Confounding An Introduction Philip la Fleur RPh MScEpidem
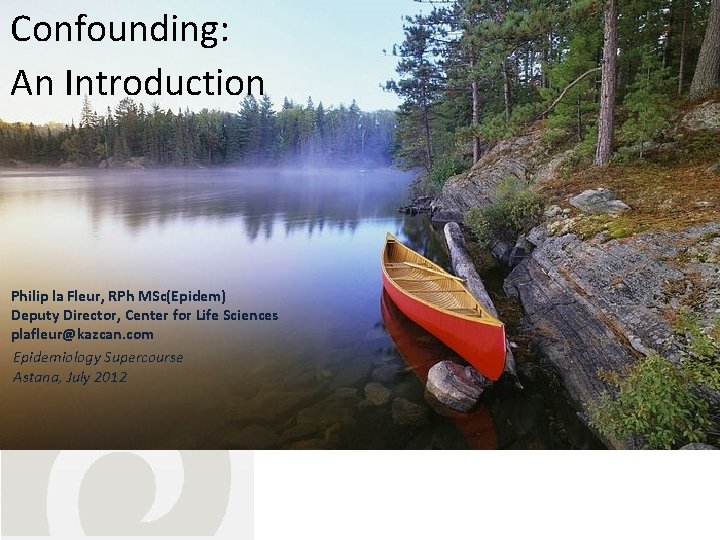
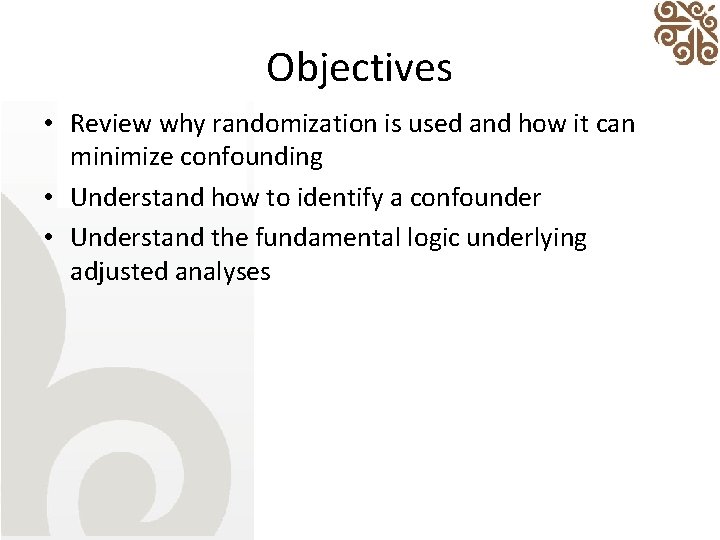
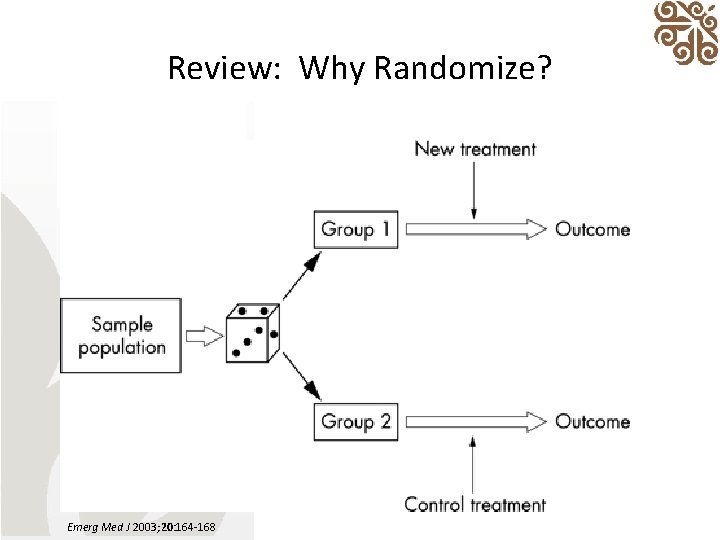
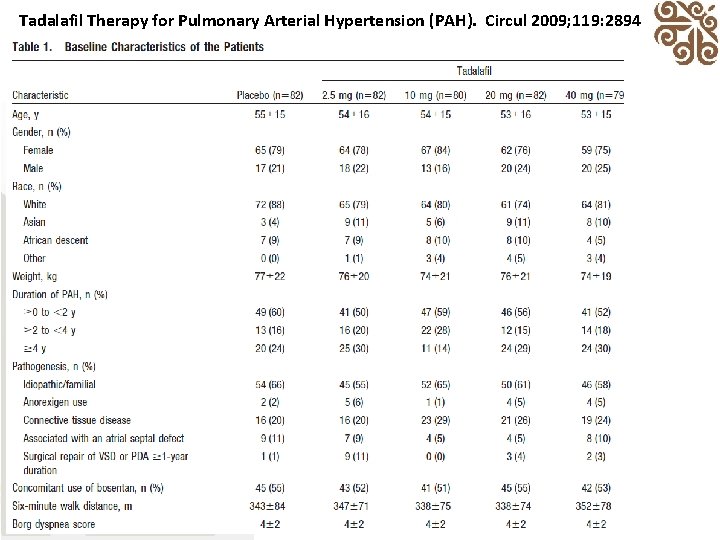
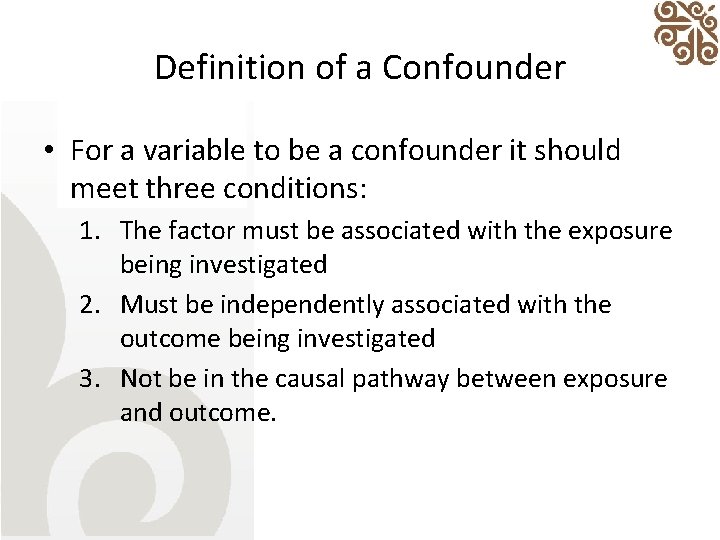
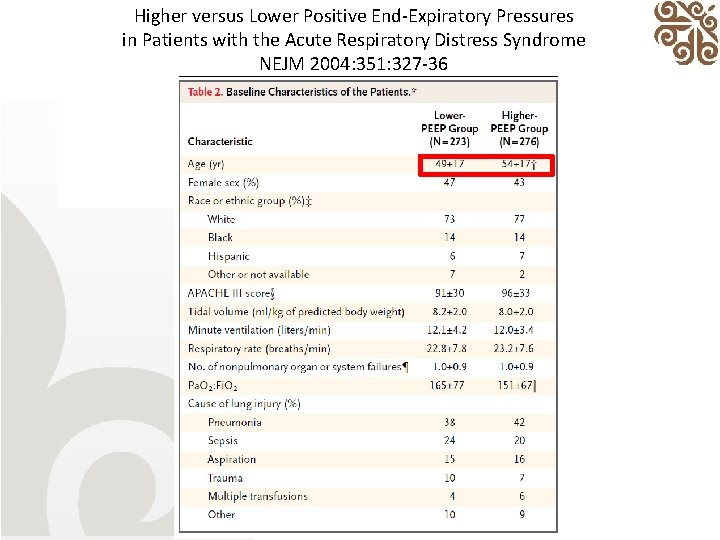
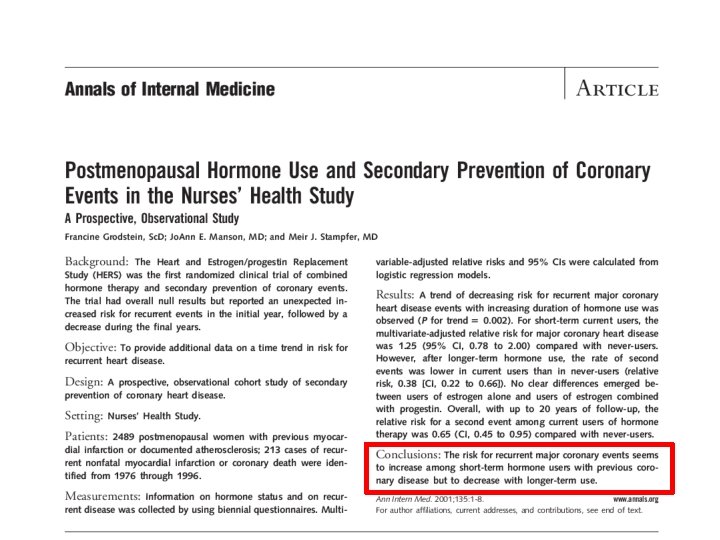
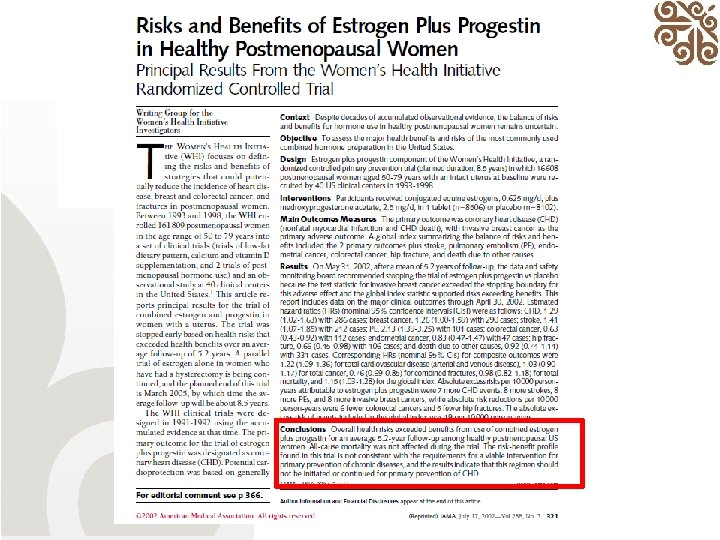
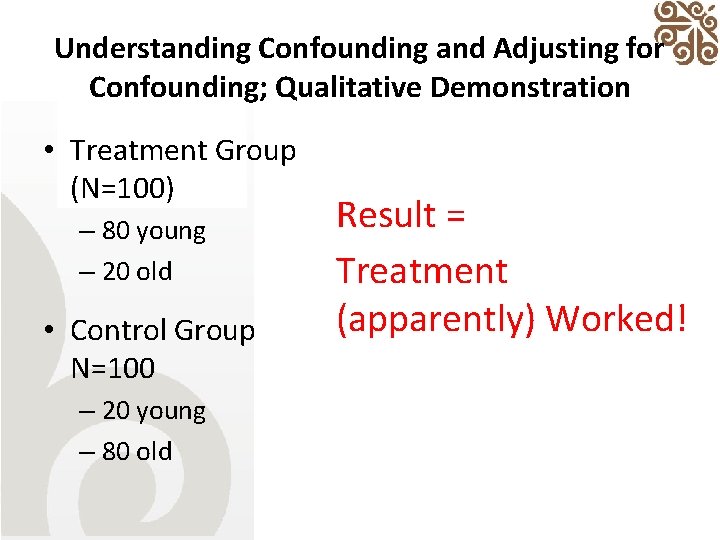
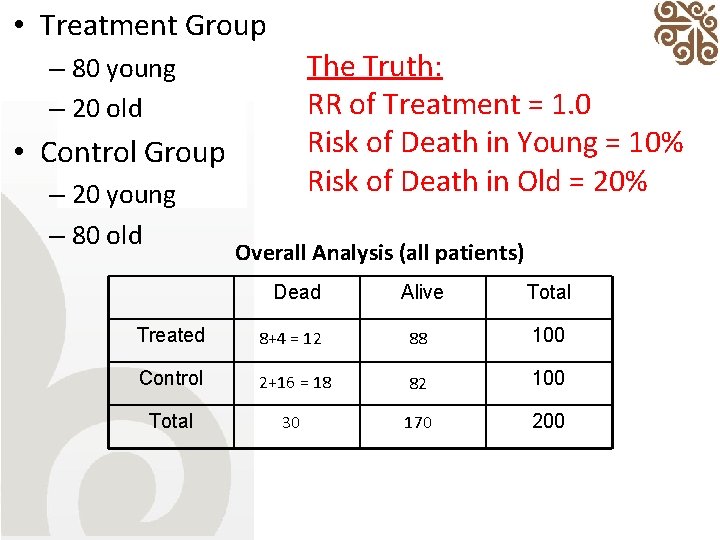
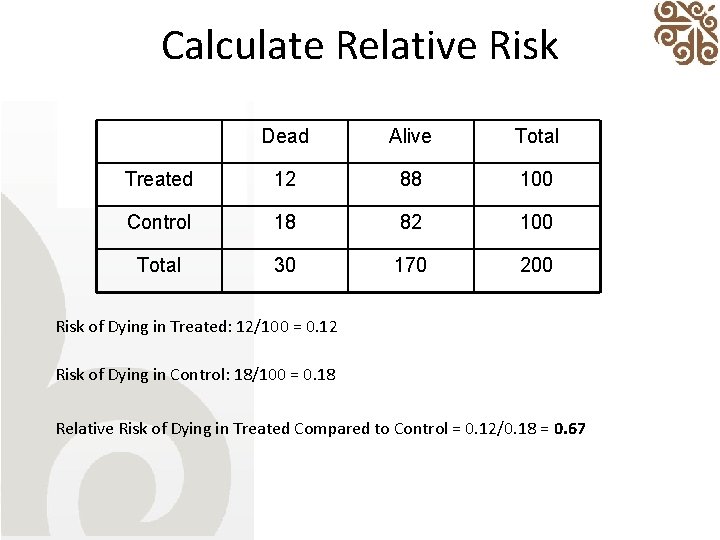
