MIT 6 441 Capacity of multiantenna Gaussian Channels
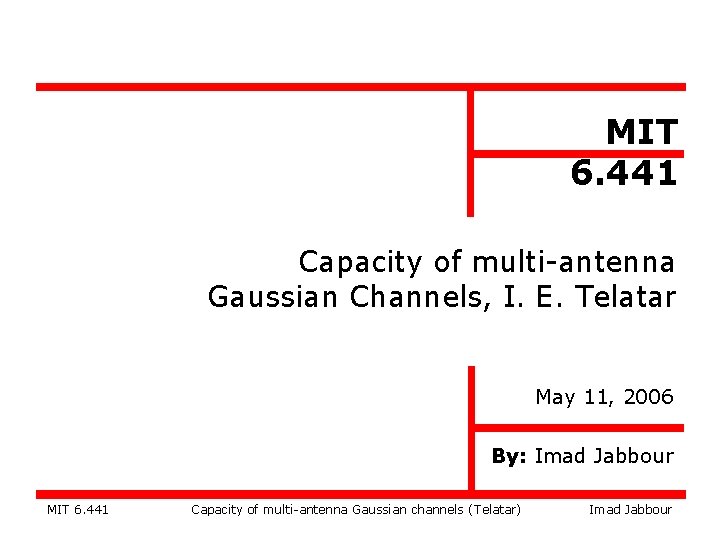
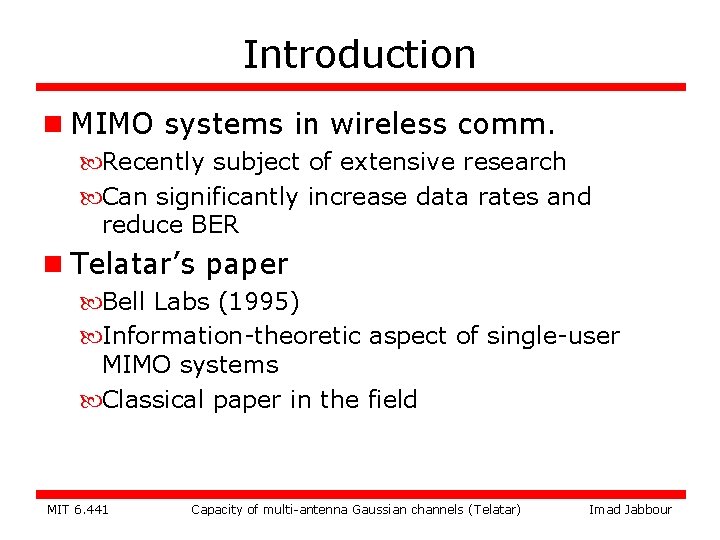
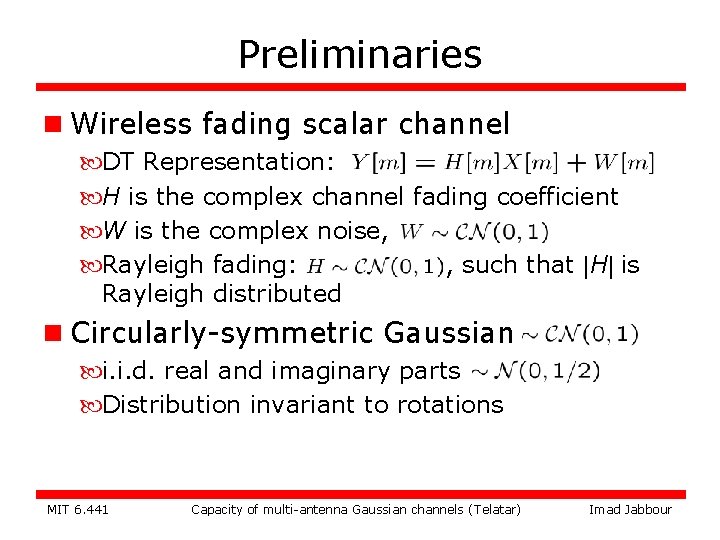
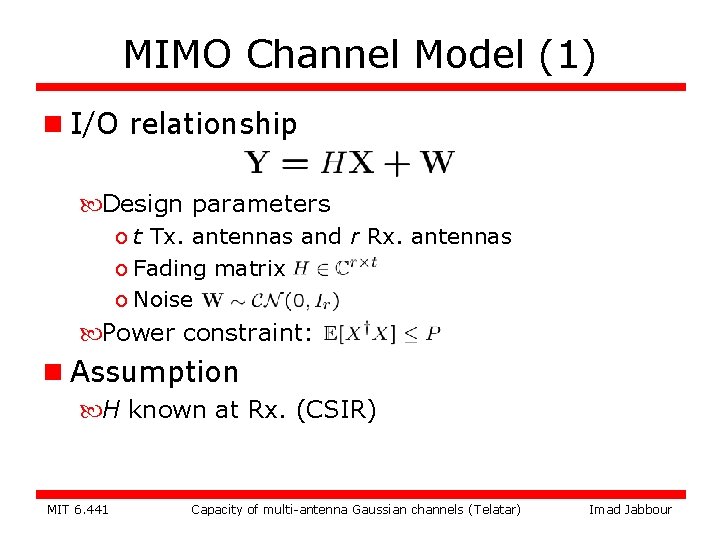
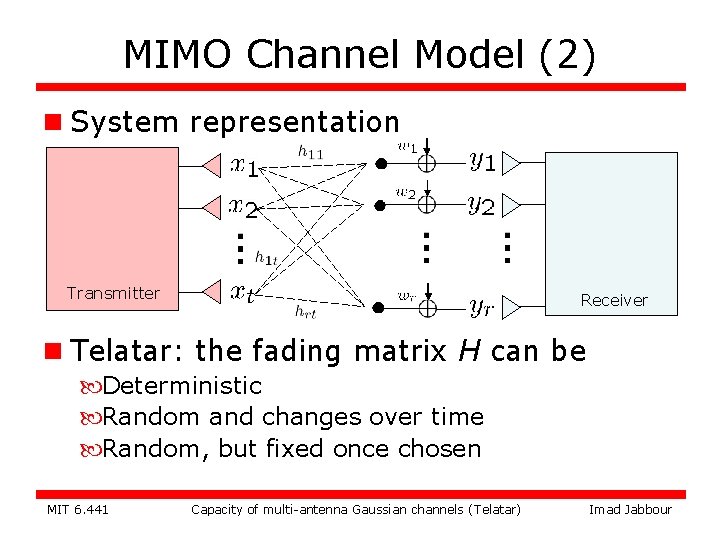
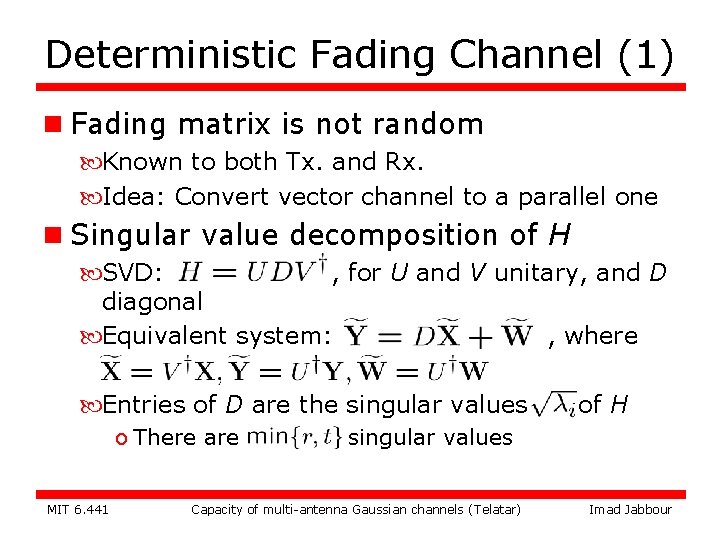
![Deterministic Fading Channel (2) n Equivalent parallel channel [nmin=min(r, t)] Tx. must know H Deterministic Fading Channel (2) n Equivalent parallel channel [nmin=min(r, t)] Tx. must know H](https://slidetodoc.com/presentation_image_h/80f95931d41a44252e3c7d9acea4ee92/image-7.jpg)
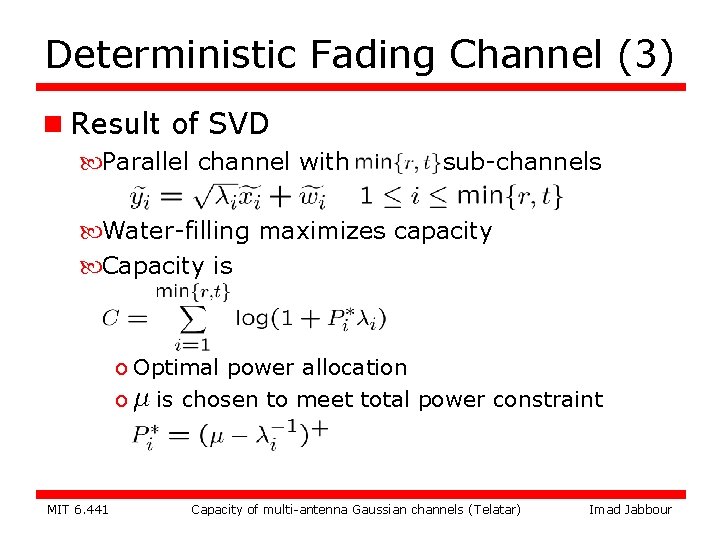
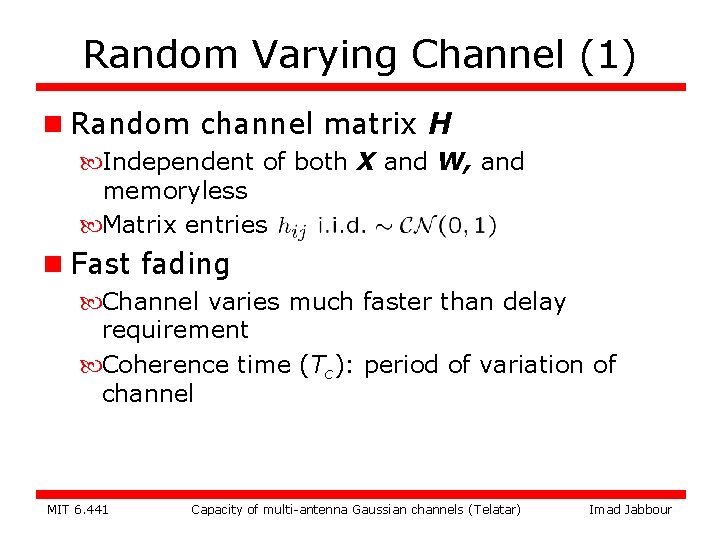
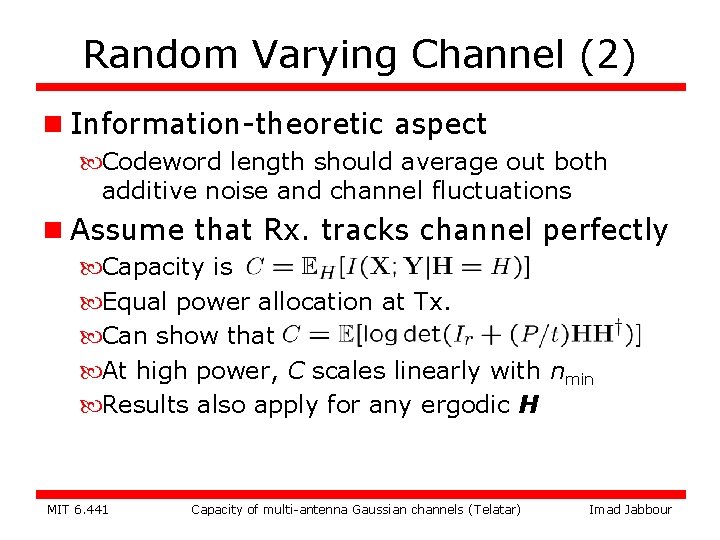
![Random Varying Channel (3) n MIMO capacity versus SNR (from [2]) MIT 6. 441 Random Varying Channel (3) n MIMO capacity versus SNR (from [2]) MIT 6. 441](https://slidetodoc.com/presentation_image_h/80f95931d41a44252e3c7d9acea4ee92/image-11.jpg)
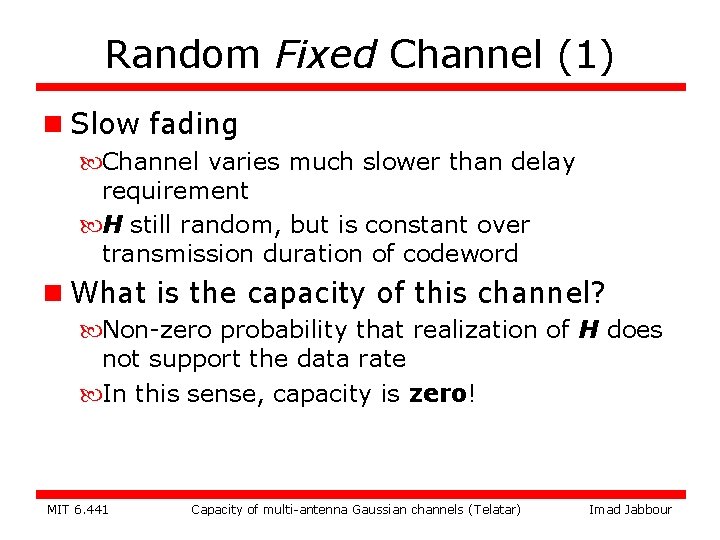
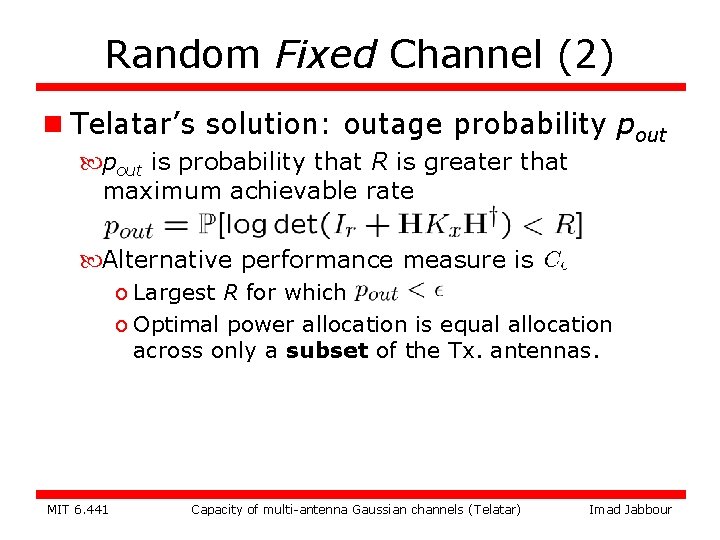
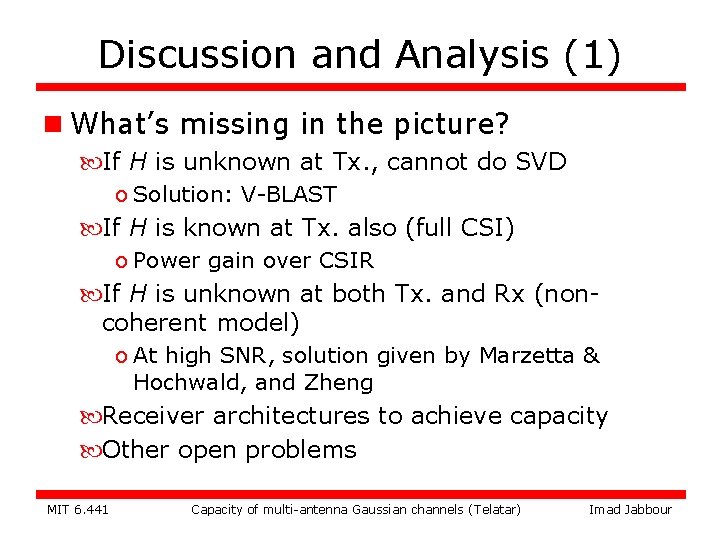
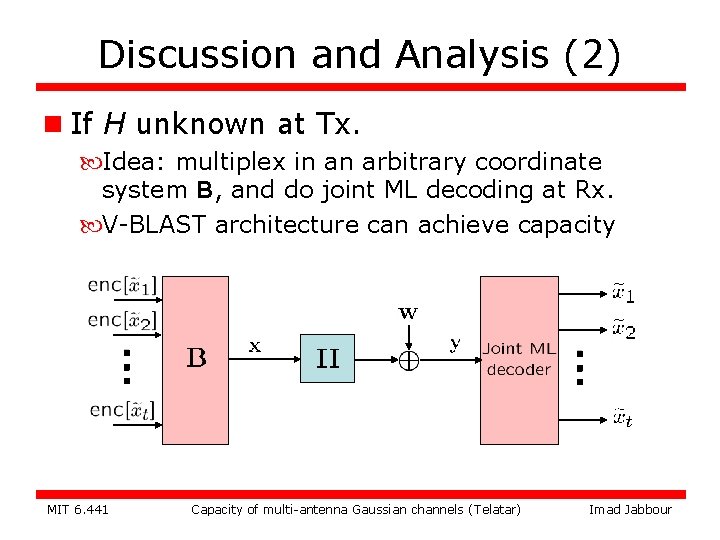
