Challenge and directions for improving GCM simulations of
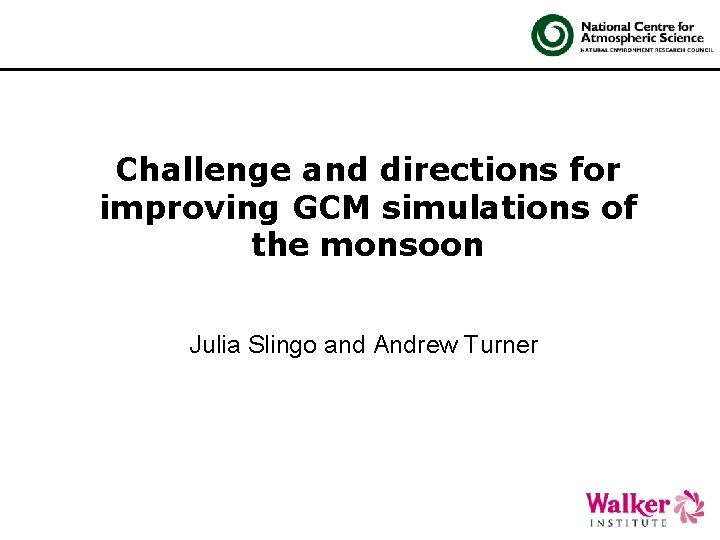
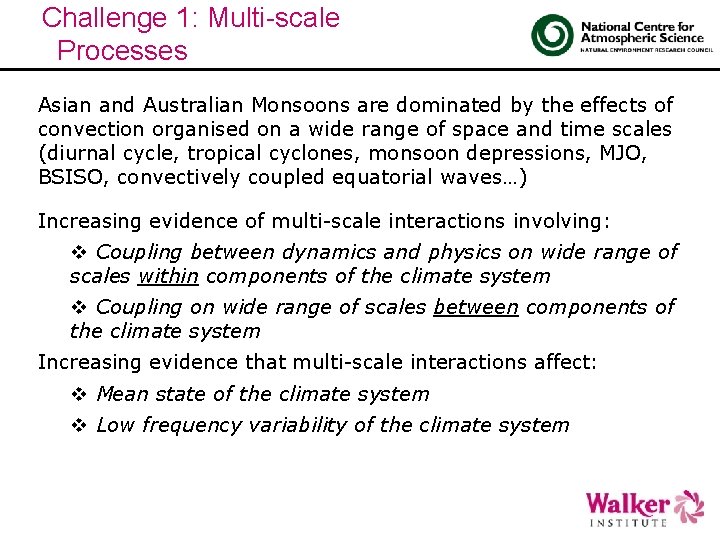
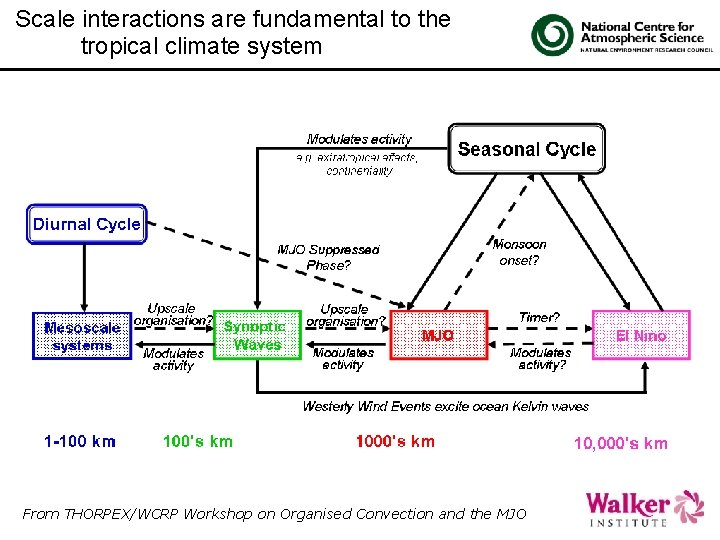
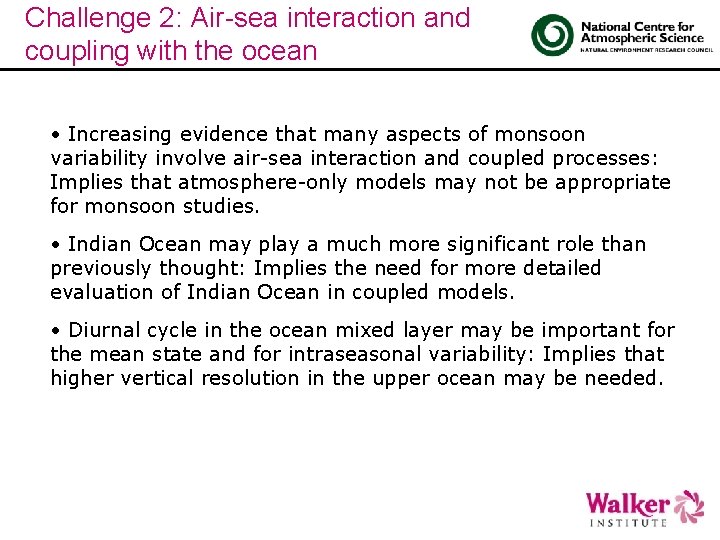
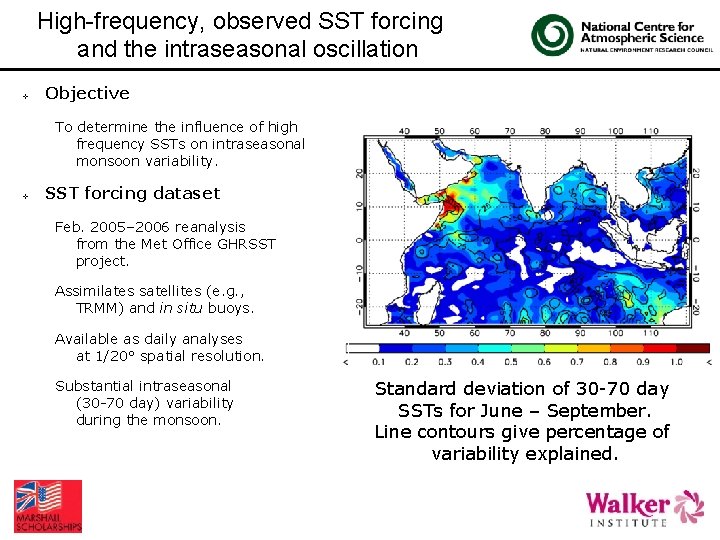
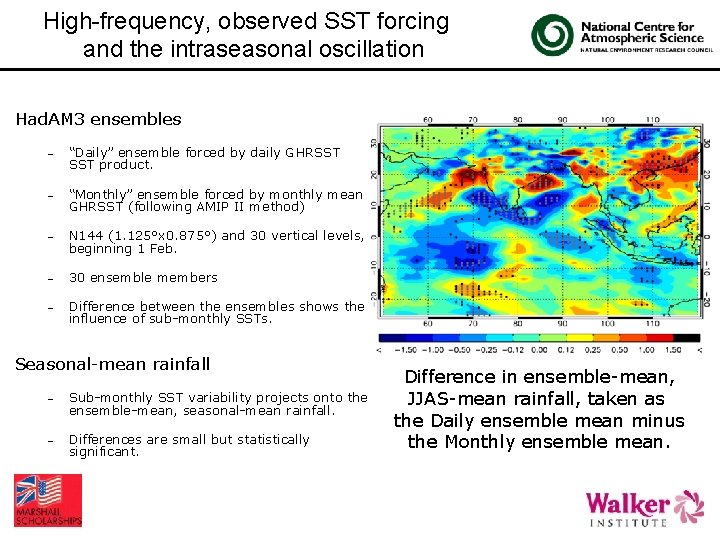
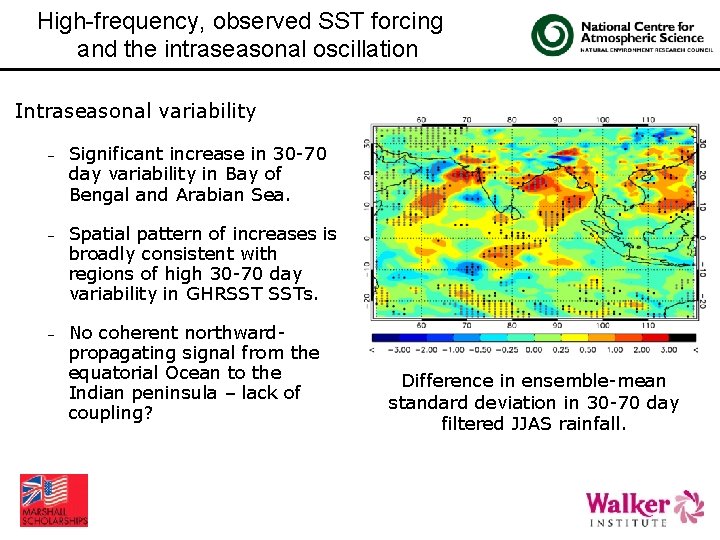
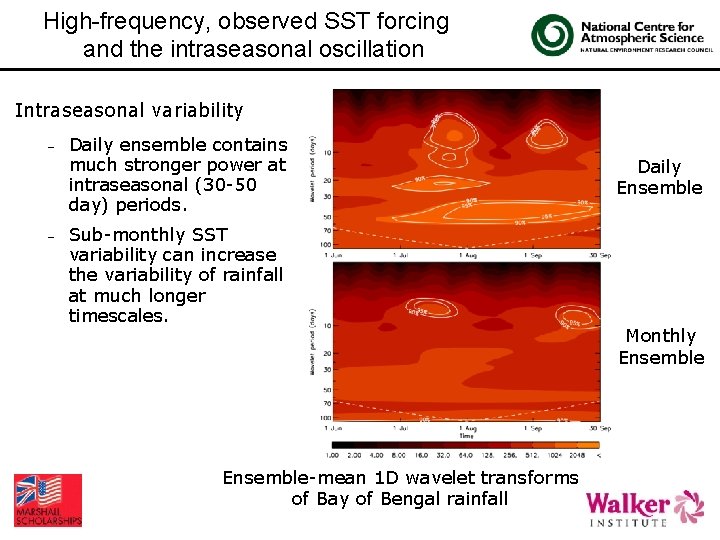
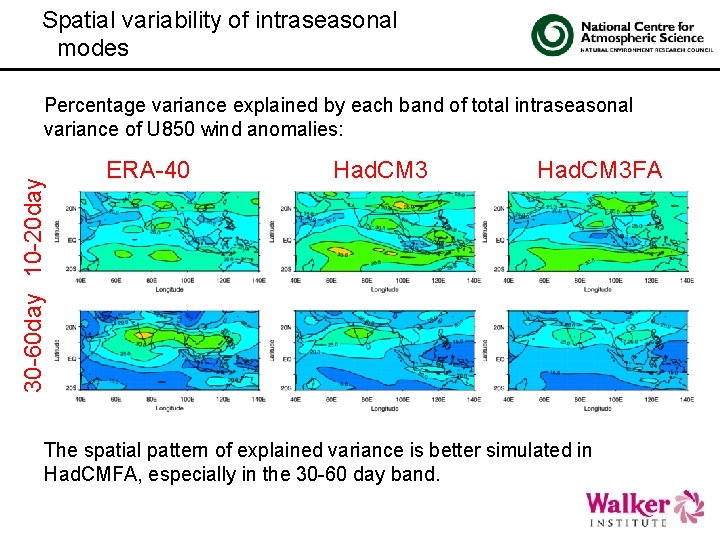
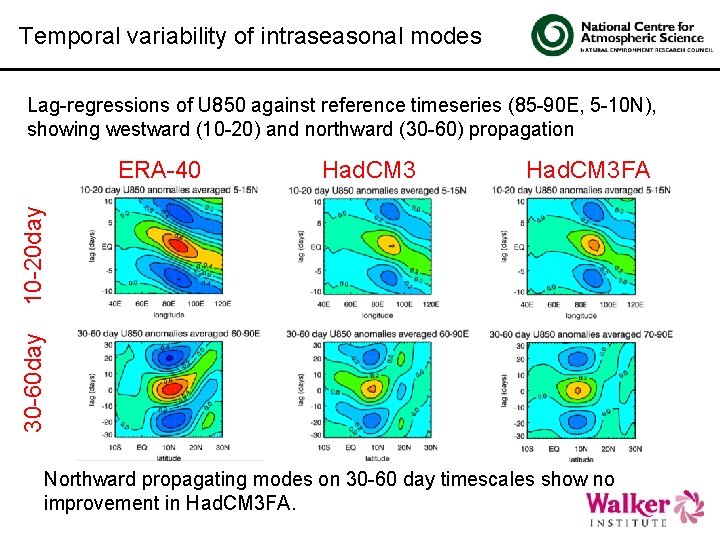
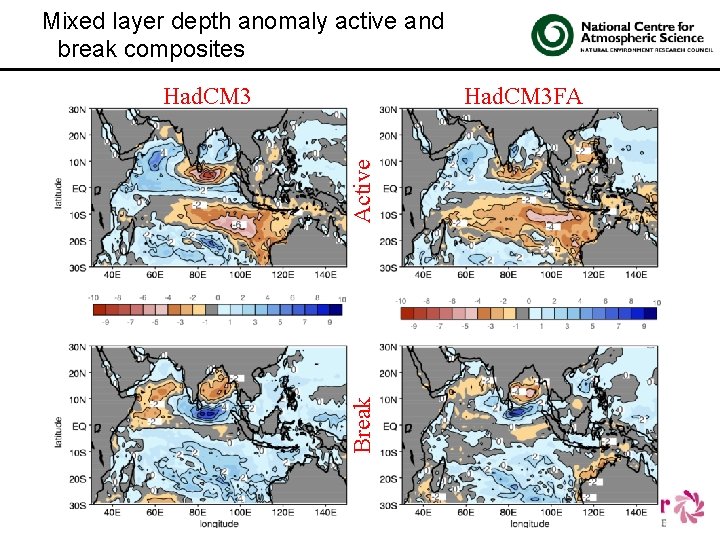
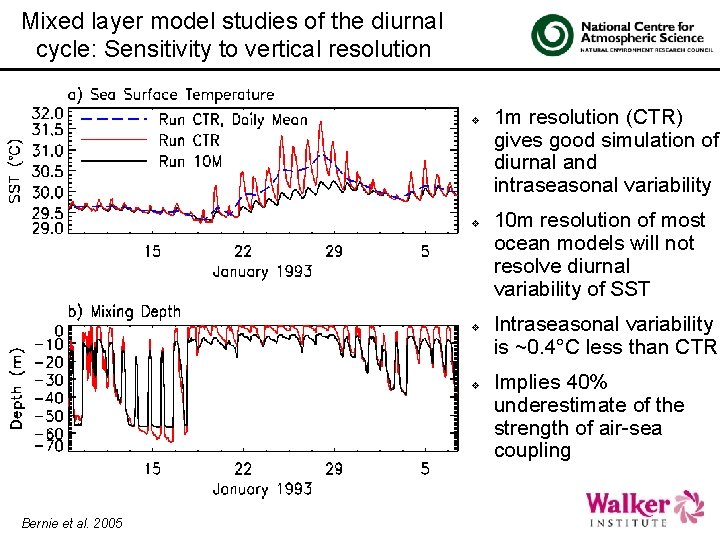
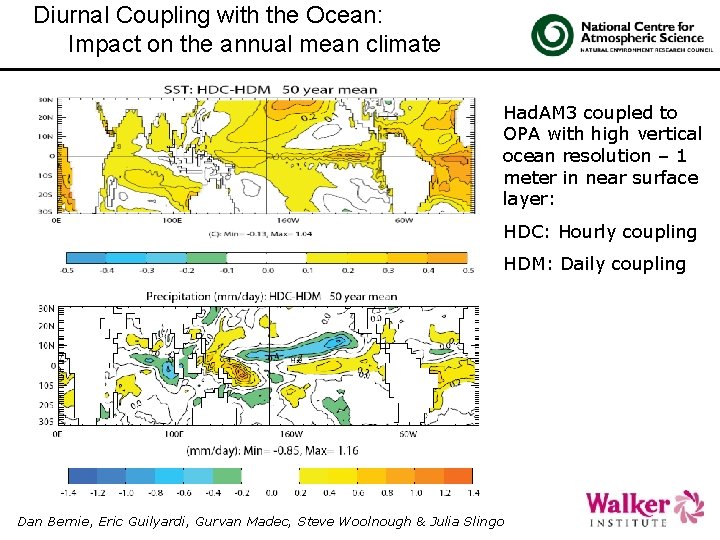
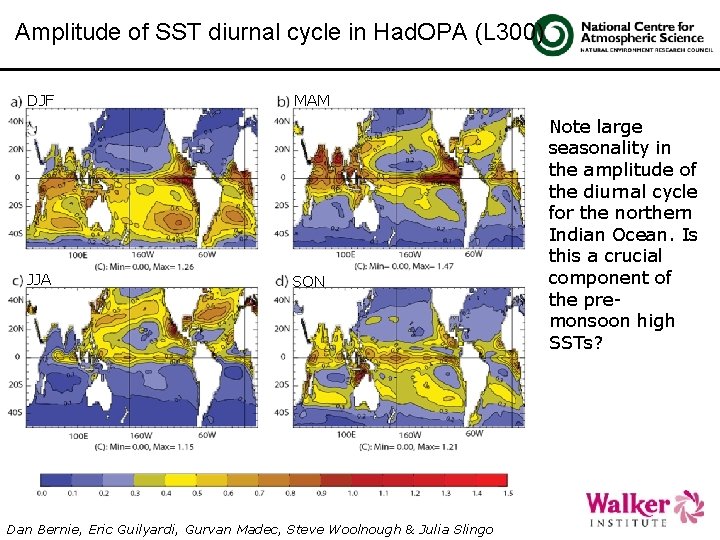
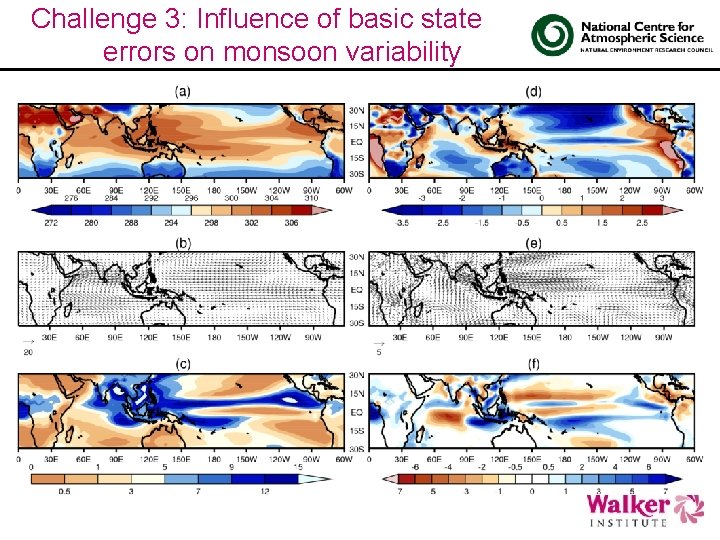
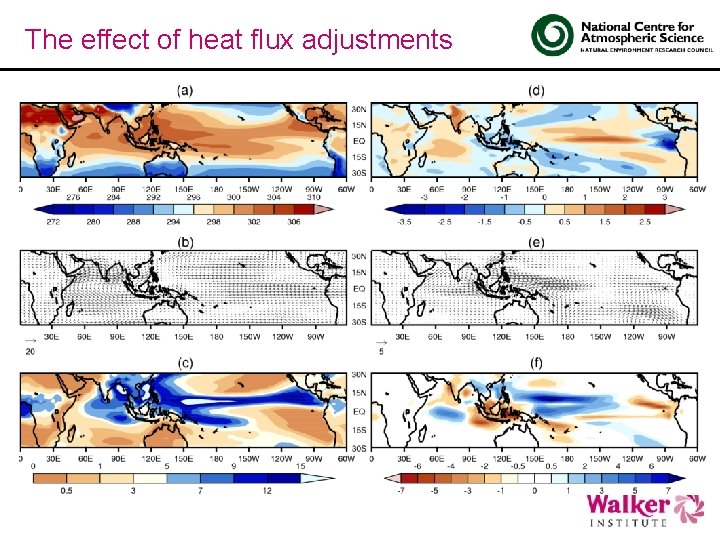
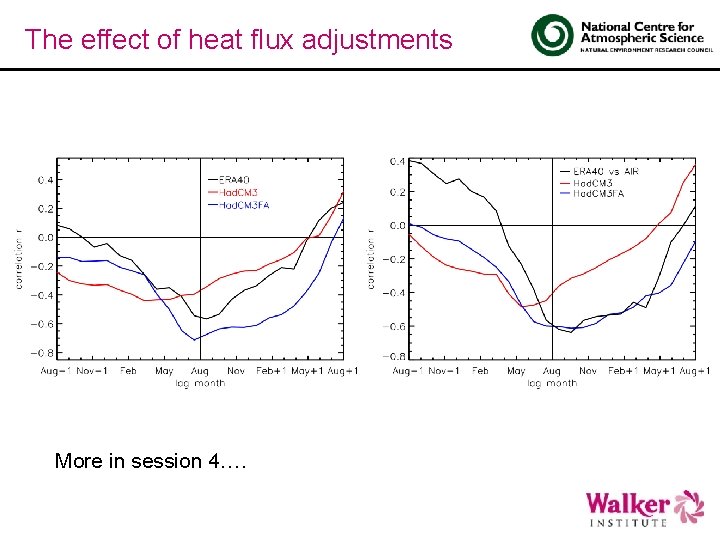
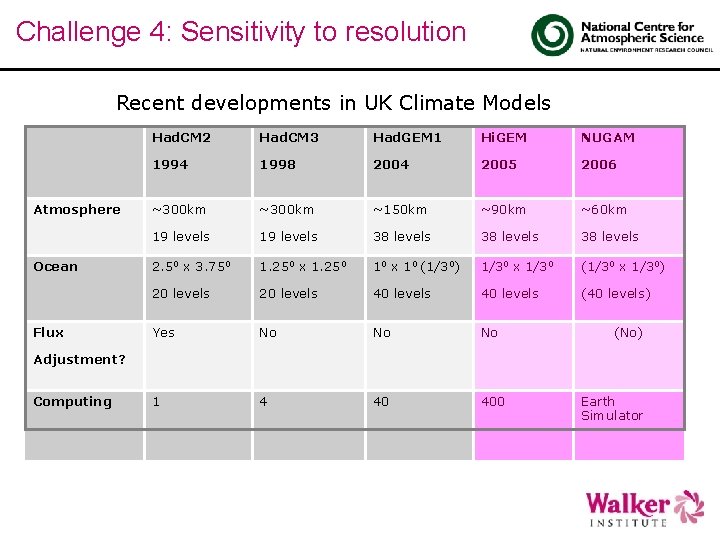
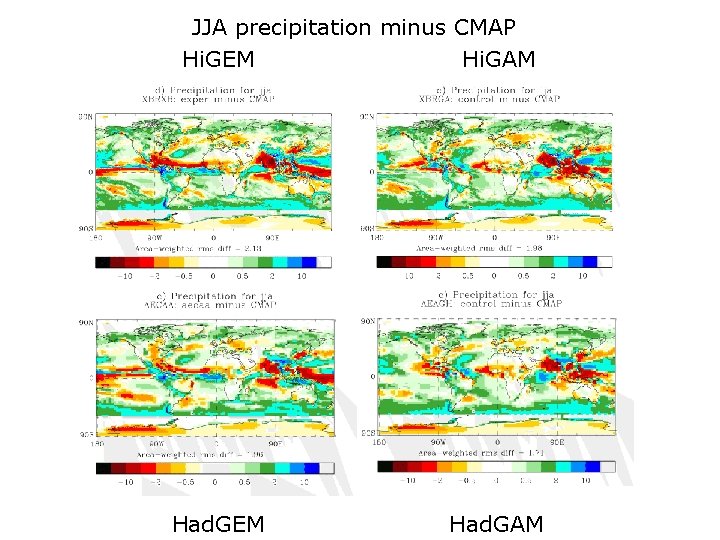
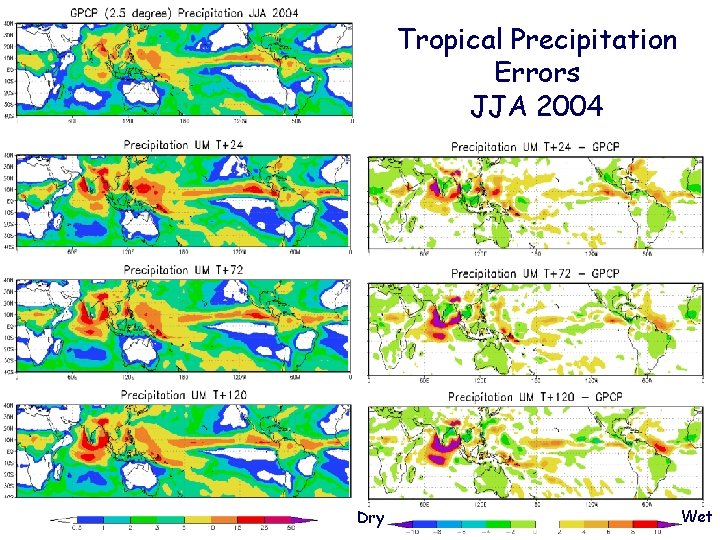
