Estimation of selectivity in Stock Synthesis lessons learned
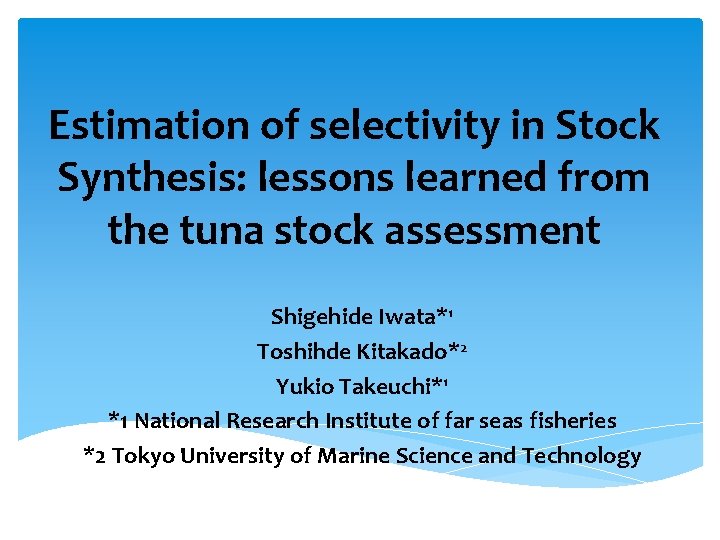
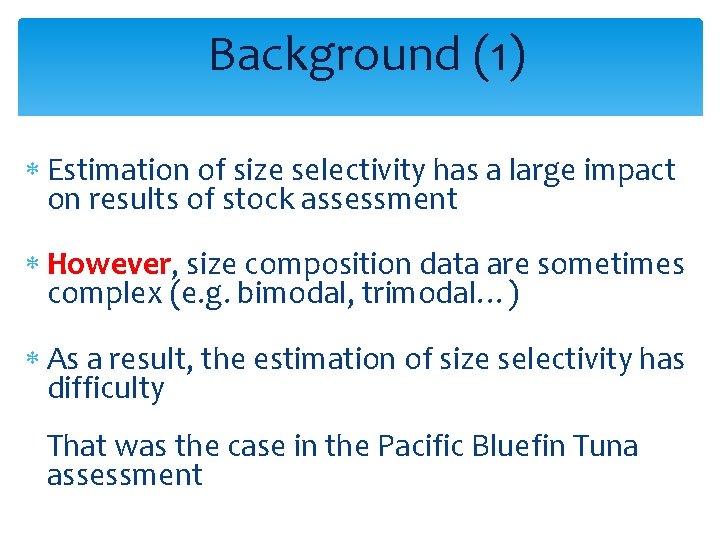
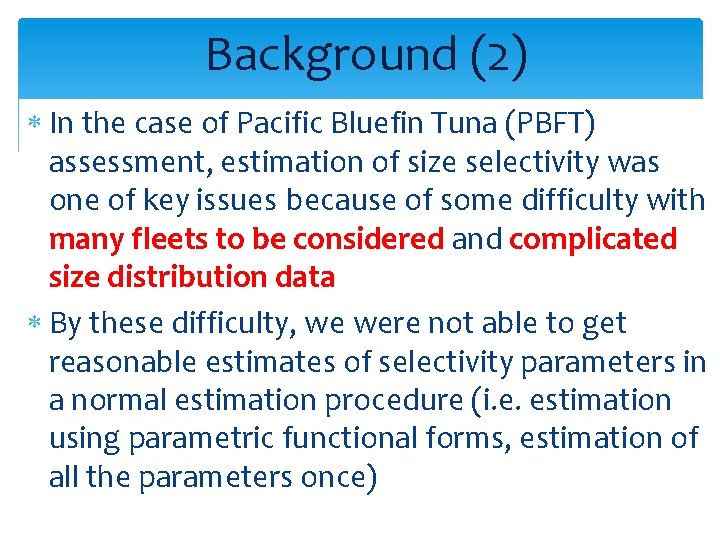
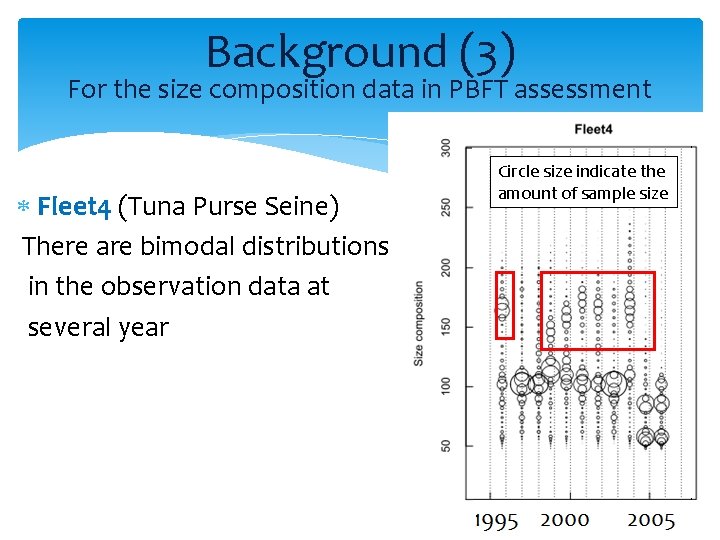
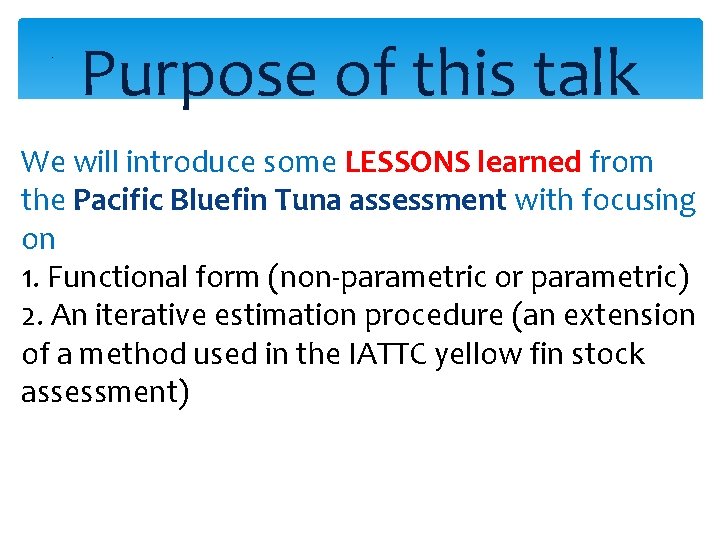
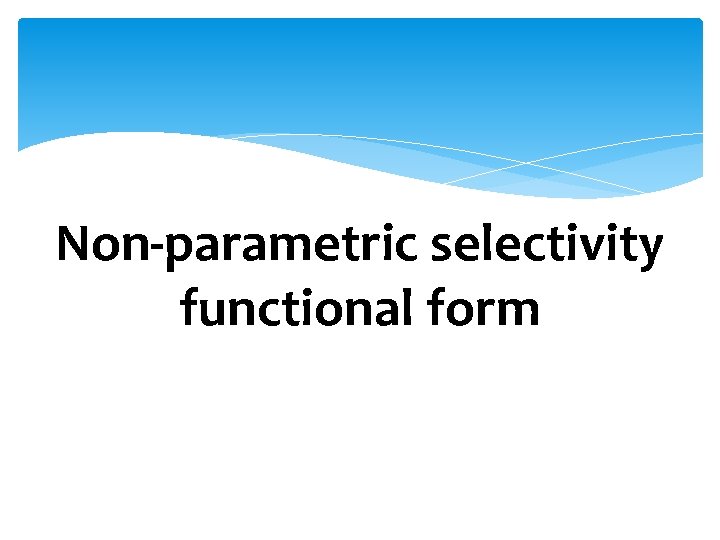
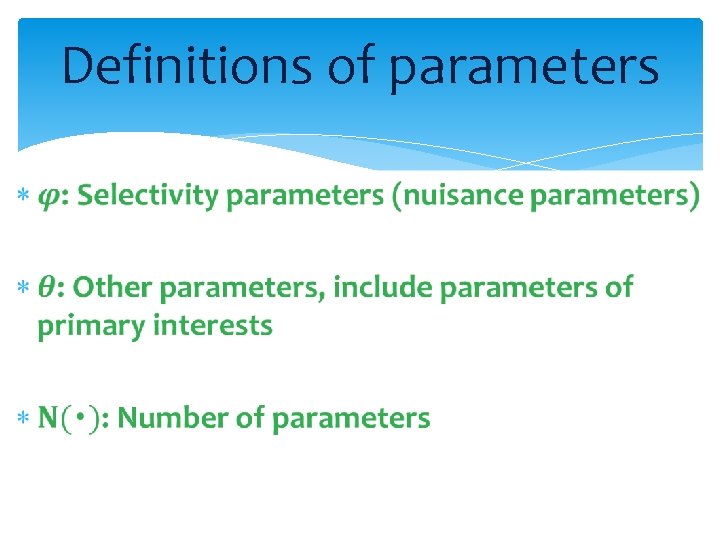
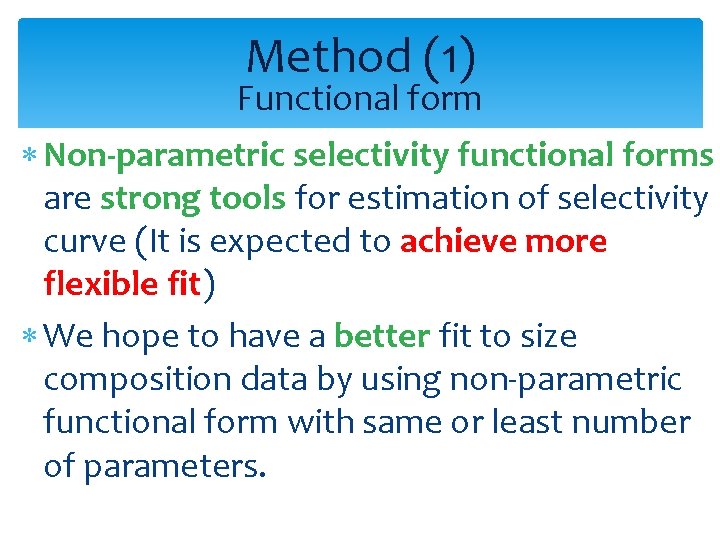
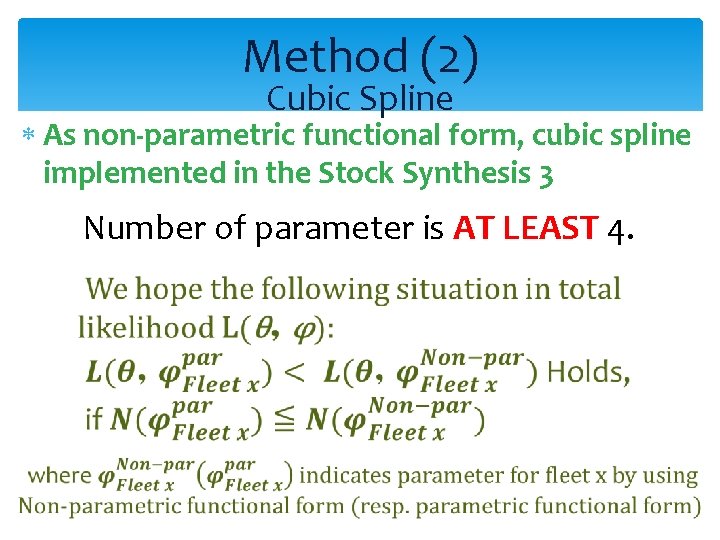
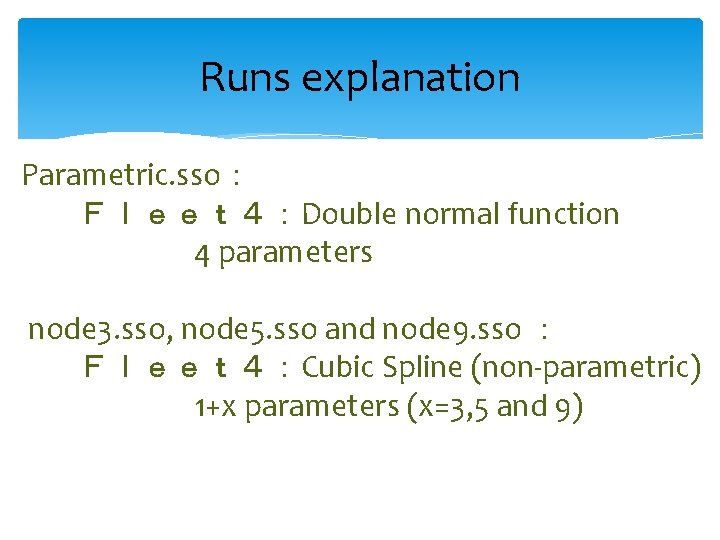
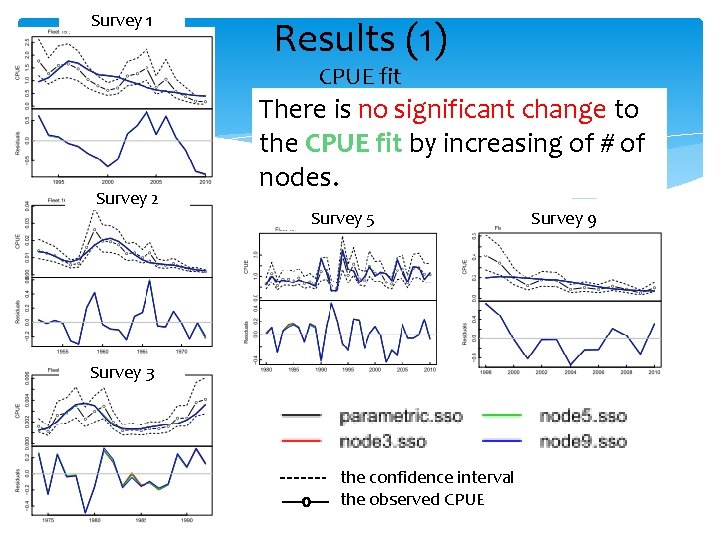
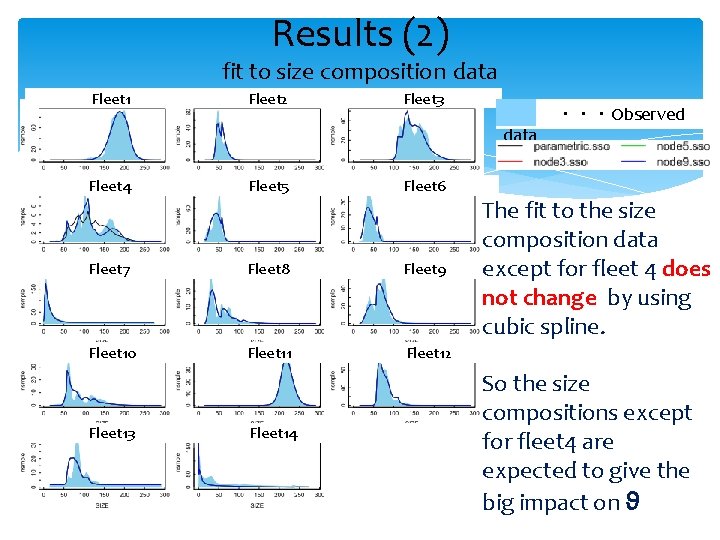
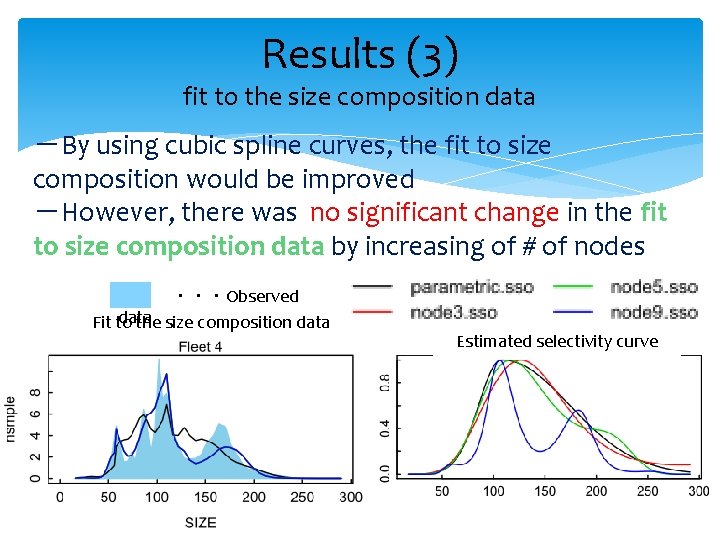
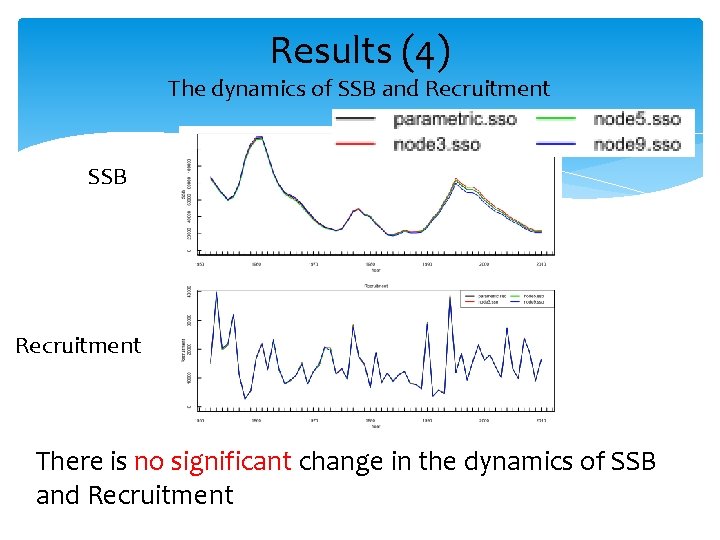
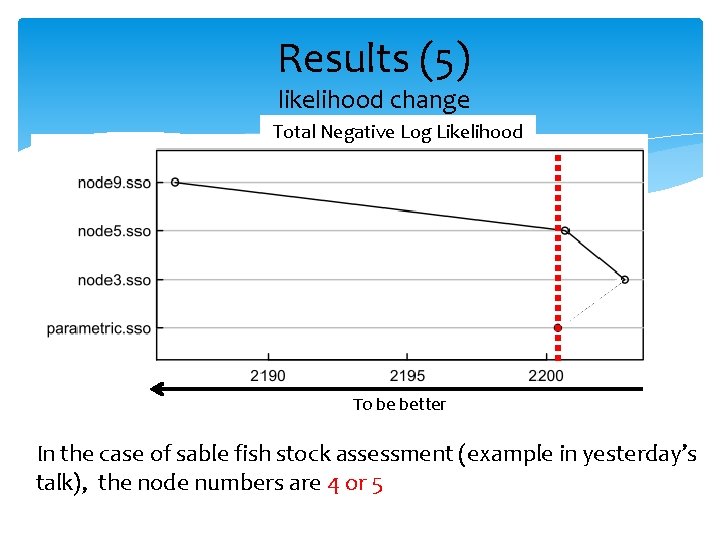
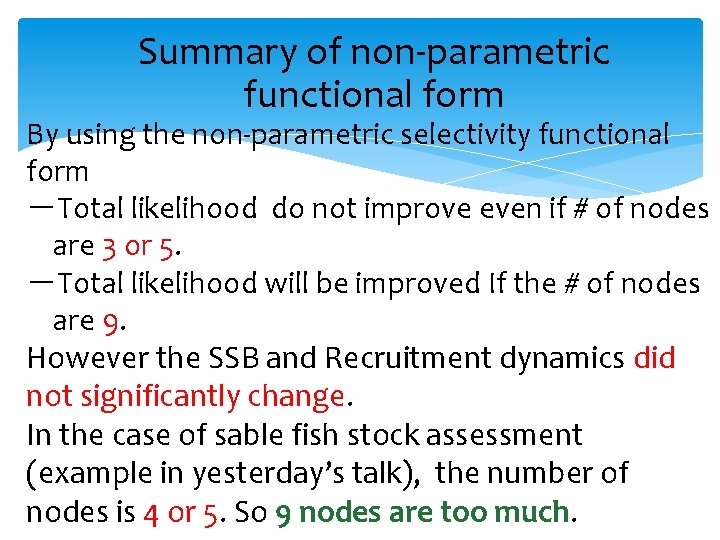