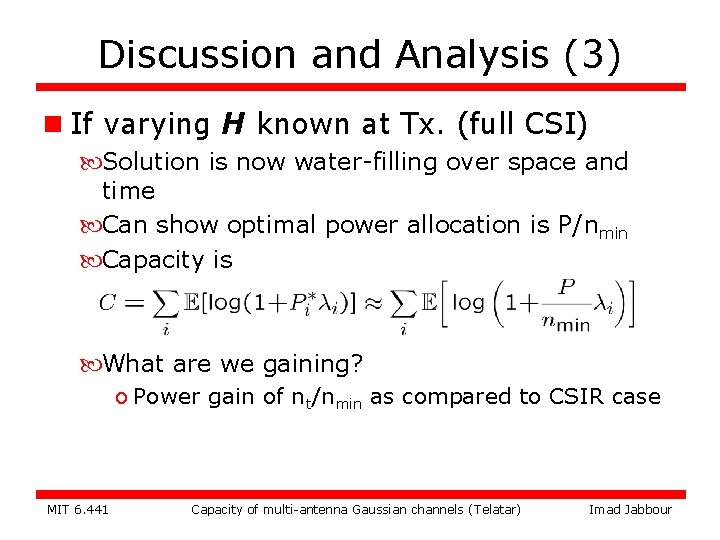
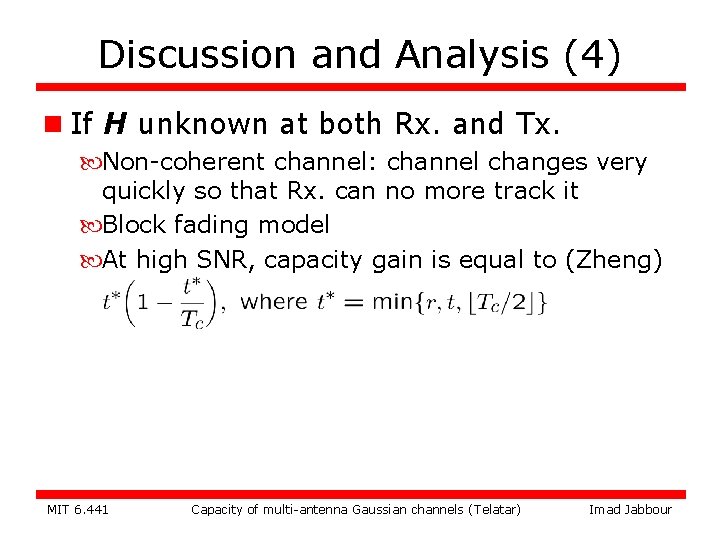
![Discussion and Analysis (5) n Receiver architectures [2] V-BLAST can achieve capacity for fast Discussion and Analysis (5) n Receiver architectures [2] V-BLAST can achieve capacity for fast](https://slidetodoc.com/presentation_image_h/80f95931d41a44252e3c7d9acea4ee92/image-18.jpg)
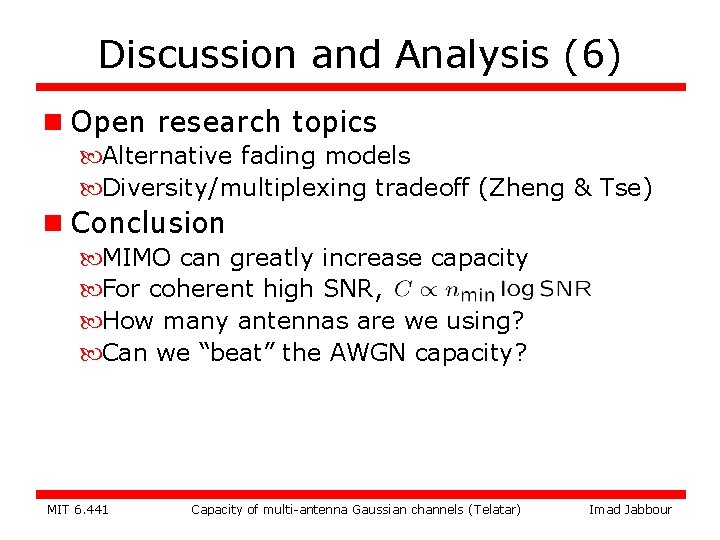
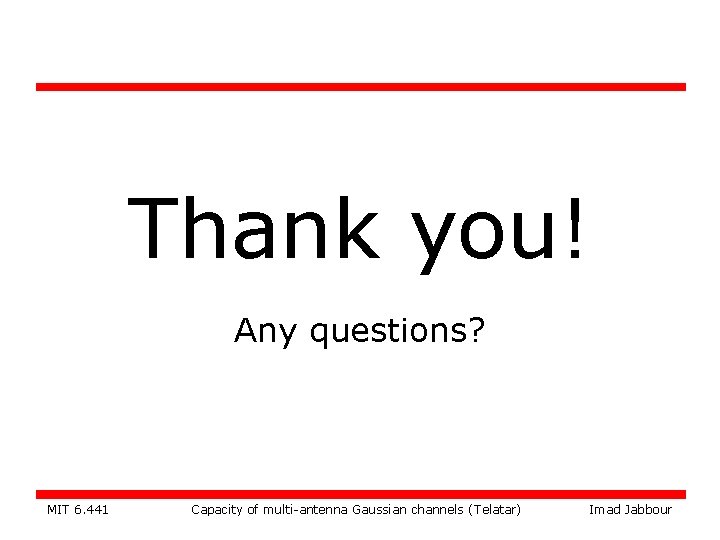
- Slides: 20
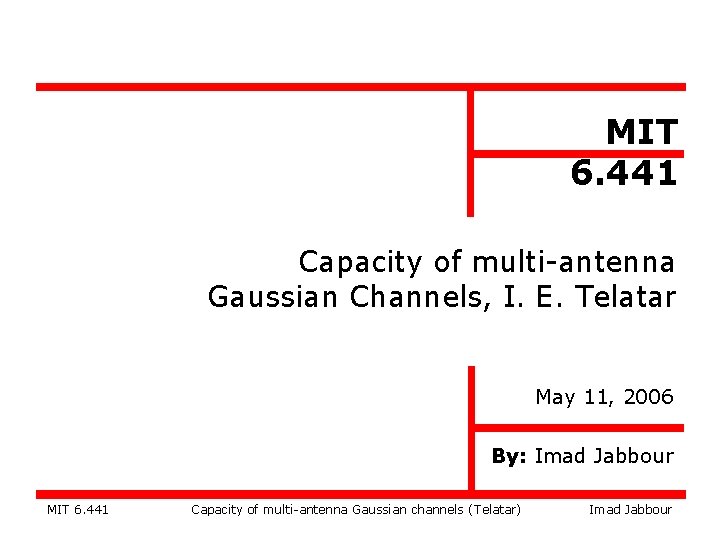
MIT 6. 441 Capacity of multi-antenna Gaussian Channels, I. E. Telatar May 11, 2006 By: Imad Jabbour MIT 6. 441 Capacity of multi-antenna Gaussian channels (Telatar) Imad Jabbour
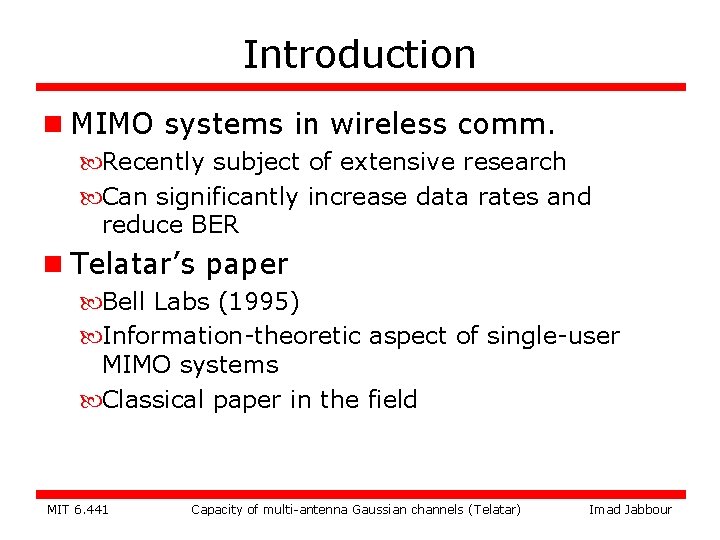
Introduction n MIMO systems in wireless comm. Recently subject of extensive research Can significantly increase data rates and reduce BER n Telatar’s paper Bell Labs (1995) Information-theoretic aspect of single-user MIMO systems Classical paper in the field MIT 6. 441 Capacity of multi-antenna Gaussian channels (Telatar) Imad Jabbour
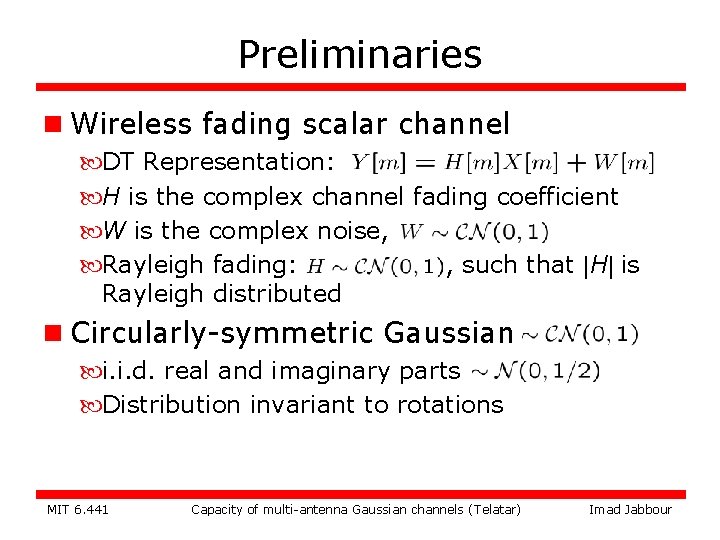
