Demand response algorithms to improve electric power system
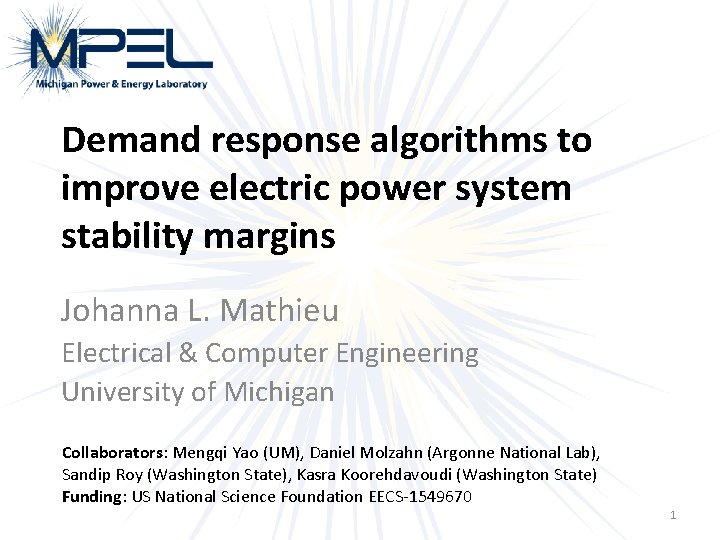
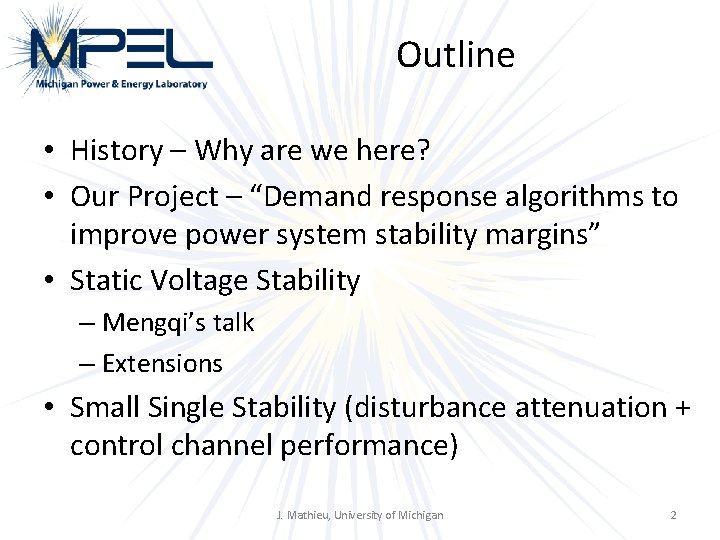
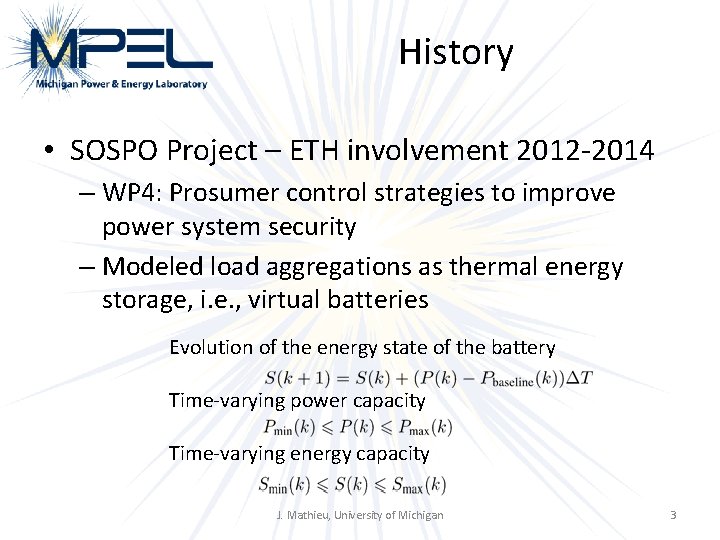
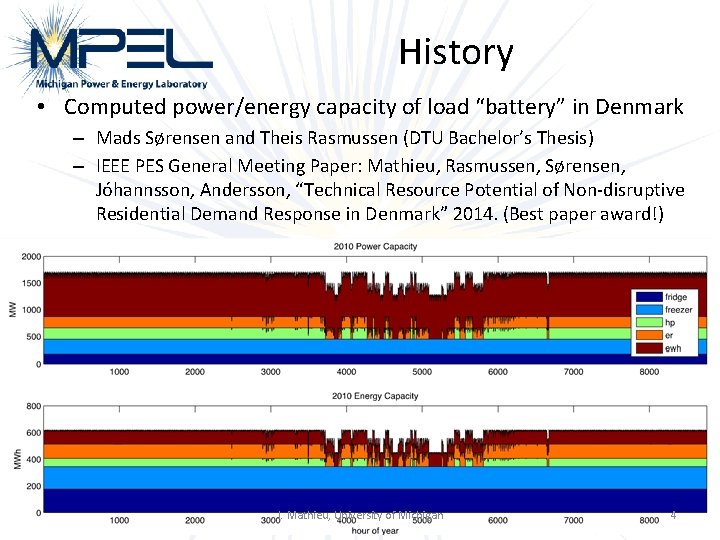
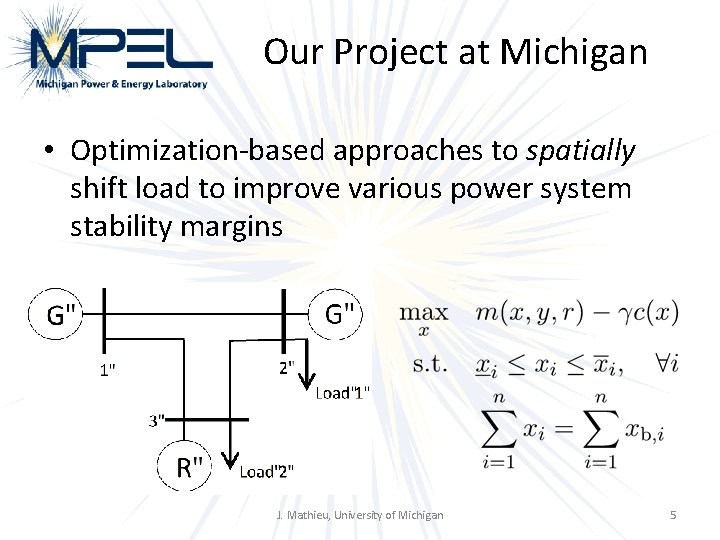
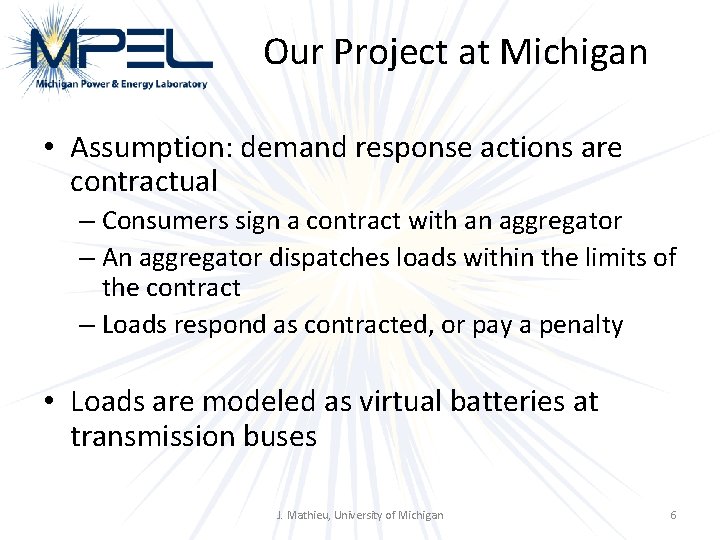
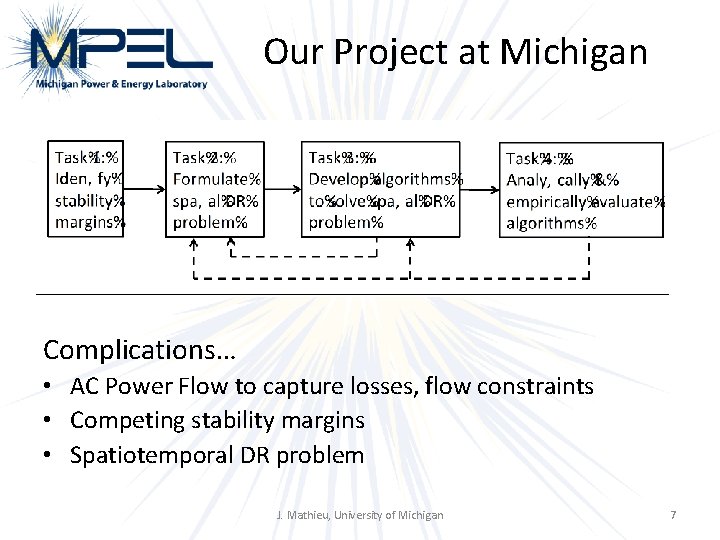
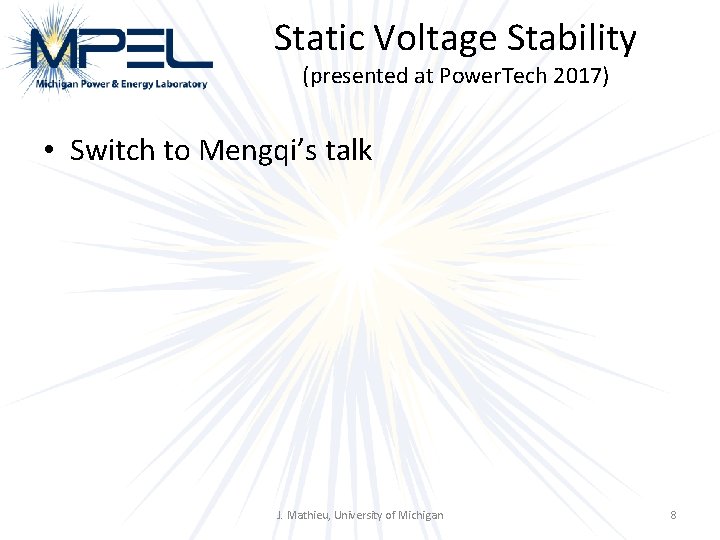
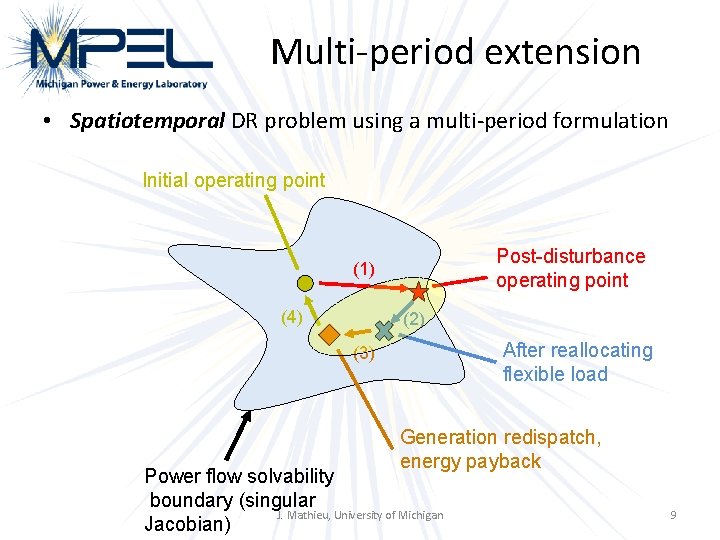
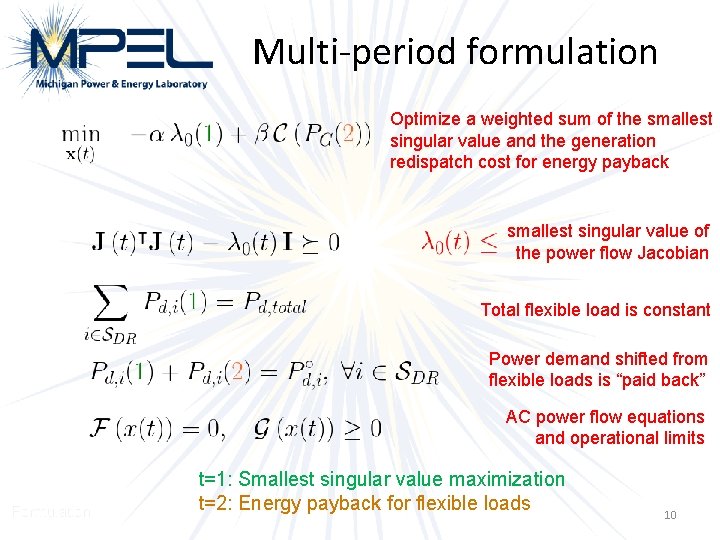
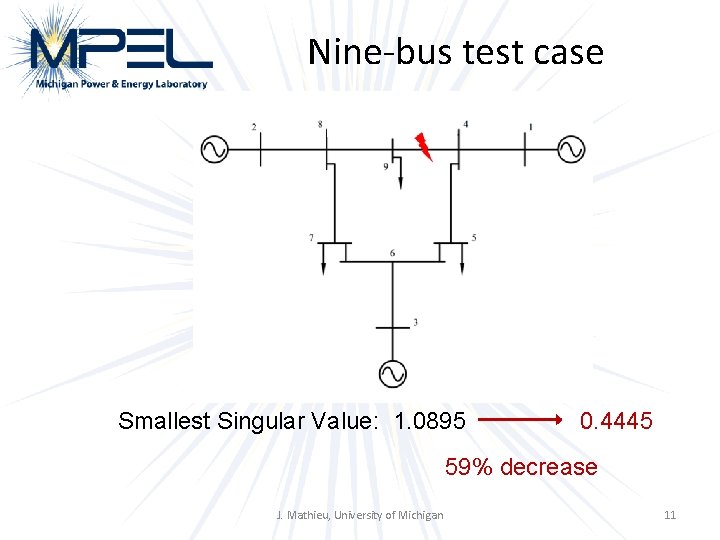
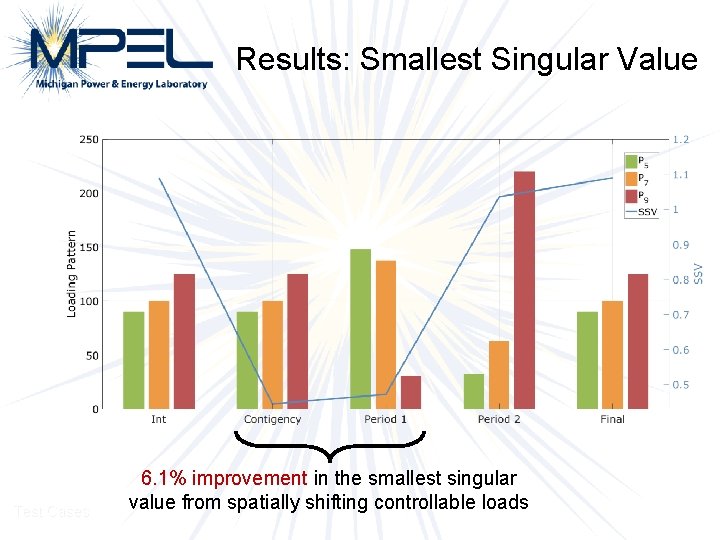
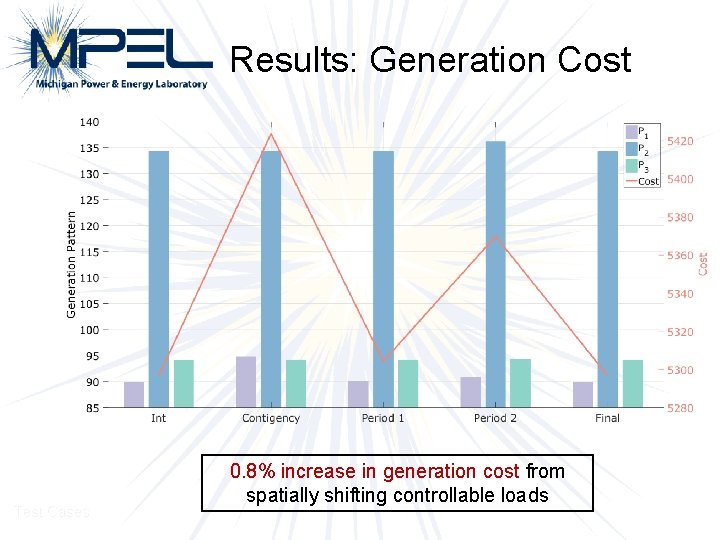
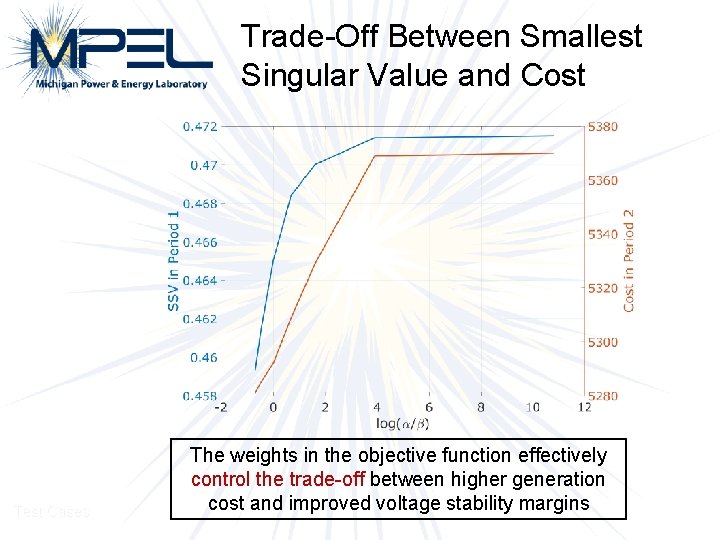
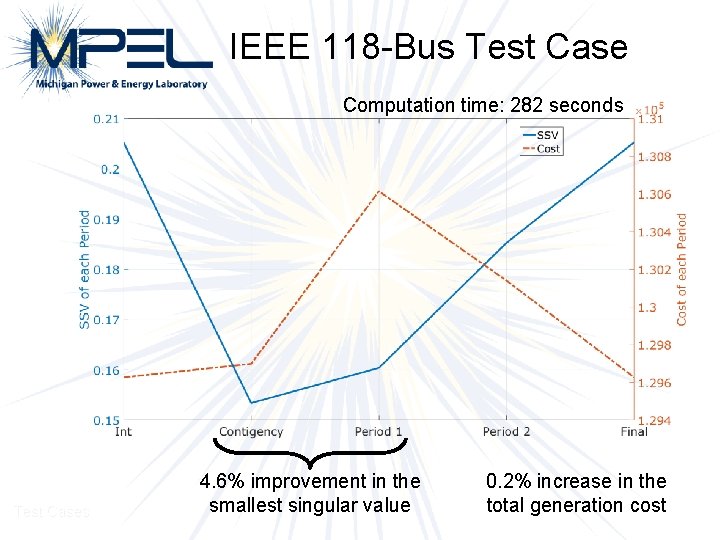
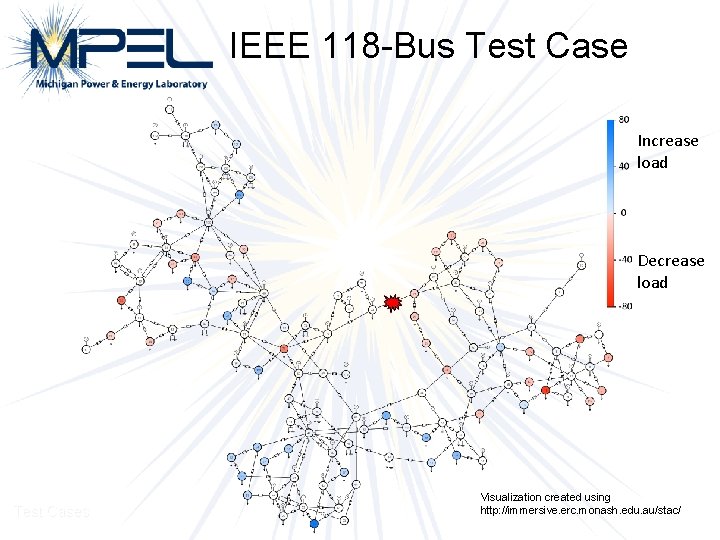
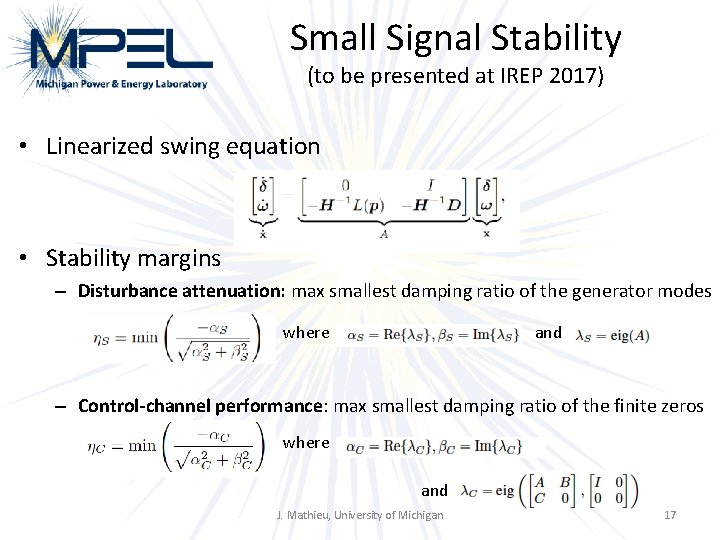
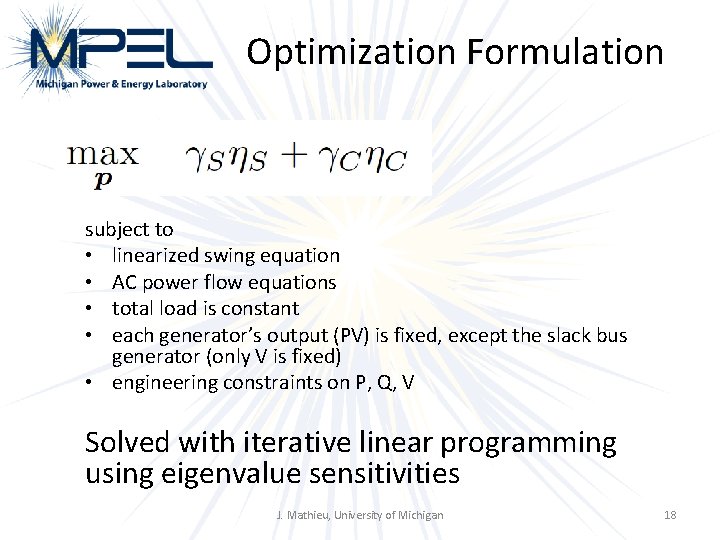
![Case Study • Kundur’s two-area 11 -bus test system [Kundur et al. 1994] • Case Study • Kundur’s two-area 11 -bus test system [Kundur et al. 1994] •](https://slidetodoc.com/presentation_image/a34c662c870b1dc254d8845f0787cb51/image-19.jpg)
