When spikes do matter speed and plasticity Thomas
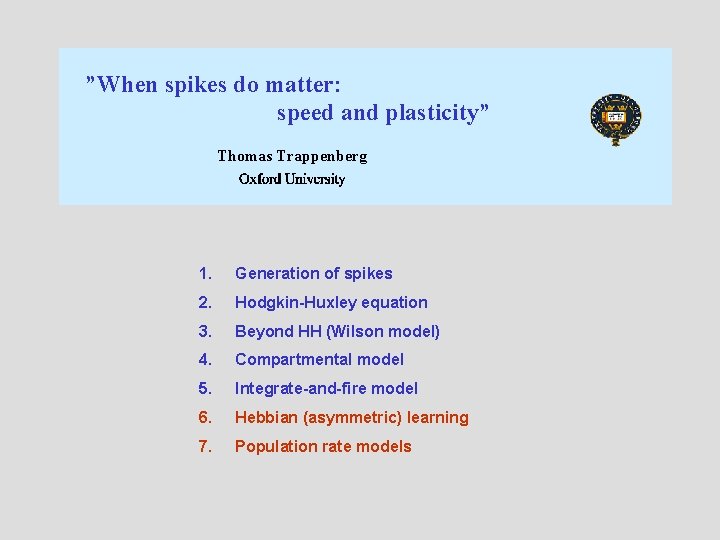
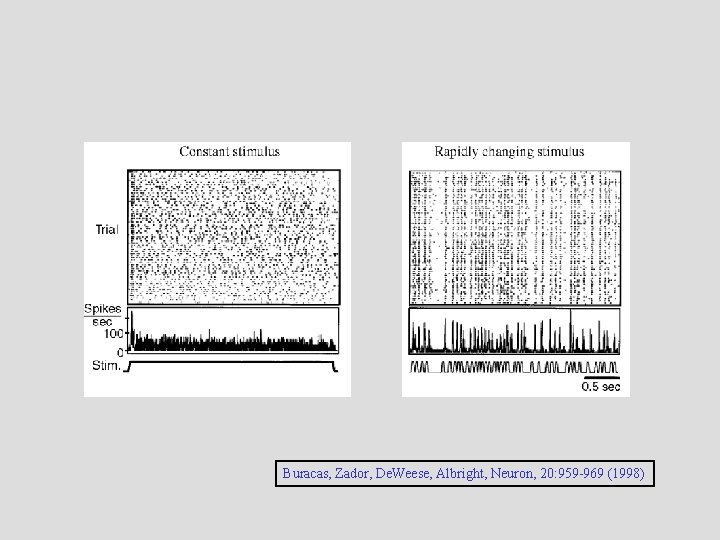
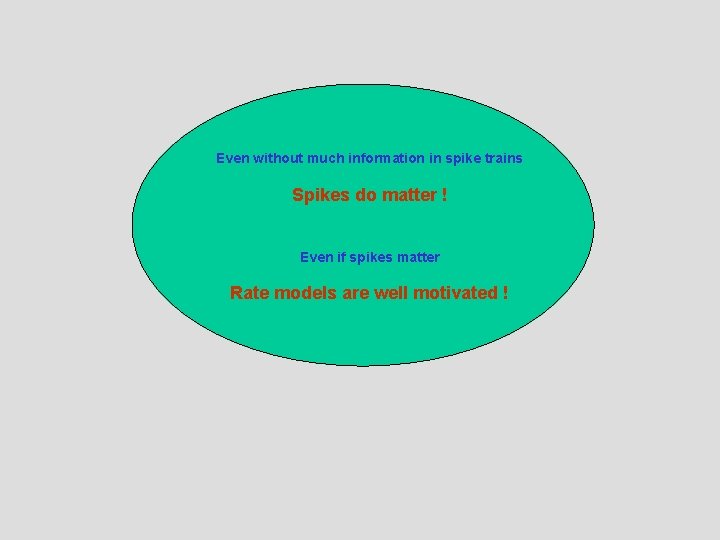
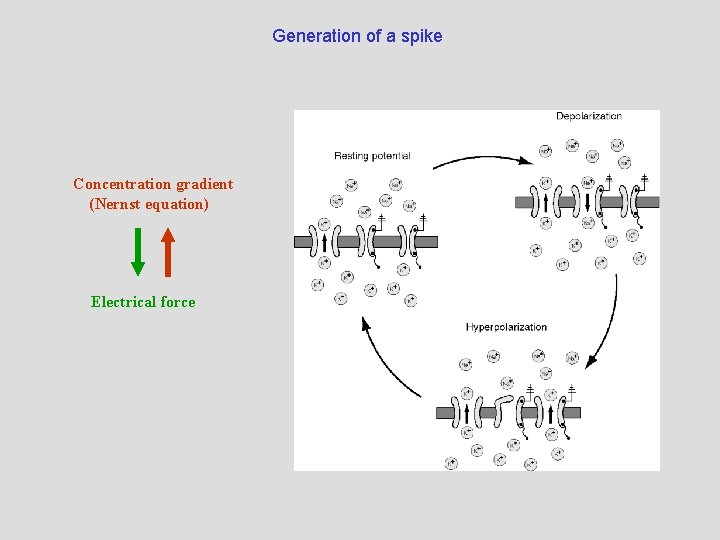
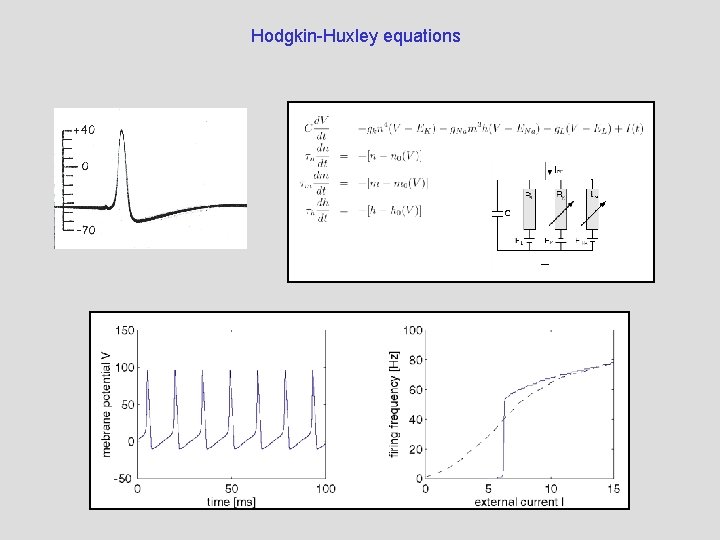
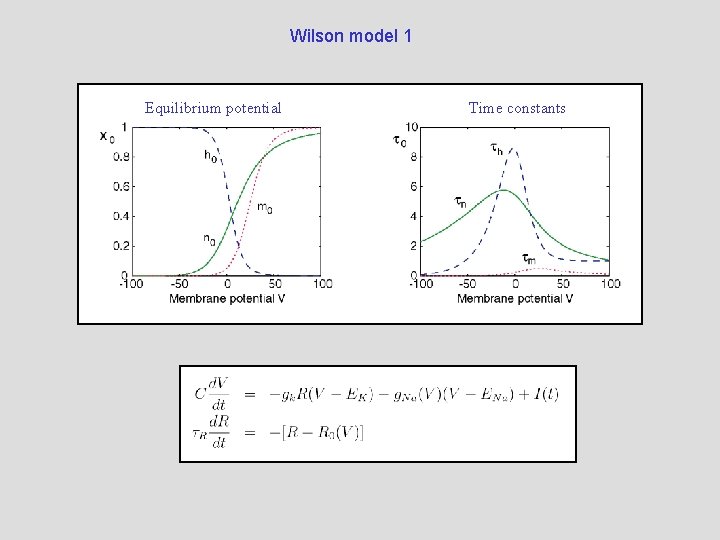
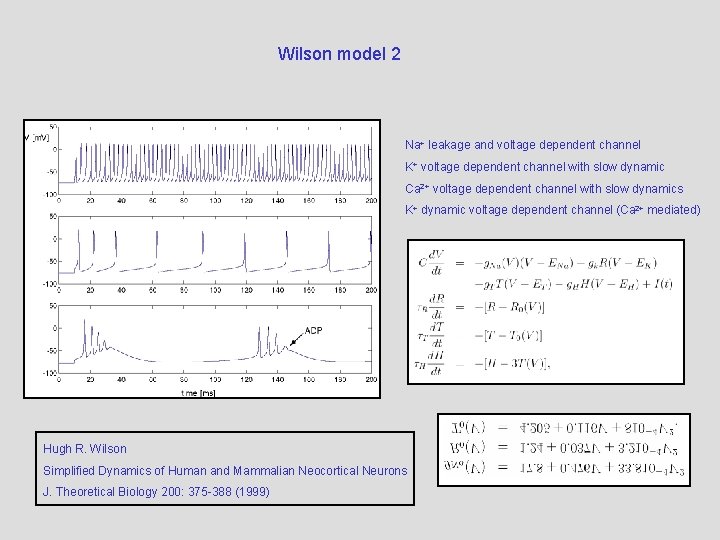
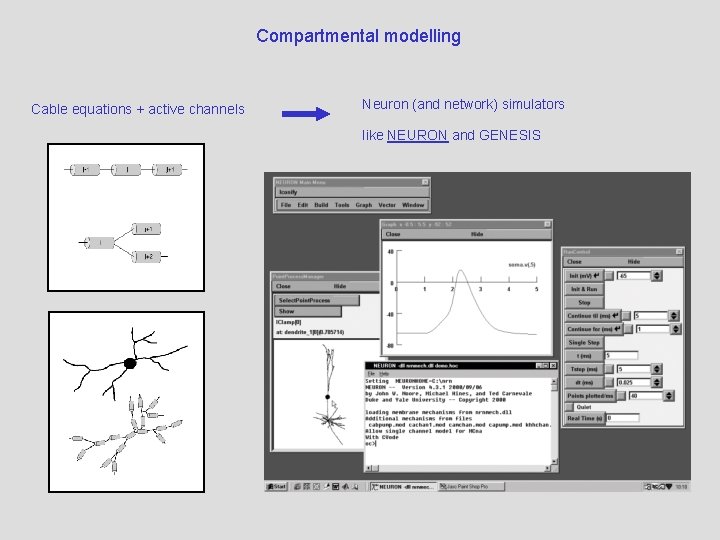
