Lecture 7 Psychology Scams etc continued Security Computer
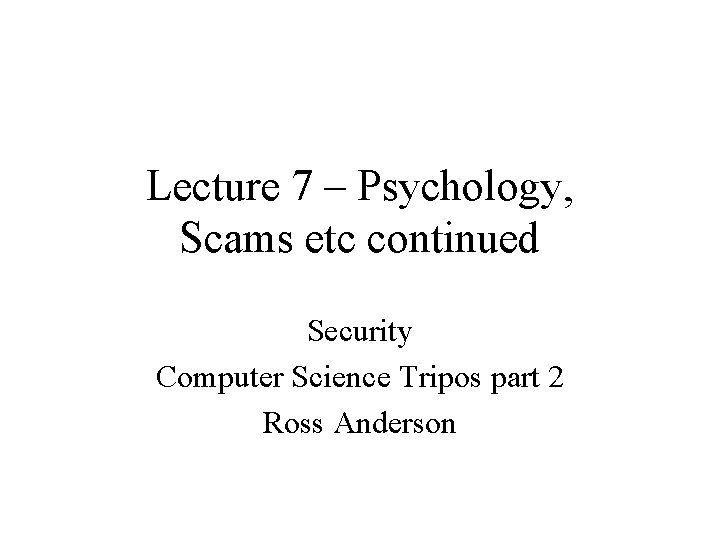
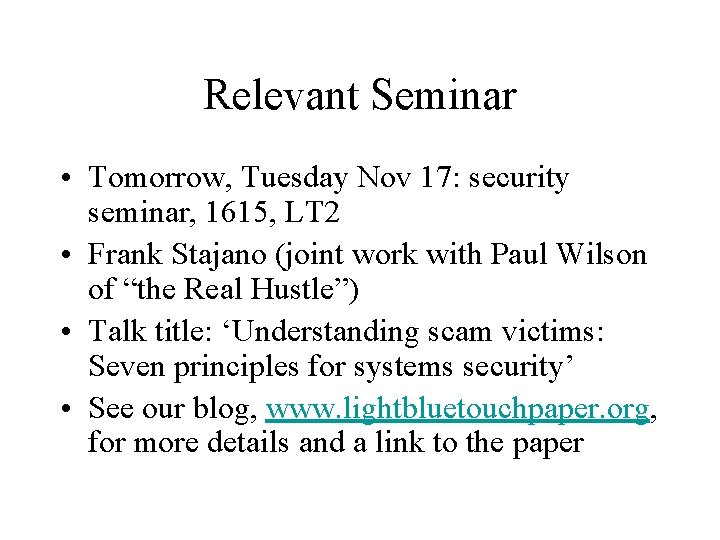
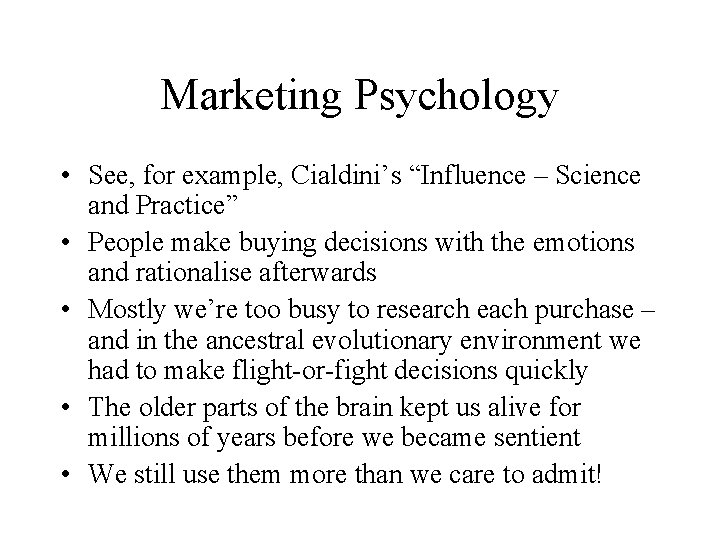
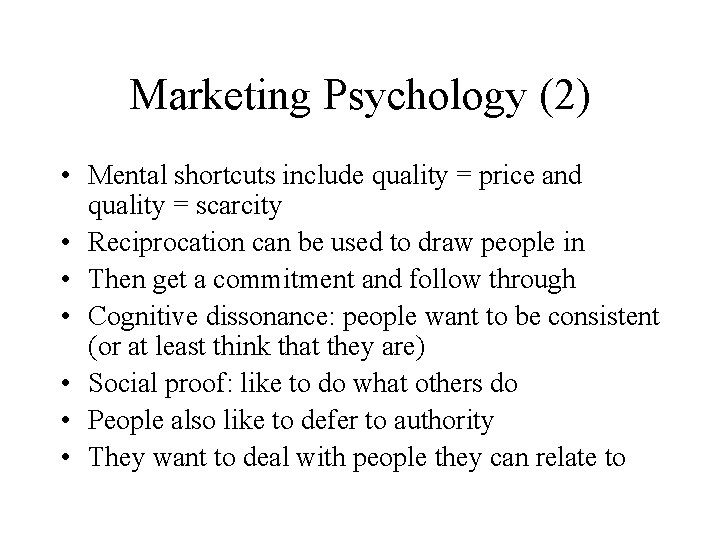
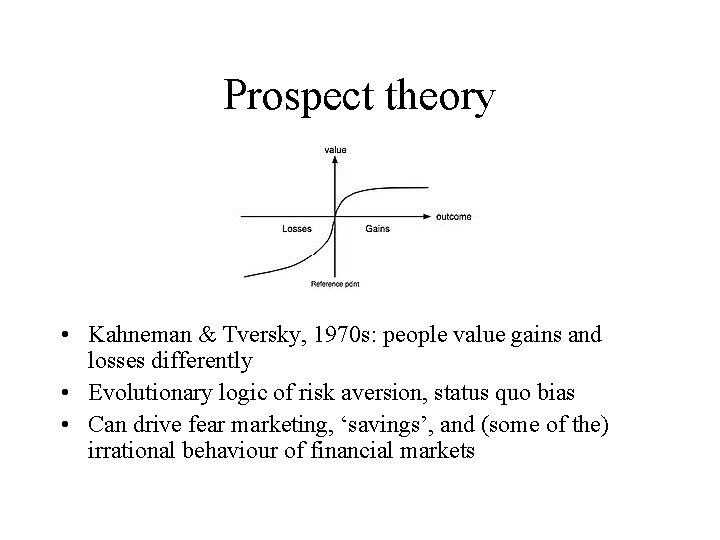
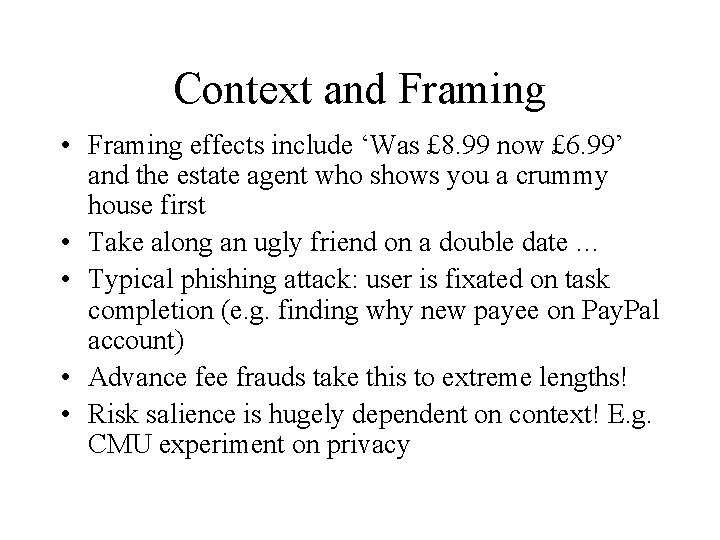
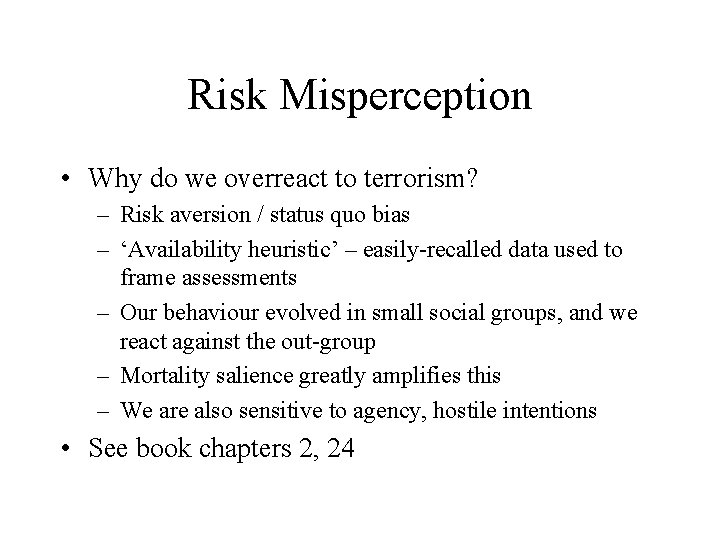
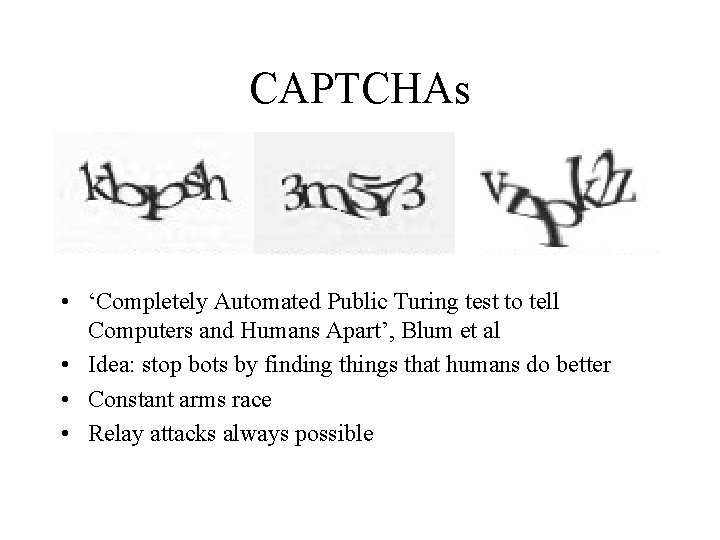
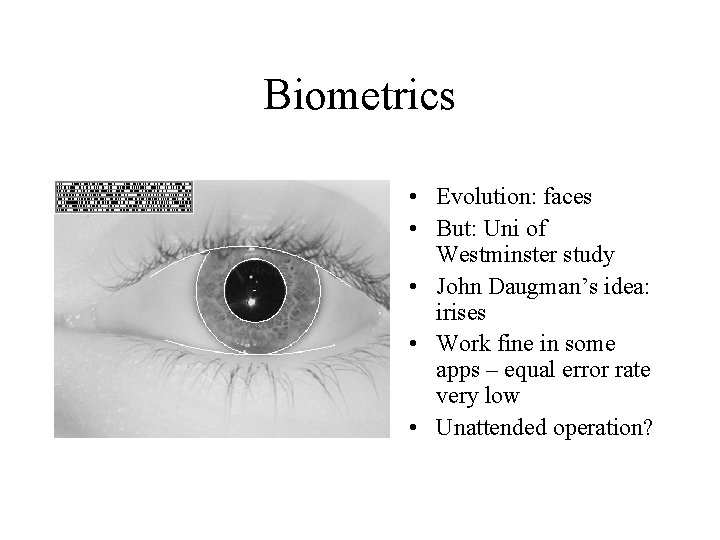
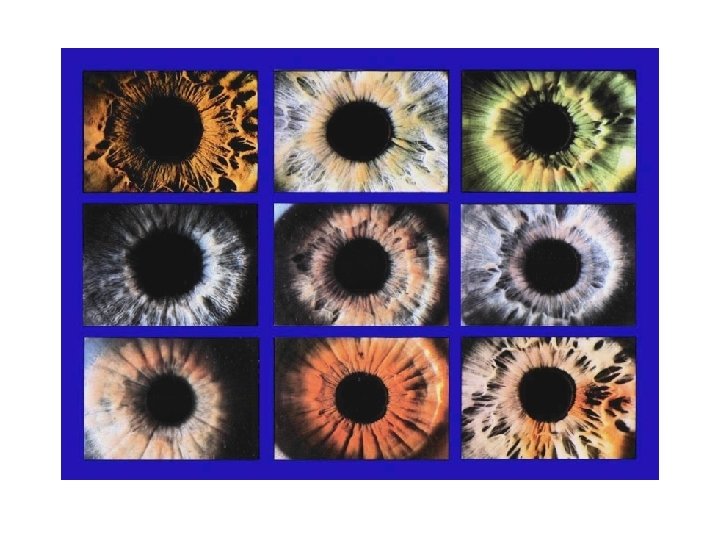
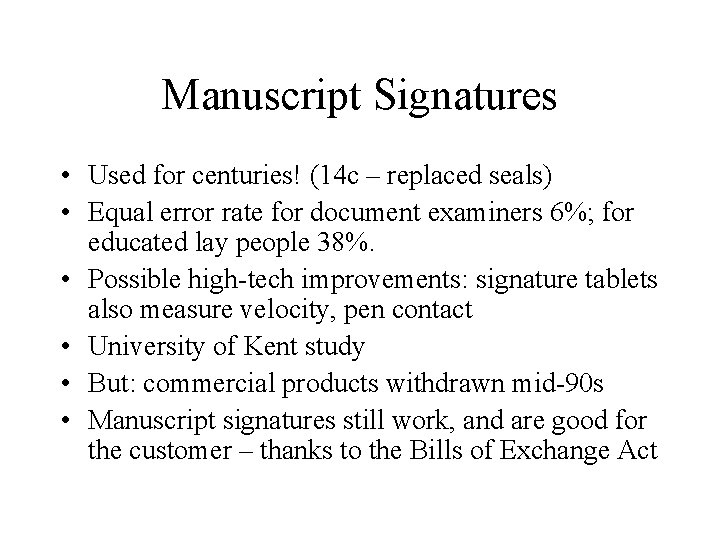