Preliminaries n Wireless fading scalar channel DT Representation: H is the complex channel fading coefficient W is the complex noise, Rayleigh fading: , such that |H| is Rayleigh distributed n Circularly-symmetric Gaussian i. i. d. real and imaginary parts Distribution invariant to rotations MIT 6. 441 Capacity of multi-antenna Gaussian channels (Telatar) Imad Jabbour
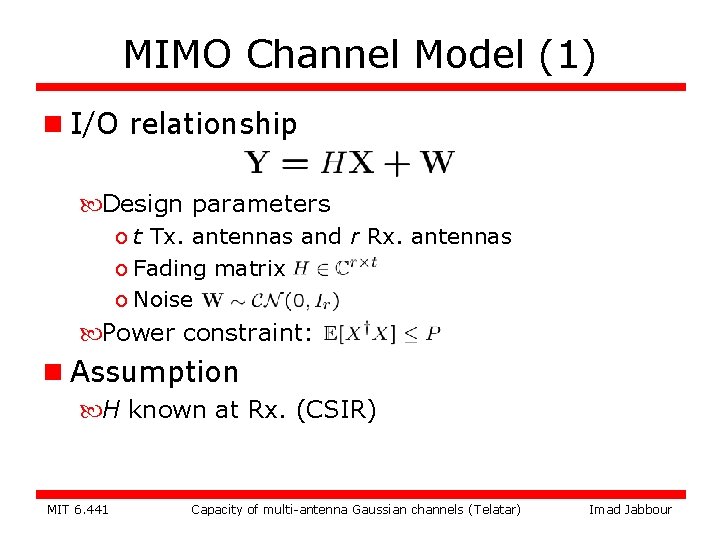
MIMO Channel Model (1) n I/O relationship Design parameters o t Tx. antennas and r Rx. antennas o Fading matrix o Noise Power constraint: n Assumption H known at Rx. (CSIR) MIT 6. 441 Capacity of multi-antenna Gaussian channels (Telatar) Imad Jabbour
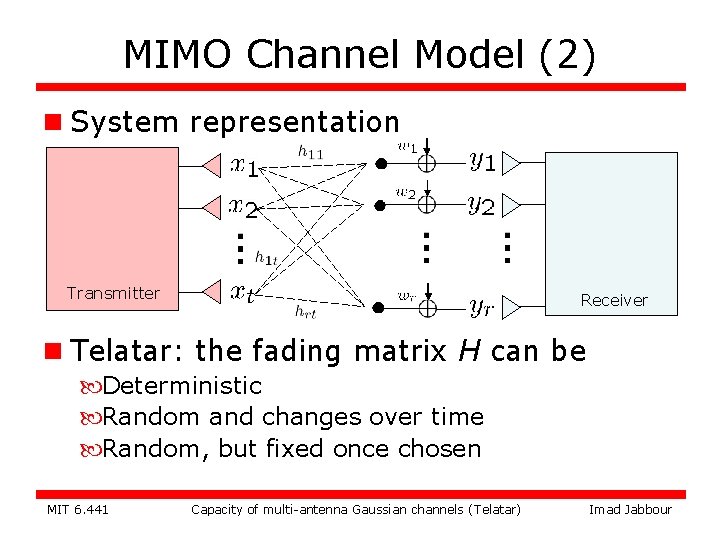
MIMO Channel Model (2) n System representation Transmitter Receiver n Telatar: the fading matrix H can be Deterministic Random and changes over time Random, but fixed once chosen MIT 6. 441 Capacity of multi-antenna Gaussian channels (Telatar) Imad Jabbour
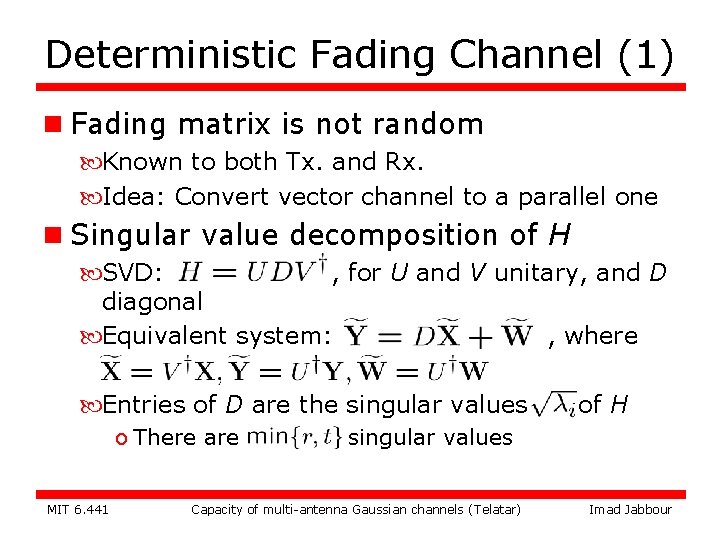
Deterministic Fading Channel (1) n Fading matrix is not random Known to both Tx. and Rx. Idea: Convert vector channel to a parallel one n Singular value decomposition of H SVD: , for U and V unitary, and D diagonal Equivalent system: , where Entries of D are the singular values o There are MIT 6. 