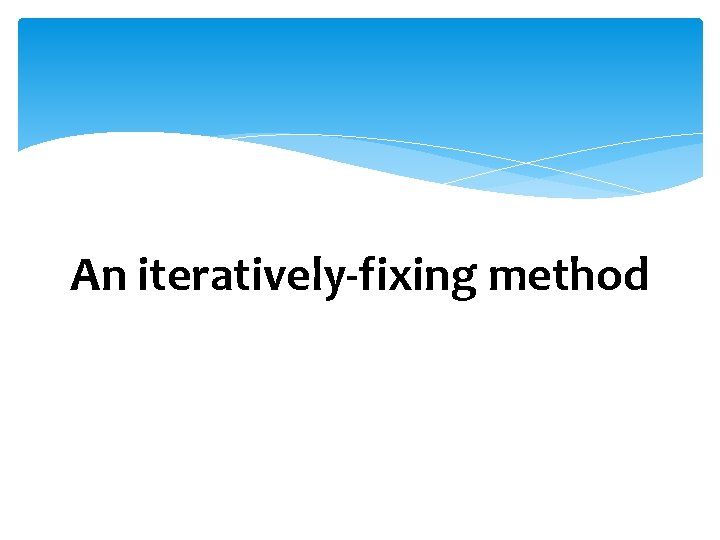
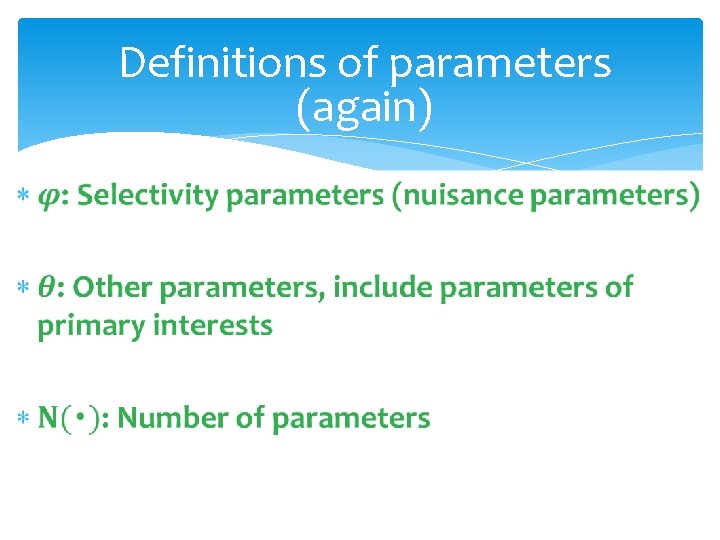
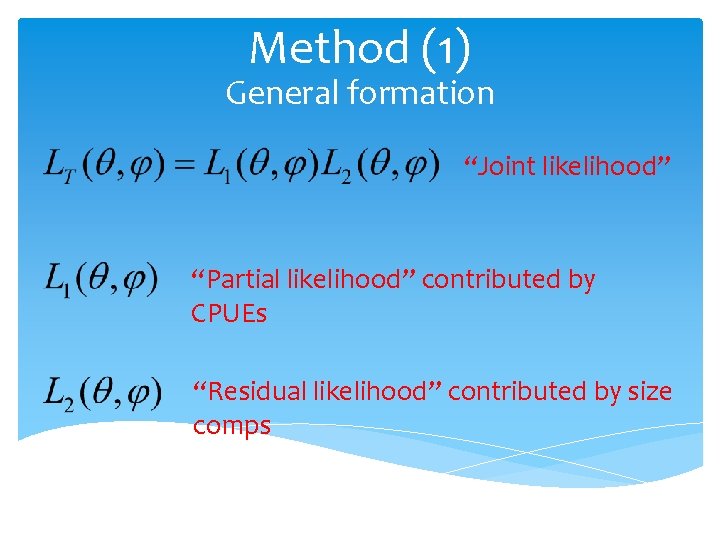
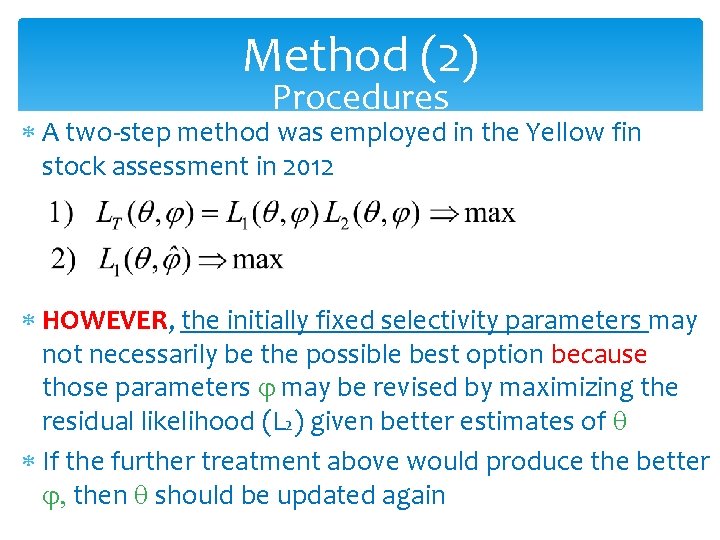
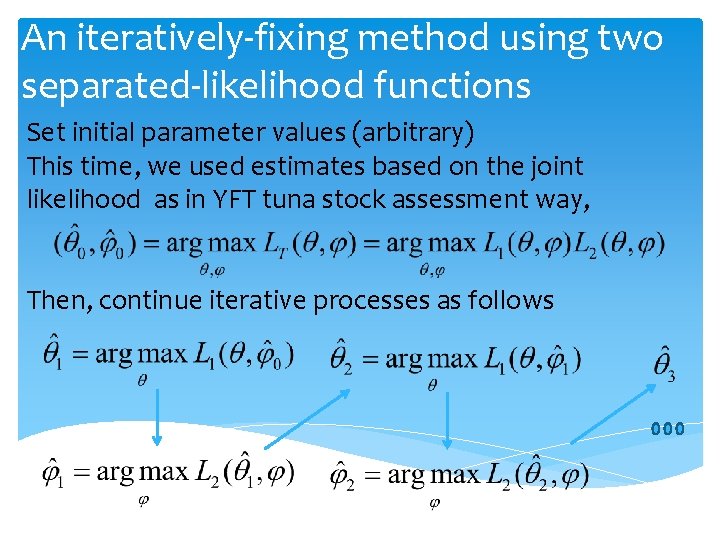
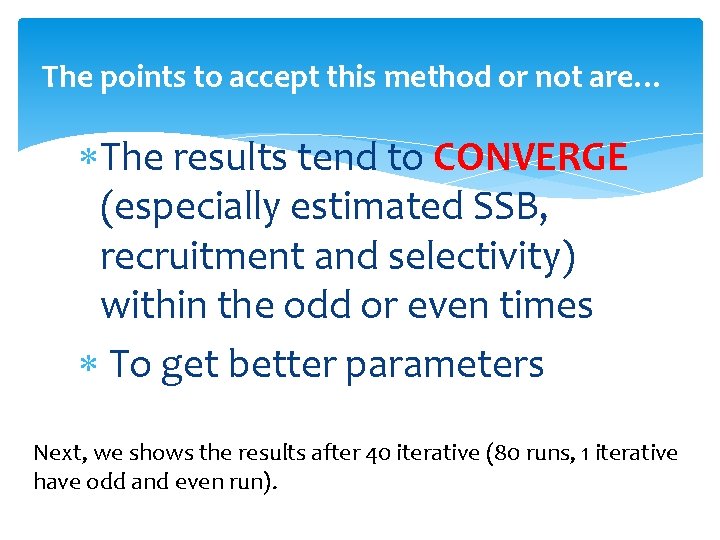
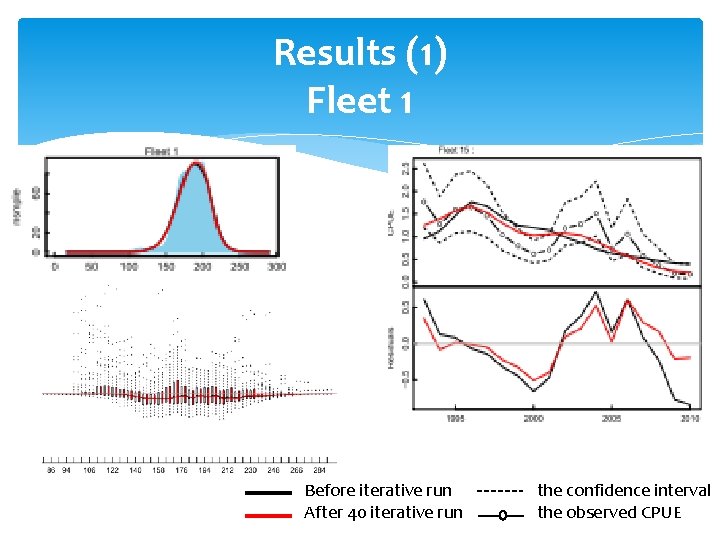
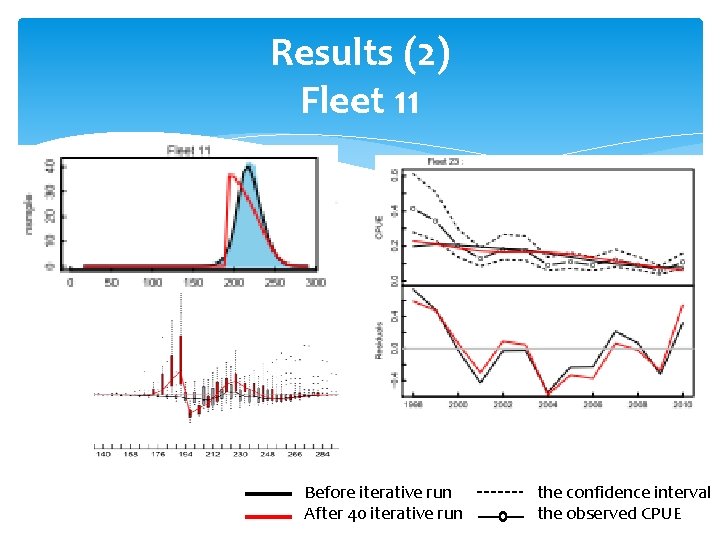
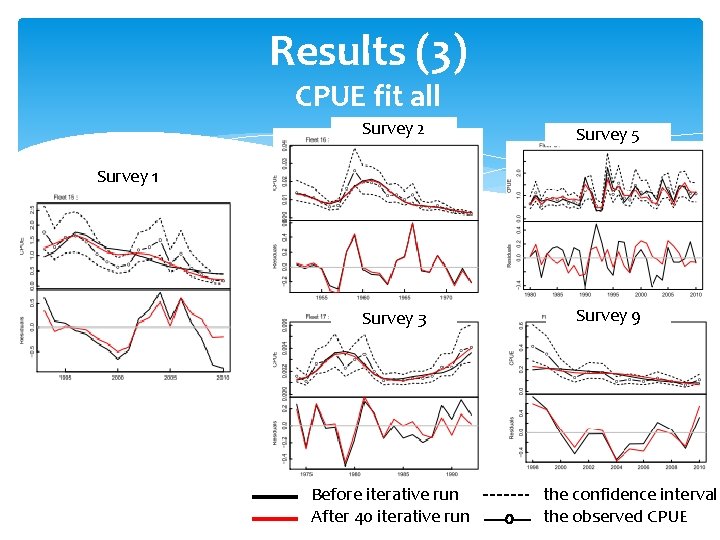
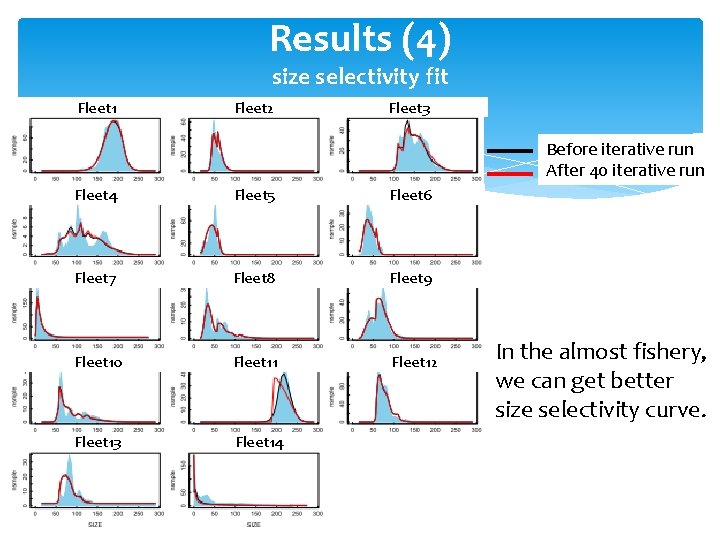
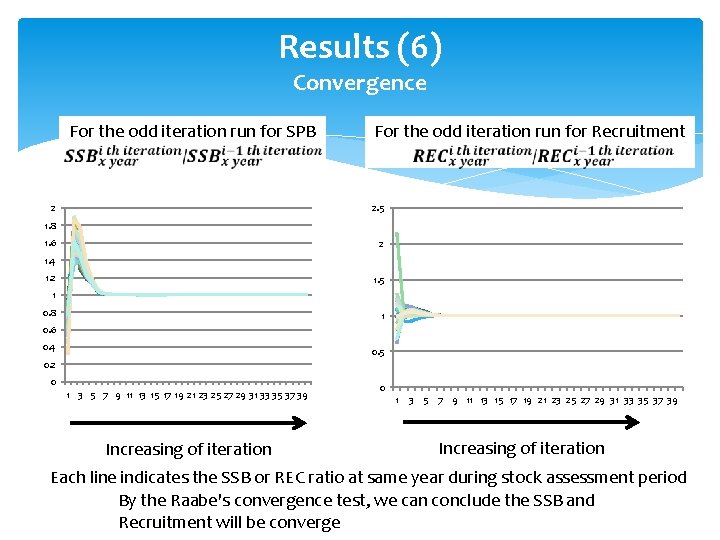
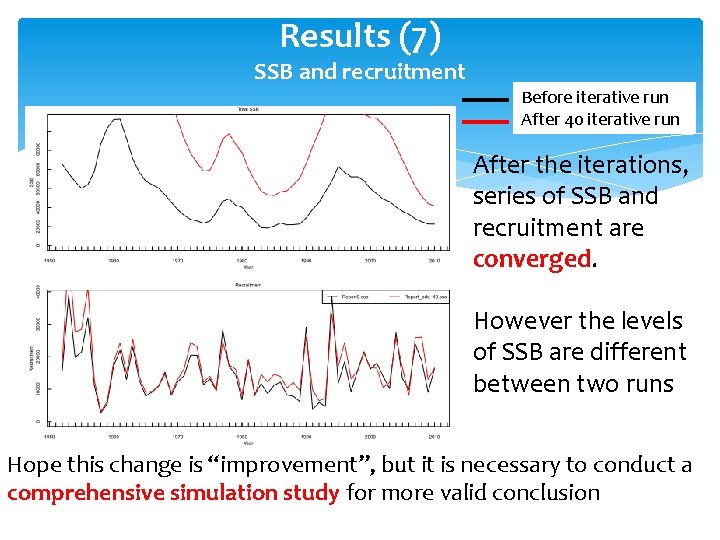
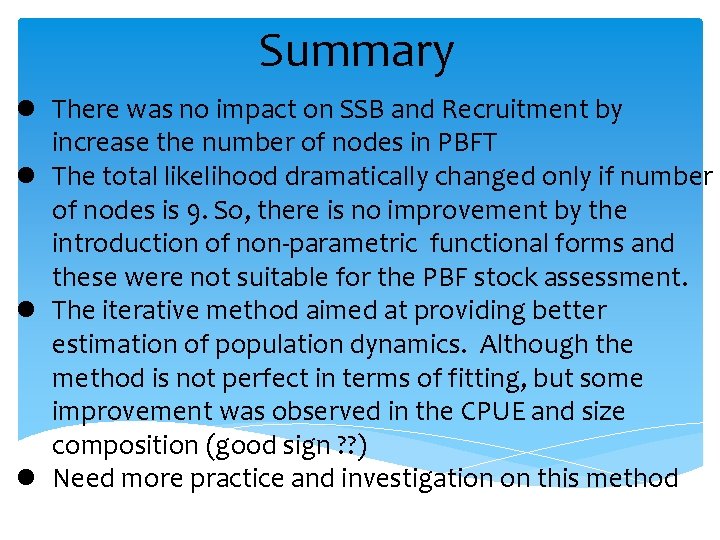
- Slides: 29
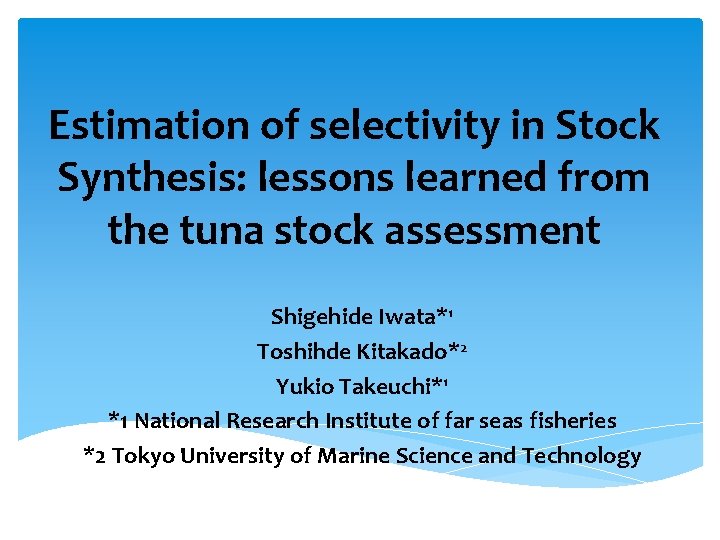
Estimation of selectivity in Stock Synthesis: lessons learned from the tuna stock assessment Shigehide Iwata*1 Toshihde Kitakado*2 Yukio Takeuchi*1 *1 National Research Institute of far seas fisheries *2 Tokyo University of Marine Science and Technology
