ESTIMATING OCEANIC PRIMARY PRODUCTIVITY AN EVALUATION OF OCEAN
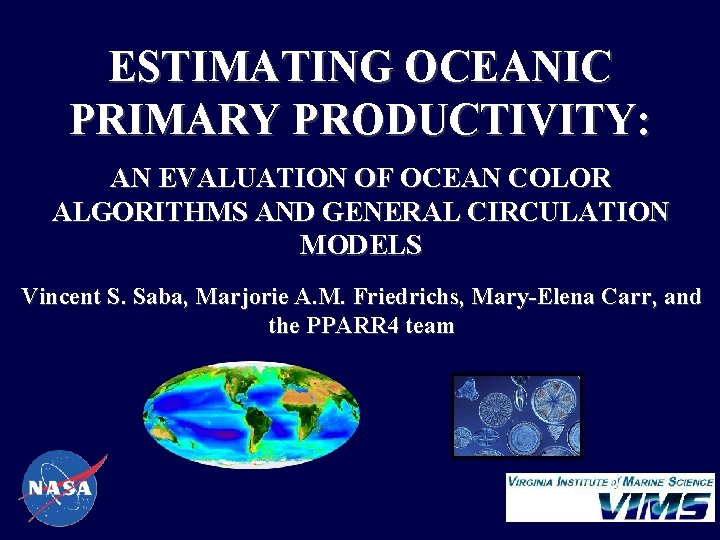
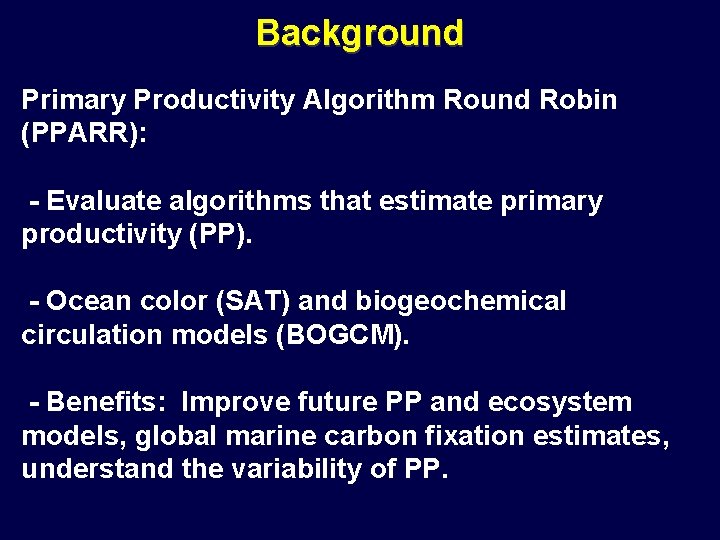
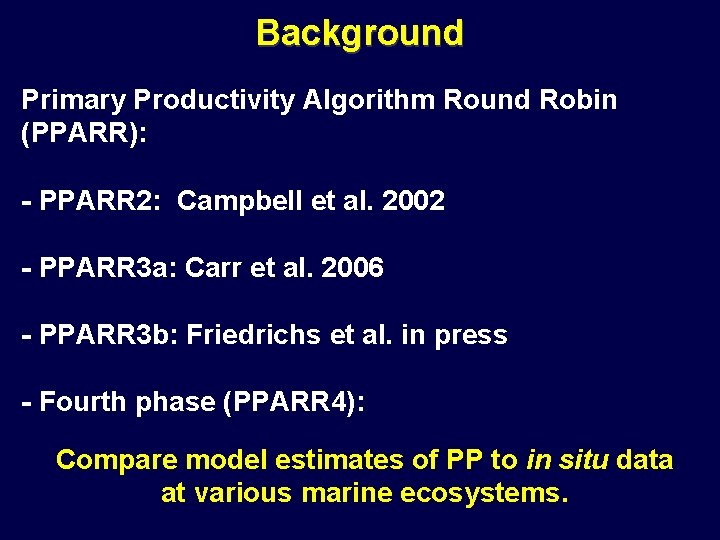
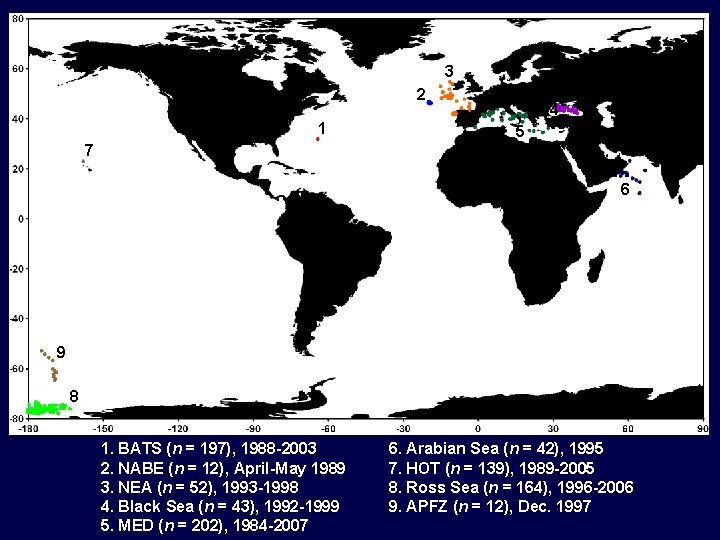
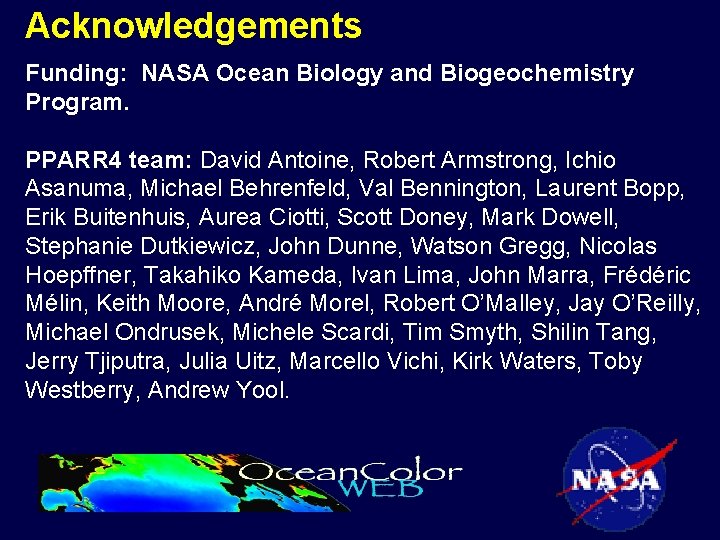
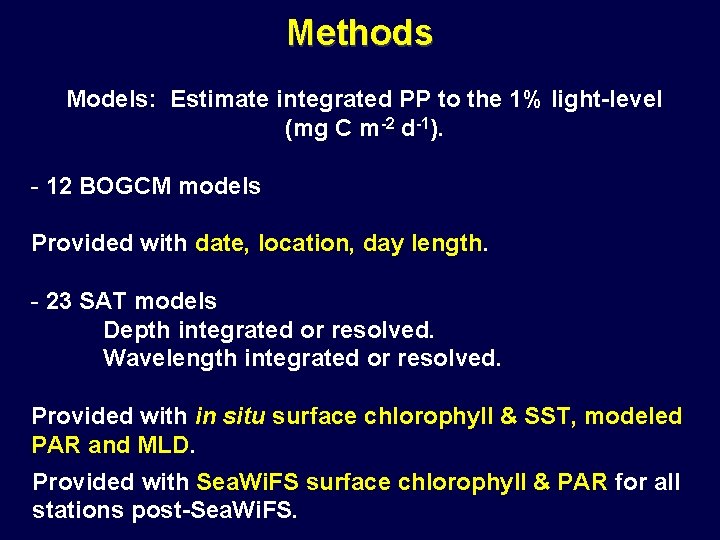
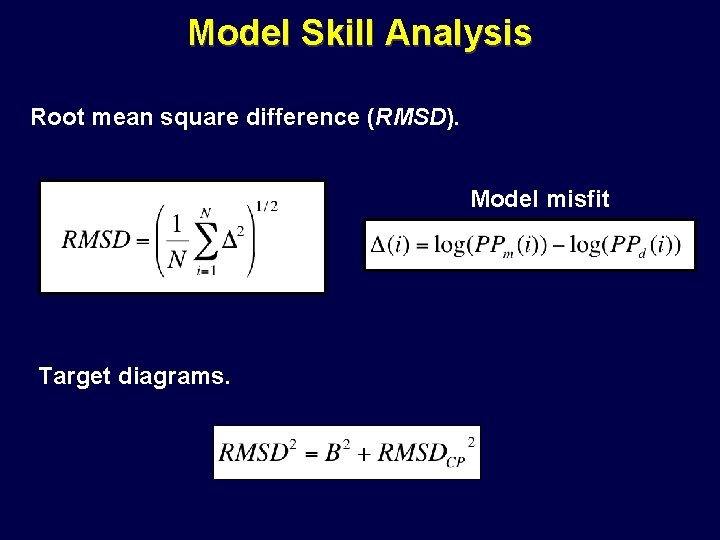
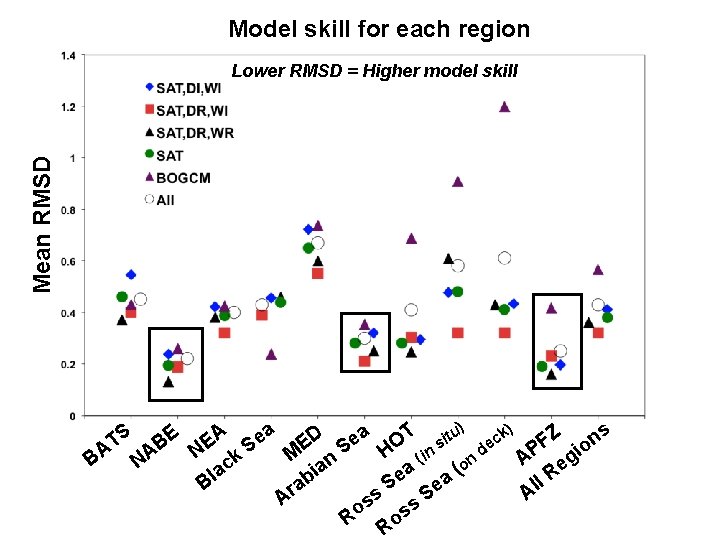
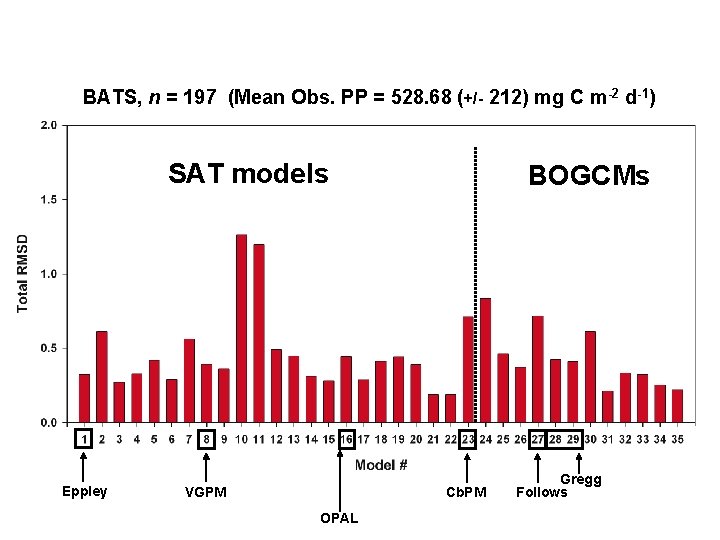
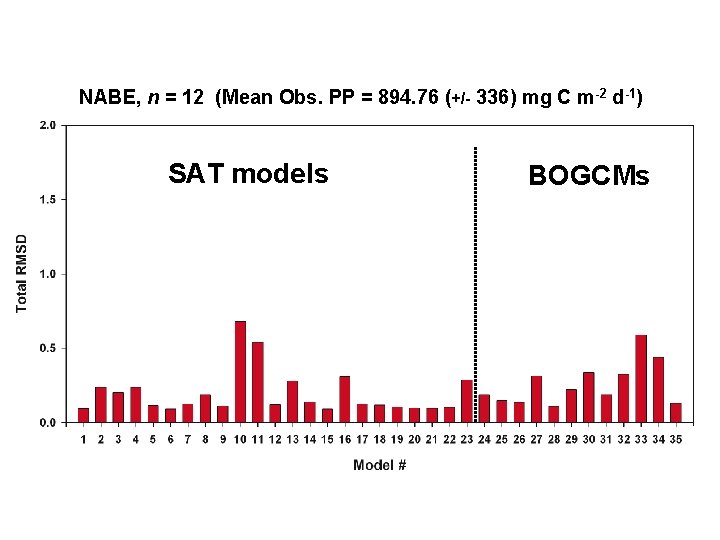