441 of H singular values Capacity of multi-antenna Gaussian channels (Telatar) Imad Jabbour
![Deterministic Fading Channel 2 n Equivalent parallel channel nminminr t Tx must know H Deterministic Fading Channel (2) n Equivalent parallel channel [nmin=min(r, t)] Tx. must know H](https://slidetodoc.com/presentation_image_h/80f95931d41a44252e3c7d9acea4ee92/image-7.jpg)
Deterministic Fading Channel (2) n Equivalent parallel channel [nmin=min(r, t)] Tx. must know H to pre-process it, and Rx. must know H to post-process it MIT 6. 441 Capacity of multi-antenna Gaussian channels (Telatar) Imad Jabbour
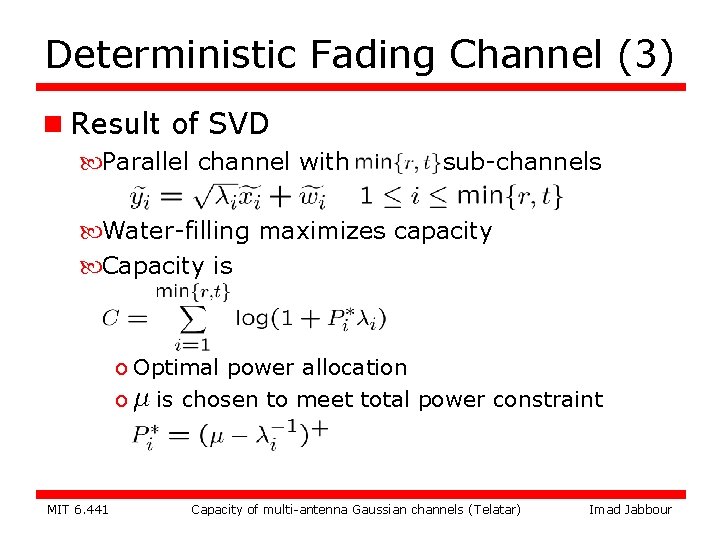
Deterministic Fading Channel (3) n Result of SVD Parallel channel with sub-channels Water-filling maximizes capacity Capacity is o Optimal power allocation o is chosen to meet total power constraint MIT 6. 441 Capacity of multi-antenna Gaussian channels (Telatar) Imad Jabbour
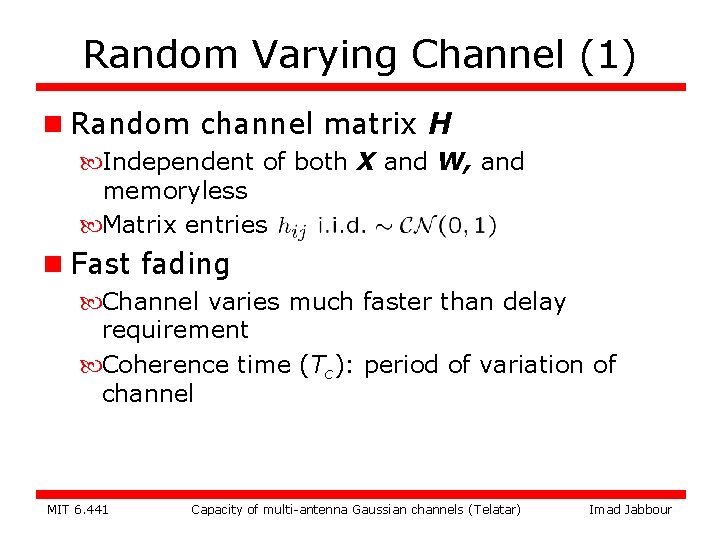