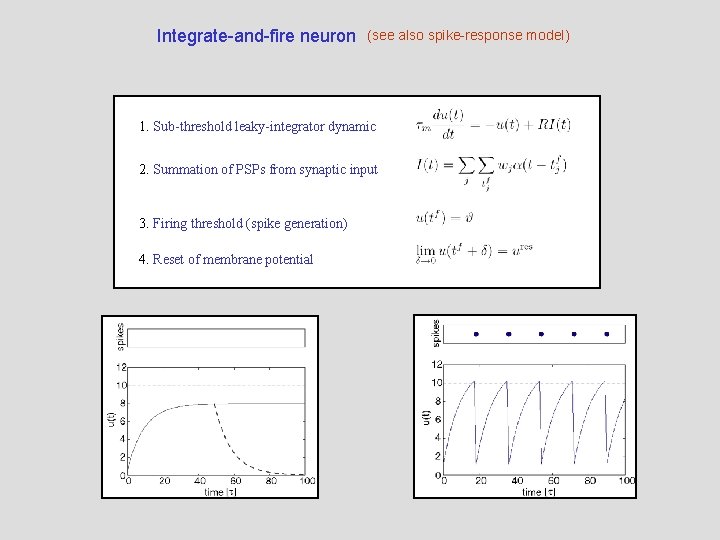
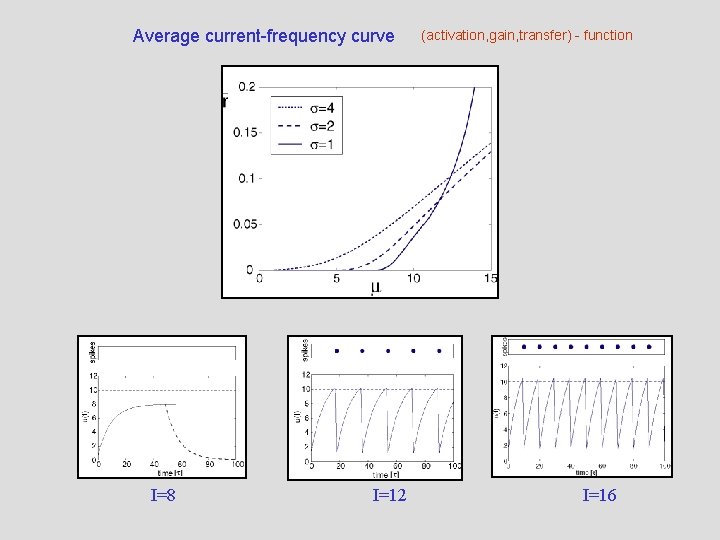
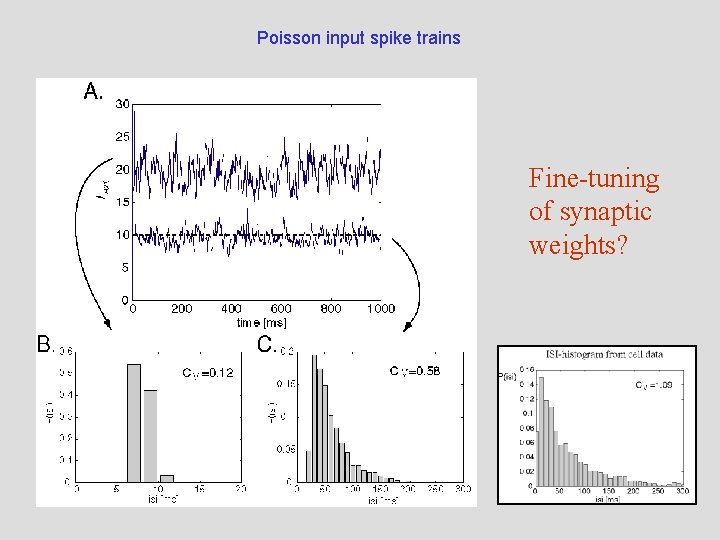
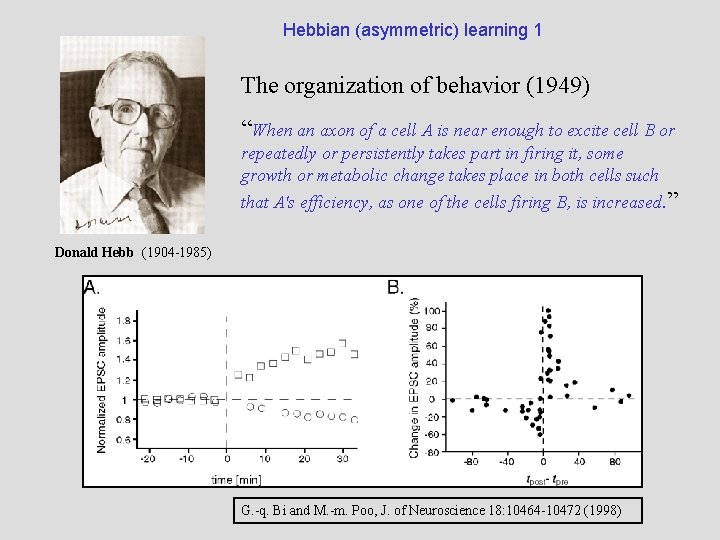
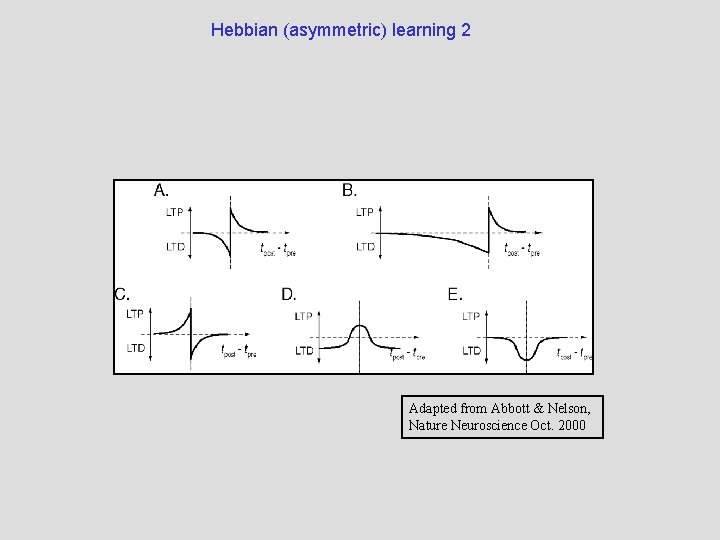
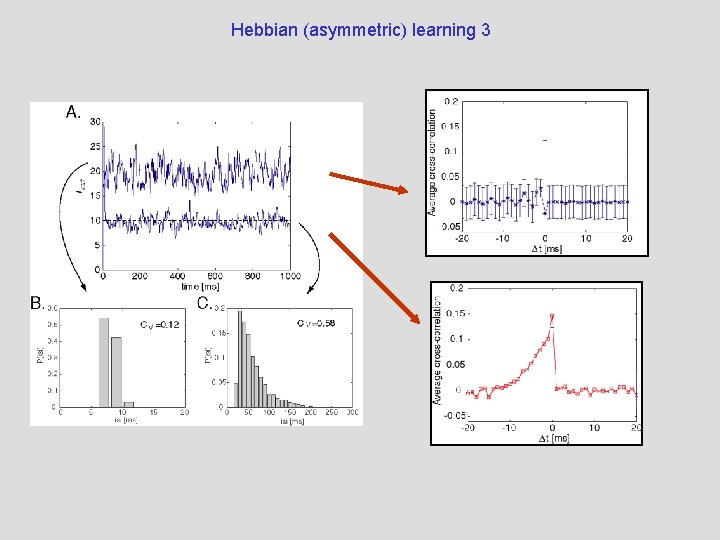
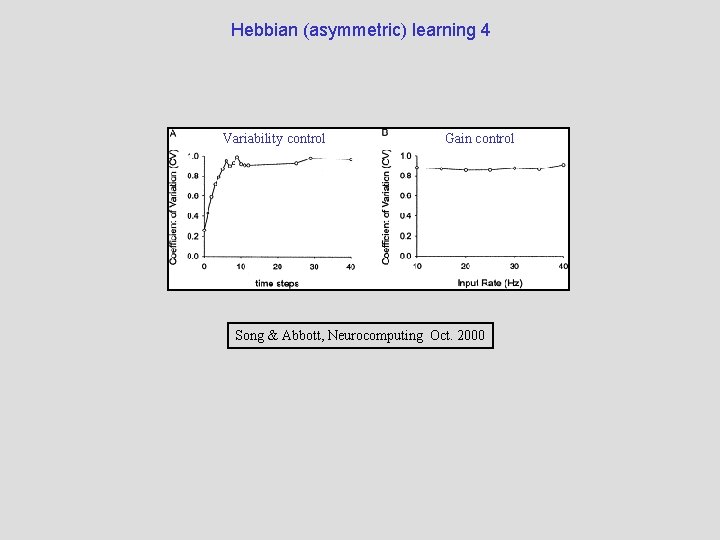
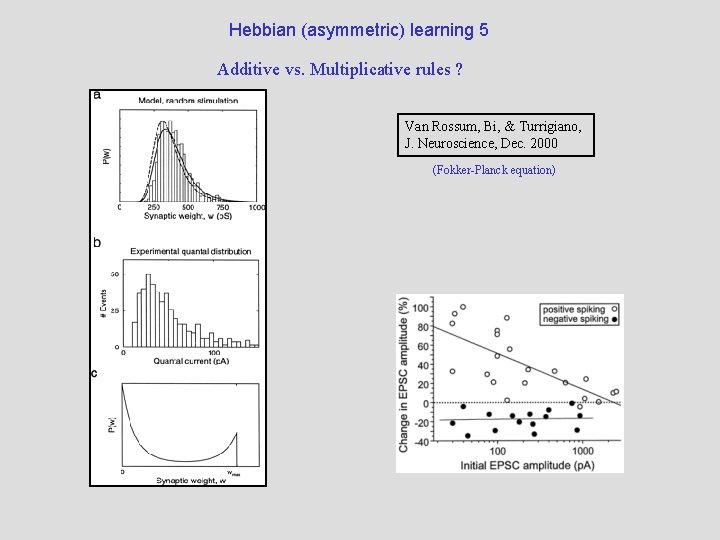
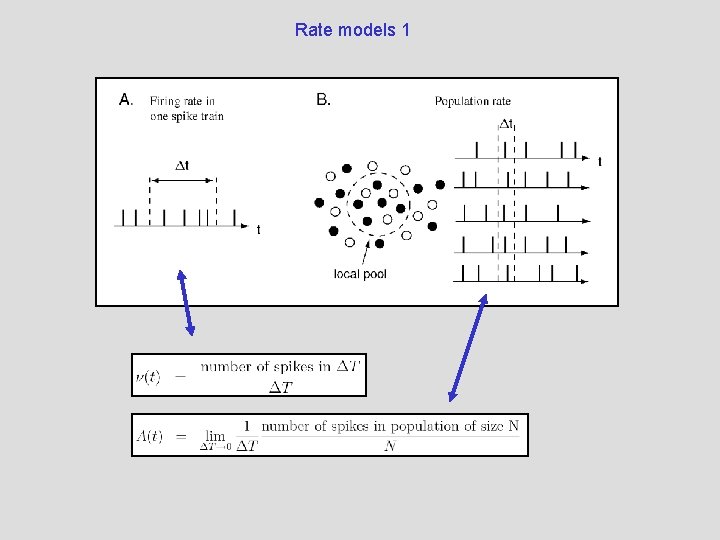
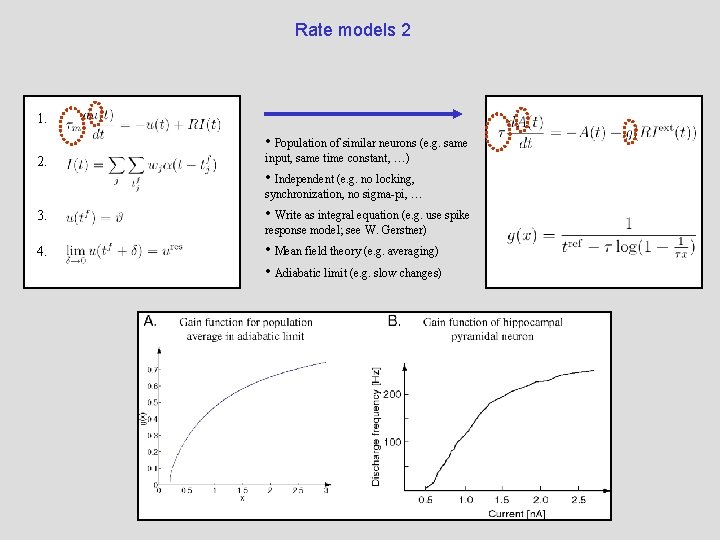
