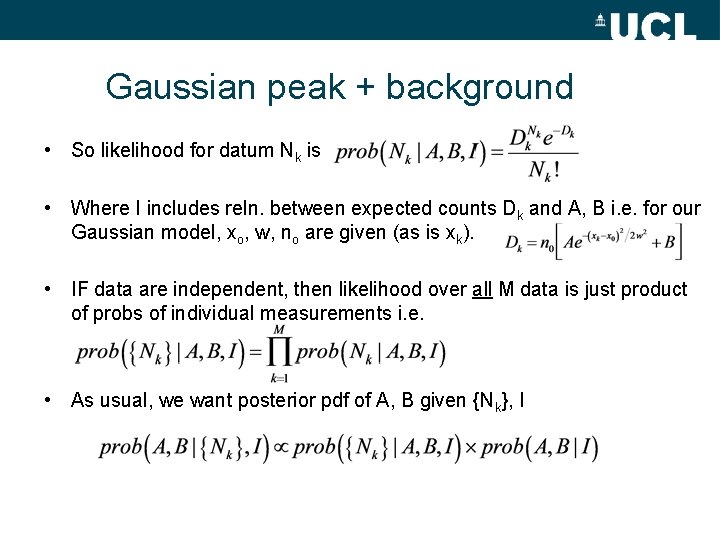
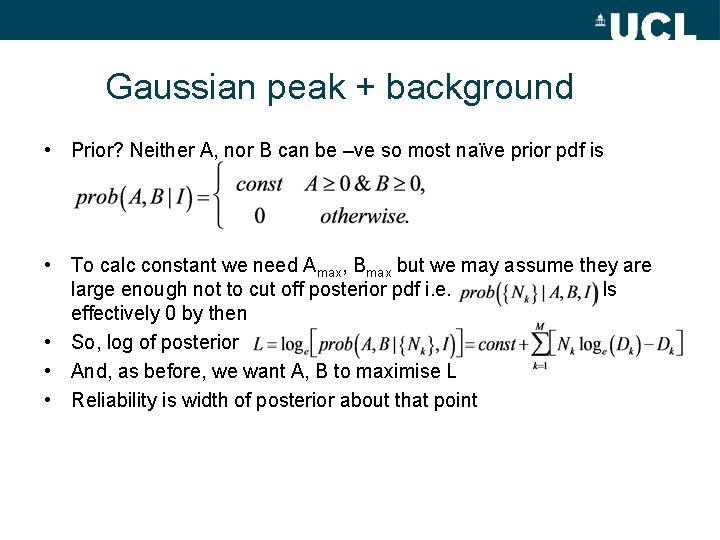
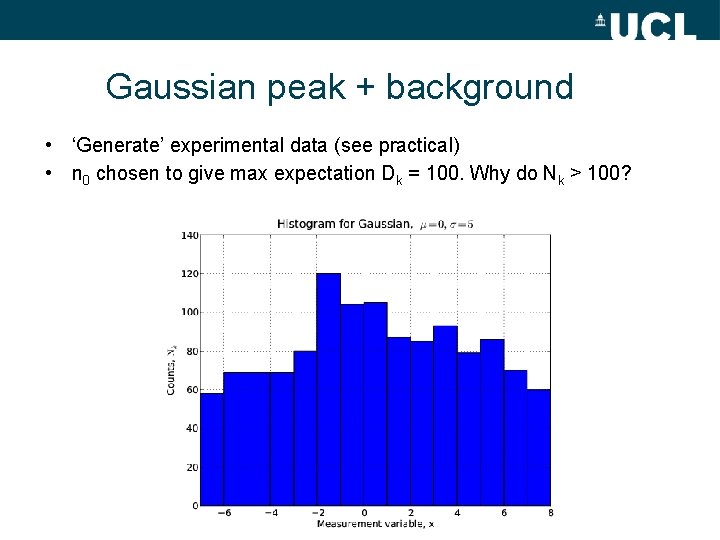
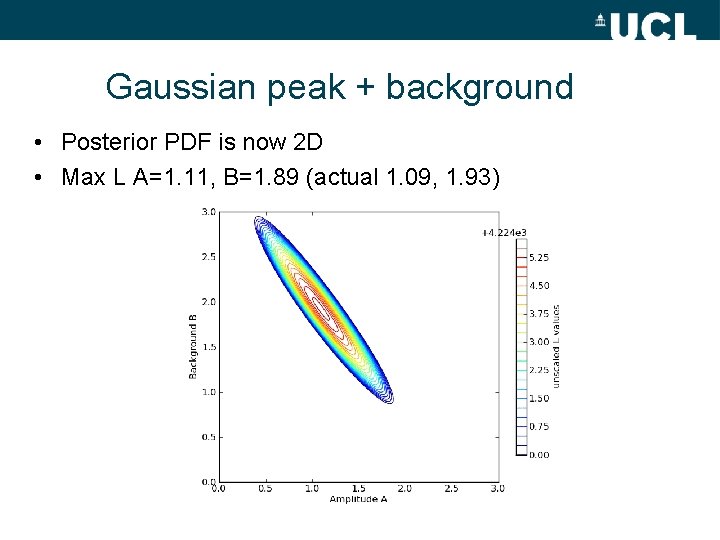
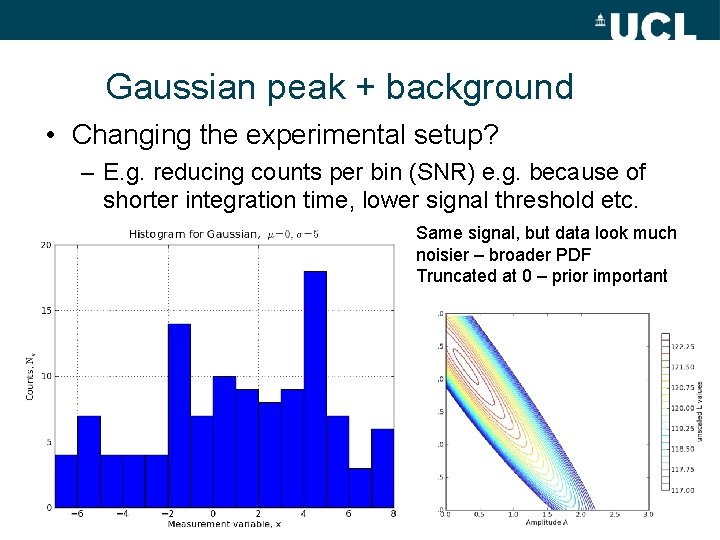
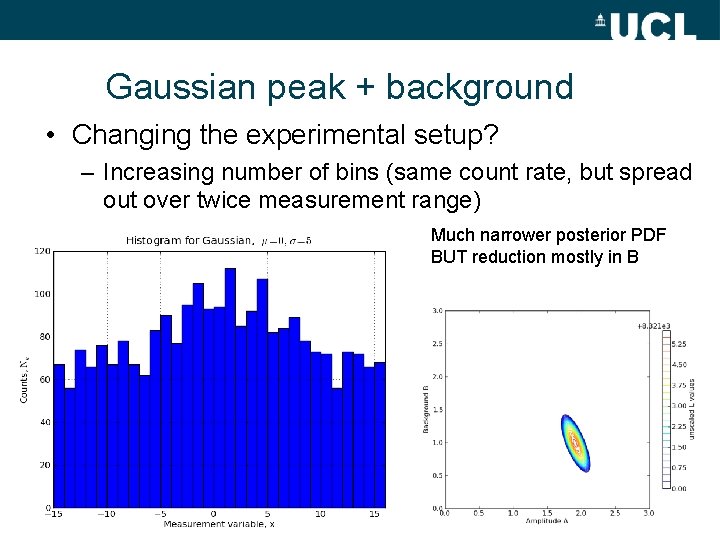
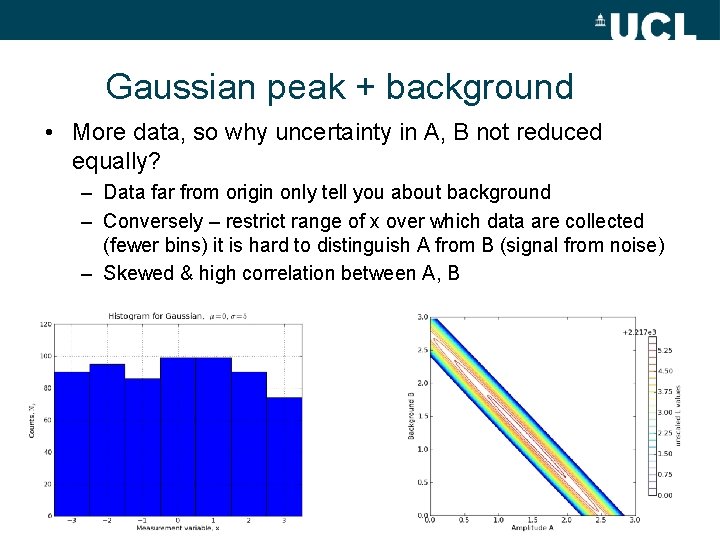
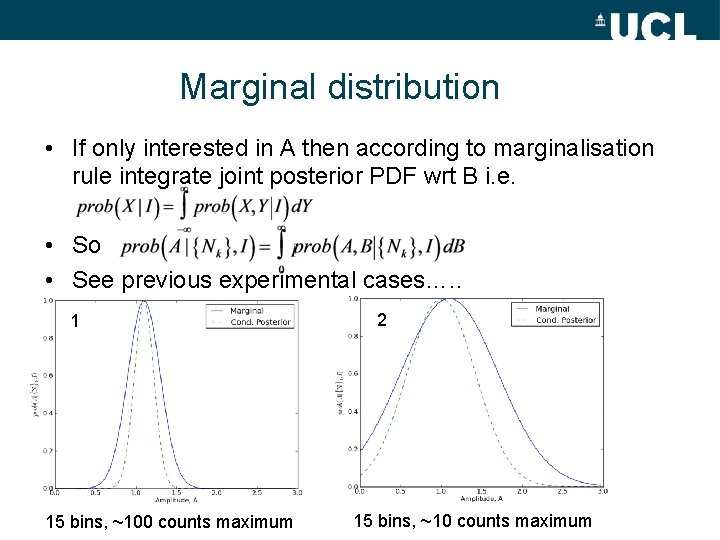
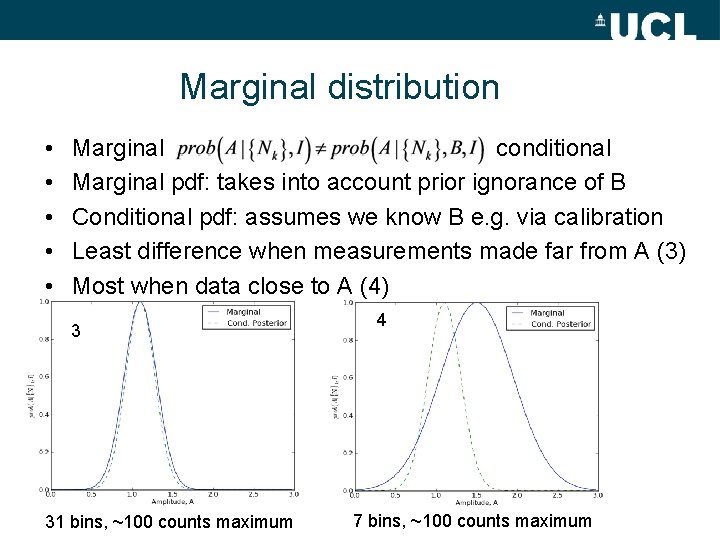
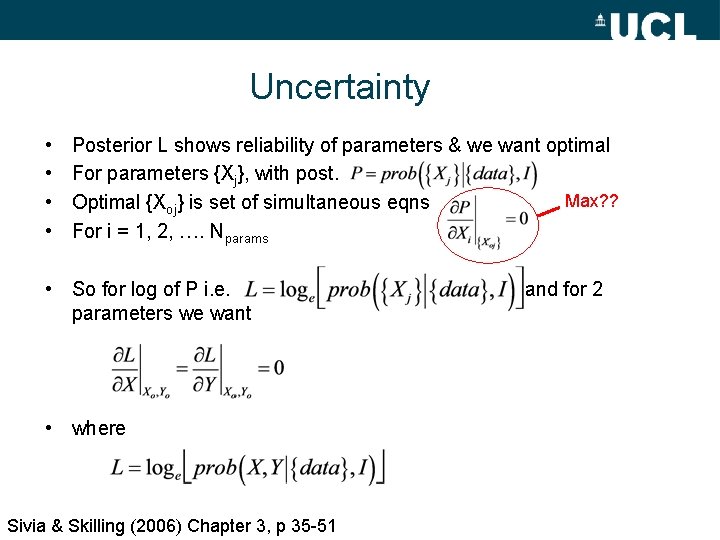
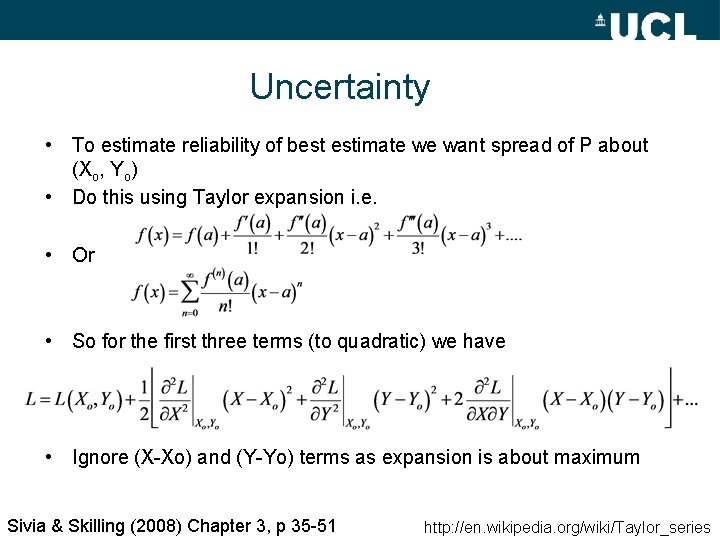
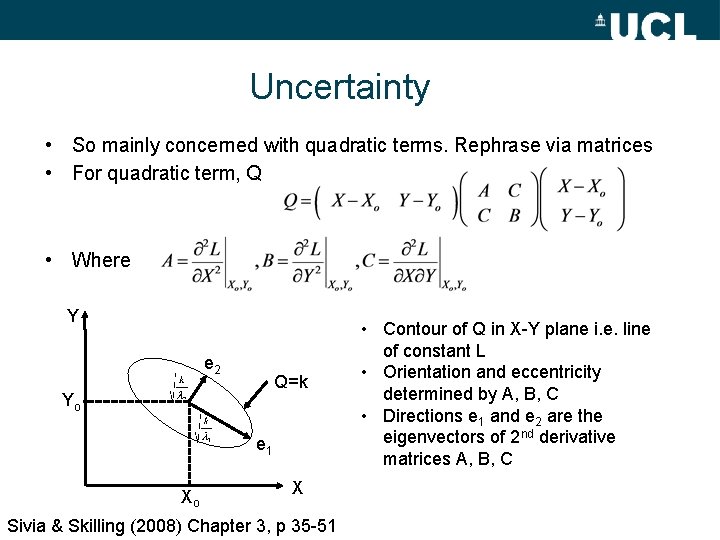
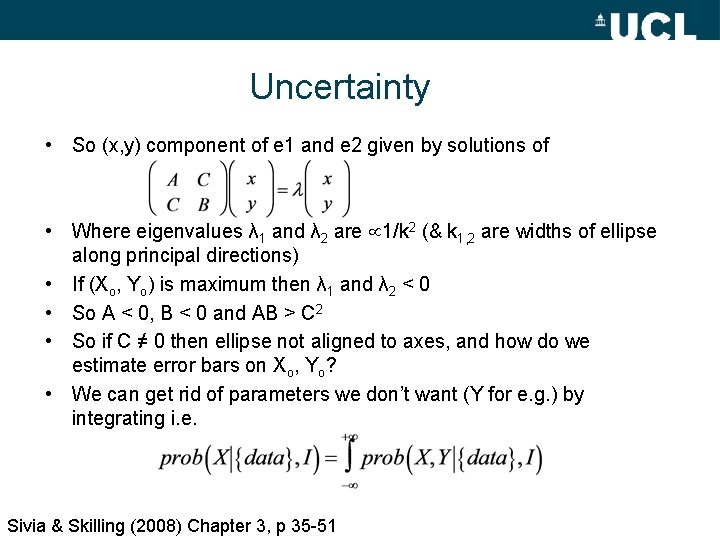
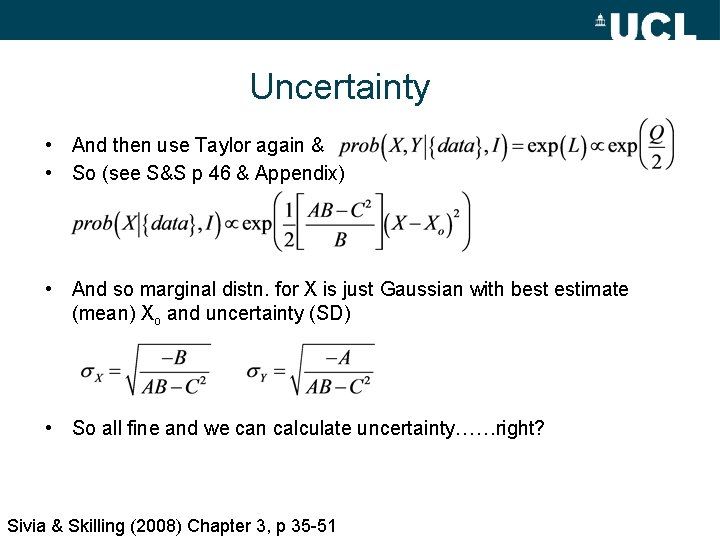
