Deep Caps Going Deeper with Capsule Networks Jathushan
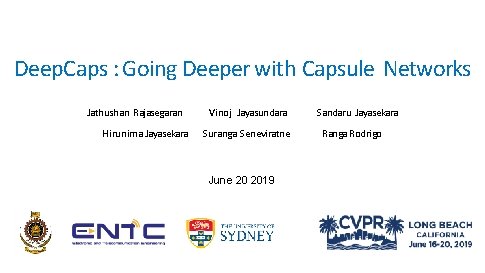
![Capsule Neural Networks [1] S. Sabour, N. Frosst, and G. E. Hinton, “Dynamic routing Capsule Neural Networks [1] S. Sabour, N. Frosst, and G. E. Hinton, “Dynamic routing](https://slidetodoc.com/presentation_image_h2/28bfe843a72e5390b1e74b4e62a12b27/image-2.jpg)
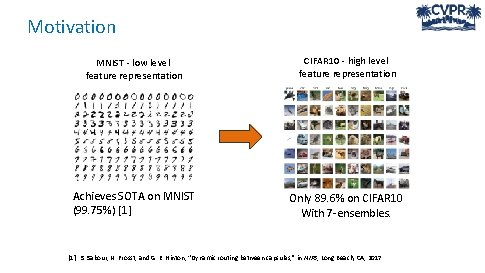
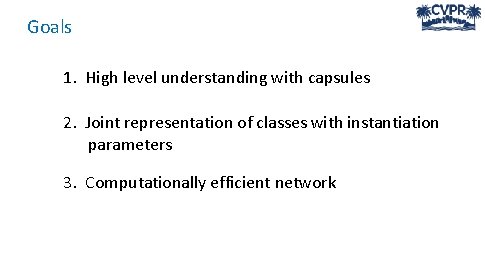
![Inspired by Conventional CNNs Alex. Net [1] VGG Net [2] Goog. Le. Net [3] Inspired by Conventional CNNs Alex. Net [1] VGG Net [2] Goog. Le. Net [3]](https://slidetodoc.com/presentation_image_h2/28bfe843a72e5390b1e74b4e62a12b27/image-5.jpg)
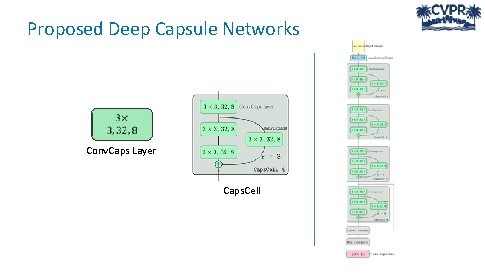
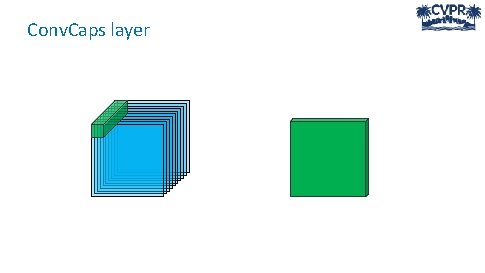
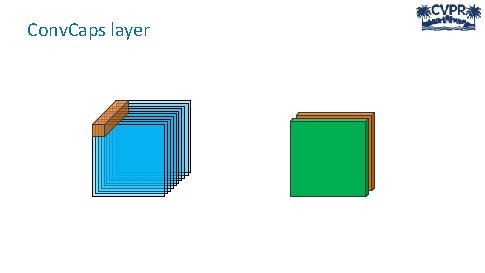
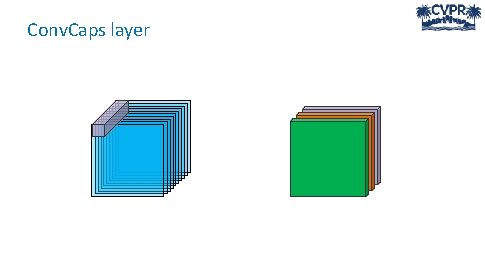
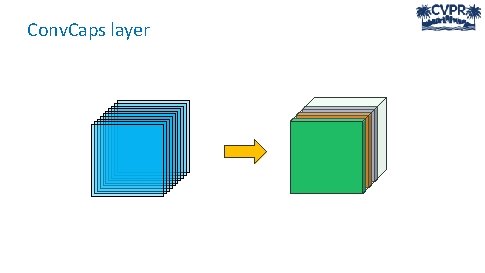
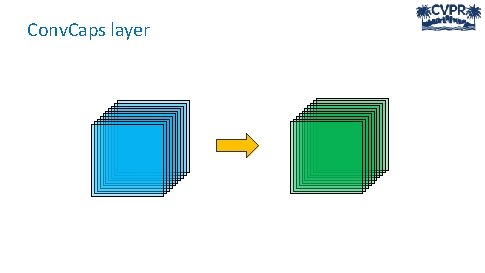
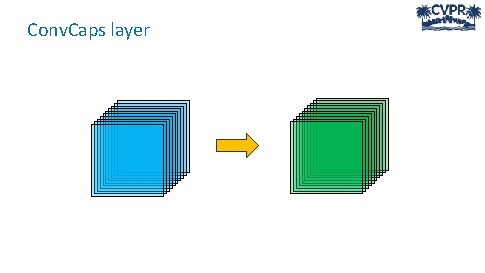
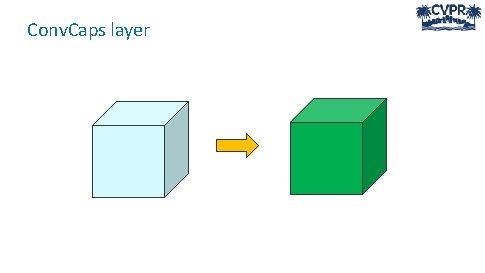
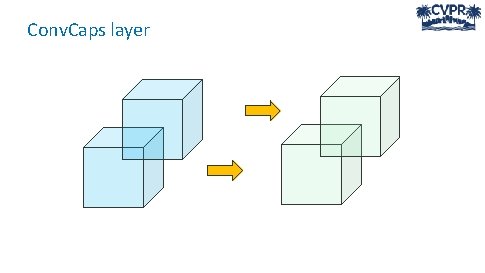
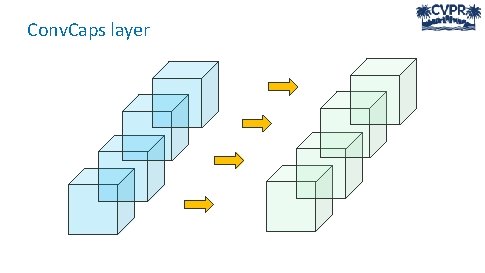
