Clustered data grids Aksel Thomsen Erik Sommer Clustered
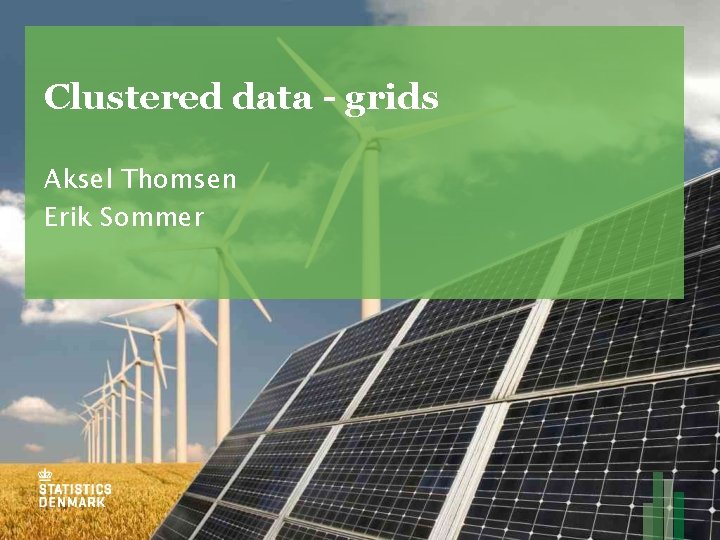
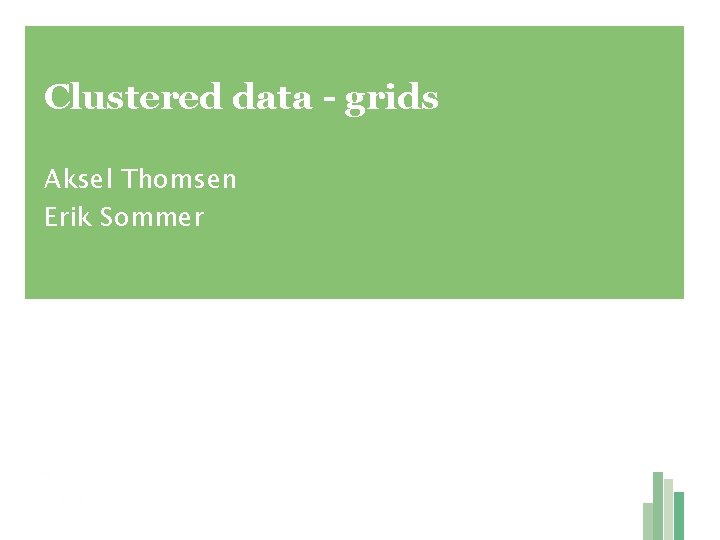
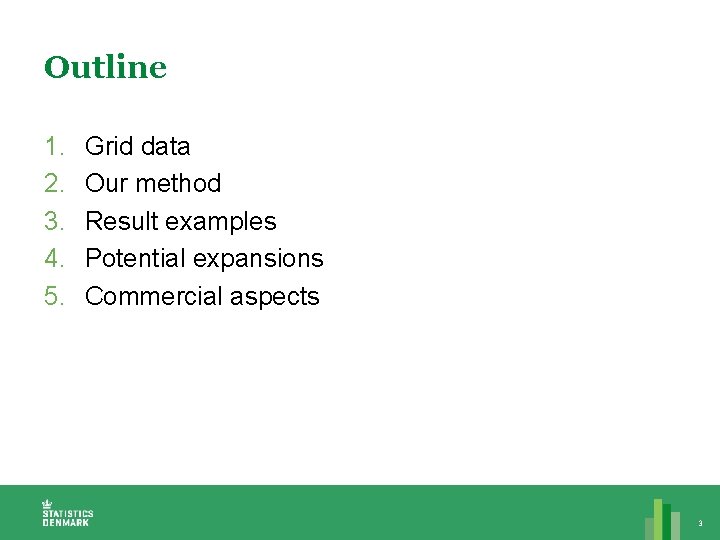
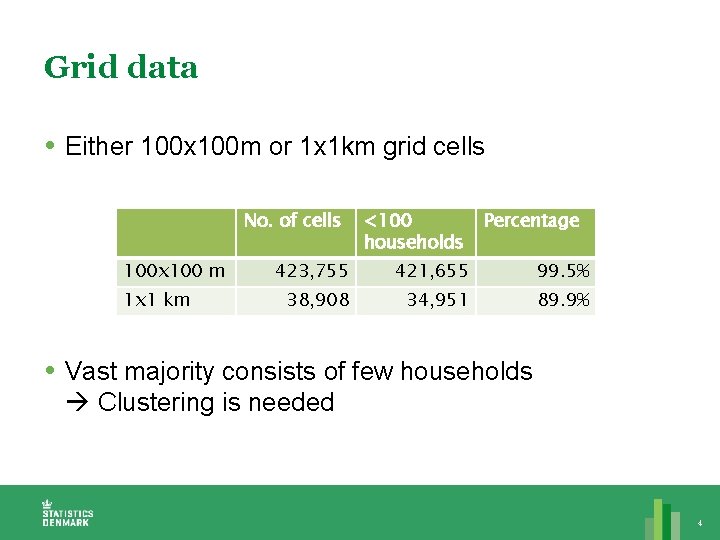
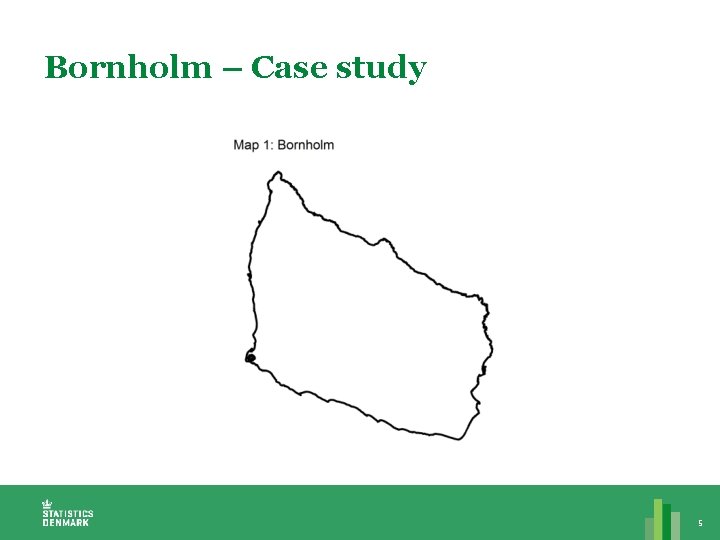
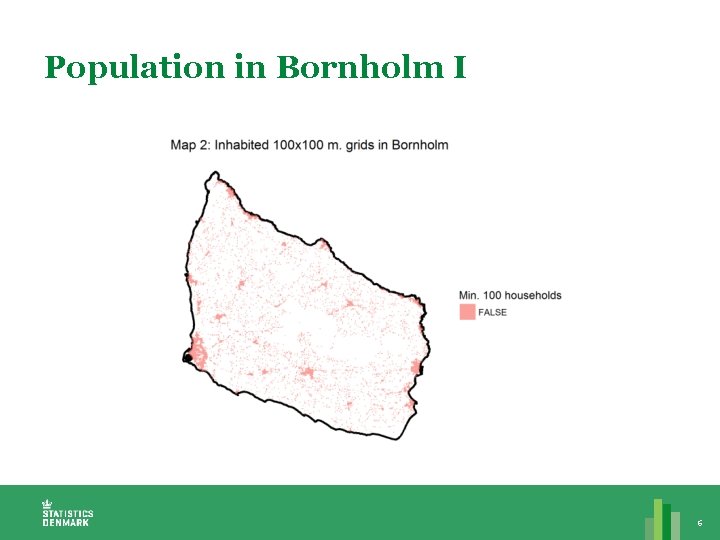
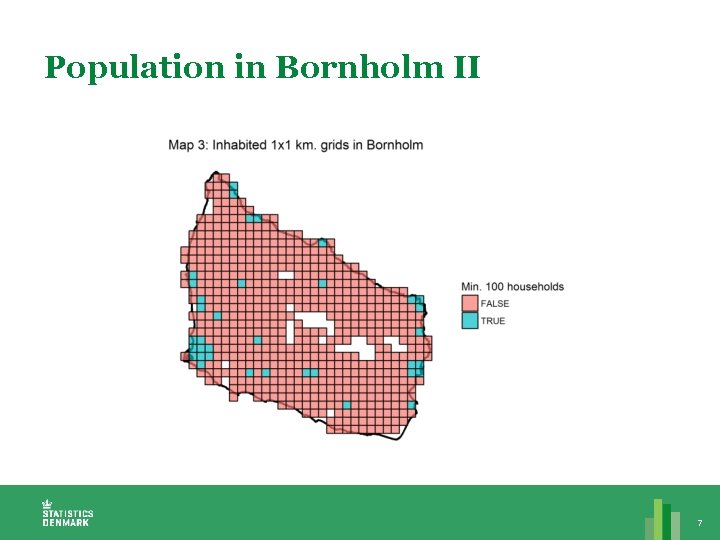
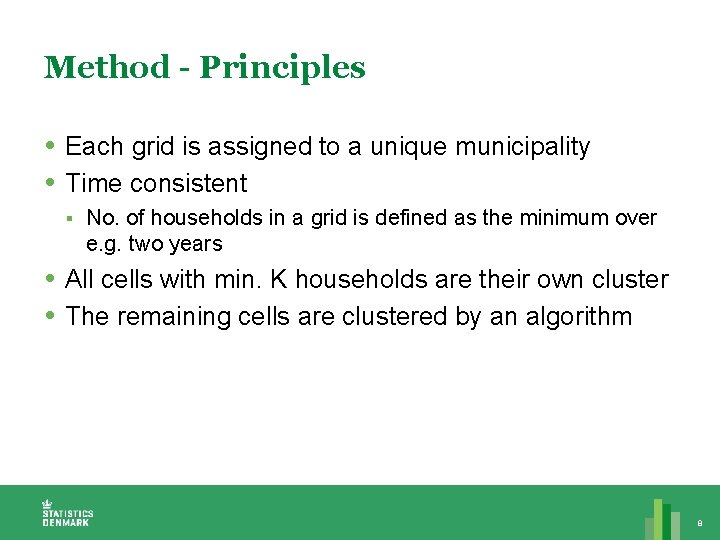
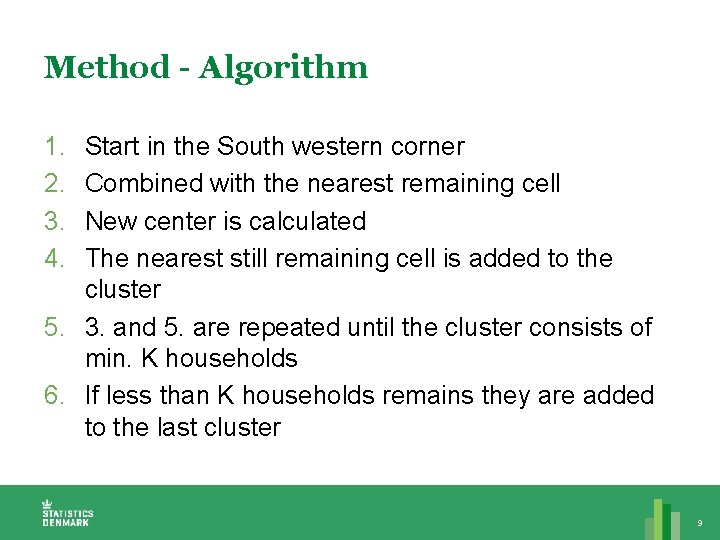
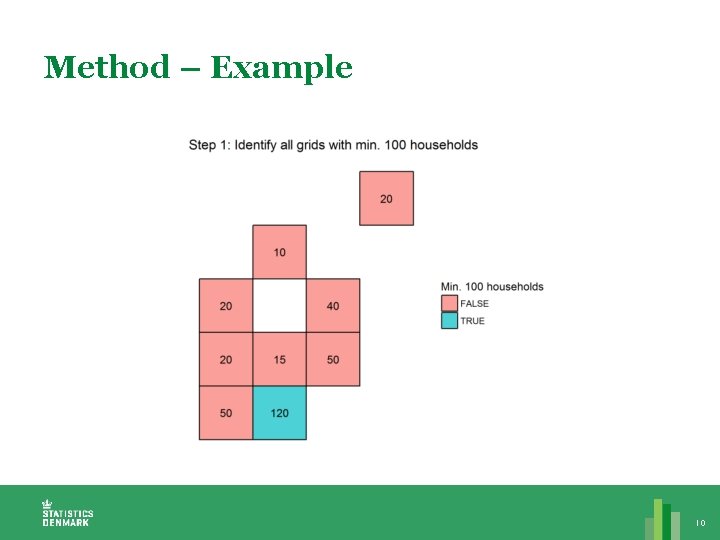
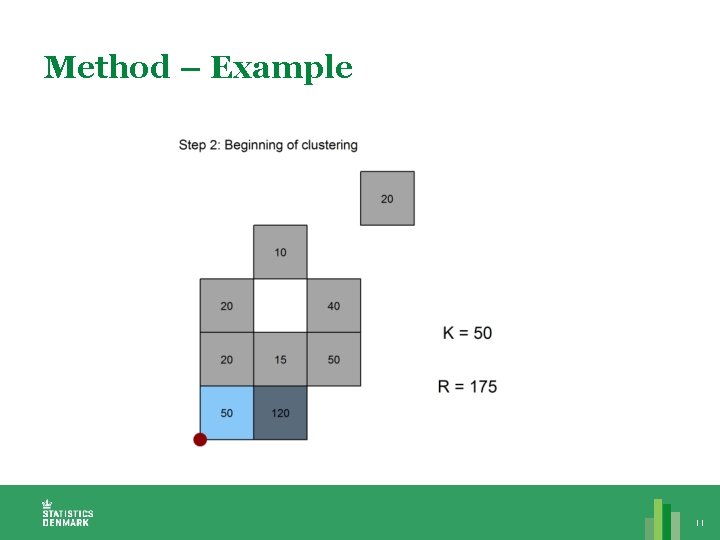
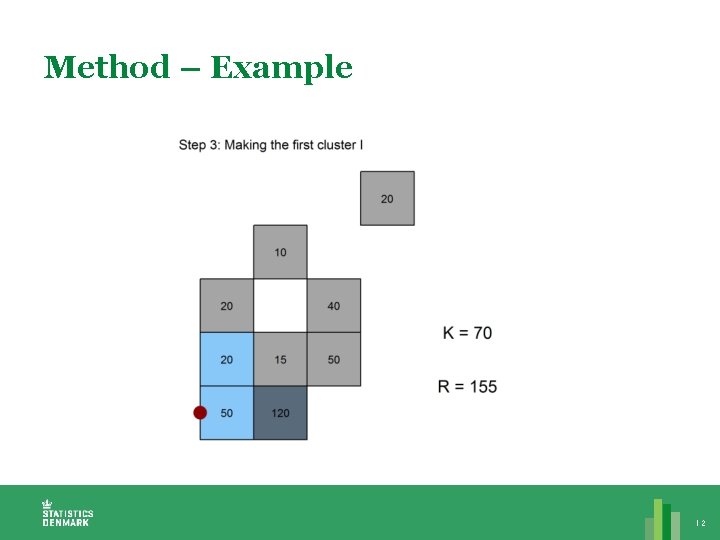
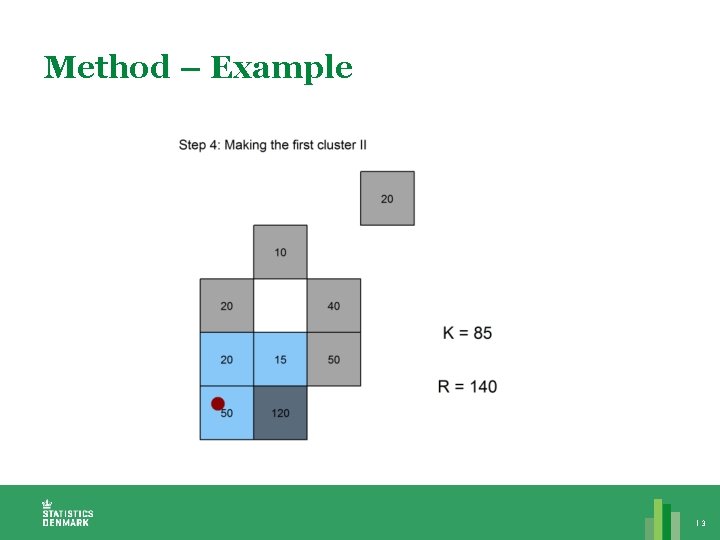
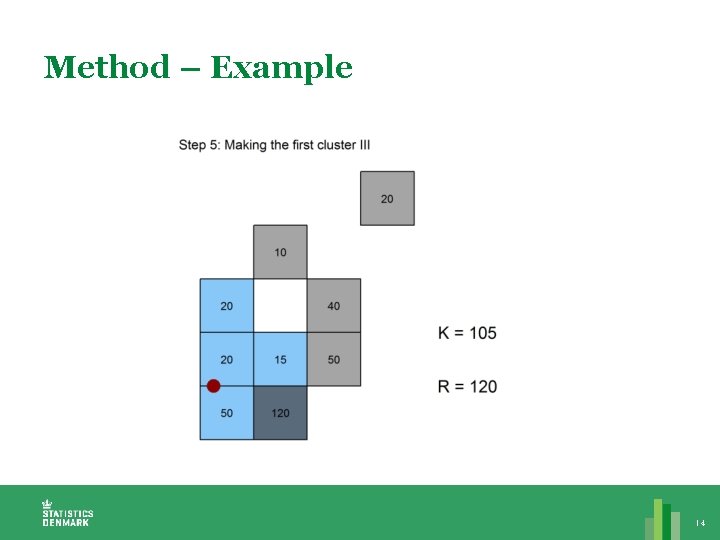
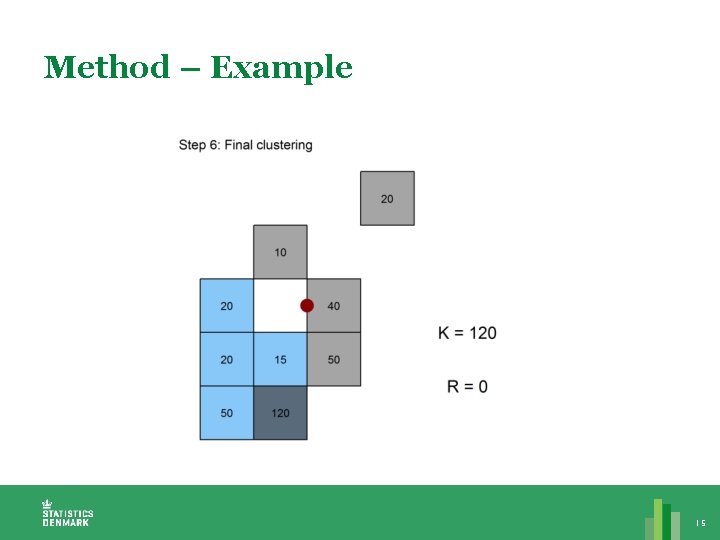
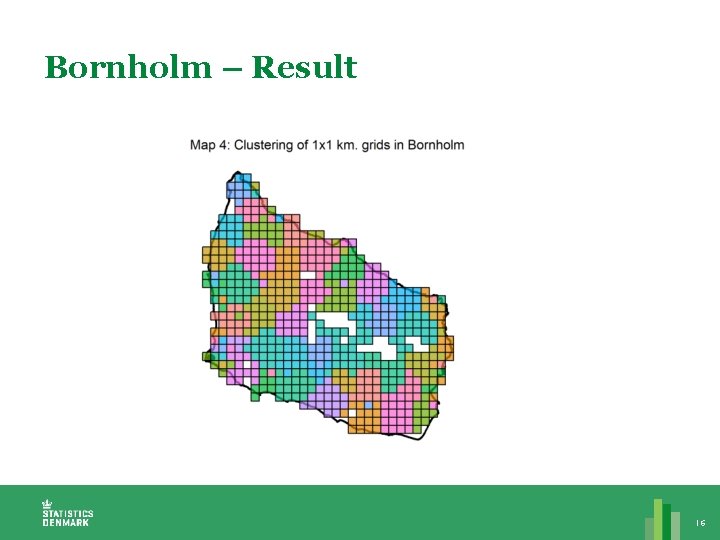
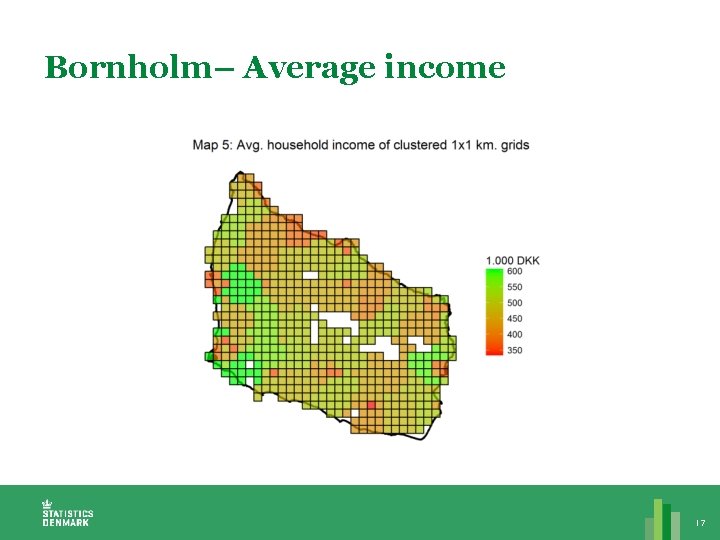
