Data Integration in Official Statistics UNECE Project Report
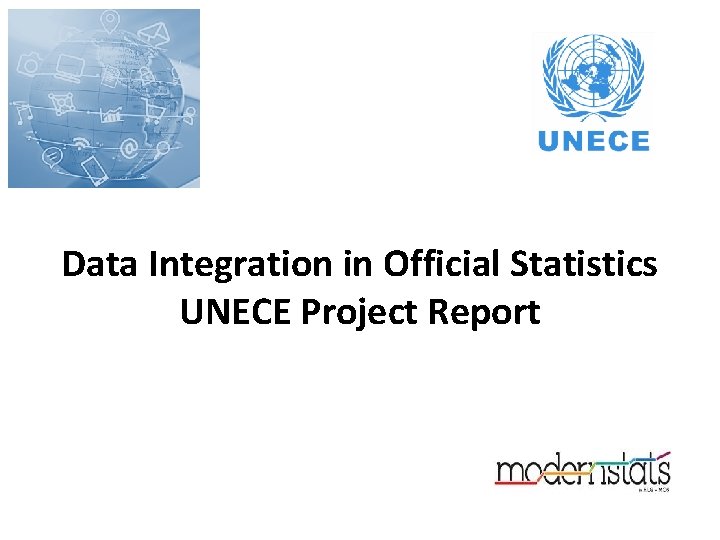
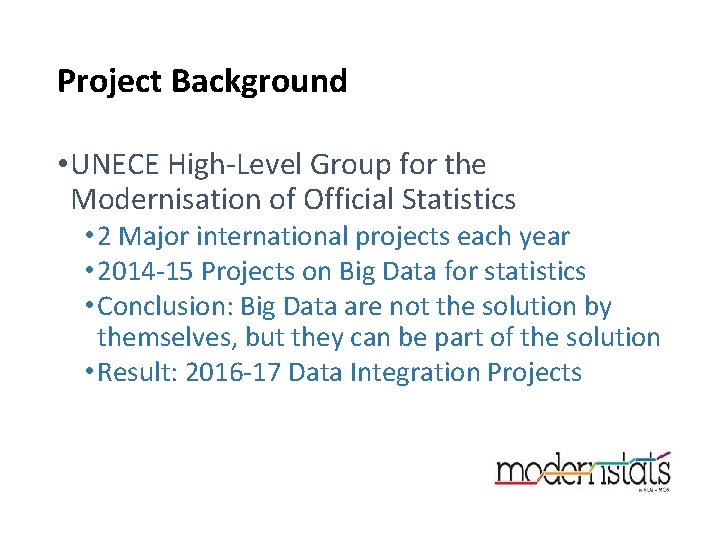
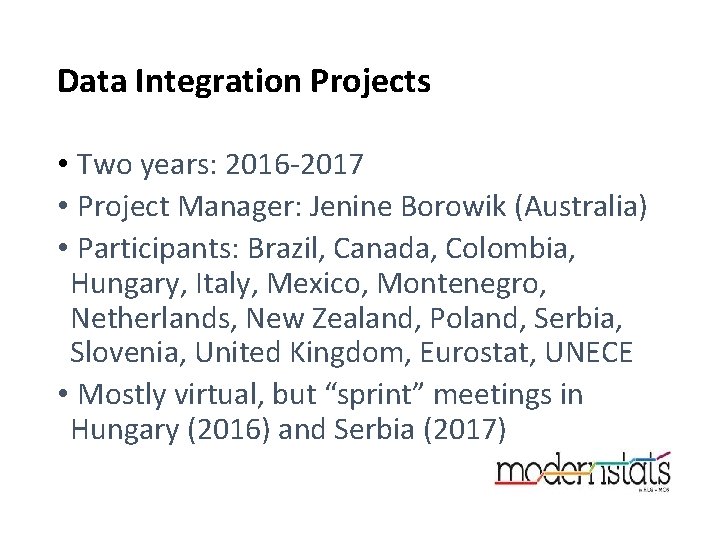
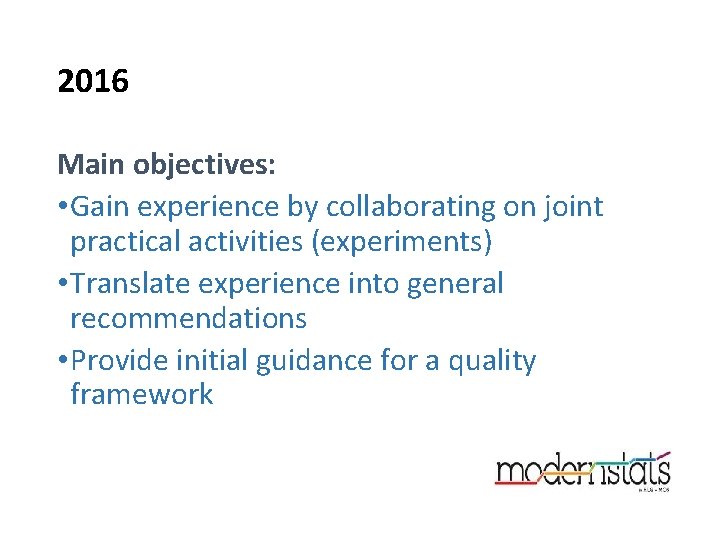
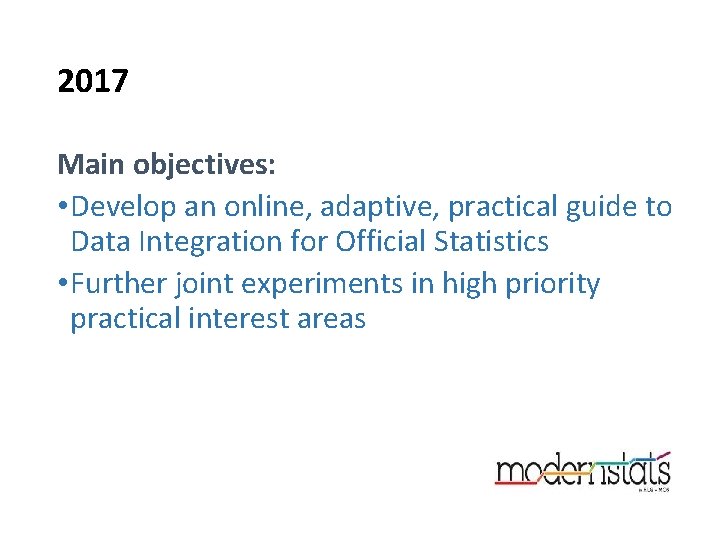
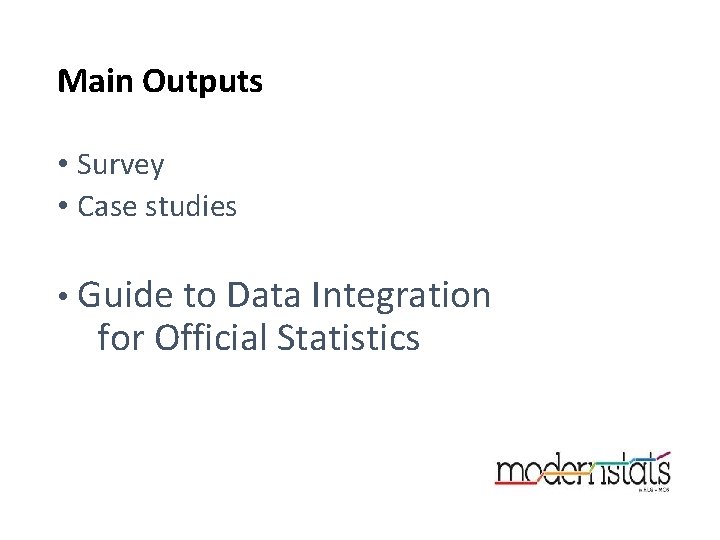
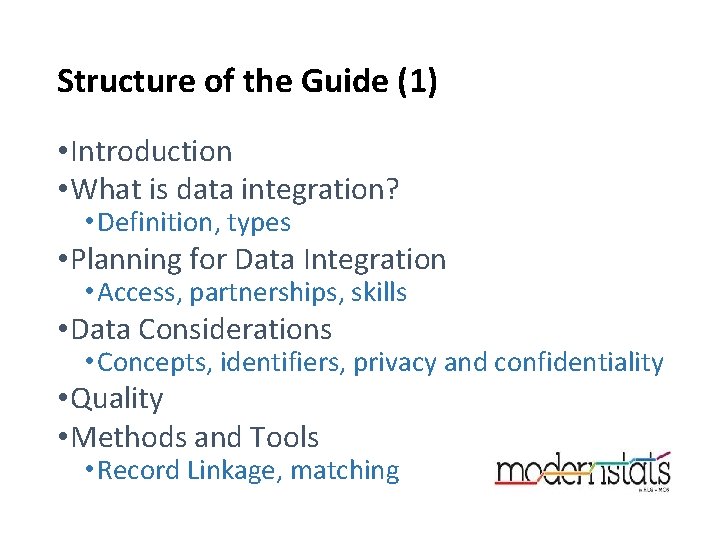
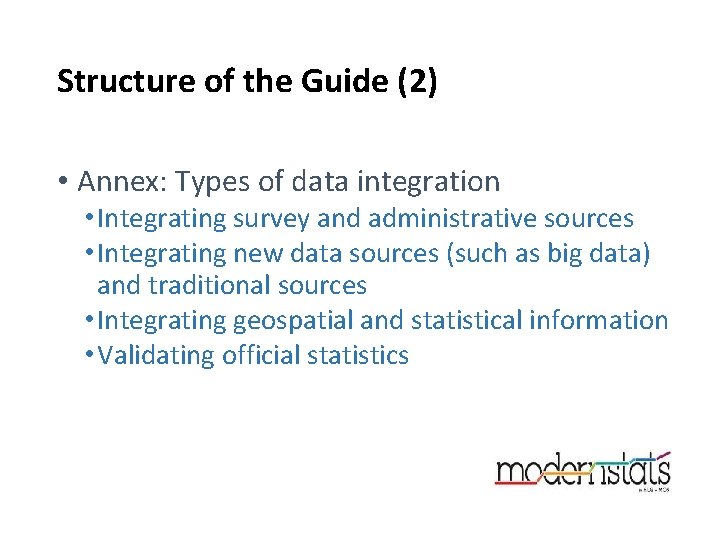
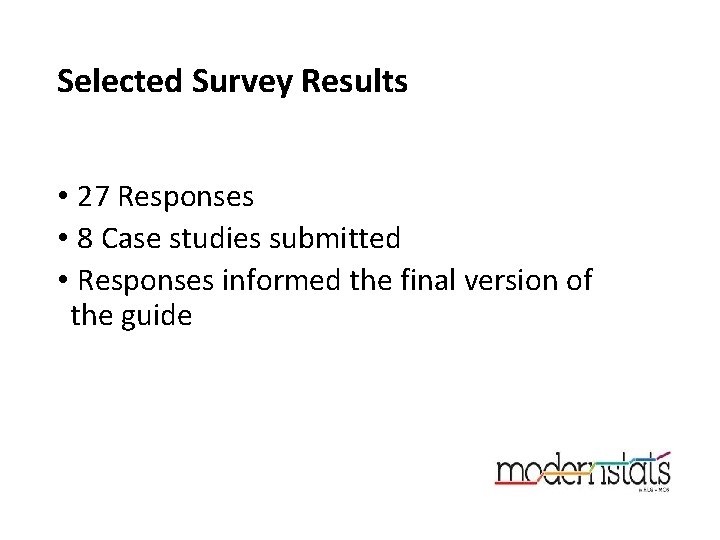
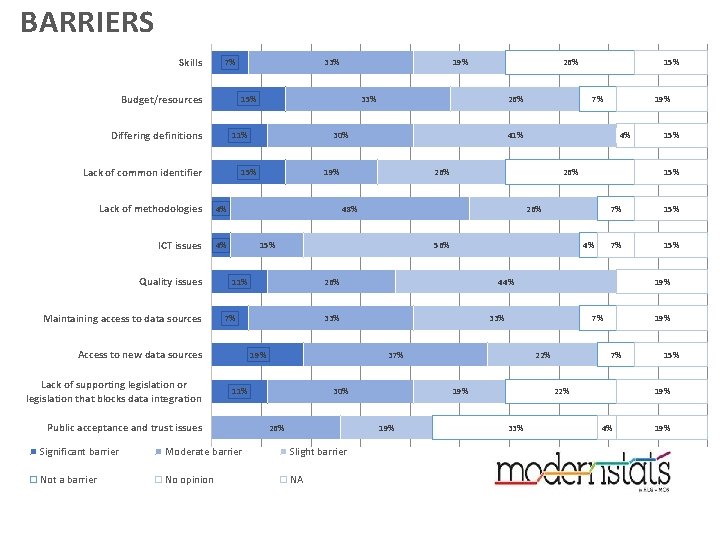
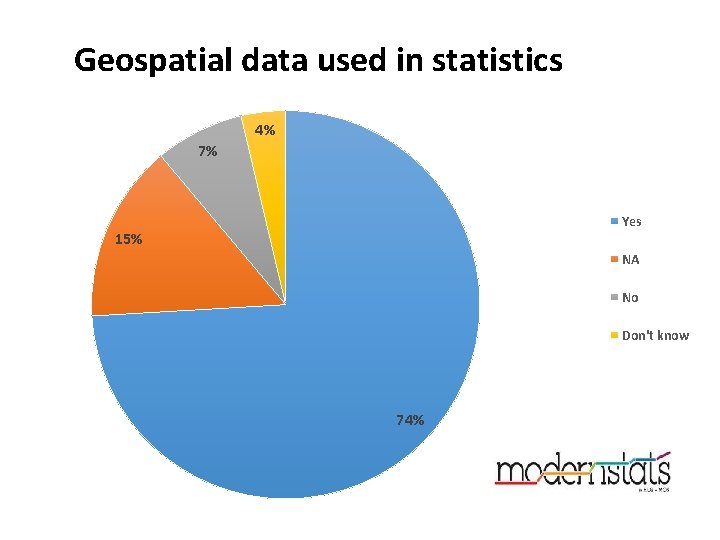
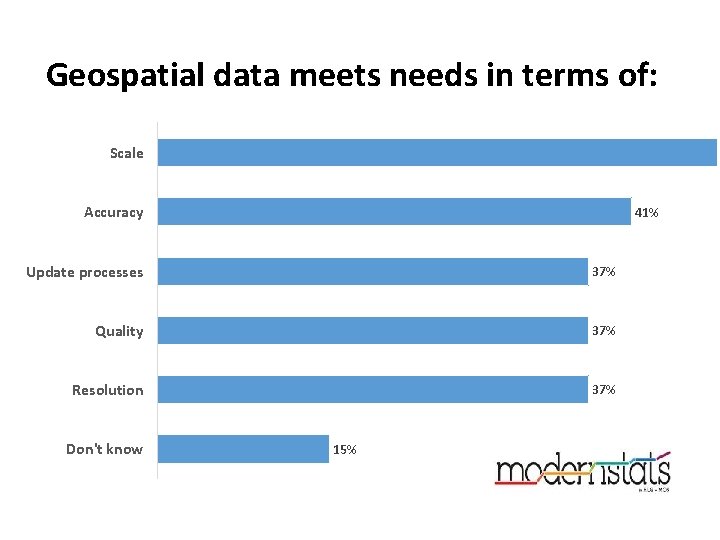
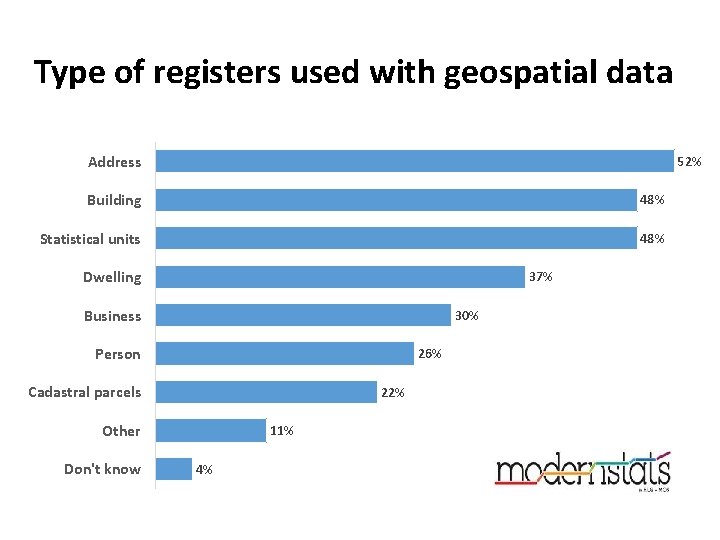
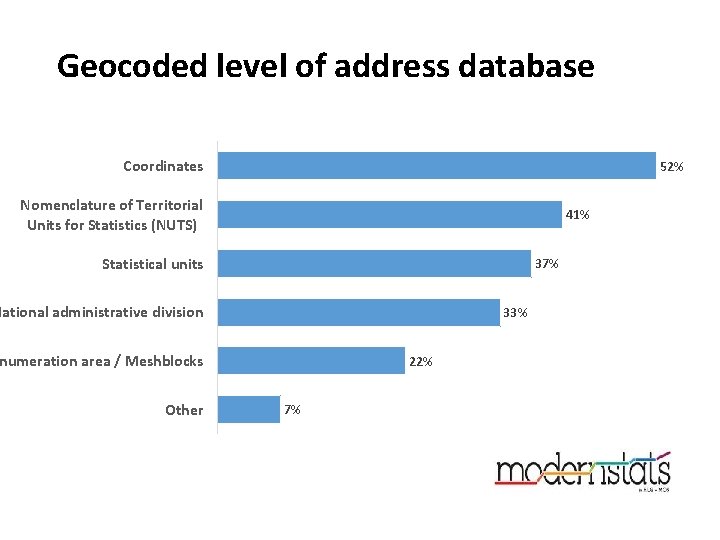
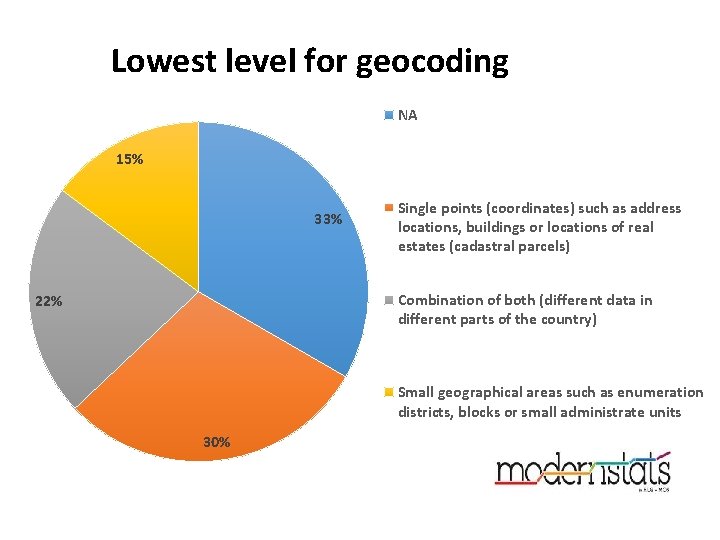
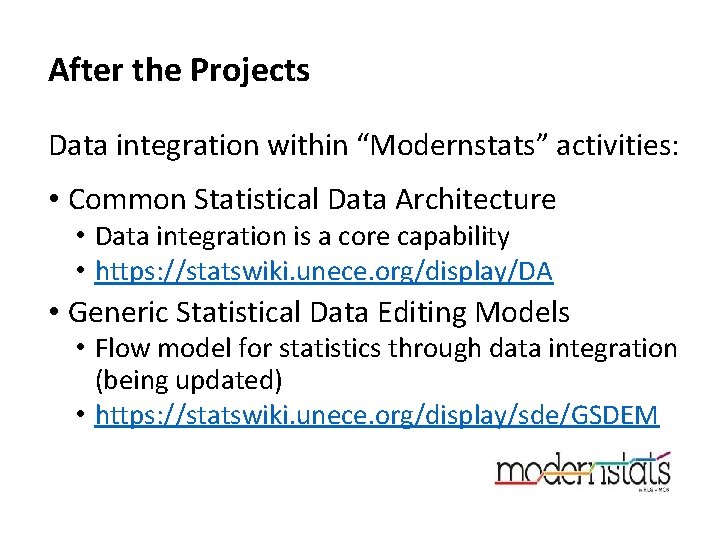
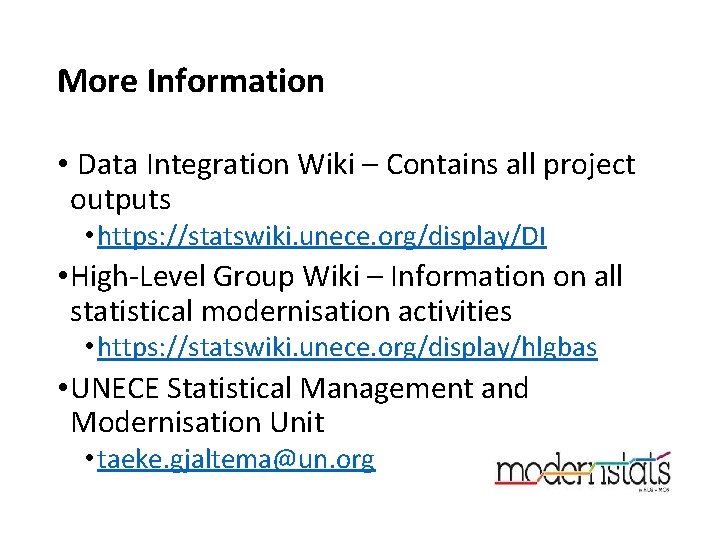