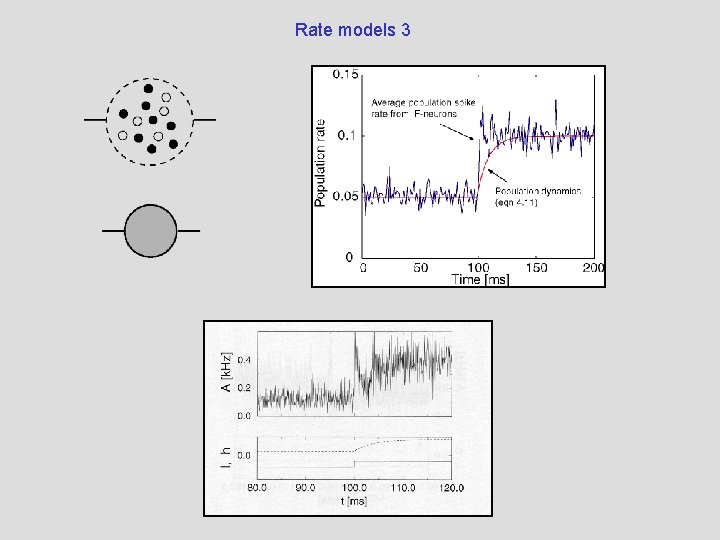
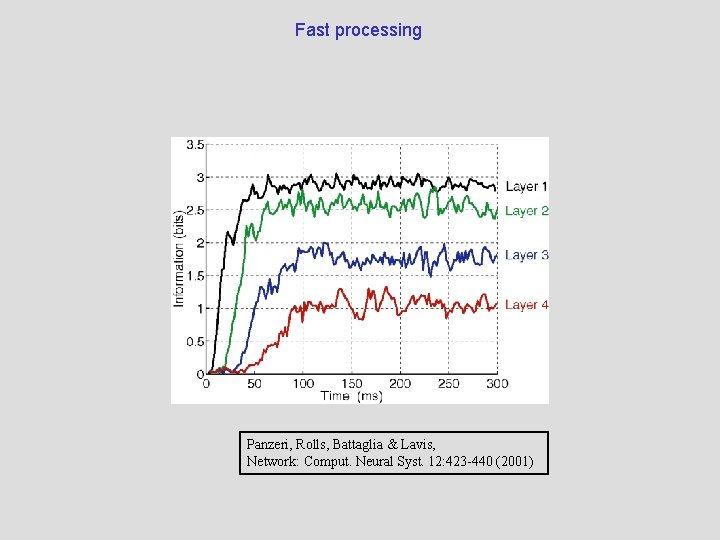
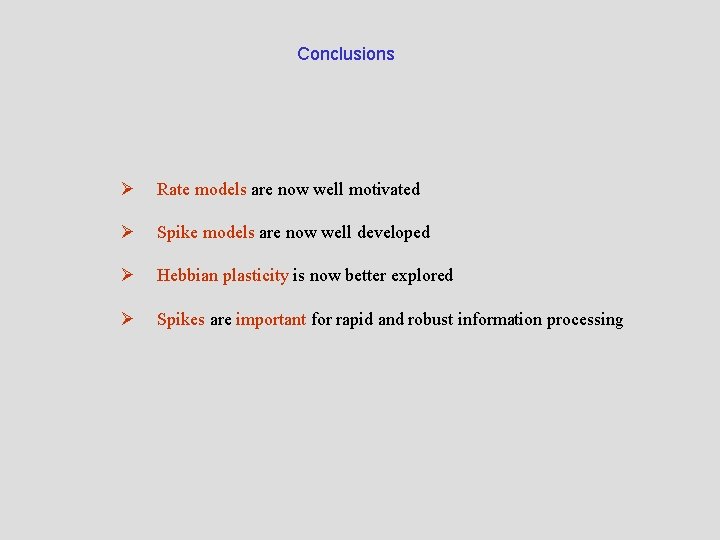
- Slides: 21
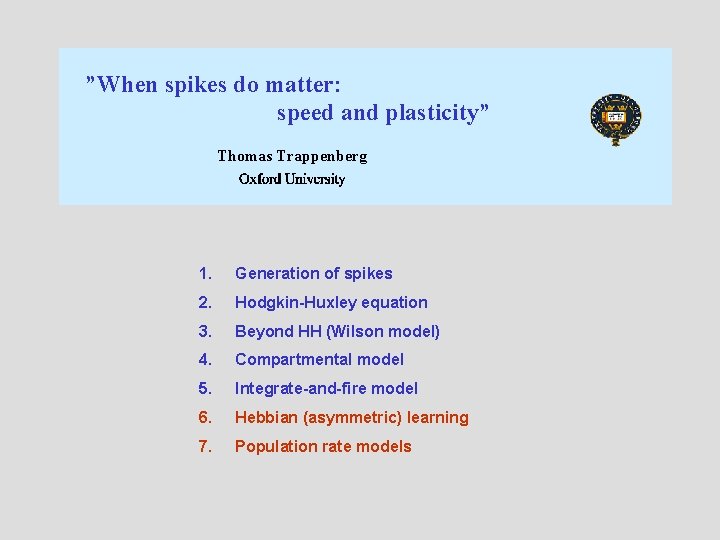
”When spikes do matter: speed and plasticity” Thomas Trappenberg 1. Generation of spikes 2. Hodgkin-Huxley equation 3. Beyond HH (Wilson model) 4. Compartmental model 5. Integrate-and-fire model 6. Hebbian (asymmetric) learning 7. Population rate models