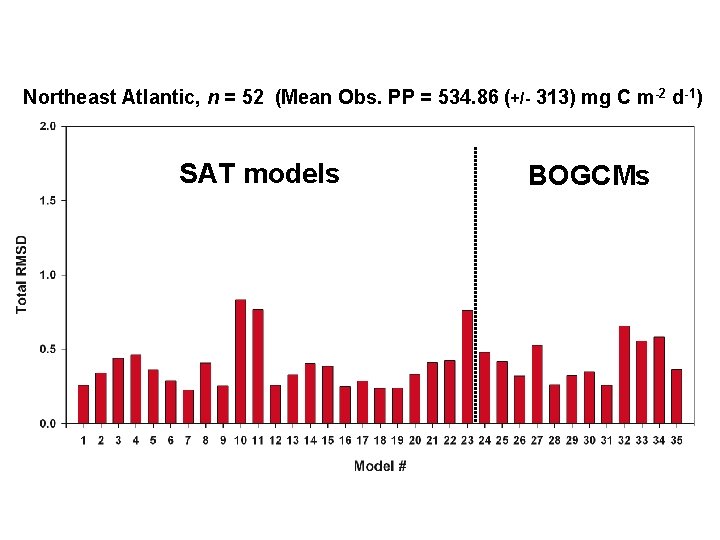
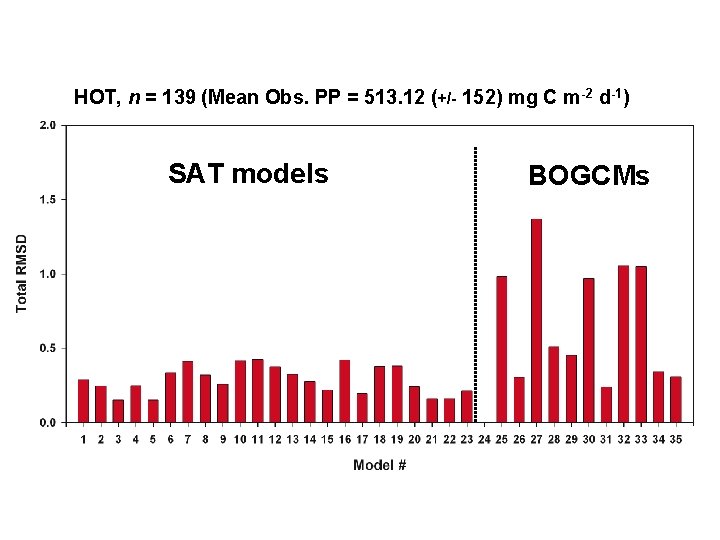
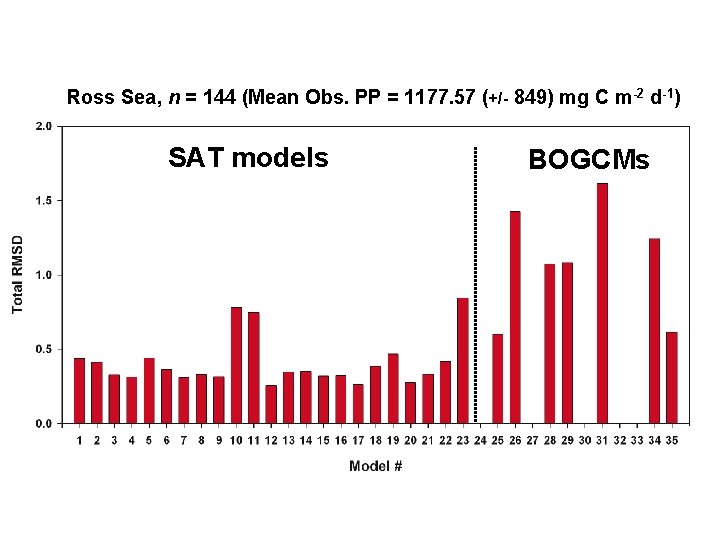
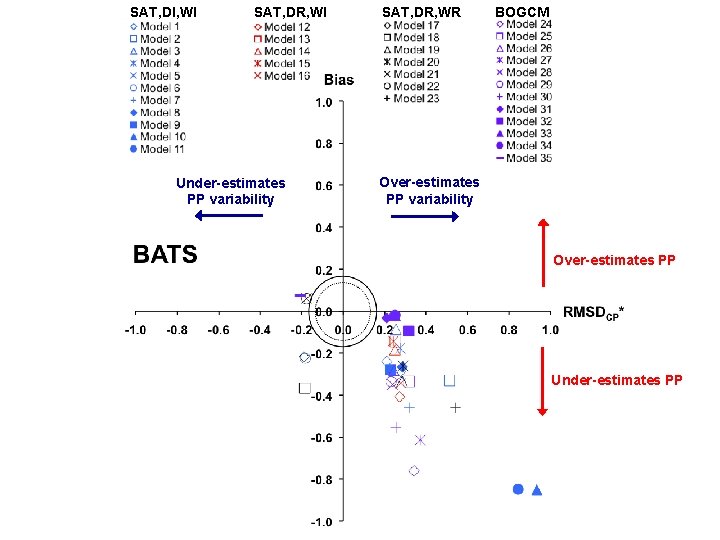
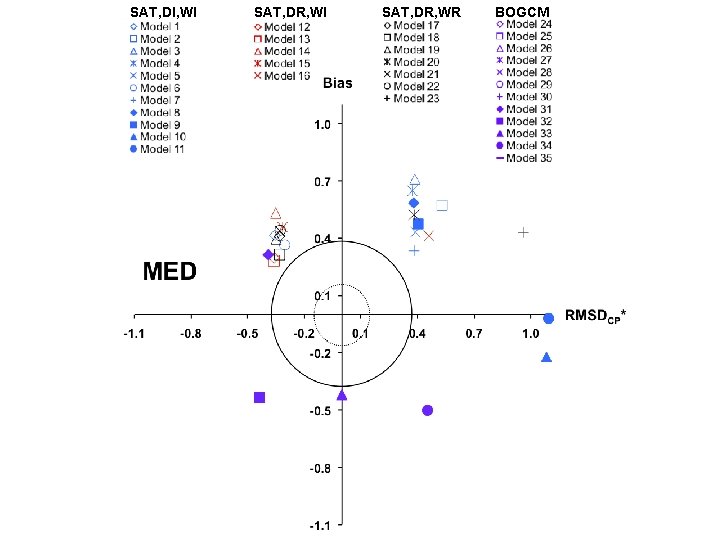
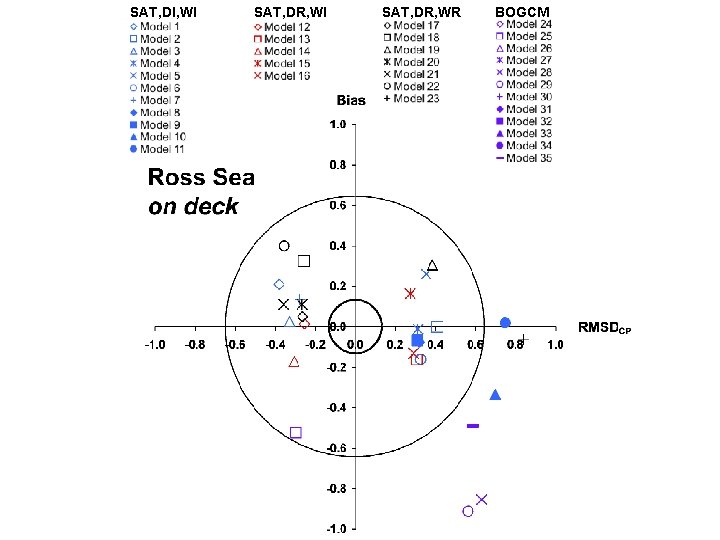
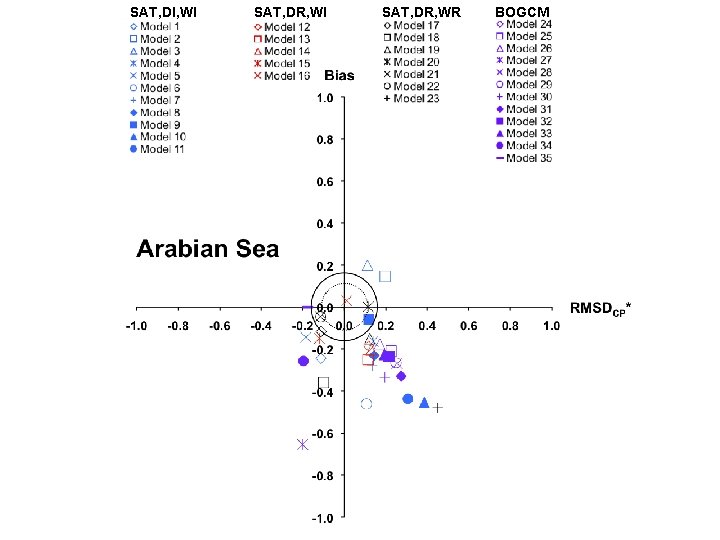
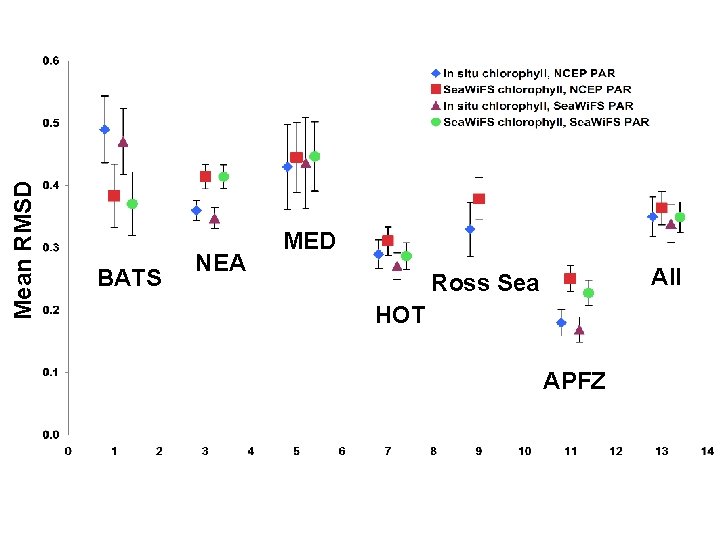
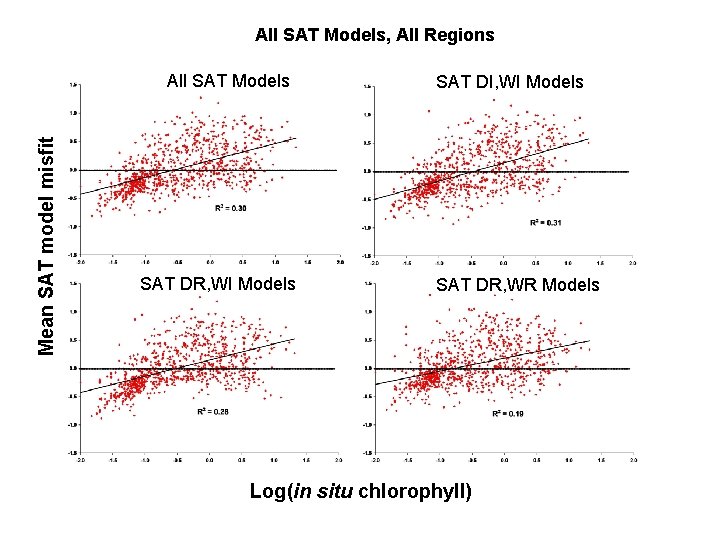
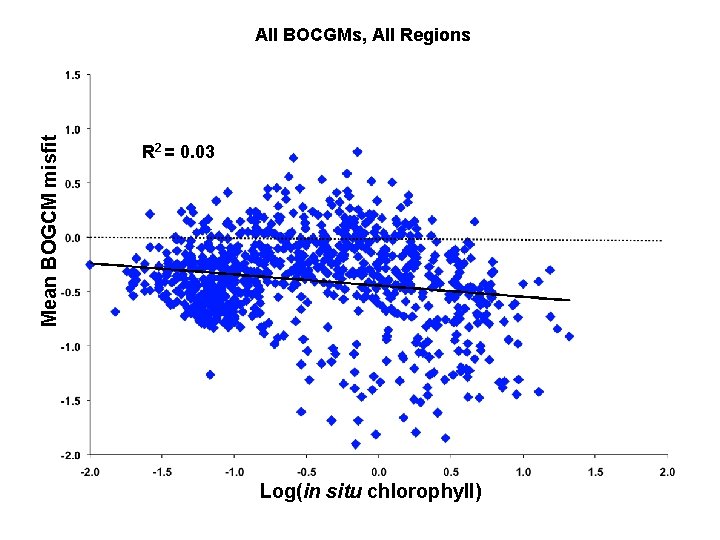
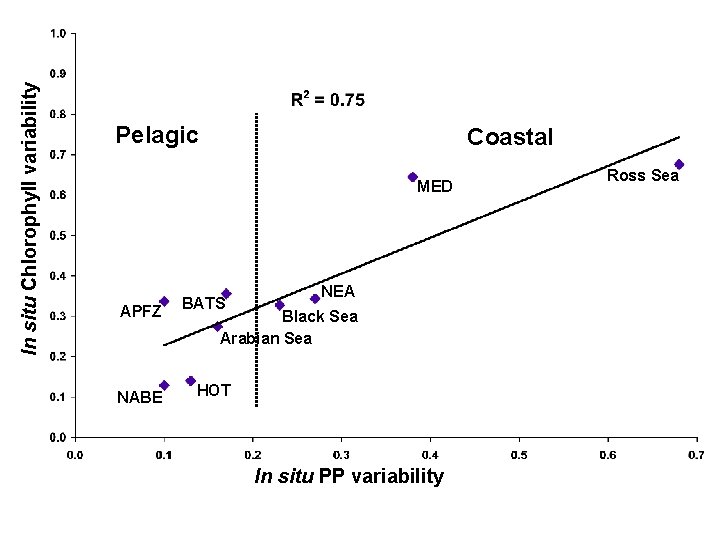