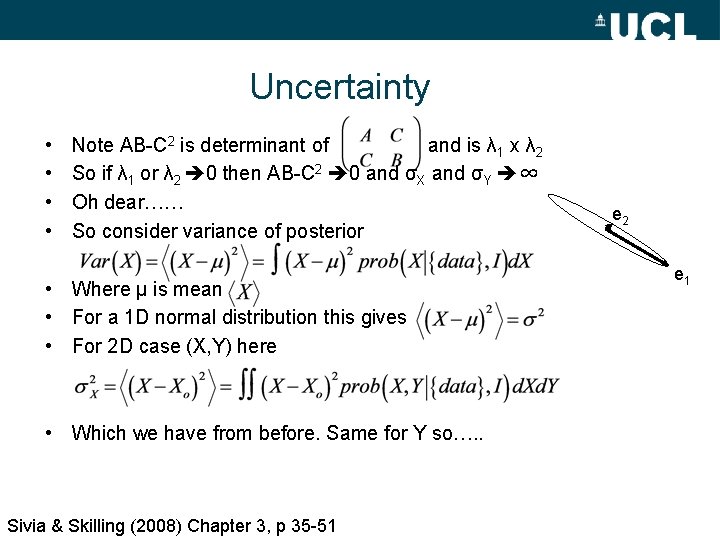
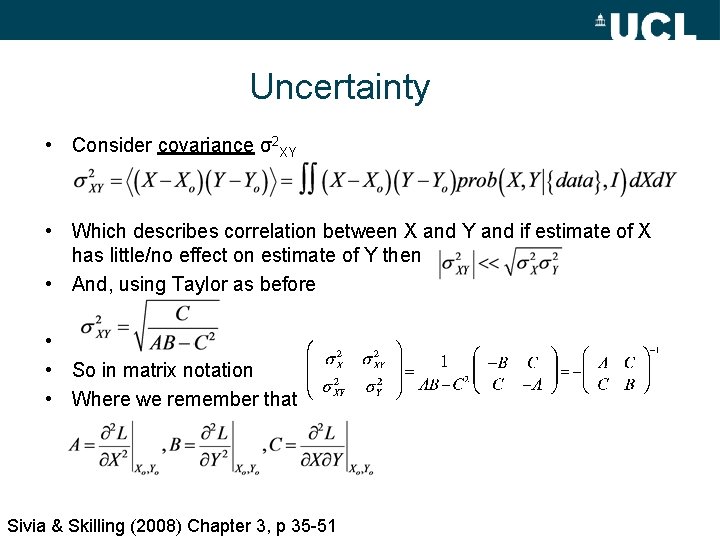
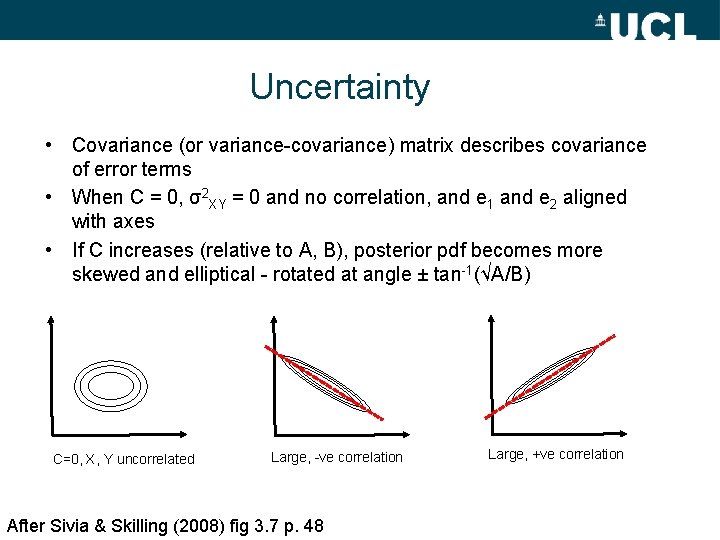
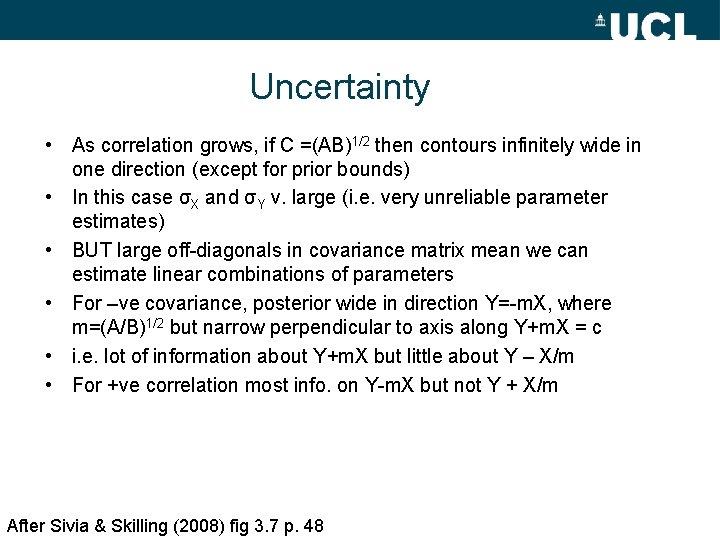
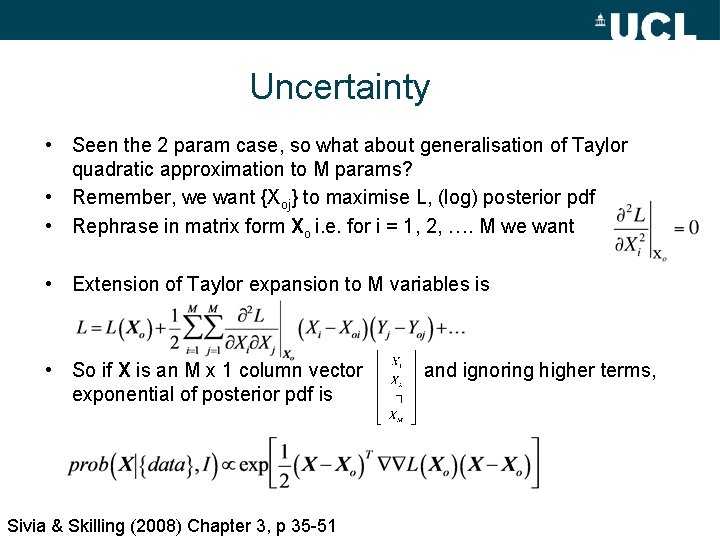
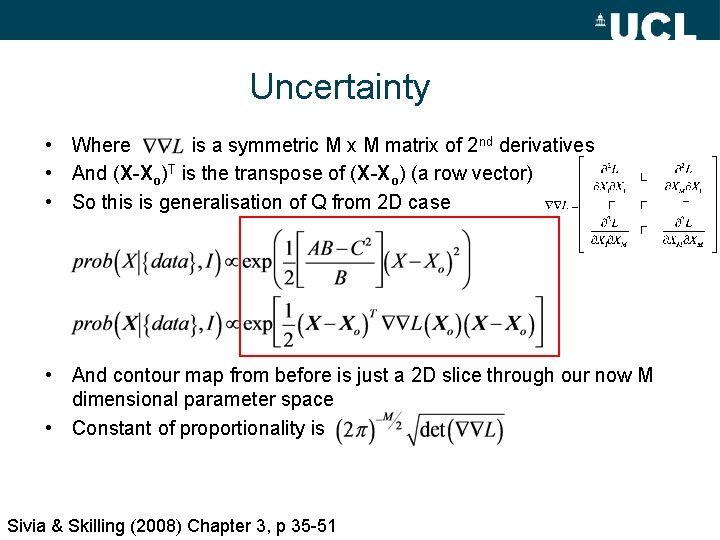
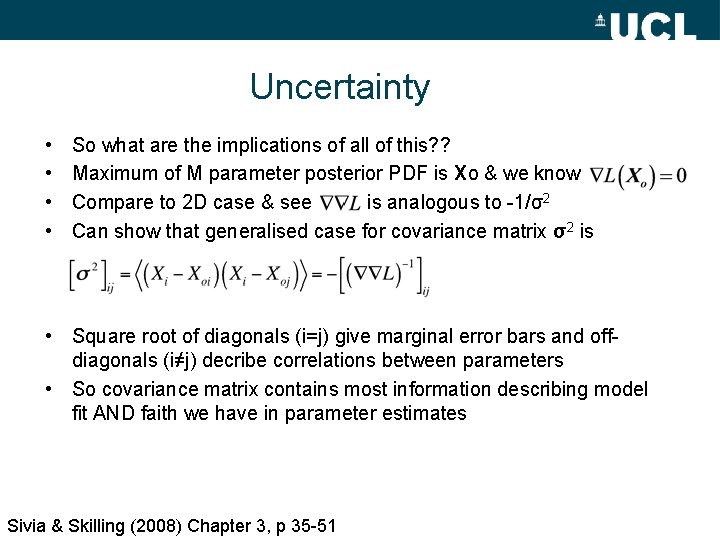
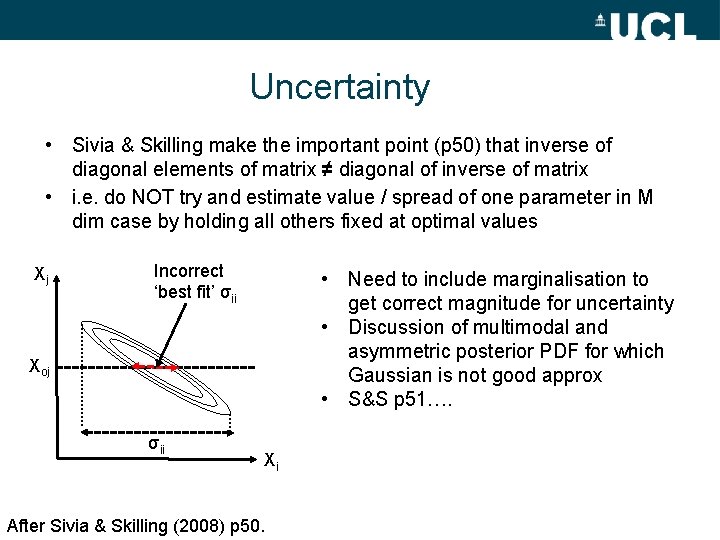
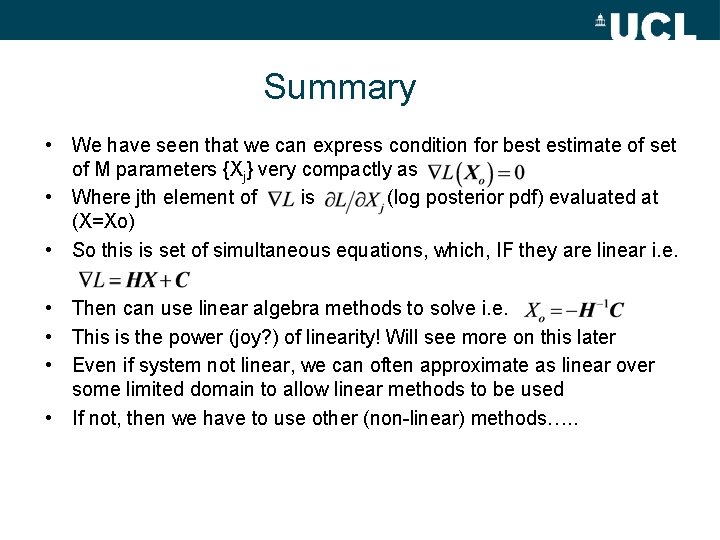
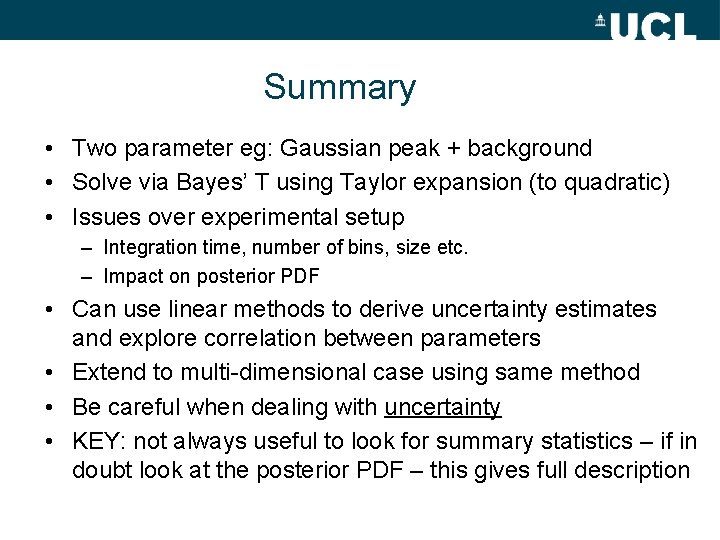
- Slides: 29
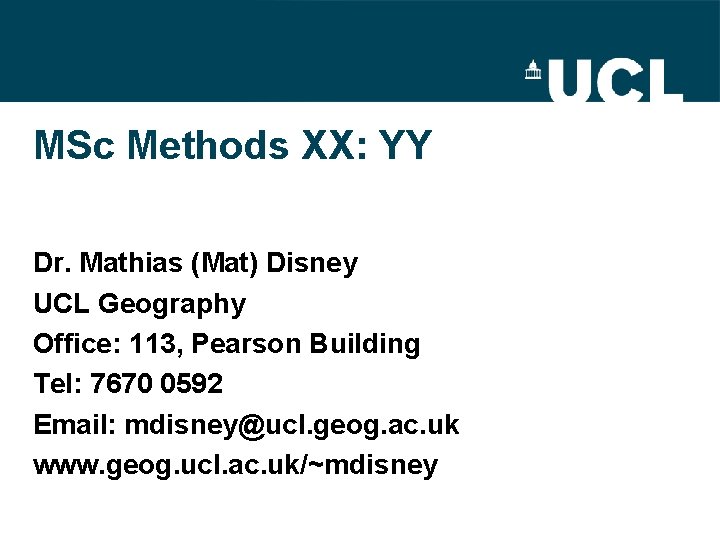
MSc Methods XX: YY Dr. Mathias (Mat) Disney UCL Geography Office: 113, Pearson Building Tel: 7670 0592 Email: mdisney@ucl. geog. ac. uk www. geog. ucl. ac. uk/~mdisney