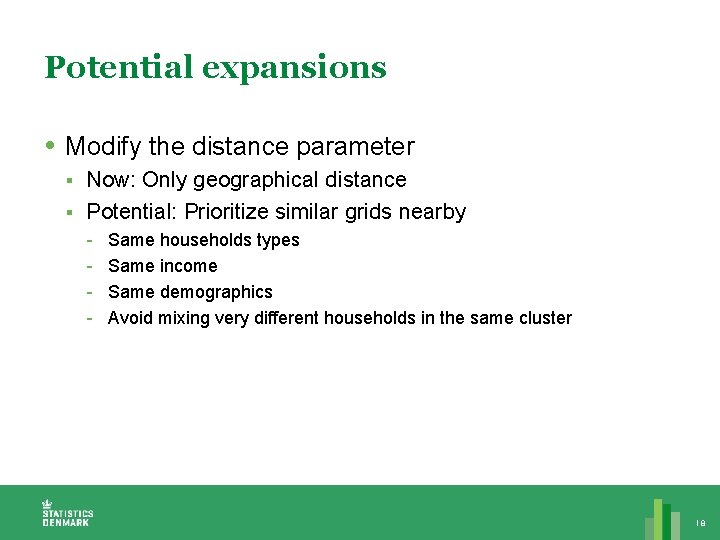
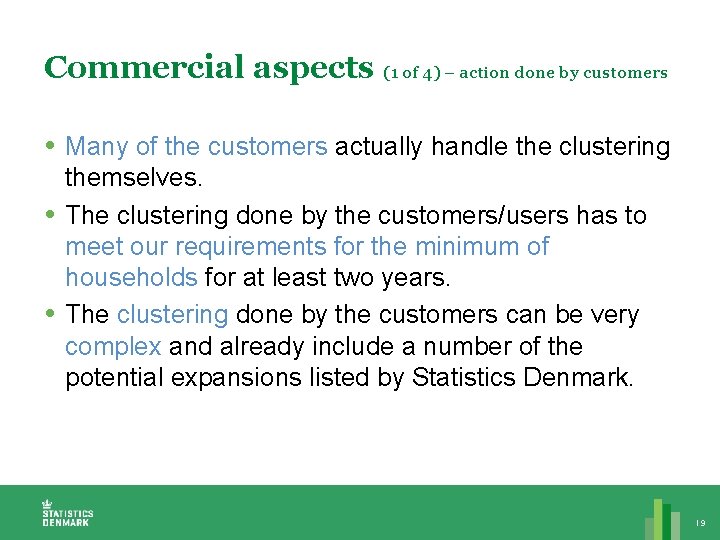
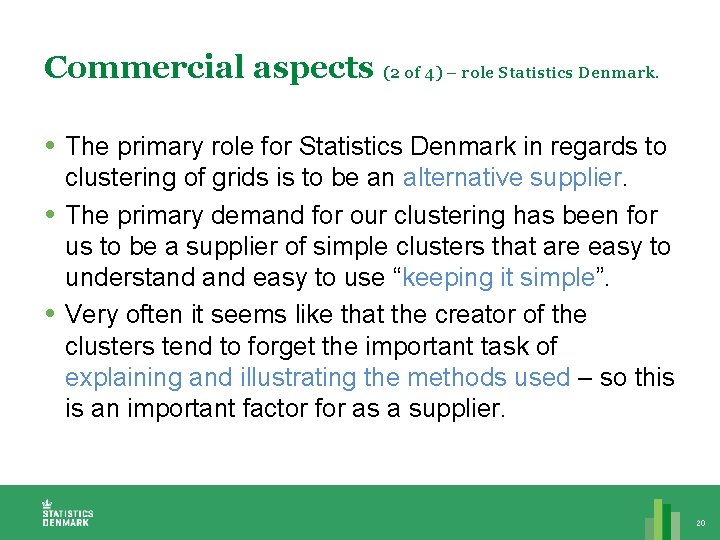
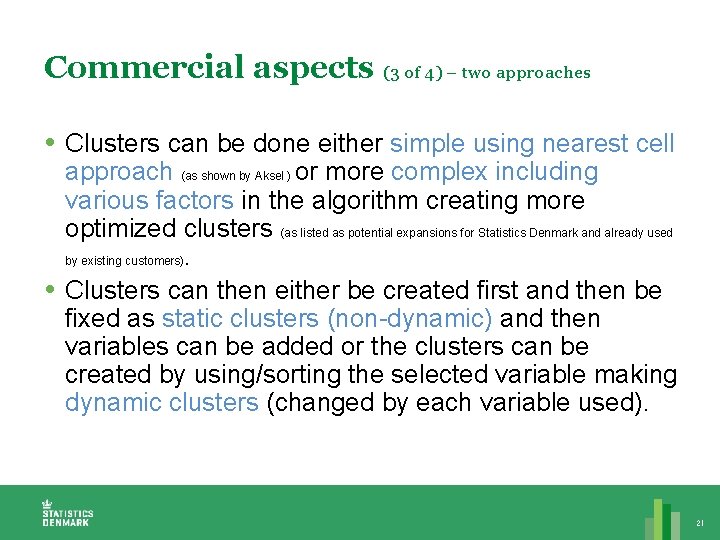
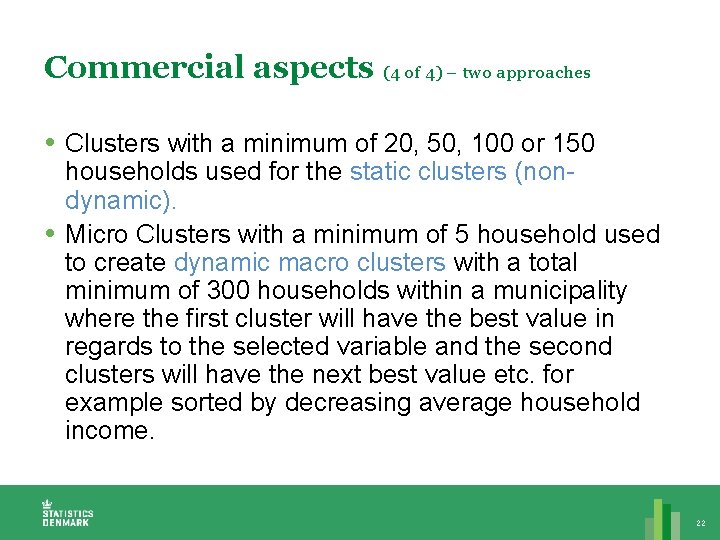
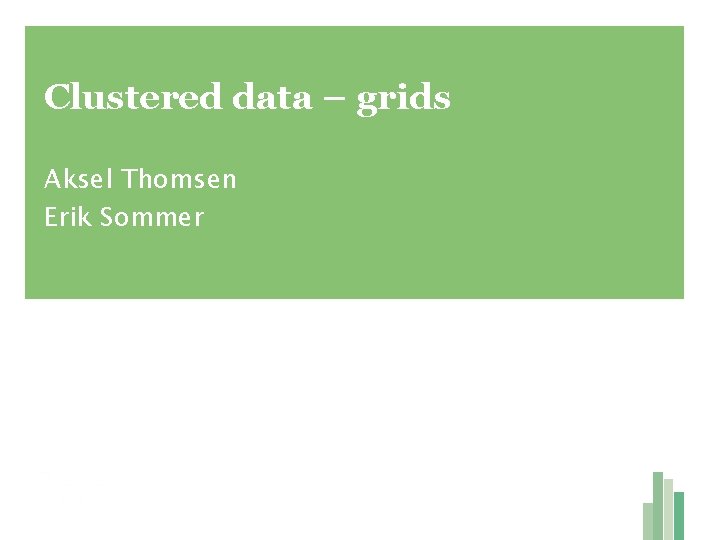
- Slides: 23
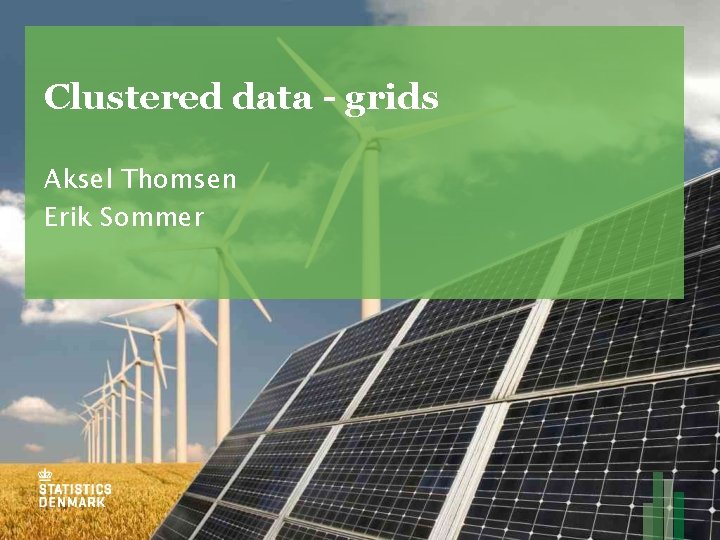
Clustered data - grids Aksel Thomsen Erik Sommer
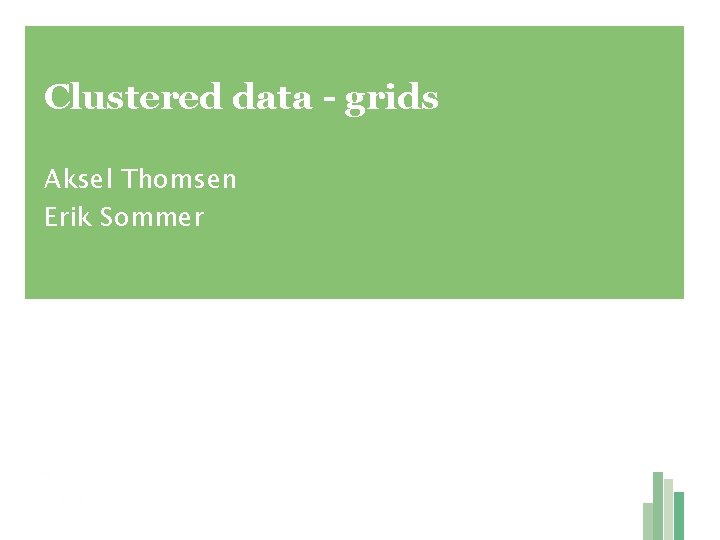
Clustered data - grids Aksel Thomsen Erik Sommer
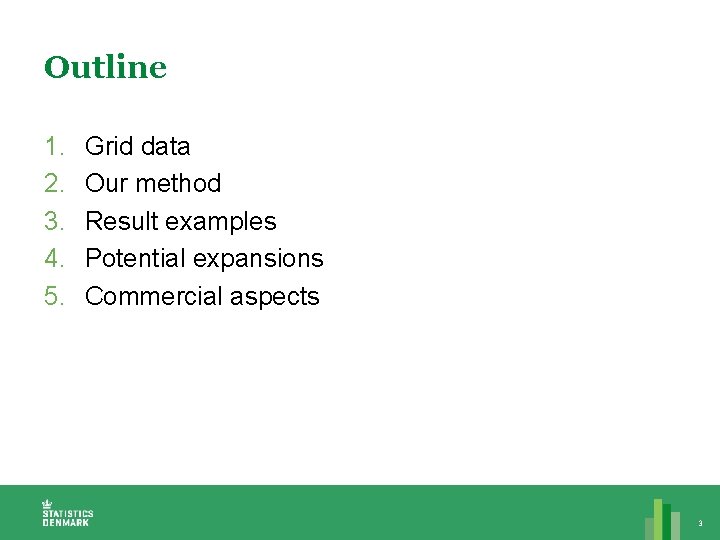
Outline 1. 2. 3. 4. 5. Grid data Our method Result examples Potential expansions Commercial aspects 3
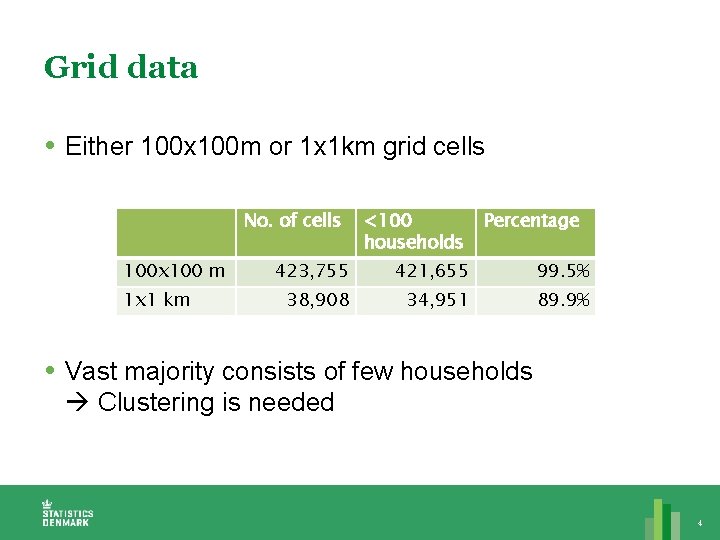
Grid data Either 100 x 100 m or 1 x 1 km grid cells No. of cells 100 x 100 m 1 x 1 km <100 households Percentage 423, 755 421, 655 99. 5% 38, 908 34, 951 89. 9% Vast majority consists of few households Clustering is needed 4
