Estimating Soil Moisture Profile Dynamics From NearSurface Soil
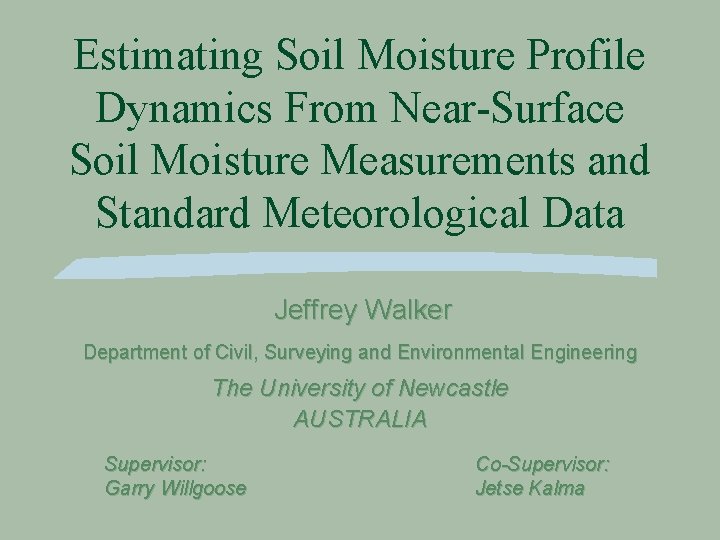
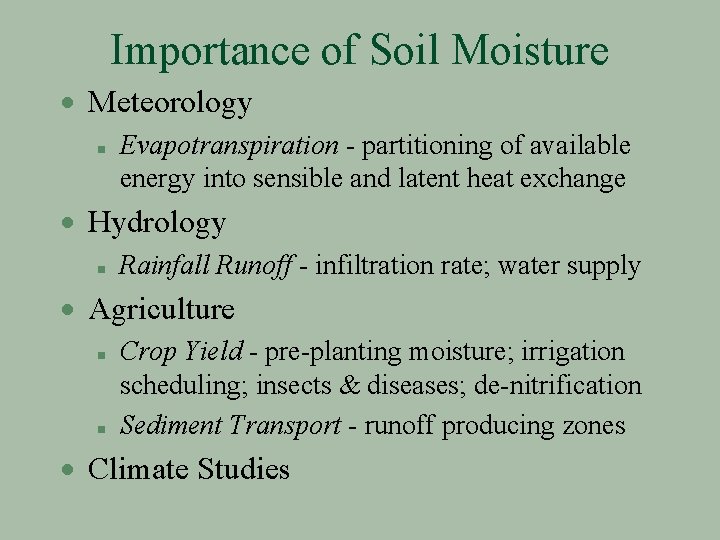
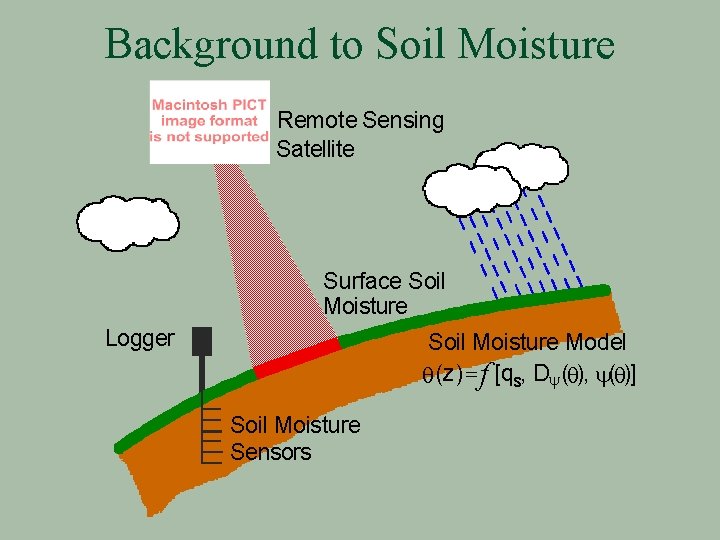
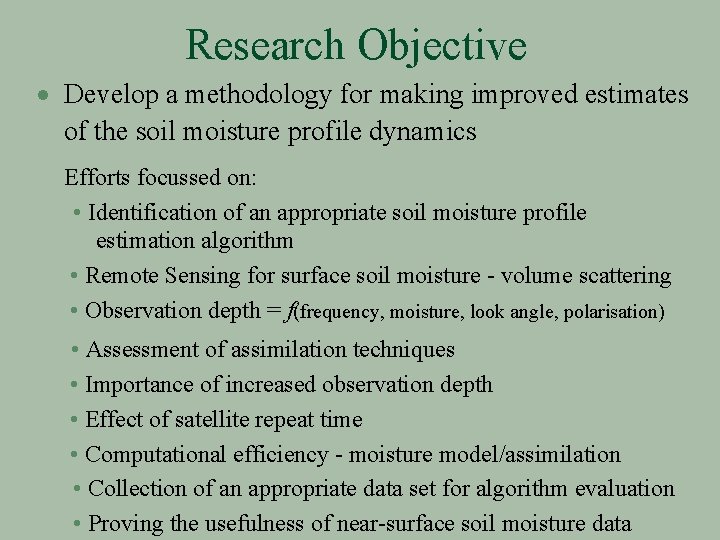
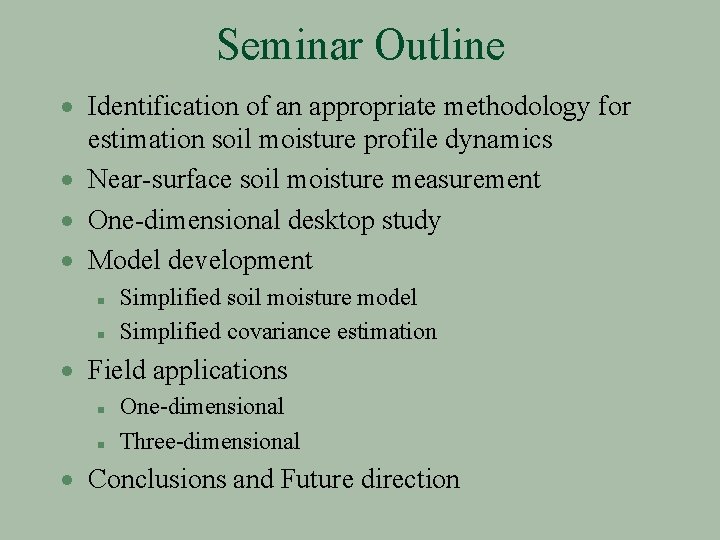
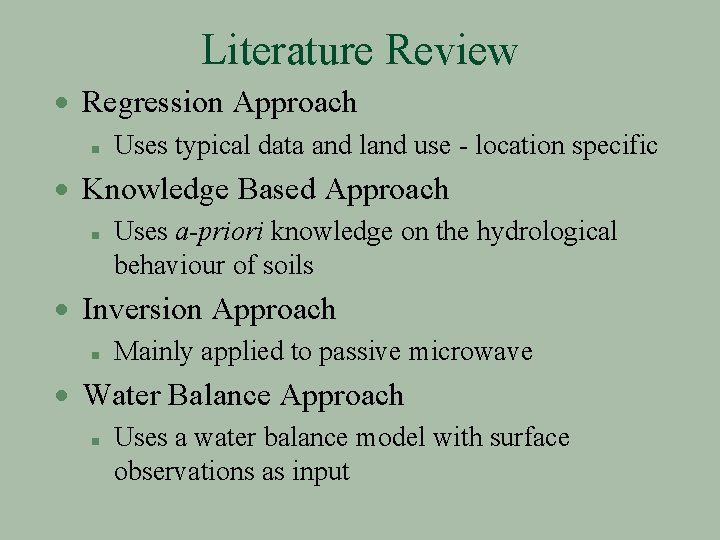
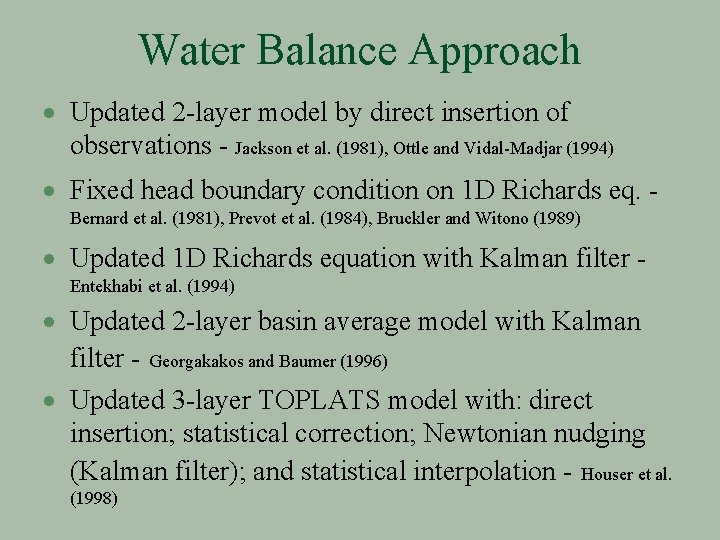
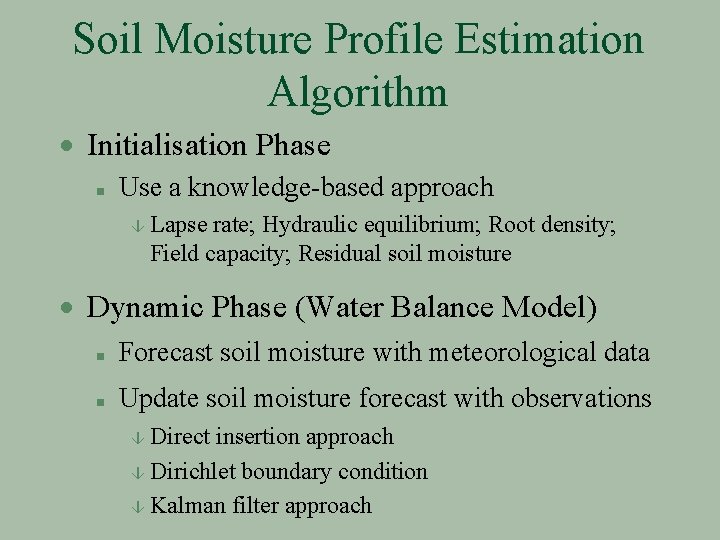
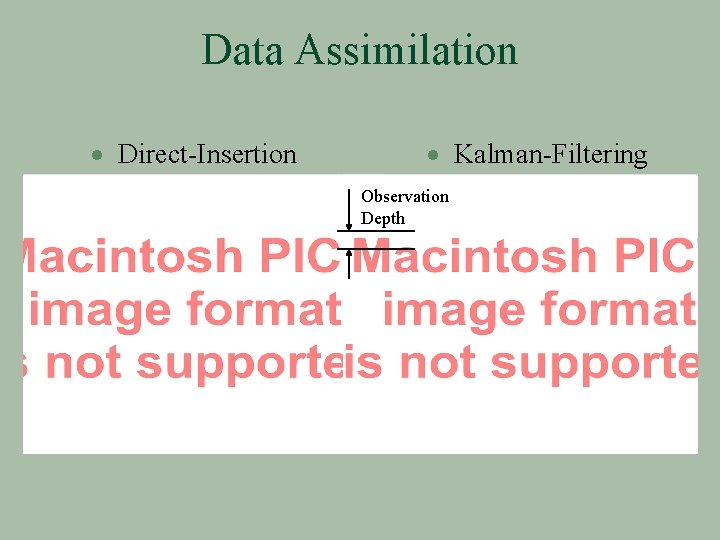
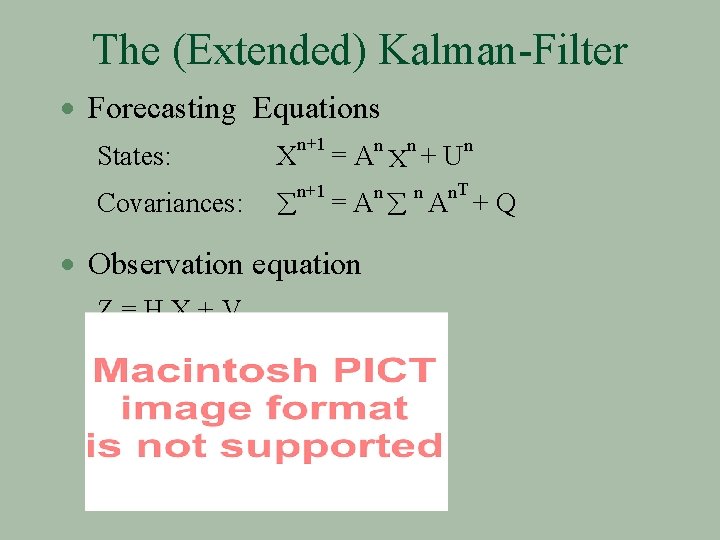
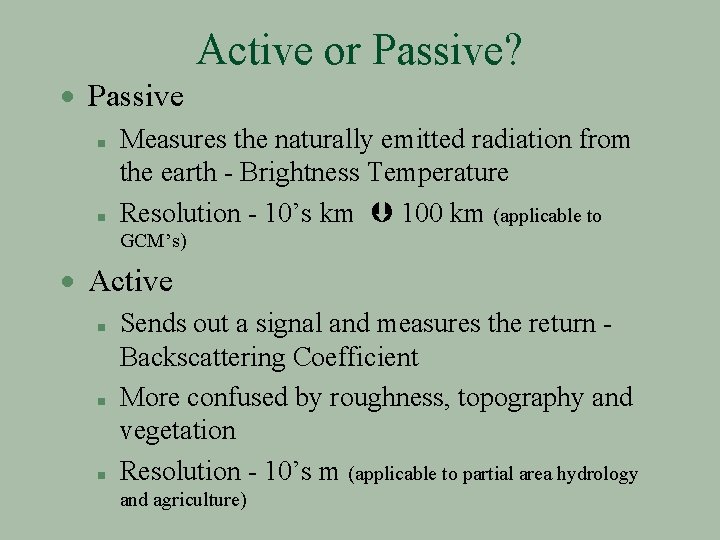
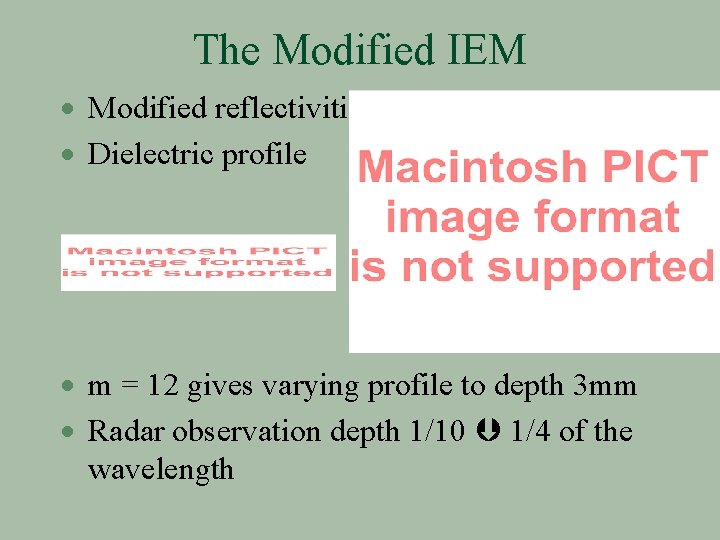
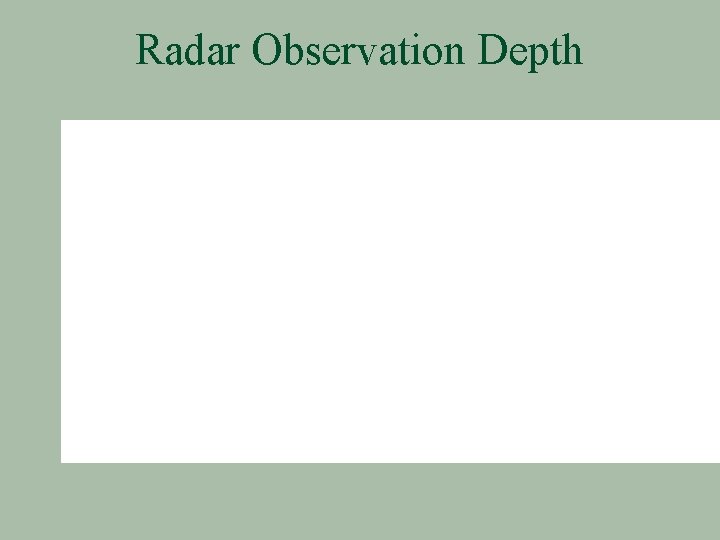
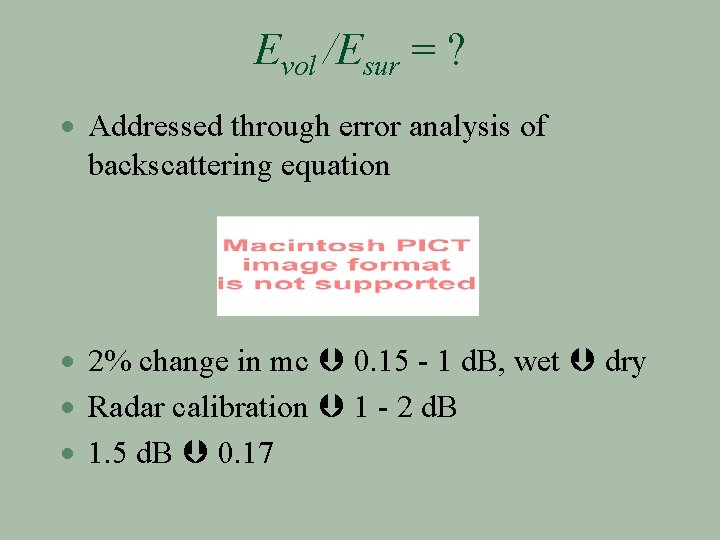
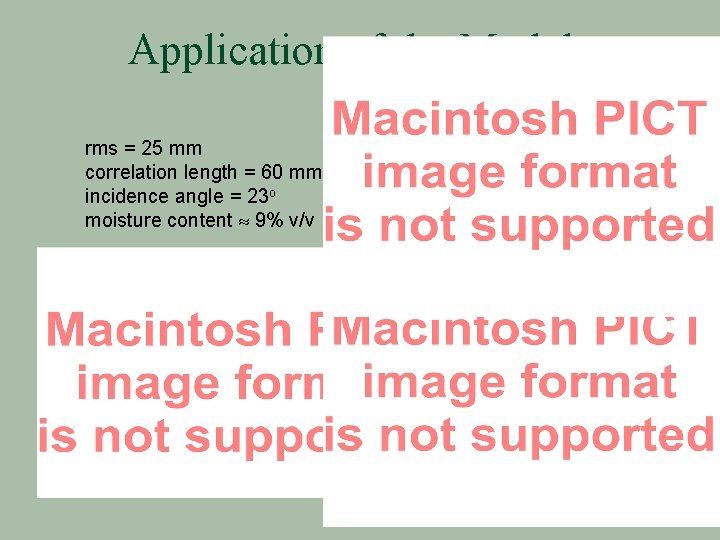
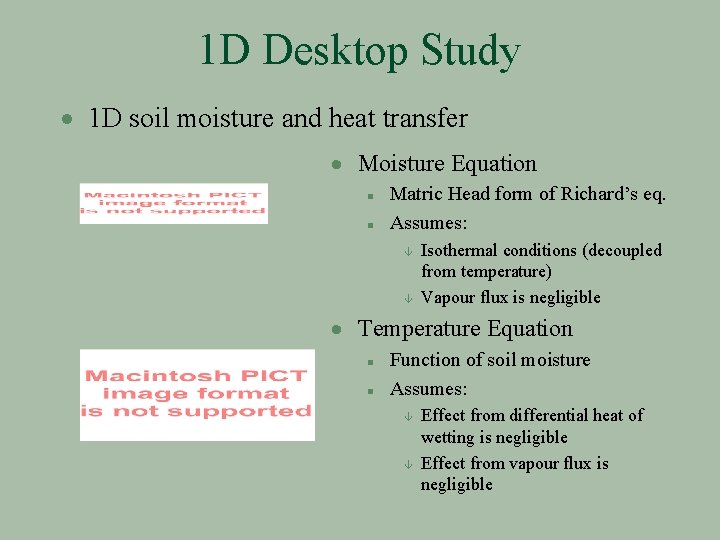
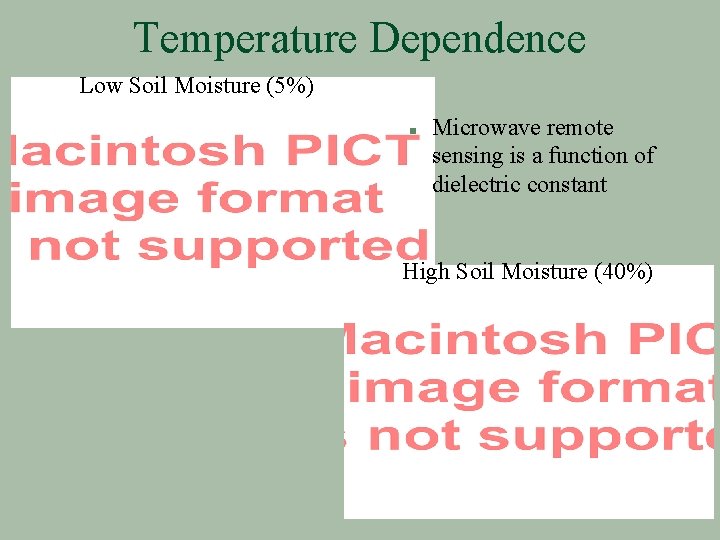
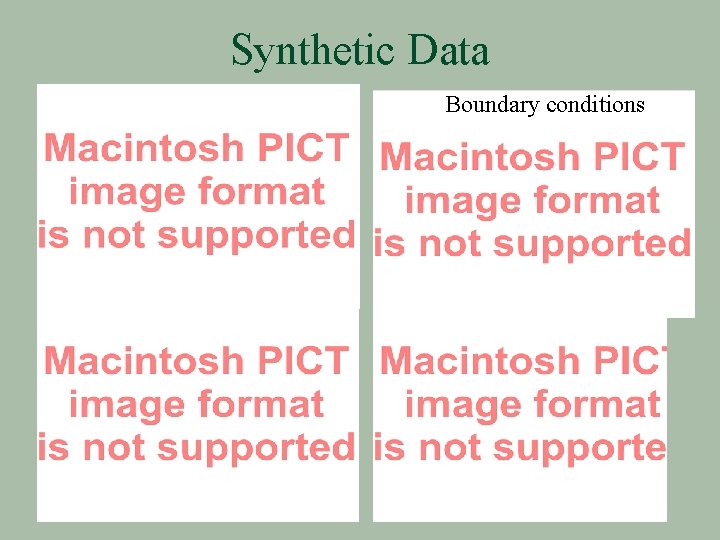
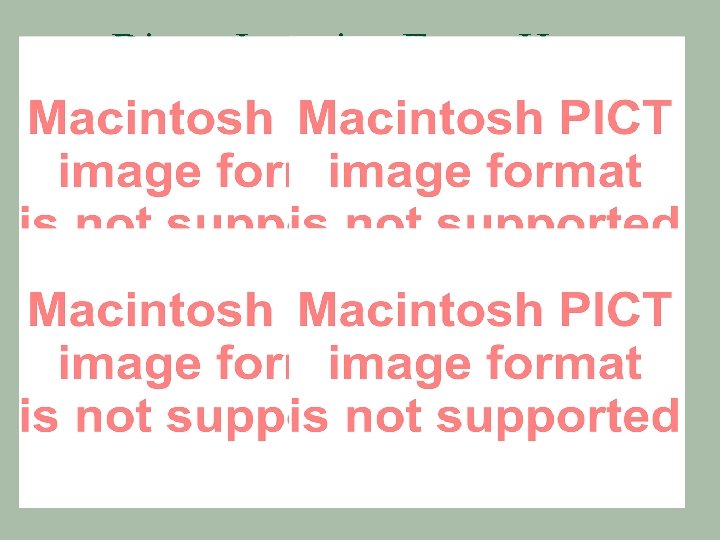
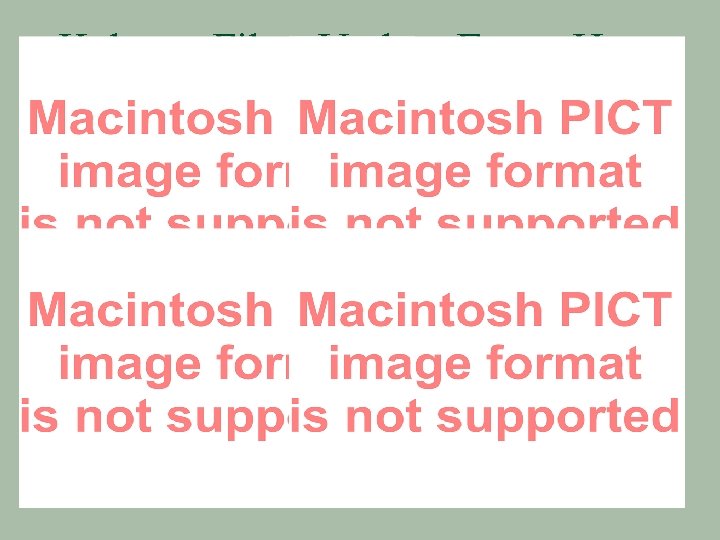
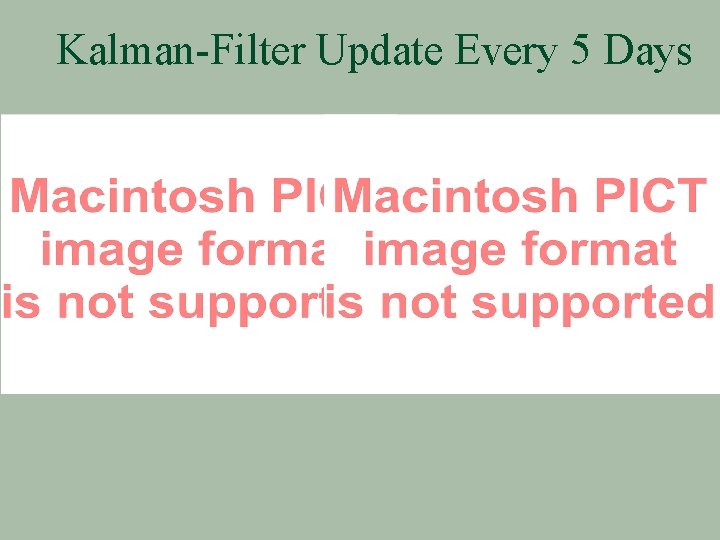
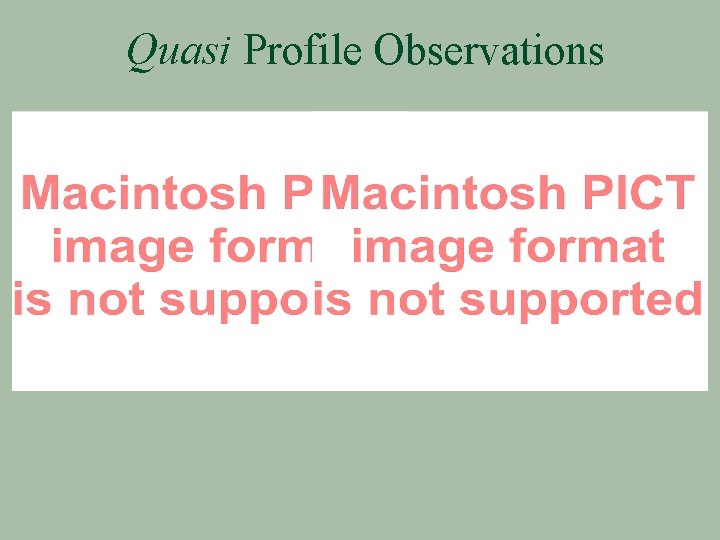
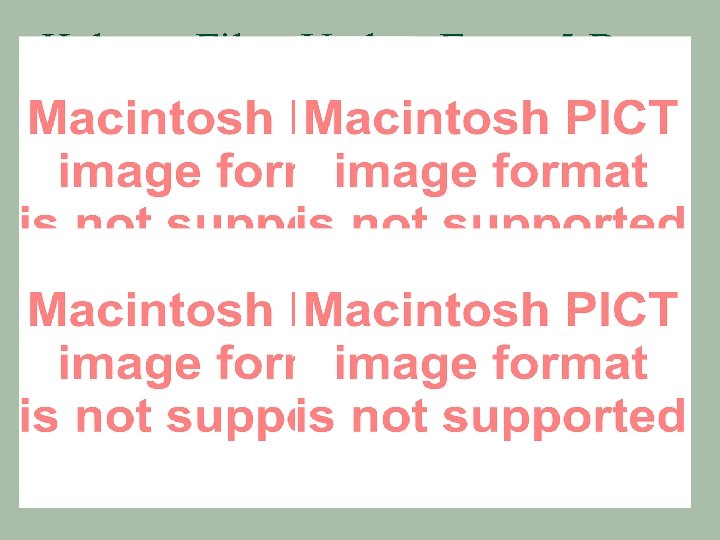
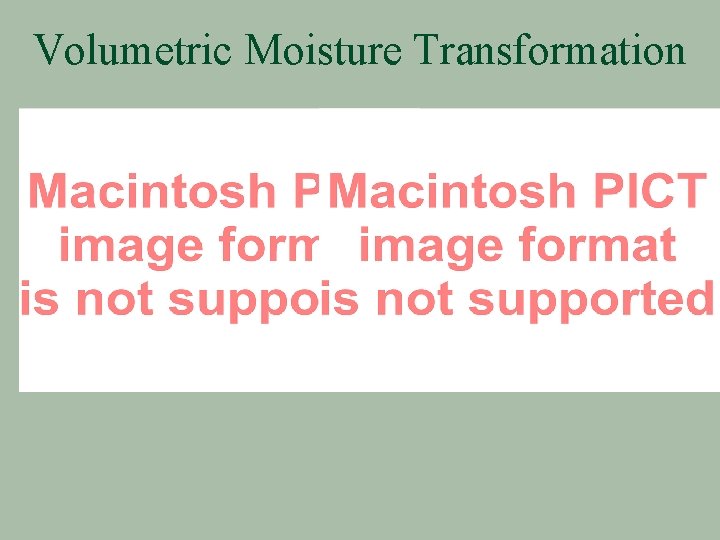
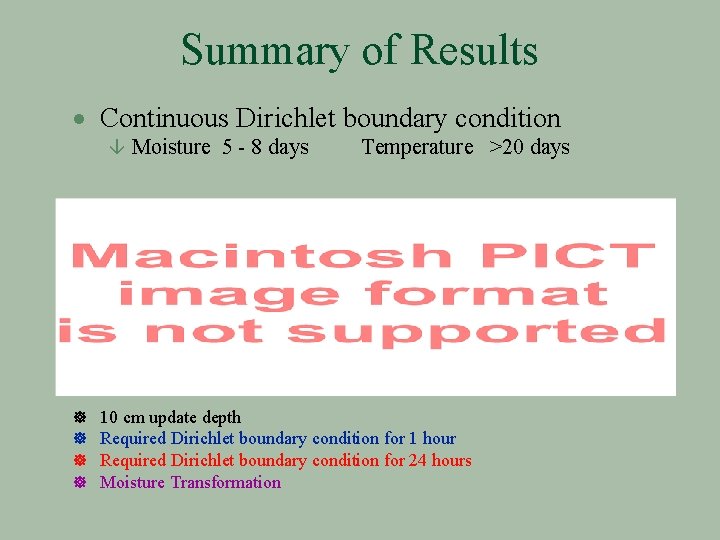
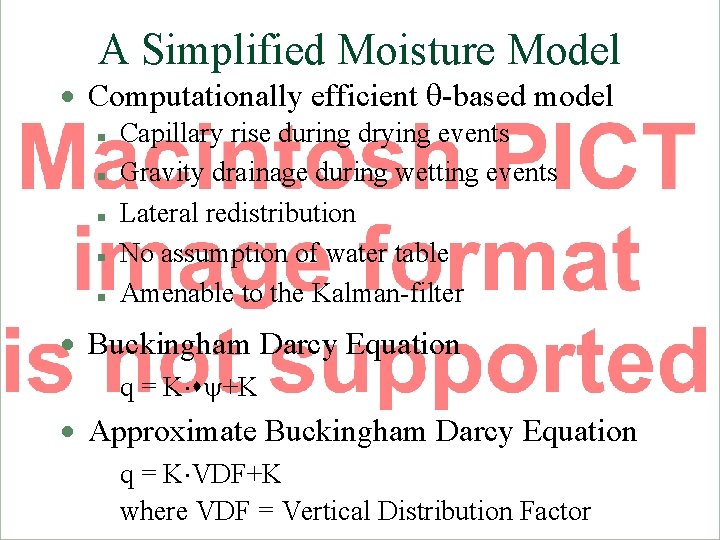
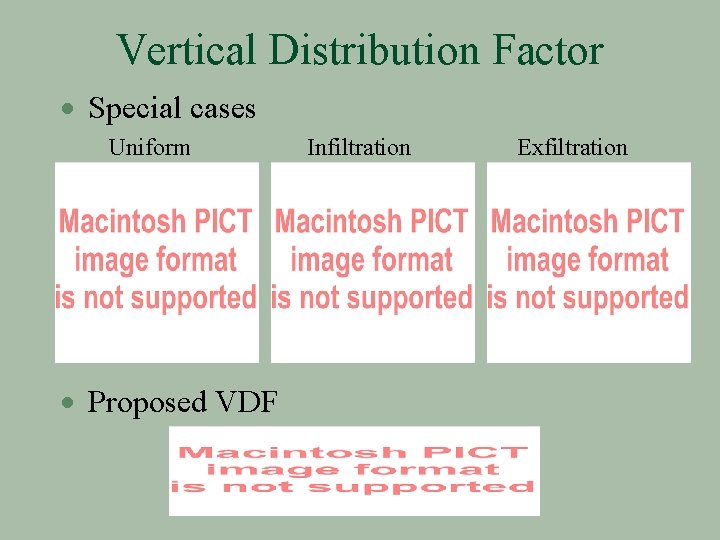
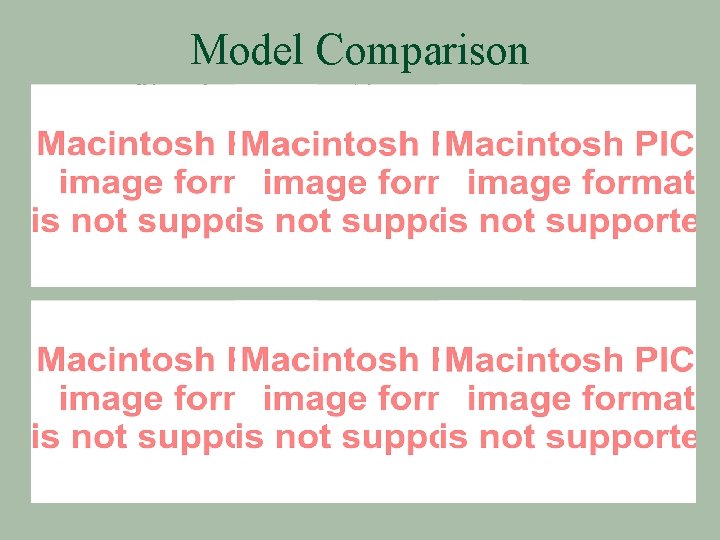
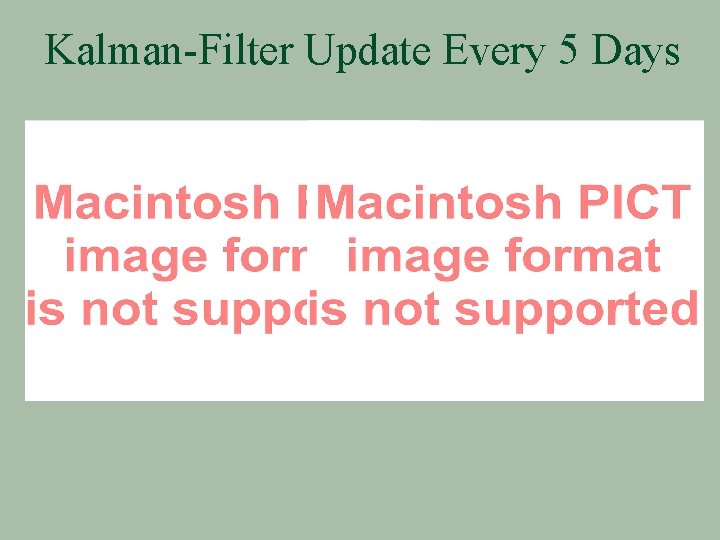
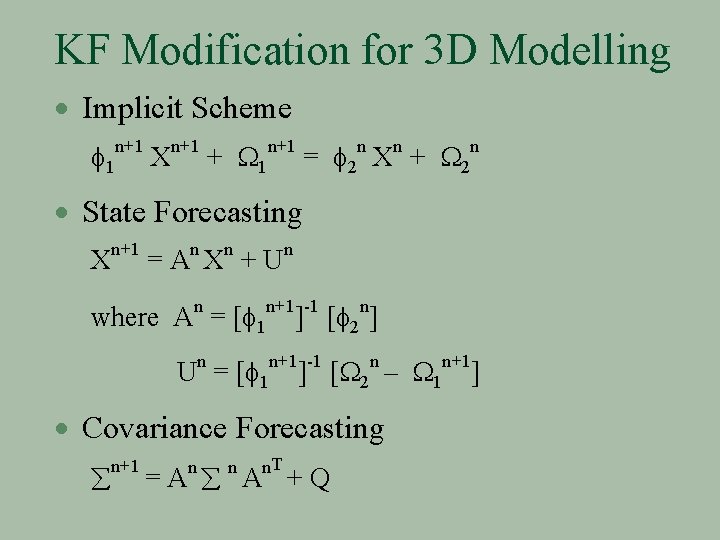
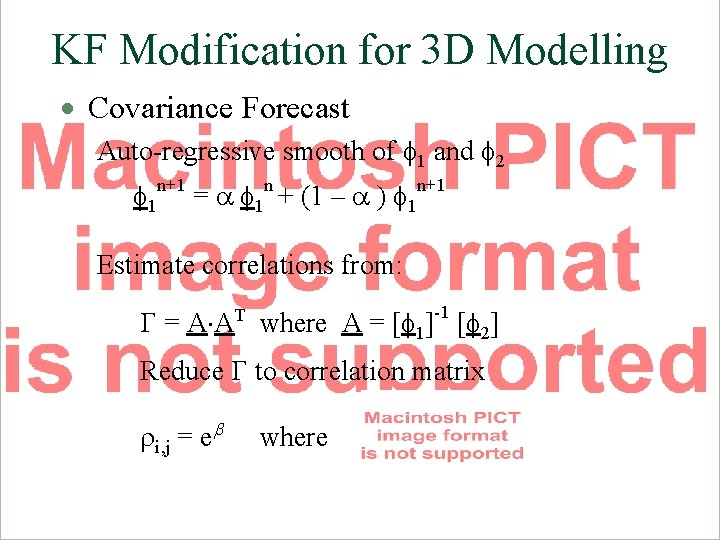
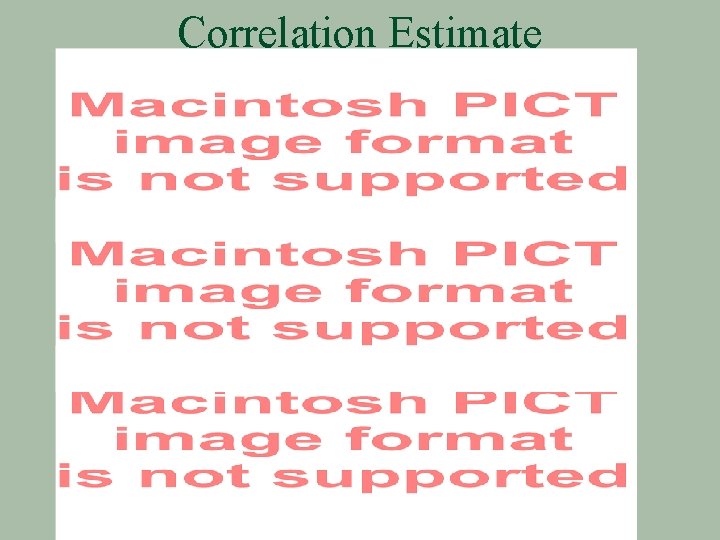
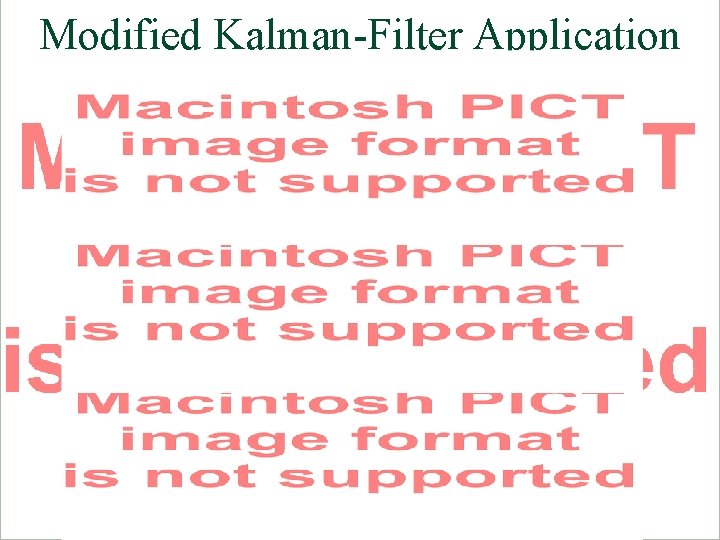
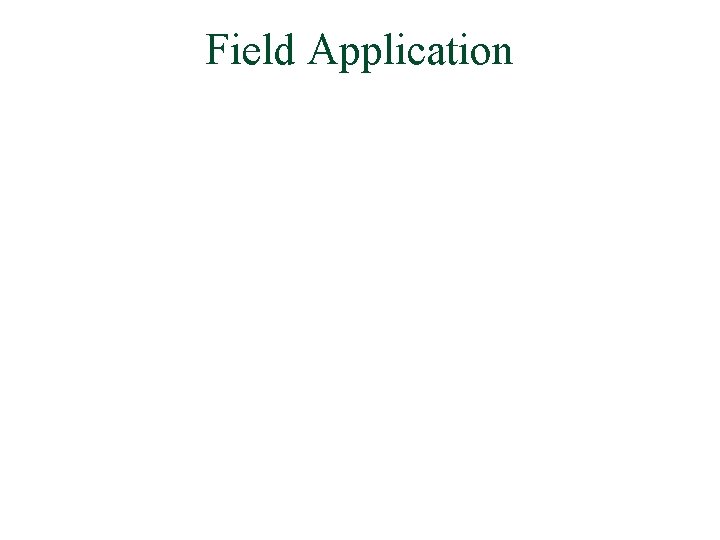
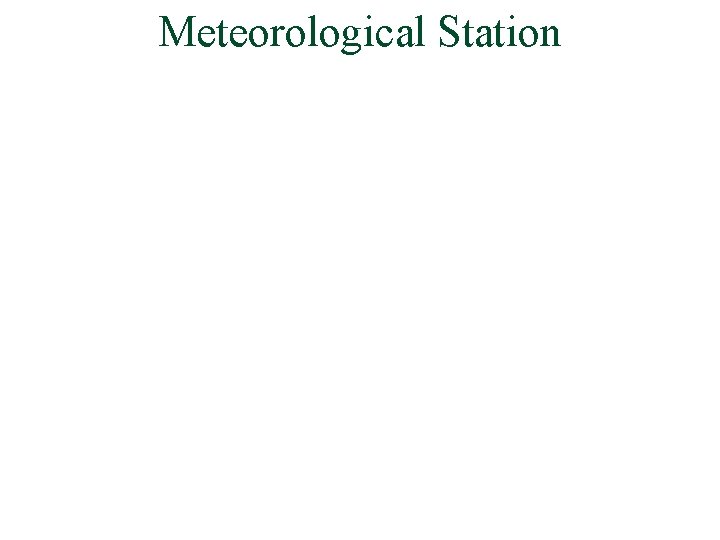
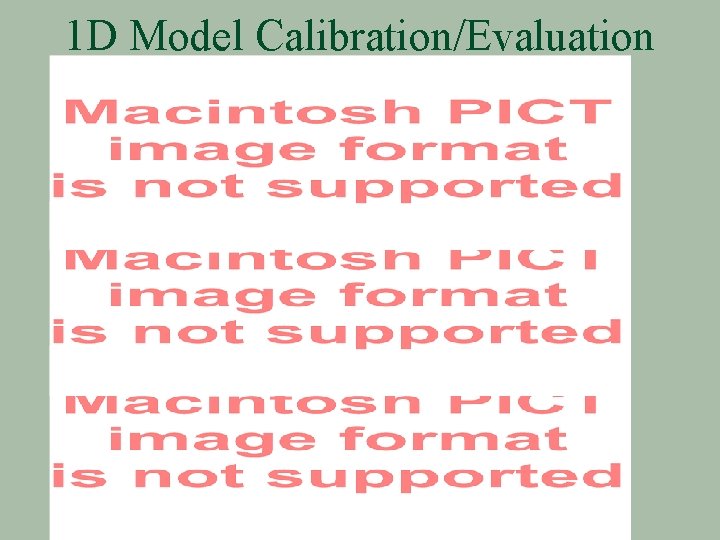
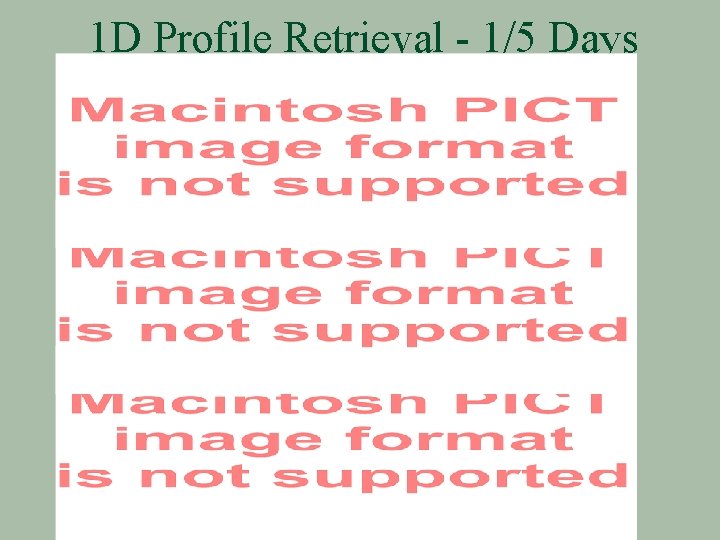