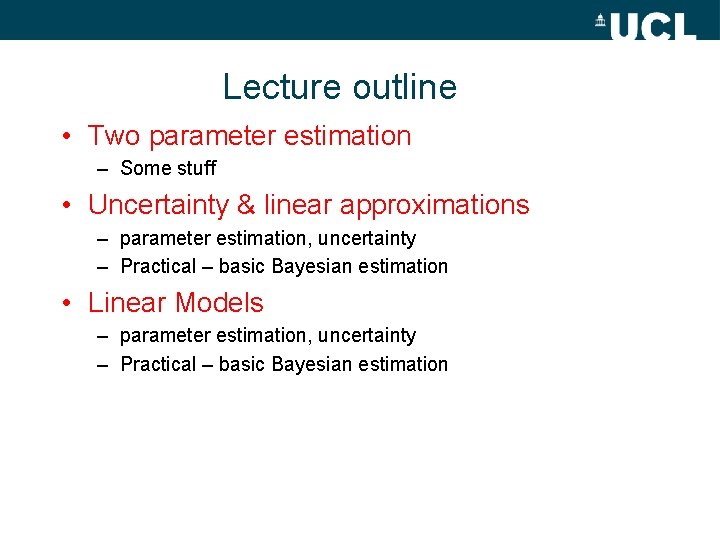
Lecture outline • Two parameter estimation – Some stuff • Uncertainty & linear approximations – parameter estimation, uncertainty – Practical – basic Bayesian estimation • Linear Models – parameter estimation, uncertainty – Practical – basic Bayesian estimation
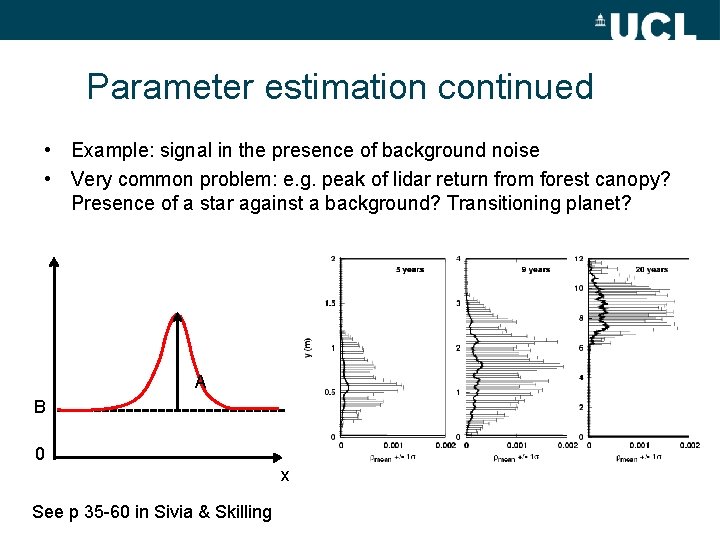
Parameter estimation continued • Example: signal in the presence of background noise • Very common problem: e. g. peak of lidar return from forest canopy? Presence of