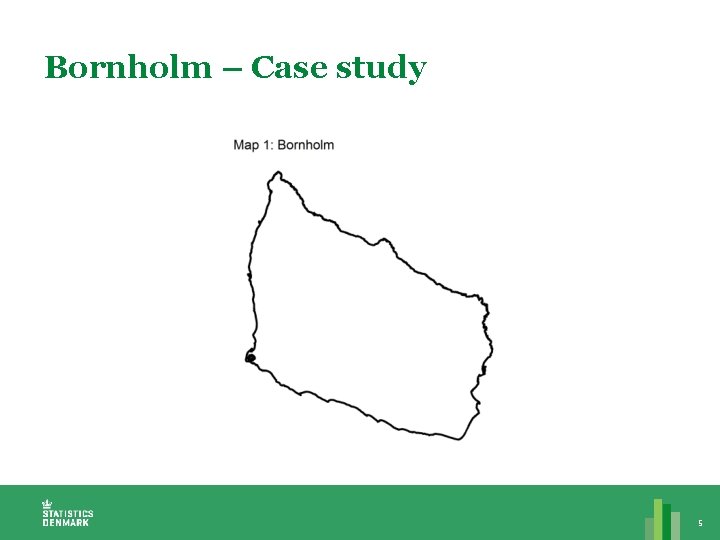
Bornholm – Case study 5
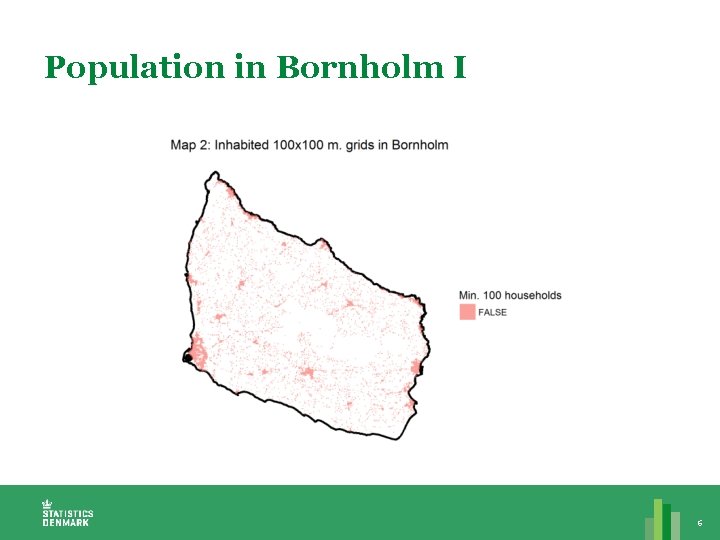
Population in Bornholm I 6
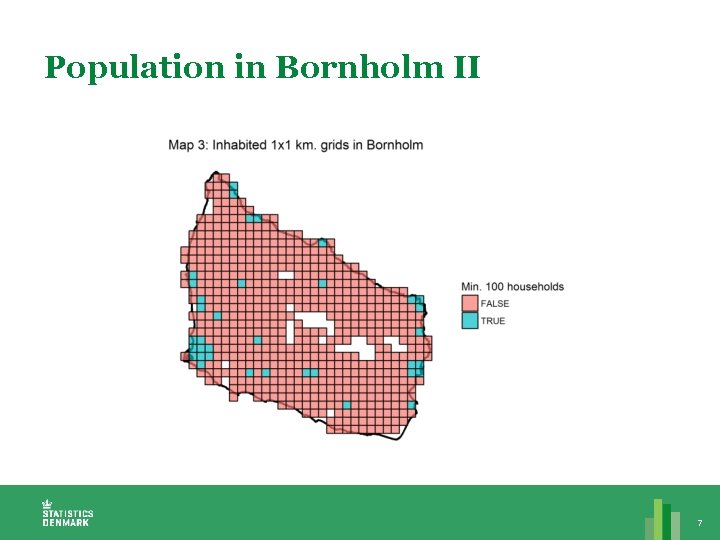
Population in Bornholm II 7
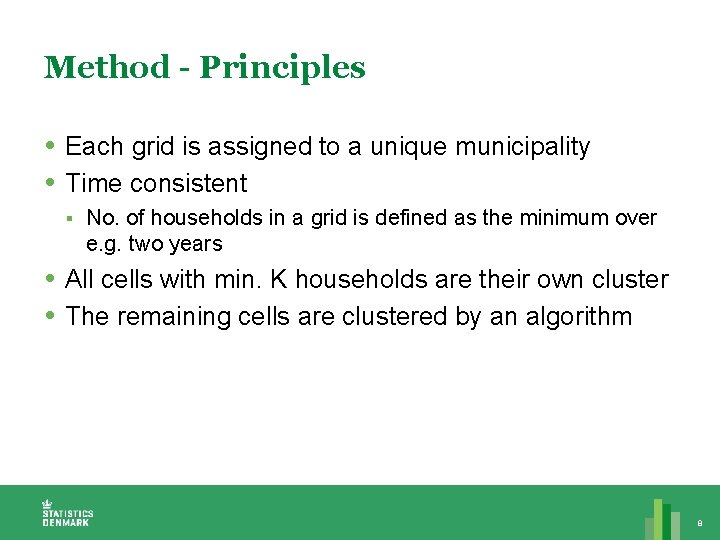
Method - Principles Each grid is assigned to a unique municipality Time consistent § No. of households in a grid is defined as the minimum over e. g. two years All cells with min. K households are their own cluster The remaining cells are clustered by an algorithm 8
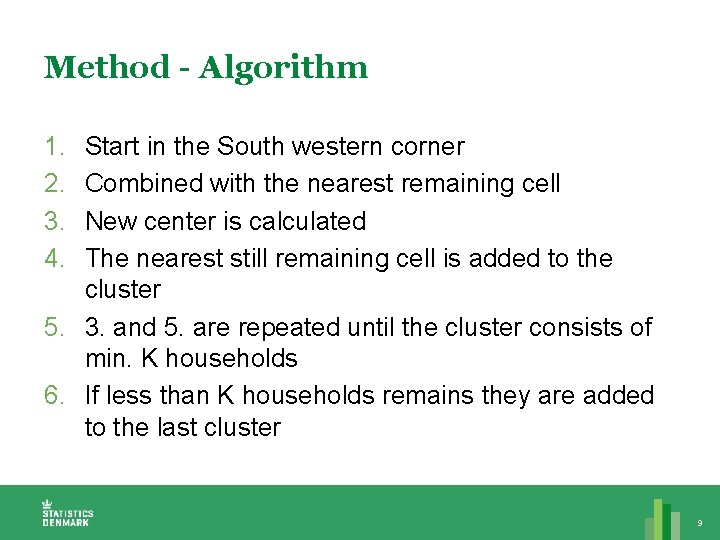