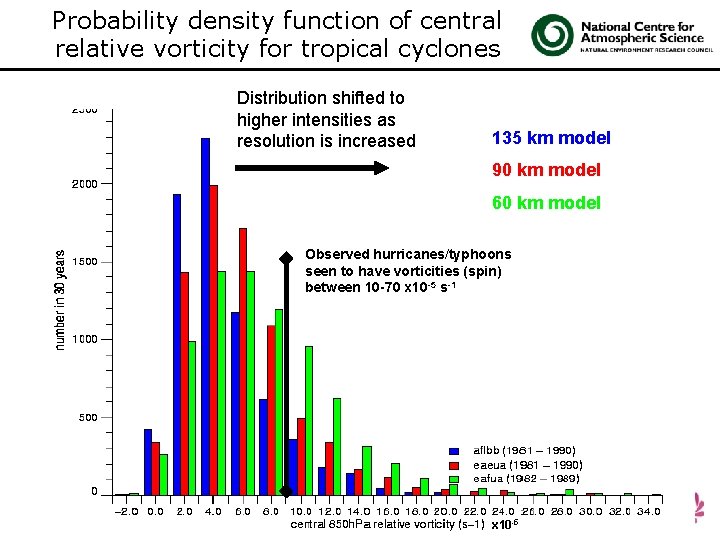
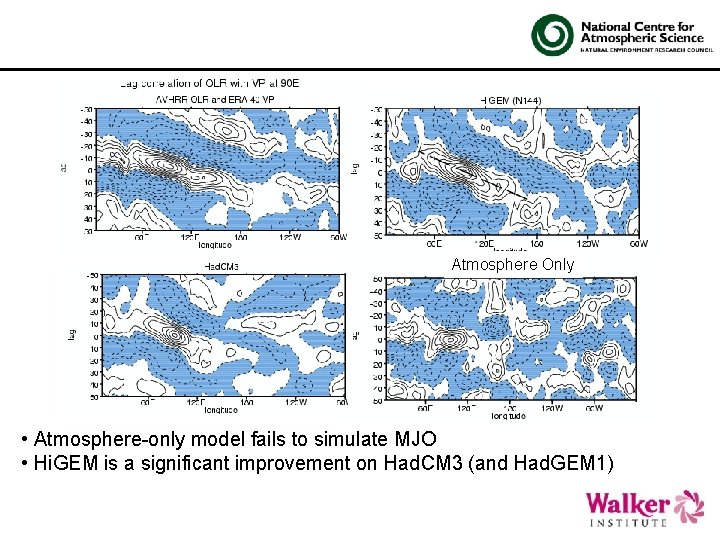
- Slides: 22
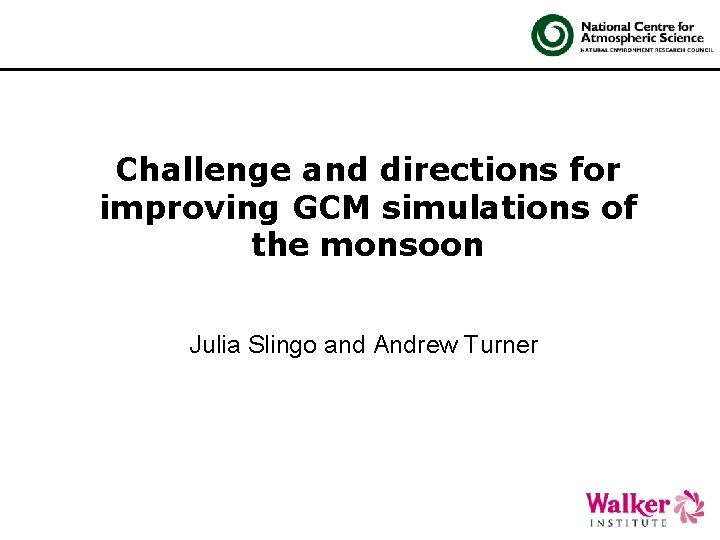
Challenge and directions for improving GCM simulations of the monsoon Julia Slingo and Andrew Turner
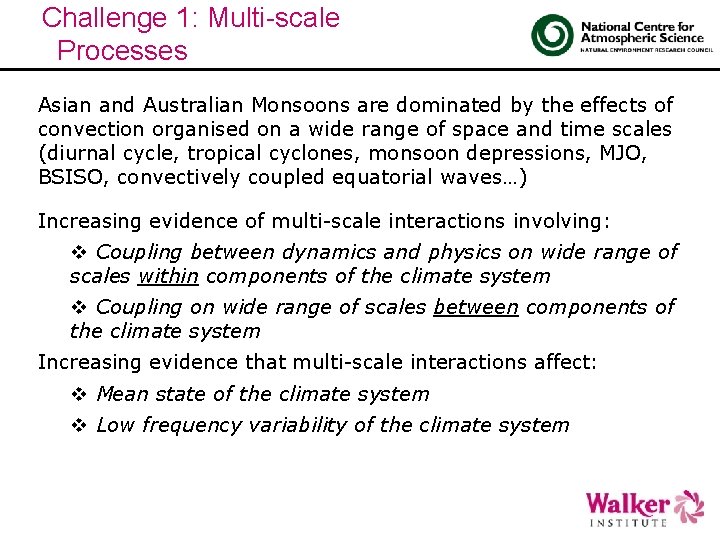
Challenge 1: Multi-scale Processes Asian and Australian Monsoons are dominated by the effects of convection organised on a wide range of space and time scales (diurnal cycle, tropical cyclones, monsoon depressions, MJO, BSISO, convectively coupled equatorial waves…) Increasing evidence of multi-scale interactions involving: v Coupling between dynamics and physics on wide range of scales within components of the climate system v Coupling on wide range of scales between components of the climate system Increasing evidence that multi-scale interactions affect: v Mean state of the climate system v Low frequency variability of the climate system