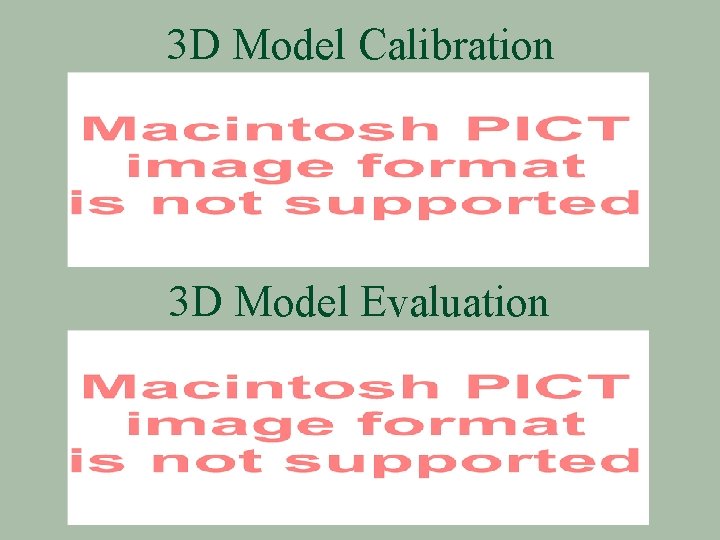
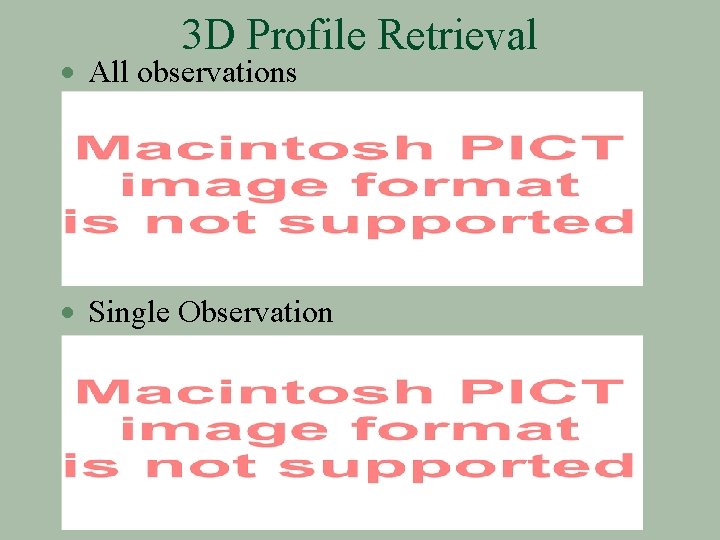
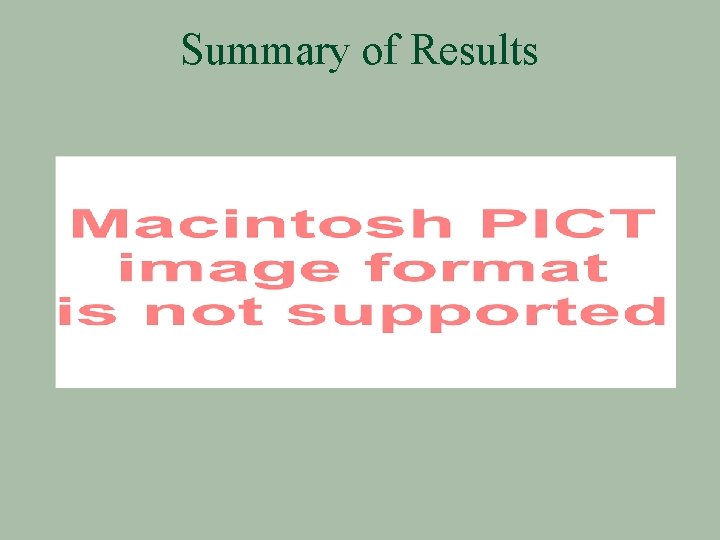
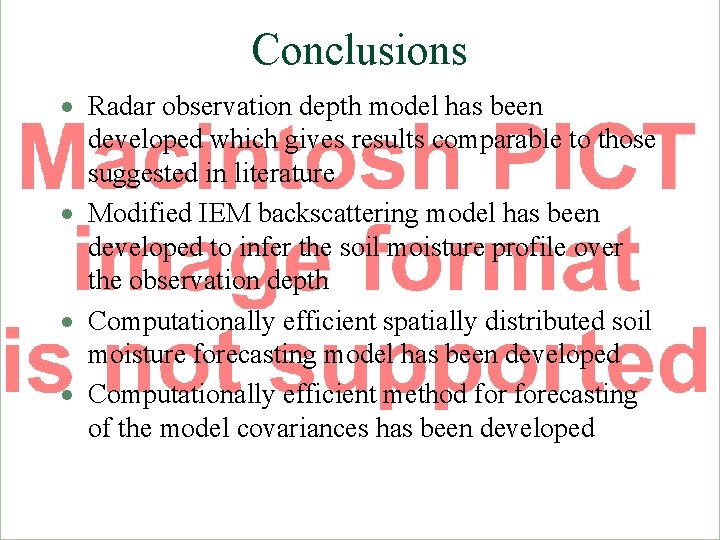
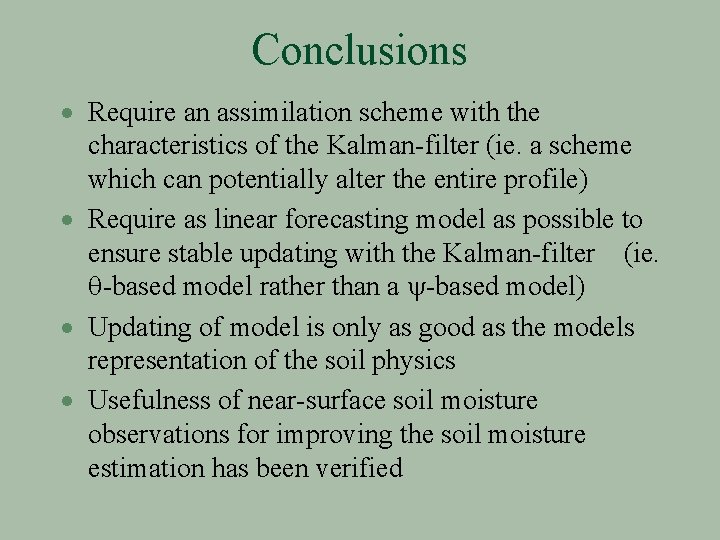
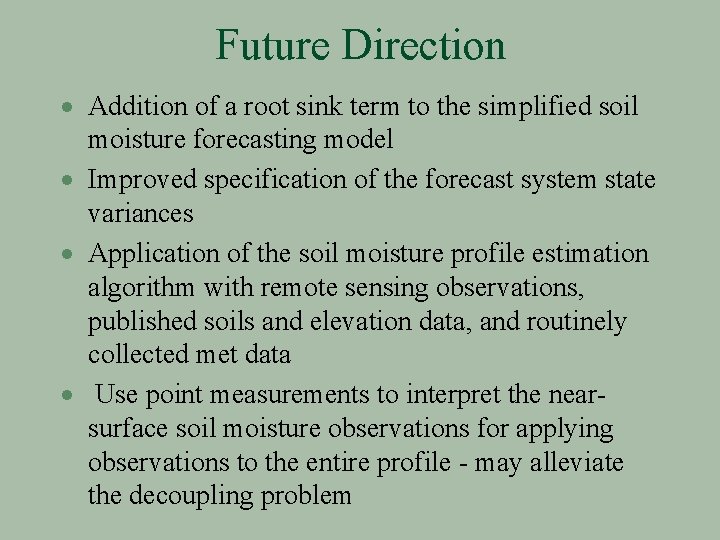
- Slides: 43
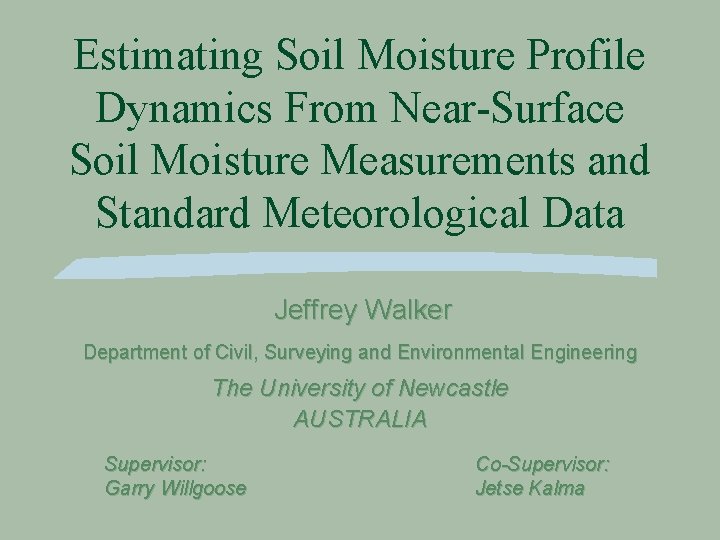
Estimating Soil Moisture Profile Dynamics From Near-Surface Soil Moisture Measurements and Standard Meteorological Data Jeffrey Walker Department of Civil, Surveying and Environmental Engineering The University of Newcastle AUSTRALIA Supervisor: Garry Willgoose Co-Supervisor: Jetse Kalma
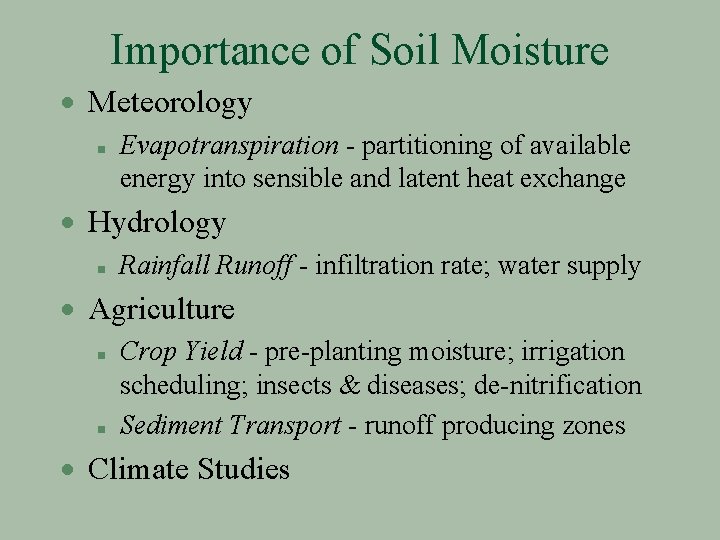
Importance of Soil Moisture · Meteorology n Evapotranspiration - partitioning of available energy into sensible and latent heat exchange · Hydrology n Rainfall Runoff - infiltration rate; water supply · Agriculture n n Crop Yield - pre-planting moisture; irrigation scheduling; insects & diseases; de-nitrification Sediment Transport - runoff producing zones · Climate Studies
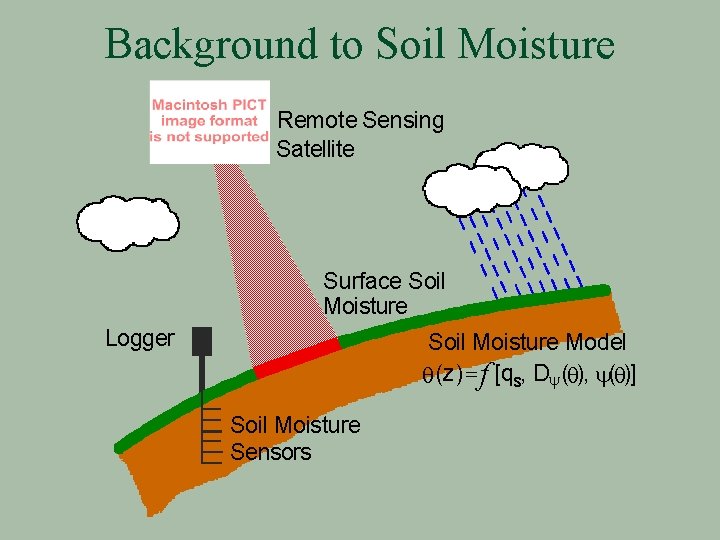
Background to Soil Moisture Remote Sensing Satellite Surface Soil Moisture Logger Soil Moisture Model (z) f [qs, D ( ), ( )] Soil Moisture Sensors
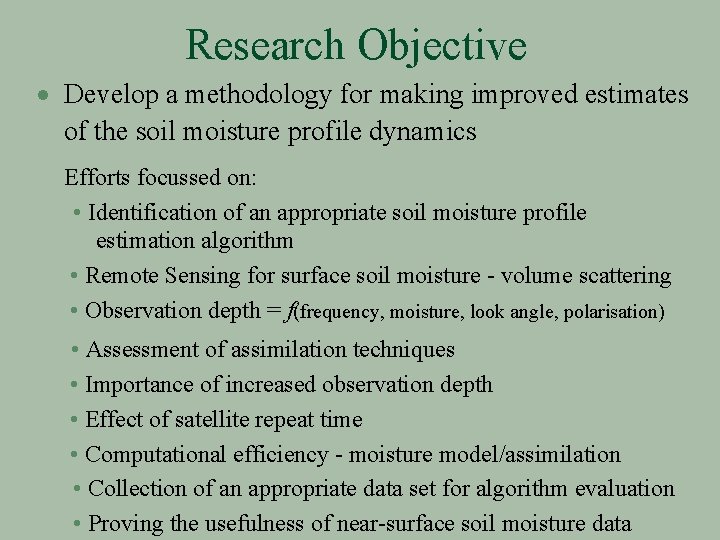
Research Objective · Develop a methodology for making improved estimates of the soil moisture profile dynamics Efforts focussed on: • Identification of an appropriate soil moisture profile estimation algorithm • Remote Sensing for surface soil moisture - volume scattering • Observation depth = f(frequency, moisture, look