Random Varying Channel (1) n Random channel matrix H Independent of both X and W, and memoryless Matrix entries n Fast fading Channel varies much faster than delay requirement Coherence time (Tc): period of variation of channel MIT 6. 441 Capacity of multi-antenna Gaussian channels (Telatar) Imad Jabbour
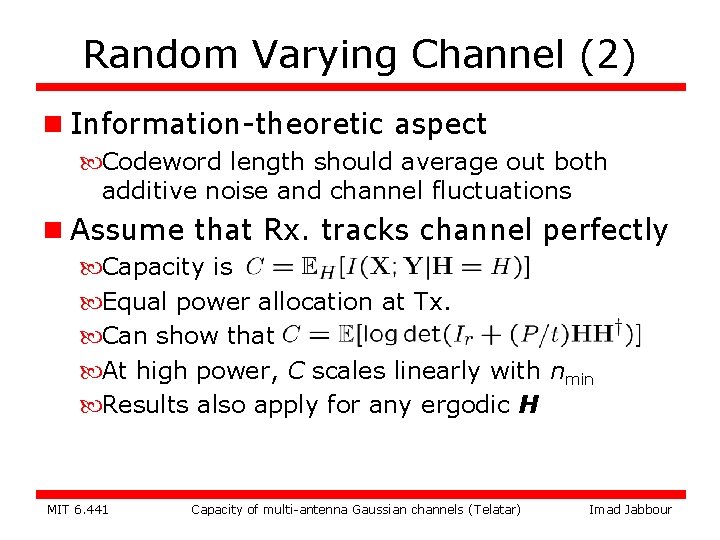
Random Varying Channel (2) n Information-theoretic aspect Codeword length should average out both additive noise and channel fluctuations n Assume that Rx. tracks channel perfectly Capacity is Equal power allocation at Tx. Can show that At high power, C scales linearly with nmin Results also apply for any ergodic H MIT 6. 441 Capacity of multi-antenna Gaussian channels (Telatar) Imad Jabbour
![Random Varying Channel 3 n MIMO capacity versus SNR from 2 MIT 6 441 Random Varying Channel (3) n MIMO capacity versus SNR (from [2]) MIT 6. 441](https://slidetodoc.com/presentation_image_h/80f95931d41a44252e3c7d9acea4ee92/image-11.jpg)
Random Varying Channel (3) n MIMO capacity versus SNR (from [2]) MIT 6. 441 Capacity of multi-antenna Gaussian channels (Telatar) Imad Jabbour
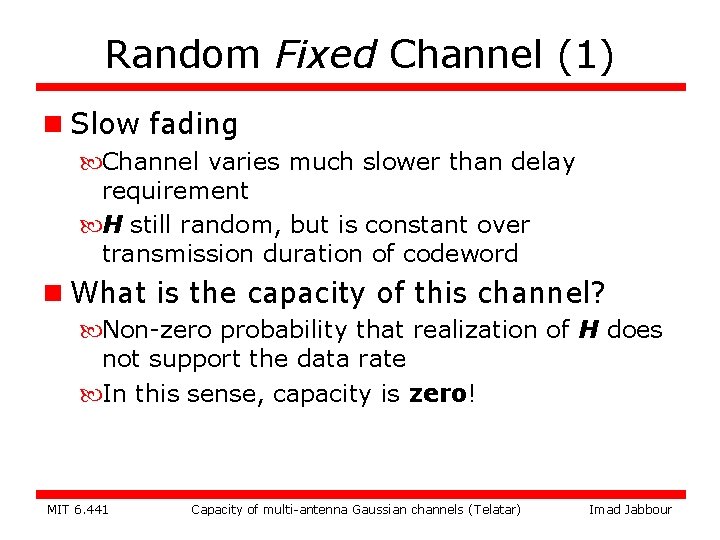