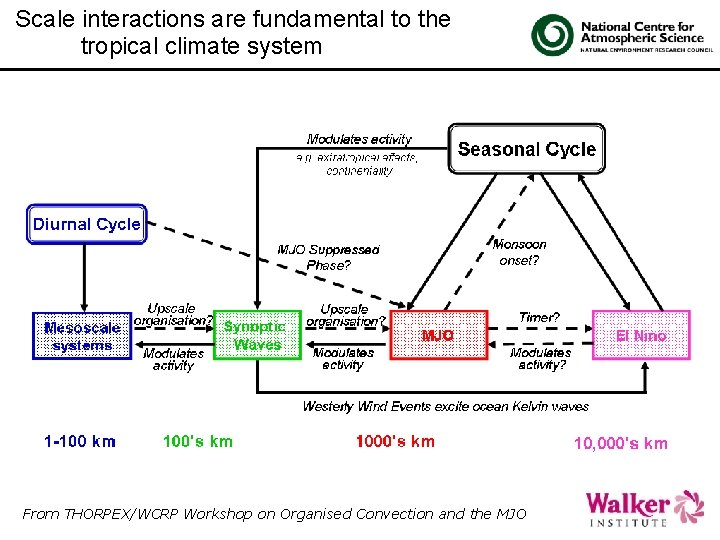
Scale interactions are fundamental to the tropical climate system From THORPEX/WCRP Workshop on Organised Convection and the MJO
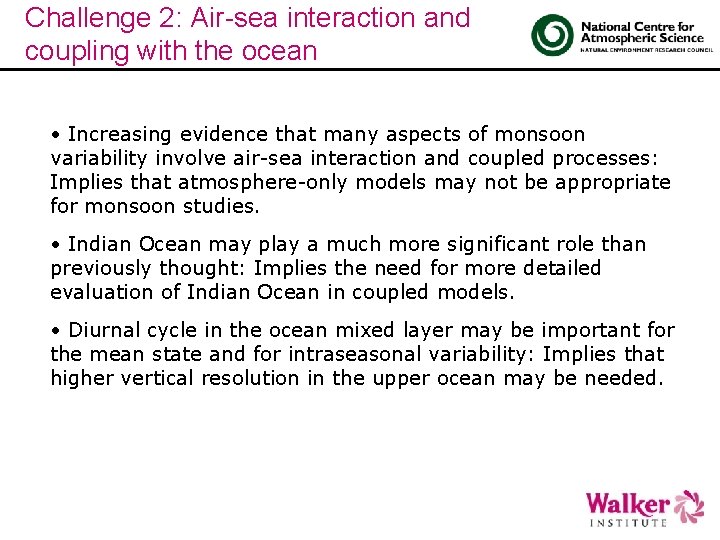
Challenge 2: Air-sea interaction and coupling with the ocean • Increasing evidence that many aspects of monsoon variability involve air-sea interaction and coupled processes: Implies that atmosphere-only models may not be appropriate for monsoon studies. • Indian Ocean may play a much more significant role than previously thought: Implies the need for more detailed evaluation of Indian Ocean in coupled models. • Diurnal cycle in the ocean mixed layer may be important for the mean state and for intraseasonal variability: Implies that higher vertical resolution in the upper ocean may be needed.
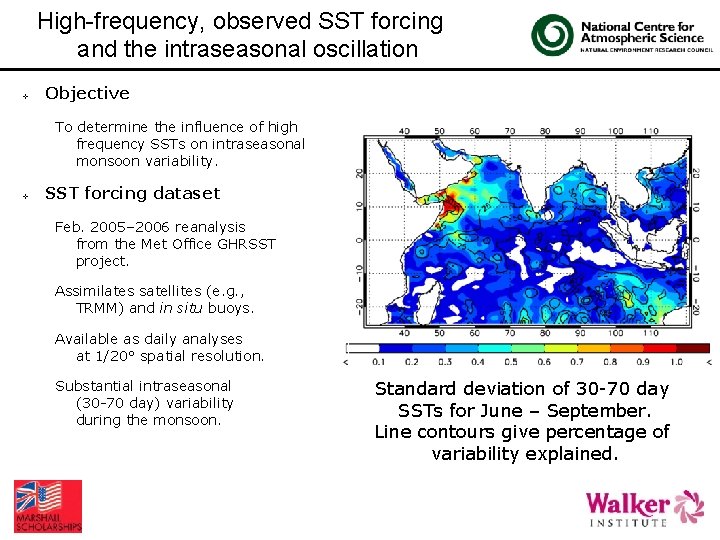