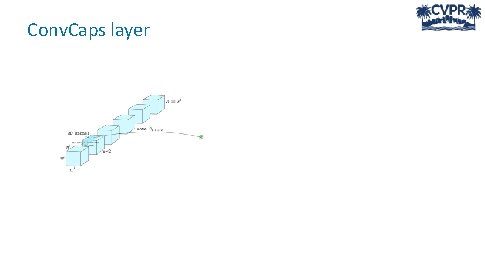
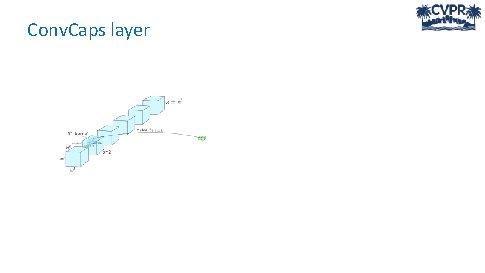
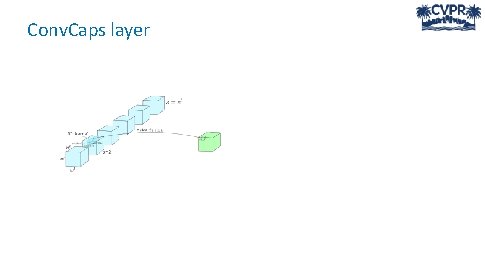
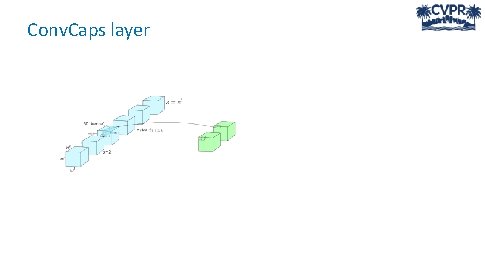
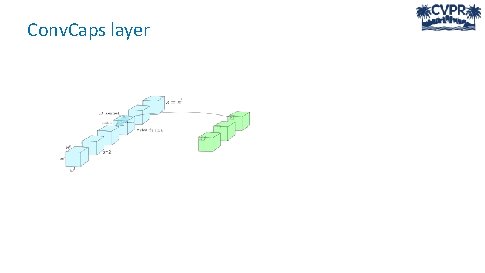
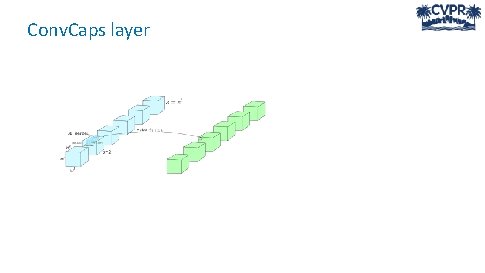
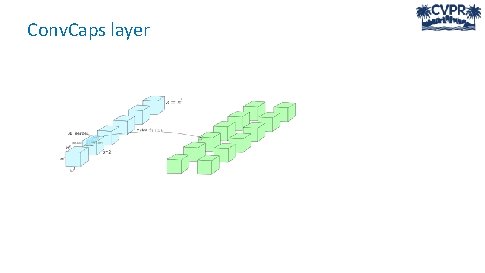
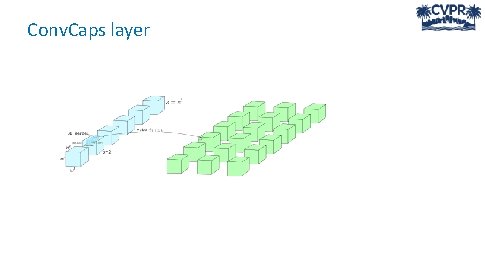
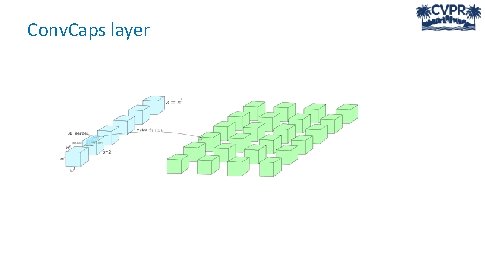
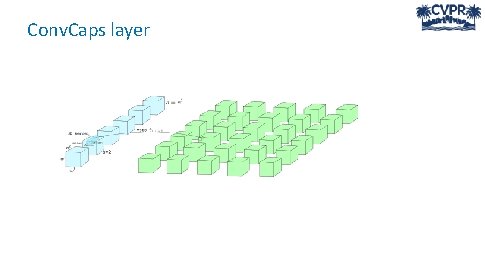
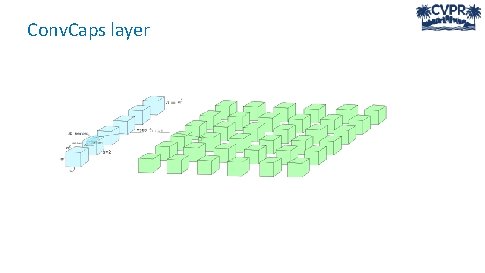
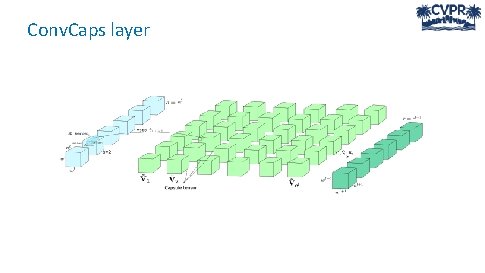
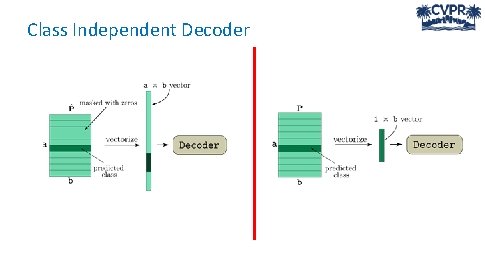
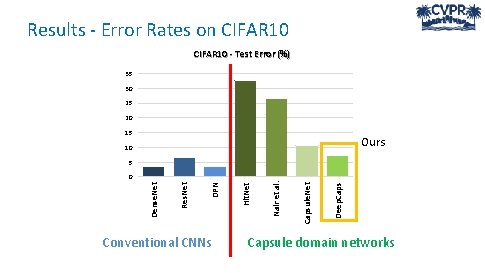
![Results - Performance on other datasets Model CIFAR 10 SVHN F-MNIST Dense. Net [1] Results - Performance on other datasets Model CIFAR 10 SVHN F-MNIST Dense. Net [1]](https://slidetodoc.com/presentation_image_h2/28bfe843a72e5390b1e74b4e62a12b27/image-30.jpg)
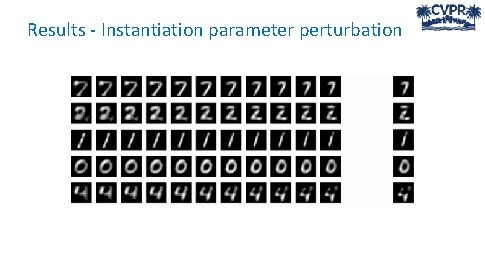
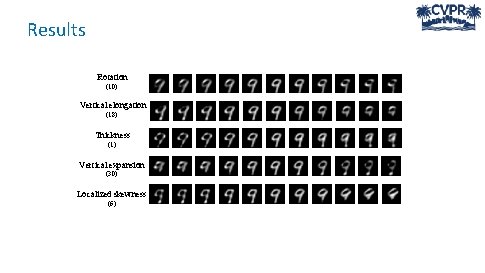
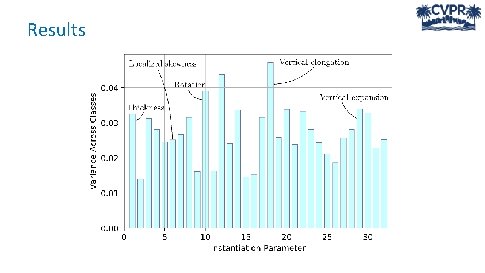
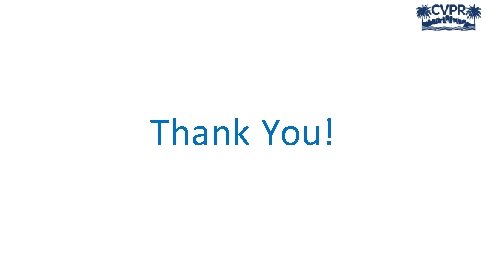
- Slides: 34
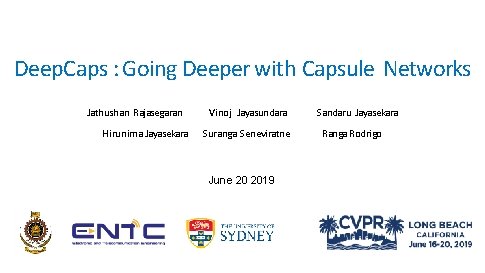