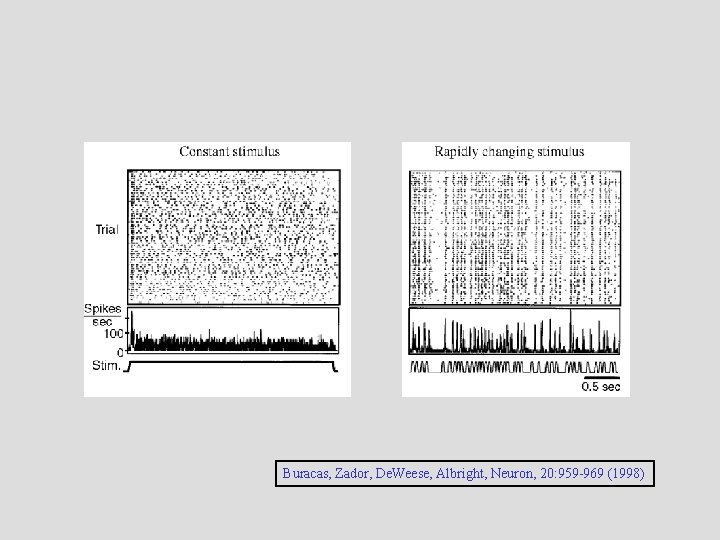
Buracas, Zador, De. Weese, Albright, Neuron, 20: 959 -969 (1998)
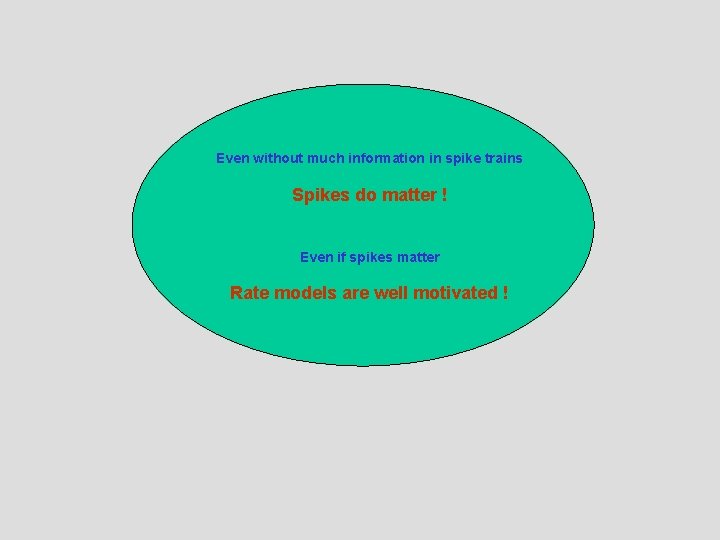
Even without much information in spike trains Spikes do matter ! Even if spikes matter Rate models are well motivated !
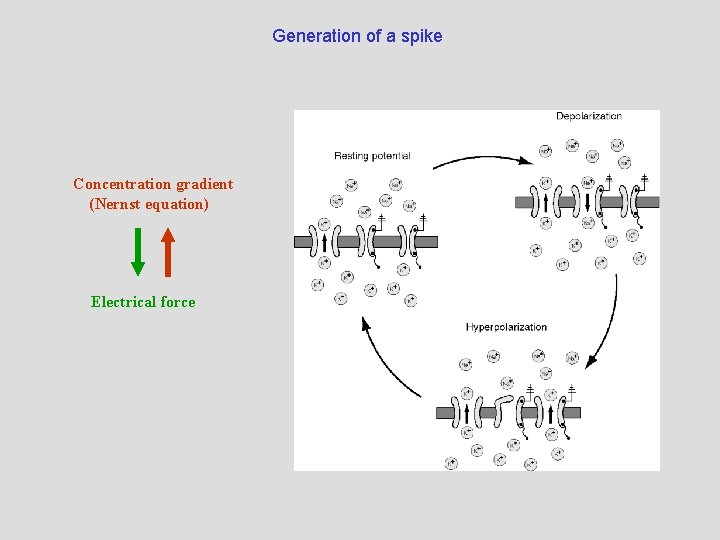
Generation of a spike Concentration gradient (Nernst equation) Electrical force