High-frequency, observed SST forcing and the intraseasonal oscillation v Objective To determine the influence of high frequency SSTs on intraseasonal monsoon variability. v SST forcing dataset Feb. 2005– 2006 reanalysis from the Met Office GHRSST project. Assimilates satellites (e. g. , TRMM) and in situ buoys. Available as daily analyses at 1/20° spatial resolution. Substantial intraseasonal (30 -70 day) variability during the monsoon. Standard deviation of 30 -70 day SSTs for June – September. Line contours give percentage of variability explained.
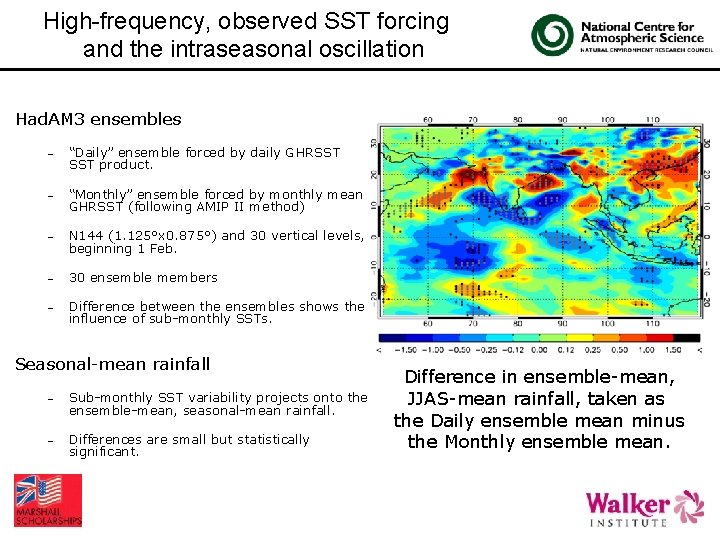
High-frequency, observed SST forcing and the intraseasonal oscillation Had. AM 3 ensembles “Daily” ensemble forced by daily GHRSST product. “Monthly” ensemble forced by monthly mean GHRSST (following AMIP II method) N 144 (1. 125°x 0. 