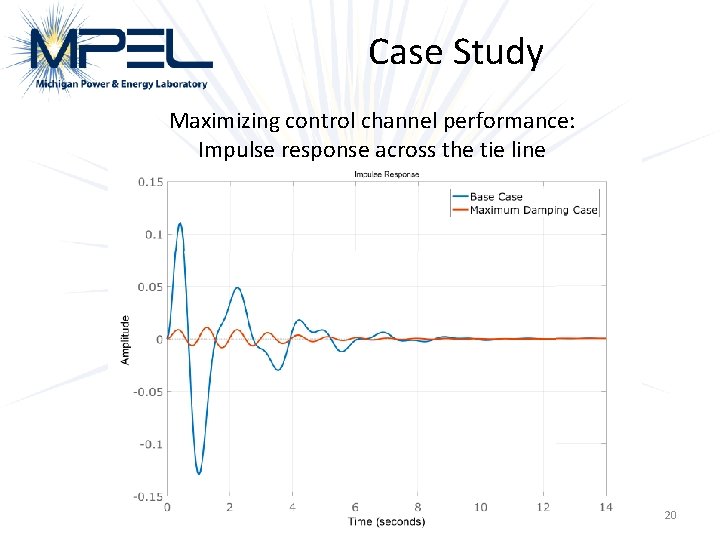
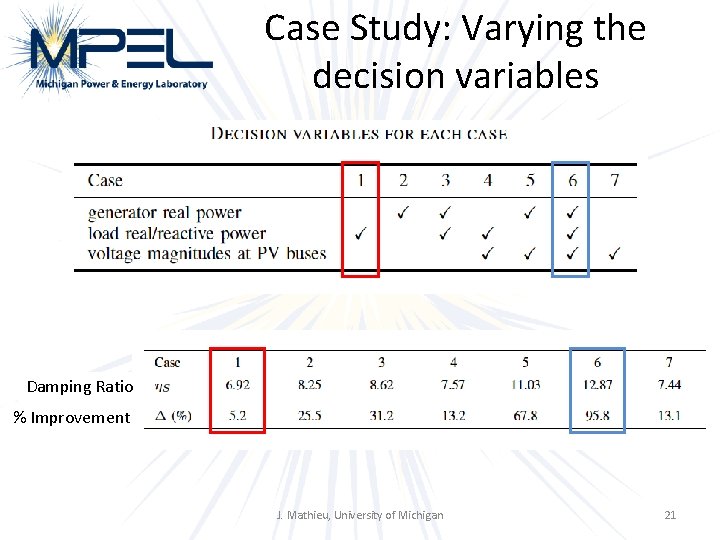
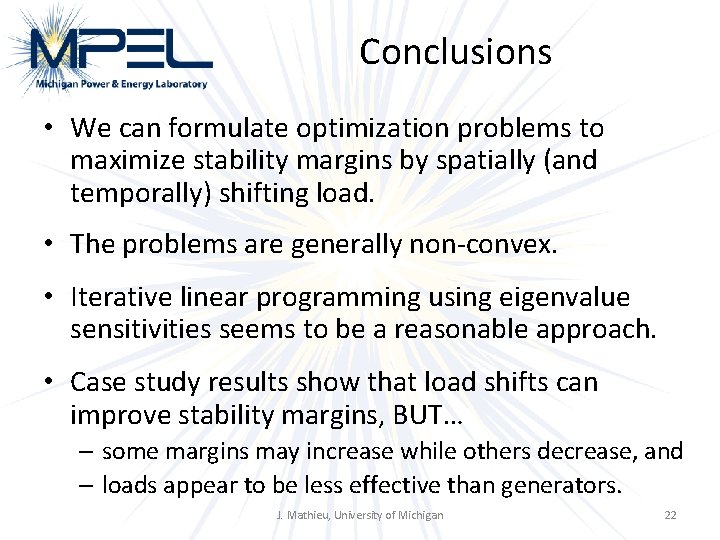
- Slides: 22
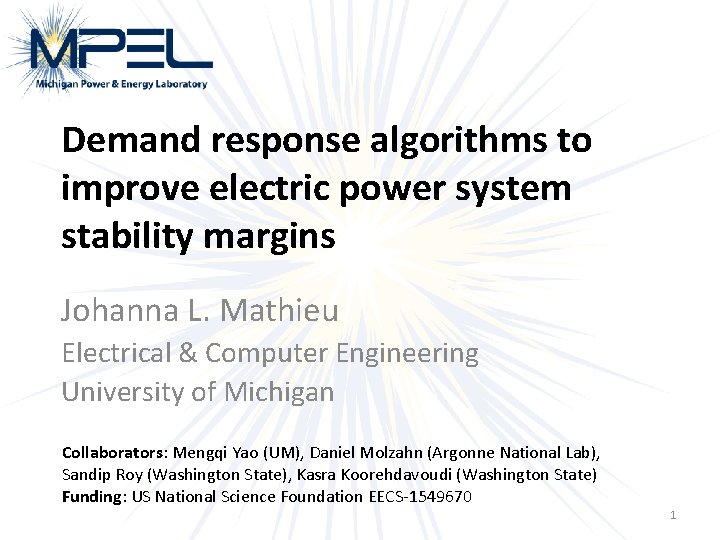
Demand response algorithms to improve electric power system stability margins Johanna L. Mathieu Electrical & Computer Engineering University of Michigan Collaborators: Mengqi Yao (UM), Daniel Molzahn (Argonne National Lab), Sandip Roy (Washington State), Kasra Koorehdavoudi (Washington State) Funding: US National Science Foundation EECS-1549670 1
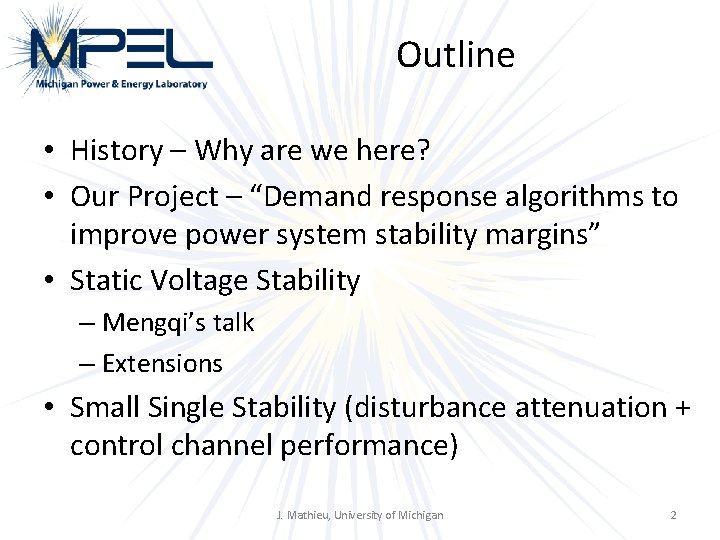
Outline • History – Why are we here? • Our Project – “Demand response algorithms to improve power system stability margins” • Static Voltage Stability – Mengqi’s talk – Extensions • Small Single Stability (disturbance attenuation + control channel performance) J. Mathieu, University of Michigan 2
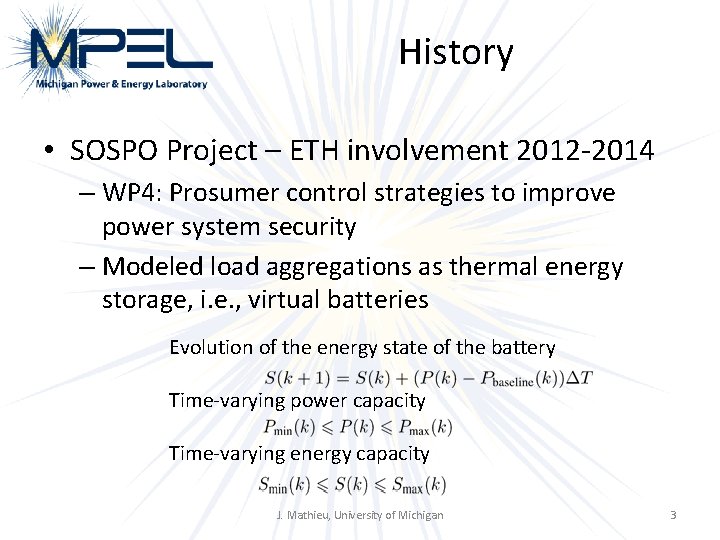