Deep. Caps : Going Deeper with Capsule Networks Jathushan Rajasegaran Hirunima Jayasekara Vinoj Jayasundara Suranga Seneviratne June 20 2019 Sandaru Jayasekara Ranga Rodrigo
![Capsule Neural Networks 1 S Sabour N Frosst and G E Hinton Dynamic routing Capsule Neural Networks [1] S. Sabour, N. Frosst, and G. E. Hinton, “Dynamic routing](https://slidetodoc.com/presentation_image_h2/28bfe843a72e5390b1e74b4e62a12b27/image-2.jpg)
Capsule Neural Networks [1] S. Sabour, N. Frosst, and G. E. Hinton, “Dynamic routing between capsules, ” in NIPS, Long Beach, CA, 2017
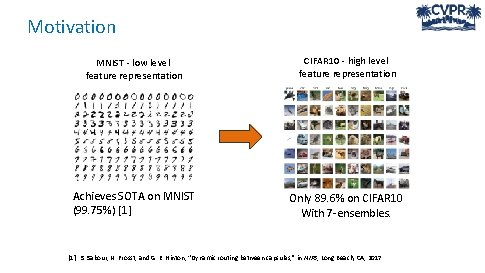
Motivation MNIST - low level feature representation CIFAR 10 - high level feature representation Achieves SOTA on MNIST (99. 75%) [1] Only 89. 6% on CIFAR 10 With 7 -ensembles. [1] S. Sabour, N. Frosst, and G. E. Hinton, “Dynamic routing between capsules, ” in NIPS, Long Beach, CA, 2017
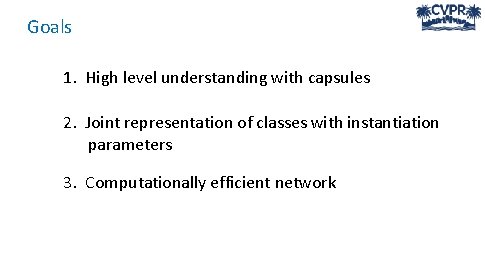
Goals 1. High level understanding with capsules 2. Joint representation of classes with instantiation parameters 3. Computationally efficient network