Method - Algorithm 1. 2. 3. 4. Start in the South western corner Combined with the nearest remaining cell New center is calculated The nearest still remaining cell is added to the cluster 5. 3. and 5. are repeated until the cluster consists of min. K households 6. If less than K households remains they are added to the last cluster 9
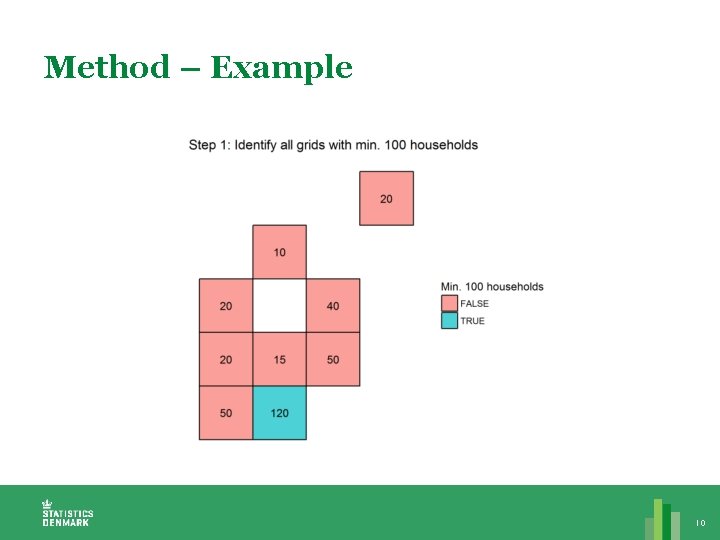
Method – Example 10
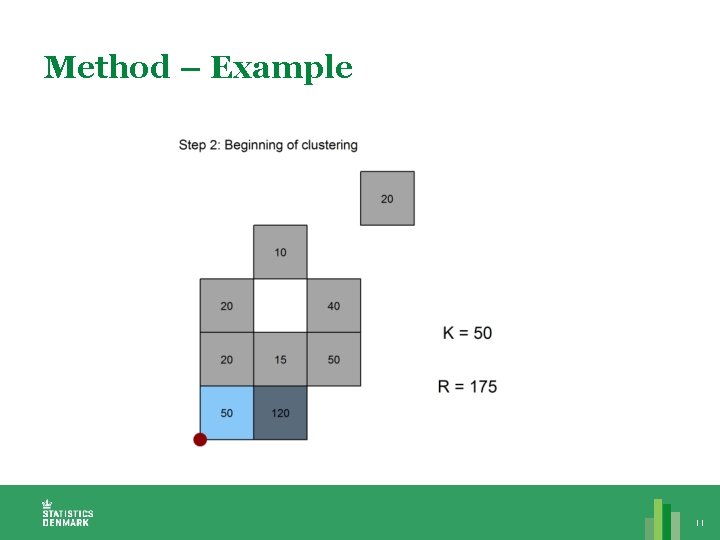
Method – Example 11
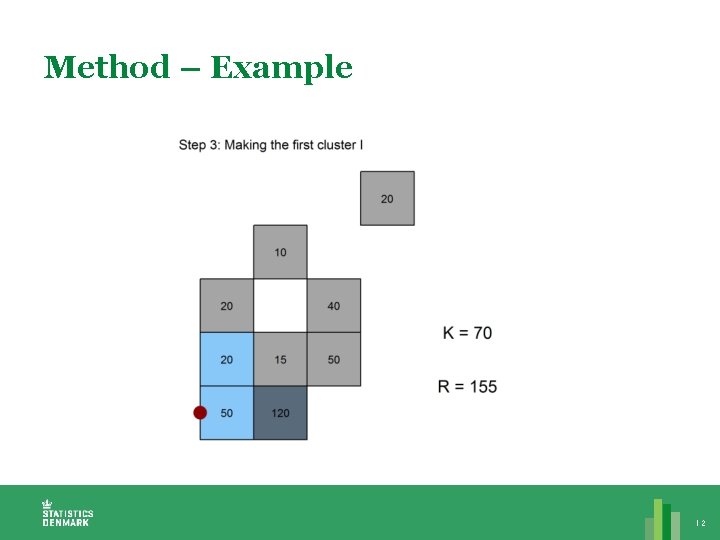
Method – Example 12
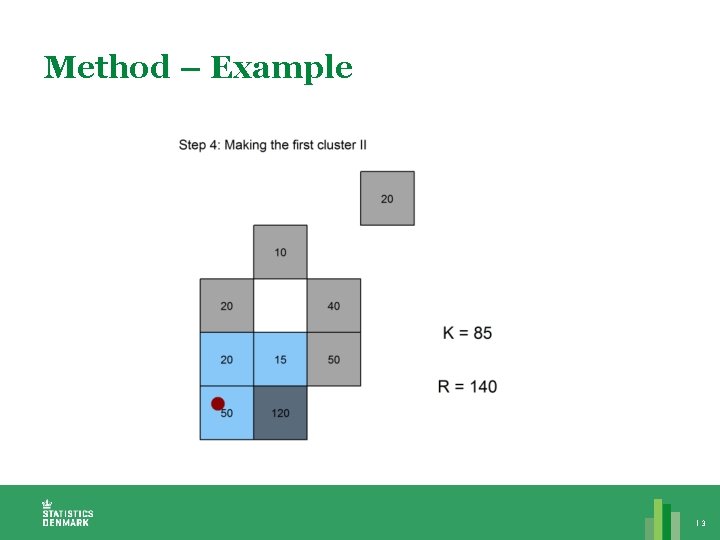
Method – Example 13
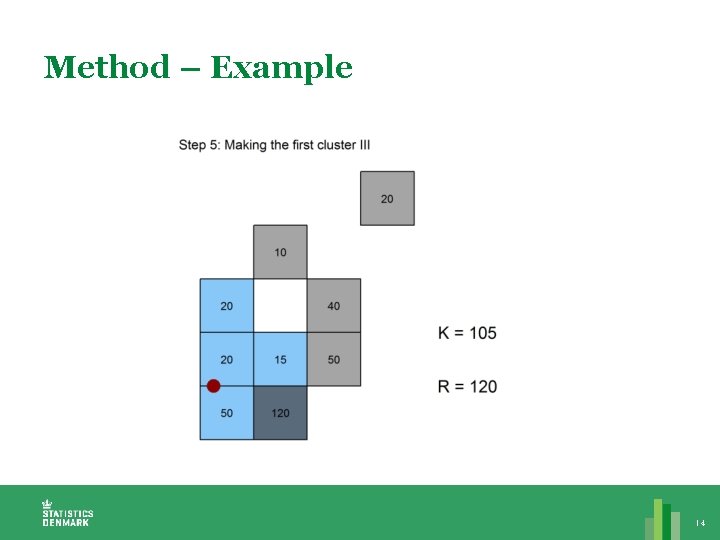
Method – Example 14
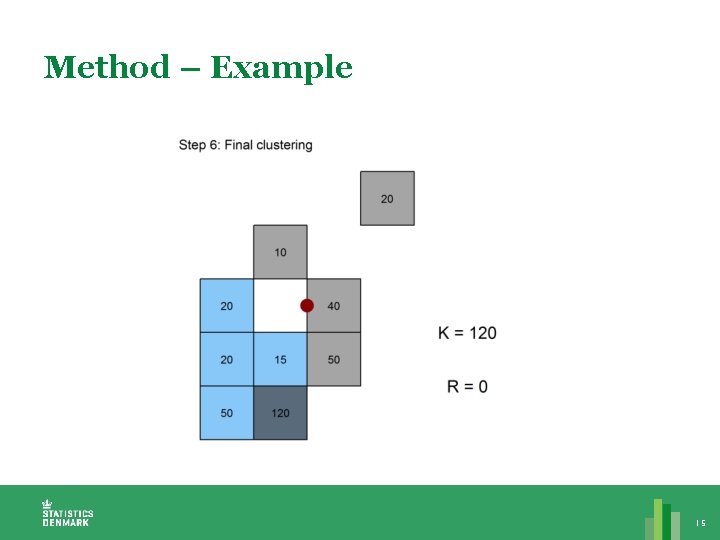
Method – Example 15
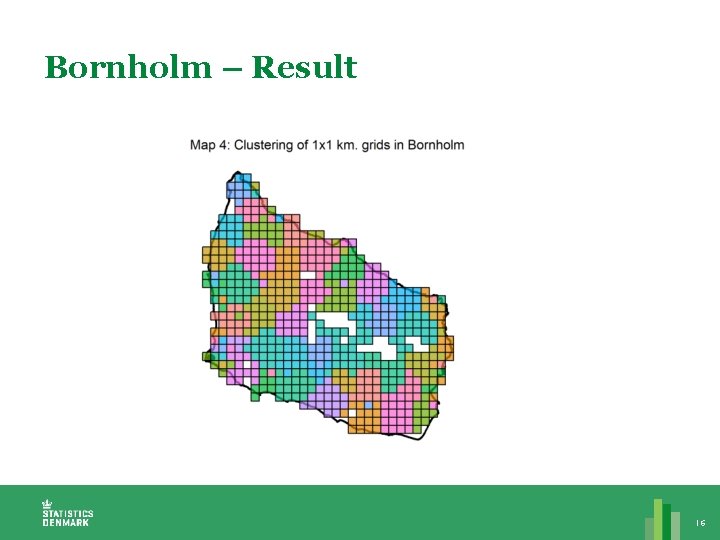
Bornholm – Result 16
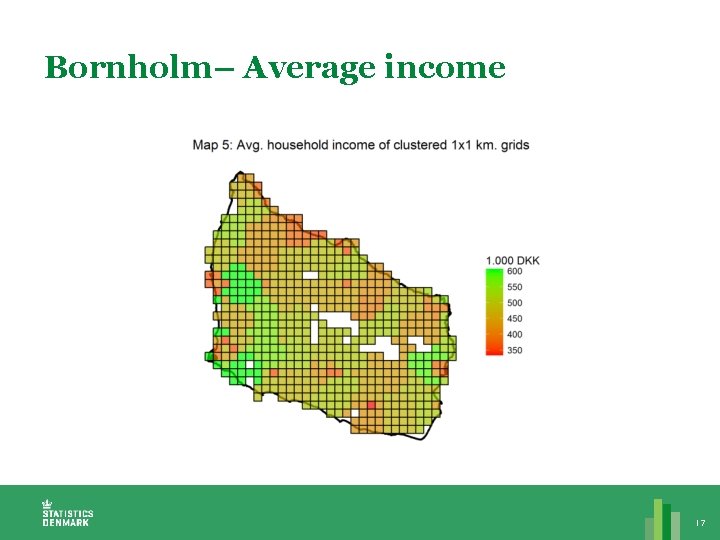
Bornholm– Average income 17
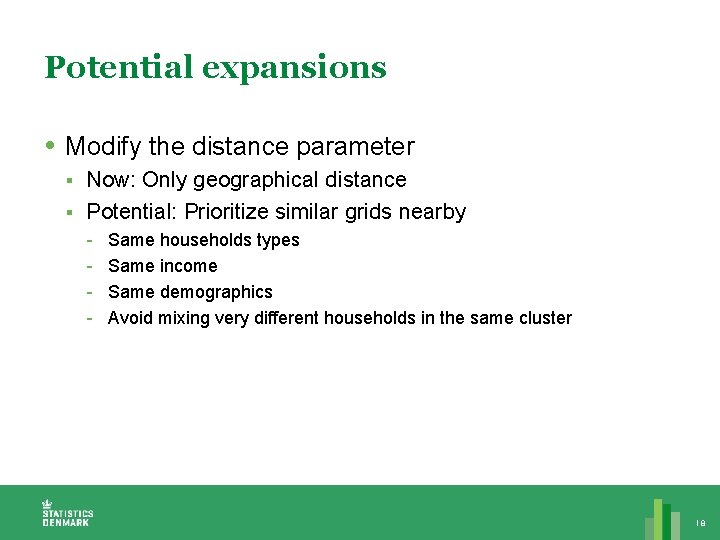