a star against a background? Transitioning planet? A B 0 x See p 35 -60 in Sivia & Skilling
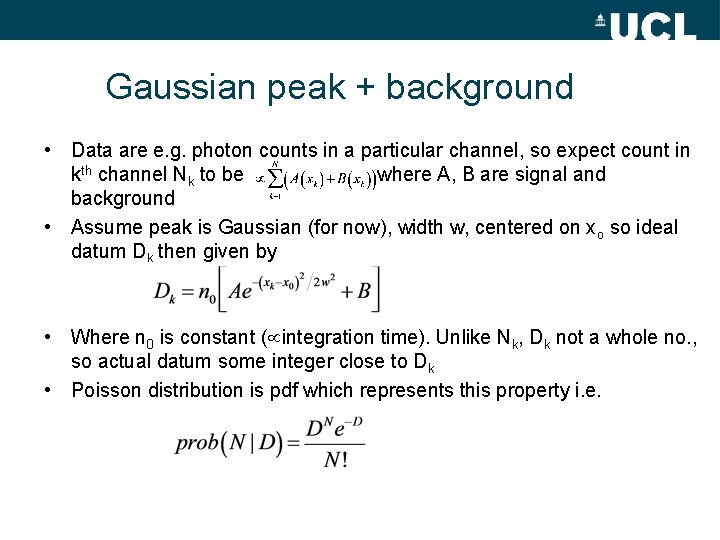
Gaussian peak + background • Data are e. g. photon counts in a particular channel, so expect count in kth channel Nk to be where A, B are signal and background • Assume peak is Gaussian (for now), width w, centered on xo so ideal datum Dk then given by • Where n 0 is constant ( integration time). Unlike Nk, Dk not a whole no. , so actual datum some integer close to Dk • Poisson distribution is pdf which represents this property i. e.