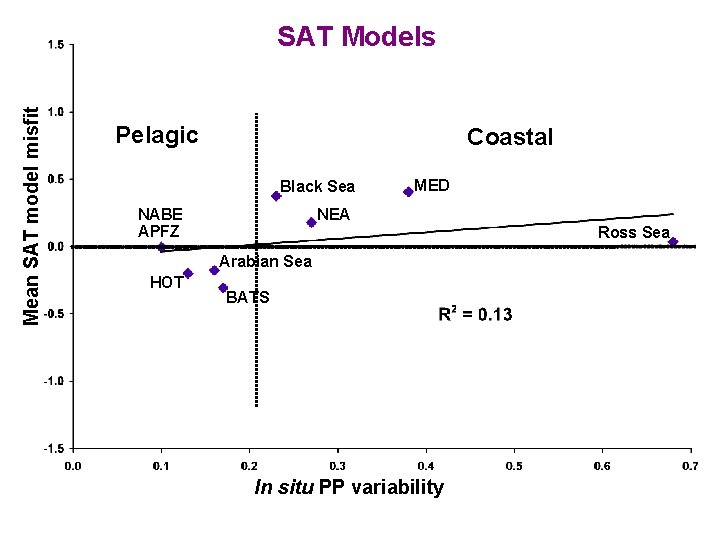
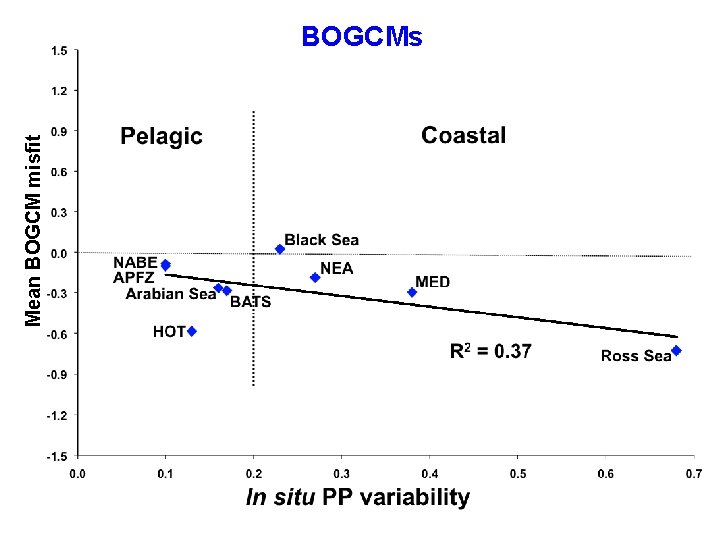
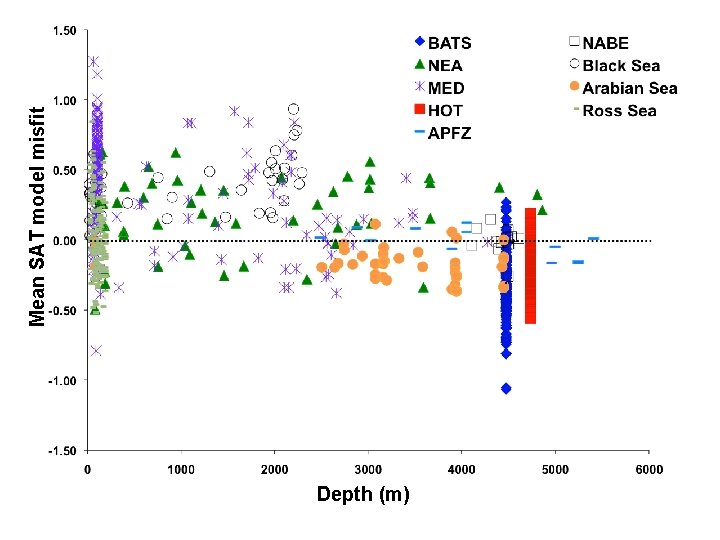
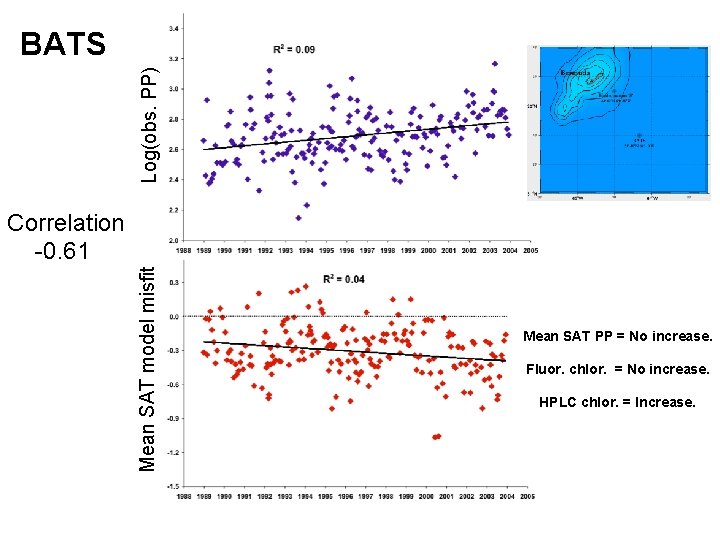
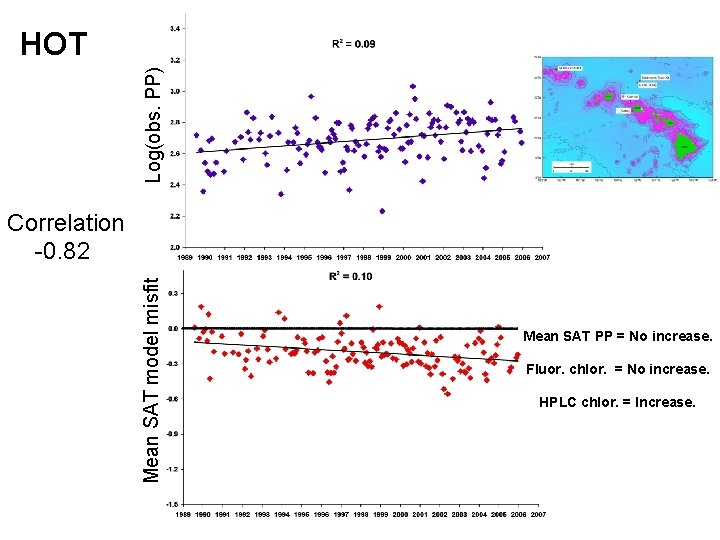
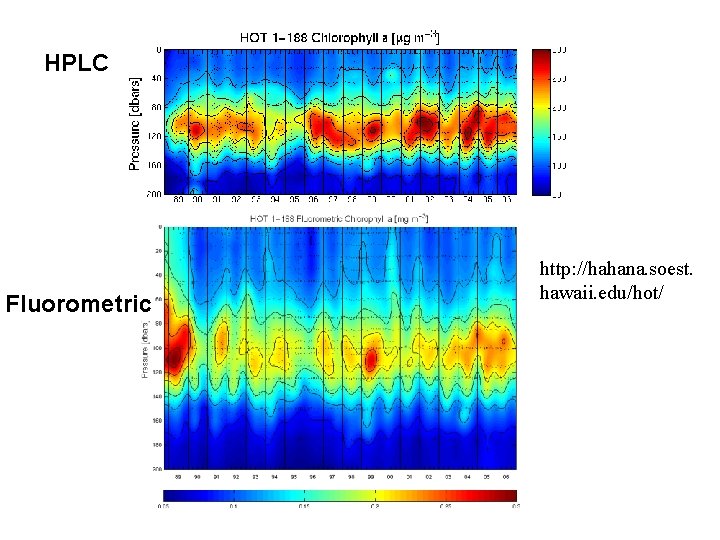
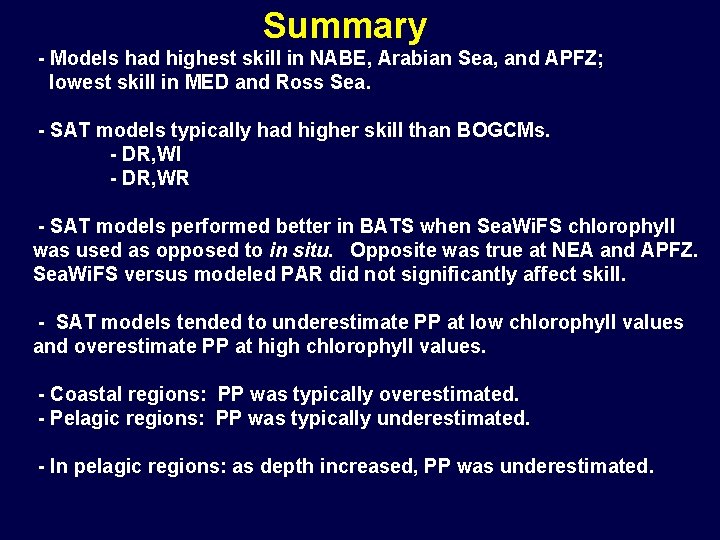
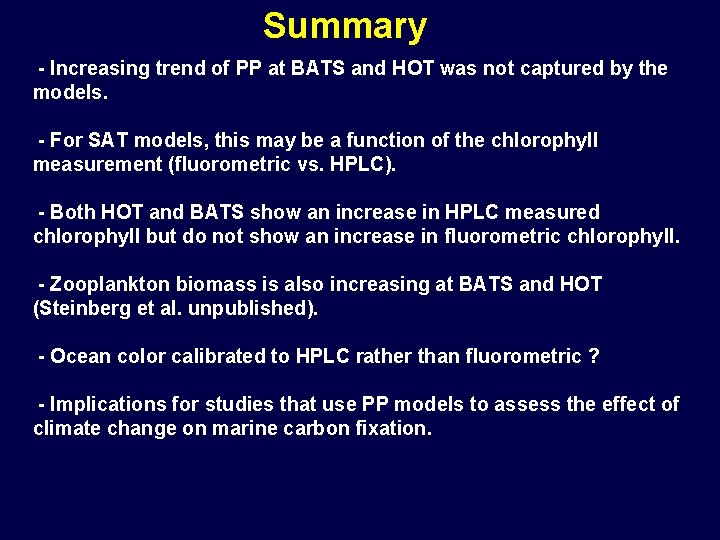
- Slides: 29
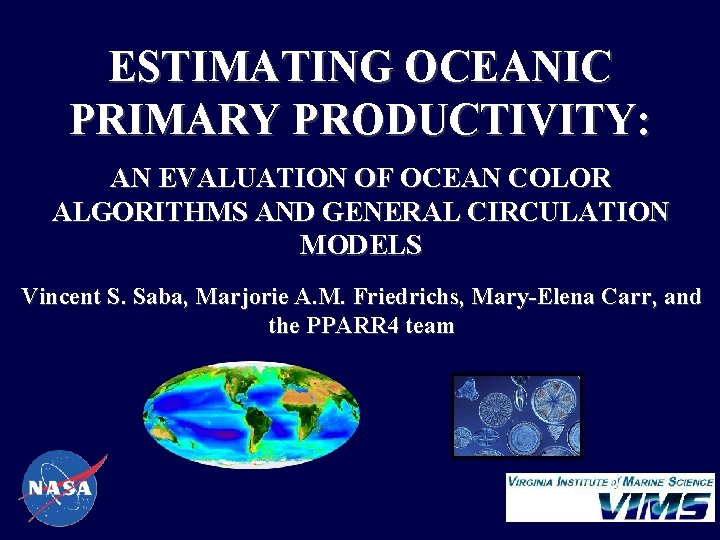
ESTIMATING OCEANIC PRIMARY PRODUCTIVITY: AN EVALUATION OF OCEAN COLOR ALGORITHMS AND GENERAL