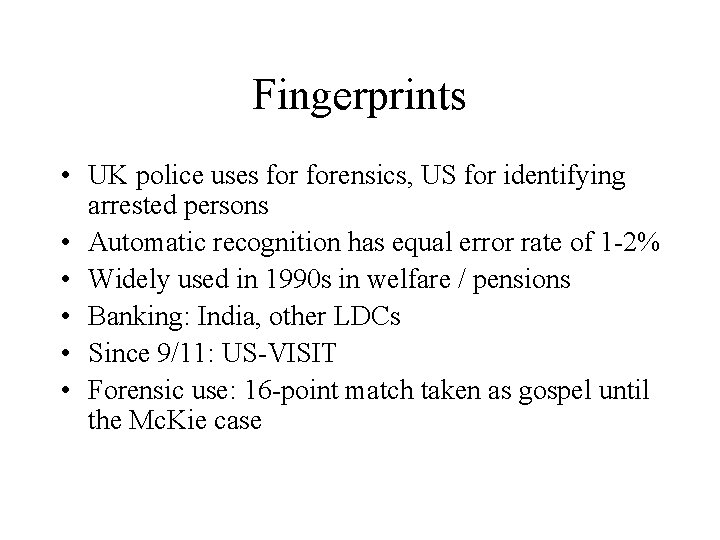
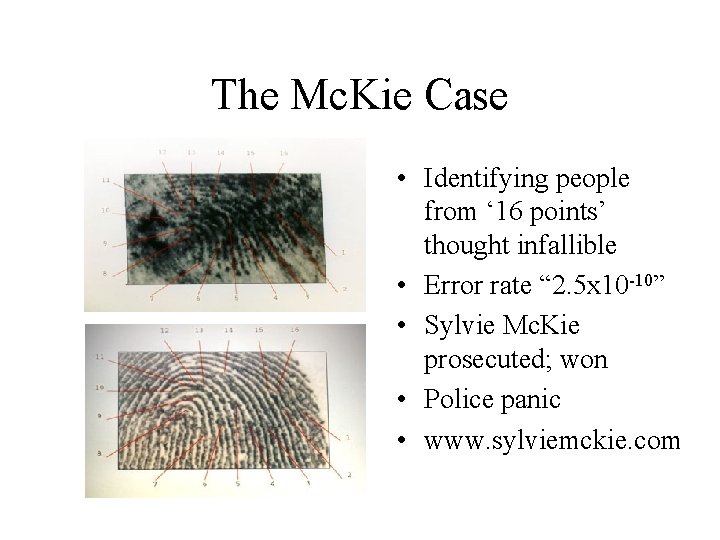
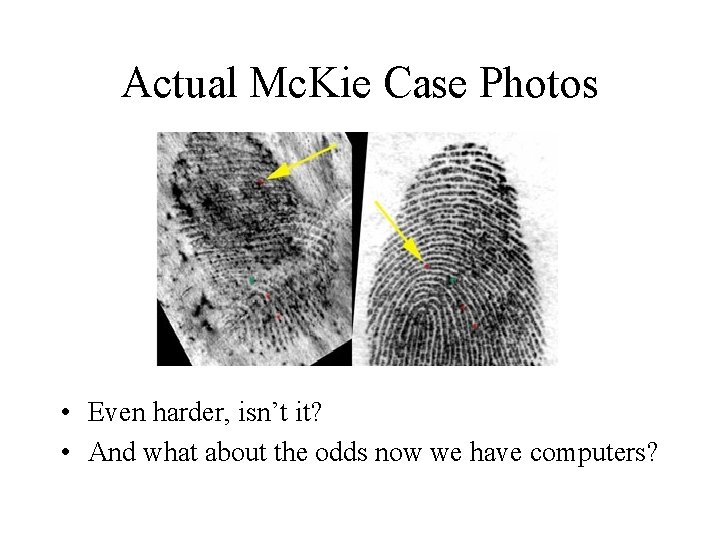
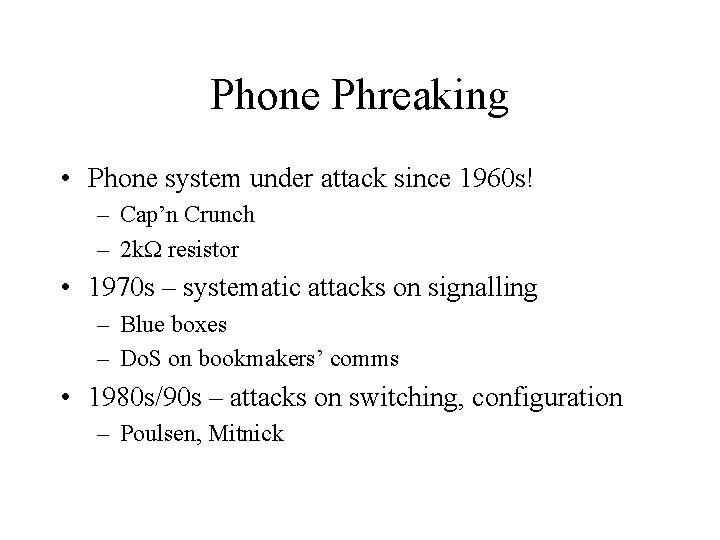
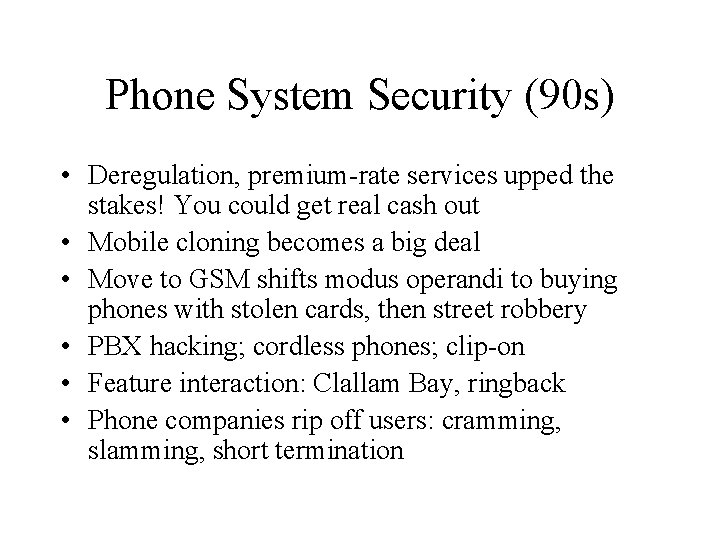
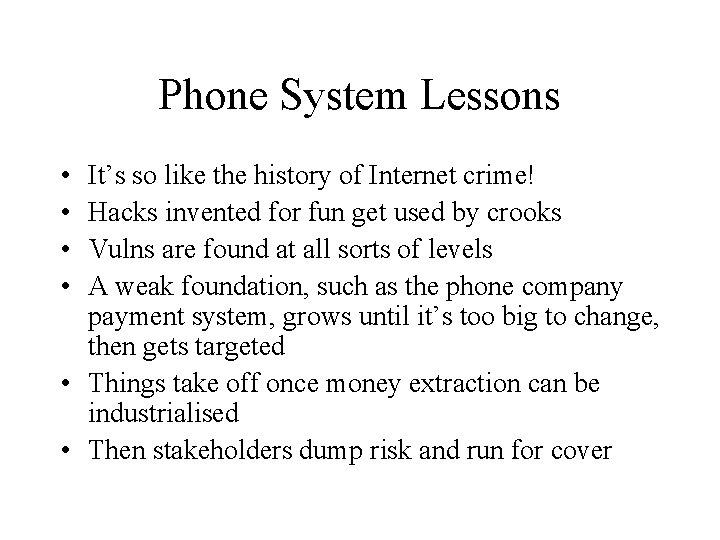
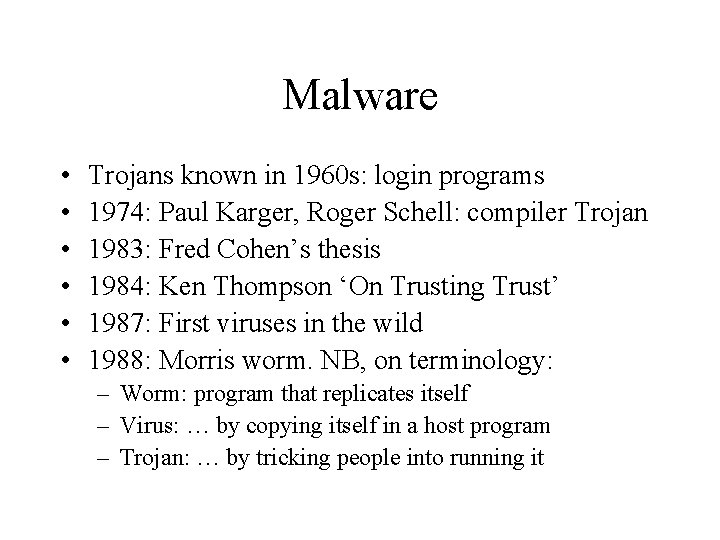
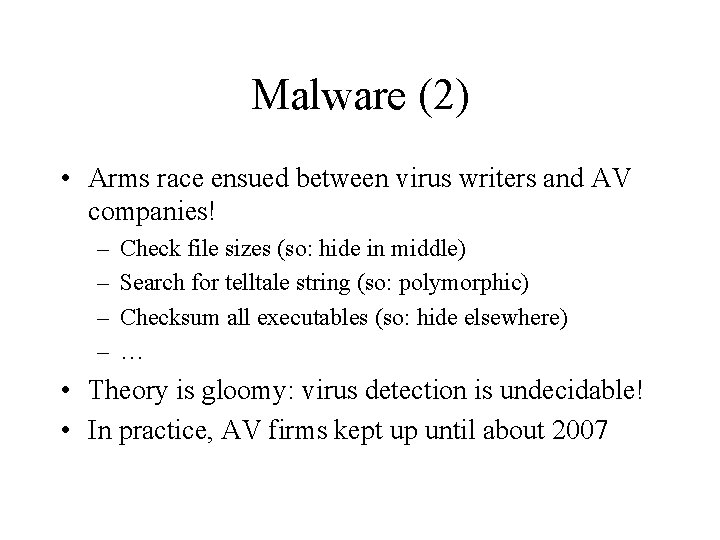
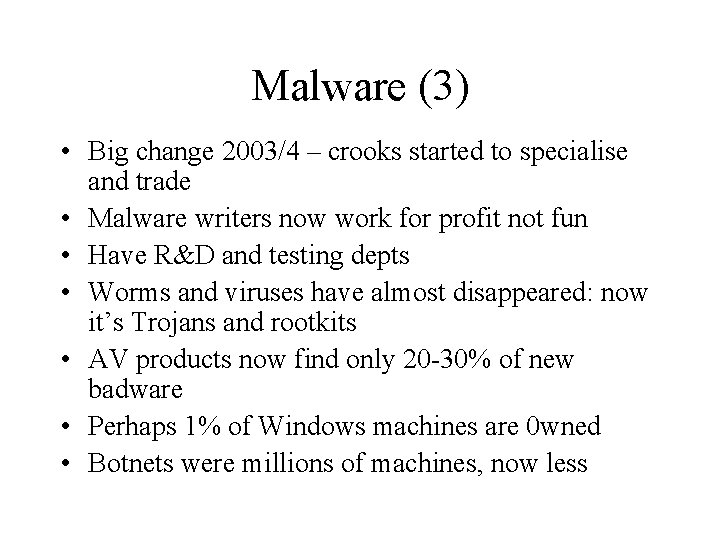
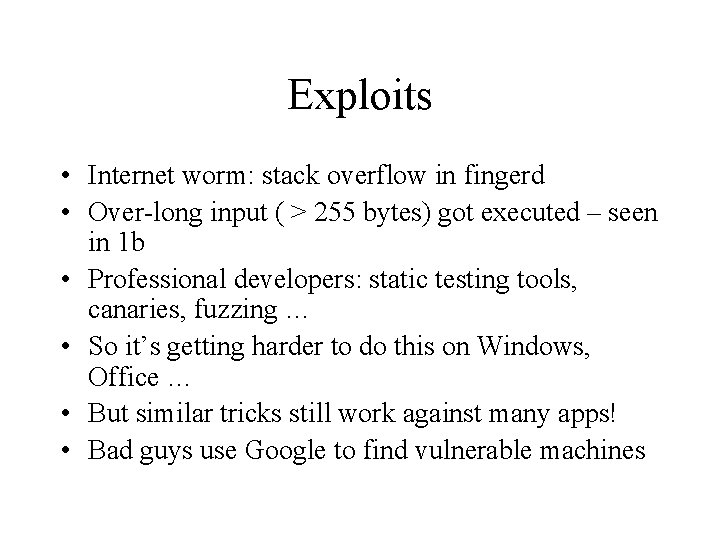
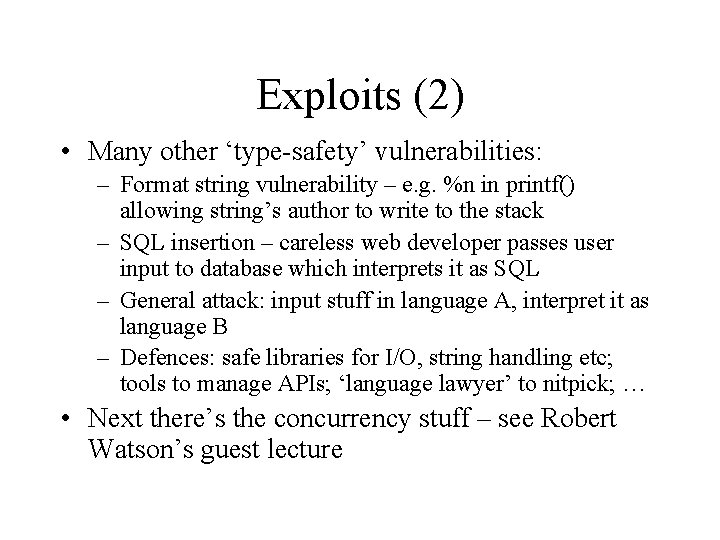
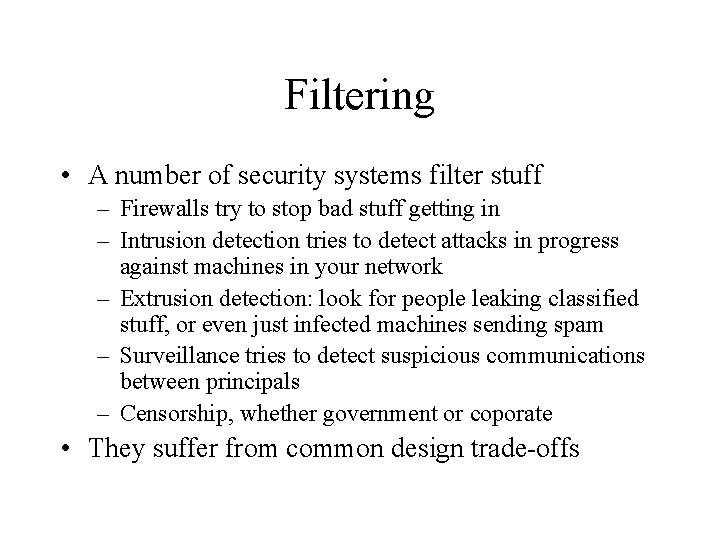