![Inspired by Conventional CNNs Alex Net 1 VGG Net 2 Goog Le Net 3 Inspired by Conventional CNNs Alex. Net [1] VGG Net [2] Goog. Le. Net [3]](https://slidetodoc.com/presentation_image_h2/28bfe843a72e5390b1e74b4e62a12b27/image-5.jpg)
Inspired by Conventional CNNs Alex. Net [1] VGG Net [2] Goog. Le. Net [3] Res. Net [4] [1] [2] [3] [4] A. Krizhevsky, I. Sutskever, and G. Hinton. Imagenet classificationwith deep convolutional neural networks. In NIPS, 2012 S. Ren, K. He, R. Girshick, and J. Sun. Faster R-CNN: Towardsreal-time object detection with region proposal networks. In NIPS, 2015 C. Szegedy, W. Liu, Y. Jia, P. Sermanet, S. Reed, D. Anguelov, D. Er-han, V. Vanhoucke, and A. Rabinovich. Going deeper with convolutions. In CVPR, 2015 K. He, X. Zhang, and S. Ren, “Deep residual learning for image recognition, ” in CVPR, 2016
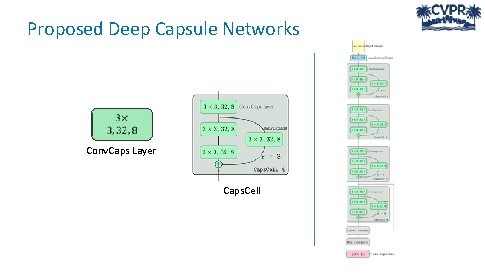
Proposed Deep Capsule Networks Conv. Caps Layer Caps. Cell
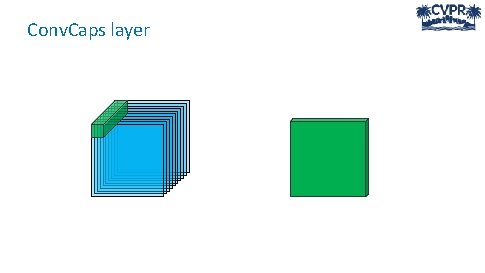
Conv. Caps layer
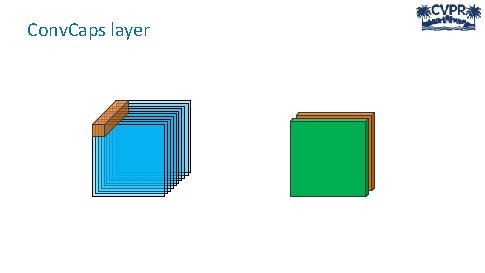
Conv. Caps layer
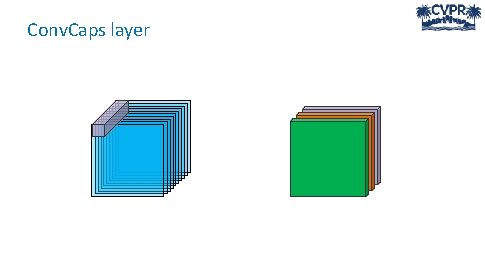
Conv. Caps layer
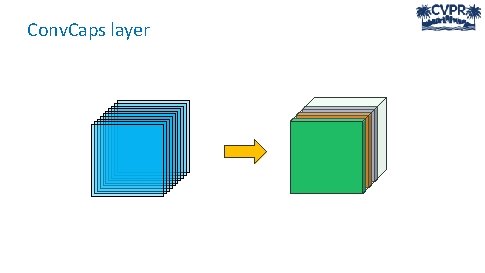
Conv. Caps layer