angle, polarisation) • Assessment of assimilation techniques • Importance of increased observation depth • Effect of satellite repeat time • Computational efficiency - moisture model/assimilation • Collection of an appropriate data set for algorithm evaluation • Proving the usefulness of near-surface soil moisture data
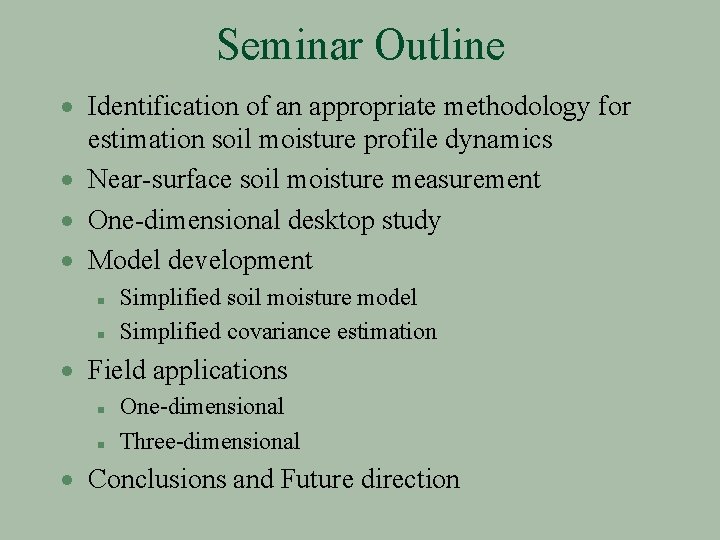
Seminar Outline · Identification of an appropriate methodology for estimation soil moisture profile dynamics · Near-surface soil moisture measurement · One-dimensional desktop study · Model development n n Simplified soil moisture model Simplified covariance estimation · Field applications n n One-dimensional Three-dimensional · Conclusions and Future direction
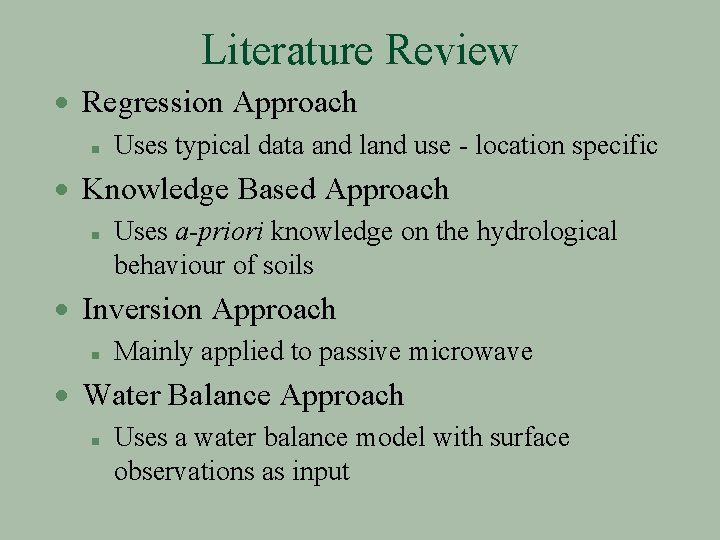
Literature Review · Regression Approach n Uses typical data and land use - location specific · Knowledge Based Approach n Uses a-priori knowledge on the hydrological behaviour of soils · Inversion Approach n Mainly applied to passive microwave · Water Balance Approach n Uses a water balance model with surface observations as input
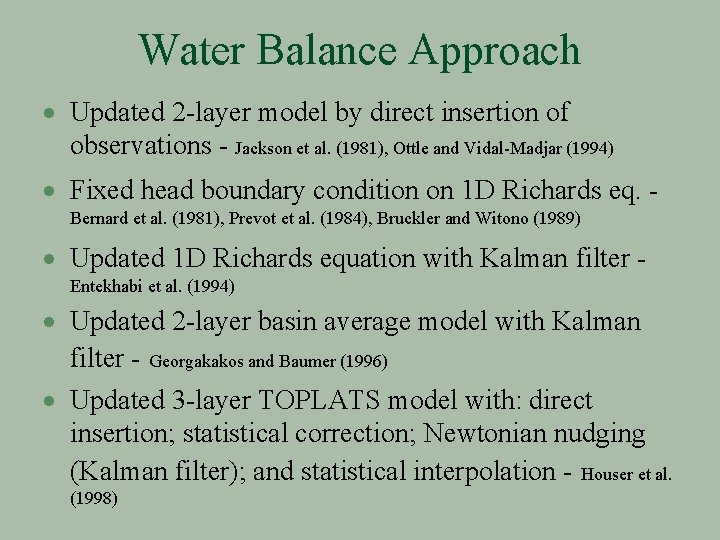
Water Balance Approach · Updated 2 -layer model by direct insertion of observations - Jackson et al. (1981), Ottle and Vidal-Madjar (1994) · Fixed head boundary condition on 1 D Richards eq. Bernard et al. (1981), Prevot et al. (1984), Bruckler and Witono (1989) · Updated 1 D Richards equation with Kalman filter Entekhabi et al. (1994) · Updated 2 -layer basin average model with Kalman filter - Georgakakos and Baumer (1996) · Updated 3 -layer TOPLATS model with: direct insertion; statistical correction; Newtonian nudging (Kalman filter); and statistical interpolation - Houser et al. (1998)