- Slides: 17
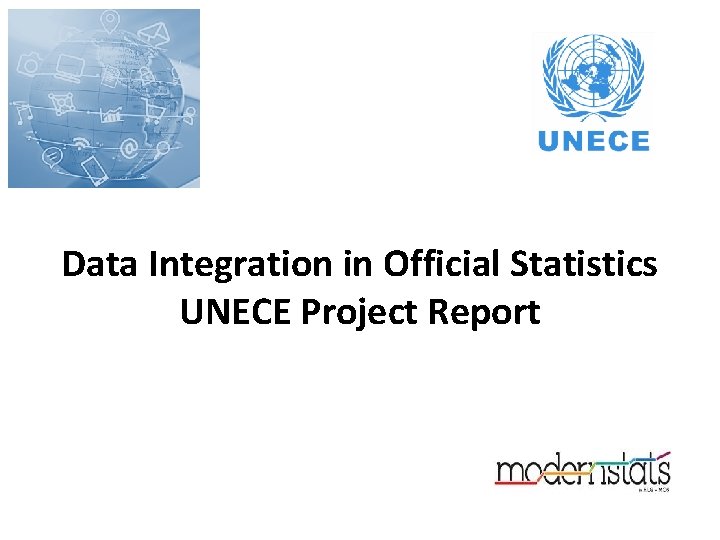
Data Integration in Official Statistics UNECE Project Report
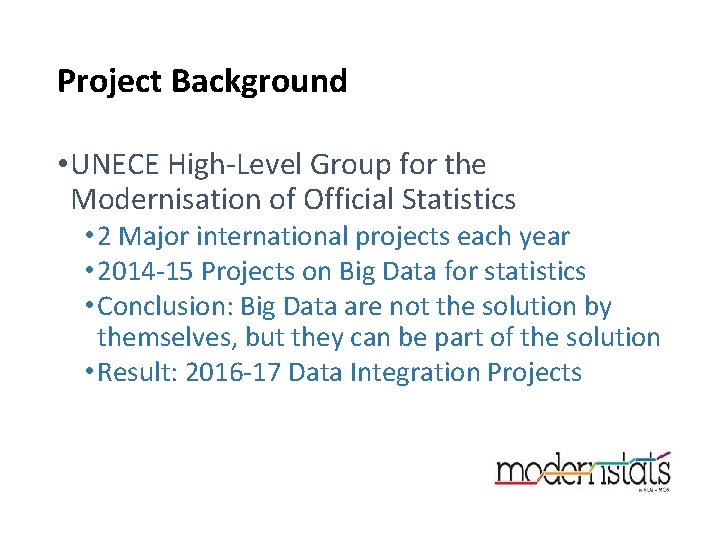
Project Background • UNECE High-Level Group for the Modernisation of Official Statistics • 2 Major international projects each year • 2014 -15 Projects on Big Data for statistics • Conclusion: Big Data are not the solution by themselves, but they can be part of the solution • Result: 2016 -17 Data Integration Projects
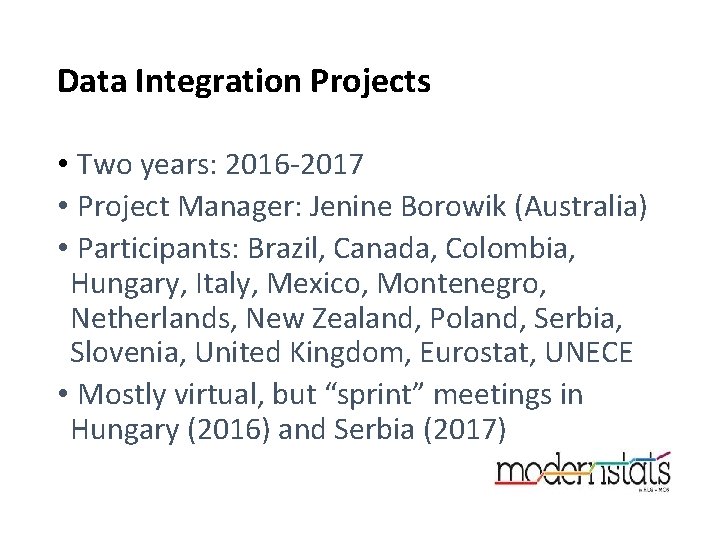
Data Integration Projects • Two years: 2016 -2017 • Project Manager: Jenine Borowik (Australia) • Participants: Brazil, Canada, Colombia, Hungary, Italy, Mexico, Montenegro, Netherlands, New