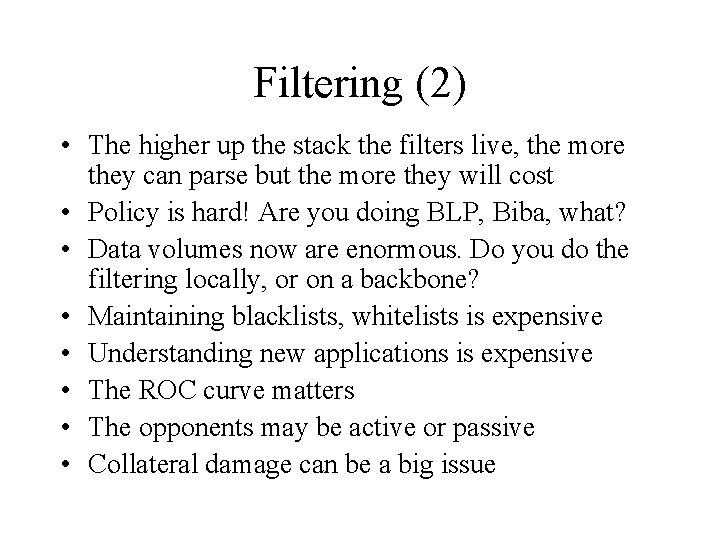
- Slides: 24
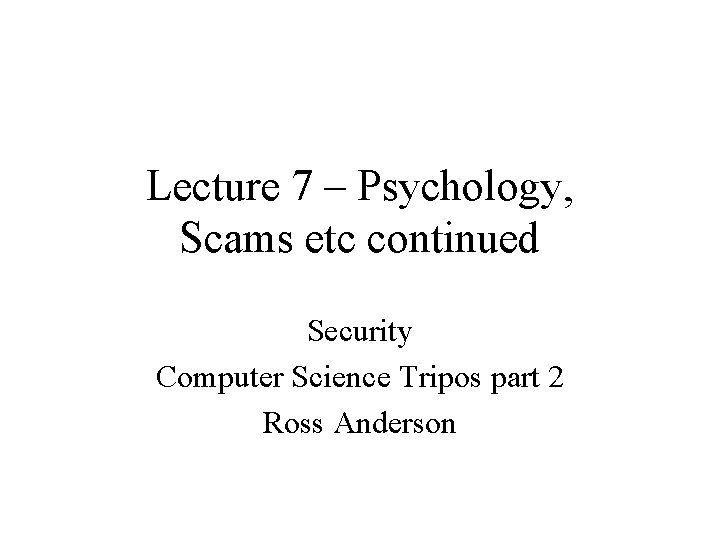
Lecture 7 – Psychology, Scams etc continued Security Computer Science Tripos part 2 Ross Anderson
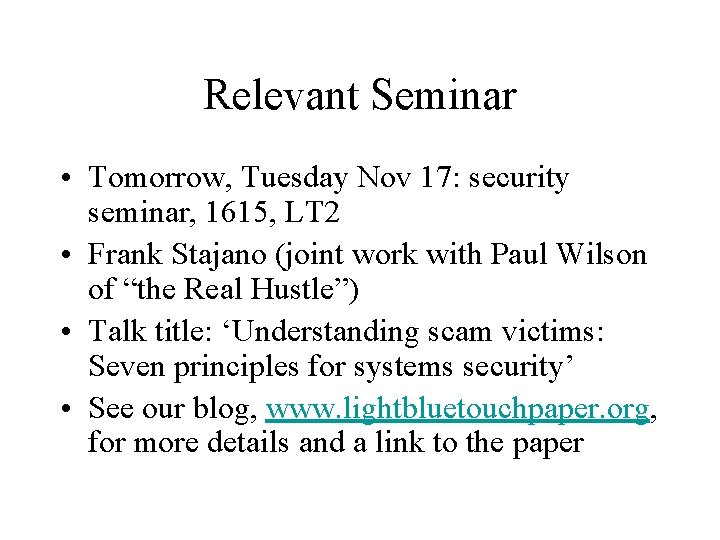
Relevant Seminar • Tomorrow, Tuesday Nov 17: security seminar, 1615, LT 2 • Frank Stajano (joint work with Paul Wilson of “the Real Hustle”) • Talk title: ‘Understanding scam victims: Seven principles for systems security’ • See our blog, www. lightbluetouchpaper. org, for more details and a link to the paper