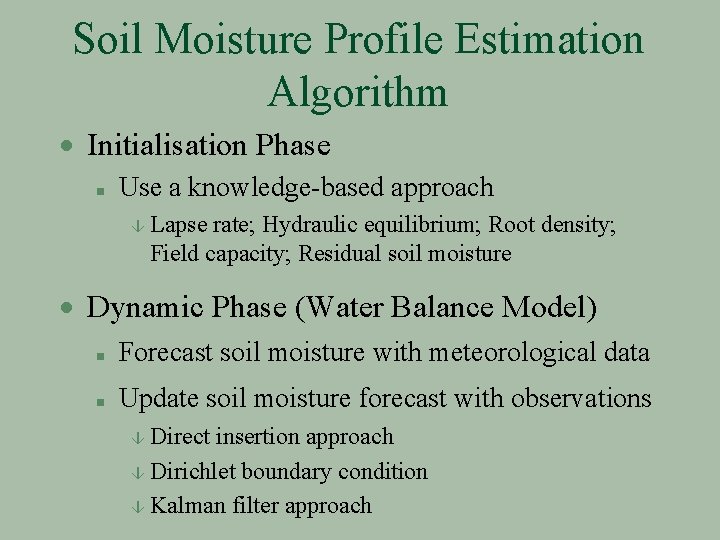
Soil Moisture Profile Estimation Algorithm · Initialisation Phase n Use a knowledge-based approach Lapse rate; Hydraulic equilibrium; Root density; Field capacity; Residual soil moisture · Dynamic Phase (Water Balance Model) n Forecast soil moisture with meteorological data n Update soil moisture forecast with observations Direct insertion approach Dirichlet boundary condition Kalman filter approach
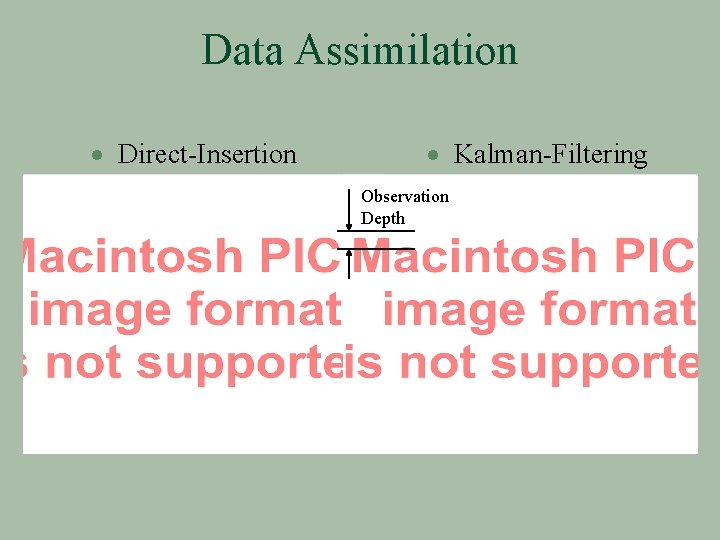
Data Assimilation · Direct-Insertion · Kalman-Filtering Observation Depth
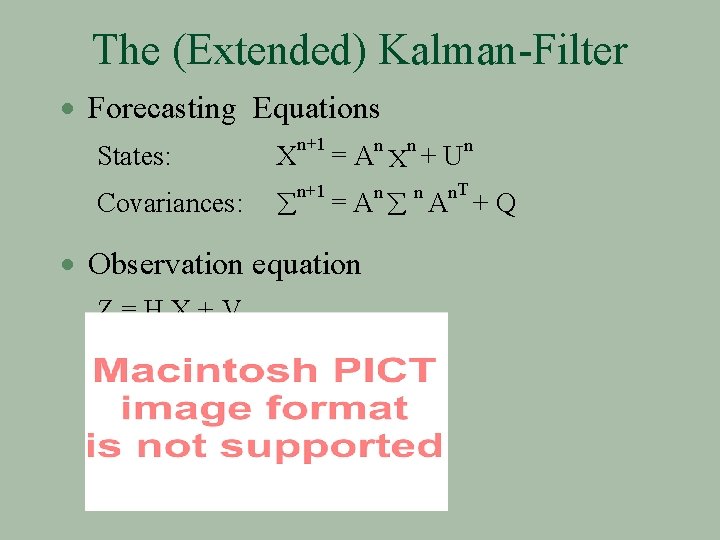
The (Extended) Kalman-Filter · Forecasting Equations States: Covariances: X n+1 n =A X +U n n. T =A A +Q · Observation equation Z=HX+V n
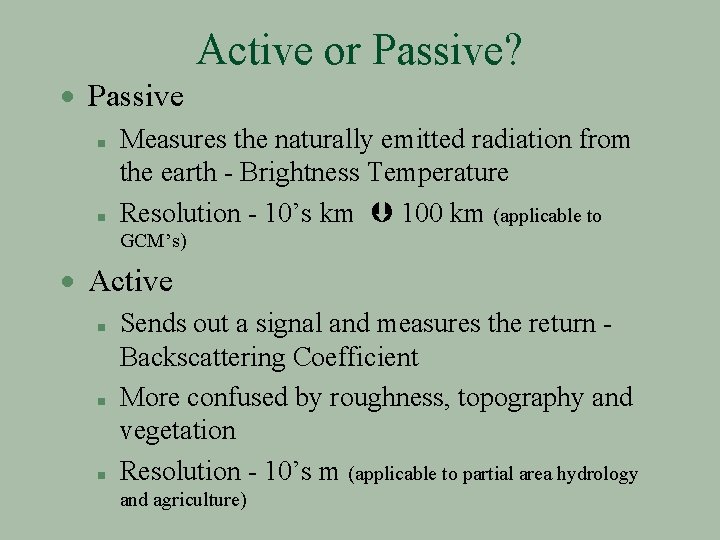
Active or Passive? · Passive n n Measures the naturally emitted radiation from the earth - Brightness Temperature Resolution - 10’s km 100 km (applicable to GCM’s) · Active n n n Sends out a signal and measures the return Backscattering Coefficient More confused by roughness, topography and vegetation Resolution - 10’s m (applicable to partial area hydrology and agriculture)
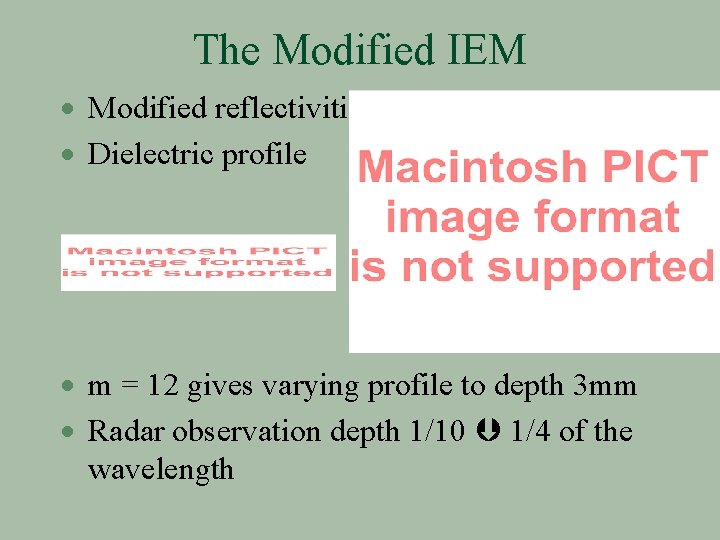
The Modified IEM · Modified reflectivities · Dielectric profile · m = 12 gives varying profile to depth 3 mm · Radar observation depth 1/10 1/4 of the wavelength
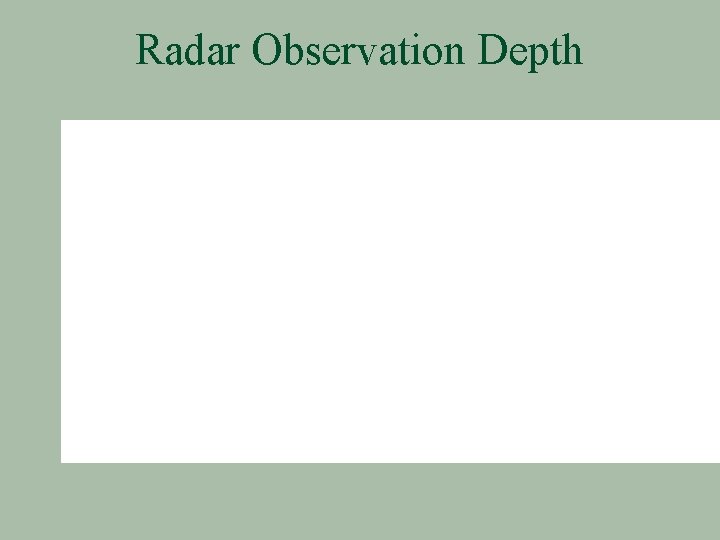