Zealand, Poland, Serbia, Slovenia, United Kingdom, Eurostat, UNECE • Mostly virtual, but “sprint” meetings in Hungary (2016) and Serbia (2017)
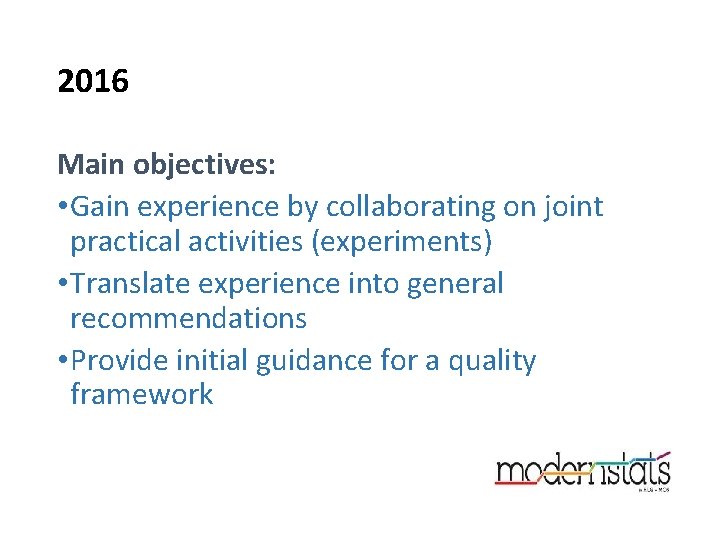
2016 Main objectives: • Gain experience by collaborating on joint practical activities (experiments) • Translate experience into general recommendations • Provide initial guidance for a quality framework
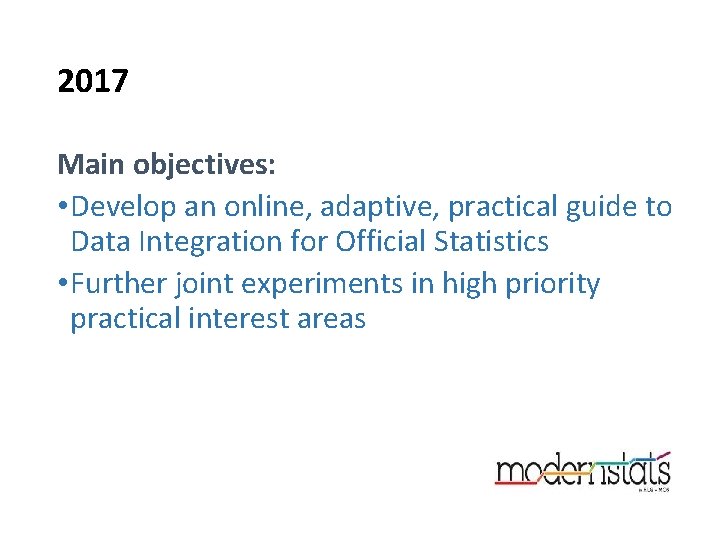
2017 Main objectives: • Develop an online, adaptive, practical guide to Data Integration for Official Statistics • Further joint experiments in high priority practical interest areas
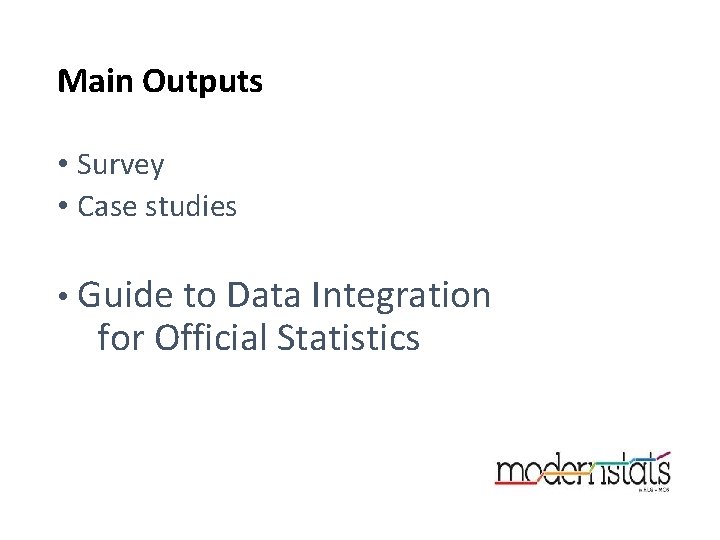
Main Outputs • Survey • Case studies • Guide to Data Integration for Official Statistics