History • SOSPO Project – ETH involvement 2012 -2014 – WP 4: Prosumer control strategies to improve power system security – Modeled load aggregations as thermal energy storage, i. e. , virtual batteries Evolution of the energy state of the battery Time-varying power capacity Time-varying energy capacity J. Mathieu, University of Michigan 3
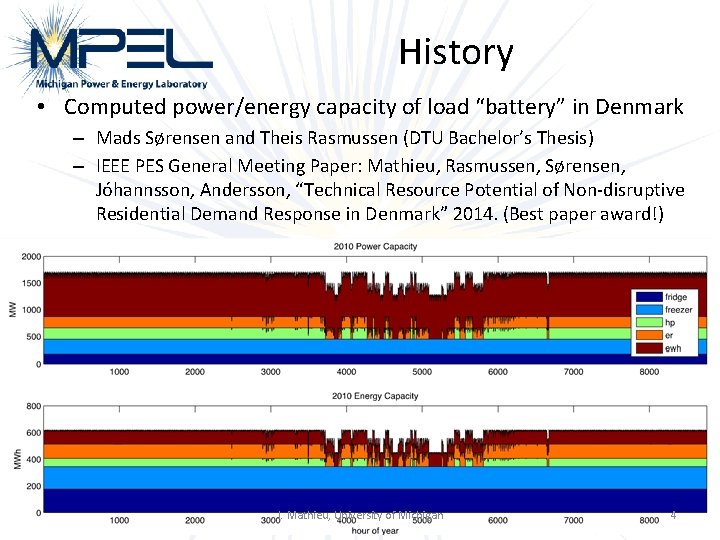
History • Computed power/energy capacity of load “battery” in Denmark – Mads Sørensen and Theis Rasmussen (DTU Bachelor’s Thesis) – IEEE PES General Meeting Paper: Mathieu, Rasmussen, Sørensen, Jóhannsson, Andersson, “Technical Resource Potential of Non-disruptive Residential Demand Response in Denmark” 2014. (Best paper award!) J. Mathieu, University of Michigan 4
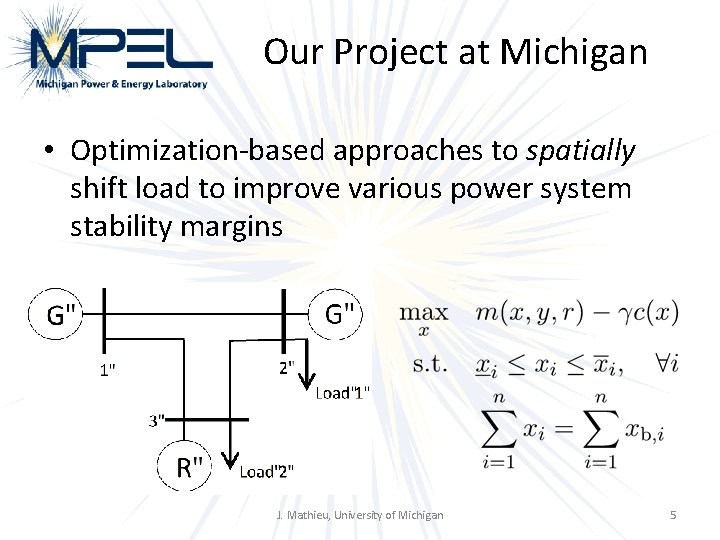
Our Project at Michigan • Optimization-based approaches to spatially shift load to improve various power system stability margins J. Mathieu, University of Michigan 5
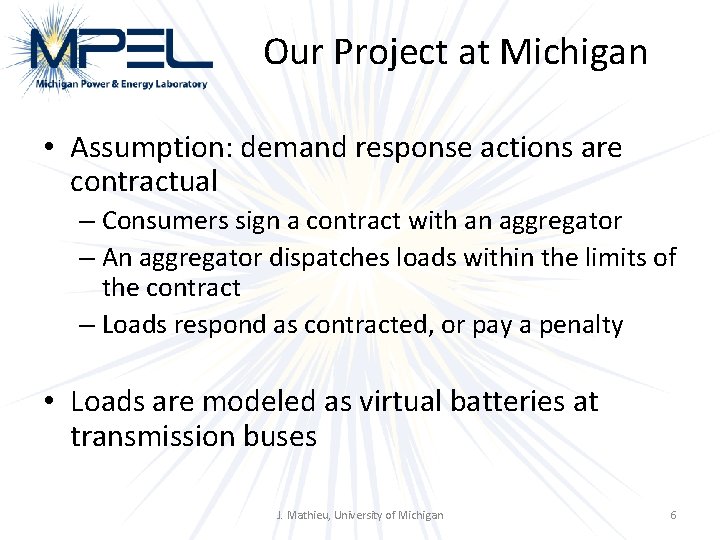
Our Project at Michigan • Assumption: demand response actions are contractual – Consumers sign a contract with an aggregator – An aggregator dispatches loads