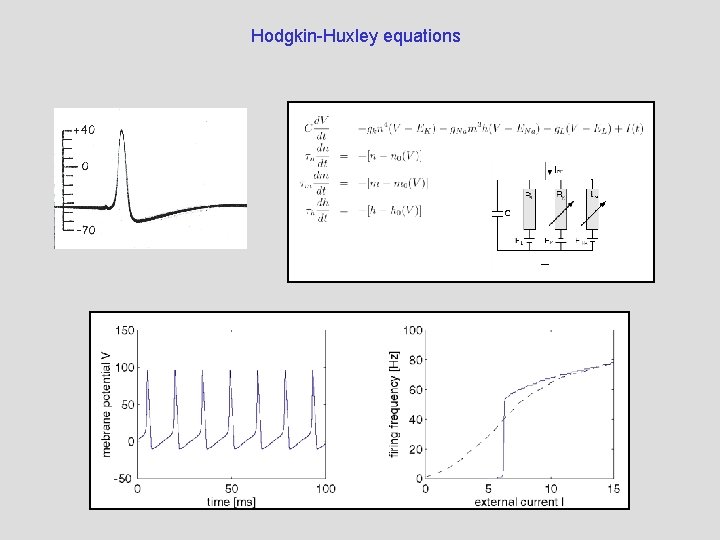
Hodgkin-Huxley equations
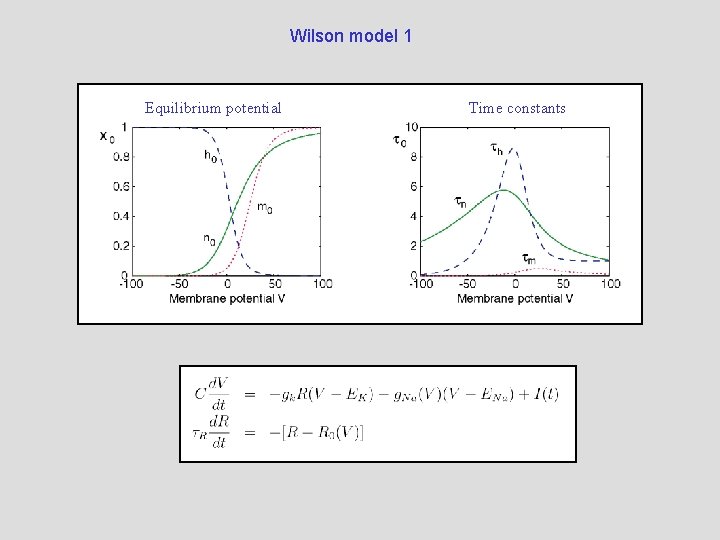
Wilson model 1 Equilibrium potential Time constants
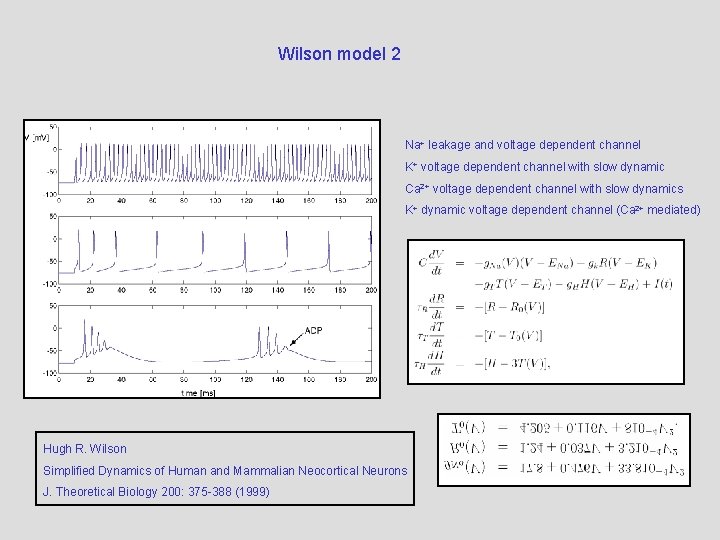
Wilson model 2 Na+ leakage and voltage dependent channel K+ voltage dependent channel with slow dynamic Ca 2+ voltage dependent channel with slow dynamics K+ dynamic voltage