875°) and 30 vertical levels, beginning 1 Feb. 30 ensemble members Difference between the ensembles shows the influence of sub-monthly SSTs. Seasonal-mean rainfall Sub-monthly SST variability projects onto the ensemble-mean, seasonal-mean rainfall. Differences are small but statistically significant. Difference in ensemble-mean, JJAS-mean rainfall, taken as the Daily ensemble mean minus the Monthly ensemble mean.
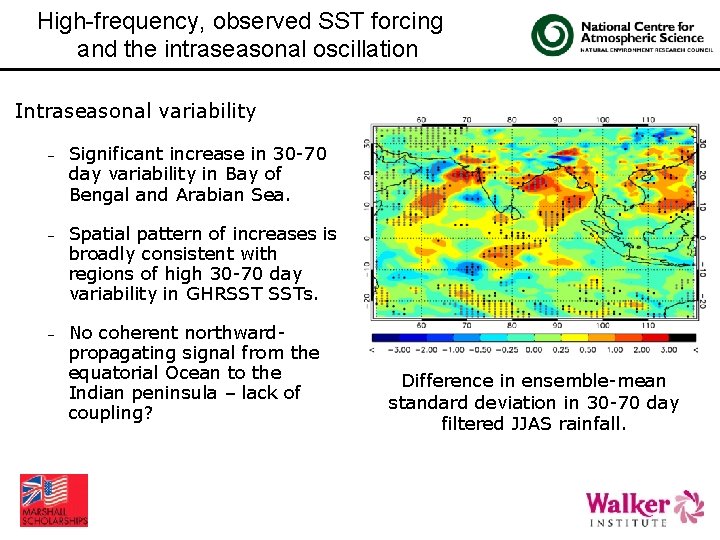
High-frequency, observed SST forcing and the intraseasonal oscillation Intraseasonal variability Significant increase in 30 -70 day variability in Bay of Bengal and Arabian Sea. Spatial pattern of increases is broadly consistent with regions of high 30 -70 day variability in GHRSST SSTs. No coherent northwardpropagating signal from the equatorial Ocean to the Indian peninsula – lack of coupling? Difference in ensemble-mean standard deviation in 30 -70 day filtered JJAS rainfall.