within the limits of the contract – Loads respond as contracted, or pay a penalty • Loads are modeled as virtual batteries at transmission buses J. Mathieu, University of Michigan 6
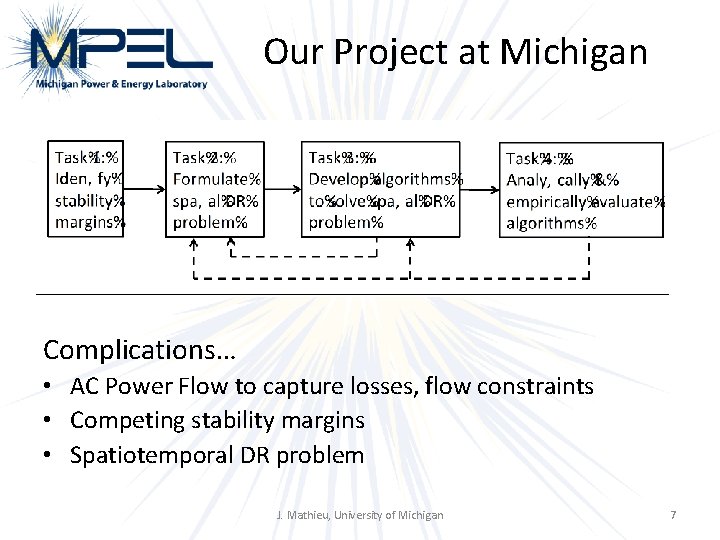
Our Project at Michigan Complications… • AC Power Flow to capture losses, flow constraints • Competing stability margins • Spatiotemporal DR problem J. Mathieu, University of Michigan 7
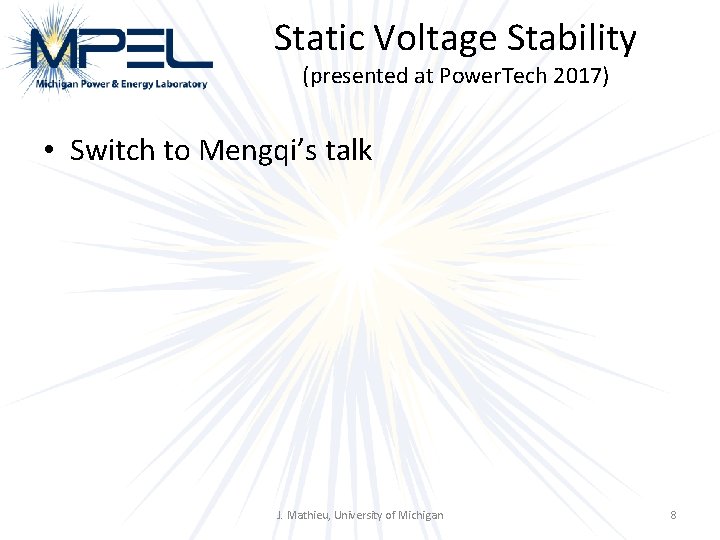
Static Voltage Stability (presented at Power. Tech 2017) • Switch to Mengqi’s talk J. Mathieu, University of Michigan 8
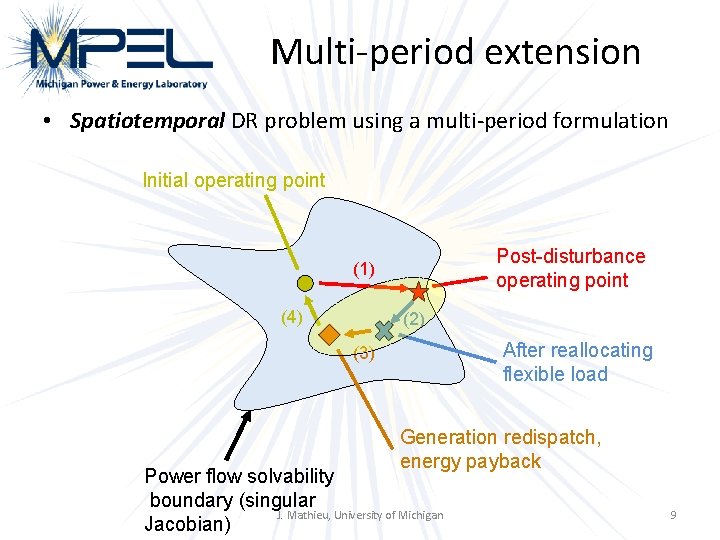
Multi-period extension • Spatiotemporal DR problem using a multi-period formulation Initial operating point Post-disturbance operating point (1) (4) (2) After reallocating flexible load (3) Generation redispatch, energy payback Power flow solvability boundary (singular J. Mathieu, University of Michigan Jacobian) 9
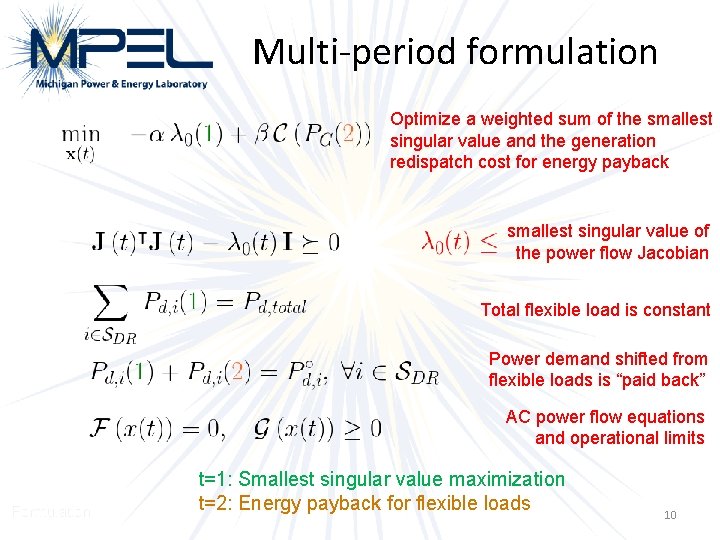