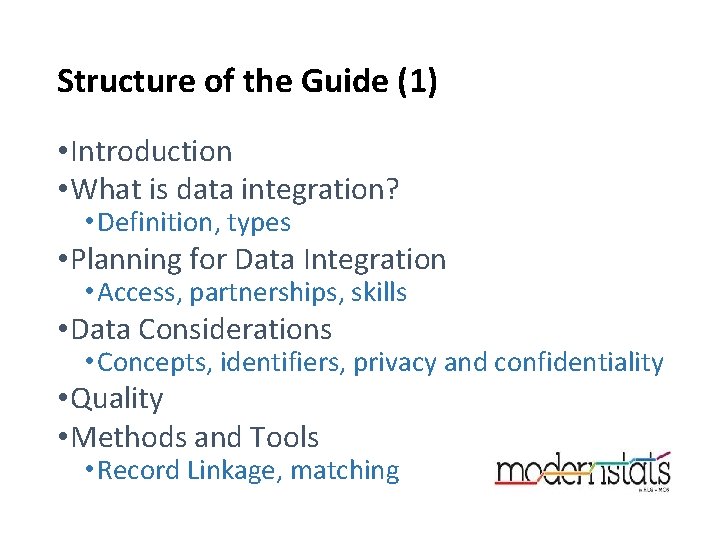
Structure of the Guide (1) • Introduction • What is data integration? • Definition, types • Planning for Data Integration • Access, partnerships, skills • Data Considerations • Concepts, identifiers, privacy and confidentiality • Quality • Methods and Tools • Record Linkage, matching
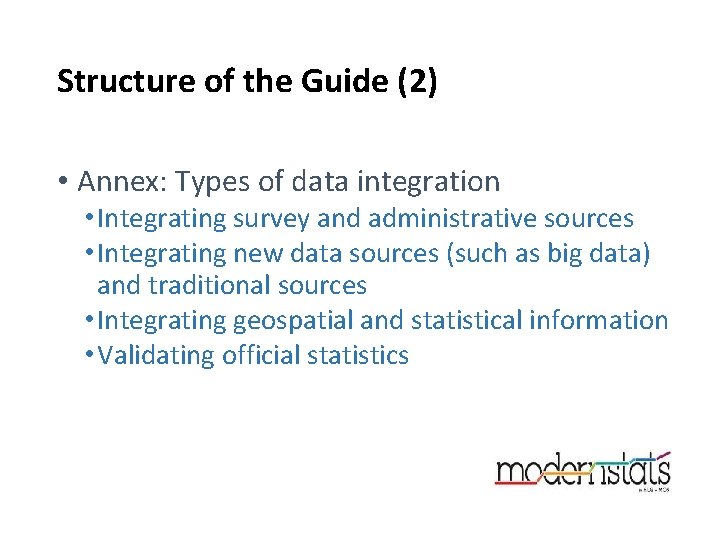
Structure of the Guide (2) • Annex: Types of data integration • Integrating survey and administrative sources • Integrating new data sources (such as big data) and traditional sources • Integrating geospatial and statistical information • Validating official statistics
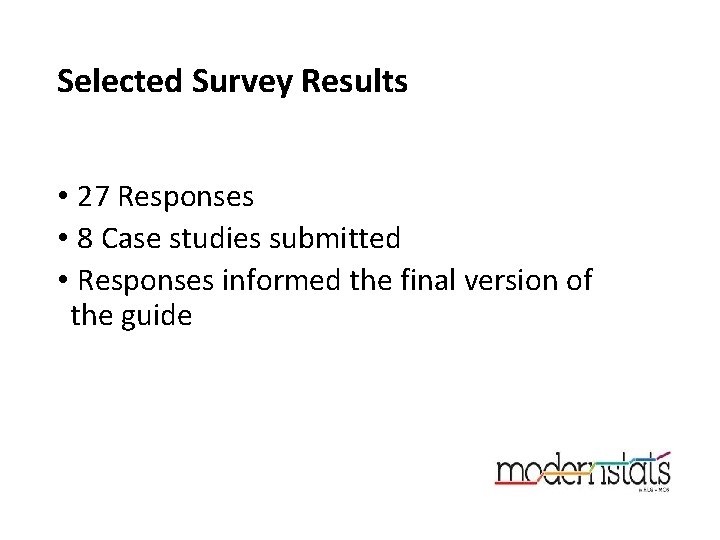
Selected Survey Results • 27 Responses • 8 Case studies submitted • Responses informed the final version of the guide