Random Fixed Channel (1) n Slow fading Channel varies much slower than delay requirement H still random, but is constant over transmission duration of codeword n What is the capacity of this channel? Non-zero probability that realization of H does not support the data rate In this sense, capacity is zero! MIT 6. 441 Capacity of multi-antenna Gaussian channels (Telatar) Imad Jabbour
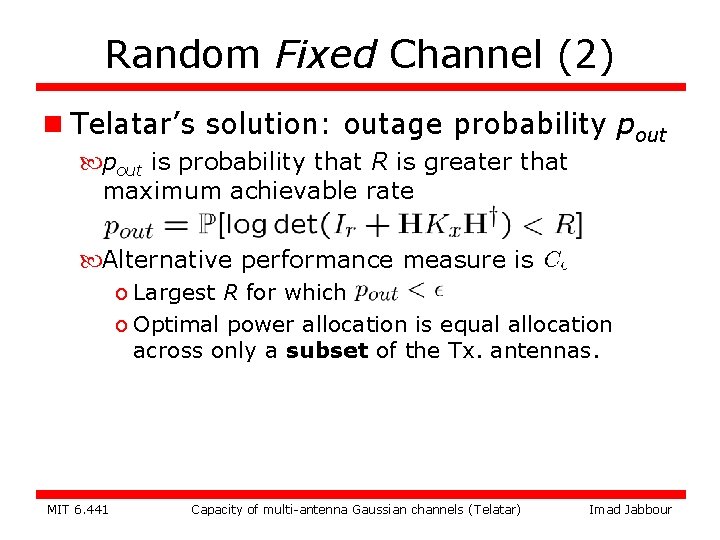
Random Fixed Channel (2) n Telatar’s solution: outage probability pout is probability that R is greater that maximum achievable rate Alternative performance measure is o Largest R for which o Optimal power allocation is equal allocation across only a subset of the Tx. antennas. MIT 6. 441 Capacity of multi-antenna Gaussian channels (Telatar) Imad Jabbour
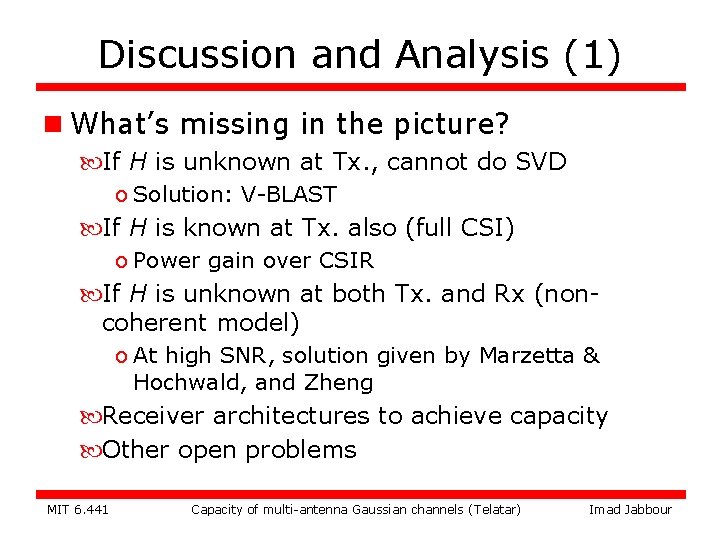
Discussion and Analysis (1) n What’s missing in the picture? If H is unknown at Tx. , cannot do SVD o Solution: V-BLAST If H is known at Tx. also (full CSI) o Power gain over CSIR If H is unknown at both Tx. and Rx (noncoherent model) o At high SNR, solution given by Marzetta & Hochwald, and Zheng Receiver architectures to achieve capacity Other open problems MIT 6. 441 Capacity of multi-antenna Gaussian channels (Telatar) Imad Jabbour