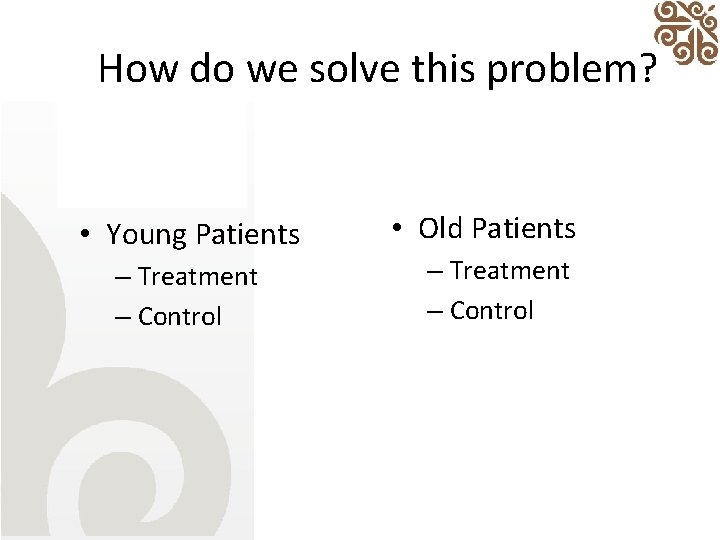
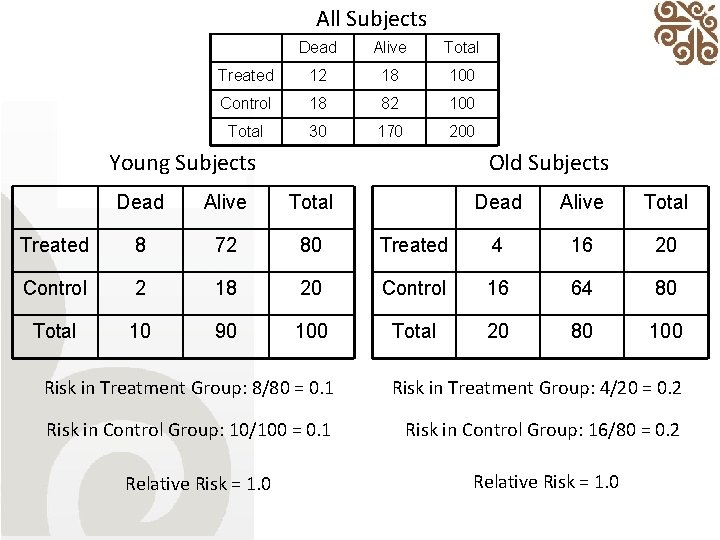
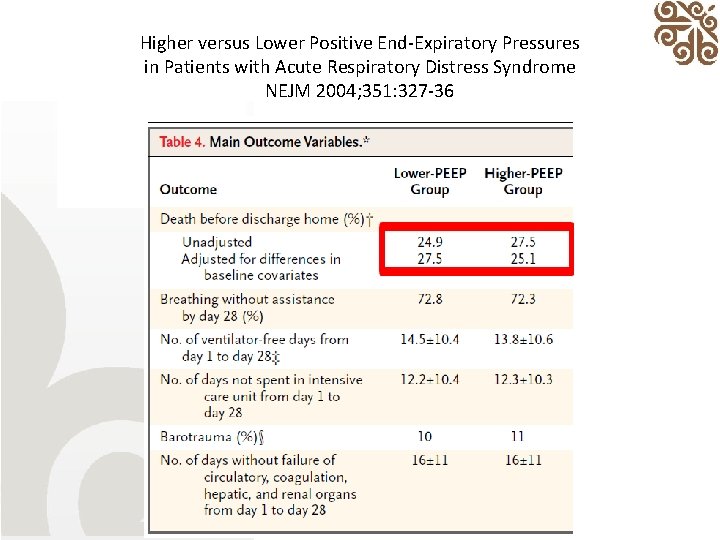
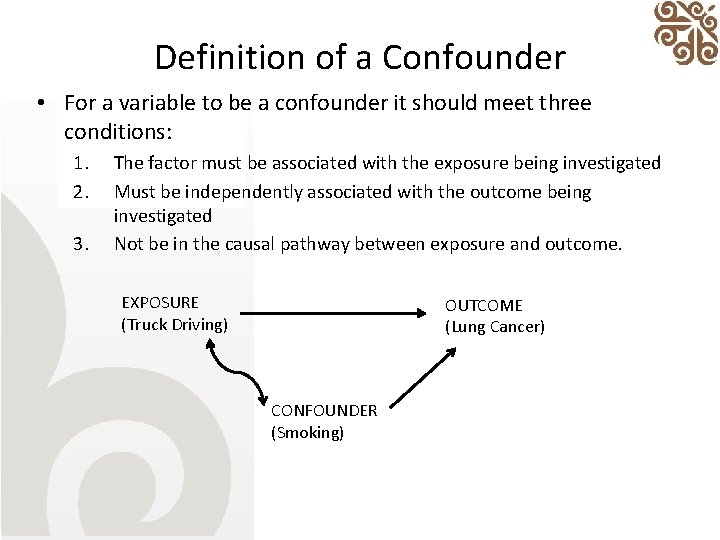
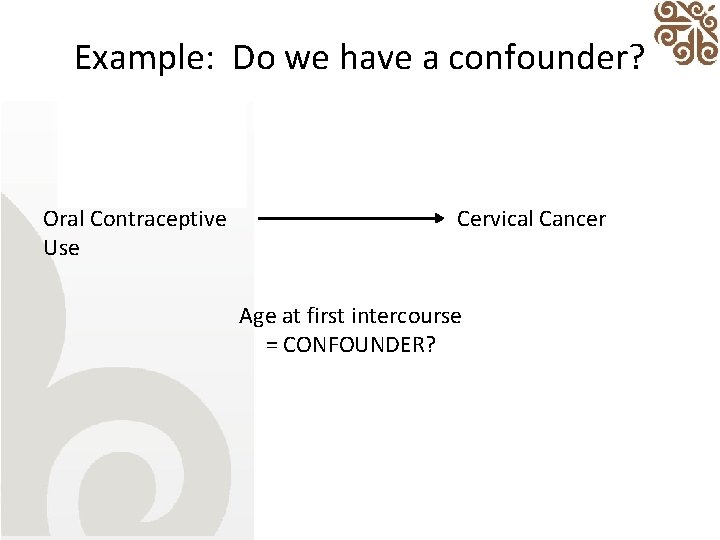
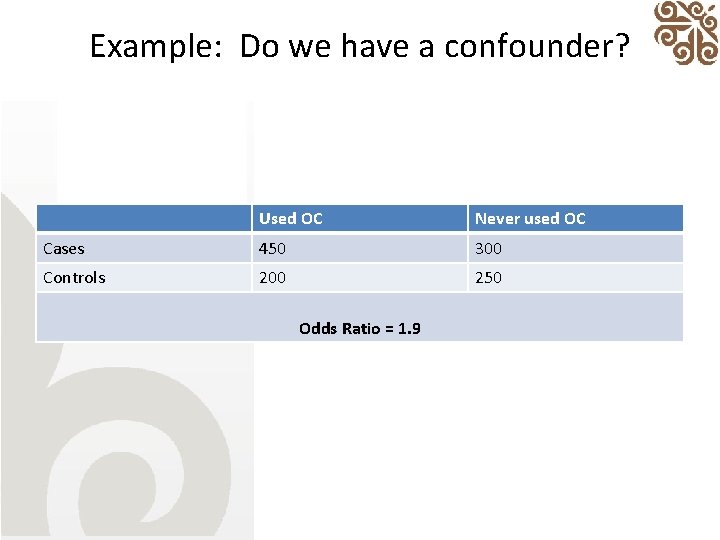
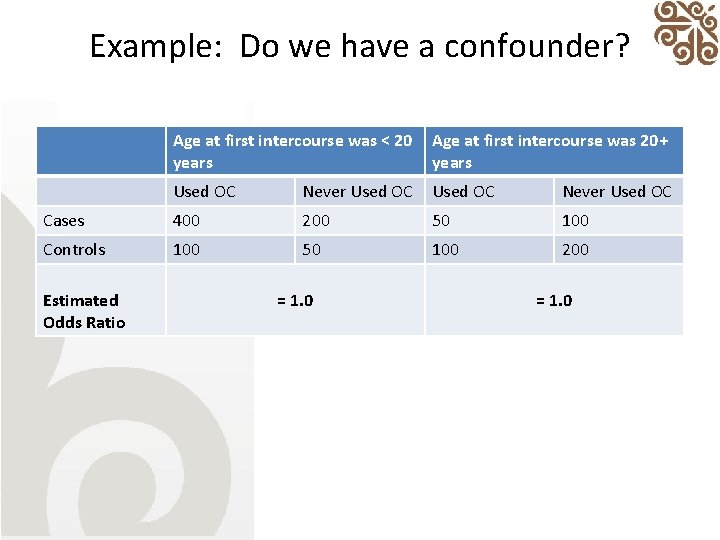
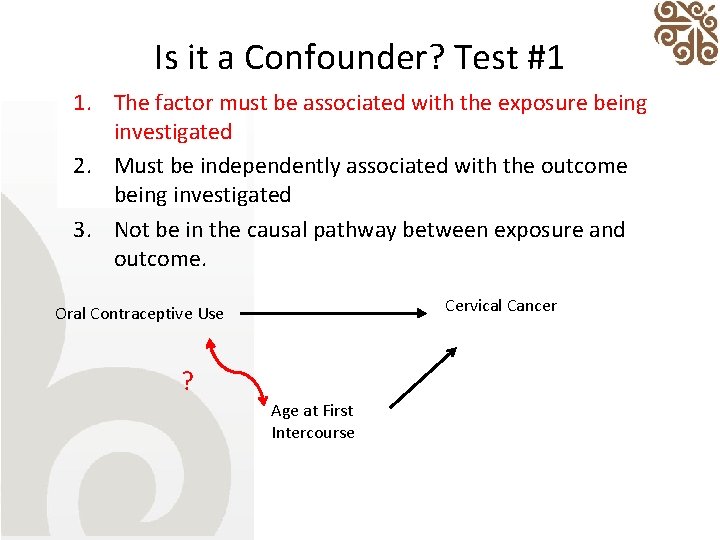
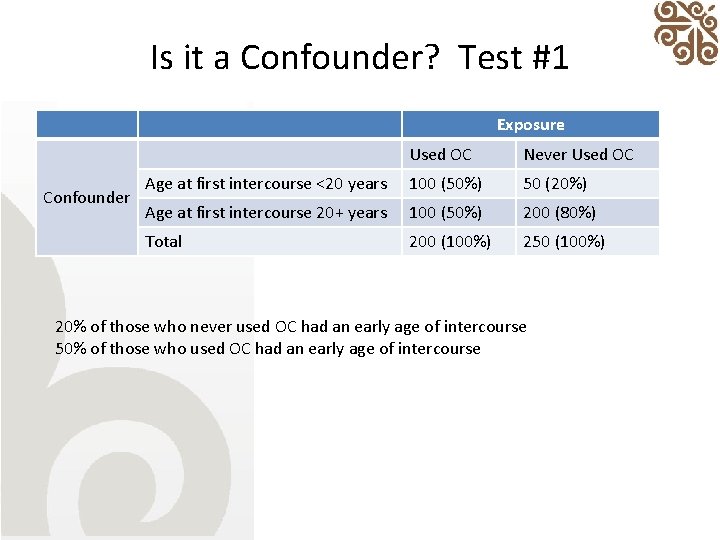
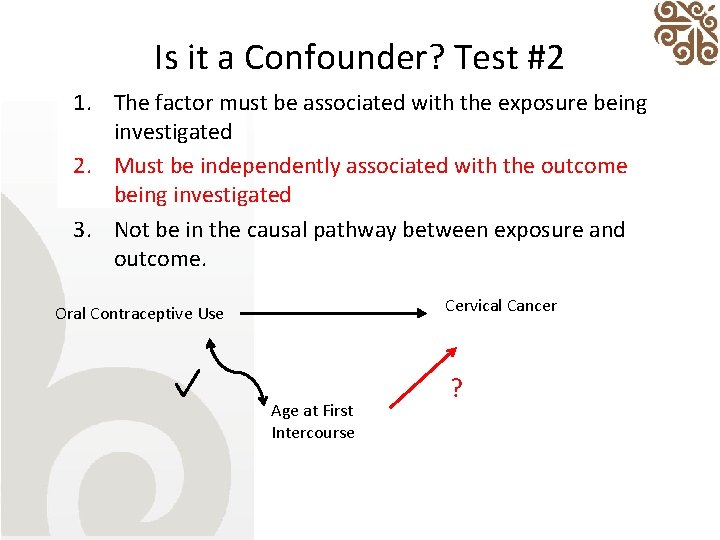
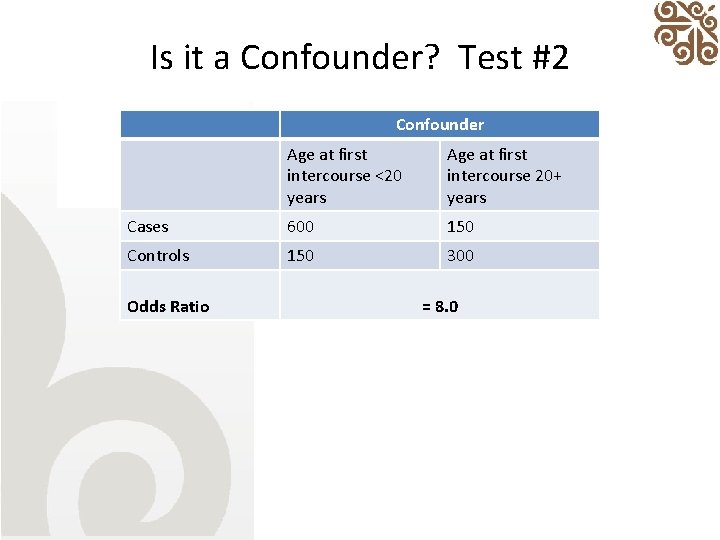
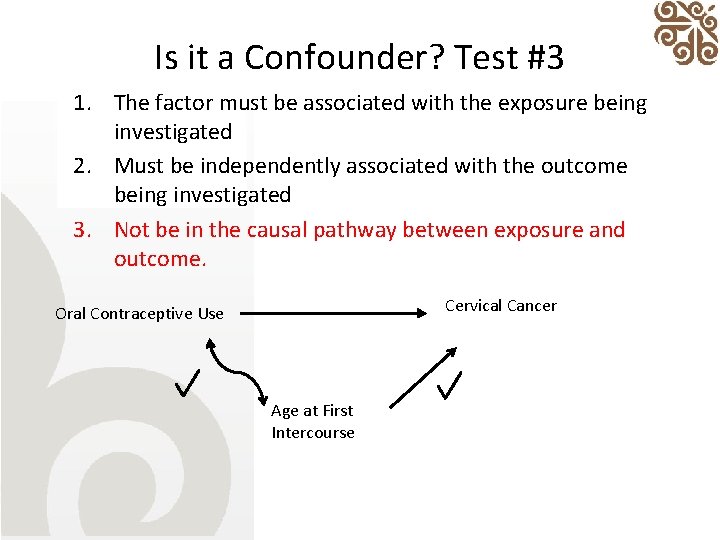
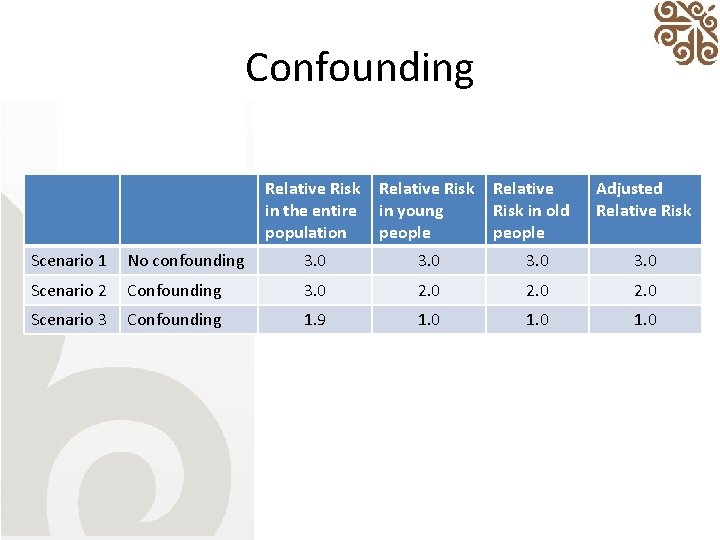