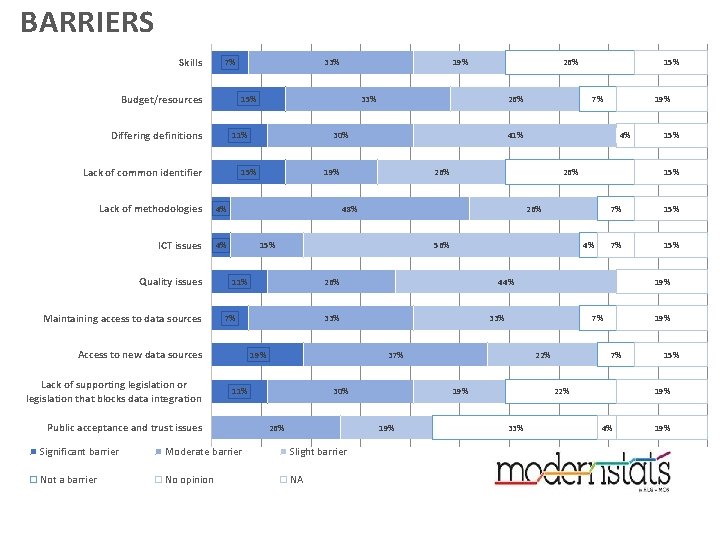
BARRIERS Skills 7% Budget/resources 4% ICT issues 4% 19% 26% 4% 30% 26% Moderate barrier Slight barrier Not a barrier No opinion NA 7% 15% 7% 22% 19% Significant barrier 15% 19% 33% 37% 11% 7% 44% 33% 19% 7% 22% 33% 15% 26% 19% 4% 56% 7% Public acceptance and trust issues 7% 26% 15% 11% 15% 41% 48% Access to new data sources Lack of supporting legislation or legislation that blocks data integration 26% 30% 15% Lack of methodologies 26% 33% 11% Lack of common identifier Maintaining access to data sources 19% 15% Differing definitions Quality issues 33% 15% 19% 4% 19%
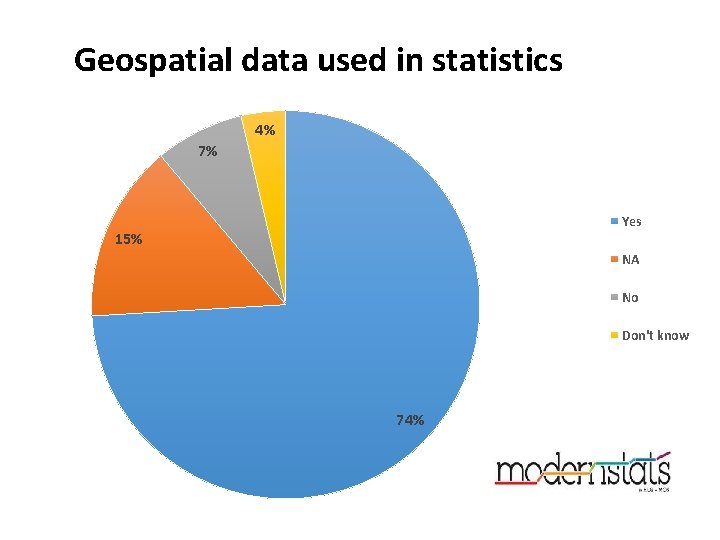
Geospatial data used in statistics 4% 7% Yes 15% NA No Don't know 74%