CIRCULATION MODELS Vincent S. Saba, Marjorie A. M. Friedrichs, Mary-Elena Carr, and the PPARR 4 team
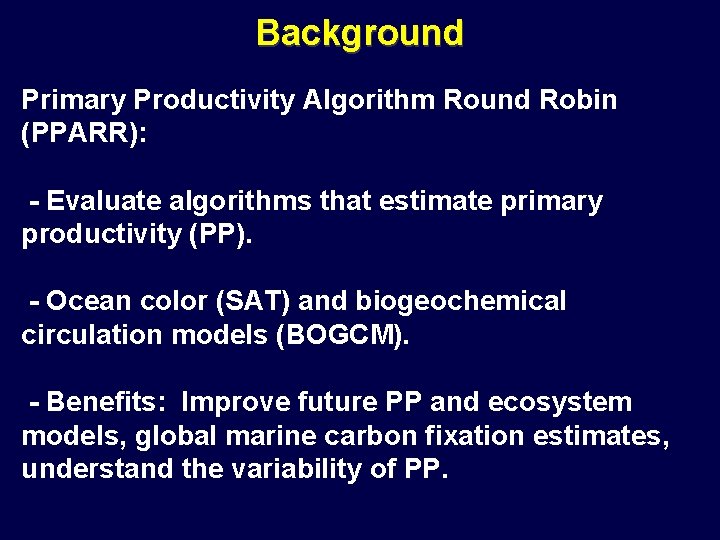
Background Primary Productivity Algorithm Round Robin (PPARR): - Evaluate algorithms that estimate primary productivity (PP). - Ocean color (SAT) and biogeochemical circulation models (BOGCM). - Benefits: Improve future PP and ecosystem models, global marine carbon fixation estimates, understand the variability of PP.