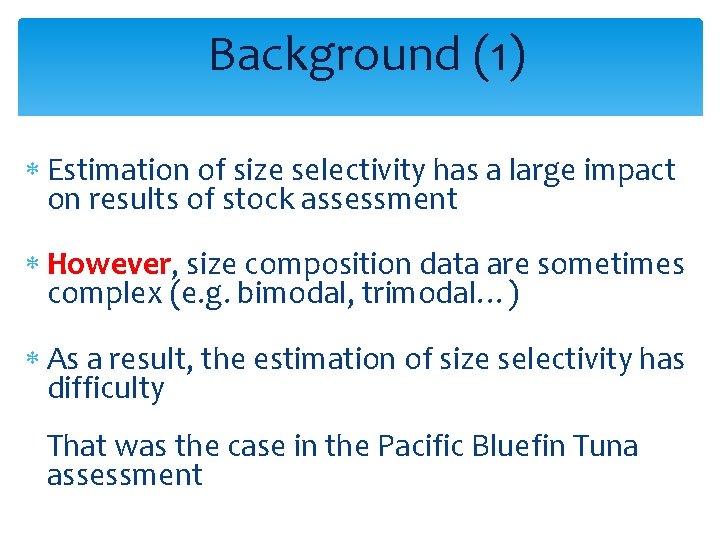
Background (1) Estimation of size selectivity has a large impact on results of stock assessment However, size composition data are sometimes complex (e. g. bimodal, trimodal…) As a result, the estimation of size selectivity has difficulty That was the case in the Pacific Bluefin Tuna assessment
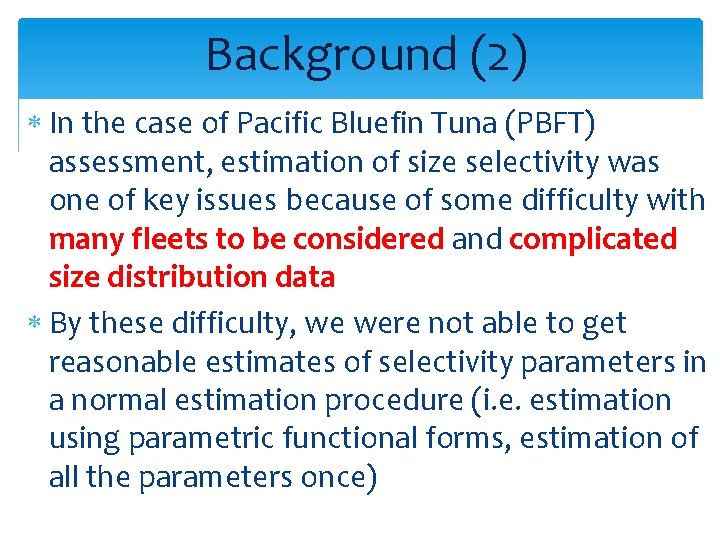
Background (2) In the case of Pacific Bluefin Tuna (PBFT) assessment, estimation of size selectivity was one of key issues because of some difficulty with many fleets to be considered and complicated size distribution data By these difficulty, we