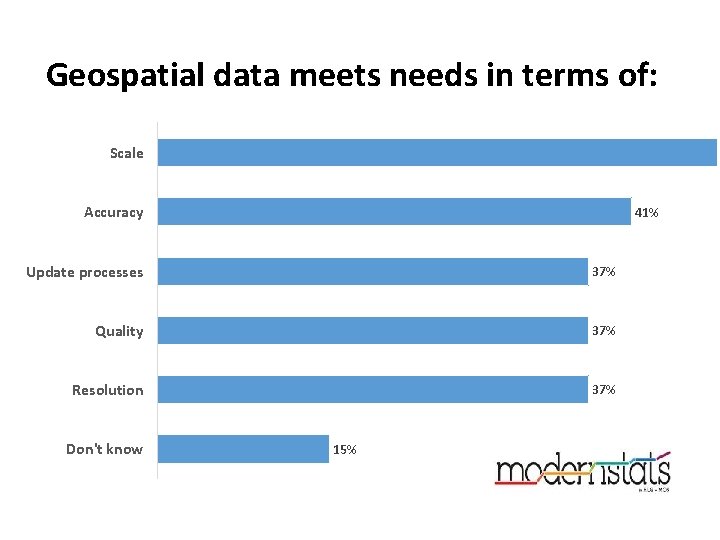
Geospatial data meets needs in terms of: Scale Accuracy 41% Update processes 37% Quality 37% Resolution 37% Don't know 15%
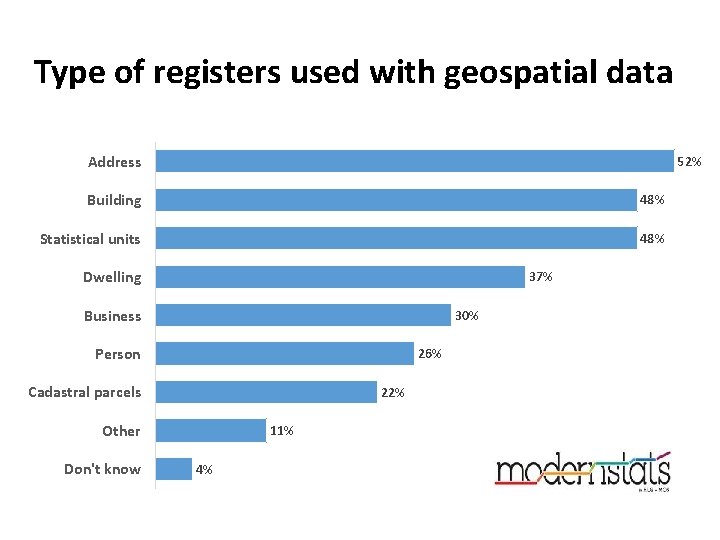
Type of registers used with geospatial data Address 52% Building 48% Statistical units 48% Dwelling 37% Business 30% Person 26% Cadastral parcels 22% Other Don't know 11% 4%
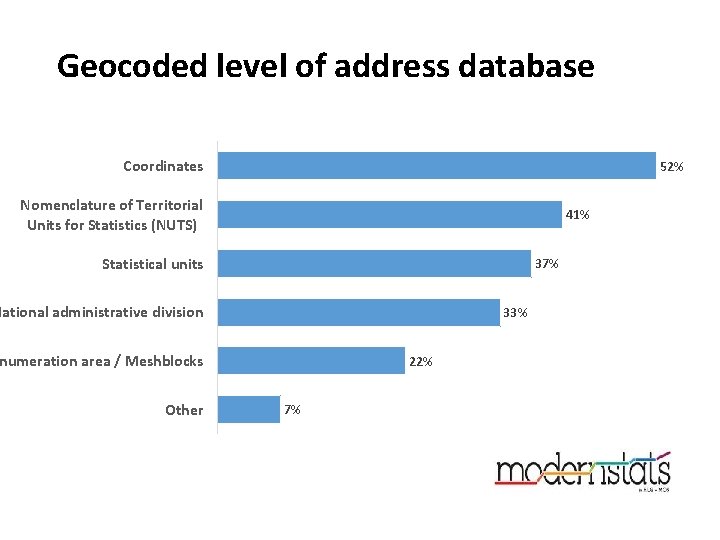
Geocoded level of address database Coordinates 52% Nomenclature of Territorial Units for Statistics (NUTS) 41% Statistical units 37% National administrative division 33% numeration area / Meshblocks Other 22% 7%
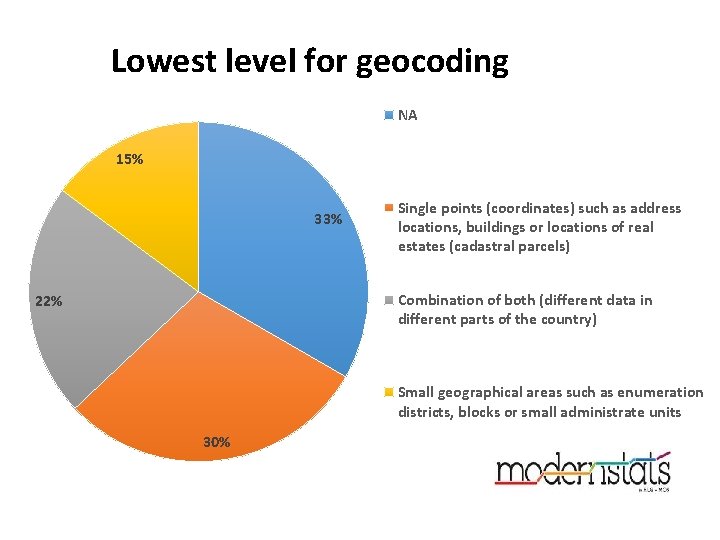