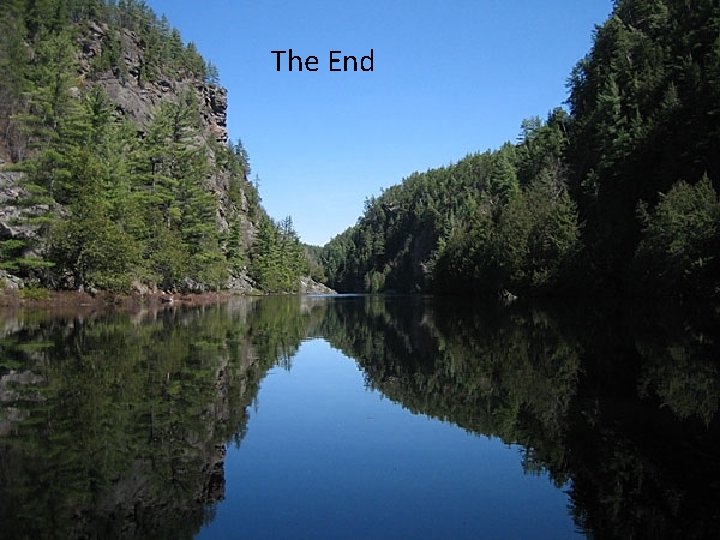
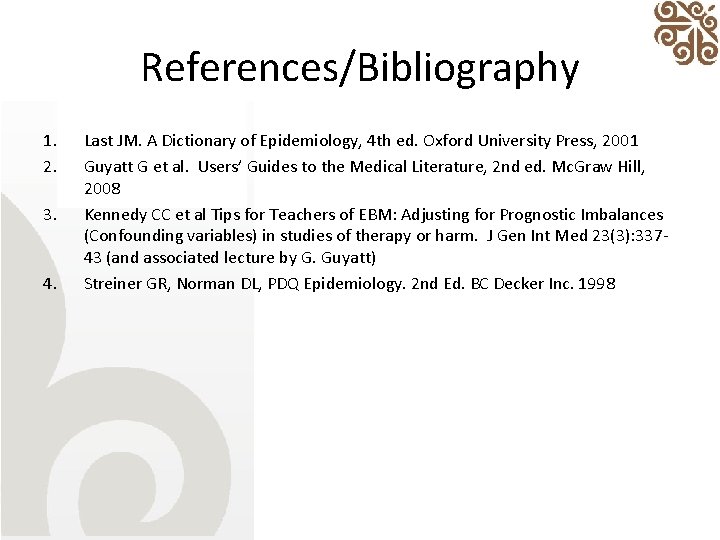
- Slides: 26
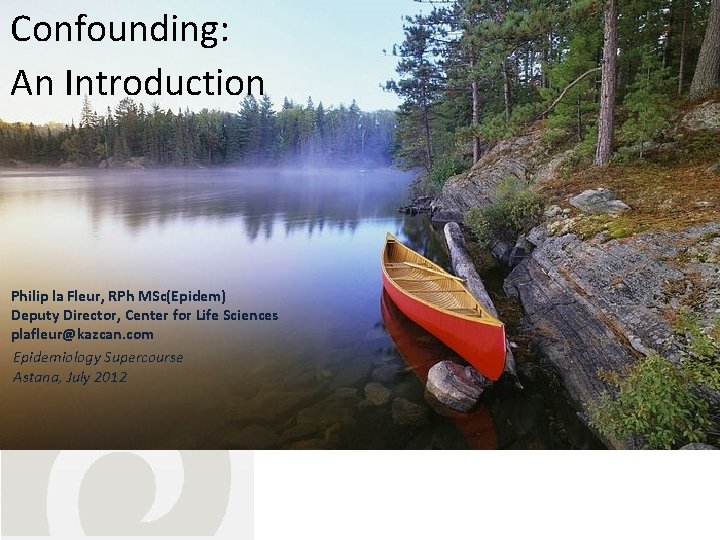
Confounding: An Introduction Philip la Fleur, RPh MSc(Epidem) Deputy Director, Center for Life Sciences plafleur@kazcan. com Epidemiology Supercourse Astana, July 2012
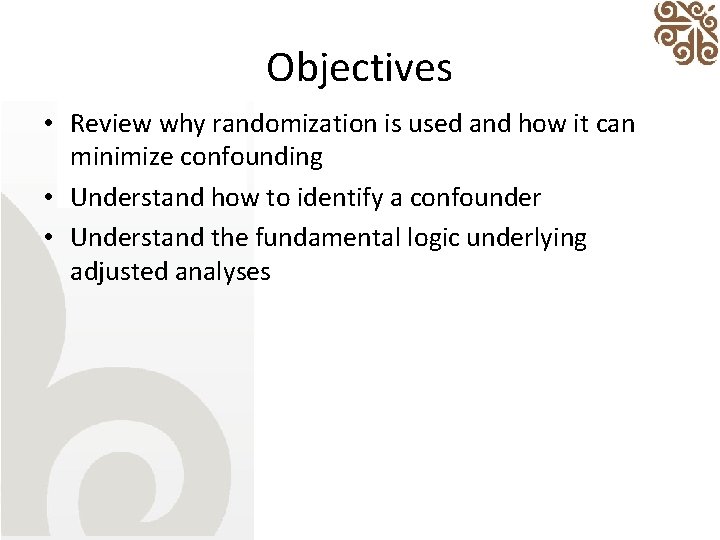
Objectives • Review why randomization is used and how it can minimize confounding • Understand how to identify a confounder • Understand the fundamental logic underlying adjusted analyses