Potential expansions Modify the distance parameter Now: Only geographical distance § Potential: Prioritize similar grids nearby § - Same households types Same income Same demographics Avoid mixing very different households in the same cluster 18
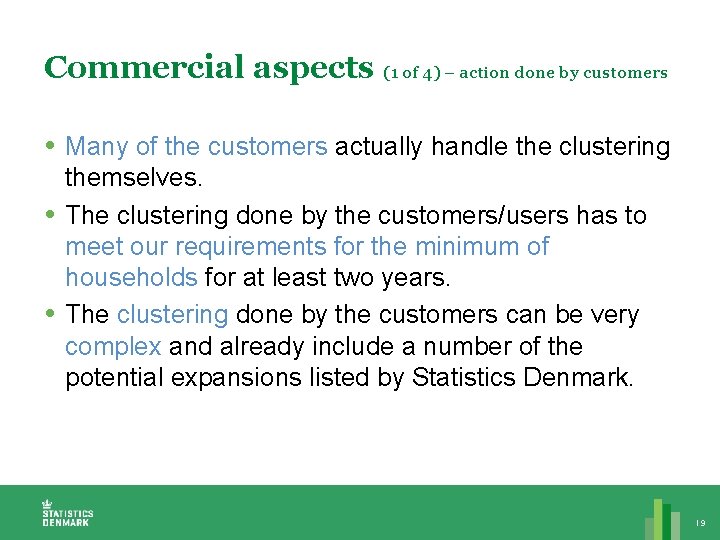
Commercial aspects (1 of 4) – action done by customers Many of the customers actually handle the clustering themselves. The clustering done by the customers/users has to meet our requirements for the minimum of households for at least two years. The clustering done by the customers can be very complex and already include a number of the potential expansions listed by Statistics Denmark. 19
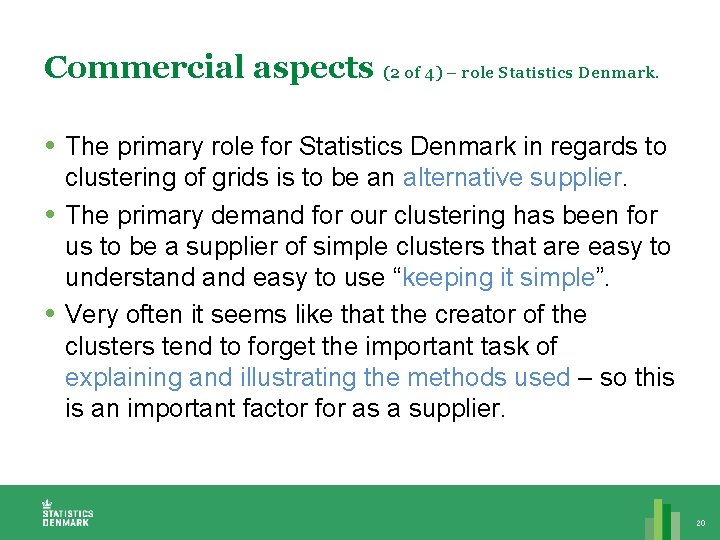