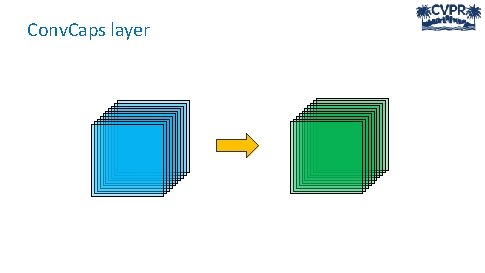
Conv. Caps layer
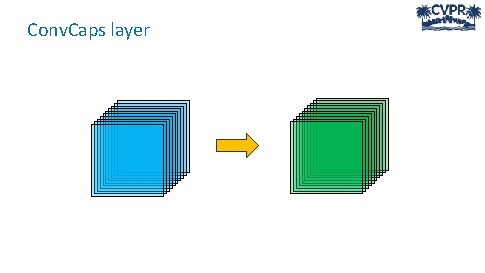
Conv. Caps layer
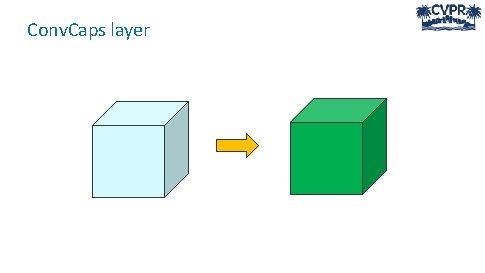
Conv. Caps layer
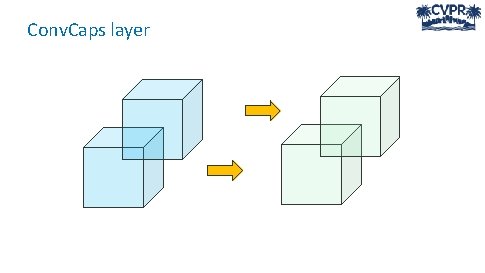
Conv. Caps layer
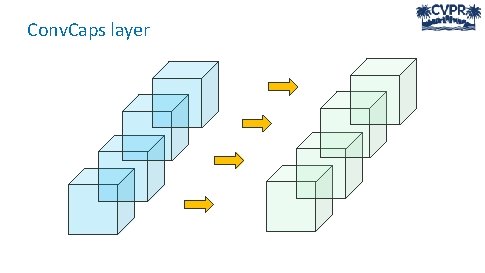
Conv. Caps layer
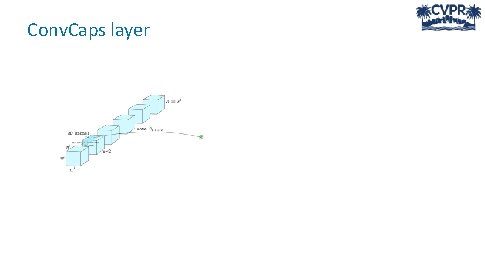
Conv. Caps layer
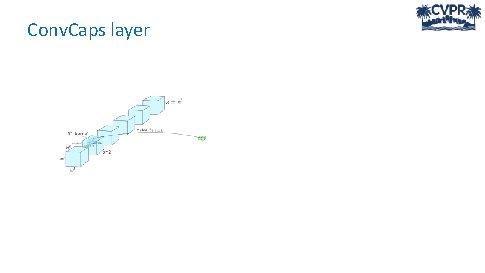
Conv. Caps layer
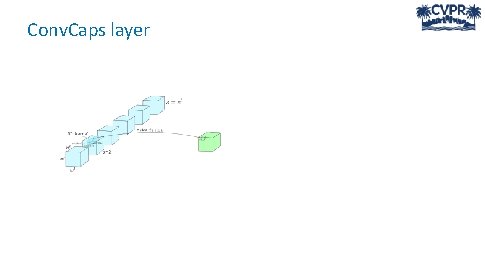
Conv. Caps layer
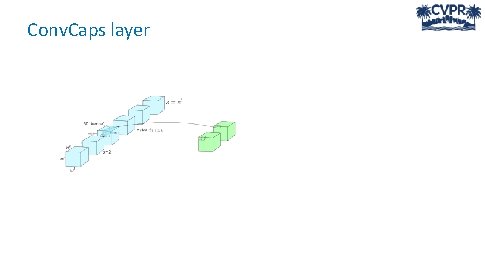
Conv. Caps layer
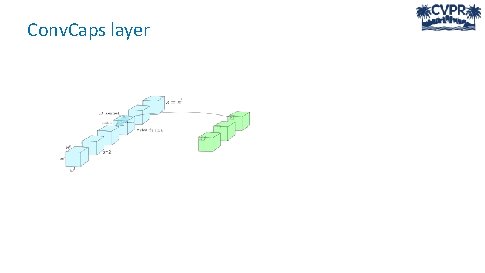
Conv. Caps layer
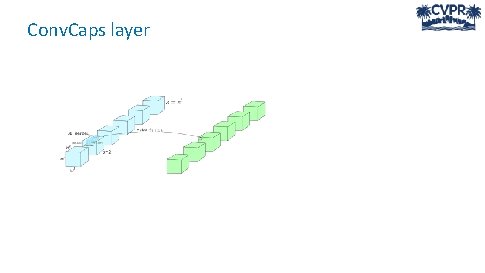
Conv. Caps layer
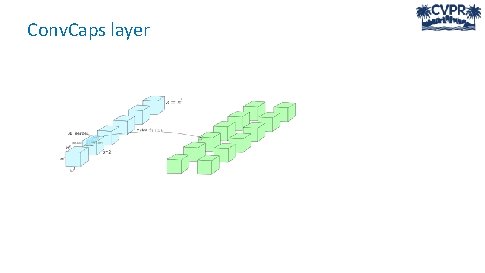
Conv. Caps layer
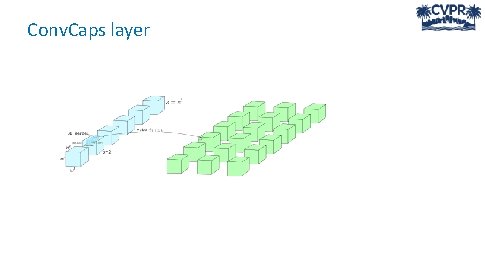
Conv. Caps layer
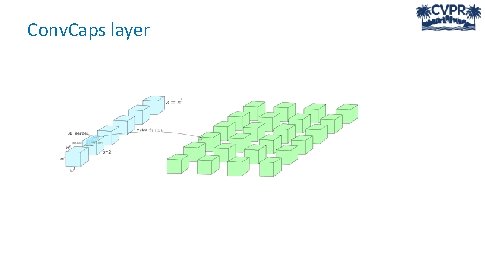
Conv. Caps layer
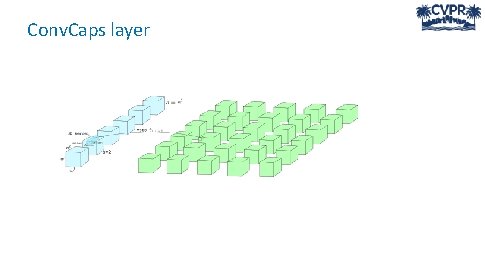
Conv. Caps layer
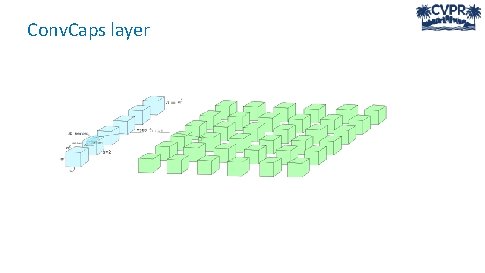
Conv. Caps layer
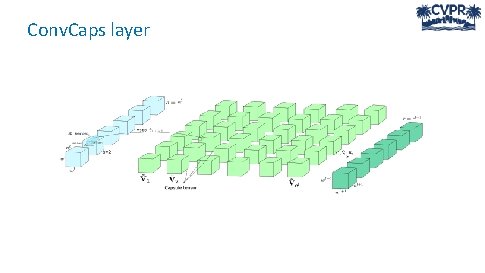
Conv. Caps layer
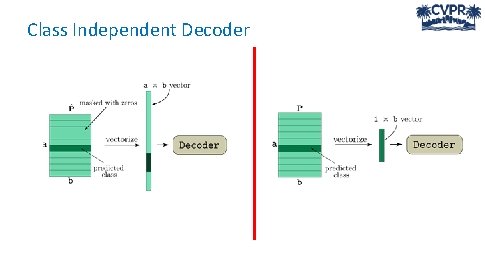
Class Independent Decoder
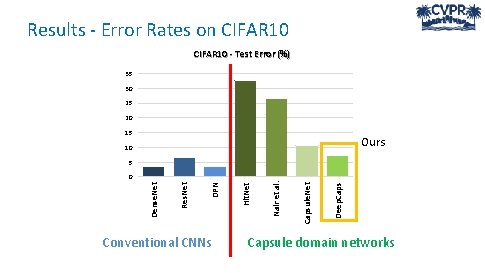