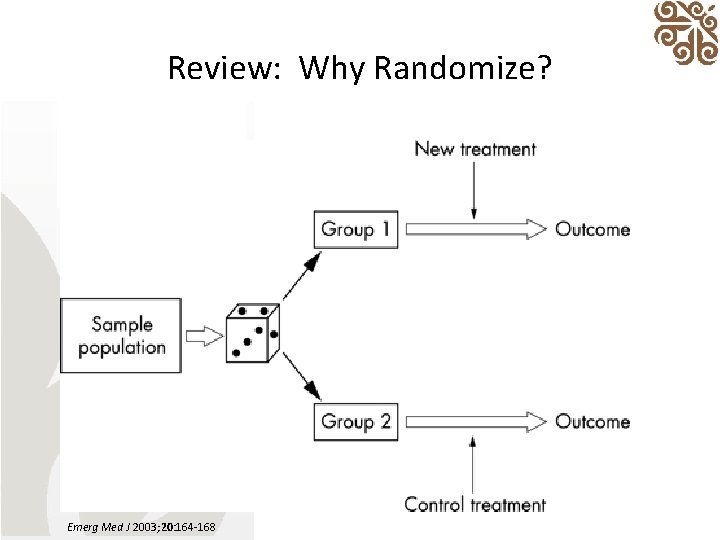
Review: Why Randomize? Emerg Med J 2003; 20: 164 -168
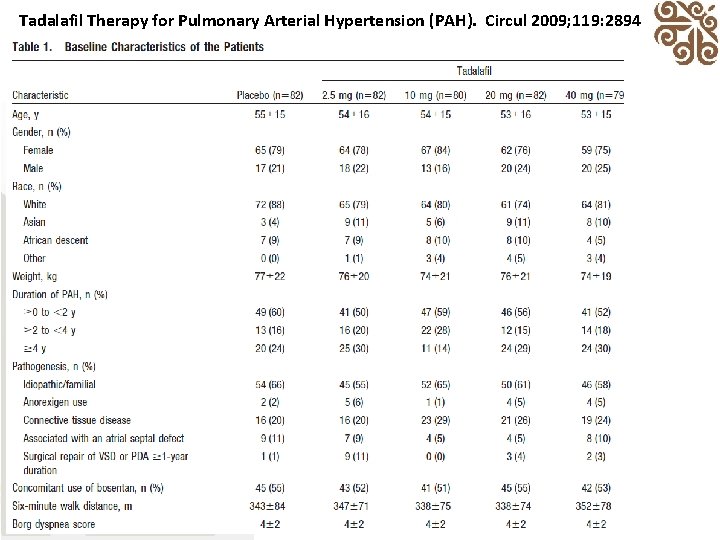
Tadalafil Therapy for Pulmonary Arterial Hypertension (PAH). Circul 2009; 119: 2894
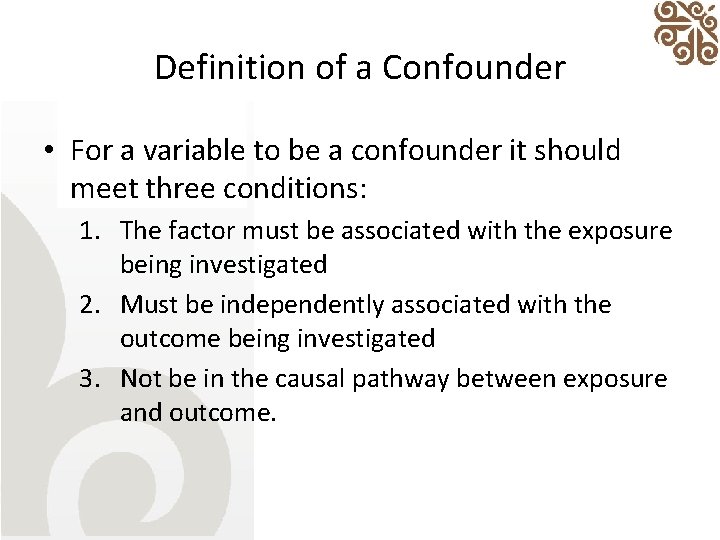
Definition of a Confounder • For a variable to be a confounder it should meet three conditions: 1. The factor must be associated with the exposure being investigated 2. Must be independently associated with the outcome being investigated 3. Not be in the causal pathway between exposure and outcome.