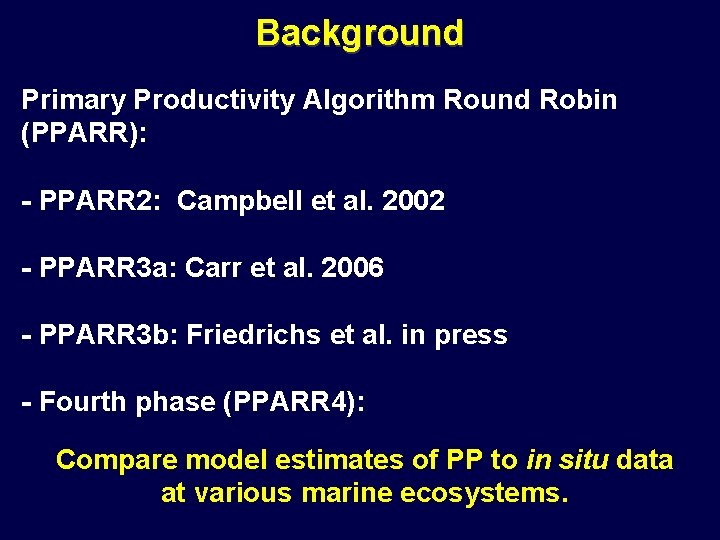
Background Primary Productivity Algorithm Round Robin (PPARR): - PPARR 2: Campbell et al. 2002 - PPARR 3 a: Carr et al. 2006 - PPARR 3 b: Friedrichs et al. in press - Fourth phase (PPARR 4): Compare model estimates of PP to in situ data at various marine ecosystems.
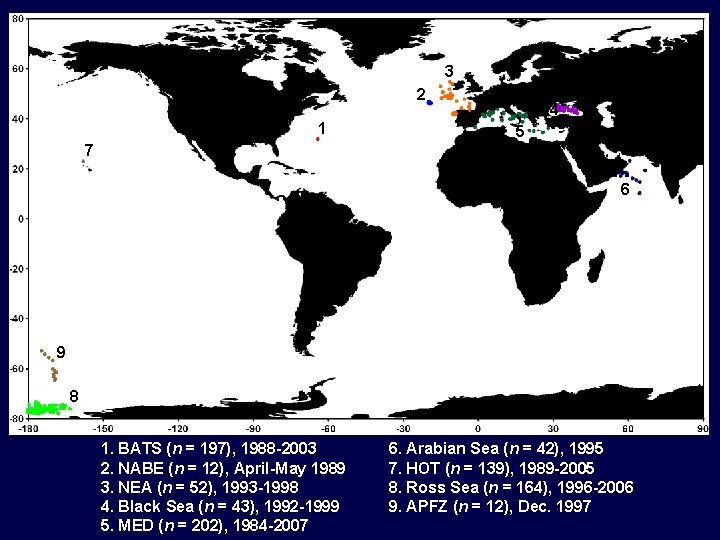
3 2 1 4 5 7 6 9 8 1. BATS (n = 197), 1988 -2003 2. NABE (n = 12), April-May 1989 3. NEA (n = 52), 1993 -1998 4. Black Sea (n = 43), 1992 -1999 5. MED (n = 202), 1984 -2007 6. Arabian Sea (n = 42), 1995 7. HOT (n = 139), 1989 -2005 8. Ross Sea (n = 164), 1996 -2006 9. APFZ (n = 12), Dec. 1997
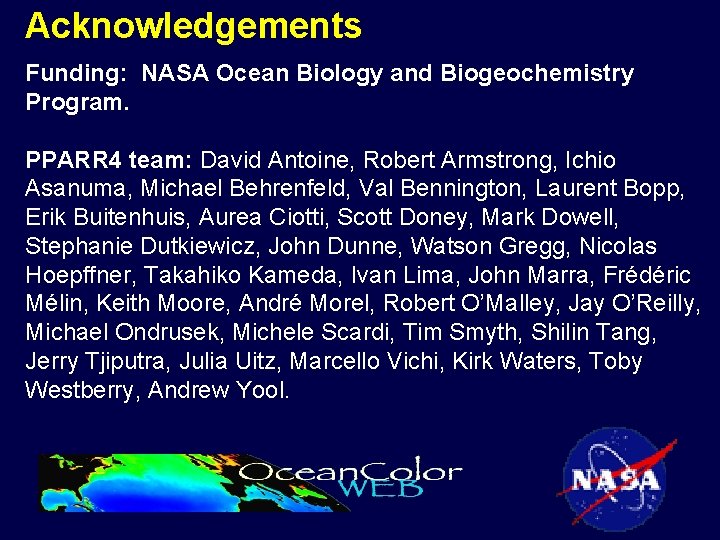
Acknowledgements Funding: NASA Ocean Biology and Biogeochemistry Program. PPARR 4 team: David Antoine, Robert Armstrong, Ichio Asanuma, Michael Behrenfeld, Val Bennington, Laurent Bopp, Erik Buitenhuis, Aurea Ciotti, Scott Doney, Mark Dowell, Stephanie Dutkiewicz, John Dunne, Watson Gregg, Nicolas Hoepffner, Takahiko Kameda, Ivan Lima, John Marra, Frédéric Mélin, Keith Moore, André Morel, Robert O’Malley, Jay O’Reilly, Michael Ondrusek, Michele Scardi, Tim Smyth, Shilin Tang, Jerry Tjiputra, Julia Uitz, Marcello Vichi, Kirk Waters, Toby Westberry, Andrew Yool.
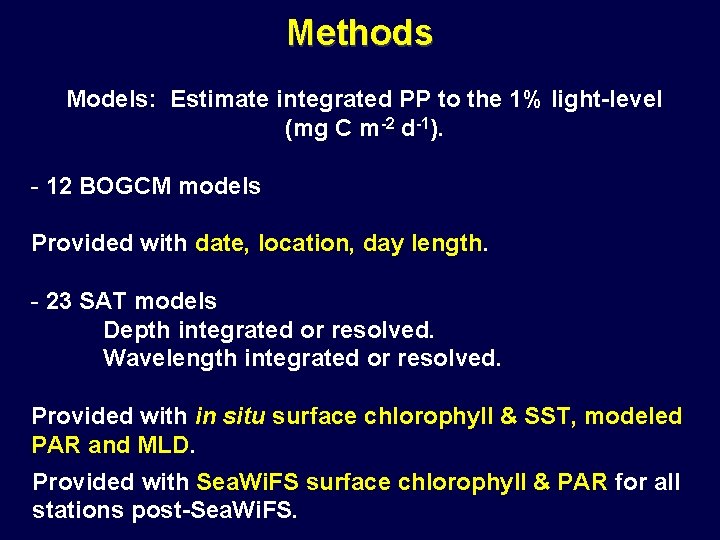
Methods Models: Estimate integrated PP to the 1% light-level (mg C m-2 d-1). - 12 BOGCM models Provided with date, location, day length. - 23 SAT models Depth integrated or resolved. Wavelength integrated or resolved. Provided with in situ surface chlorophyll & SST, modeled PAR and MLD. Provided with Sea. Wi. FS surface chlorophyll & PAR for all stations post-Sea. Wi. FS.
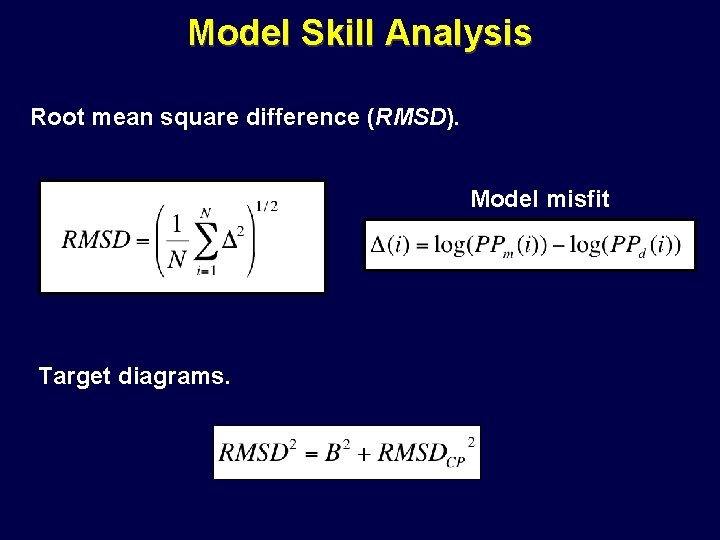
Model Skill Analysis Root mean square difference (RMSD). Model misfit Target diagrams.