dependent channel (Ca 2+ mediated) Hugh R. Wilson Simplified Dynamics of Human and Mammalian Neocortical Neurons J. Theoretical Biology 200: 375 -388 (1999)
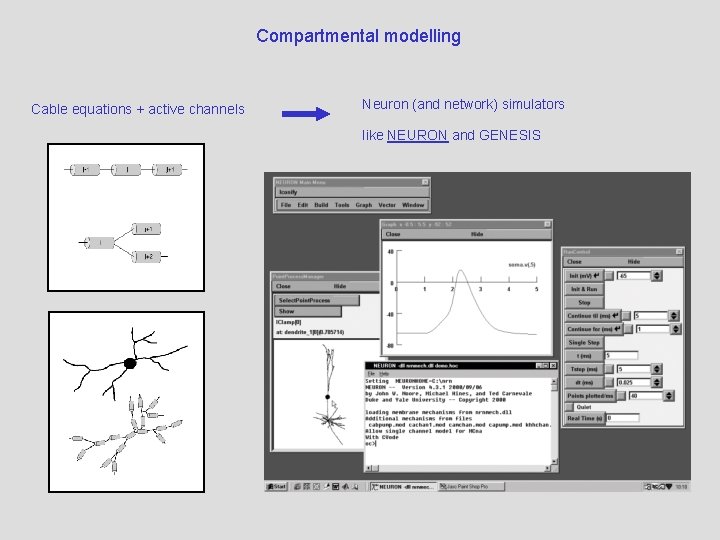
Compartmental modelling Cable equations + active channels Neuron (and network) simulators like NEURON and GENESIS
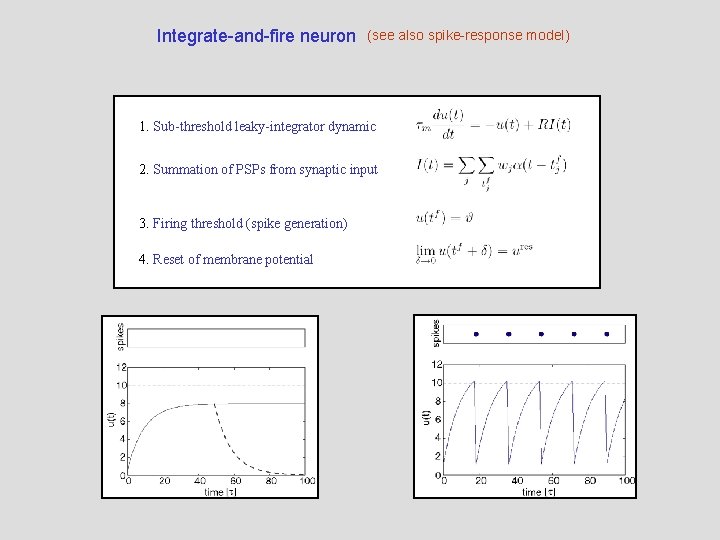