Multi-period formulation Optimize a weighted sum of the smallest singular value and the generation redispatch cost for energy payback smallest singular value of the power flow Jacobian Total flexible load is constant Power demand shifted from flexible loads is “paid back” AC power flow equations and operational limits Formulation t=1: Smallest singular value maximization t=2: Energy payback for flexible loads 10
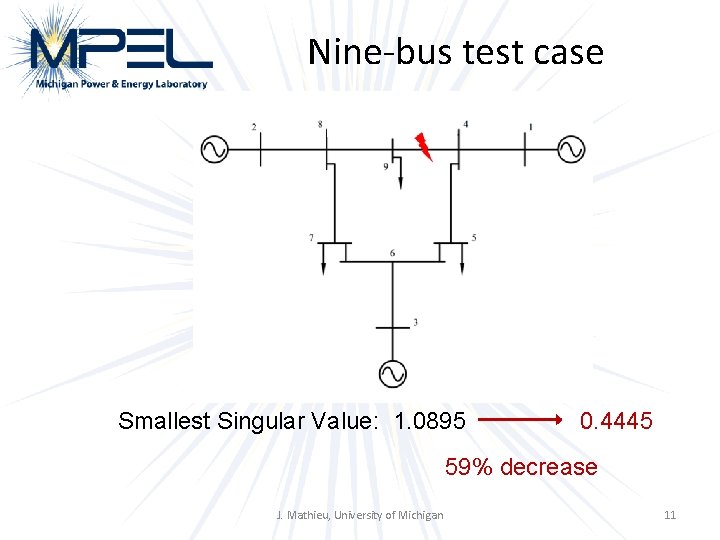
Nine-bus test case Smallest Singular Value: 1. 0895 0. 4445 59% decrease J. Mathieu, University of Michigan 11
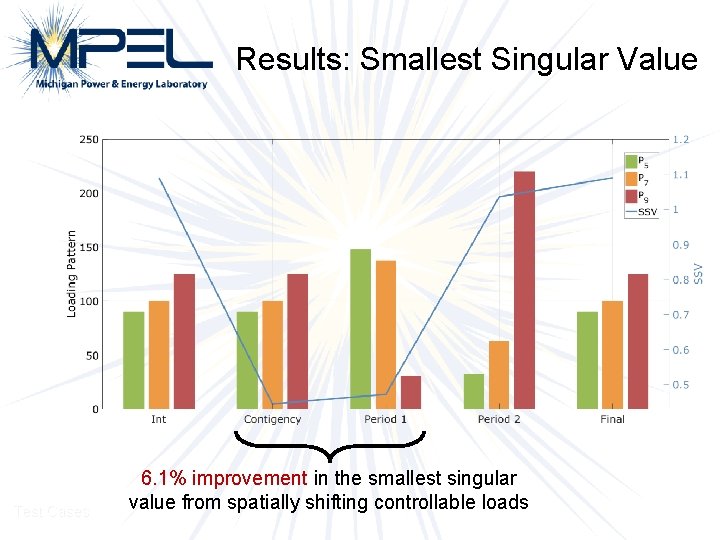
Results: Smallest Singular Value Test Cases 6. 1% improvement in the smallest singular value from spatially shifting controllable loads
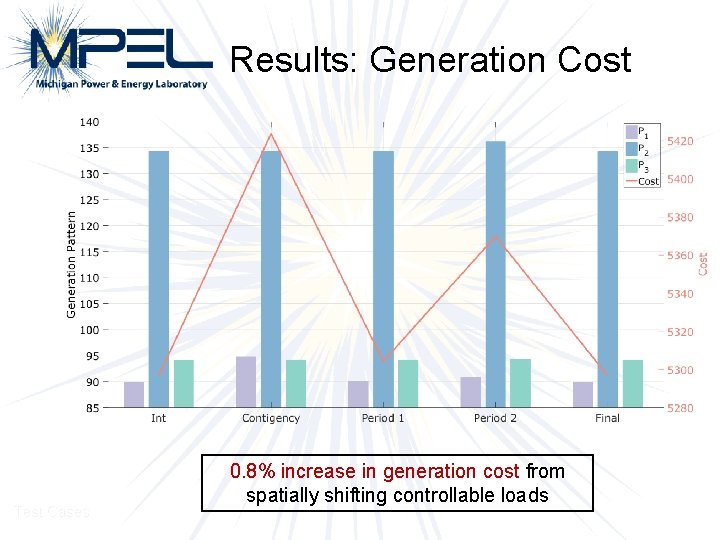
Results: Generation Cost Test Cases 0. 8% increase in generation cost from spatially shifting controllable loads
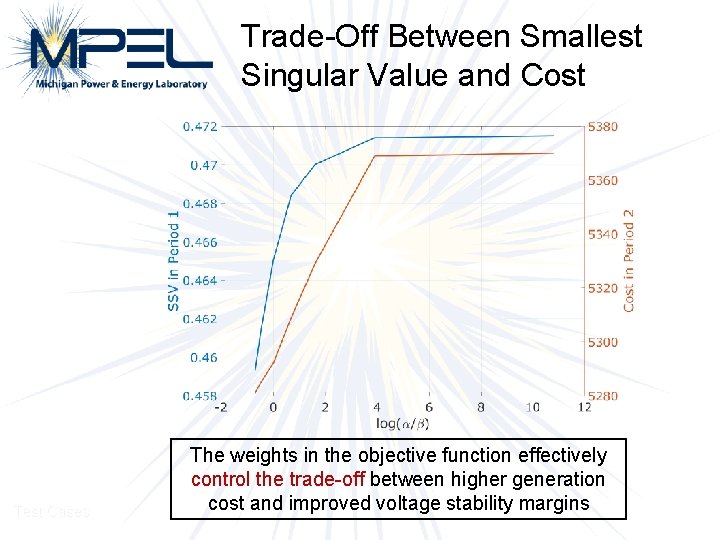
Trade-Off Between Smallest Singular Value and Cost Test Cases The weights in the objective function effectively control the trade-off between higher generation cost and improved voltage stability margins