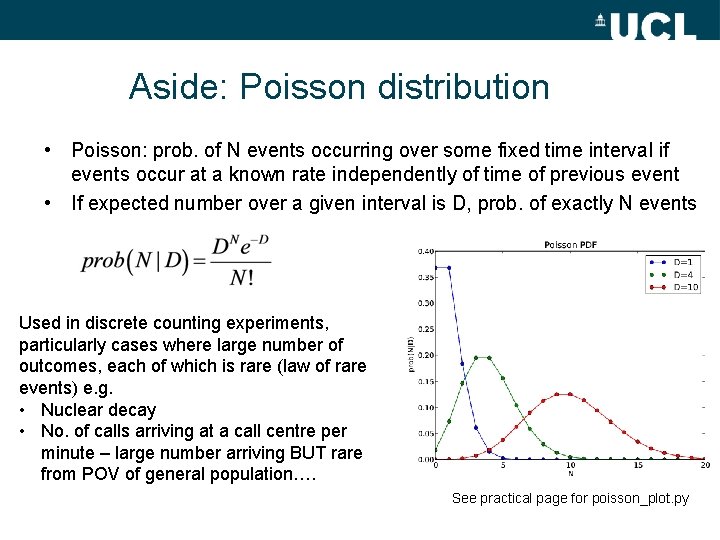
Aside: Poisson distribution • Poisson: prob. of N events occurring over some fixed time interval if events occur at a known rate independently of time of previous event • If expected number over a given interval is D, prob. of exactly N events Used in discrete counting experiments, particularly cases where large number of outcomes, each of which is rare (law of rare events) e. g. • Nuclear decay • No. of calls arriving at a call centre per minute – large number arriving BUT rare from POV of general population…. See practical page for poisson_plot. py
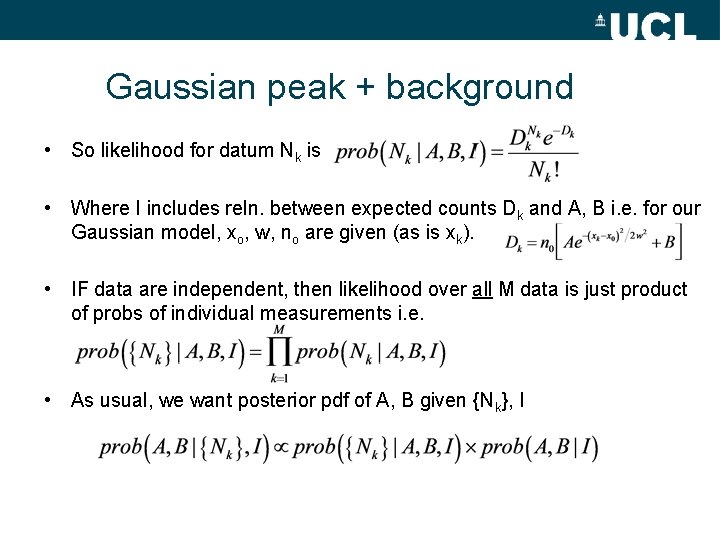
Gaussian peak + background • So likelihood for datum Nk is • Where I includes reln. between expected counts Dk and A, B i. e. for our Gaussian model, xo, w, no are given (as is xk). • IF data are independent, then likelihood over all M data is just product of probs of individual measurements i. e. • As usual, we want posterior pdf of A, B given {Nk}, I
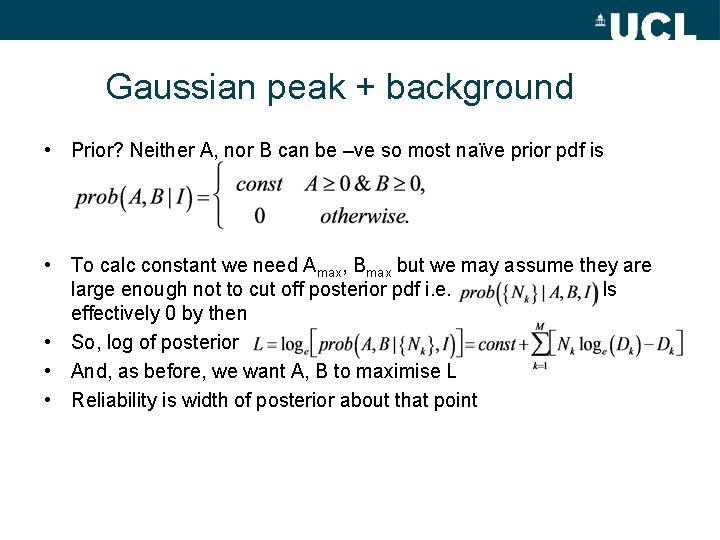
Gaussian peak + background • Prior? Neither A, nor B can be –ve so most naïve prior pdf is • To calc constant we need Amax, Bmax but we may assume they are large enough not to cut off posterior pdf i. e. Is effectively 0 by then • So, log of posterior • And, as before, we want A, B to maximise L • Reliability is width of posterior about that point
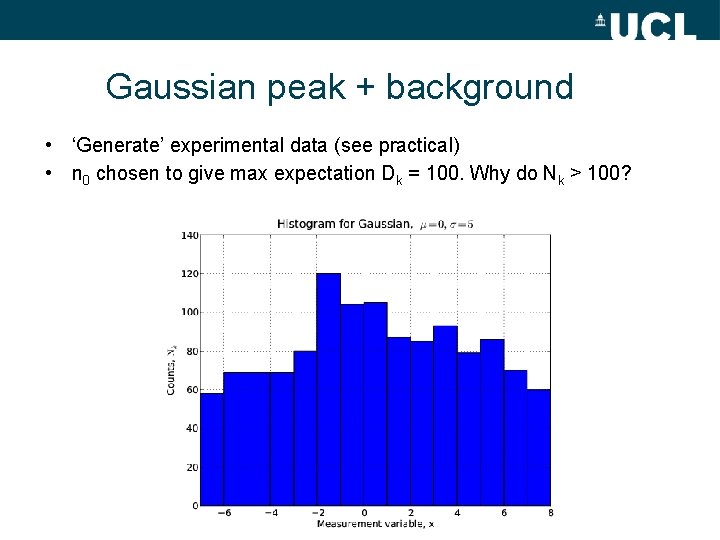
Gaussian peak + background • ‘Generate’ experimental data (see practical) • n 0 chosen to give max expectation Dk = 100. Why do Nk > 100?
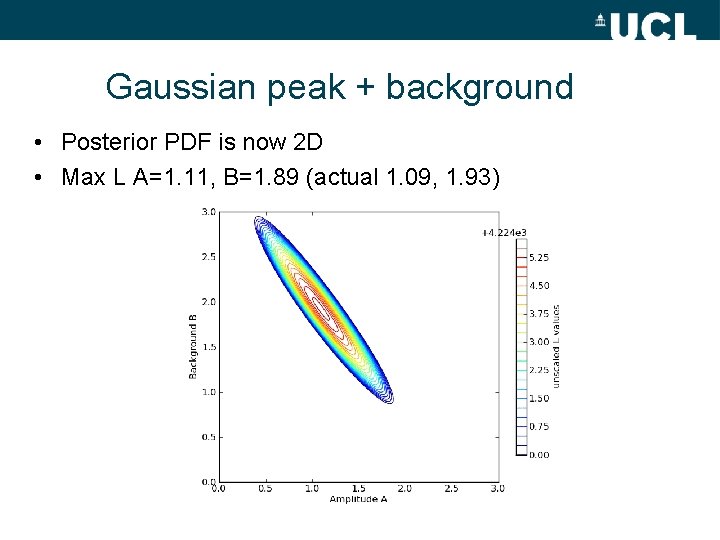
Gaussian peak + background • Posterior PDF is now 2 D • Max L A=1. 11, B=1. 89 (actual 1. 09, 1. 93)
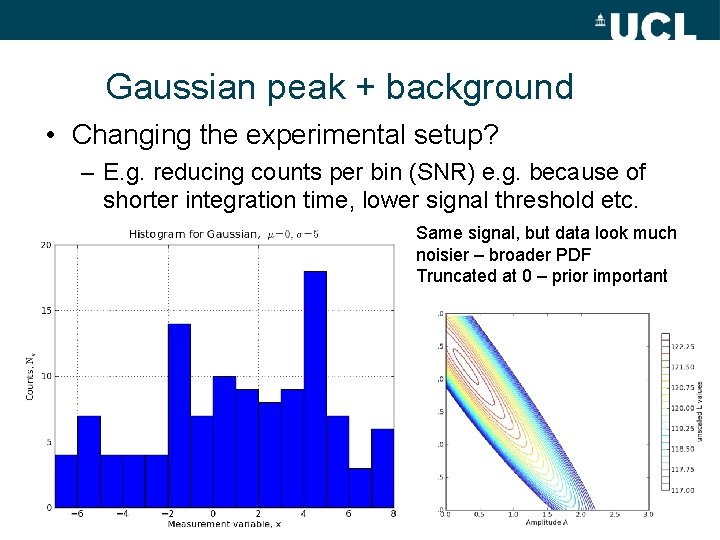
Gaussian peak + background • Changing the experimental setup? – E. g. reducing counts per bin (SNR) e. g. because of shorter integration time, lower signal threshold etc. Same signal, but data look much noisier – broader PDF Truncated at 0 – prior important
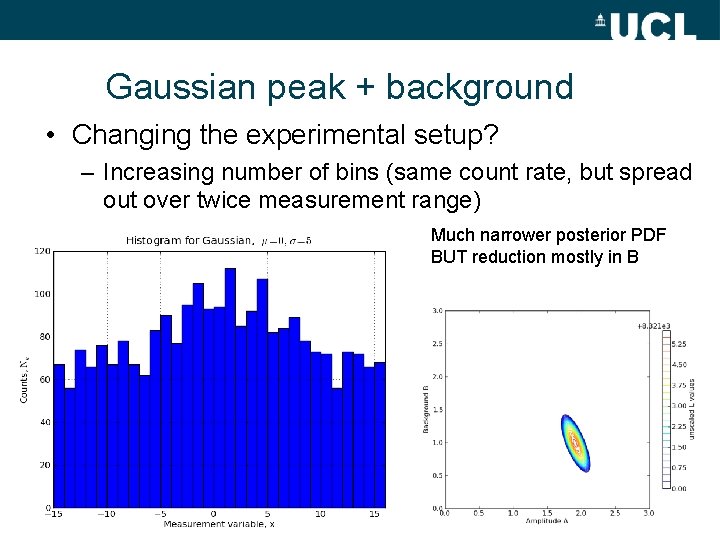
Gaussian peak + background • Changing the experimental setup? – Increasing number of bins (same count rate, but spread out over twice measurement range) Much narrower posterior PDF BUT reduction mostly in B
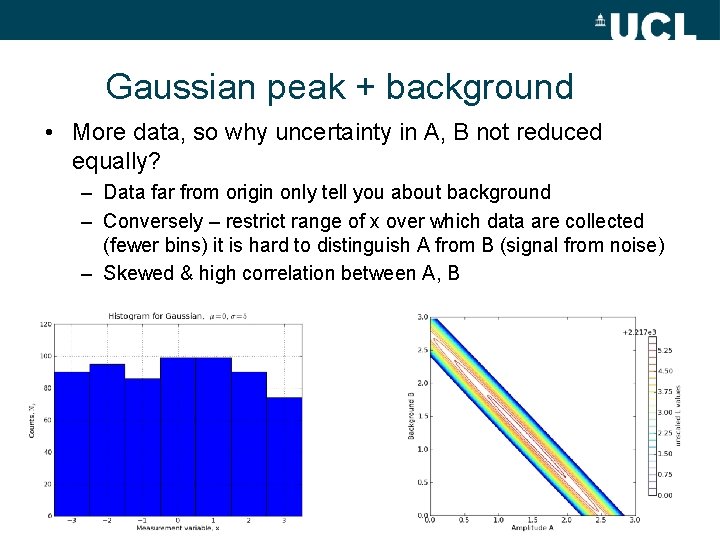
Gaussian peak + background • More data, so why uncertainty in A, B not reduced equally? – Data far from origin only tell you about background – Conversely – restrict range of x over which data are collected (fewer bins) it is hard to distinguish A from B (signal from noise) – Skewed & high correlation between A, B
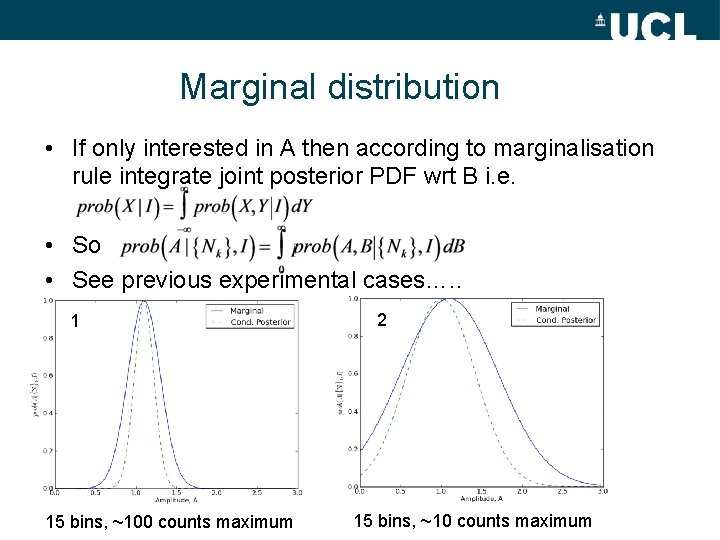