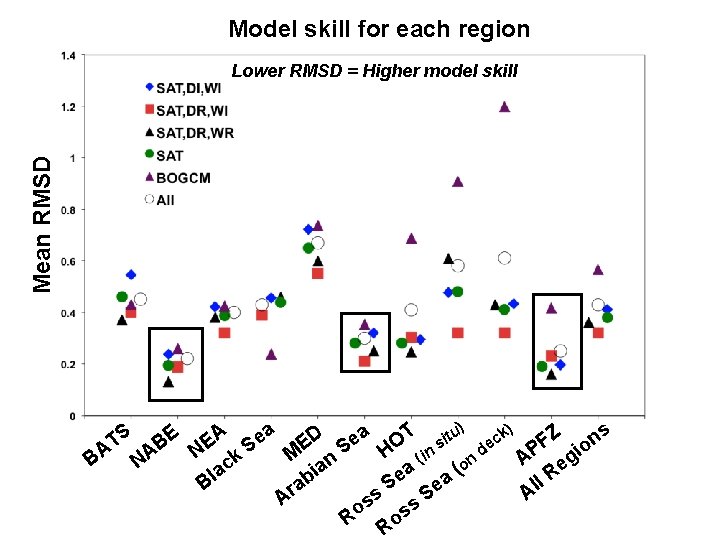
Model skill for each region Mean RMSD Lower RMSD = Higher model skill B S T A NA BE s a k) a OT itu) Z D c e n e F s e E S d P gio N k. S H (in M n A n o e a a ( i ac R l e a l B S l ab e r A s S A s s Ro os R EA
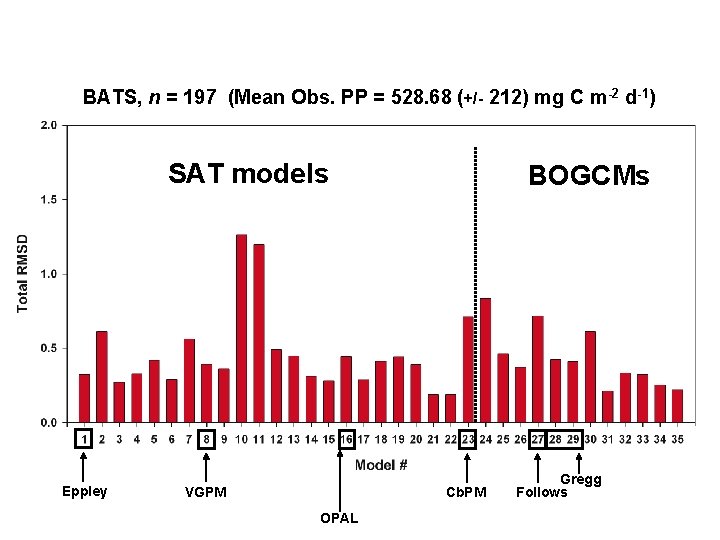
BATS, n = 197 (Mean Obs. PP = 528. 68 (+/- 212) mg C m-2 d-1) SAT models Eppley VGPM BOGCMs Cb. PM OPAL Gregg Follows
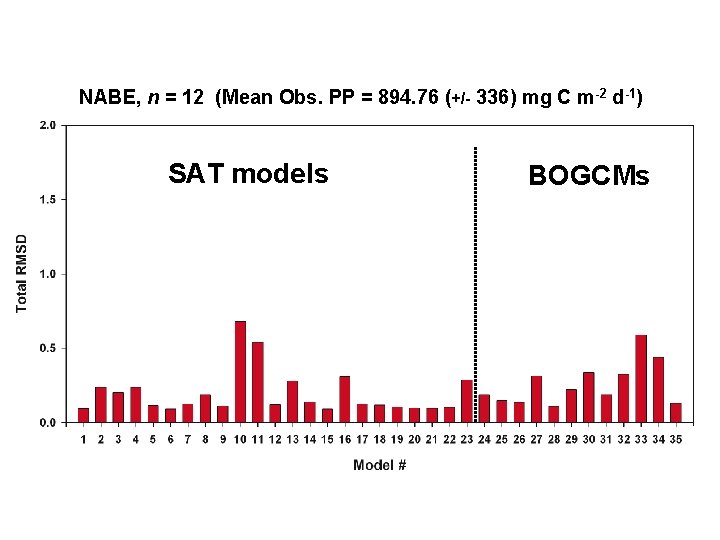
NABE, n = 12 (Mean Obs. PP = 894. 76 (+/- 336) mg C m-2 d-1) SAT models BOGCMs
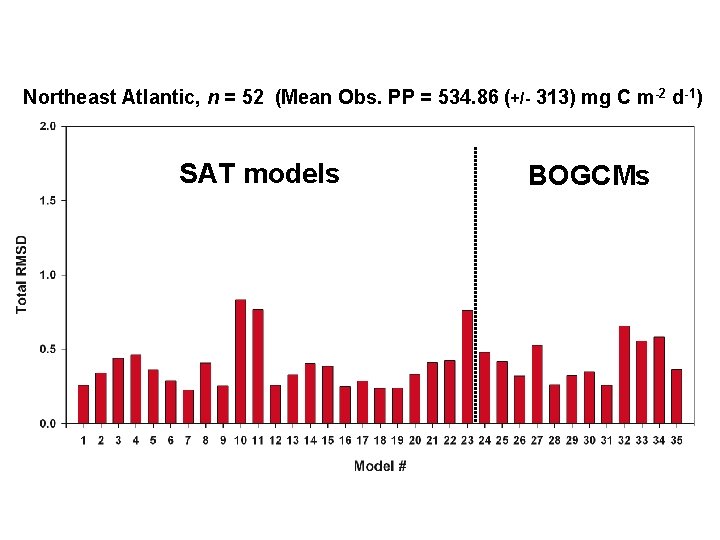
Northeast Atlantic, n = 52 (Mean Obs. PP = 534. 86 (+/- 313) mg C m-2 d-1) SAT models BOGCMs
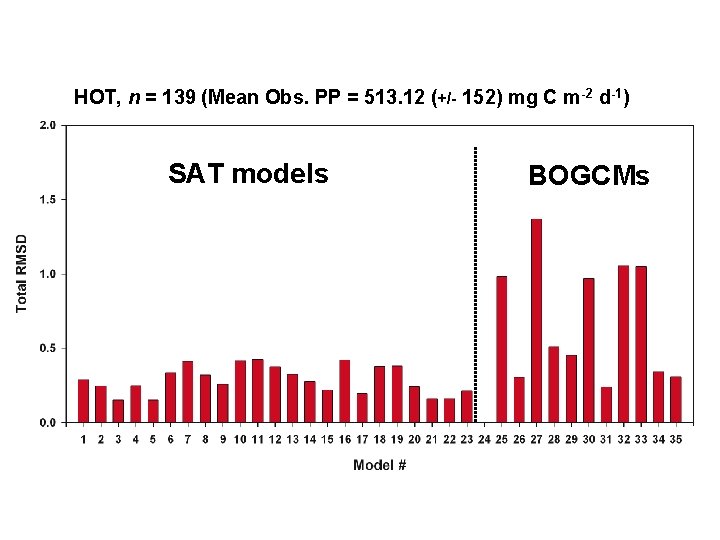
HOT, n = 139 (Mean Obs. PP = 513. 12 (+/- 152) mg C m-2 d-1) SAT models BOGCMs
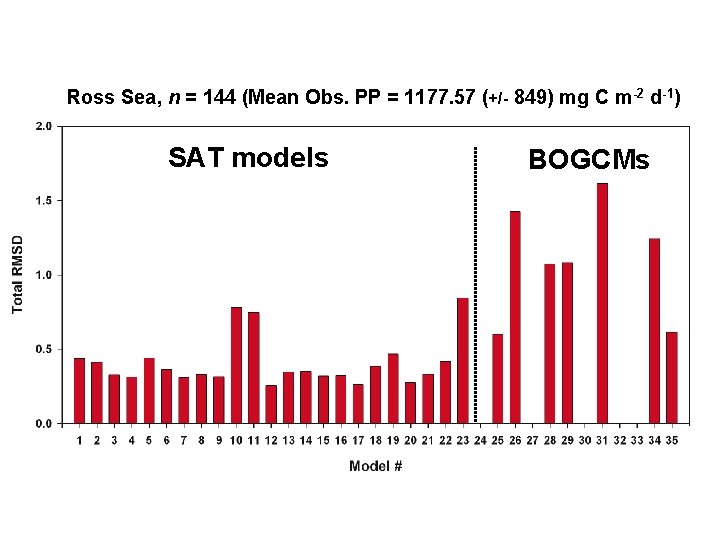
Ross Sea, n = 144 (Mean Obs. PP = 1177. 57 (+/- 849) mg C m-2 d-1) SAT models BOGCMs
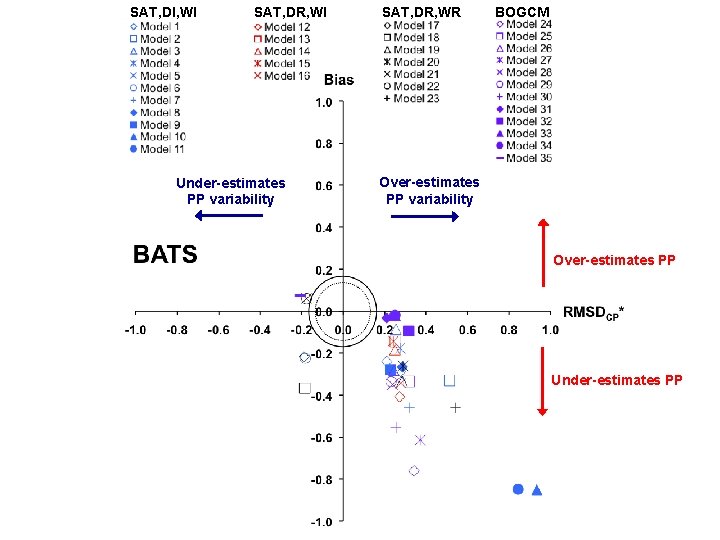
SAT, DI, WI SAT, DR, WI Under-estimates PP variability SAT, DR, WR BOGCM Over-estimates PP variability Over-estimates PP Under-estimates PP
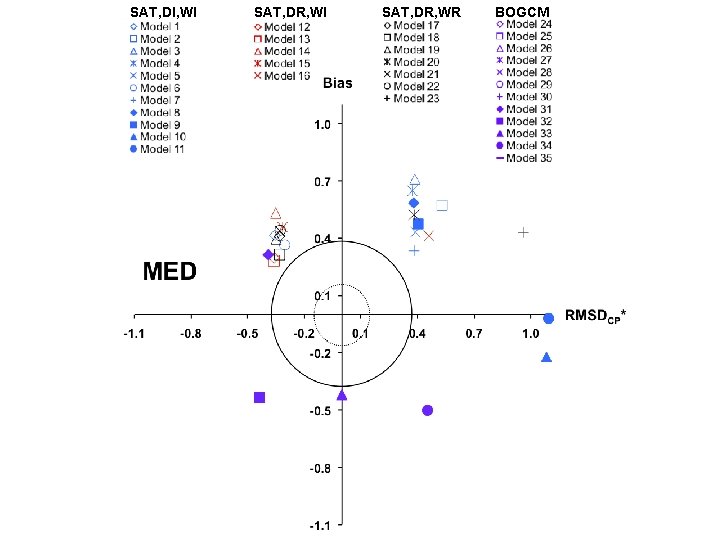
SAT, DI, WI SAT, DR, WR BOGCM