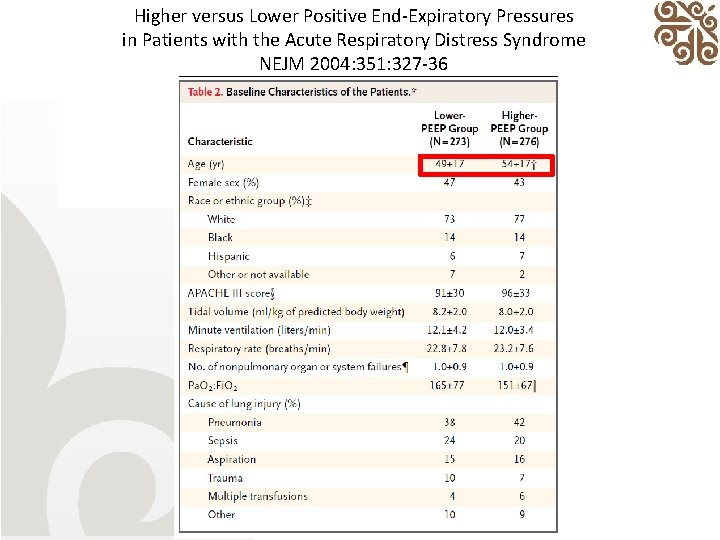
Higher versus Lower Positive End-Expiratory Pressures in Patients with the Acute Respiratory Distress Syndrome NEJM 2004; 351: 327 -36
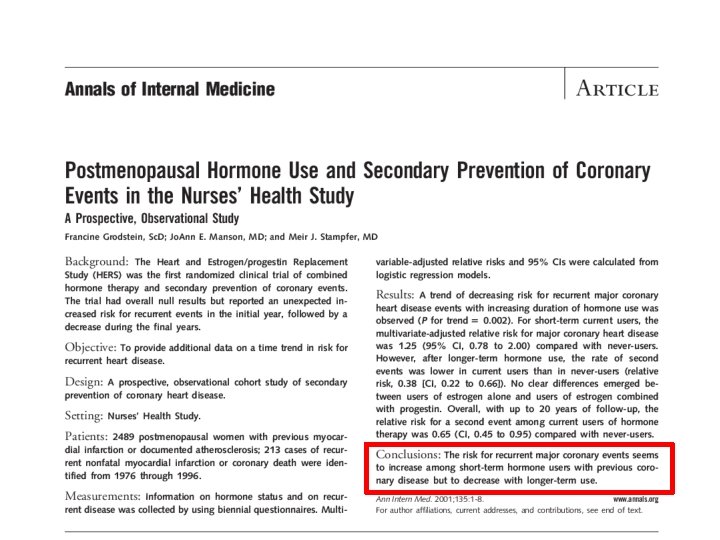
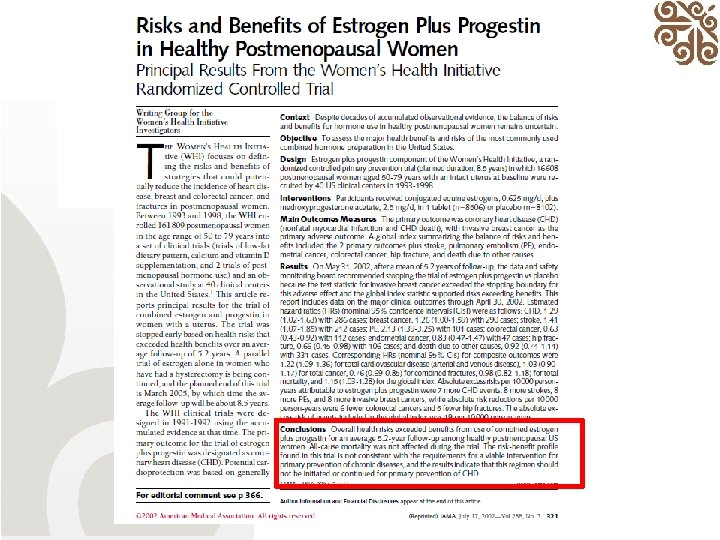
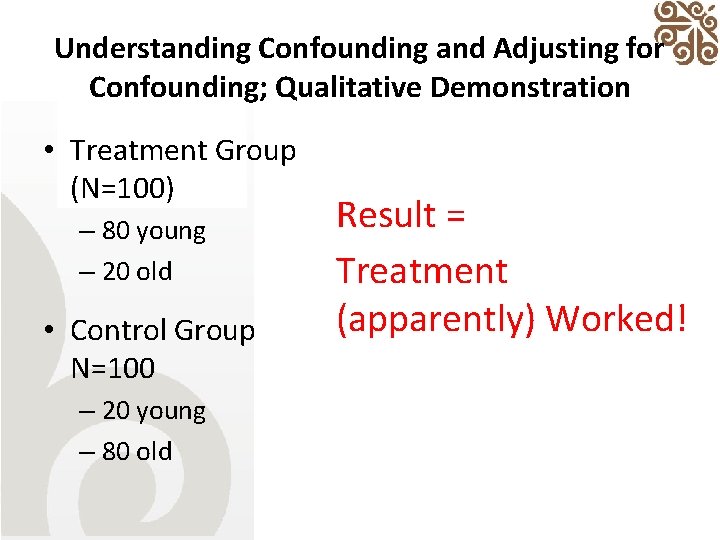
Understanding Confounding and Adjusting for Confounding; Qualitative Demonstration • Treatment Group (N=100) – 80 young – 20 old • Control Group N=100 – 20 young – 80 old Result = Treatment (apparently) Worked!