were not able to get reasonable estimates of selectivity parameters in a normal estimation procedure (i. e. estimation using parametric functional forms, estimation of all the parameters once)
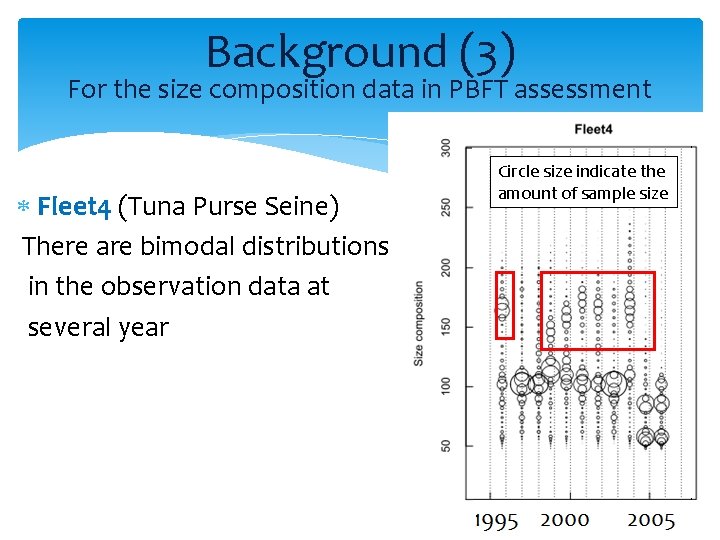
Background (3) For the size composition data in PBFT assessment Fleet 4 (Tuna Purse Seine) There are bimodal distributions in the observation data at several year Circle size indicate the amount of sample size
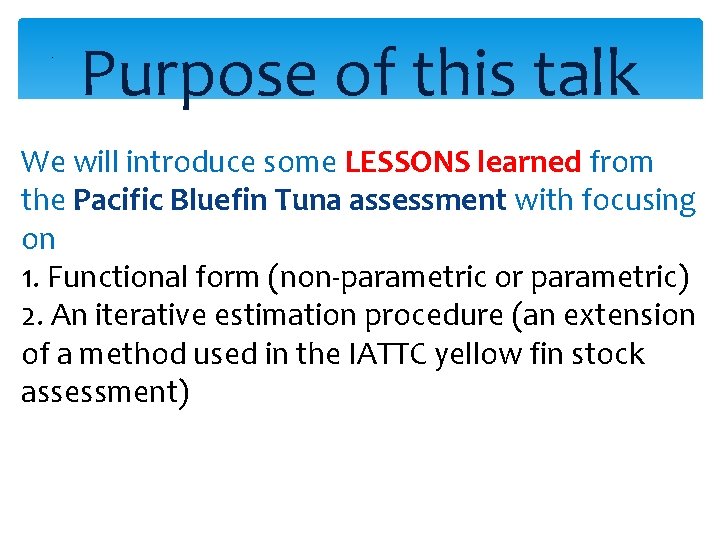
. Purpose of this talk We will introduce some LESSONS learned from the Pacific Bluefin Tuna assessment with focusing on 1. Functional form (non-parametric or parametric) 2. An iterative estimation procedure (an extension of a method used in the IATTC yellow fin stock assessment)