Lowest level for geocoding NA 15% 33% Single points (coordinates) such as address locations, buildings or locations of real estates (cadastral parcels) Combination of both (different data in different parts of the country) 22% Small geographical areas such as enumeration districts, blocks or small administrate units 30%
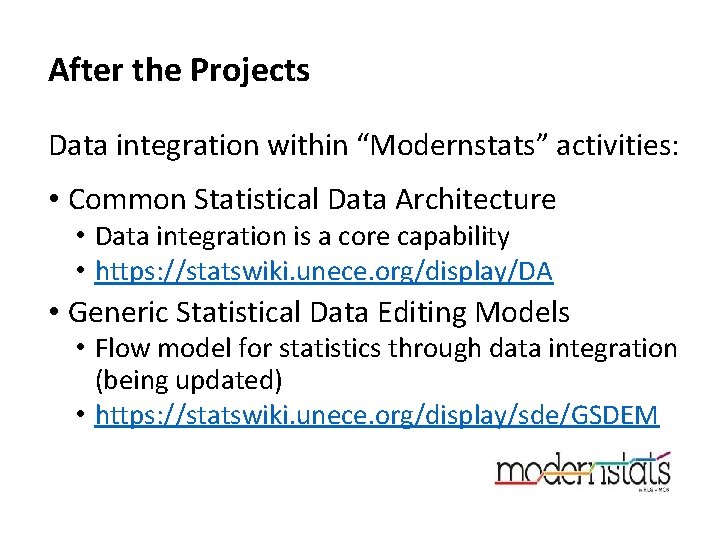
After the Projects Data integration within “Modernstats” activities: • Common Statistical Data Architecture • Data integration is a core capability • https: //statswiki. unece. org/display/DA • Generic Statistical Data Editing Models • Flow model for statistics through data integration (being updated) • https: //statswiki. unece. org/display/sde/GSDEM
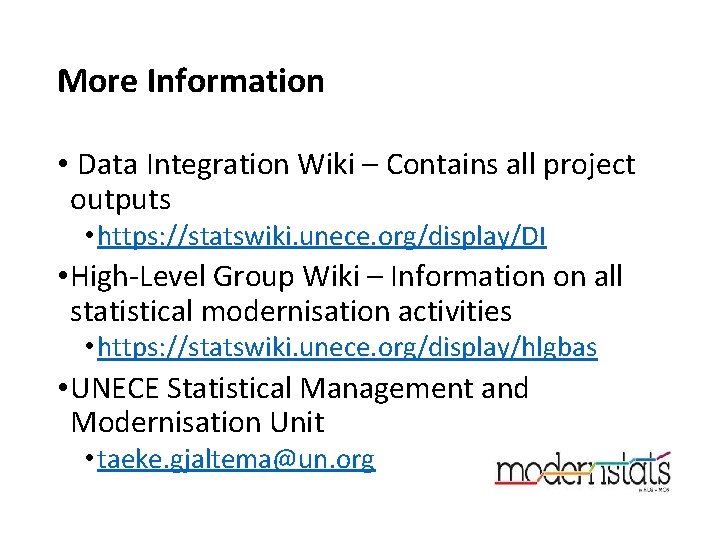
More Information • Data Integration Wiki – Contains all project outputs • https: //statswiki. unece. org/display/DI • High-Level Group Wiki – Information on all statistical modernisation activities • https: //statswiki. unece. org/display/hlgbas • UNECE Statistical Management and Modernisation Unit • taeke. gjaltema@un. org
Big data official statistics
Big data official statistics
Big data official statistics
Official statistics from mobile phone data
Difference between official and non official compendia
Data integration statistics
European master on official statistics
Official statistics of sweden
European master in official statistics
Unece
Unece
Cenelec vs atex
Impactnexus
Tailor information
Unece
Unece nuclear energy
Unece nuclear energy
Unece standards