Integrate-and-fire neuron (see also spike-response model) 1. Sub-threshold leaky-integrator dynamic 2. Summation of PSPs from synaptic input 3. Firing threshold (spike generation) 4. Reset of membrane potential
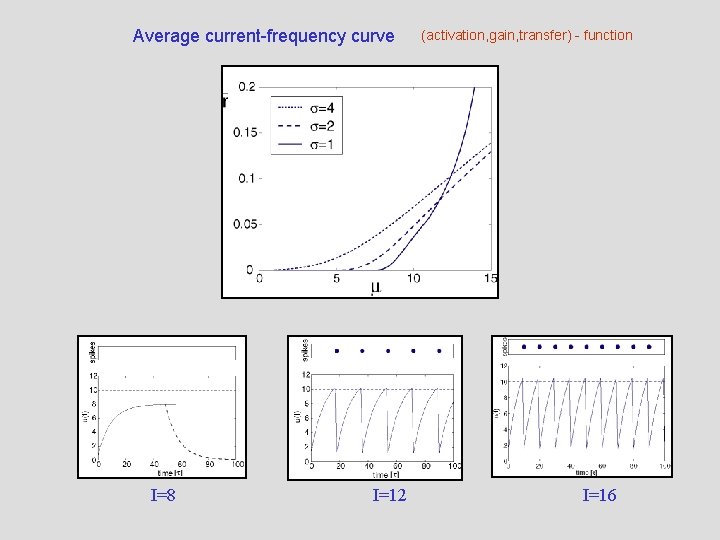
Average current-frequency curve I=8 I=12 (activation, gain, transfer) - function I=16
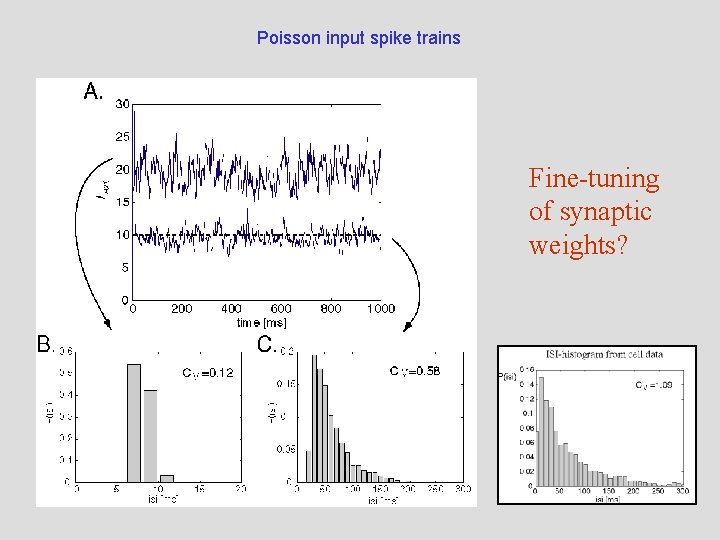
Poisson input spike trains Fine-tuning of synaptic weights?