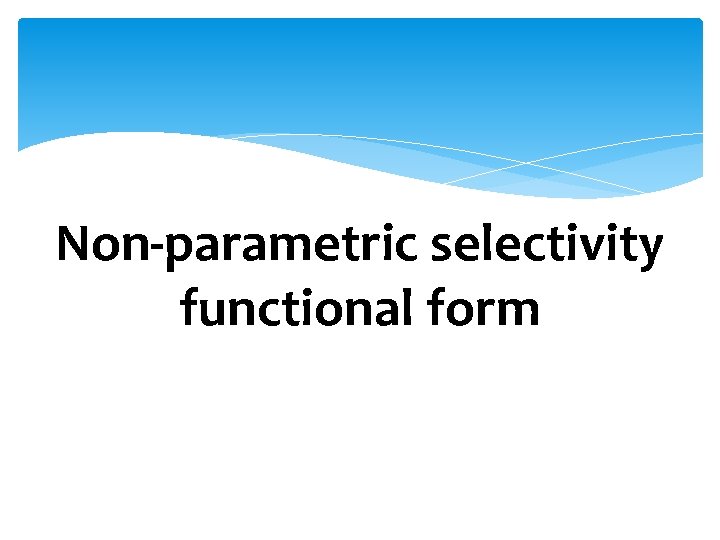
Non-parametric selectivity functional form
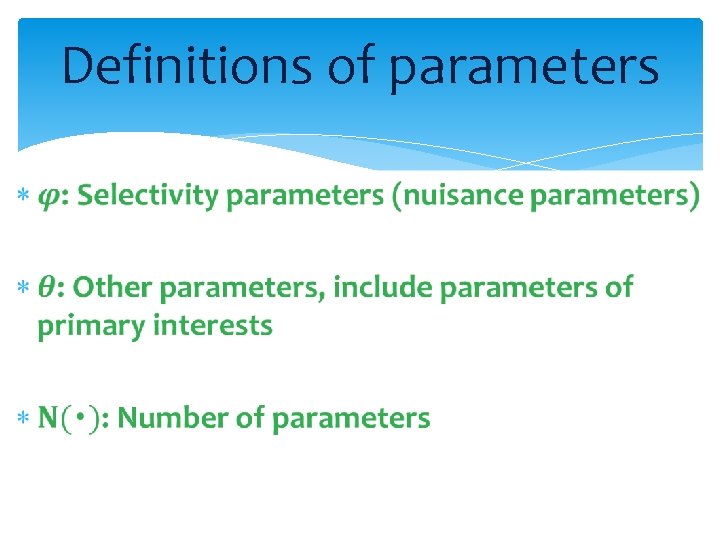
Definitions of parameters
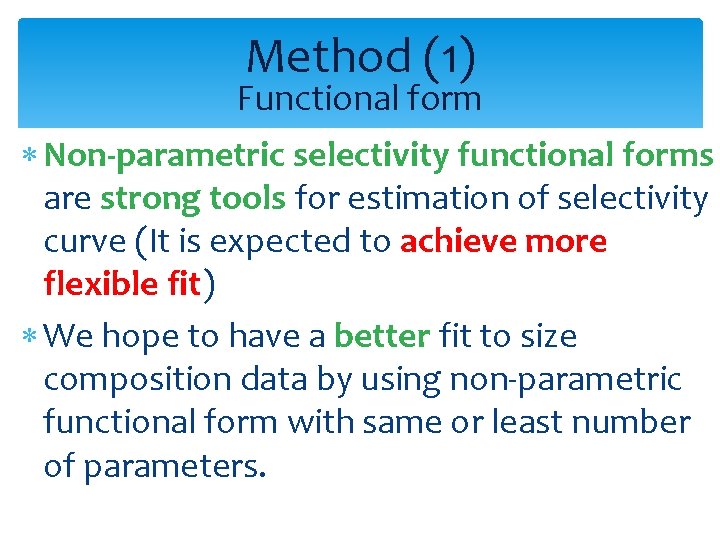
Method (1) Functional form Non-parametric selectivity functional forms are strong tools for estimation of selectivity curve (It is expected to achieve more flexible fit) We hope to have a better fit to size composition data by using non-parametric functional form with same or least number of parameters.
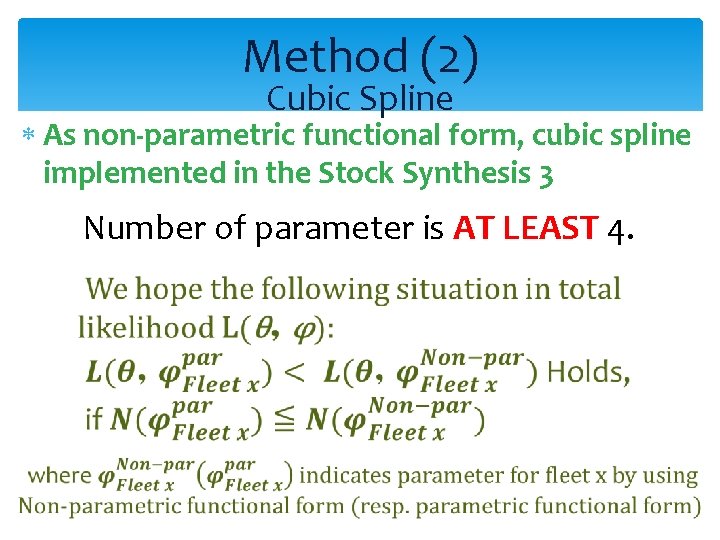
Method (2) Cubic Spline As non-parametric functional form, cubic spline implemented in the Stock Synthesis 3 Number of parameter is AT LEAST 4.