Commercial aspects (2 of 4) – role Statistics Denmark. The primary role for Statistics Denmark in regards to clustering of grids is to be an alternative supplier. The primary demand for our clustering has been for us to be a supplier of simple clusters that are easy to understand easy to use “keeping it simple”. Very often it seems like that the creator of the clusters tend to forget the important task of explaining and illustrating the methods used – so this is an important factor for as a supplier. 20
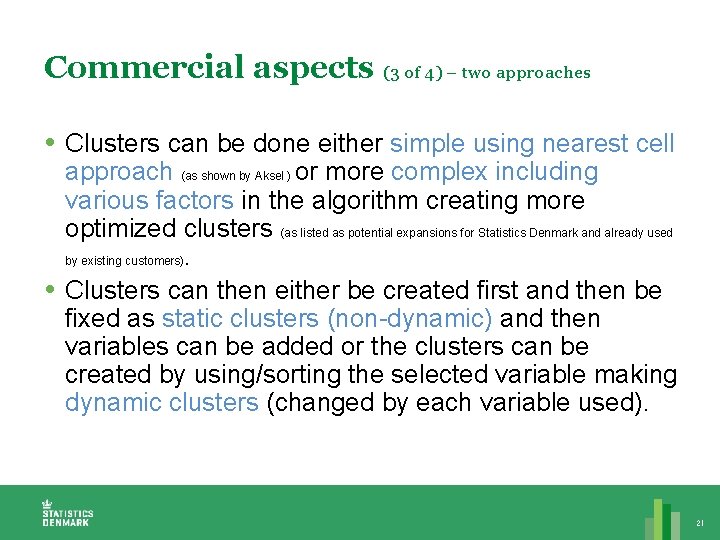
Commercial aspects (3 of 4) – two approaches Clusters can be done either simple using nearest cell approach (as shown by Aksel ) or more complex including various factors in the algorithm creating more optimized clusters (as listed