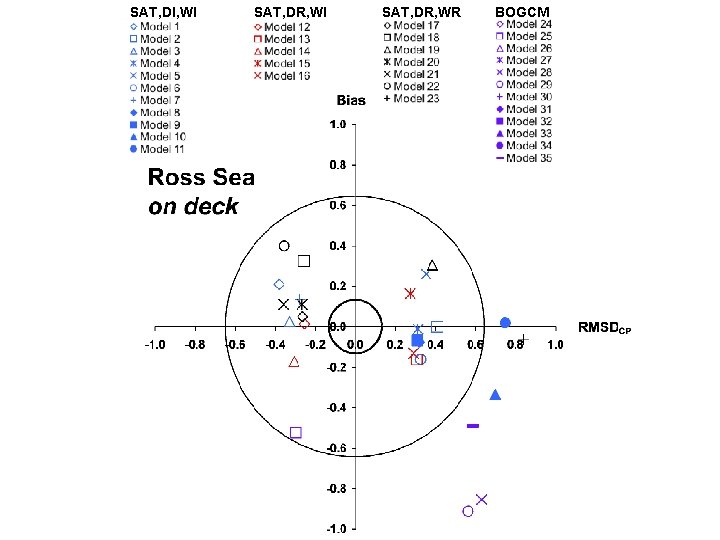
SAT, DI, WI SAT, DR, WR BOGCM
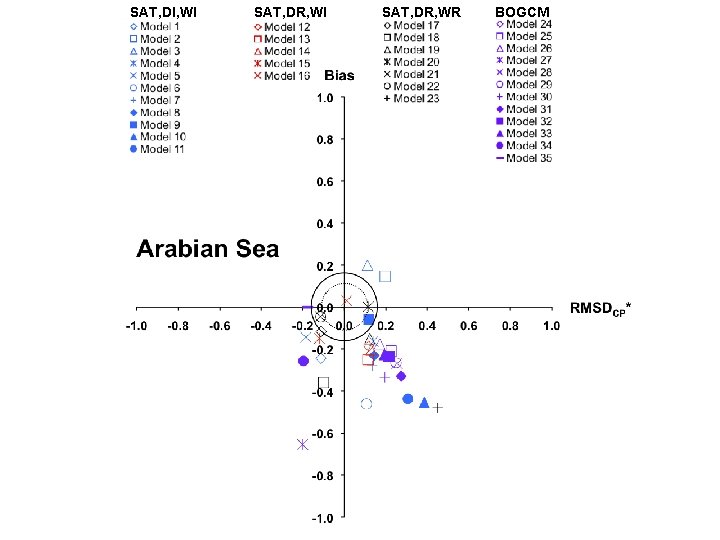
SAT, DI, WI SAT, DR, WR BOGCM
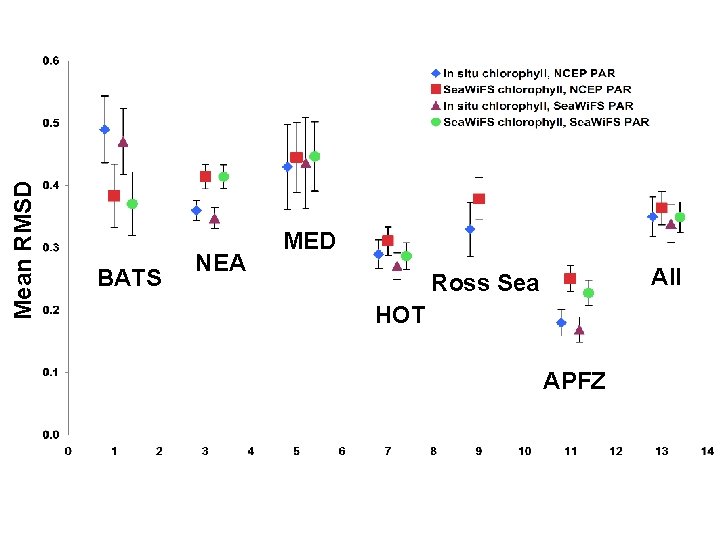
Mean RMSD BATS NEA MED All Ross Sea HOT APFZ
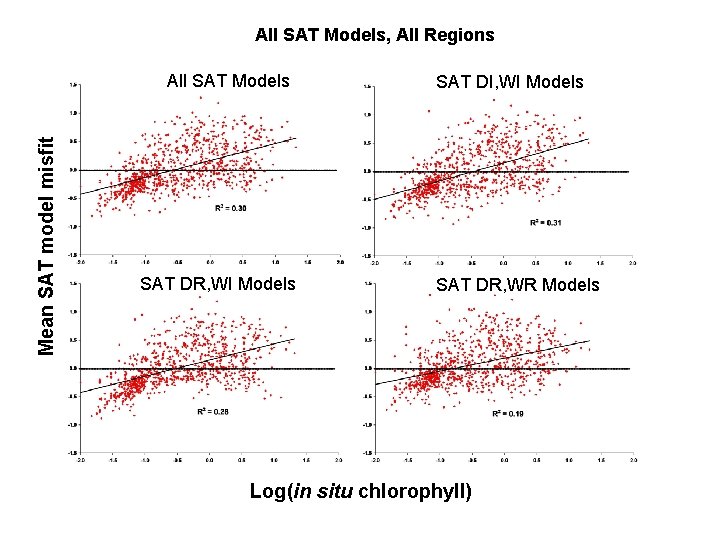
All SAT Models, All Regions Mean SAT model misfit All SAT Models SAT DR, WI Models SAT DI, WI Models SAT DR, WR Models Log(in situ chlorophyll)
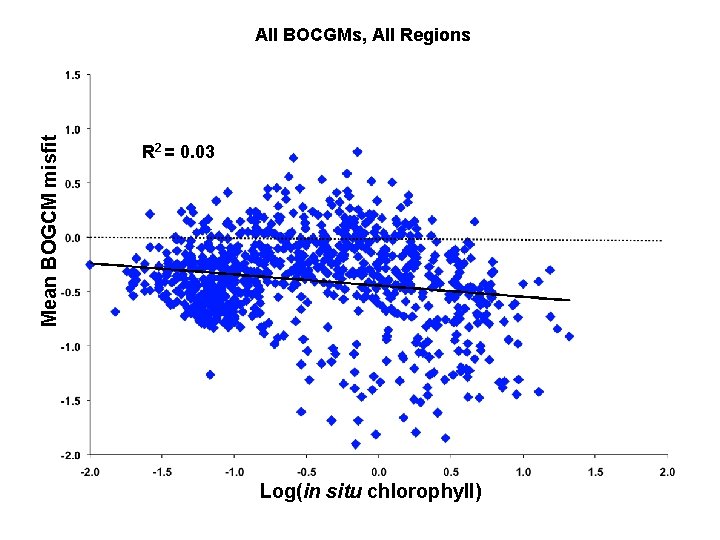
Mean BOGCM misfit All BOCGMs, All Regions R 2 = 0. 03 Log(in situ chlorophyll)
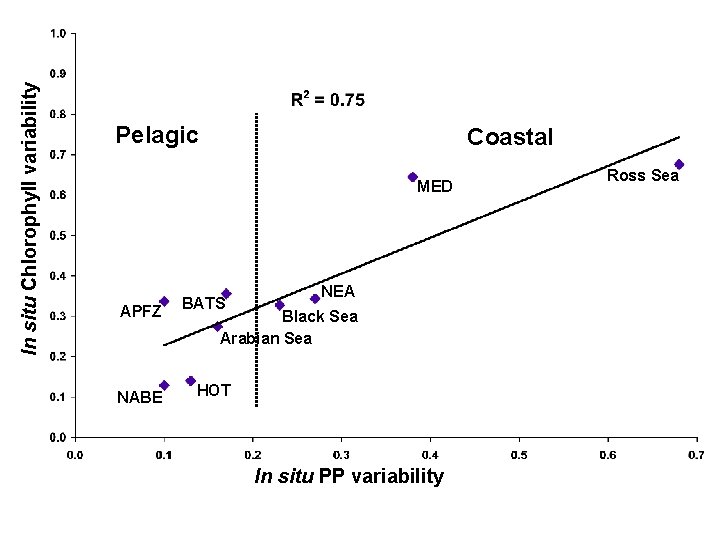
In situ Chlorophyll variability Pelagic Coastal MED APFZ NABE BATS NEA Black Sea Arabian Sea HOT In situ PP variability Ross Sea
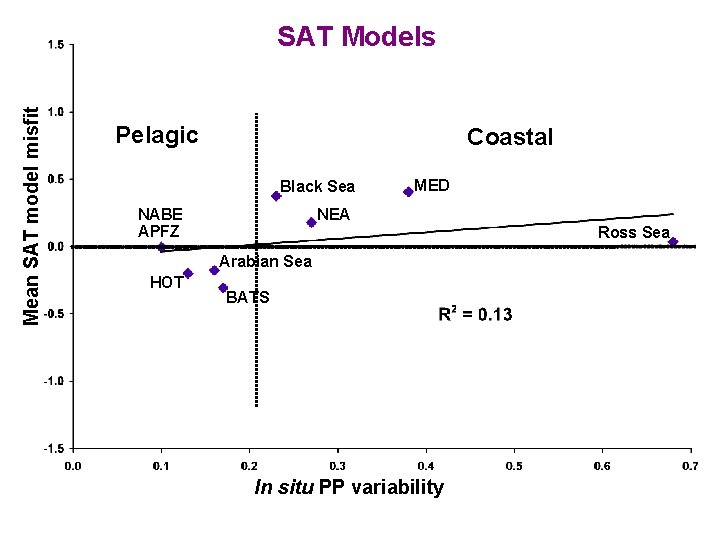
Mean SAT model misfit SAT Models Pelagic Coastal Black Sea MED NEA NABE APFZ Ross Sea Arabian Sea HOT BATS In situ PP variability
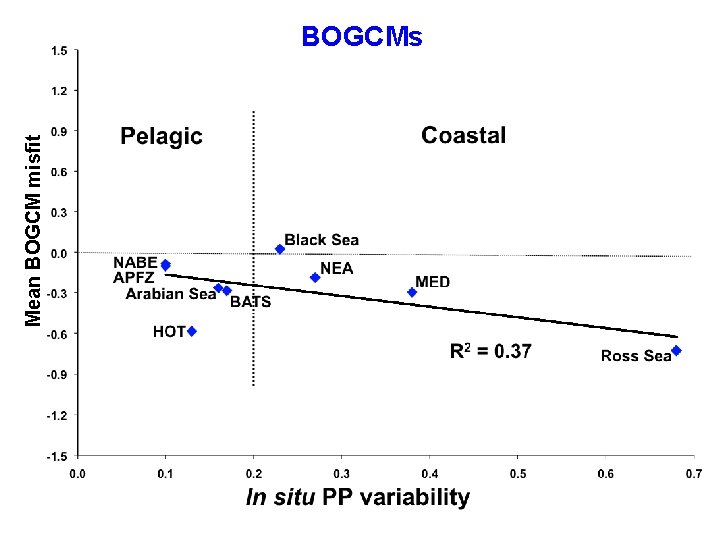
Mean BOGCM misfit BOGCMs
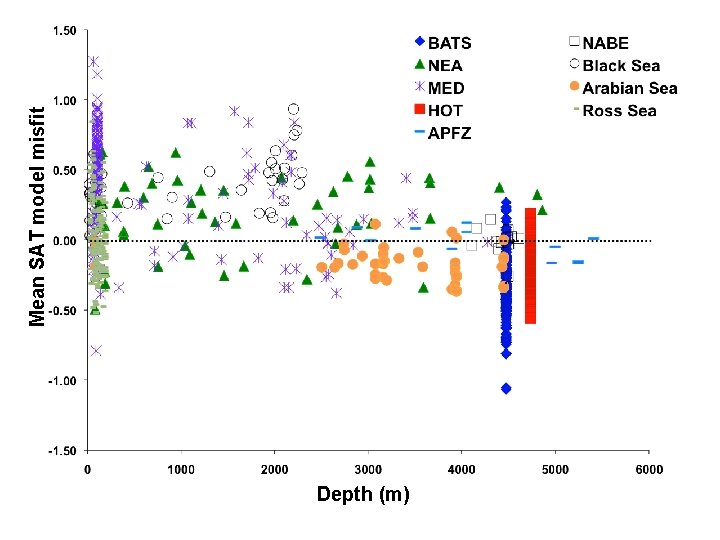
Depth (m) Mean SAT model misfit
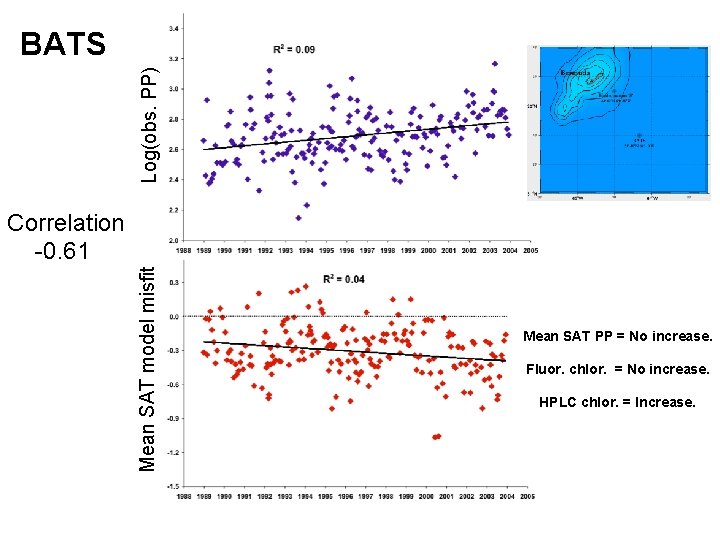
Log(obs. PP) BATS Mean SAT model misfit Correlation -0. 61 Mean SAT PP = No increase. Fluor. chlor. = No increase. HPLC chlor. = Increase.