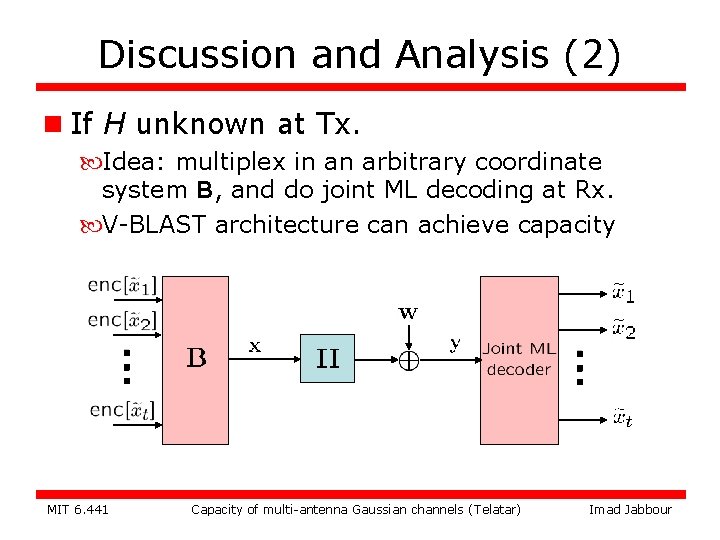
Discussion and Analysis (2) n If H unknown at Tx. Idea: multiplex in an arbitrary coordinate system B, and do joint ML decoding at Rx. V-BLAST architecture can achieve capacity MIT 6. 441 Capacity of multi-antenna Gaussian channels (Telatar) Imad Jabbour
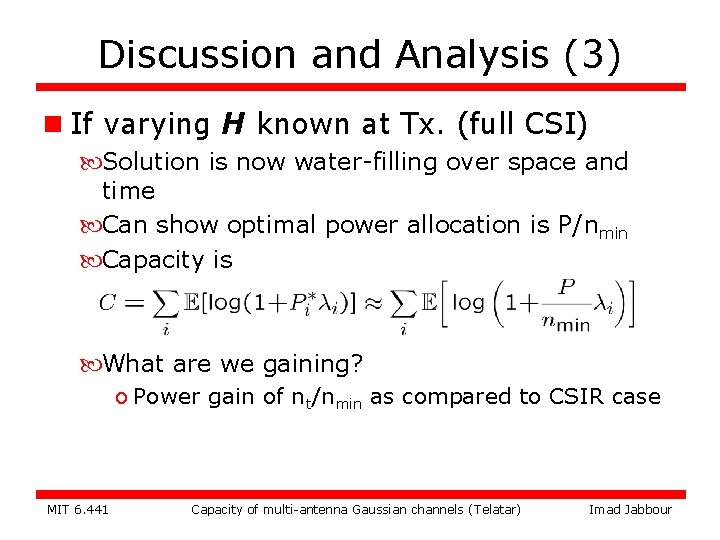
Discussion and Analysis (3) n If varying H known at Tx. (full CSI) Solution is now water-filling over space and time Can show optimal power allocation is P/nmin Capacity is What are we gaining? o Power gain of nt/nmin as compared to CSIR case MIT 6. 441 Capacity of multi-antenna Gaussian channels (Telatar) Imad Jabbour
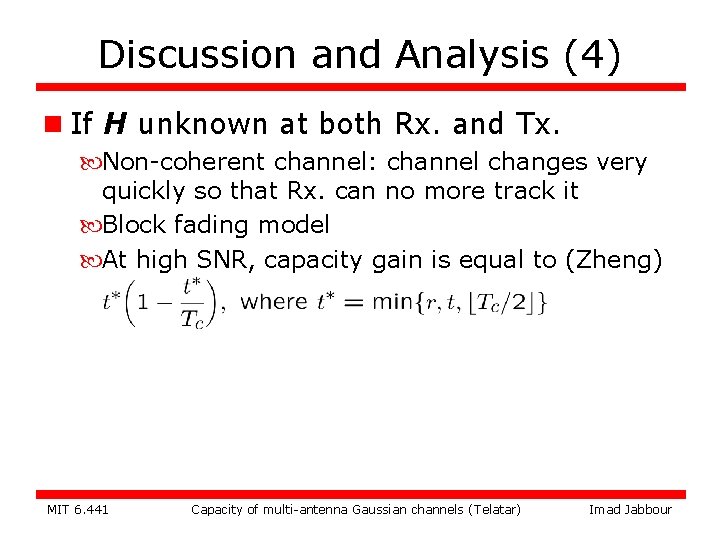
Discussion and Analysis (4) n If H unknown at both Rx. and Tx. Non-coherent channel: channel changes very quickly so that Rx. can no more track it Block fading model At high SNR, capacity gain is equal to (Zheng) MIT 6. 441 Capacity of multi-antenna Gaussian channels (Telatar) Imad Jabbour