Radar Observation Depth
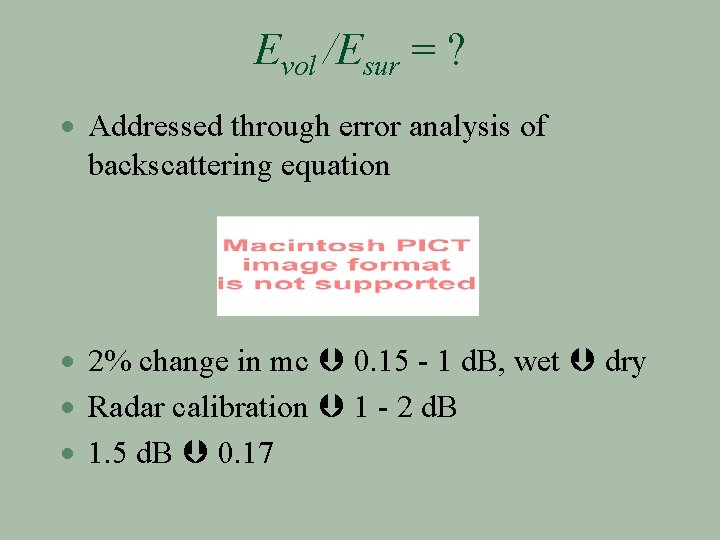
Evol /Esur = ? · Addressed through error analysis of backscattering equation · 2% change in mc 0. 15 - 1 d. B, wet dry · Radar calibration 1 - 2 d. B · 1. 5 d. B 0. 17
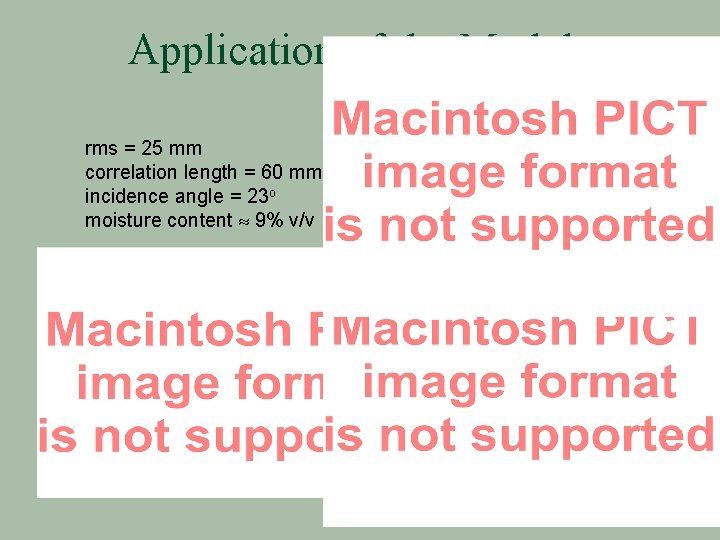
Application of the Models rms = 25 mm correlation length = 60 mm incidence angle = 23 o moisture content 9% v/v hh polarisation vv polarisation
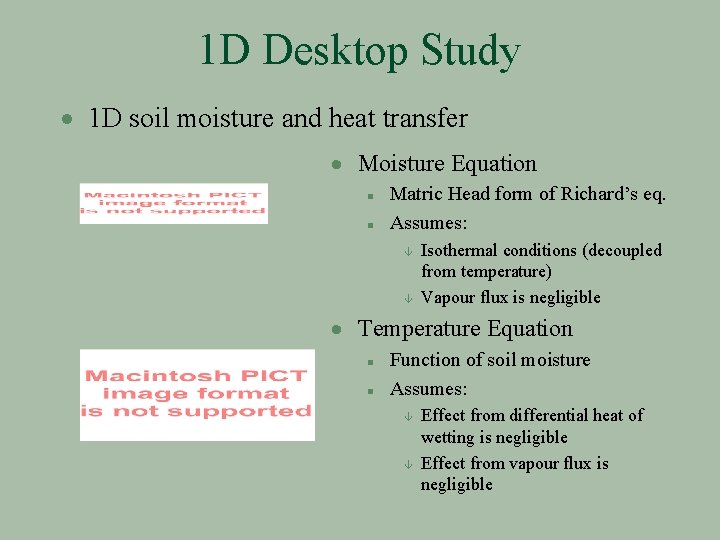
1 D Desktop Study · 1 D soil moisture and heat transfer · Moisture Equation n n Matric Head form of Richard’s eq. Assumes: Isothermal conditions (decoupled from temperature) Vapour flux is negligible · Temperature Equation n n Function of soil moisture Assumes: Effect from differential heat of wetting is negligible Effect from vapour flux is negligible
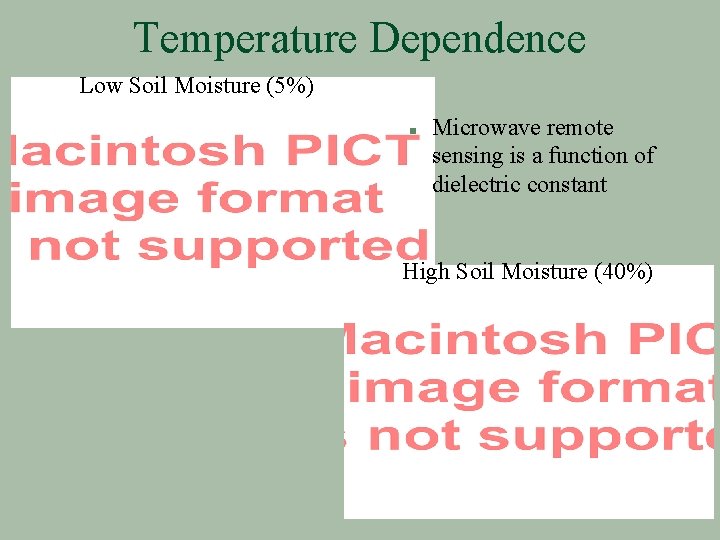
Temperature Dependence Low Soil Moisture (5%) n Microwave remote sensing is a function of dielectric constant High Soil Moisture (40%)
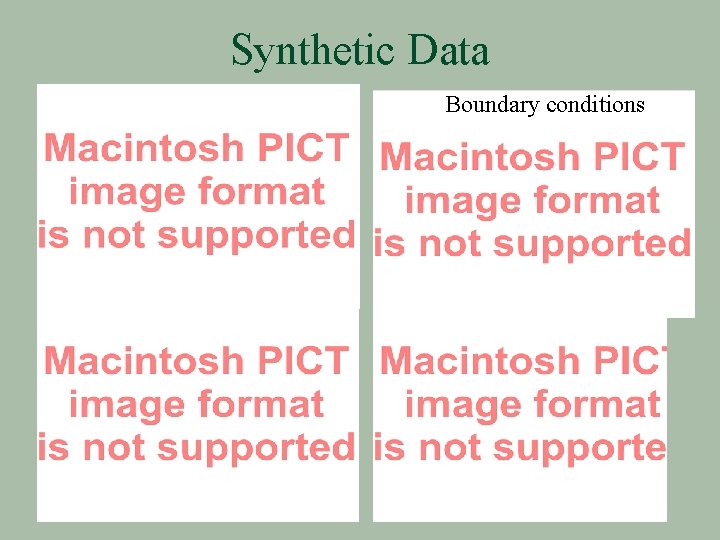
Synthetic Data Initial conditions Boundary conditions
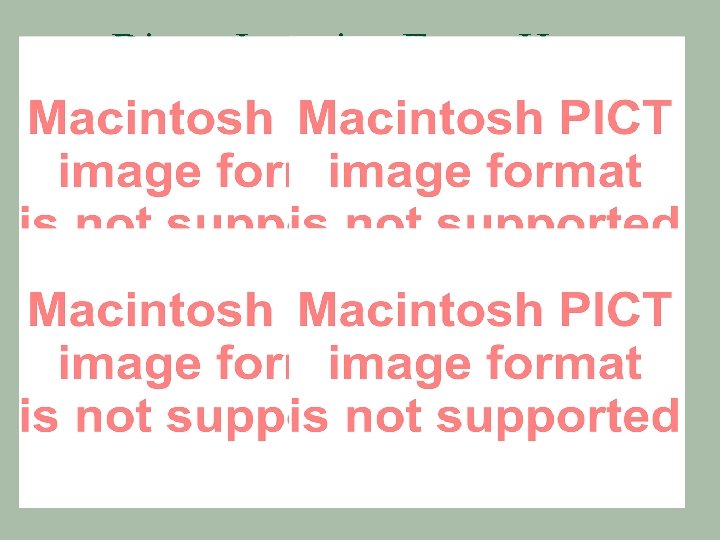
Direct-Insertion Every Hour
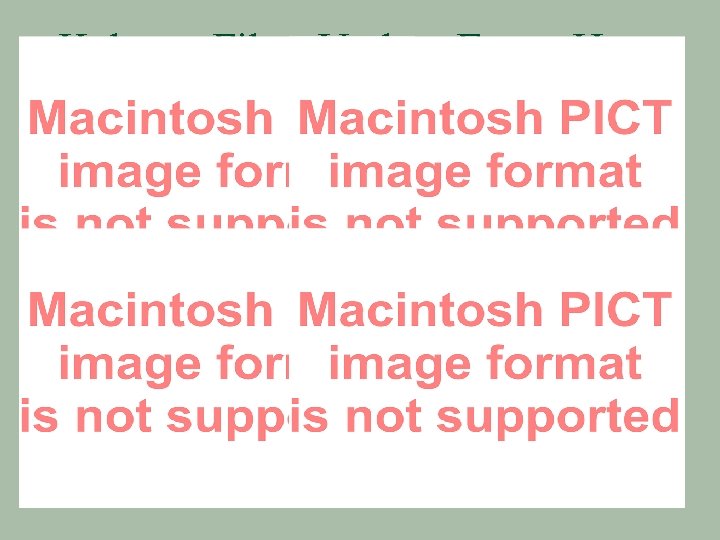
Kalman-Filter Update Every Hour
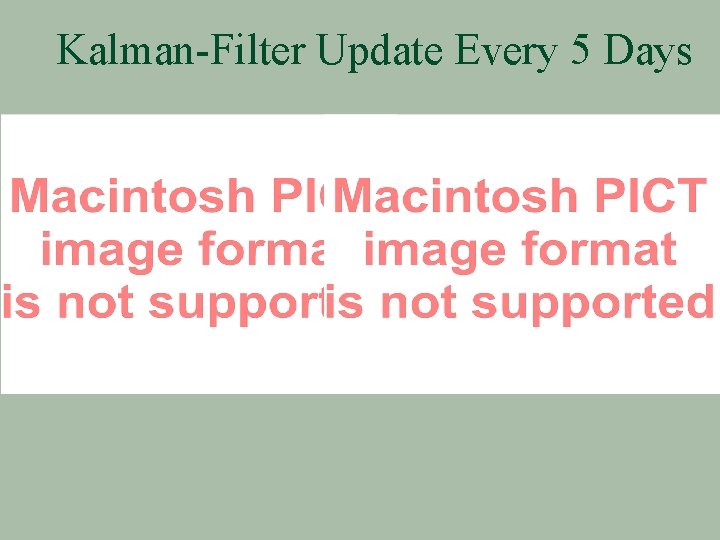
Kalman-Filter Update Every 5 Days
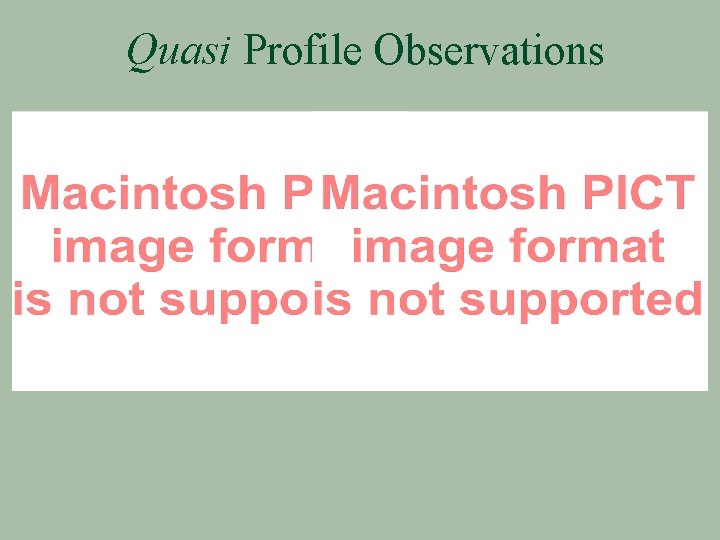
Quasi Profile Observations
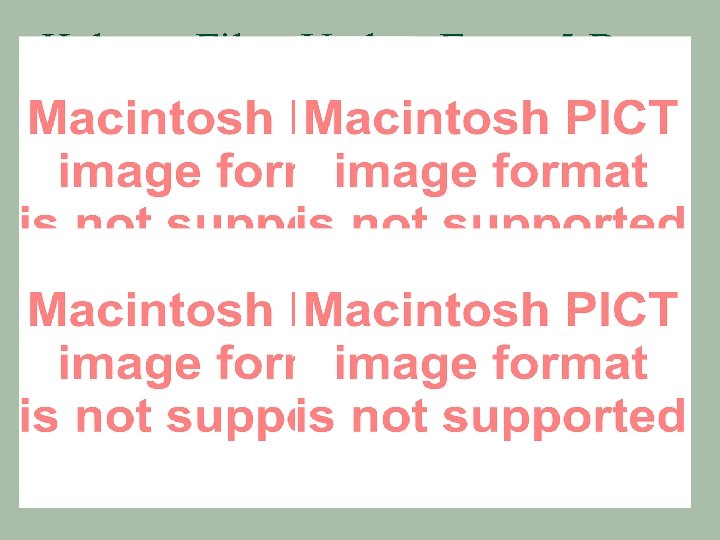
Kalman-Filter Update Every 5 Days
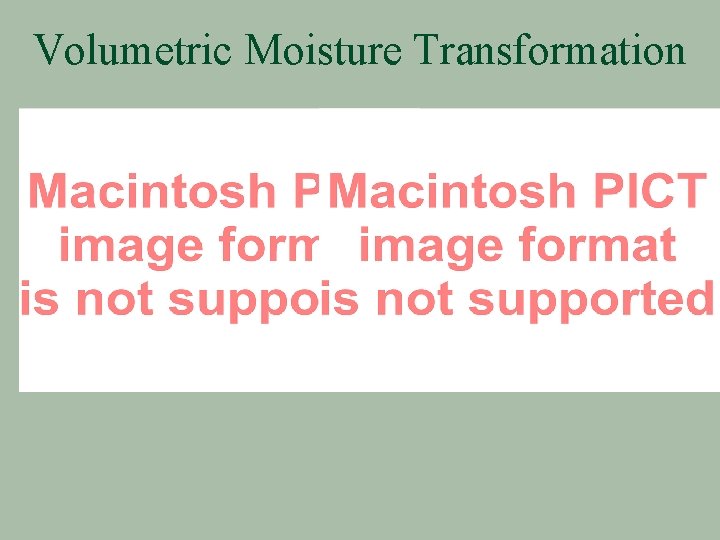
Volumetric Moisture Transformation
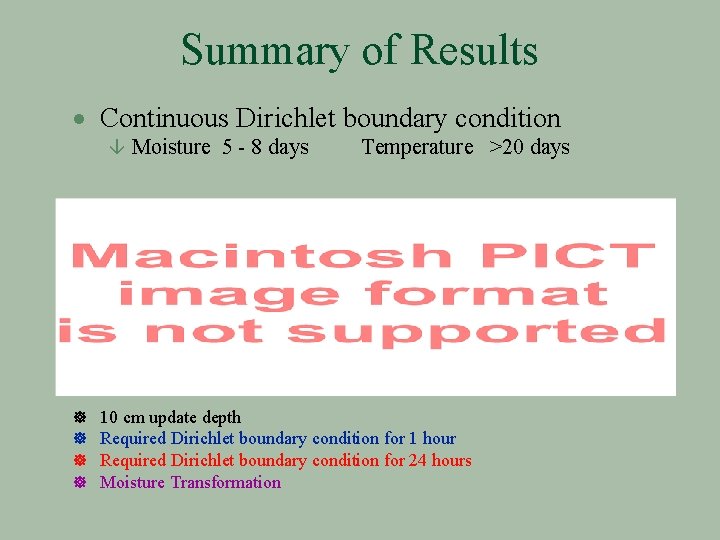