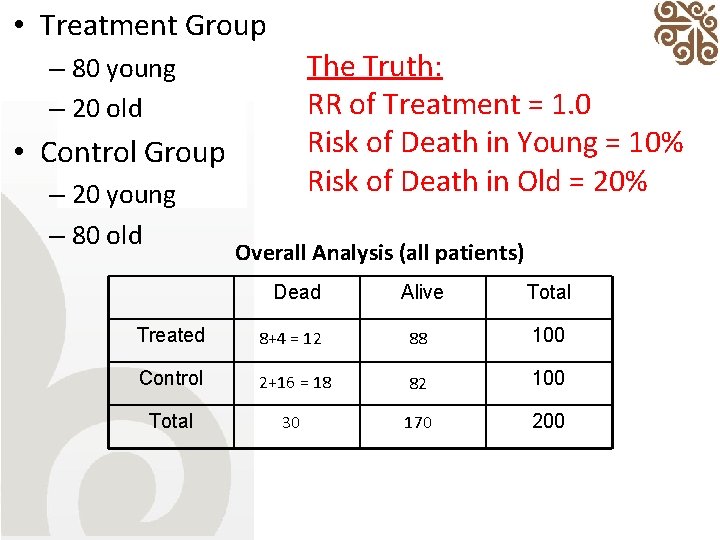
• Treatment Group The Truth: RR of Treatment = 1. 0 Risk of Death in Young = 10% Risk of Death in Old = 20% – 80 young – 20 old • Control Group – 20 young – 80 old Overall Analysis (all patients) Dead Alive Total Treated 8+4 = 12 88 100 Control 2+16 = 18 82 100 Total 30 170 200
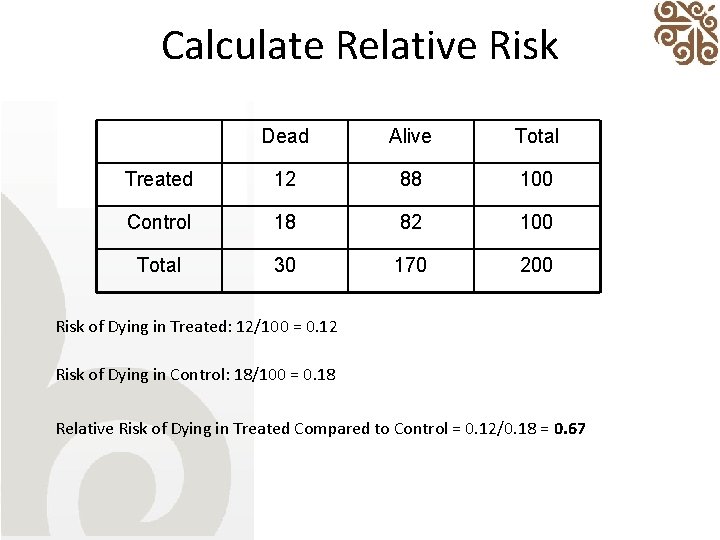
Calculate Relative Risk Dead Alive Total Treated 12 88 100 Control 18 82 100 Total 30 170 200 Risk of Dying in Treated: 12/100 = 0. 12 Risk of Dying in Control: 18/100 = 0. 18 Relative Risk of Dying in Treated Compared to Control = 0. 12/0. 18 = 0. 67
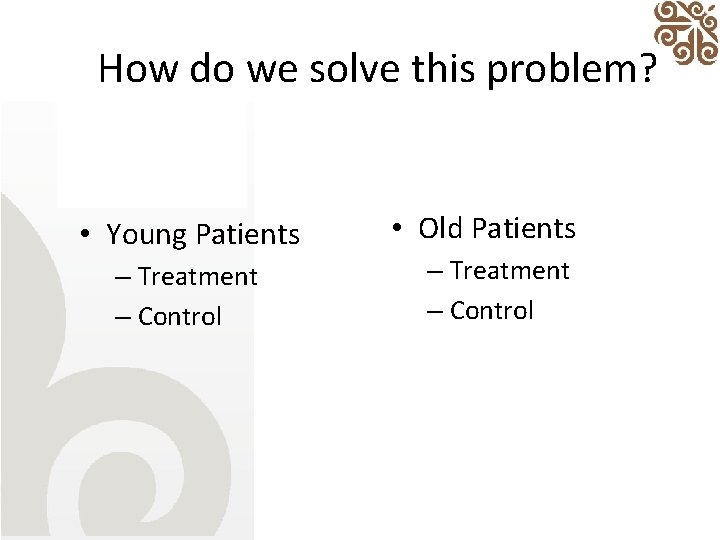
How do we solve this problem? • Young Patients – Treatment – Control • Old Patients – Treatment – Control
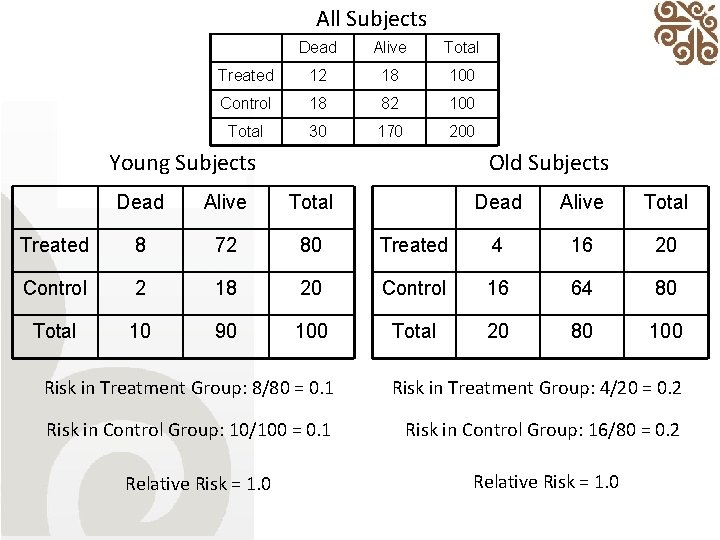
All Subjects Dead Alive Total Treated 12 18 100 Control 18 82 100 Total 30 170 200 Young Subjects Old Subjects Dead Alive Total Treated 8 72 80 Treated 4 16 20 Control 2 18 20 Control 16 64 80 Total 10 90 100 Total 20 80 100 Risk in Treatment Group: 8/80 = 0. 1 Risk in Treatment Group: 4/20 = 0. 2 Risk in Control Group: 10/100 = 0. 1 Risk in Control Group: 16/80 = 0. 2 Relative Risk = 1. 0
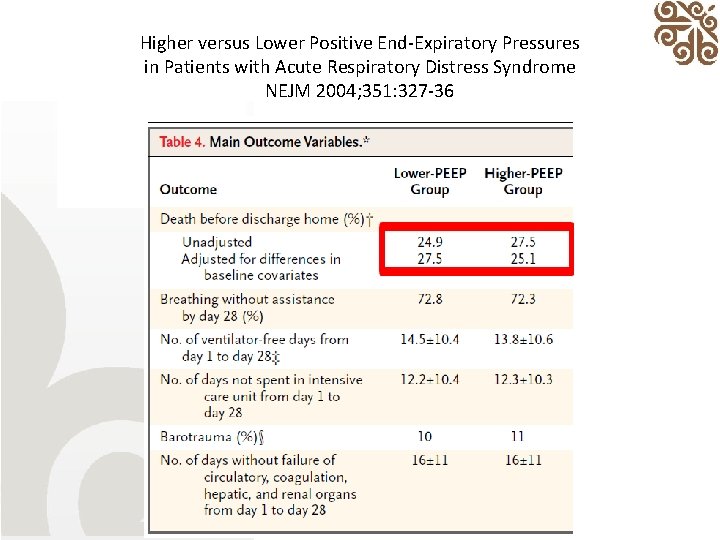
Higher versus Lower Positive End-Expiratory Pressures in Patients with Acute Respiratory Distress Syndrome NEJM 2004; 351: 327 -36
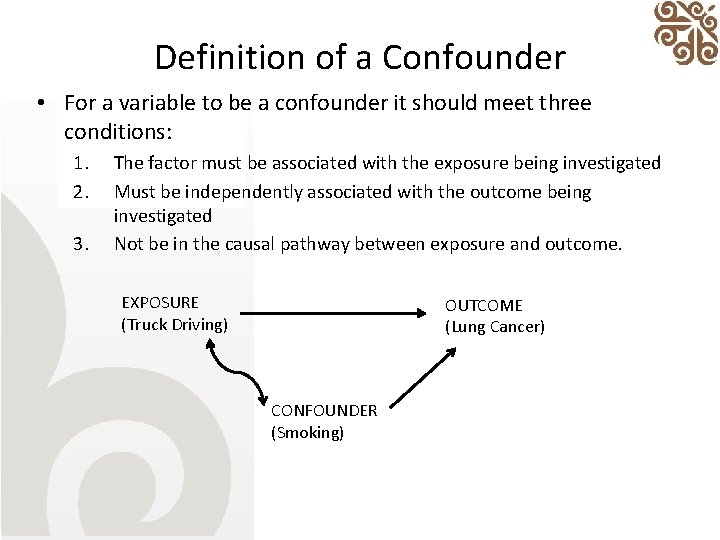
Definition of a Confounder • For a variable to be a confounder it should meet three conditions: 1. 2. 3. The factor must be associated with the exposure being investigated Must be independently associated with the outcome being investigated Not be in the causal pathway between exposure and outcome. EXPOSURE (Truck Driving) OUTCOME (Lung Cancer) CONFOUNDER (Smoking)
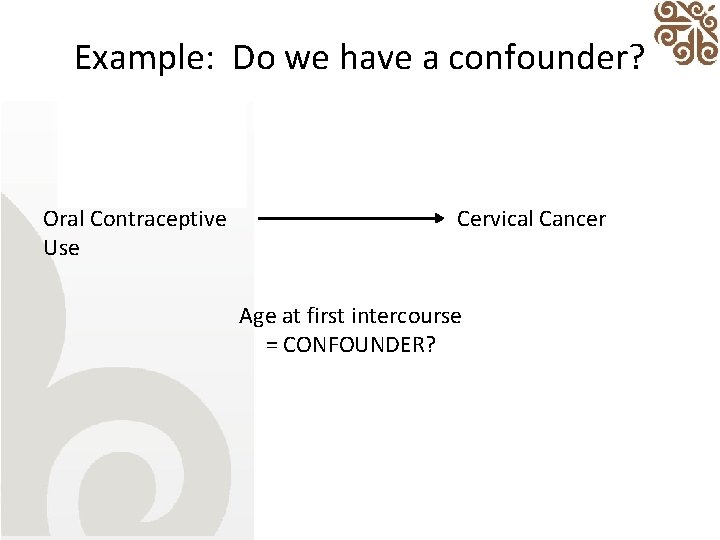
Example: Do we have a confounder? Oral Contraceptive Use Cervical Cancer Age at first intercourse = CONFOUNDER?