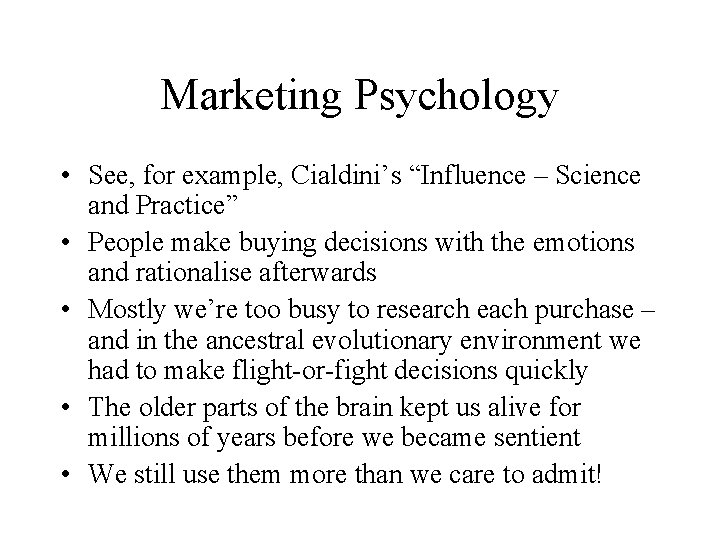
Marketing Psychology • See, for example, Cialdini’s “Influence – Science and Practice” • People make buying decisions with the emotions and rationalise afterwards • Mostly we’re too busy to research each purchase – and in the ancestral evolutionary environment we had to make flight-or-fight decisions quickly • The older parts of the brain kept us alive for millions of years before we became sentient • We still use them more than we care to admit!