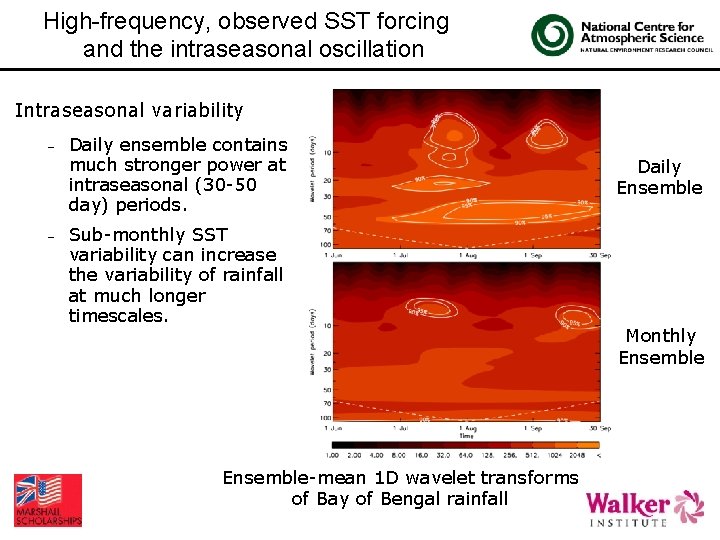
High-frequency, observed SST forcing and the intraseasonal oscillation Intraseasonal variability Daily ensemble contains much stronger power at intraseasonal (30 -50 day) periods. Sub-monthly SST variability can increase the variability of rainfall at much longer timescales. Ensemble-mean 1 D wavelet transforms of Bay of Bengal rainfall Daily Ensemble Monthly Ensemble
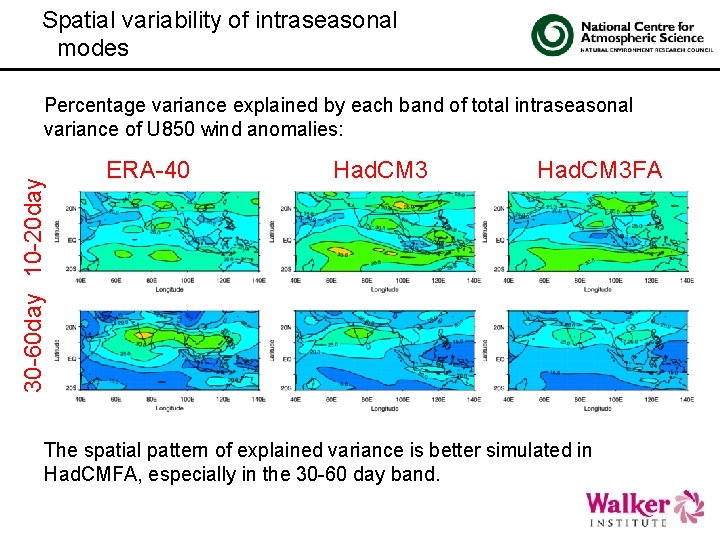
Spatial variability of intraseasonal modes 30 -60 day 10 -20 day Percentage variance explained by each band of total intraseasonal variance of U 850 wind anomalies: ERA-40 Had. CM 3 FA The spatial pattern of explained variance is better simulated in Had. CMFA, especially in the 30 -60 day band.
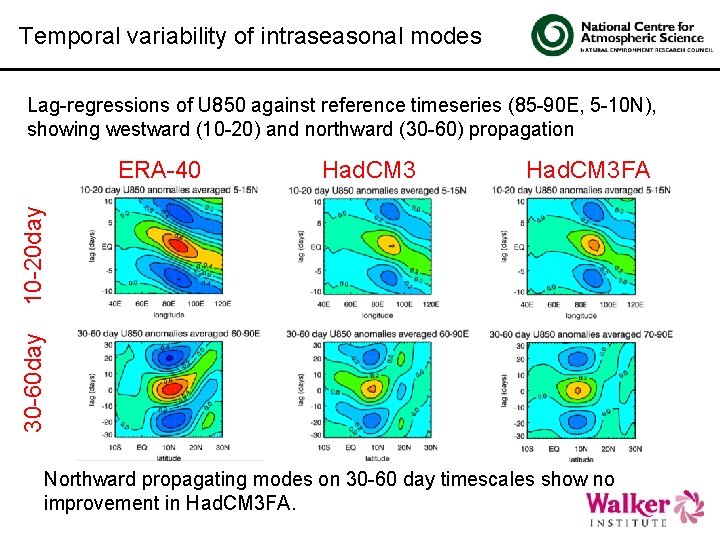
Temporal variability of intraseasonal modes Lag-regressions of U 850 against reference timeseries (85 -90 E, 5 -10 N), showing westward (10 -20) and northward (30 -60) propagation Had. CM 3 FA 30 -60 day 10 -20 day ERA-40 Northward propagating modes on 30 -60 day timescales show no improvement in Had. CM 3 FA.
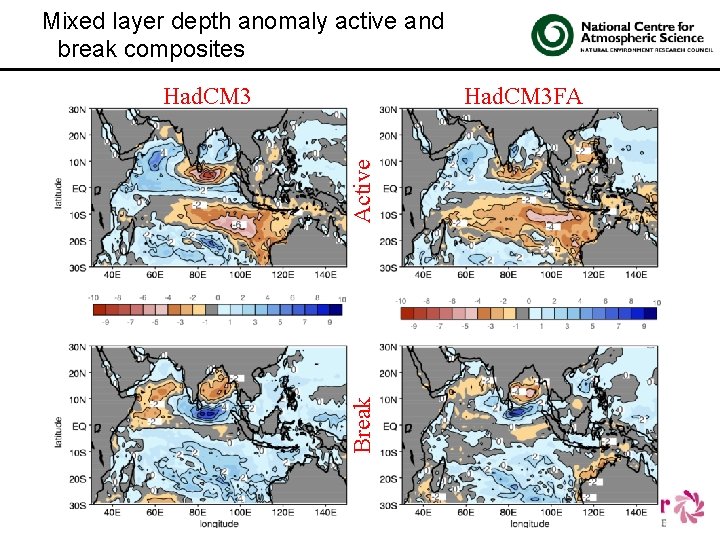
Mixed layer depth anomaly active and break composites Had. CM 3 FA Break Active Had. CM 3
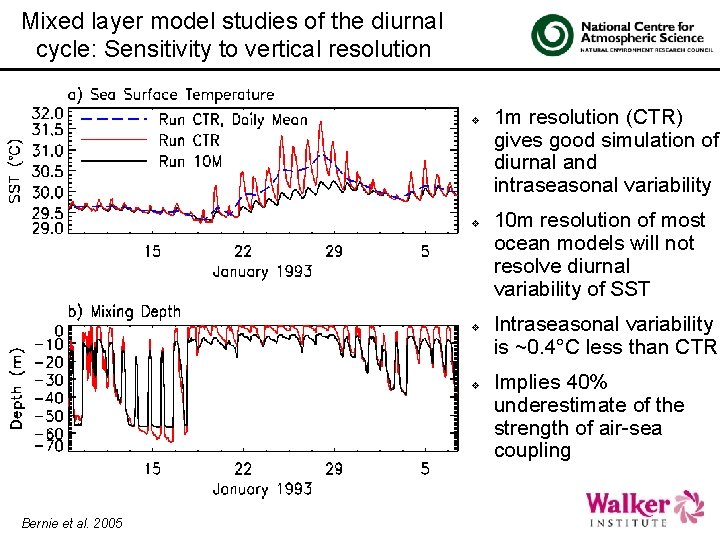