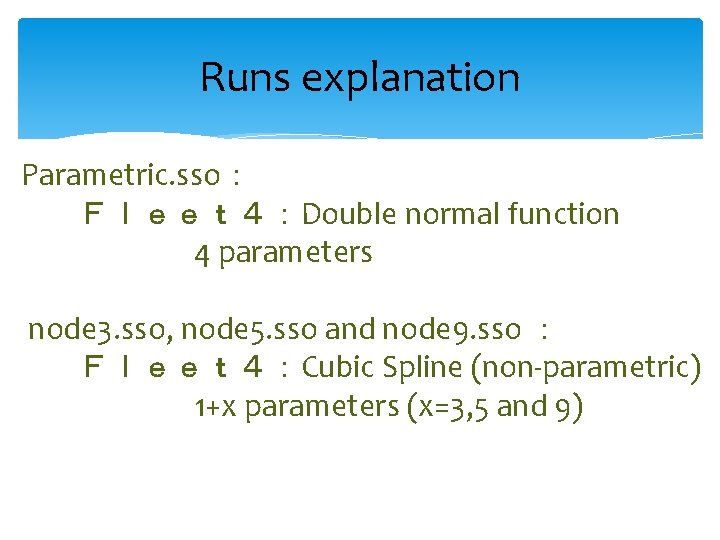
Runs explanation Parametric. sso: Fleet4:Double normal function 4 parameters node 3. sso, node 5. sso and node 9. sso : Fleet4:Cubic Spline (non-parametric) 1+x parameters (x=3, 5 and 9)
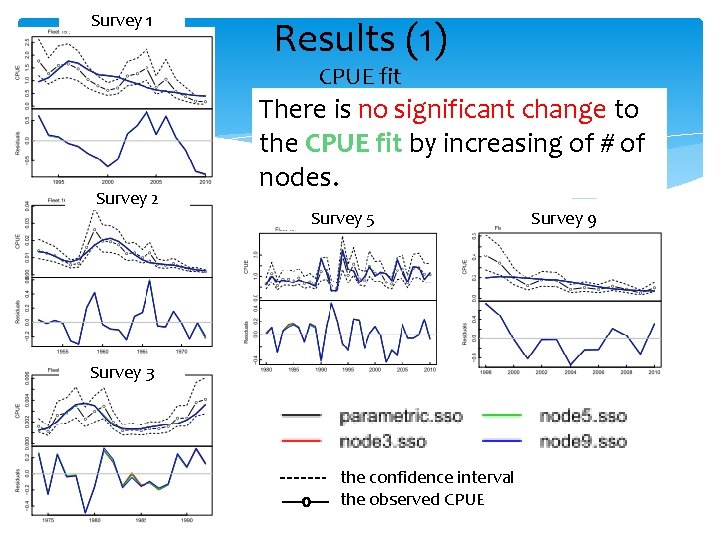
Survey 1 Results (1) CPUE fit Survey 2 There is no significant change to the CPUE fit by increasing of # of nodes. Survey 5 Survey 3 the confidence interval the observed CPUE Survey 9
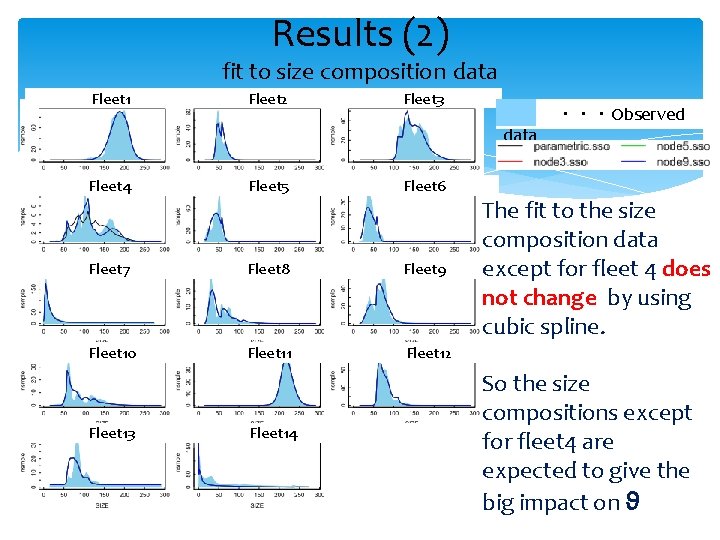
Results (2) fit to size composition data Fleet 1 Fleet 2 Fleet 3 Fleet 4 Fleet 5 Fleet 6 Fleet 7 Fleet 8 Fleet 9 Fleet 10 Fleet 11 Fleet 12 Fleet 13 Fleet 14 ・・・Observed data The fit to the size composition data except for fleet 4 does not change by using cubic spline. So the size compositions except for fleet 4 are expected to give the big impact on θ
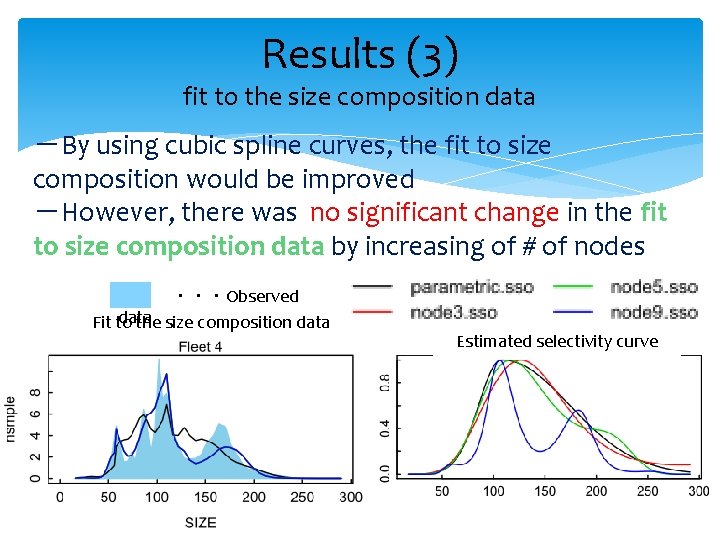
Results (3) fit to the size composition data -By using cubic spline curves, the fit to size composition would be improved -However, there was no significant change in the fit to size composition data by increasing of # of nodes ・・・Observed data Fit to the size composition data Estimated selectivity curve
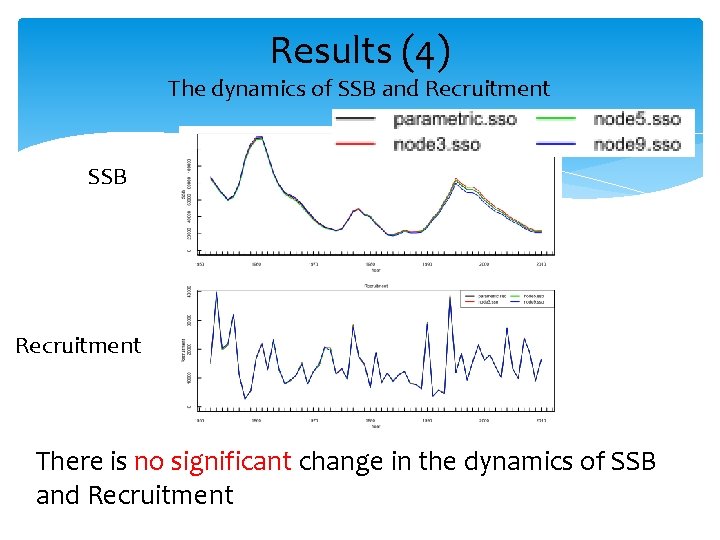
Results (4) The dynamics of SSB and Recruitment SSB Recruitment There is no significant change in the dynamics of SSB and Recruitment
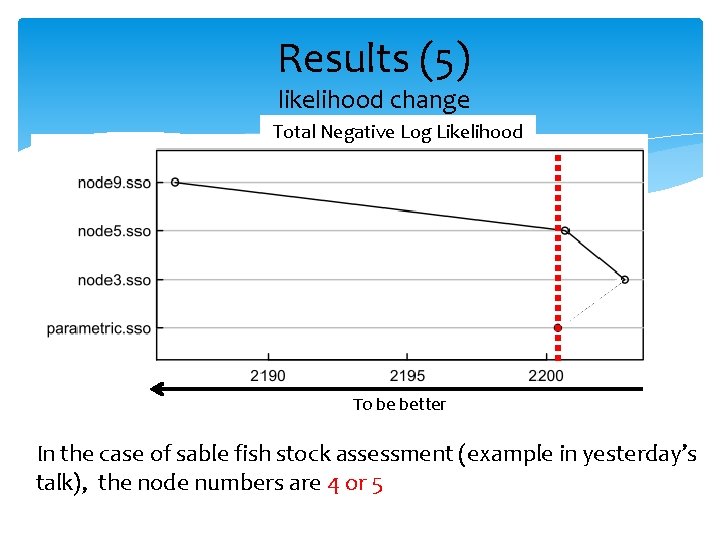
Results (5) likelihood change Total Negative Log Likelihood To be better In the case of sable fish stock assessment (example in yesterday’s talk), the node numbers are 4 or 5
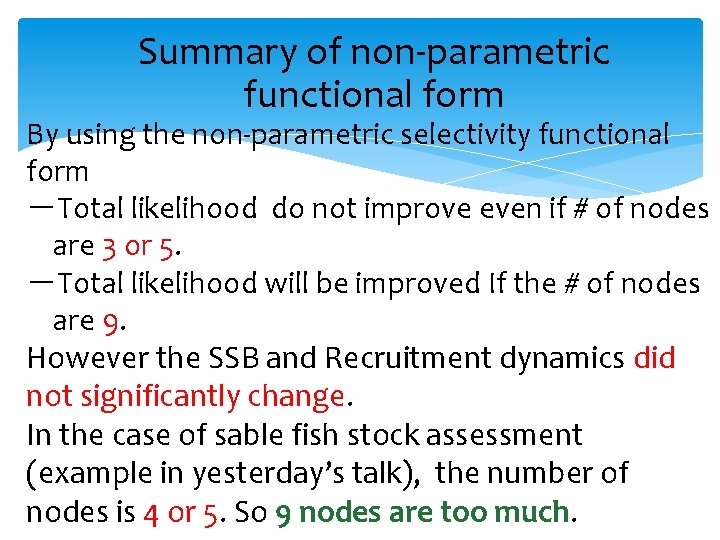
Summary of non-parametric functional form By using the non-parametric selectivity functional form -Total likelihood do not improve even if # of nodes are 3 or 5. -Total likelihood will be improved If the # of nodes are 9. However the SSB and Recruitment dynamics did not significantly change. In the case of sable fish stock assessment (example in yesterday’s talk), the number of nodes is 4 or 5. So 9 nodes are too much.