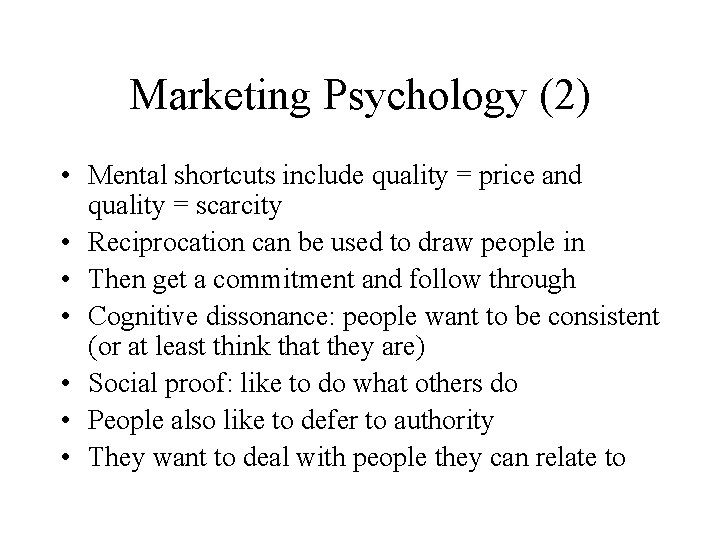
Marketing Psychology (2) • Mental shortcuts include quality = price and quality = scarcity • Reciprocation can be used to draw people in • Then get a commitment and follow through • Cognitive dissonance: people want to be consistent (or at least think that they are) • Social proof: like to do what others do • People also like to defer to authority • They want to deal with people they can relate to
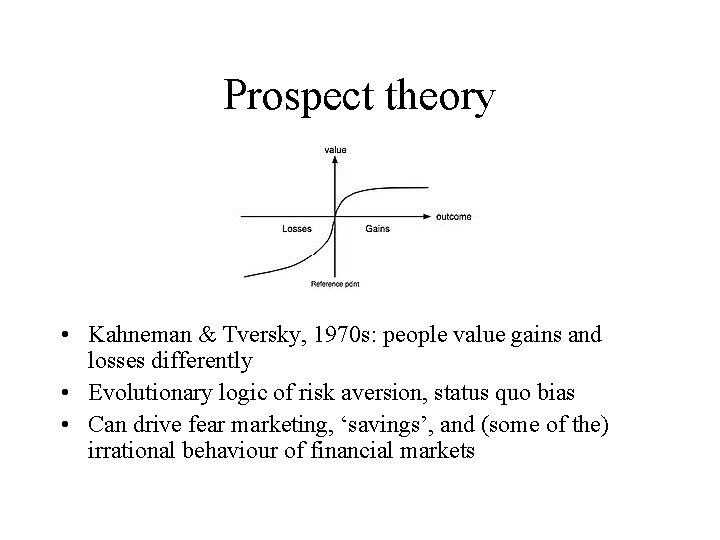
Prospect theory • Kahneman & Tversky, 1970 s: people value gains and losses differently • Evolutionary logic of risk aversion, status quo bias • Can drive fear marketing, ‘savings’, and (some of the) irrational behaviour of financial markets
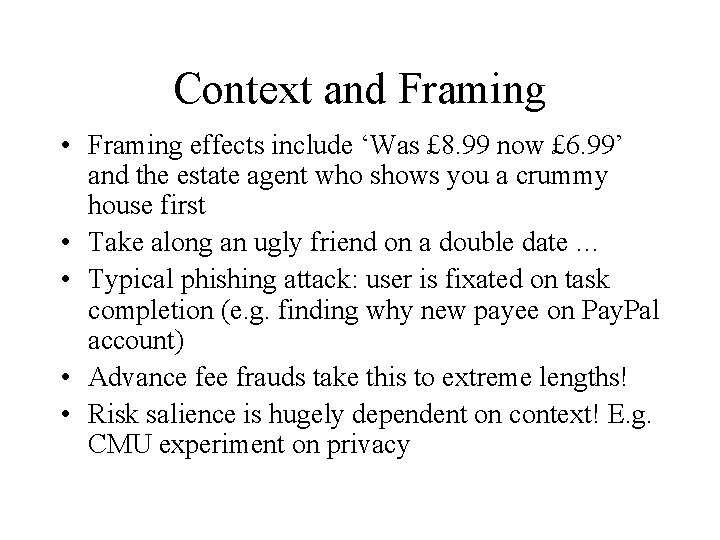
Context and Framing • Framing effects include ‘Was £ 8. 99 now £ 6. 99’ and the estate agent who shows you a crummy house first • Take along an ugly friend on a double date … • Typical phishing attack: user is fixated on task completion (e. g. finding why new payee on Pay. Pal account) • Advance fee frauds take this to extreme lengths! • Risk salience is hugely dependent on context! E. g. CMU experiment on privacy
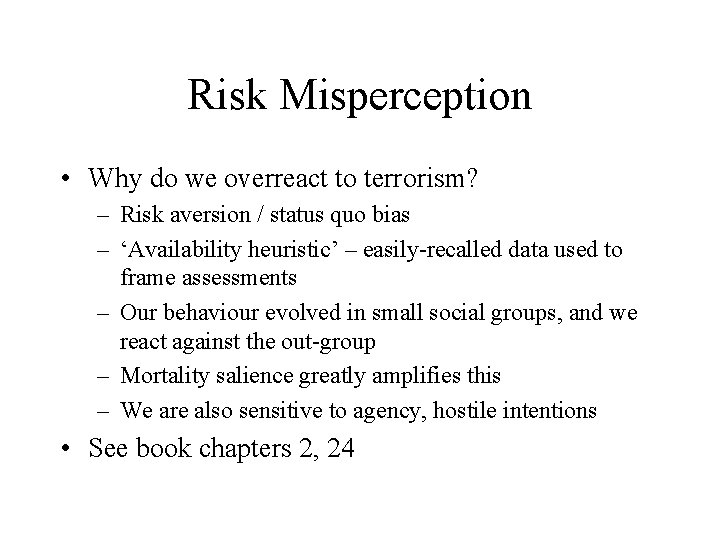
Risk Misperception • Why do we overreact to terrorism? – Risk aversion / status quo bias – ‘Availability heuristic’ – easily-recalled data used to frame assessments – Our behaviour evolved in small social groups, and we react against the out-group – Mortality salience greatly amplifies this – We are also sensitive to agency, hostile intentions • See book chapters 2, 24
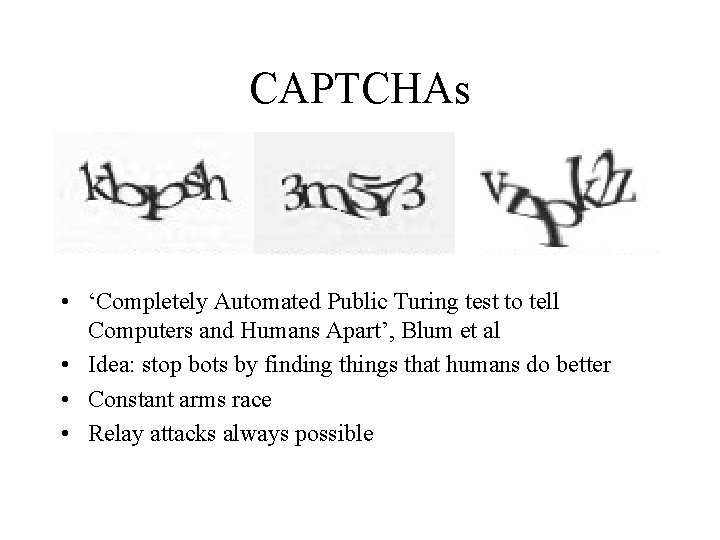
CAPTCHAs • ‘Completely Automated Public Turing test to tell Computers and Humans Apart’, Blum et al • Idea: stop bots by finding things that humans do better • Constant arms race • Relay attacks always possible
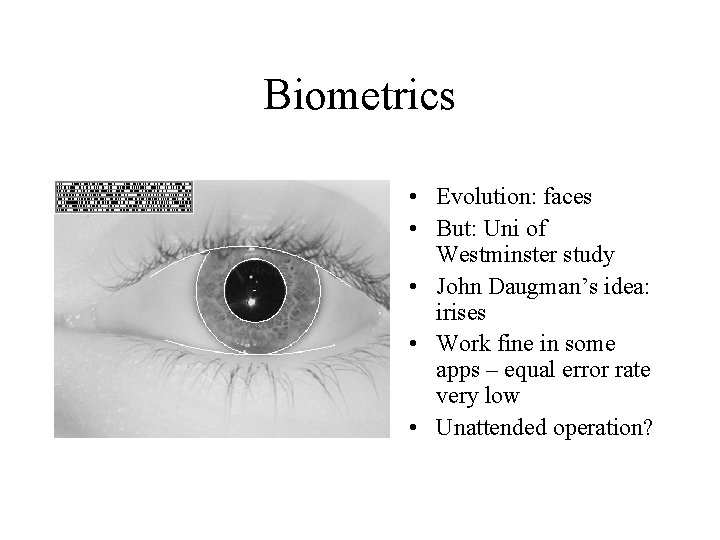
Biometrics • Evolution: faces • But: Uni of Westminster study • John Daugman’s idea: irises • Work fine in some apps – equal error rate very low • Unattended operation?