Summary of Results · Continuous Dirichlet boundary condition Moisture 5 - 8 days Temperature >20 days 10 cm update depth Required Dirichlet boundary condition for 1 hour Required Dirichlet boundary condition for 24 hours Moisture Transformation
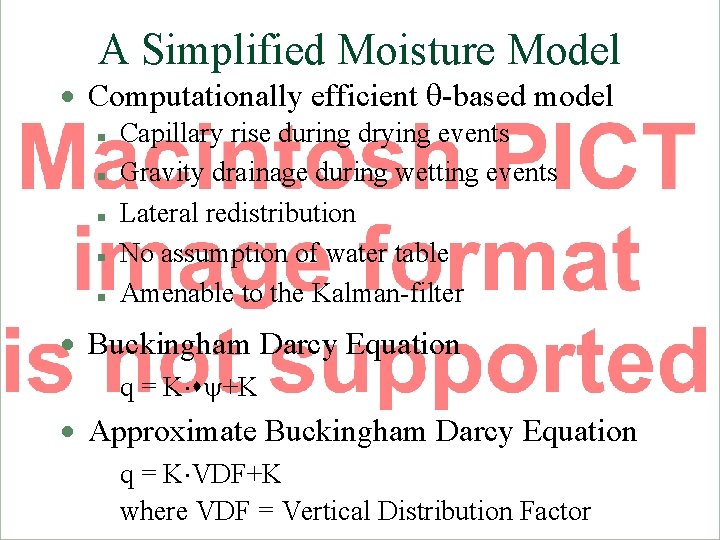
A Simplified Moisture Model · Computationally efficient -based model n n n Capillary rise during drying events Gravity drainage during wetting events Lateral redistribution No assumption of water table Amenable to the Kalman-filter · Buckingham Darcy Equation q = K +K · Approximate Buckingham Darcy Equation q = K VDF+K where VDF = Vertical Distribution Factor
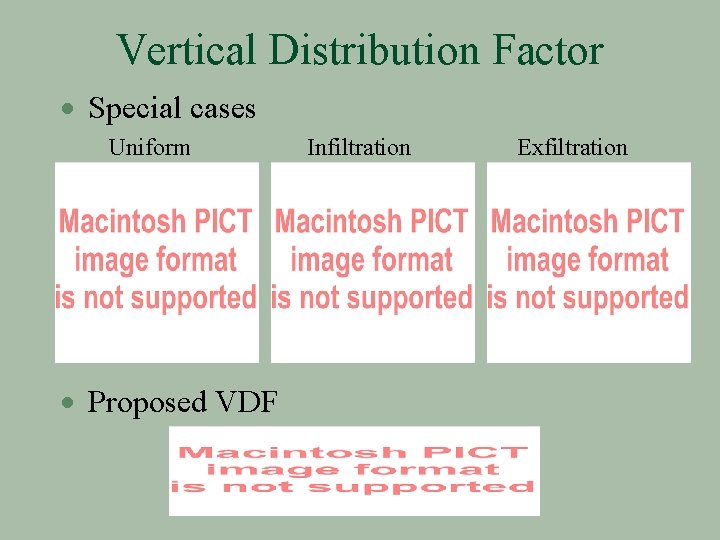
Vertical Distribution Factor · Special cases Uniform · Proposed VDF Infiltration Exfiltration
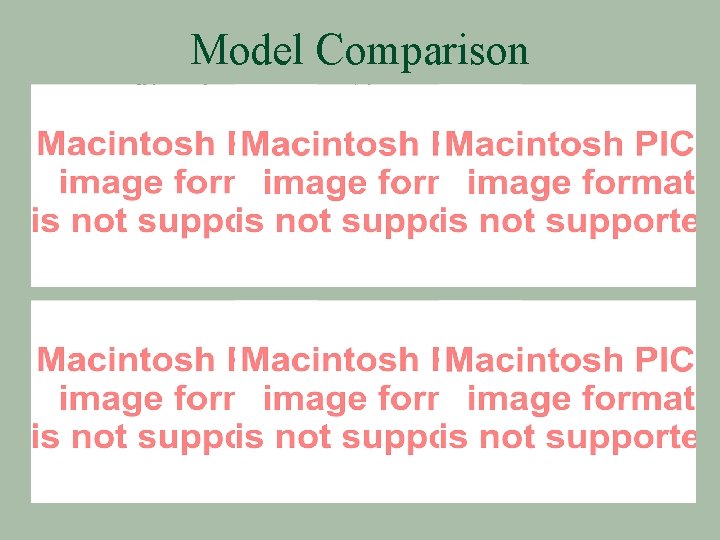
Model Comparison · Exfiltration (0. 5 cm/day) · Infiltration (10 mm/hr)
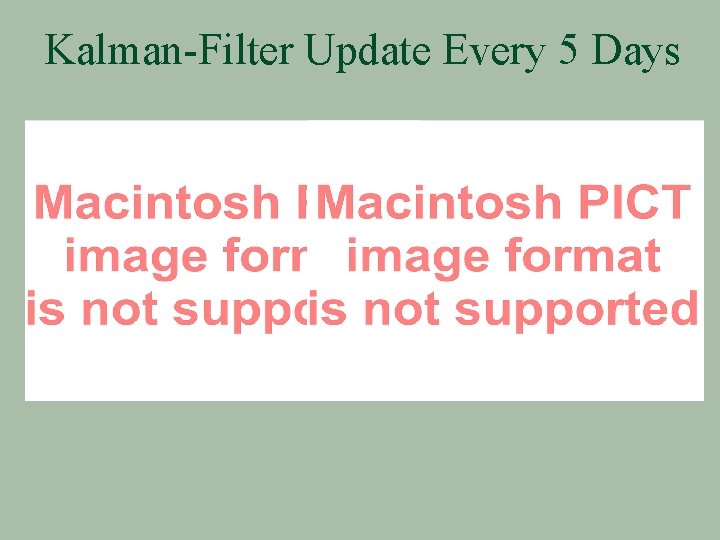
Kalman-Filter Update Every 5 Days
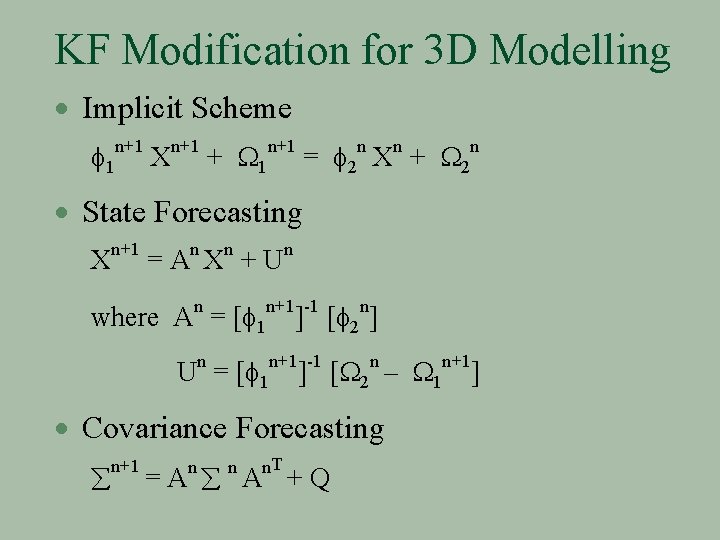
KF Modification for 3 D Modelling · Implicit Scheme 1 n+1 Xn+1 + 1 n+1 = 2 n Xn + 2 n · State Forecasting X n+1 n n =A X +U n n n+1 -1 n [ 2 ] n n+1 -1 n [ 2 – where A = [ 1 U = [ 1 ] ] · Covariance Forecasting n+1 n n n. T =A A +Q n+1 1 ]
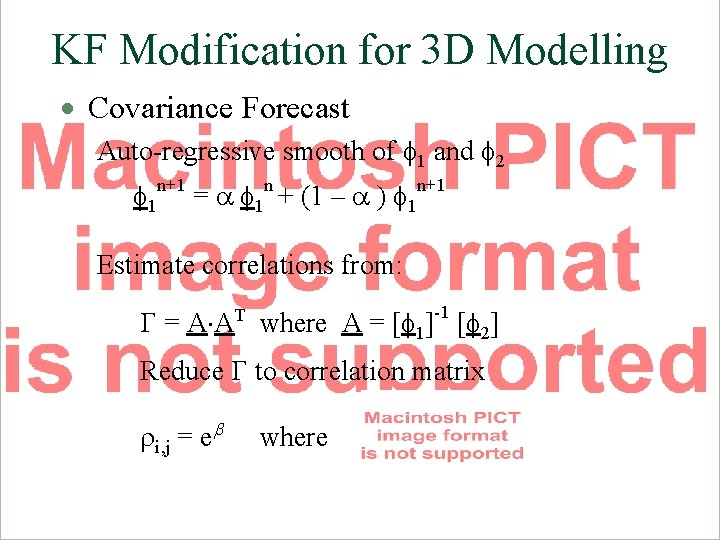
KF Modification for 3 D Modelling · Covariance Forecast Auto-regressive smooth of 1 and 2 n+1 1 n = 1 + (1 – ) n+1 1 Estimate correlations from: = A AT where A = [ 1]-1 [ 2] Reduce to correlation matrix i, j = e where
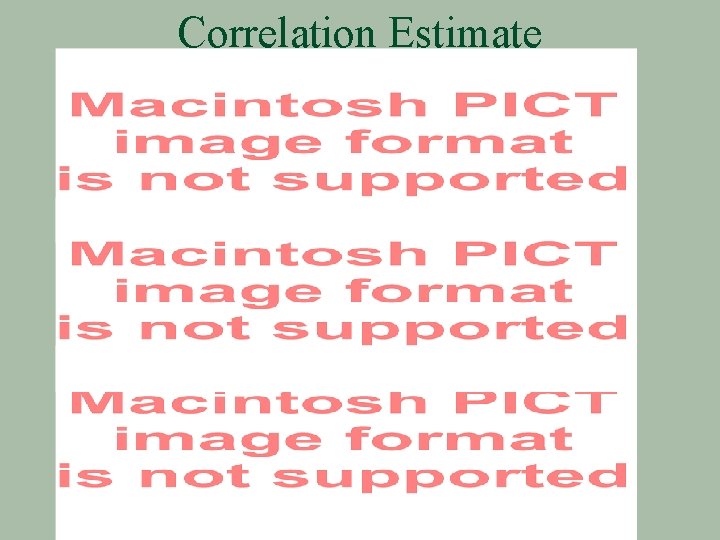
Correlation Estimate