Results - Error Rates on CIFAR 10 - Test Error (%) 35 30 25 20 15 Ours 10 5 Conventional CNNs Deep. Capsule. Net Nair et al. Hit. Net DPN Res. Net Dense. Net 0 Capsule domain networks
![Results Performance on other datasets Model CIFAR 10 SVHN FMNIST Dense Net 1 Results - Performance on other datasets Model CIFAR 10 SVHN F-MNIST Dense. Net [1]](https://slidetodoc.com/presentation_image_h2/28bfe843a72e5390b1e74b4e62a12b27/image-30.jpg)
Results - Performance on other datasets Model CIFAR 10 SVHN F-MNIST Dense. Net [1] Res. Net [2] DPN [3] Wan et al. [4] Zhong et al. [5] Sabour et al. [6] Nair et al. [7] Hit. Net [8] Deep. Caps (7 -ensembles) 96. 40% 93. 57% 96. 35% 96. 92% 89. 40% 67. 53% 73. 30% 91. 01% 92. 74% 98. 41% 95. 70% 91. 06 % 94. 50% 97. 16% 97. 56% 95. 40% 95. 70% 96. 35% 93. 60% 89. 80% 92. 30% 94. 46% 94. 73% 99. 59% 99. 75% 99. 50% 99. 68% 99. 72% - [1] G. Huang, Z. Liu, L. Van Der Maaten, and K. Q. Wein- berger, “Densely connected convolutional networks. ” in CVPR, 2017. [2] K. He, X. Zhang, S. Ren, and. J. Sun, “Deepresiduallearning for image recognition, ” in CVPR , 