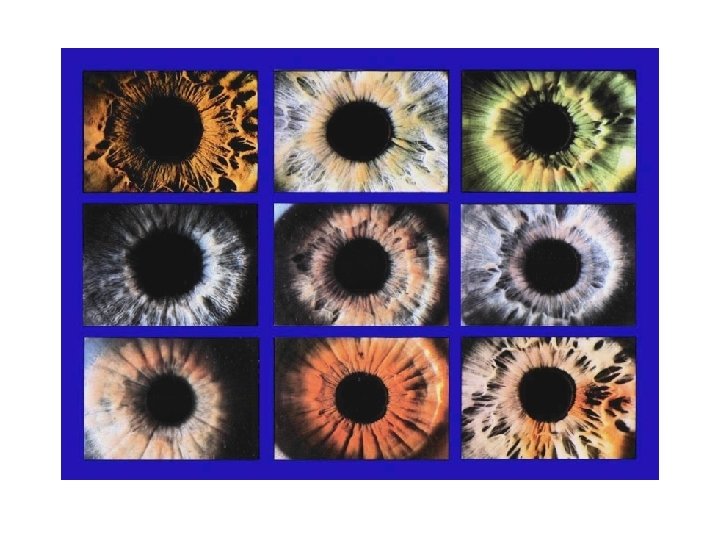
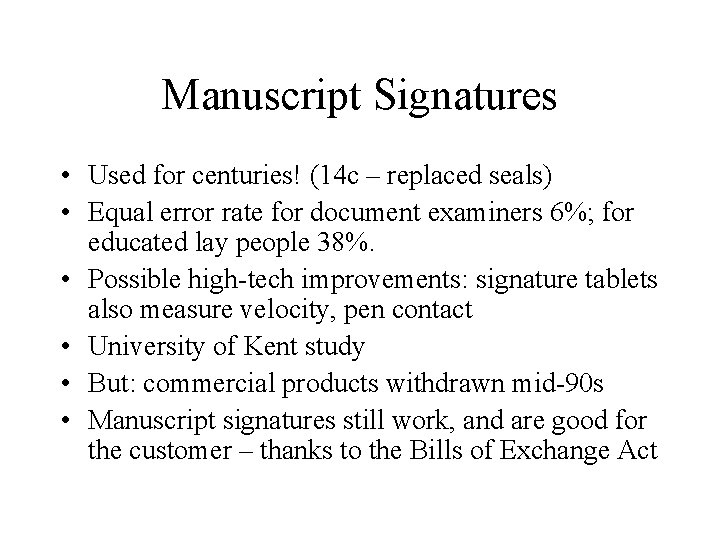
Manuscript Signatures • Used for centuries! (14 c – replaced seals) • Equal error rate for document examiners 6%; for educated lay people 38%. • Possible high-tech improvements: signature tablets also measure velocity, pen contact • University of Kent study • But: commercial products withdrawn mid-90 s • Manuscript signatures still work, and are good for the customer – thanks to the Bills of Exchange Act
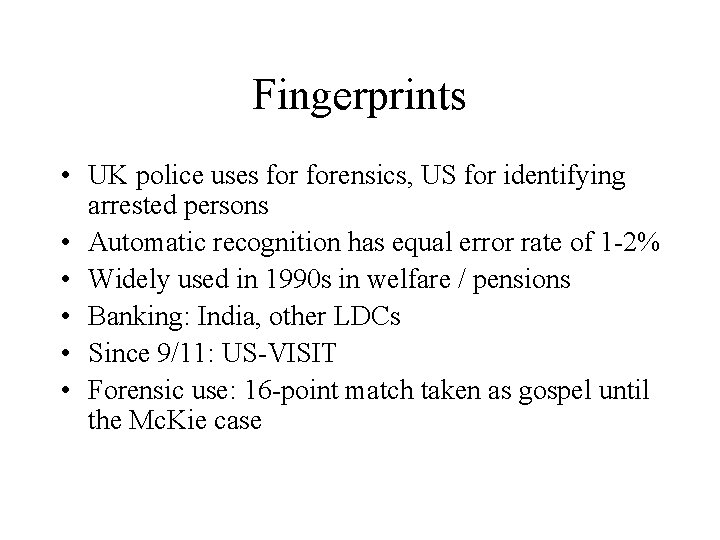
Fingerprints • UK police uses forensics, US for identifying arrested persons • Automatic recognition has equal error rate of 1 -2% • Widely used in 1990 s in welfare / pensions • Banking: India, other LDCs • Since 9/11: US-VISIT • Forensic use: 16 -point match taken as gospel until the Mc. Kie case
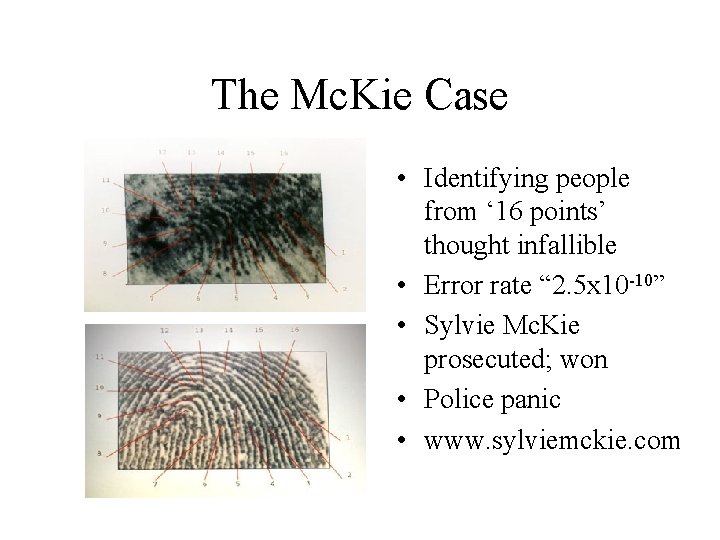
The Mc. Kie Case • Identifying people from ‘ 16 points’ thought infallible • Error rate “ 2. 5 x 10 -10” • Sylvie Mc. Kie prosecuted; won • Police panic • www. sylviemckie. com
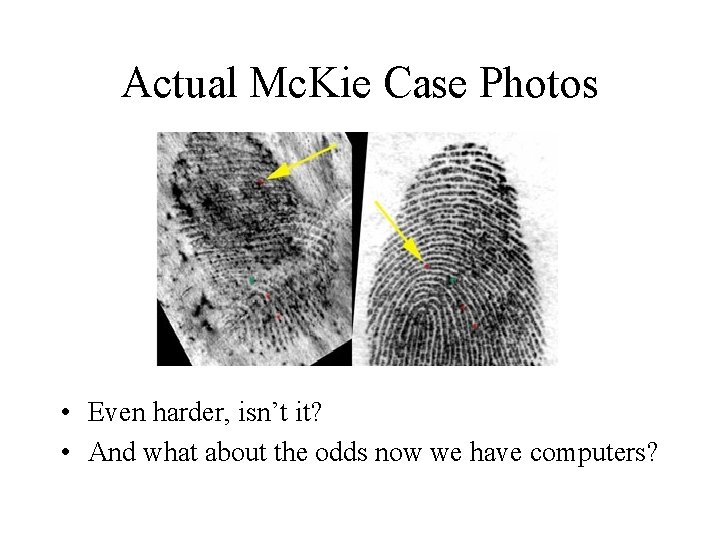
Actual Mc. Kie Case Photos • Even harder, isn’t it? • And what about the odds now we have computers?