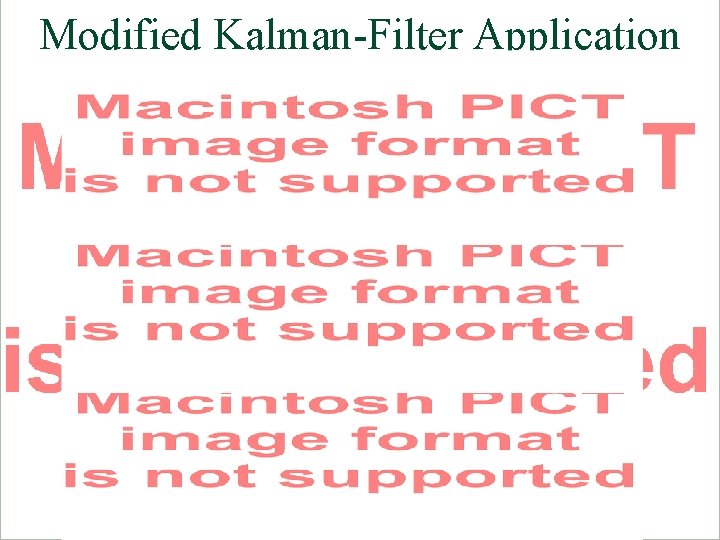
Modified Kalman-Filter Application
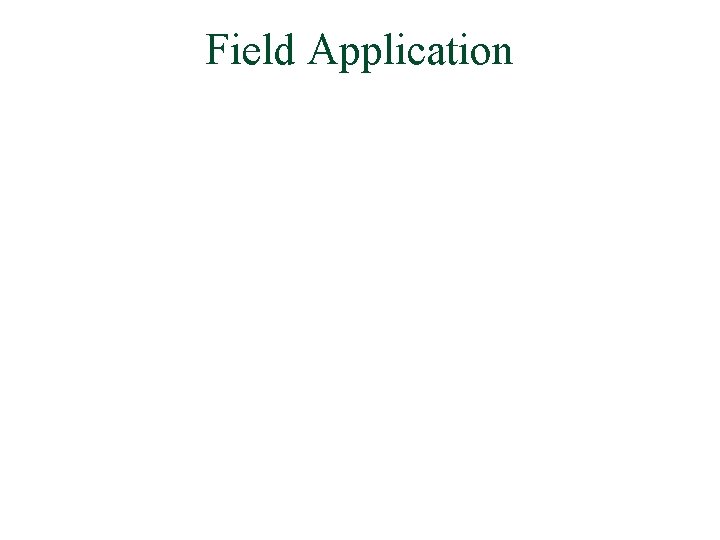
Field Application
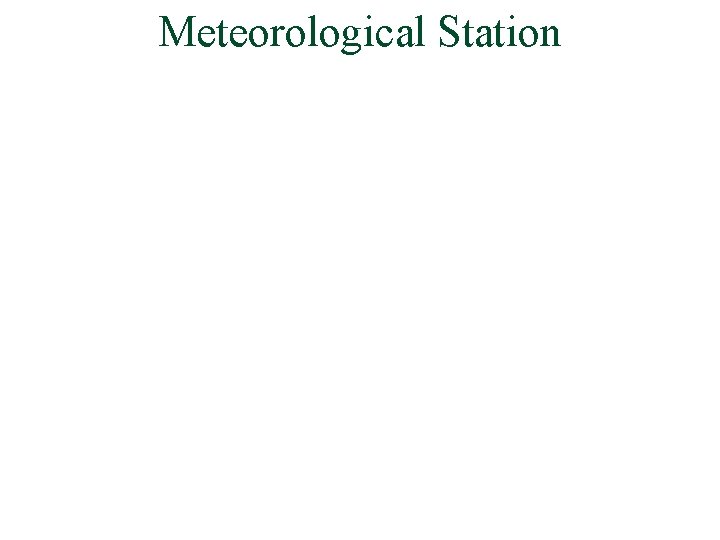
Meteorological Station
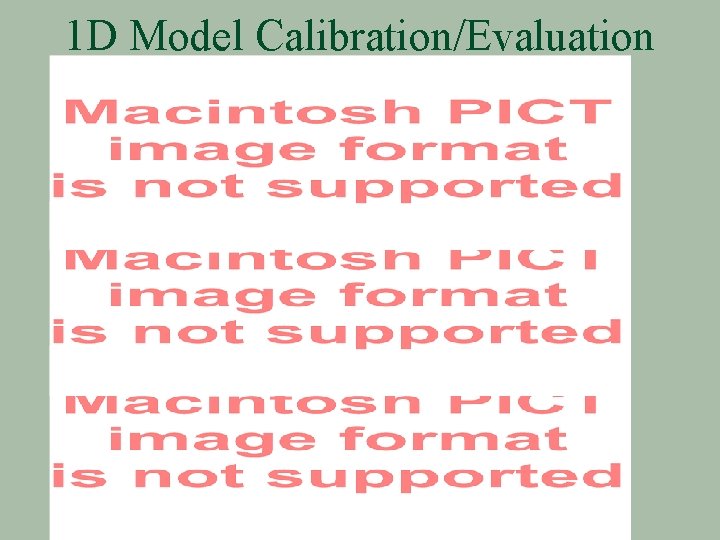
1 D Model Calibration/Evaluation
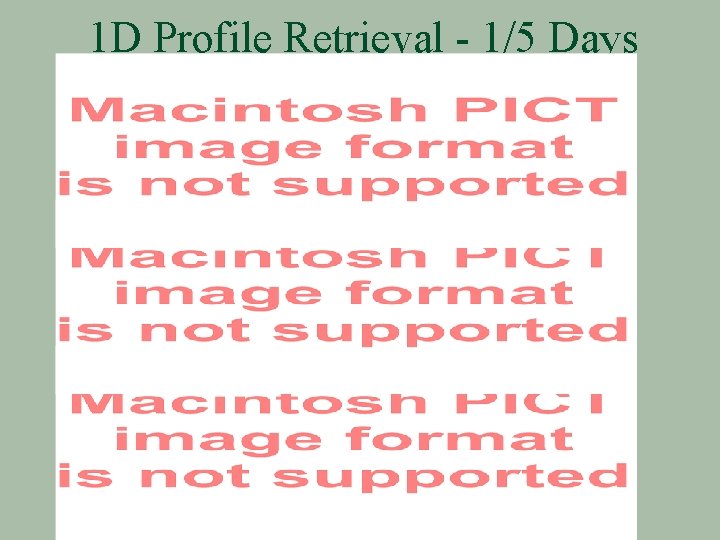
1 D Profile Retrieval - 1/5 Days
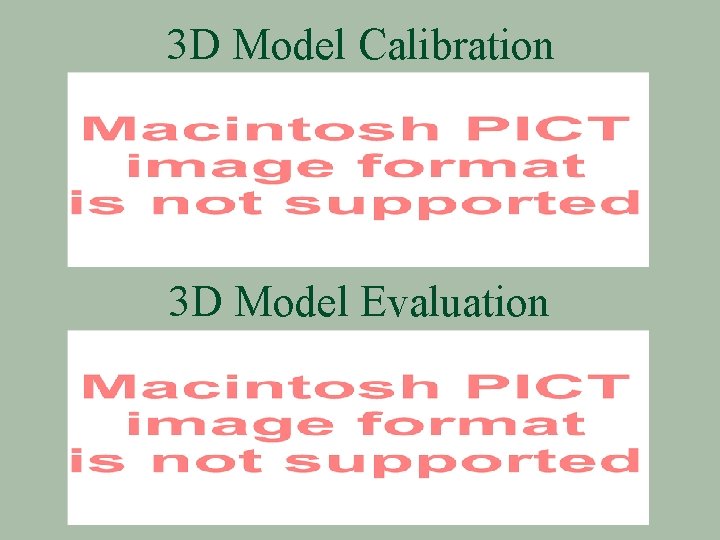
3 D Model Calibration 3 D Model Evaluation
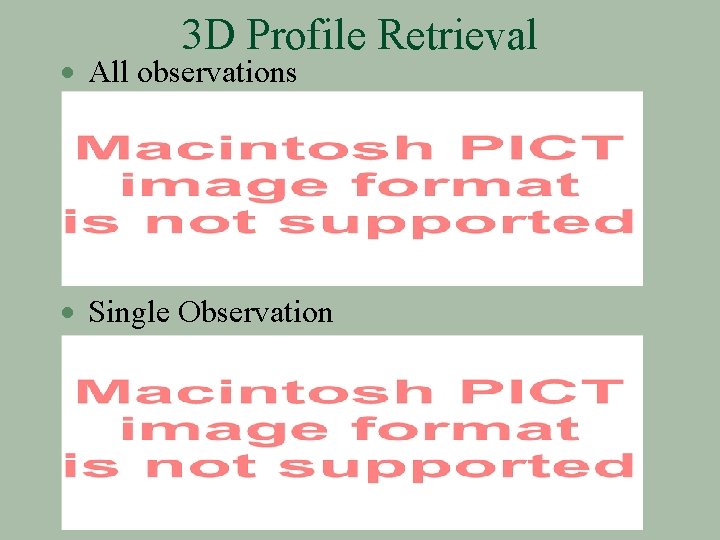
3 D Profile Retrieval · All observations · Single Observation
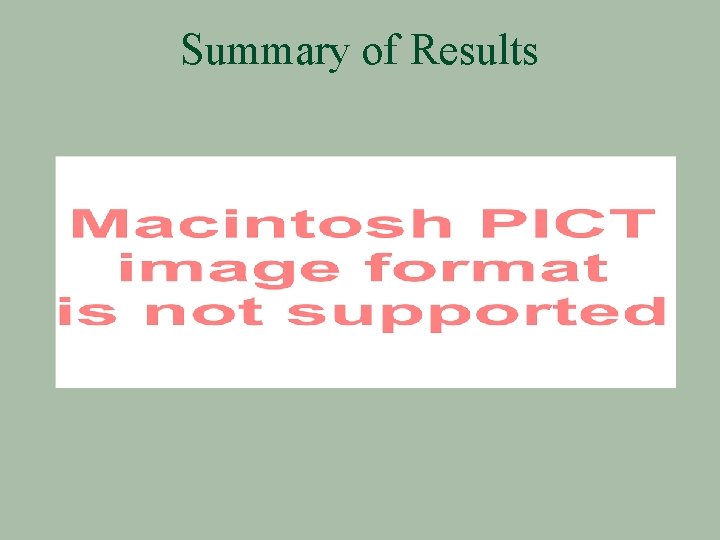
Summary of Results
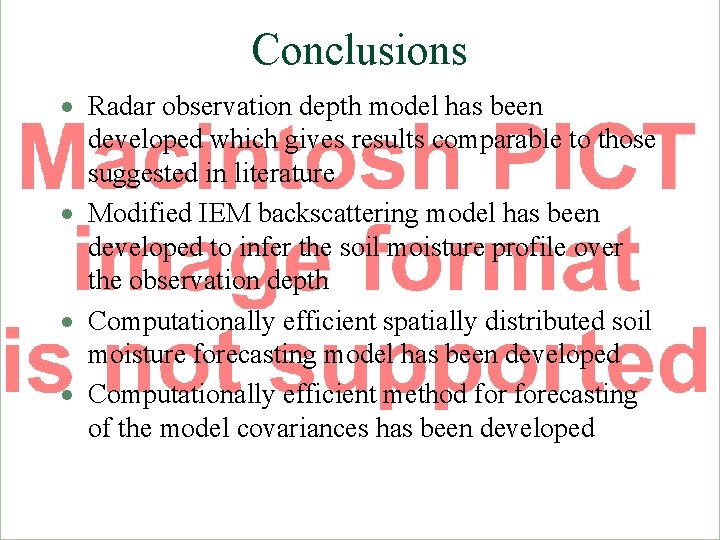
Conclusions · Radar observation depth model has been developed which gives results comparable to those suggested in literature · Modified IEM backscattering model has been developed to infer the soil moisture profile over the observation depth · Computationally efficient spatially distributed soil moisture forecasting model has been developed · Computationally efficient method forecasting of the model covariances has been developed
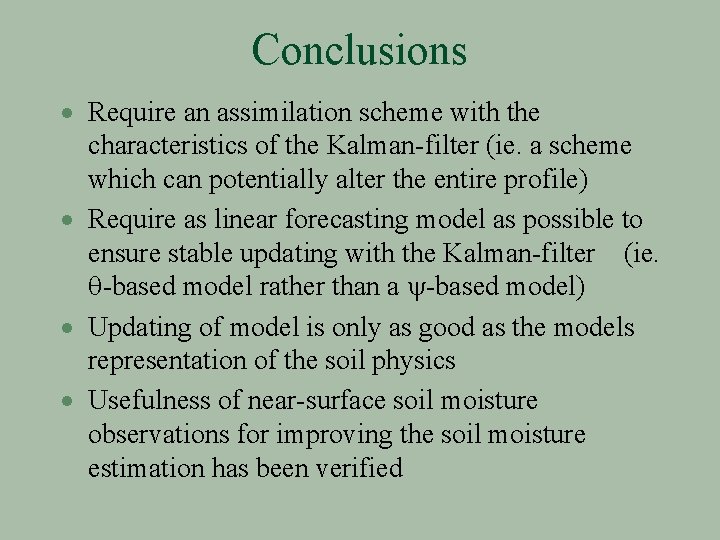
Conclusions · Require an assimilation scheme with the characteristics of the Kalman-filter (ie. a scheme which can potentially alter the entire profile) · Require as linear forecasting model as possible to ensure stable updating with the Kalman-filter (ie. -based model rather than a -based model) · Updating of model is only as good as the models representation of the soil physics · Usefulness of near-surface soil moisture observations for improving the soil moisture estimation has been verified