as potential expansions for Statistics Denmark and already used by existing customers). Clusters can then either be created first and then be fixed as static clusters (non-dynamic) and then variables can be added or the clusters can be created by using/sorting the selected variable making dynamic clusters (changed by each variable used). 21
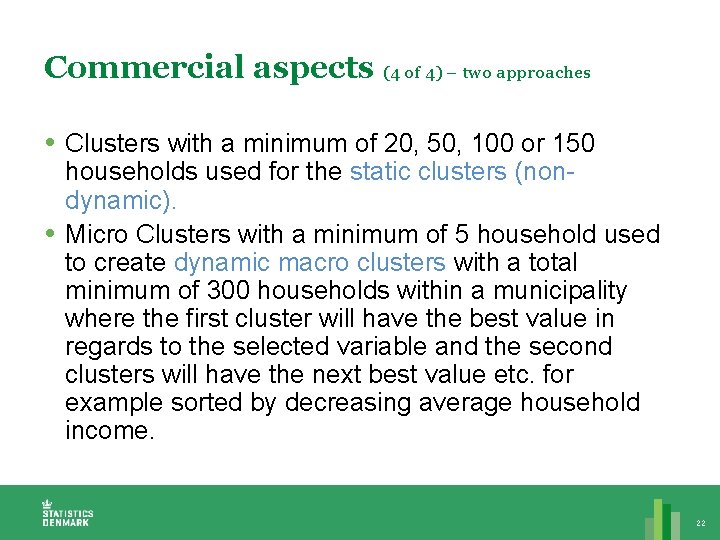
Commercial aspects (4 of 4) – two approaches Clusters with a minimum of 20, 50, 100 or 150 households used for the static clusters (nondynamic). Micro Clusters with a minimum of 5 household used to create dynamic macro clusters with a total minimum of 300 households within a municipality where the first cluster will have the best value in regards to the selected