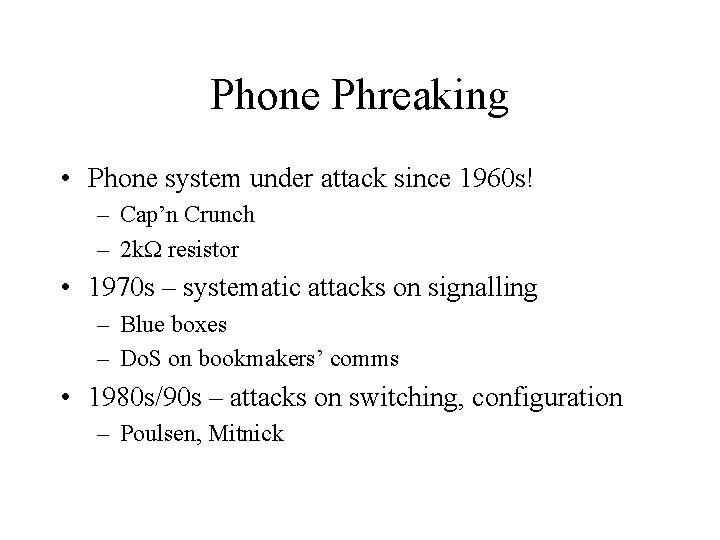
Phone Phreaking • Phone system under attack since 1960 s! – Cap’n Crunch – 2 k resistor • 1970 s – systematic attacks on signalling – Blue boxes – Do. S on bookmakers’ comms • 1980 s/90 s – attacks on switching, configuration – Poulsen, Mitnick
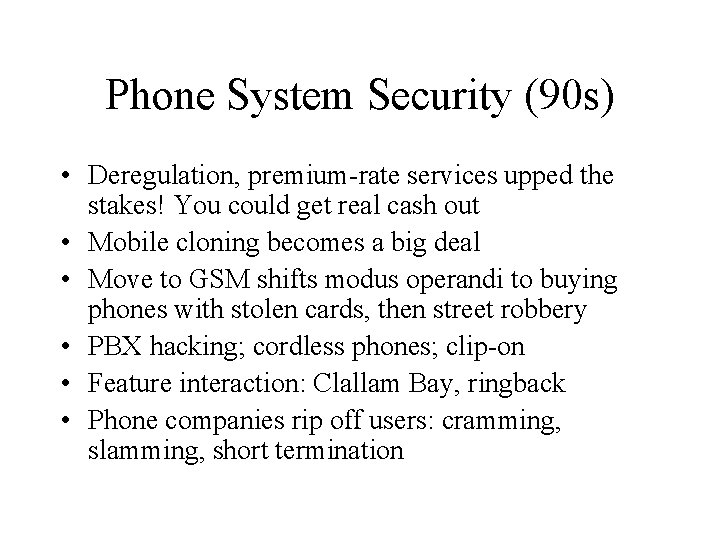
Phone System Security (90 s) • Deregulation, premium-rate services upped the stakes! You could get real cash out • Mobile cloning becomes a big deal • Move to GSM shifts modus operandi to buying phones with stolen cards, then street robbery • PBX hacking; cordless phones; clip-on • Feature interaction: Clallam Bay, ringback • Phone companies rip off users: cramming, slamming, short termination
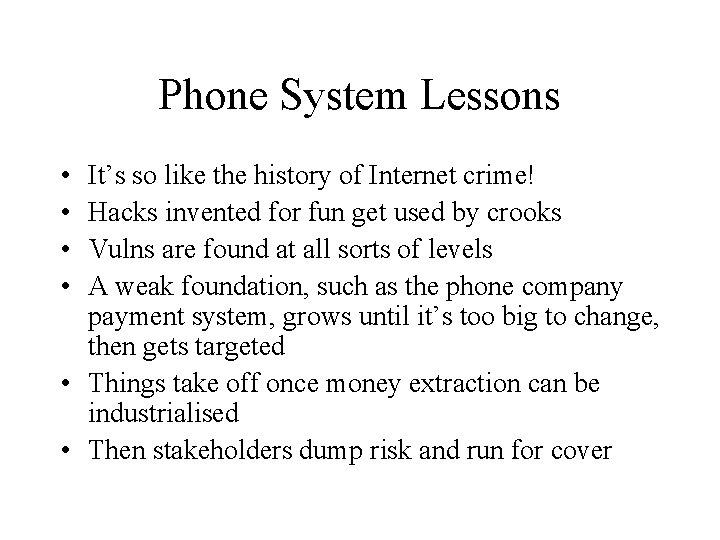
Phone System Lessons • • It’s so like the history of Internet crime! Hacks invented for fun get used by crooks Vulns are found at all sorts of levels A weak foundation, such as the phone company payment system, grows until it’s too big to change, then gets targeted • Things take off once money extraction can be industrialised • Then stakeholders dump risk and run for cover
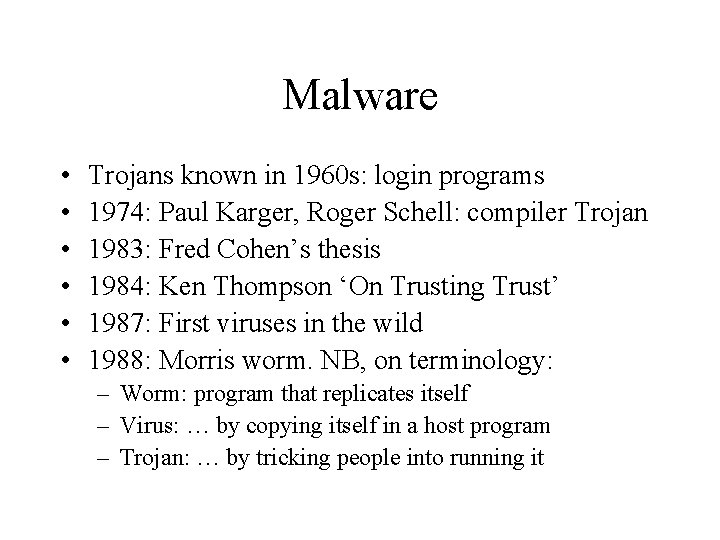
Malware • • • Trojans known in 1960 s: login programs 1974: Paul Karger, Roger Schell: compiler Trojan 1983: Fred Cohen’s thesis 1984: Ken Thompson ‘On Trusting Trust’ 1987: First viruses in the wild 1988: Morris worm. NB, on terminology: – Worm: program that replicates itself – Virus: … by copying itself in a host program – Trojan: … by tricking people into running it
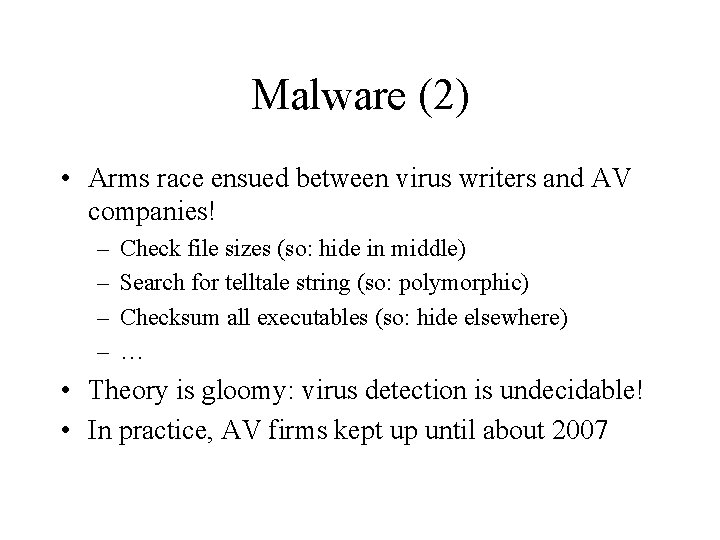
Malware (2) • Arms race ensued between virus writers and AV companies! – – Check file sizes (so: hide in middle) Search for telltale string (so: polymorphic) Checksum all executables (so: hide elsewhere) … • Theory is gloomy: virus detection is undecidable! • In practice, AV firms kept up until about 2007
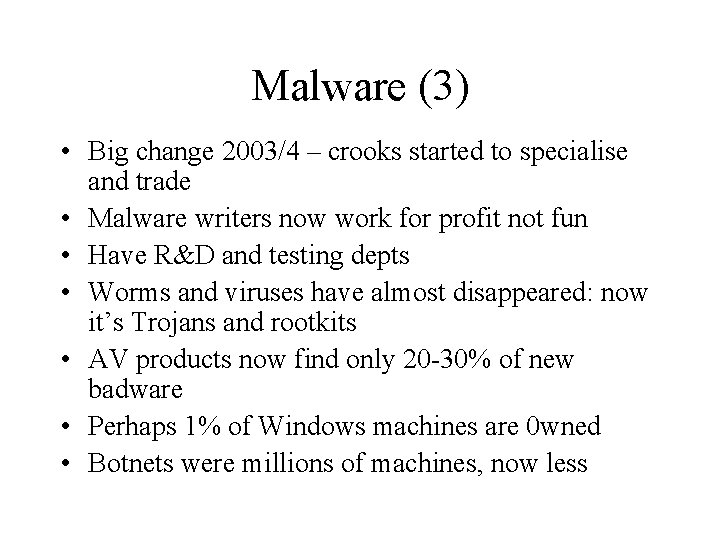
Malware (3) • Big change 2003/4 – crooks started to specialise and trade • Malware writers now work for profit not fun • Have R&D and testing depts • Worms and viruses have almost disappeared: now it’s Trojans and rootkits • AV products now find only 20 -30% of new badware • Perhaps 1% of Windows machines are 0 wned • Botnets were millions of machines, now less
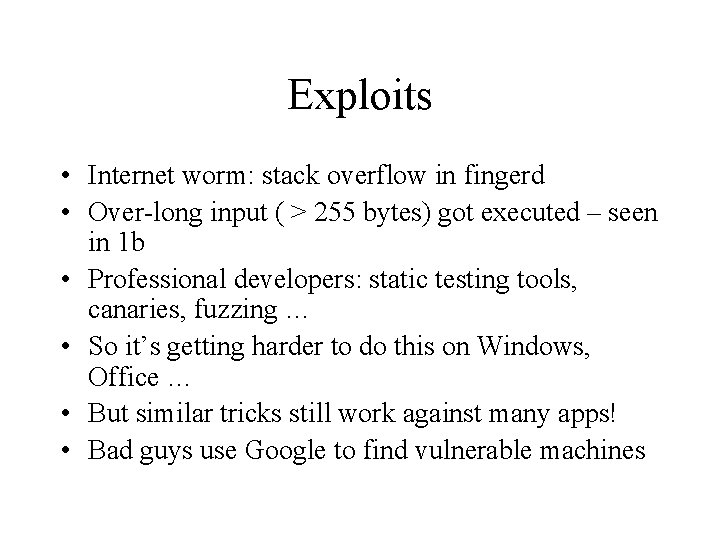
Exploits • Internet worm: stack overflow in fingerd • Over-long input ( > 255 bytes) got executed – seen in 1 b • Professional developers: static testing tools, canaries, fuzzing … • So it’s getting harder to do this on Windows, Office … • But similar tricks still work against many apps! • Bad guys use Google to find vulnerable machines