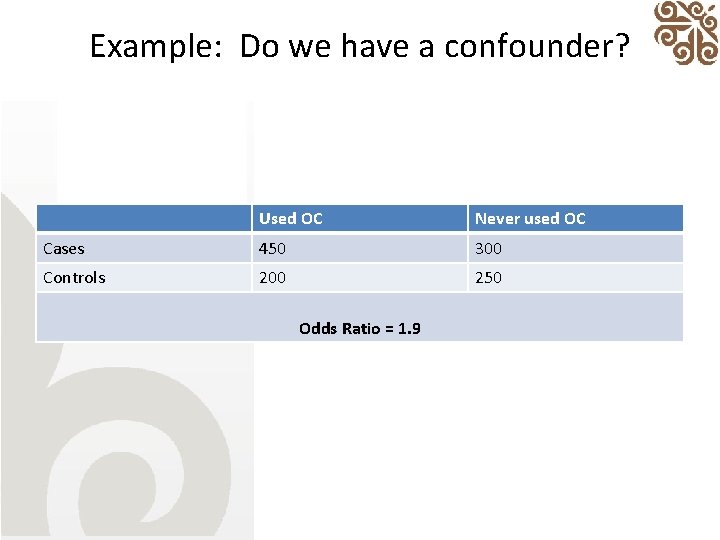
Example: Do we have a confounder? Used OC Never used OC Cases 450 300 Controls 200 250 Odds Ratio = 1. 9
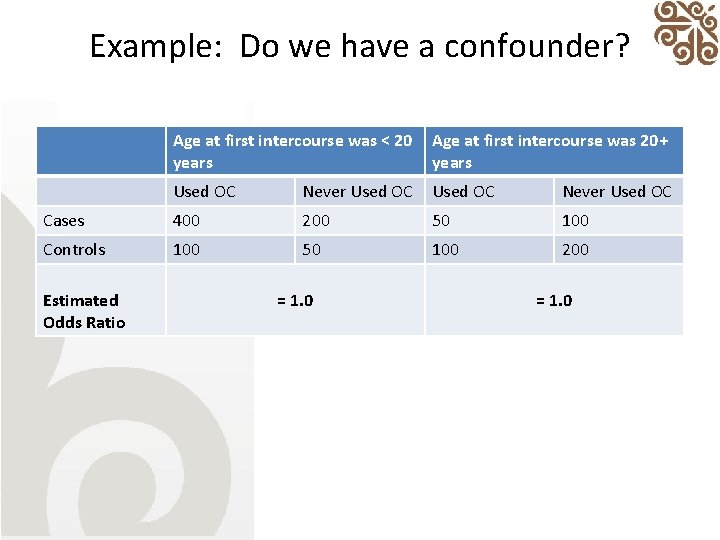
Example: Do we have a confounder? Age at first intercourse was < 20 years Age at first intercourse was 20+ years Used OC Never Used OC Cases 400 200 50 100 Controls 100 50 100 200 Estimated Odds Ratio = 1. 0
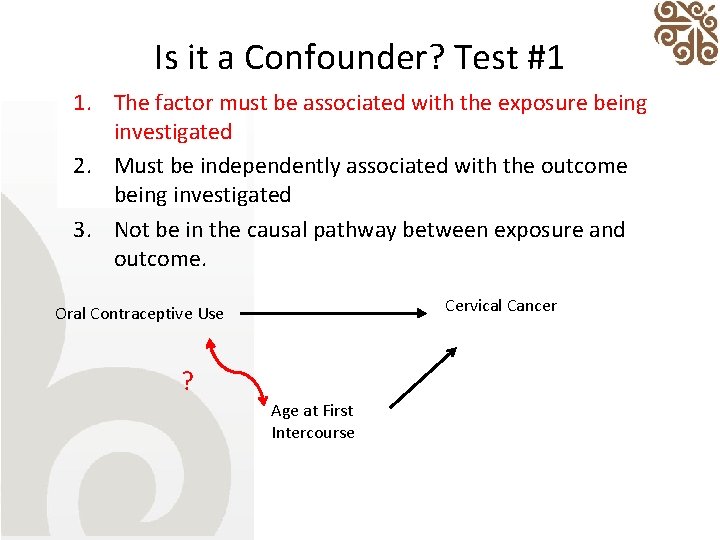
Is it a Confounder? Test #1 1. The factor must be associated with the exposure being investigated 2. Must be independently associated with the outcome being investigated 3. Not be in the causal pathway between exposure and outcome. Cervical Cancer Oral Contraceptive Use ? Age at First Intercourse
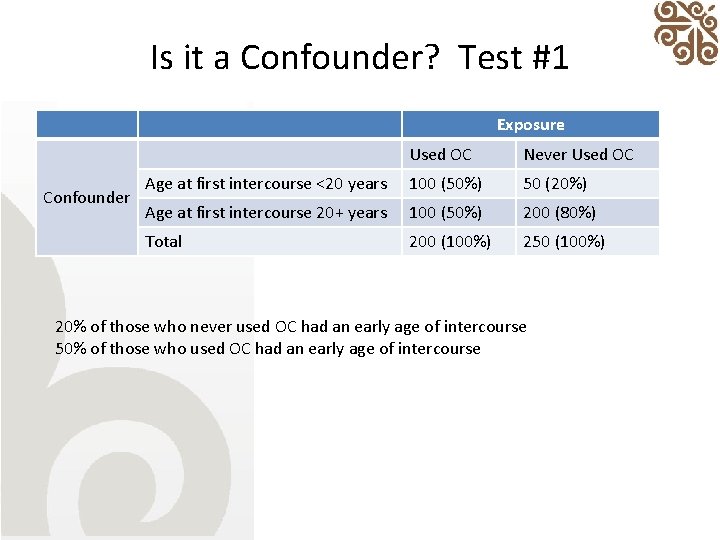
Is it a Confounder? Test #1 Exposure Confounder Used OC Never Used OC Age at first intercourse <20 years 100 (50%) 50 (20%) Age at first intercourse 20+ years 100 (50%) 200 (80%) Total 200 (100%) 250 (100%) 20% of those who never used OC had an early age of intercourse 50% of those who used OC had an early age of intercourse
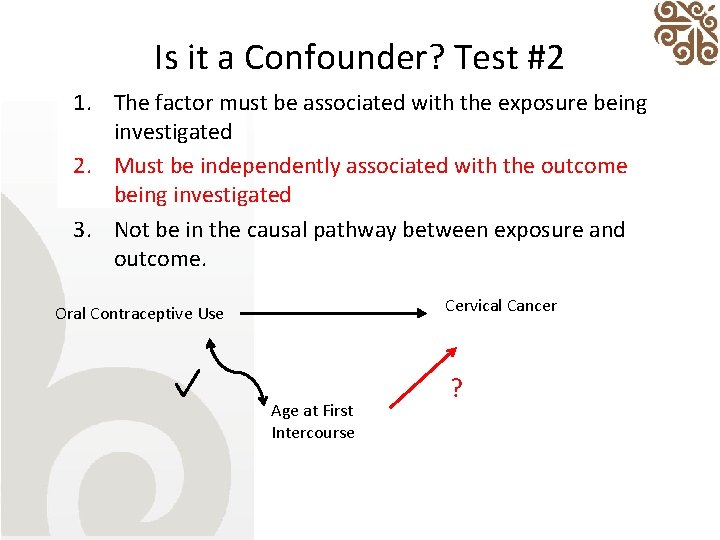
Is it a Confounder? Test #2 1. The factor must be associated with the exposure being investigated 2. Must be independently associated with the outcome being investigated 3. Not be in the causal pathway between exposure and outcome. Cervical Cancer Oral Contraceptive Use Age at First Intercourse ?