Marginal distribution • If only interested in A then according to marginalisation rule integrate joint posterior PDF wrt B i. e. • So • See previous experimental cases…. . 1 15 bins, ~100 counts maximum 2 15 bins, ~10 counts maximum
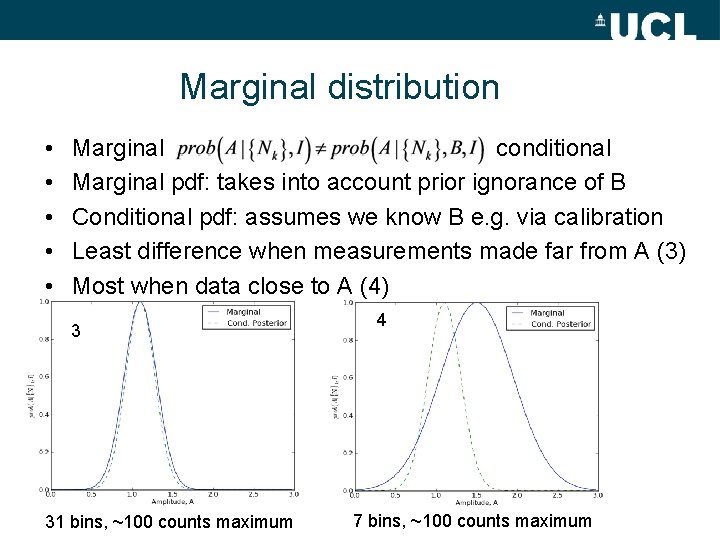
Marginal distribution • • • Marginal conditional Marginal pdf: takes into account prior ignorance of B Conditional pdf: assumes we know B e. g. via calibration Least difference when measurements made far from A (3) Most when data close to A (4) 3 31 bins, ~100 counts maximum 4 7 bins, ~100 counts maximum
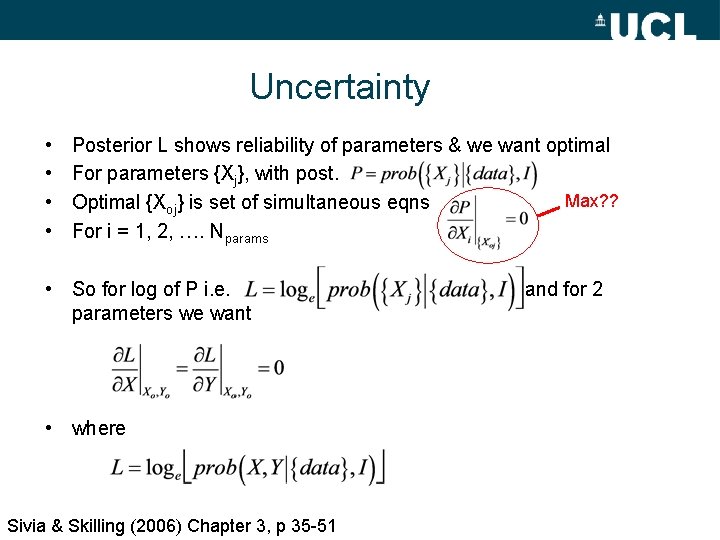
Uncertainty • • Posterior L shows reliability of parameters & we want optimal For parameters {Xj}, with post. Max? ? Optimal {Xoj} is set of simultaneous eqns For i = 1, 2, …. Nparams • So for log of P i. e. parameters we want • where Sivia & Skilling (2006) Chapter 3, p 35 -51 and for 2
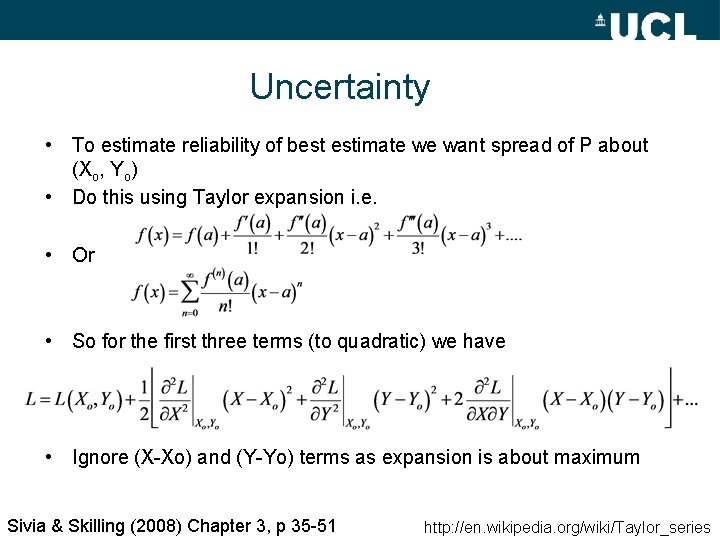
Uncertainty • To estimate reliability of best estimate we want spread of P about (Xo, Yo) • Do this using Taylor expansion i. e. • Or • So for the first three terms (to quadratic) we have • Ignore (X-Xo) and (Y-Yo) terms as expansion is about maximum Sivia & Skilling (2008) Chapter 3, p 35 -51 http: //en. wikipedia. org/wiki/Taylor_series
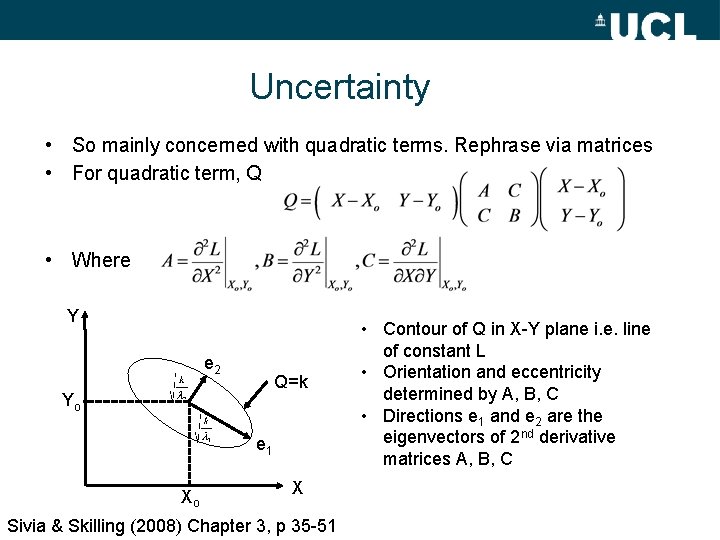
Uncertainty • So mainly concerned with quadratic terms. Rephrase via matrices • For quadratic term, Q • Where Y e 2 Q=k Yo e 1 Xo X Sivia & Skilling (2008) Chapter 3, p 35 -51 • Contour of Q in X-Y plane i. e. line of constant L • Orientation and eccentricity determined by A, B, C • Directions e 1 and e 2 are the eigenvectors of 2 nd derivative matrices A, B, C
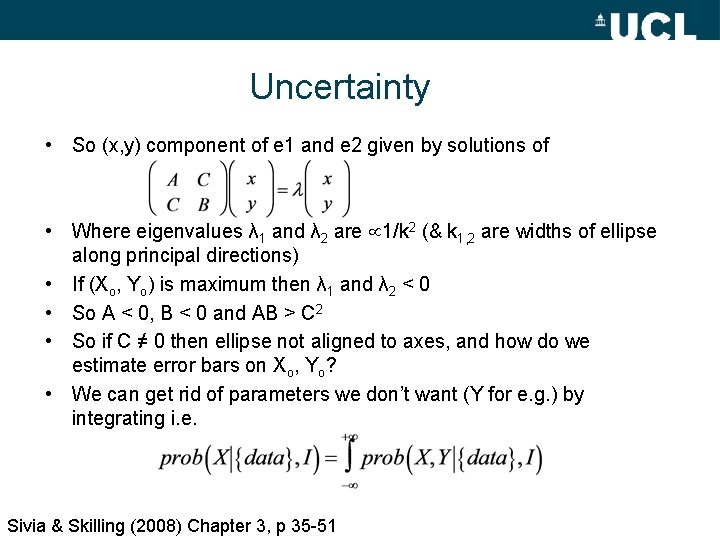
Uncertainty • So (x, y) component of e 1 and e 2 given by solutions of • Where eigenvalues λ 1 and λ 2 are 1/k 2 (& k 1, 2 are widths of ellipse along principal directions) • If (Xo, Yo) is maximum then λ 1 and λ 2 < 0 • So A < 0, B < 0 and AB > C 2 • So if C ≠ 0 then ellipse not aligned to axes, and how do we estimate error bars on Xo, Yo? • We can get rid of parameters we don’t want (Y for e. g. ) by integrating i. e. Sivia & Skilling (2008) Chapter 3, p 35 -51
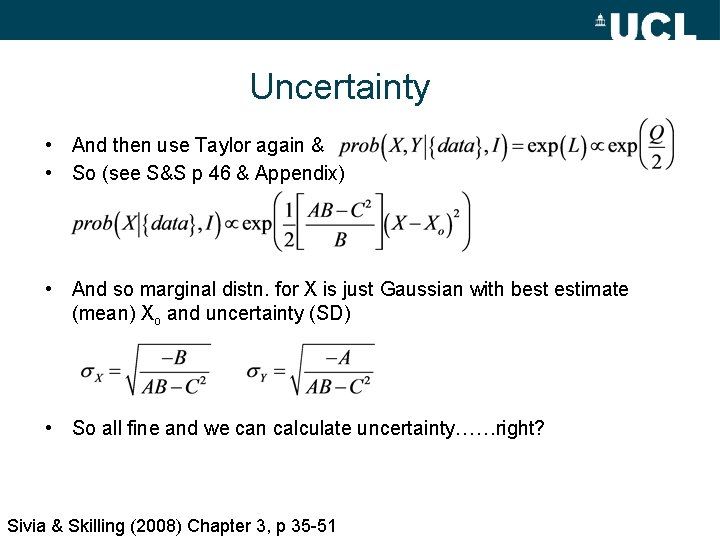
Uncertainty • And then use Taylor again & • So (see S&S p 46 & Appendix) • And so marginal distn. for X is just Gaussian with best estimate (mean) Xo and uncertainty (SD) • So all fine and we can calculate uncertainty……right? Sivia & Skilling (2008) Chapter 3, p 35 -51
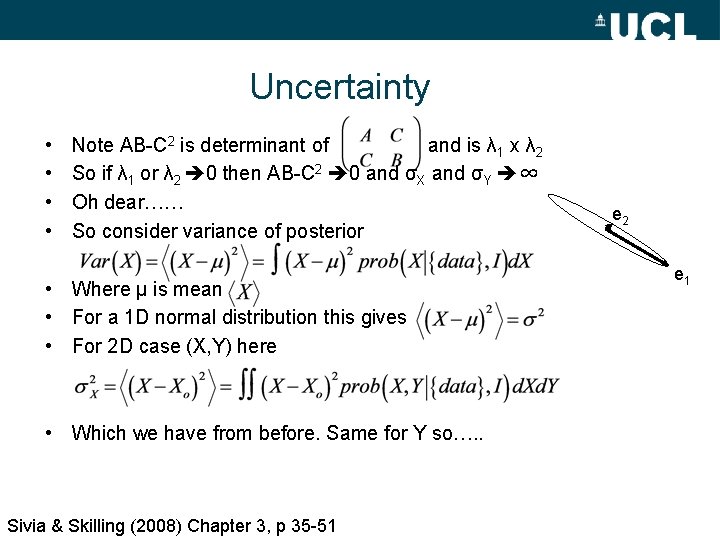