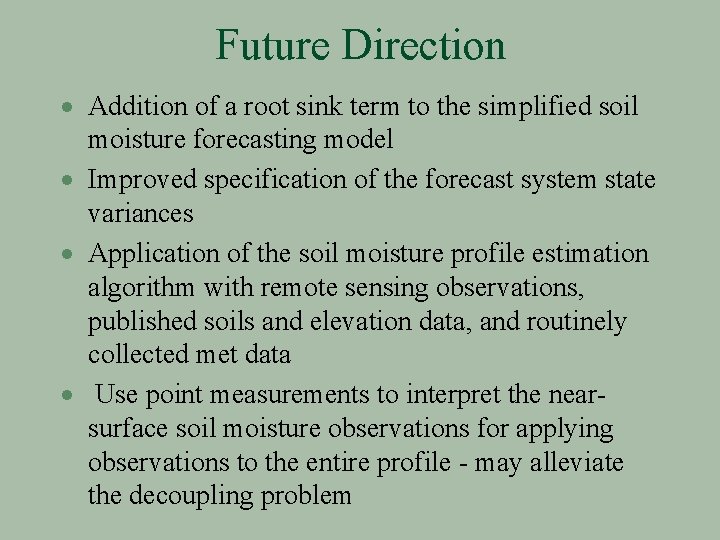
Future Direction · Addition of a root sink term to the simplified soil moisture forecasting model · Improved specification of the forecast system state variances · Application of the soil moisture profile estimation algorithm with remote sensing observations, published soils and elevation data, and routinely collected met data · Use point measurements to interpret the nearsurface soil moisture observations for applying observations to the entire profile - may alleviate the decoupling problem
Estimating soil moisture by feel and appearance
Tanya hoogerwerf
Resistive soil moisture sensor working principle
A capillary tube
Soil moisture sensor
Soil moisture meter argos
Sentek soil moisture
Conflict dynamics profile
Soil profile
Puising
Brown earth soil profile
Red latosol
Soil profile
Clay soil profile
Gley soil higher geography
Soil characteristic
Soil profile
Higher geography biosphere
Soil profile is a vertical cross section
Loose weathered rock and organic material
Red latosols
Food web soil profile
Brown earth soil ireland
Living soil vs dead soil
Living soil vs dead soil
Surface moisture content formula
Chapter 36 moisture control
Moisture holding capacity
Moisture clouds and precipitation
All kinesises
Lab 5 atmospheric moisture
Atum
Moisture and total solids analysis
Frontal wedging
Stand over
Bonide leaf shine and moisture guard
"concrete curing"
Antecedent moisture condition
Moisture equation
Drying rate formula
Danbunama
Wisepac
What is water
Grain moisture content measurement