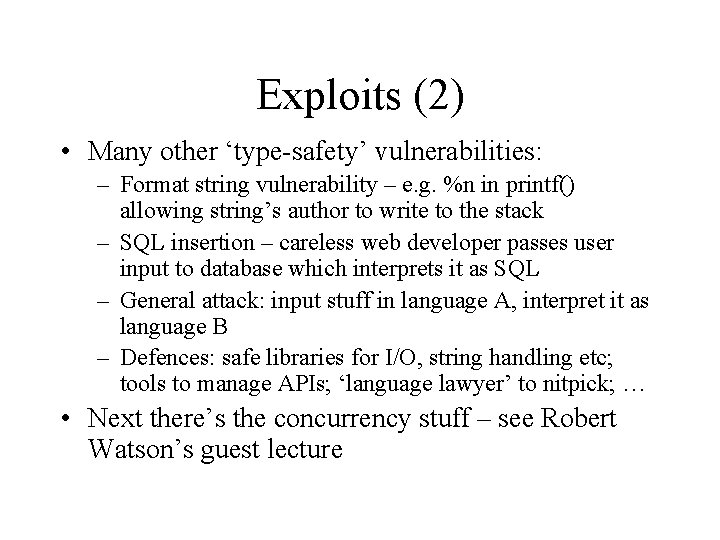
Exploits (2) • Many other ‘type-safety’ vulnerabilities: – Format string vulnerability – e. g. %n in printf() allowing string’s author to write to the stack – SQL insertion – careless web developer passes user input to database which interprets it as SQL – General attack: input stuff in language A, interpret it as language B – Defences: safe libraries for I/O, string handling etc; tools to manage APIs; ‘language lawyer’ to nitpick; … • Next there’s the concurrency stuff – see Robert Watson’s guest lecture
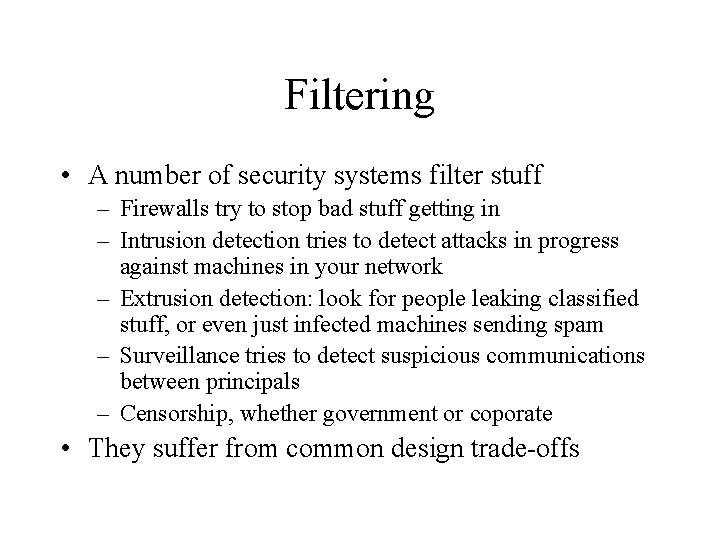
Filtering • A number of security systems filter stuff – Firewalls try to stop bad stuff getting in – Intrusion detection tries to detect attacks in progress against machines in your network – Extrusion detection: look for people leaking classified stuff, or even just infected machines sending spam – Surveillance tries to detect suspicious communications between principals – Censorship, whether government or coporate • They suffer from common design trade-offs
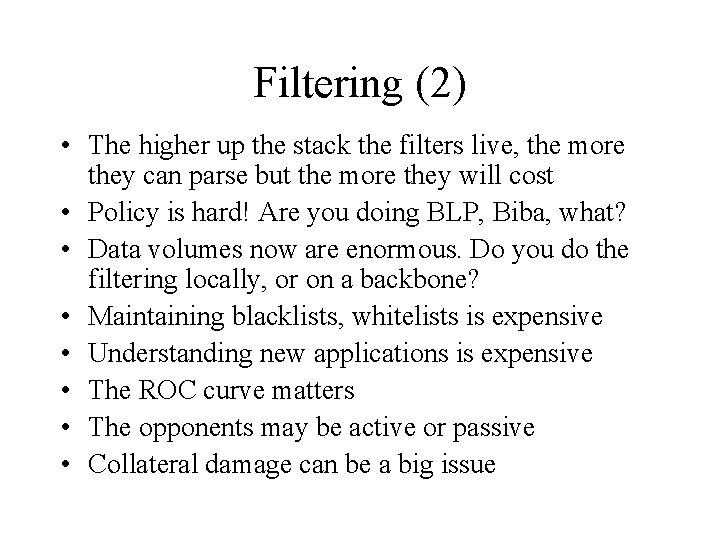
Filtering (2) • The higher up the stack the filters live, the more they can parse but the more they will cost • Policy is hard! Are you doing BLP, Biba, what? • Data volumes now are enormous. Do you do the filtering locally, or on a backbone? • Maintaining blacklists, whitelists is expensive • Understanding new applications is expensive • The ROC curve matters • The opponents may be active or passive • Collateral damage can be a big issue
Computer security 161 cryptocurrency lecture
Private secuirty
01:640:244 lecture notes - lecture 15: plat, idah, farad
Social psychology lecture
Forensic psychology lecture
Introduction to psychology lecture
Psychology lecture for medical students
Computer-aided drug design lecture notes
Computer architecture lecture notes
Isa computer architecture
Osi security architecture in network security
Guide to network security
Wireless security in cryptography
Visa international security model diagram
Electronic mail security in network security
Information security
Electronic commerce security
Software security building security in
Security guide to network security fundamentals
Security guide to network security fundamentals
Romeo and juliet act ii scene 2
Dramatic irony in act 4 of romeo and juliet
Capítulo 4a indirect object pronouns (continued answers)
Position cost distribution
Example of signal word