Uncertainty • • Note AB-C 2 is determinant of and is λ 1 x λ 2 So if λ 1 or λ 2 0 then AB-C 2 0 and σX and σY ∞ Oh dear…… So consider variance of posterior • Where μ is mean • For a 1 D normal distribution this gives • For 2 D case (X, Y) here • Which we have from before. Same for Y so…. . Sivia & Skilling (2008) Chapter 3, p 35 -51 e 2 e 1
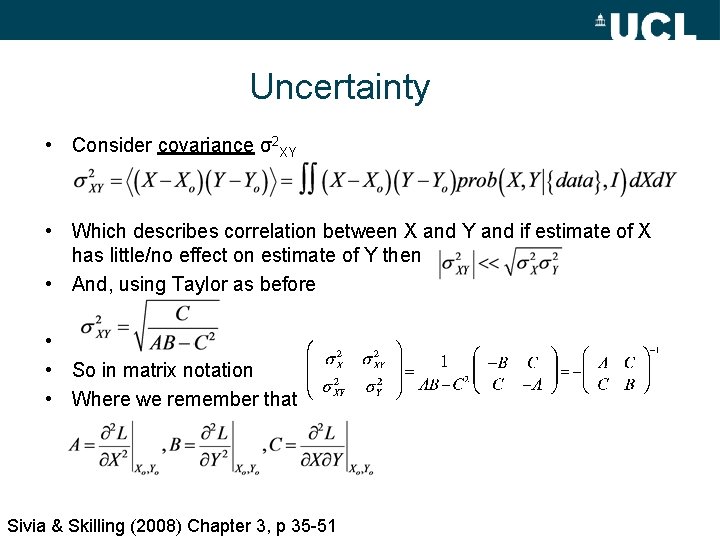
Uncertainty • Consider covariance σ2 XY • Which describes correlation between X and Y and if estimate of X has little/no effect on estimate of Y then • And, using Taylor as before • • So in matrix notation • Where we remember that Sivia & Skilling (2008) Chapter 3, p 35 -51
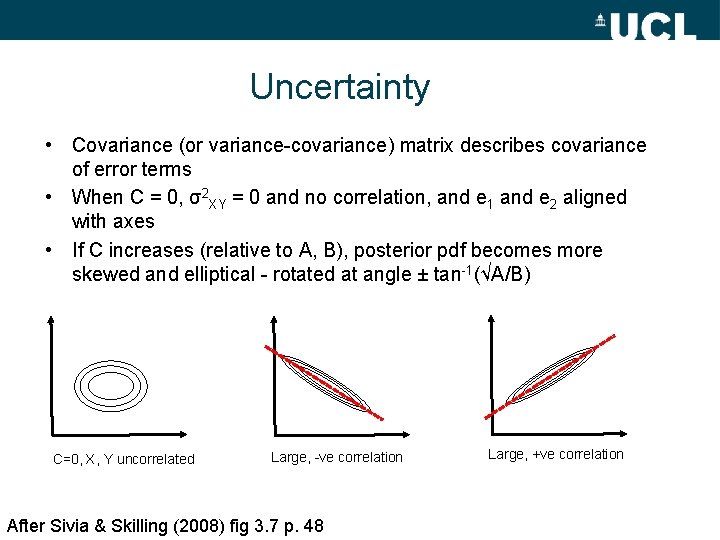
Uncertainty • Covariance (or variance-covariance) matrix describes covariance of error terms • When C = 0, σ2 XY = 0 and no correlation, and e 1 and e 2 aligned with axes • If C increases (relative to A, B), posterior pdf becomes more skewed and elliptical - rotated at angle ± tan-1(√A/B) C=0, X, Y uncorrelated Large, -ve correlation After Sivia & Skilling (2008) fig 3. 7 p. 48 Large, +ve correlation
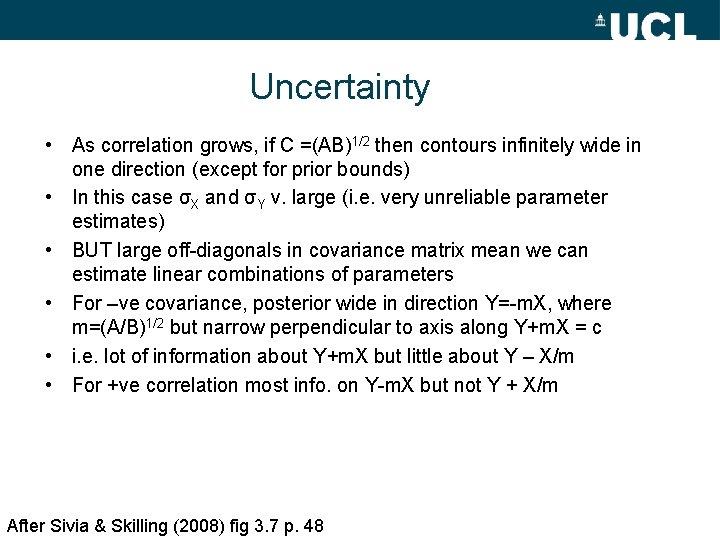
Uncertainty • As correlation grows, if C =(AB)1/2 then contours infinitely wide in one direction (except for prior bounds) • In this case σX and σY v. large (i. e. very unreliable parameter estimates) • BUT large off-diagonals in covariance matrix mean we can estimate linear combinations of parameters • For –ve covariance, posterior wide in direction Y=-m. X, where m=(A/B)1/2 but narrow perpendicular to axis along Y+m. X = c • i. e. lot of information about Y+m. X but little about Y – X/m • For +ve correlation most info. on Y-m. X but not Y + X/m After Sivia & Skilling (2008) fig 3. 7 p. 48
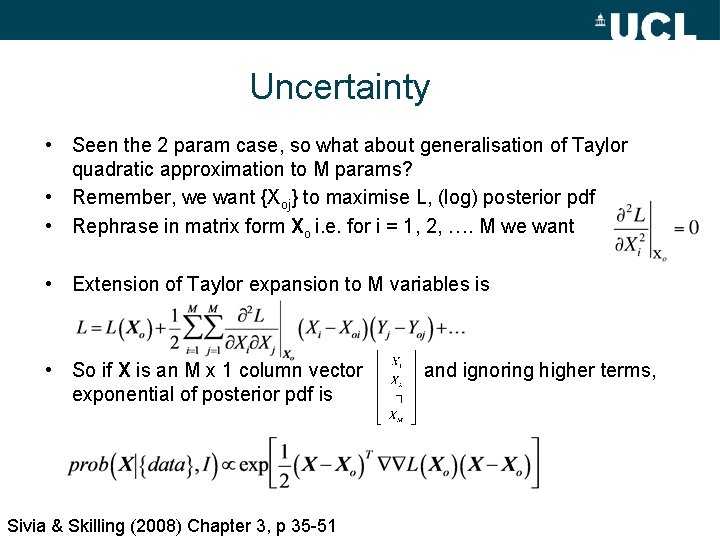
Uncertainty • Seen the 2 param case, so what about generalisation of Taylor quadratic approximation to M params? • Remember, we want {Xoj} to maximise L, (log) posterior pdf • Rephrase in matrix form Xo i. e. for i = 1, 2, …. M we want • Extension of Taylor expansion to M variables is • So if X is an M x 1 column vector exponential of posterior pdf is Sivia & Skilling (2008) Chapter 3, p 35 -51 and ignoring higher terms,
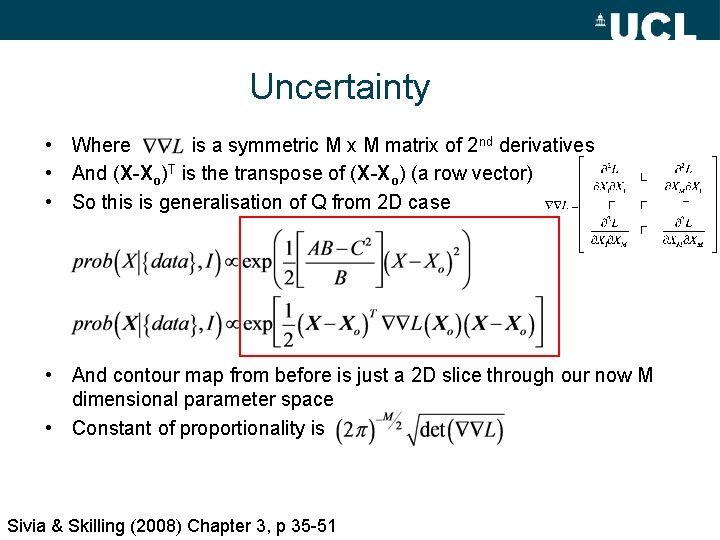
Uncertainty • Where is a symmetric M x M matrix of 2 nd derivatives • And (X-Xo)T is the transpose of (X-Xo) (a row vector) • So this is generalisation of Q from 2 D case • And contour map from before is just a 2 D slice through our now M dimensional parameter space • Constant of proportionality is Sivia & Skilling (2008) Chapter 3, p 35 -51
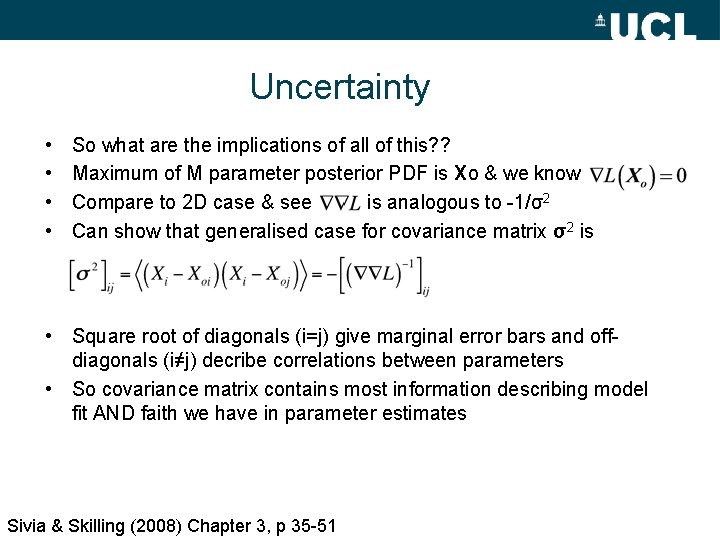