Mixed layer model studies of the diurnal cycle: Sensitivity to vertical resolution v v Bernie et al. 2005 1 m resolution (CTR) gives good simulation of diurnal and intraseasonal variability 10 m resolution of most ocean models will not resolve diurnal variability of SST Intraseasonal variability is ~0. 4°C less than CTR Implies 40% underestimate of the strength of air-sea coupling
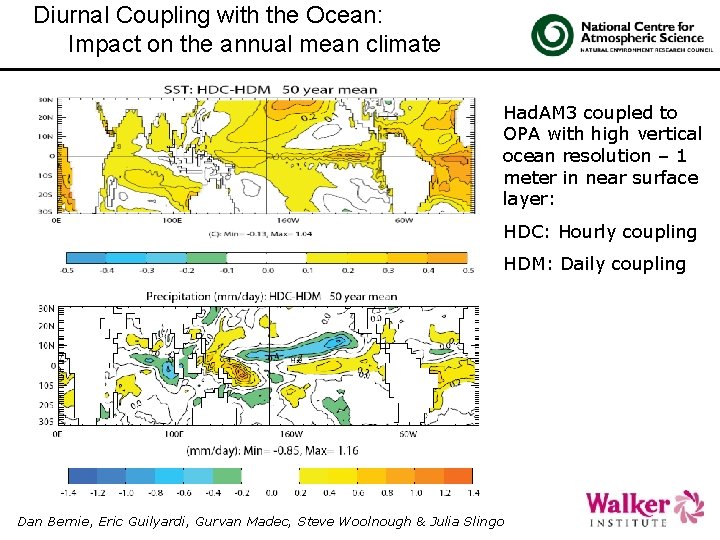
Diurnal Coupling with the Ocean: Impact on the annual mean climate Had. AM 3 coupled to OPA with high vertical ocean resolution – 1 meter in near surface layer: HDC: Hourly coupling HDM: Daily coupling Dan Bernie, Eric Guilyardi, Gurvan Madec, Steve Woolnough & Julia Slingo
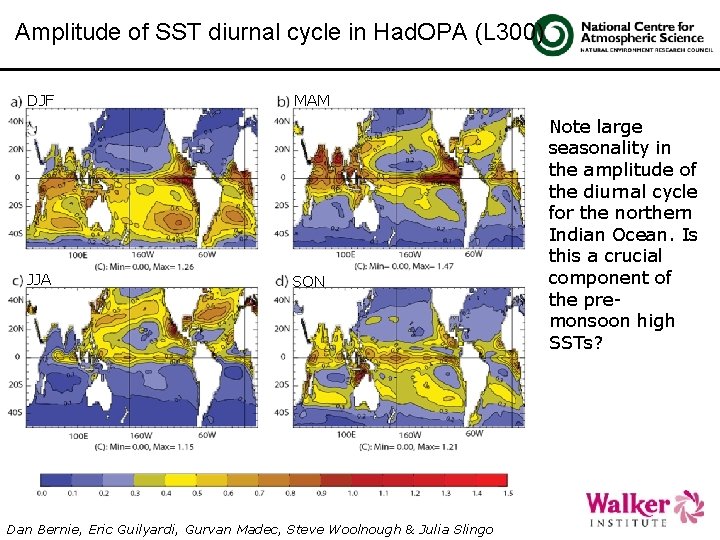
Amplitude of SST diurnal cycle in Had. OPA (L 300) DJF JJA MAM SON Dan Bernie, Eric Guilyardi, Gurvan Madec, Steve Woolnough & Julia Slingo Note large seasonality in the amplitude of the diurnal cycle for the northern Indian Ocean. Is this a crucial component of the premonsoon high SSTs?
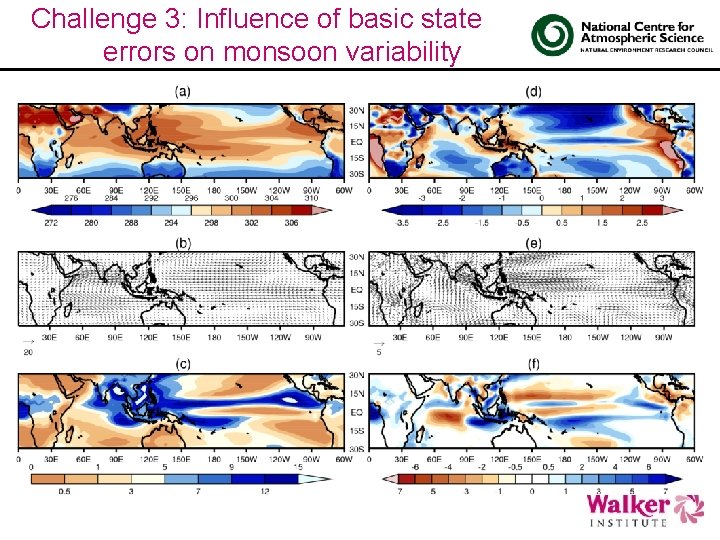
Challenge 3: Influence of basic state errors on monsoon variability A very interesting talk
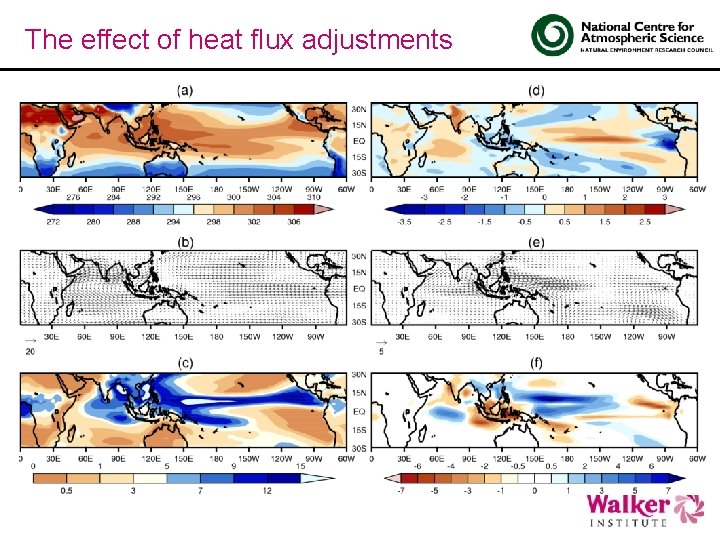
The effect of heat flux adjustments
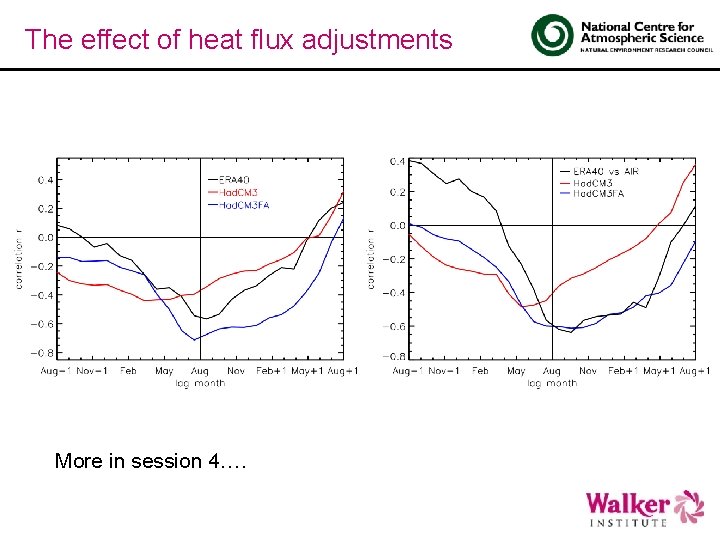
The effect of heat flux adjustments More in session 4….