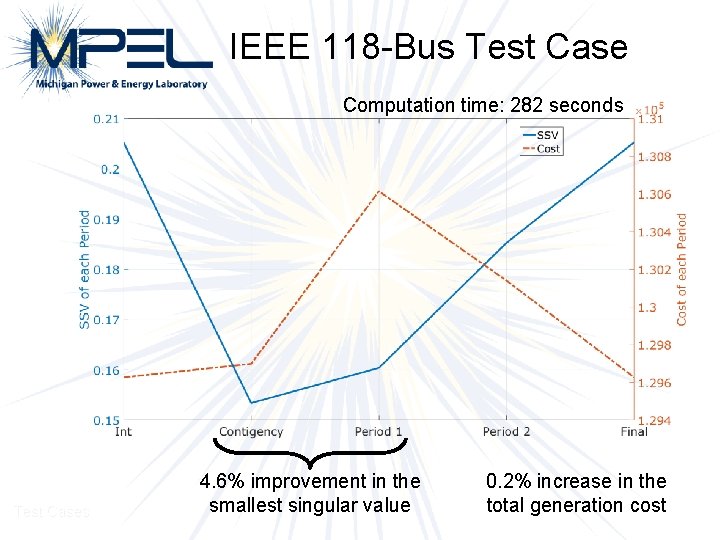
IEEE 118 -Bus Test Case Computation time: 282 seconds Test Cases 4. 6% improvement in the smallest singular value 0. 2% increase in the total generation cost
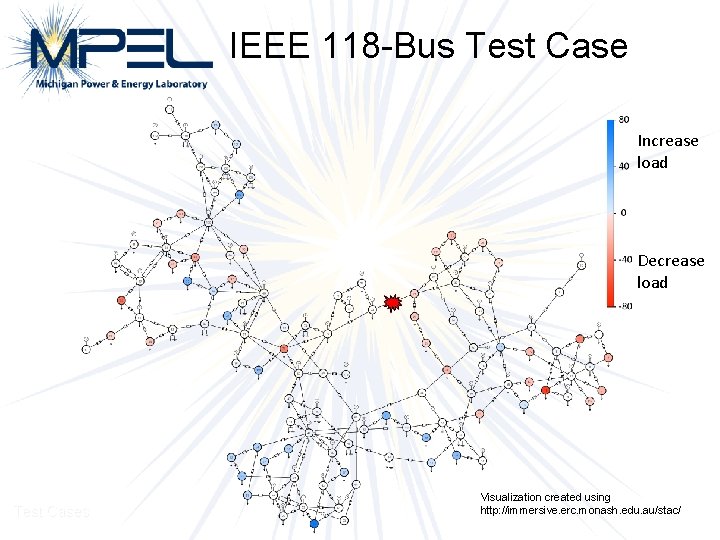
IEEE 118 -Bus Test Case Increase load Decrease load Test Cases Visualization created using http: //immersive. erc. monash. edu. au/stac/
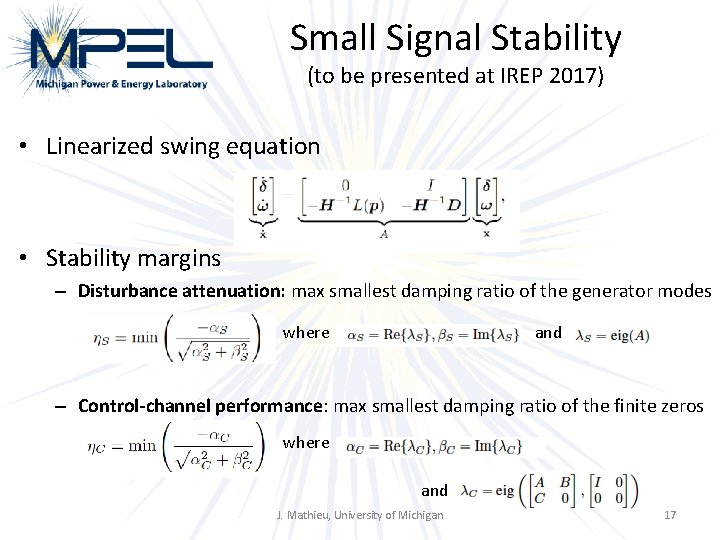
Small Signal Stability (to be presented at IREP 2017) • Linearized swing equation • Stability margins – Disturbance attenuation: max smallest damping ratio of the generator modes where and – Control-channel performance: max smallest damping ratio of the finite zeros where and J. Mathieu, University of Michigan 17
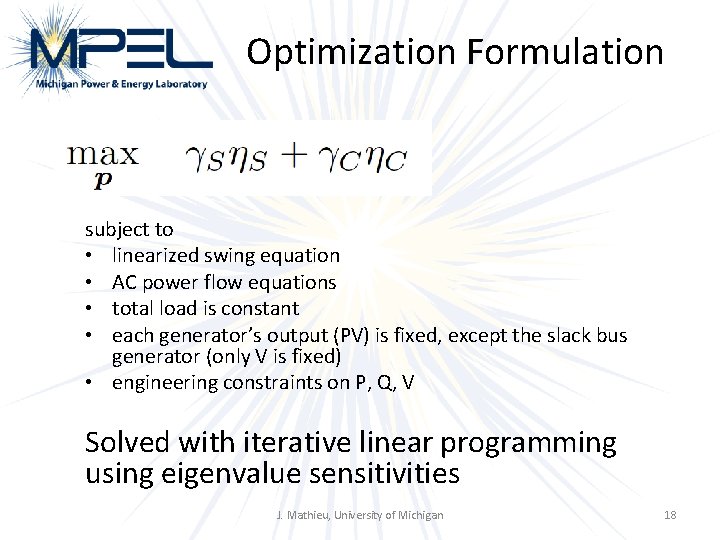
Optimization Formulation subject to • linearized swing equation • AC power flow equations • total load is constant • each generator’s output (PV) is fixed, except the slack bus generator (only V is fixed) • engineering constraints on P, Q, V Solved with iterative linear programming using eigenvalue sensitivities J. Mathieu, University of Michigan 18