Uncertainty • • So what are the implications of all of this? ? Maximum of M parameter posterior PDF is Xo & we know Compare to 2 D case & see is analogous to -1/σ2 Can show that generalised case for covariance matrix σ2 is • Square root of diagonals (i=j) give marginal error bars and offdiagonals (i≠j) decribe correlations between parameters • So covariance matrix contains most information describing model fit AND faith we have in parameter estimates Sivia & Skilling (2008) Chapter 3, p 35 -51
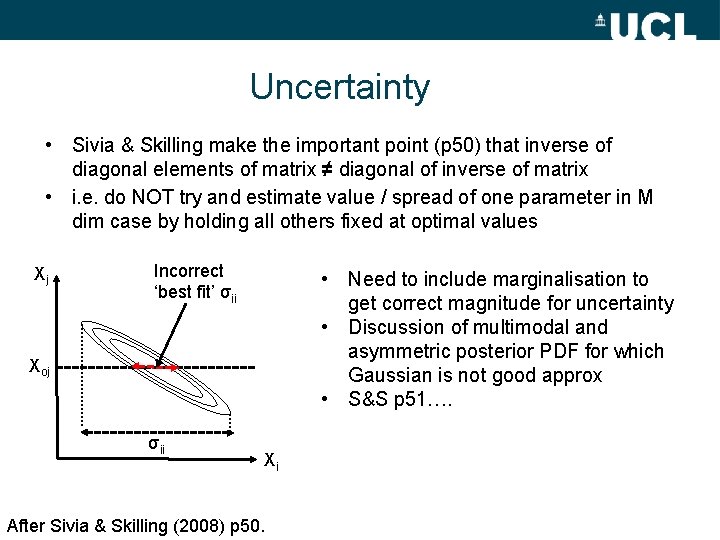
Uncertainty • Sivia & Skilling make the important point (p 50) that inverse of diagonal elements of matrix ≠ diagonal of inverse of matrix • i. e. do NOT try and estimate value / spread of one parameter in M dim case by holding all others fixed at optimal values Xj Incorrect ‘best fit’ σii • Need to include marginalisation to get correct magnitude for uncertainty • Discussion of multimodal and asymmetric posterior PDF for which Gaussian is not good approx • S&S p 51…. Xoj σii Xi After Sivia & Skilling (2008) p 50.
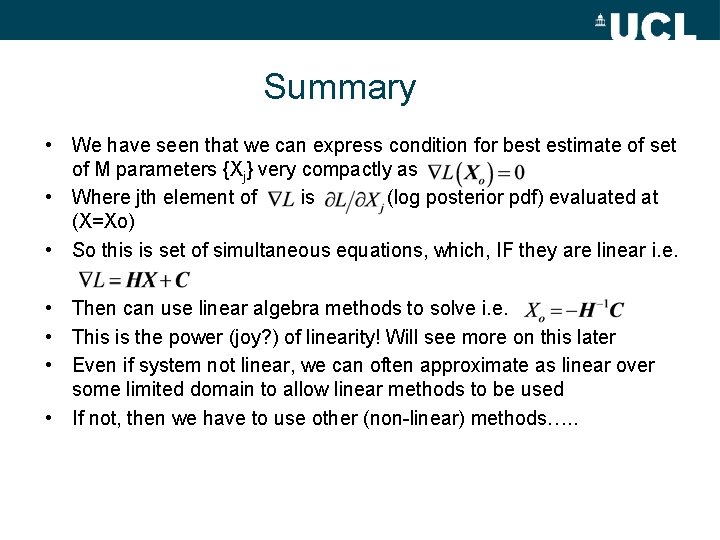
Summary • We have seen that we can express condition for best estimate of set of M parameters {Xj} very compactly as • Where jth element of is (log posterior pdf) evaluated at (X=Xo) • So this is set of simultaneous equations, which, IF they are linear i. e. • Then can use linear algebra methods to solve i. e. • This is the power (joy? ) of linearity! Will see more on this later • Even if system not linear, we can often approximate as linear over some limited domain to allow linear methods to be used • If not, then we have to use other (non-linear) methods…. .
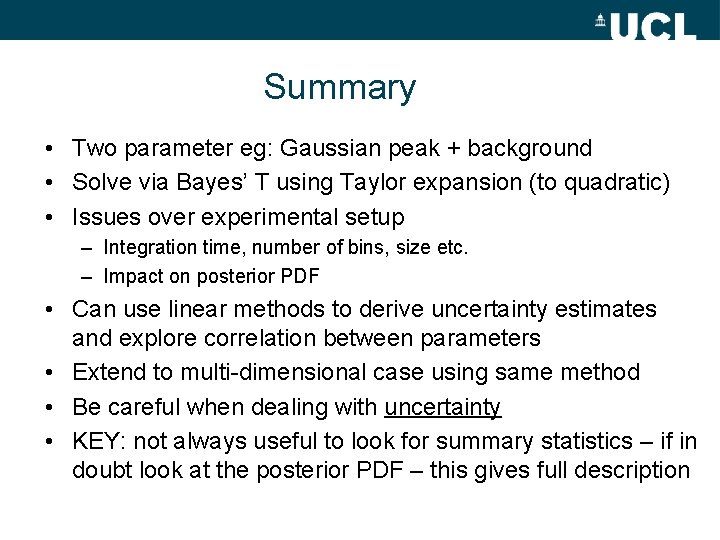
Summary • Two parameter eg: Gaussian peak + background • Solve via Bayes’ T using Taylor expansion (to quadratic) • Issues over experimental setup – Integration time, number of bins, size etc. – Impact on posterior PDF • Can use linear methods to derive uncertainty estimates and explore correlation between parameters • Extend to multi-dimensional case using same method • Be careful when dealing with uncertainty • KEY: not always useful to look for summary statistics – if in doubt look at the posterior PDF – this gives full description
Mathias temot
Mathias mæhle
Porr h
Fraunhofer moez
Seminari montfort pondok kebijaksanaan
Lms
Mathias loris
Mathias lux
Mathias bienstman
Mathias temot
Mathias loris
Somatiseringstilstand
Mathias jourdan
Mathias coiffard
Mathias fünfstück
Mathias temot
Mathias reinecke
Mathias reinecke
Mathias thaler
Rudolf virchow
Chapter 4 cell theory and cell study
Direct wax pattern
Msc traction
Msc pelago
Fsvad
Solid-state lighting (ssl)
Struktur msc
Rna transfer
Msc
Msc sandra