2016. [3] Y. Chen, J. Li, H. Xiao, X. Jin, S. Yan, and J. Feng, “Dual path networks, ” in NIPS, 2017. [4] L. Wan, M. Zeiler, S. Zhang, Y. L. Cun, and. R. Fergus, “Reg- ularization of neural networks using dropconnect, ” ICML , 2013. [5] Z. Zhong, L. Zheng, G. Kang, S. Li, and Y. Yang, “Random erasing data augmentation, ” Co. RR, 2017. [6] S. Sabour, N. Frosst, and G. E. Hinton, “Dynamic routing be- tween capsules, ” in NIPS, 2017. [7] P. Q. Nair, R. Doshi, and S. Keselj, “Pushing the limits of capsule networks, ” 2018. [8] A. Delie`ge, A. Cioppa, and. M. Van. Droogenbroeck, “Hitnet: a neural network with capsules embedded in a hit-or-miss layer, extended with hybrid data augmentation and ghost capsules, ” Co. RR, 2018.
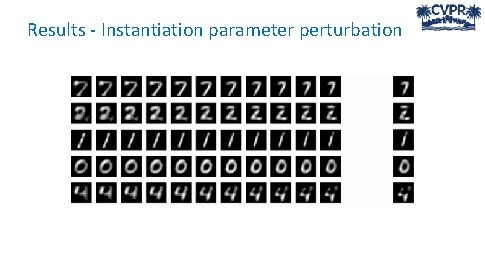
Results - Instantiation parameter perturbation
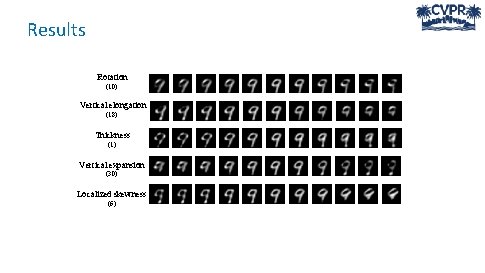
Results Rotation (10) Vertical elongation (18) Thickness (1) Vertical expansion (30) Localized skewness (6)
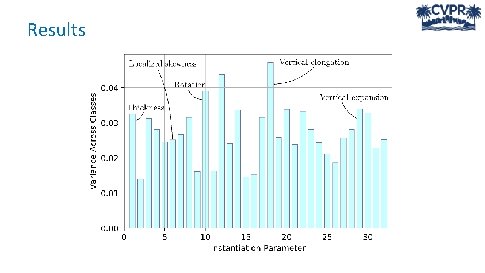
Results
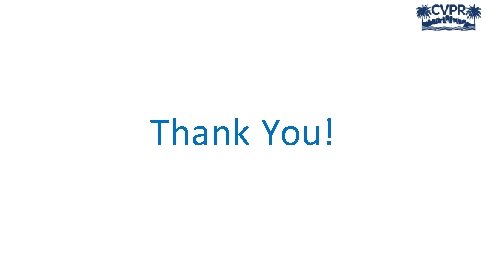
Thank You!
Into the heart of jesus deeper and deeper i go
Tic tac toe going high going low going criss cross lollipop
Definition of soft gelatin capsule
Deep forest: towards an alternative to deep neural networks
Capsule networks tutorial
If you are going through hell, keep going
Deep asleep deep asleep it lies
深哉深哉
Mippers
Deep convolutional networks
Image super resolution using deep convolutional networks
Convolutional deep belief networks
Distbelief
Efficient processing of deep neural networks pdf
Greedy layer wise training of deep networks
Difference between virtual circuit and datagram
Basestore iptv
Dive deep synonyms
Growing deeper in god
Dclm netherlands
Deeper life netherlands
Deeper life netherlands
Deeper life bible church netherlands search the scriptures
Deeper life canada
"we thinkers" curriculum or methodology or vocabulary
Deeper life bible church netherlands
Promote together
Deeper life bible church netherlands
Netherlands
Deeper exam
Deeper life netherlands
Deeper life netherlands
Your love is deeper than the ocean higher than the heavens
Deeper life bible church
Deeper life bible church netherlands search the scriptures