![Case Study Kundurs twoarea 11 bus test system Kundur et al 1994 Case Study • Kundur’s two-area 11 -bus test system [Kundur et al. 1994] •](https://slidetodoc.com/presentation_image/a34c662c870b1dc254d8845f0787cb51/image-19.jpg)
Case Study • Kundur’s two-area 11 -bus test system [Kundur et al. 1994] • Maximizing disturbance attenuation Nominal System Optimized Damping ratio: 6. 57 Damping ratio: 6. 92 19
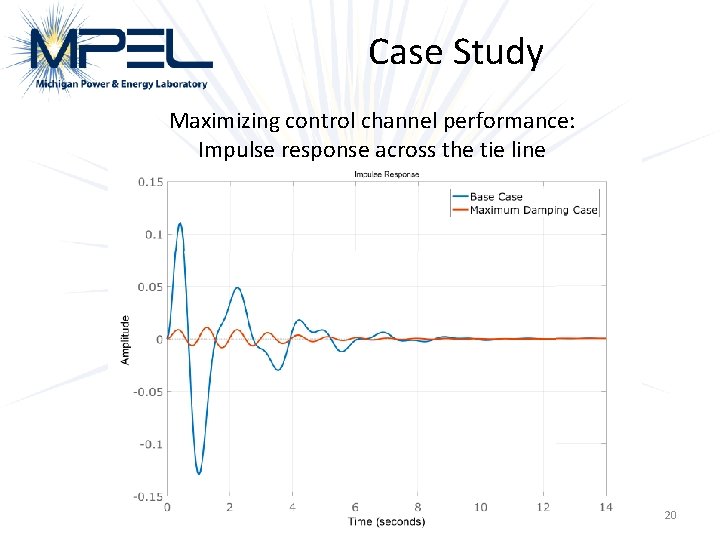
Case Study Maximizing control channel performance: Impulse response across the tie line 20
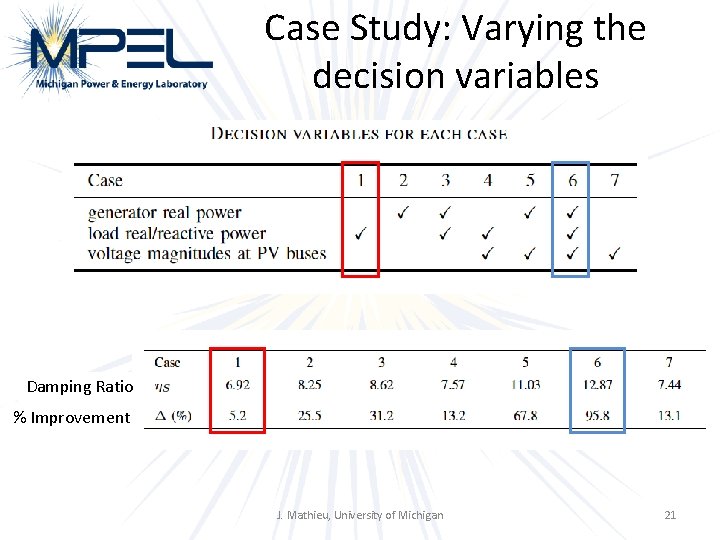
Case Study: Varying the decision variables Damping Ratio % Improvement J. Mathieu, University of Michigan 21
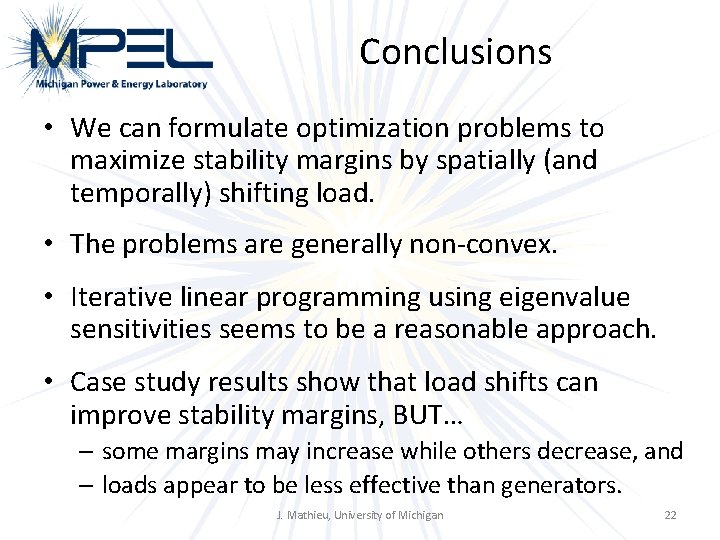
Conclusions • We can formulate optimization problems to maximize stability margins by spatially (and temporally) shifting load. • The problems are generally non-convex. • Iterative linear programming using eigenvalue sensitivities seems to be a reasonable approach. • Case study results show that load shifts can improve stability margins, BUT… – some margins may increase while others decrease, and – loads appear to be less effective than generators. J. Mathieu, University of Michigan 22
Power triangle diagram
Electrical potential formula
Electric potential due to a dipole
Electric field lines
Electric field from electric potential
A suitable electric pump in an electric circuit is a
Chapter 21 electric charge and electric field
Chapter 21 electric charge and electric field
K constant unit
Dc o/d per item charge
Electric charges and electric forces lesson outline
Natural response and forced response
What is natural response
A subsequent
Demand response in smart grids
Cpower demand response
Demand response research center
Stochastic inventory model example
Deficient demand and excess demand
Market demand curve
Demand dependent inventory
Demand schedule tagalog
Dependent demand example