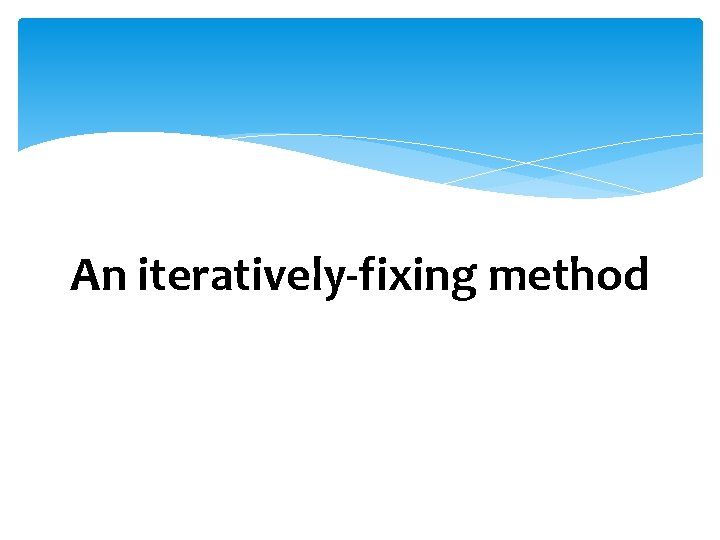
An iteratively-fixing method
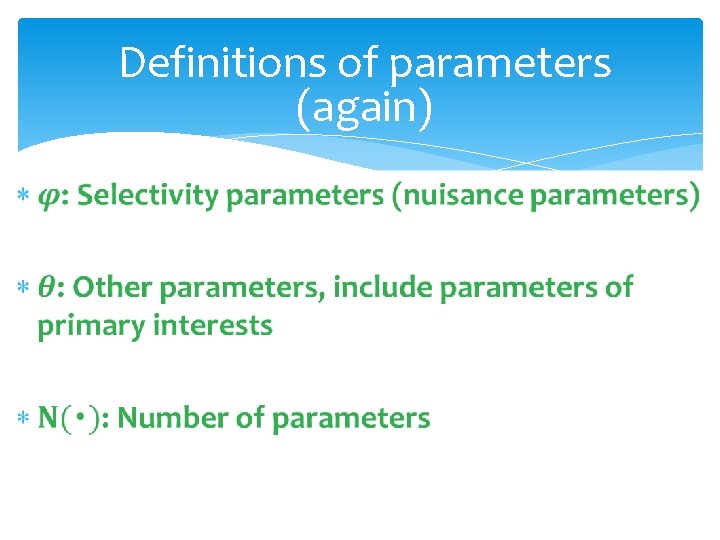
Definitions of parameters (again)
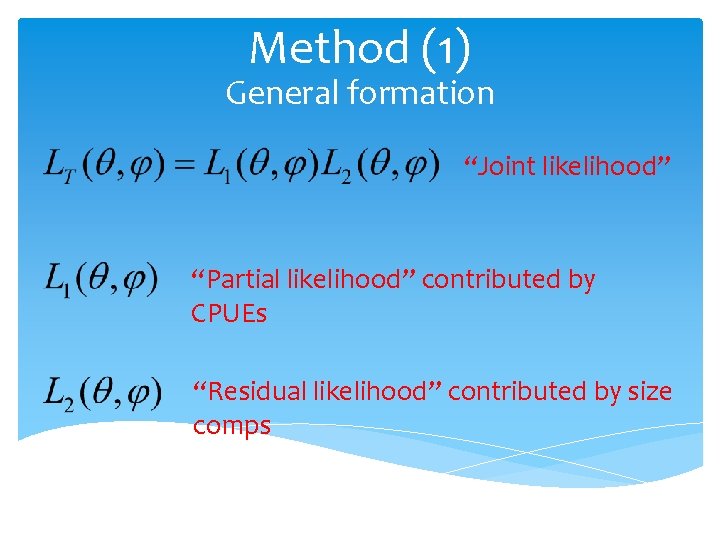
Method (1) General formation “Joint likelihood” “Partial likelihood” contributed by CPUEs “Residual likelihood” contributed by size comps
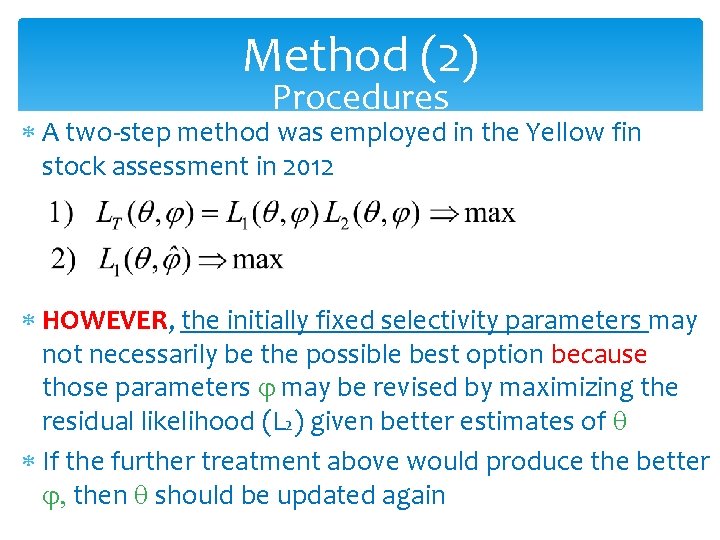
Method (2) Procedures A two-step method was employed in the Yellow fin stock assessment in 2012 HOWEVER, the initially fixed selectivity parameters may not necessarily be the possible best option because those parameters j may be revised by maximizing the residual likelihood (L 2) given better estimates of q If the further treatment above would produce the better j, then q should be updated again
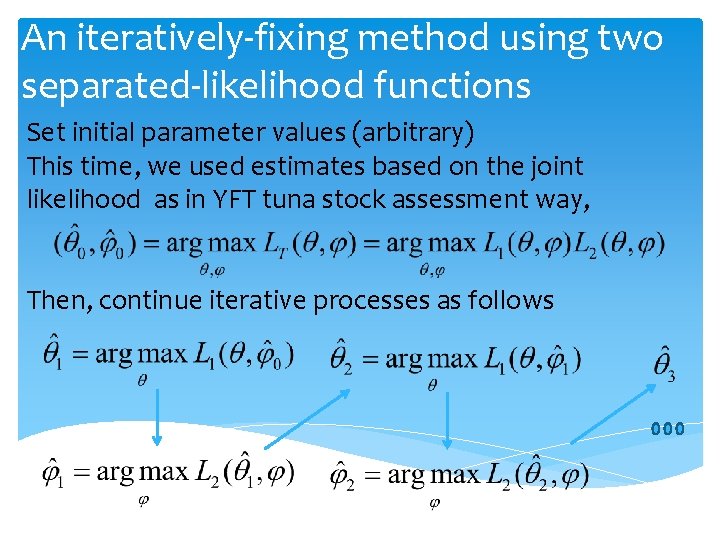
An iteratively-fixing method using two separated-likelihood functions Set initial parameter values (arbitrary) This time, we used estimates based on the joint likelihood as in YFT tuna stock assessment way, Then, continue iterative processes as follows
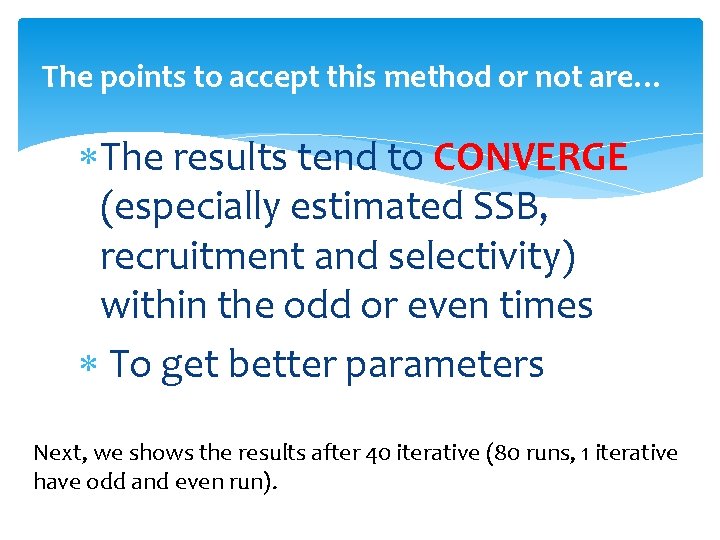
The points to accept this method or not are… The results tend to CONVERGE (especially estimated SSB, recruitment and selectivity) within the odd or even times To get better parameters Next, we shows the results after 40 iterative (80 runs, 1 iterative have odd and even run).