variable and the second clusters will have the next best value etc. for example sorted by decreasing average household income. 22
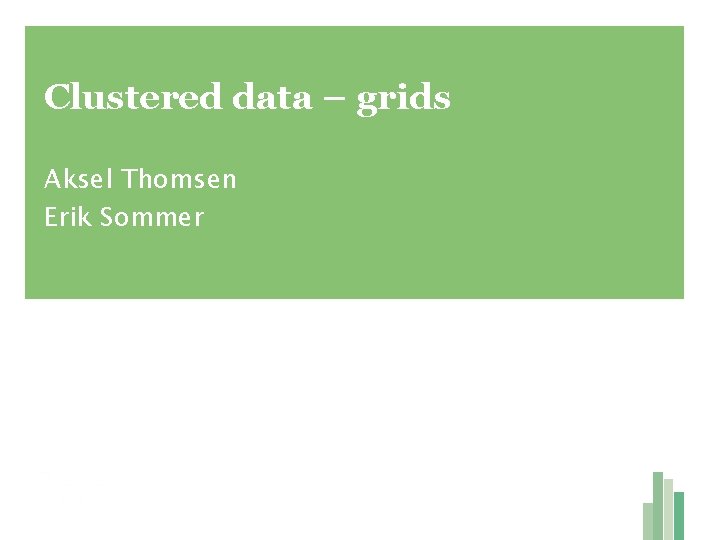
Clustered data – grids Aksel Thomsen Erik Sommer
Clustered index và non clustered index
Aksel hallin
Amcor istanbul
Aksel hallin
Grid conversion factor radiology
Glow discharge tem grids
Layered architecture for web services and grids
Decimal using grids
Parallel research kernels
Demand response in smart grids
Mccc salary grid
Bootstrap 4 osztály
Differentiation grids
Simon nørskov thomsen
Ulla serup thomsen
�rhus karriere
Thomsen diagrams
Flowchart symbols
Astrid bruus thomsen
Lone leth thomsen
H thomsen lohnunternehmen
Wachsgedicht aufbau
Flaggtider nord norge
Sommer stumpenhorst