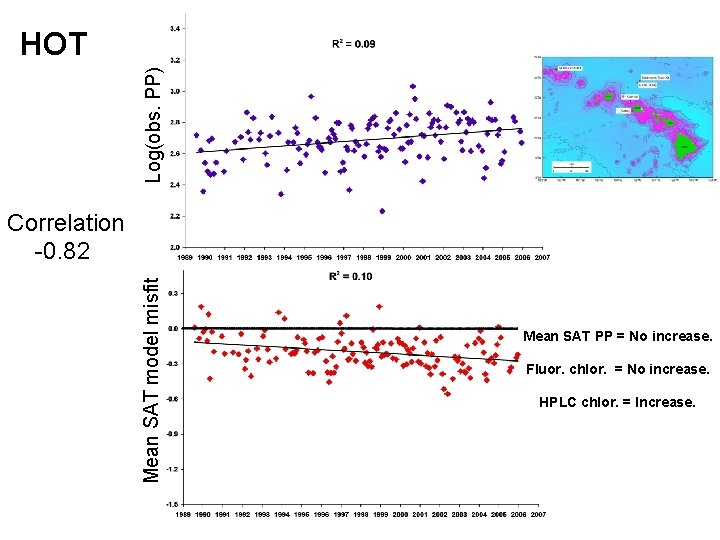
Log(obs. PP) HOT Mean SAT model misfit Correlation -0. 82 Mean SAT PP = No increase. Fluor. chlor. = No increase. HPLC chlor. = Increase.
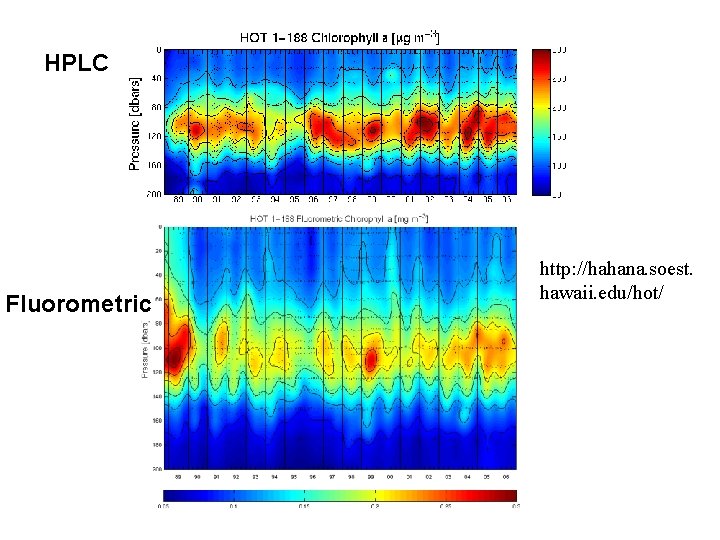
HPLC Fluorometric http: //hahana. soest. hawaii. edu/hot/
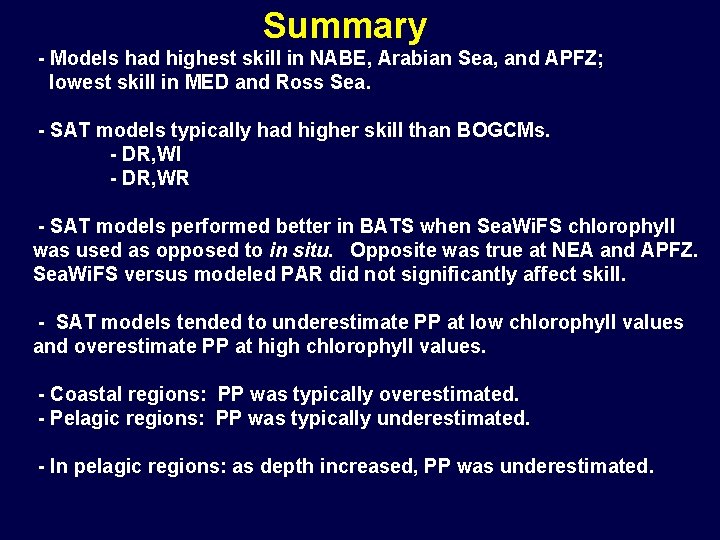
Summary - Models had highest skill in NABE, Arabian Sea, and APFZ; lowest skill in MED and Ross Sea. - SAT models typically had higher skill than BOGCMs. - DR, WI - DR, WR - SAT models performed better in BATS when Sea. Wi. FS chlorophyll was used as opposed to in situ. Opposite was true at NEA and APFZ. Sea. Wi. FS versus modeled PAR did not significantly affect skill. - SAT models tended to underestimate PP at low chlorophyll values and overestimate PP at high chlorophyll values. - Coastal regions: PP was typically overestimated. - Pelagic regions: PP was typically underestimated. - In pelagic regions: as depth increased, PP was underestimated.
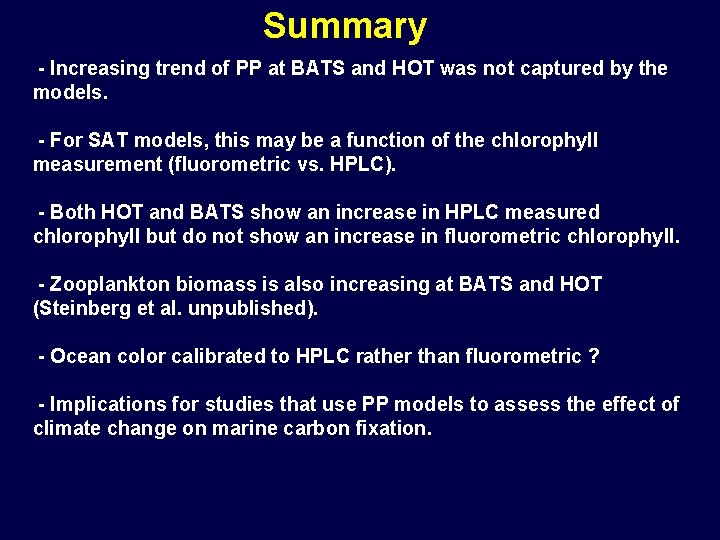
Summary - Increasing trend of PP at BATS and HOT was not captured by the models. - For SAT models, this may be a function of the chlorophyll measurement (fluorometric vs. HPLC). - Both HOT and BATS show an increase in HPLC measured chlorophyll but do not show an increase in fluorometric chlorophyll. - Zooplankton biomass is also increasing at BATS and HOT (Steinberg et al. unpublished). - Ocean color calibrated to HPLC rather than fluorometric ? - Implications for studies that use PP models to assess the effect of climate change on marine carbon fixation.
Ocean to ocean convergent boundary
Convergent continental boundary
Section 15.3 oceanic productivity
Divergent convergent transform
Ocean ocean convergent boundary
Convergent plate boundaries
Ocean ocean convergent boundary
Chapter 15 ocean water and ocean life wordwise answer key
Ice age crack
Red ocean vs blue ocean
Process of primary productivity
Gross primary productivity
Simple ecosystem drawing
Macroalgae are:
Primary producers in the ocean
What is the primary driving force behind surface currents?
Neritic province and oceanic province
What happens when two oceanic plates collide
Continental and oceanic crust
Divergent boundary real life example
Pelagic zones
Oceanic divisions
New york oceanic
Oceanic crust vip #1
Neritic zone climate
A denser oceanic plate collides with a continental plate
Oceanic group of companies
Happens when two oceanic plates collide
The cross section below depicts magnetized oceanic crust
Convergence of two oceanic plates oreo