![Discussion and Analysis 5 n Receiver architectures 2 VBLAST can achieve capacity for fast Discussion and Analysis (5) n Receiver architectures [2] V-BLAST can achieve capacity for fast](https://slidetodoc.com/presentation_image_h/80f95931d41a44252e3c7d9acea4ee92/image-18.jpg)
Discussion and Analysis (5) n Receiver architectures [2] V-BLAST can achieve capacity for fast Rayleigh -fading channels Caveat: Complexity of joint decoding Solution: simpler linear decoders o Zero-forcing receiver (decorrelator) o MMSE receiver o MMSE can achieve capacity if SIC is used MIT 6. 441 Capacity of multi-antenna Gaussian channels (Telatar) Imad Jabbour
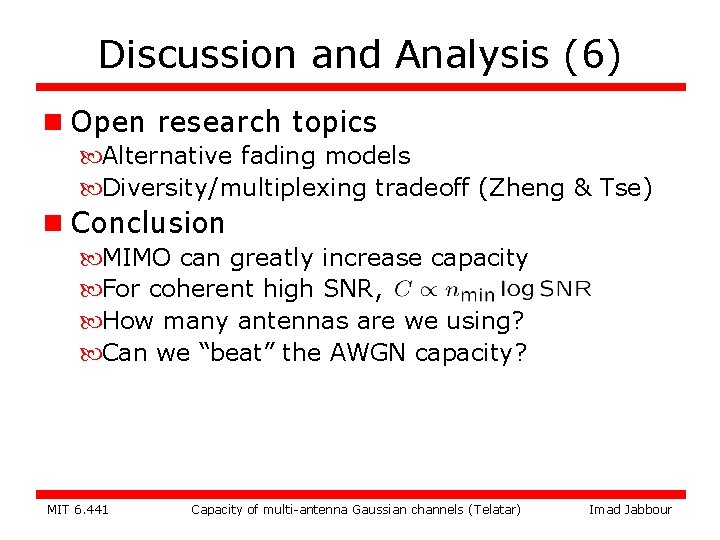
Discussion and Analysis (6) n Open research topics Alternative fading models Diversity/multiplexing tradeoff (Zheng & Tse) n Conclusion MIMO can greatly increase capacity For coherent high SNR, How many antennas are we using? Can we “beat” the AWGN capacity? MIT 6. 441 Capacity of multi-antenna Gaussian channels (Telatar) Imad Jabbour
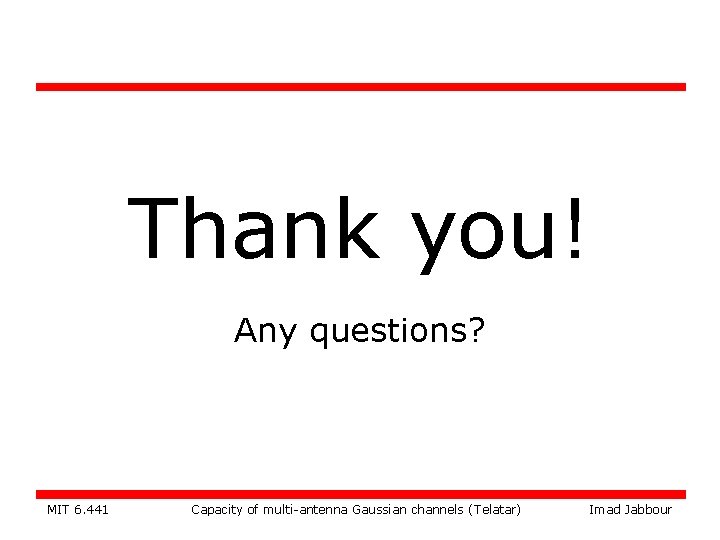
Thank you! Any questions? MIT 6. 441 Capacity of multi-antenna Gaussian channels (Telatar) Imad Jabbour