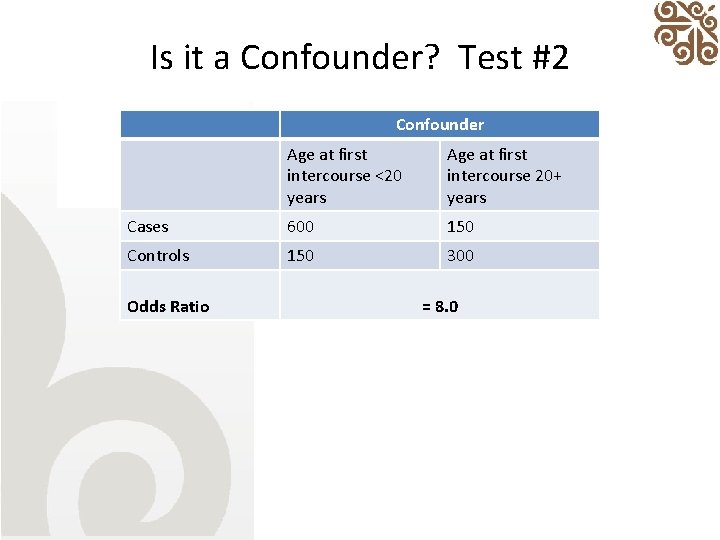
Is it a Confounder? Test #2 Confounder Age at first intercourse <20 years Age at first intercourse 20+ years Cases 600 150 Controls 150 300 Odds Ratio = 8. 0
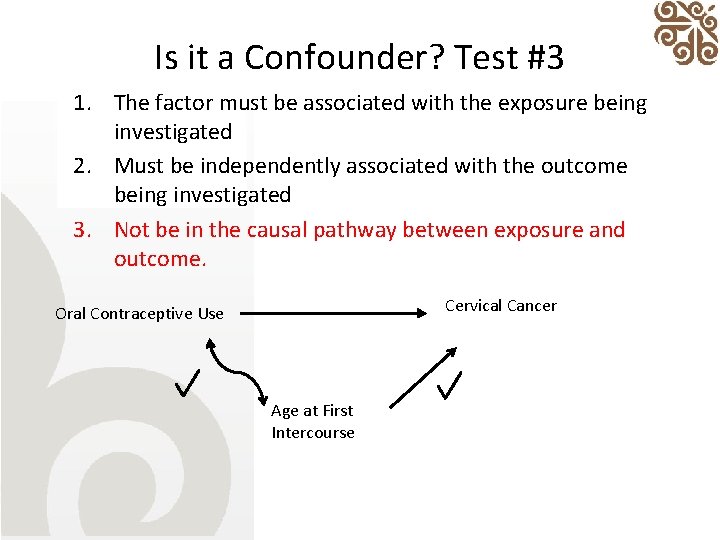
Is it a Confounder? Test #3 1. The factor must be associated with the exposure being investigated 2. Must be independently associated with the outcome being investigated 3. Not be in the causal pathway between exposure and outcome. Cervical Cancer Oral Contraceptive Use Age at First Intercourse
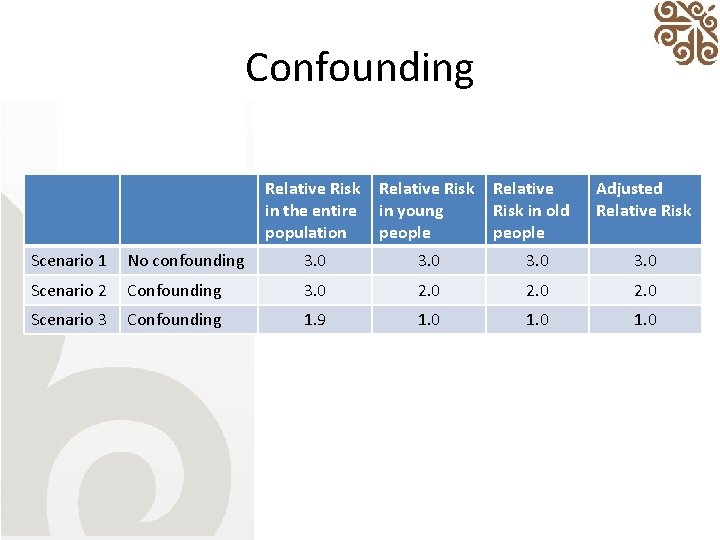
Confounding Relative Risk in the entire population Relative Risk in young people Relative Risk in old people Adjusted Relative Risk Scenario 1 No confounding 3. 0 Scenario 2 Confounding 3. 0 2. 0 Scenario 3 Confounding 1. 9 1. 0
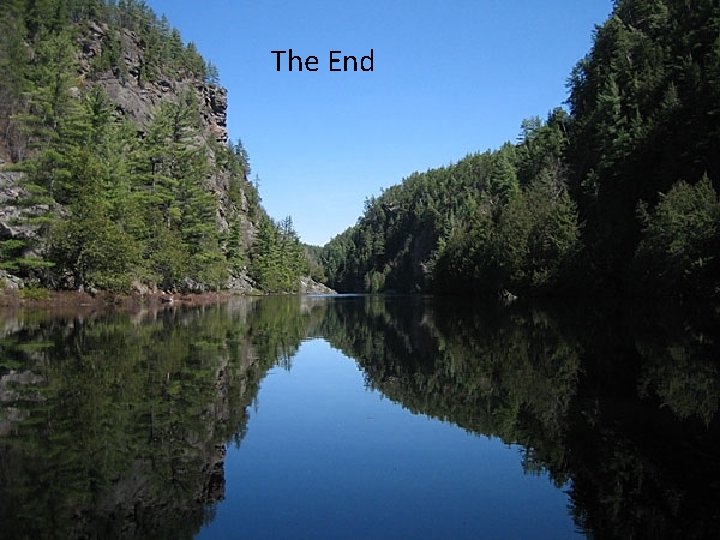
The End
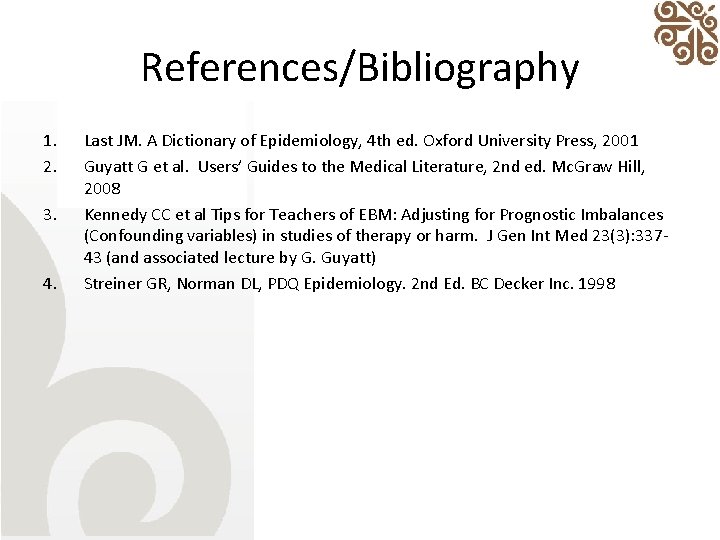
References/Bibliography 1. 2. 3. 4. Last JM. A Dictionary of Epidemiology, 4 th ed. Oxford University Press, 2001 Guyatt G et al. Users’ Guides to the Medical Literature, 2 nd ed. Mc. Graw Hill, 2008 Kennedy CC et al Tips for Teachers of EBM: Adjusting for Prognostic Imbalances (Confounding variables) in studies of therapy or harm. J Gen Int Med 23(3): 33743 (and associated lecture by G. Guyatt) Streiner GR, Norman DL, PDQ Epidemiology. 2 nd Ed. BC Decker Inc. 1998
How to control for confounding variables in regression
What are extraneous variables
Effect modification vs confounding
Effect modifier vs confounder
What is extraneous variables in research
Confounding variables in an experiment
How to control for confounding
Confounding vs effect modification
Effect modification vs confounding
Effect modification vs confounding
Confounding vs effect modification
Confounding variable psychology
What is a confounding variable example
How to control for confounding
Obscuring variable
Misclassification bias
Henri matisse fleur
J'ai cueilli cette fleur pour toi sur la colline
La fleur de l'estime de soi
Fleur onrust
Arriver comme une fleur expression
Se pointer comme une fleur
Fleur claessens
Golf le fleur pronunciation
Comme une belle fleur assise entre les fleurs
Fleur emblème île maurice
La fleur jalousie