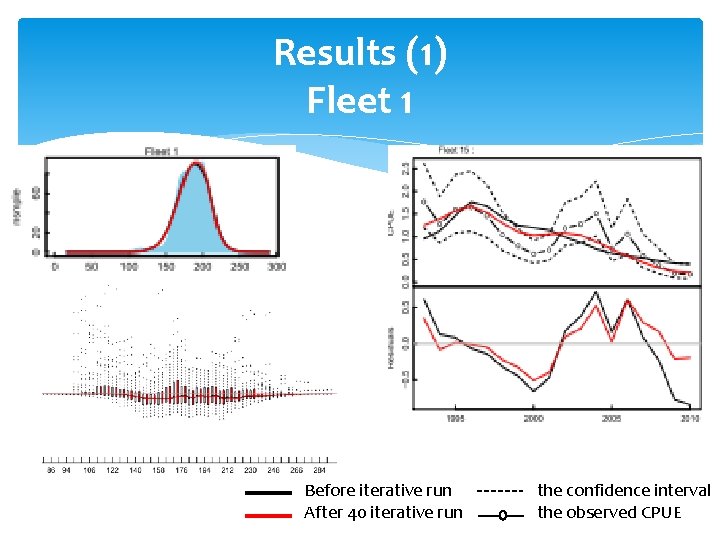
Results (1) Fleet 1 Before iterative run After 40 iterative run the confidence interval the observed CPUE
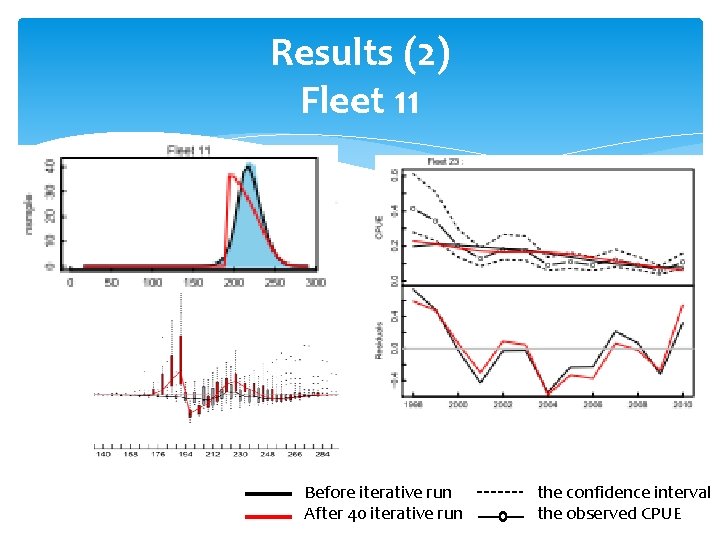
Results (2) Fleet 11 Before iterative run After 40 iterative run the confidence interval the observed CPUE
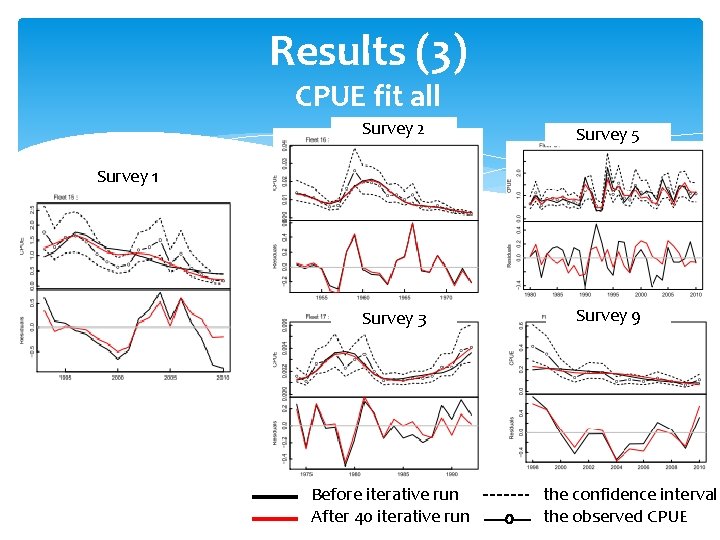
Results (3) CPUE fit all Survey 2 Survey 5 Survey 3 Survey 9 Survey 1 Before iterative run After 40 iterative run the confidence interval the observed CPUE
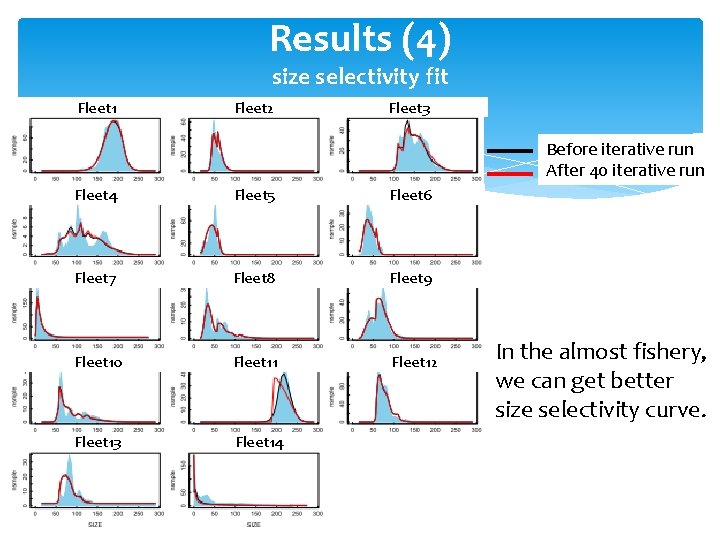
Results (4) size selectivity fit Fleet 1 Fleet 2 Fleet 3 Before iterative run After 40 iterative run Fleet 4 Fleet 5 Fleet 6 Fleet 7 Fleet 8 Fleet 9 Fleet 10 Fleet 11 Fleet 12 Fleet 13 Fleet 14 In the almost fishery, we can get better size selectivity curve.
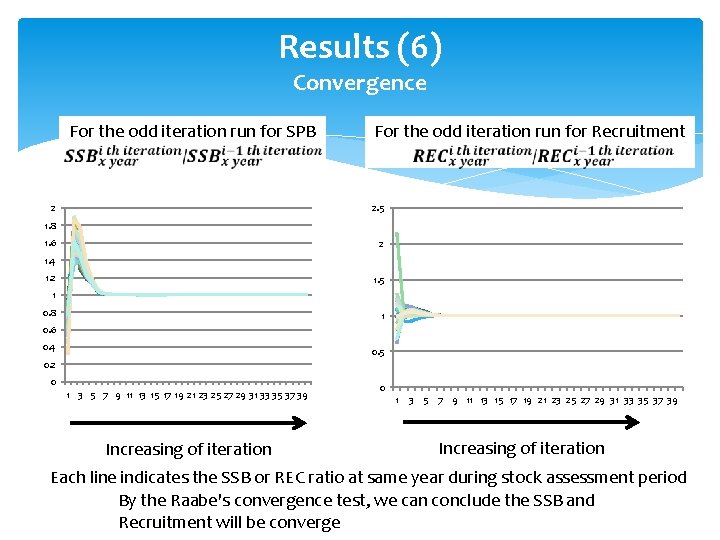
Results (6) Convergence For the odd iteration run for SPB 2 For the odd iteration run for Recruitment 2. 5 1. 8 1. 6 2 1. 4 1. 2 1. 5 1 0. 8 1 0. 6 0. 4 0. 5 0. 2 0 1 3 5 7 9 11 13 15 17 19 21 23 25 27 29 31 33 35 37 39 Increasing of iteration Each line indicates the SSB or REC ratio at same year during stock assessment period By the Raabe's convergence test, we can conclude the SSB and Recruitment will be converge
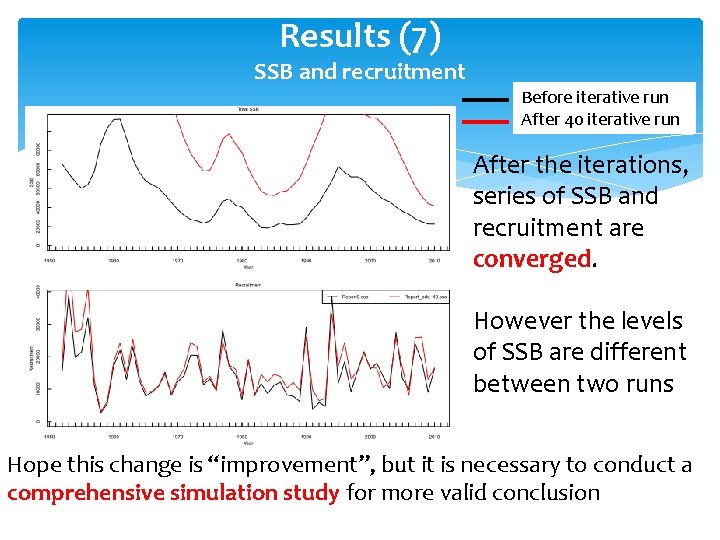
Results (7) SSB and recruitment Before iterative run After 40 iterative run After the iterations, series of SSB and recruitment are converged. However the levels of SSB are different between two runs Hope this change is “improvement”, but it is necessary to conduct a comprehensive simulation study for more valid conclusion
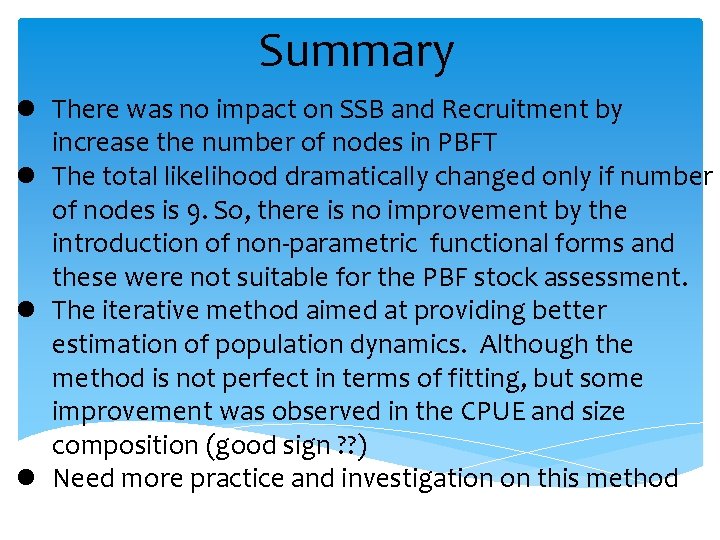
Summary l There was no impact on SSB and Recruitment by increase the number of nodes in PBFT l The total likelihood dramatically changed only if number of nodes is 9. So, there is no improvement by the introduction of non-parametric functional forms and these were not suitable for the PBF stock assessment. l The iterative method aimed at providing better estimation of population dynamics. Although the method is not perfect in terms of fitting, but some improvement was observed in the CPUE and size composition (good sign ? ? ) l Need more practice and investigation on this method
Tac lessons learned
Hydrotopia
2 chronicles 34 children's lesson
Lessons learned ground rules
Lessons not learned in blood
Change management lessons learned
Dps project title
Lessons learned repository
Lesson learned register
Character trait of an ant
Workday implementation lessons learned
Obadiah (1 kings)
Strawman report
Lessons learned suomeksi
Six sigma lessons learned
Lessons learned faa
Life lessons in life of pi
Lessons learned from mount carmel contest
Is josiah luke a dwarf
Lesson learned in typhoon yolanda
Nerc lessons learned
Hyatt regency collapse
Stock synthesis
Brown stock adalah
Characteristics of common stock and preferred stock
Stock final de marchandises
Long-term debt preferred stock and common stock
Character analysis worksheet for actors
Robert peck's stages of psychological development
Nsaid selectivity