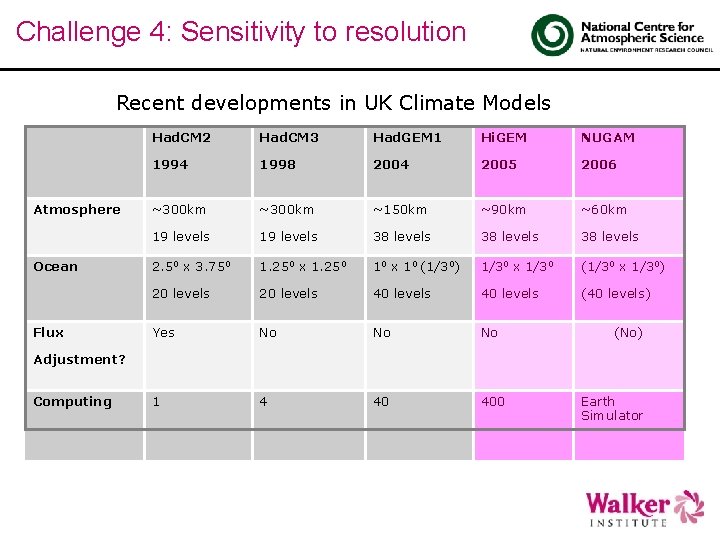
Challenge 4: Sensitivity to resolution Recent developments in UK Climate Models Atmosphere Ocean Flux Had. CM 2 Had. CM 3 Had. GEM 1 Hi. GEM NUGAM 1994 1998 2004 2005 2006 ~300 km ~150 km ~90 km ~60 km 19 levels 38 levels 2. 50 x 3. 750 1. 250 x 1. 250 10 x 10 (1/30) 1/30 x 1/30 (1/30 x 1/30) 20 levels 40 levels (40 levels) Yes No No No 1 4 40 400 (No) Adjustment? Computing Earth Simulator
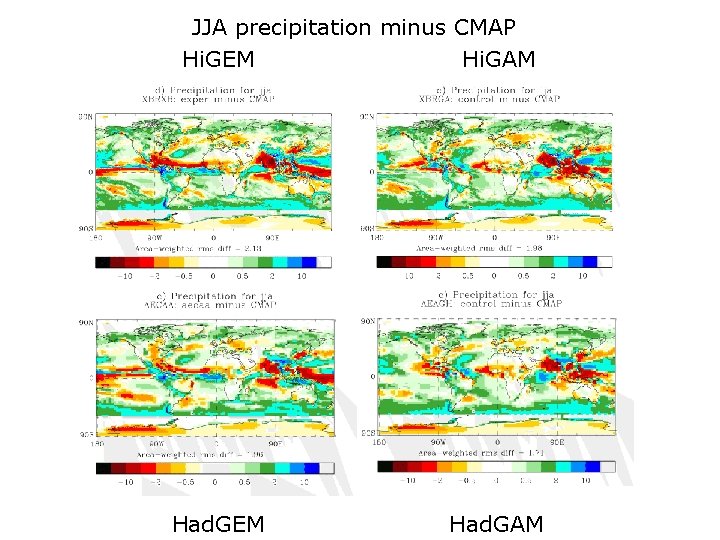
JJA precipitation minus CMAP Hi. GEM Hi. GAM Had. GEM Had. GAM
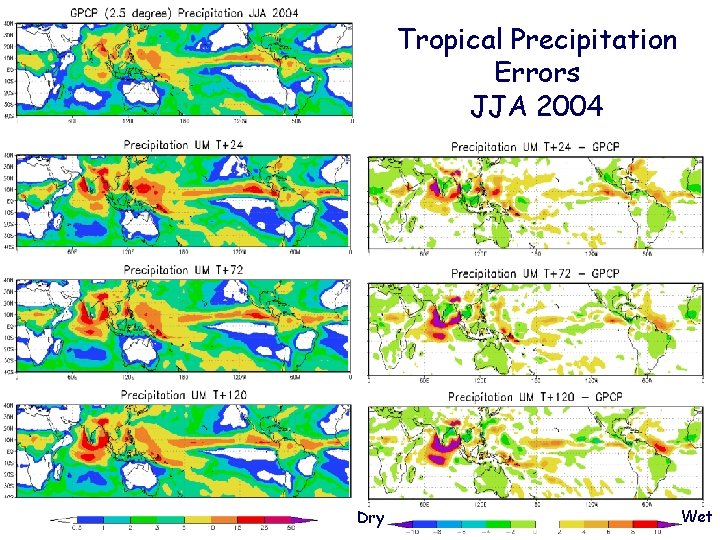
Tropical Precipitation Errors JJA 2004 © Crown copyright 2006 Dry Wet Page 20
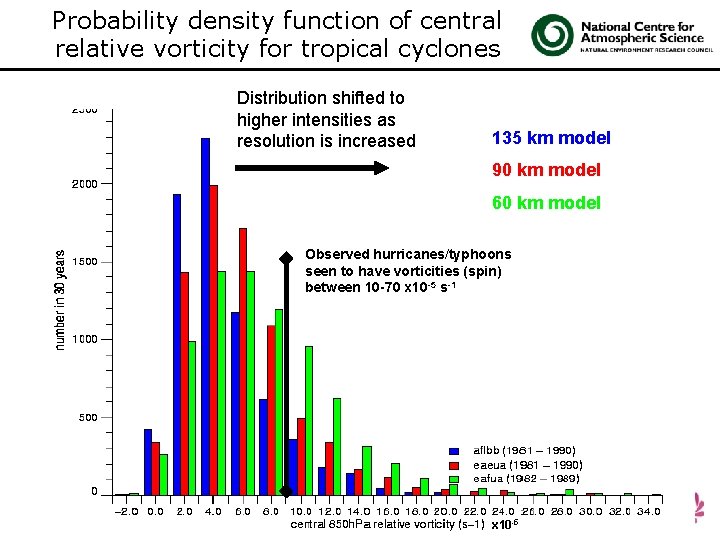
Probability density function of central relative vorticity for tropical cyclones Distribution shifted to higher intensities as resolution is increased 135 km model 90 km model 60 km model Observed hurricanes/typhoons seen to have vorticities (spin) between 10 -70 x 10 -5 s-1 x 10 -5
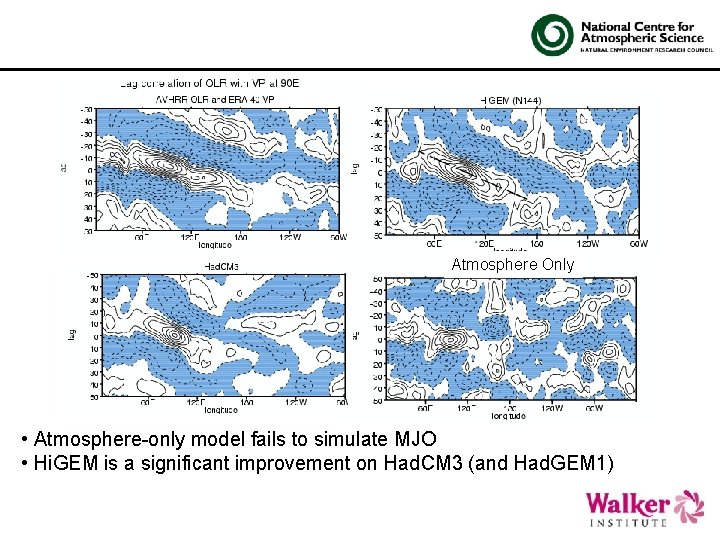
Atmosphere Only • Atmosphere-only model fails to simulate MJO • Hi. GEM is a significant improvement on Had. CM 3 (and Had. GEM 1)
Gcf
Gcm
George c marshall high school athletics
D=m/v triangle
Gcm
Gcmlab
Fallon sherrock smoking
Yes or no
Clinical simulations in nursing education
Chris harding simulations
Tcad simulations
World history simulations
Simulations for solid state physics
Payroll card
Pinpoint simulations 777
Baton simulations
Service quality and productivity
Quality business a level
Improving own learning and performance examples
Aliens meaning
Improving vocabulary with word parts and context clues
Improving decision making and managing knowledge
Fspos