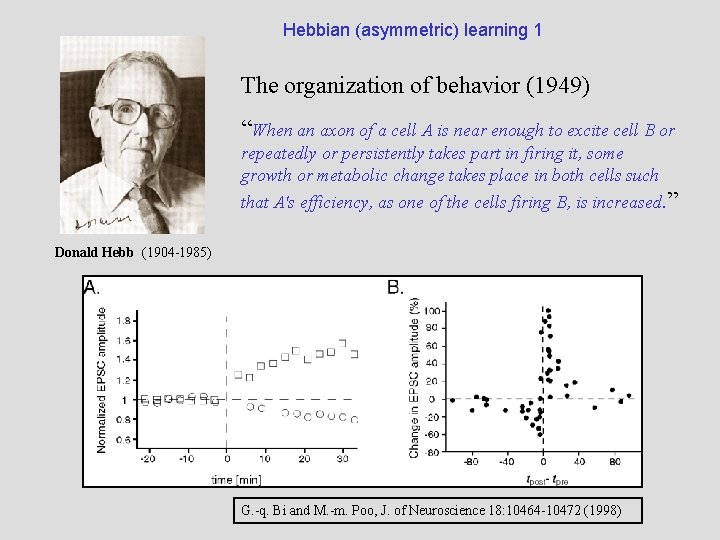
Hebbian (asymmetric) learning 1 The organization of behavior (1949) “When an axon of a cell A is near enough to excite cell B or repeatedly or persistently takes part in firing it, some growth or metabolic change takes place in both cells such that A's efficiency, as one of the cells firing B, is increased. ” Donald Hebb (1904 -1985) G. -q. Bi and M. -m. Poo, J. of Neuroscience 18: 10464 -10472 (1998)
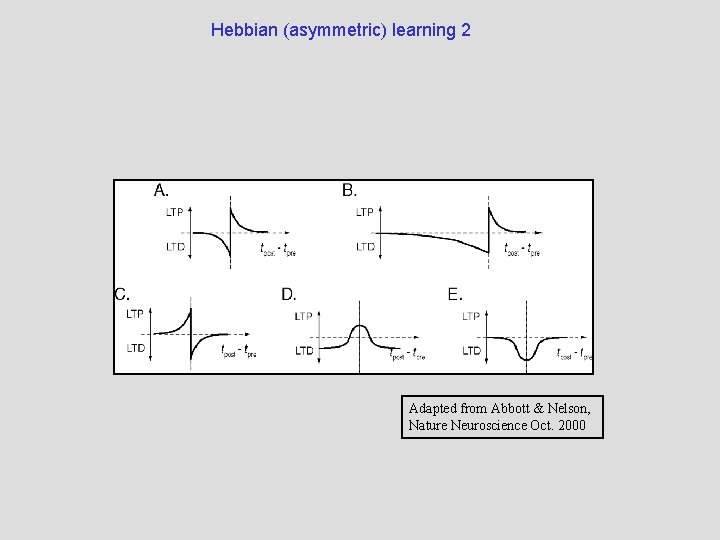
Hebbian (asymmetric) learning 2 Adapted from Abbott & Nelson, Nature Neuroscience Oct. 2000
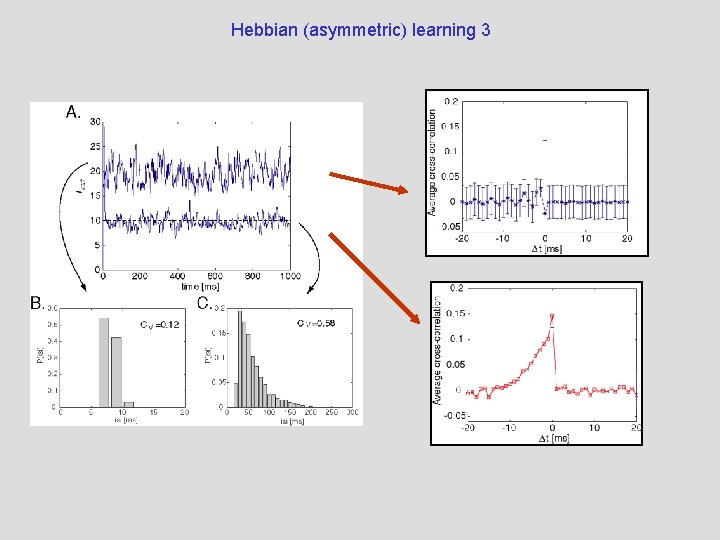
Hebbian (asymmetric) learning 3
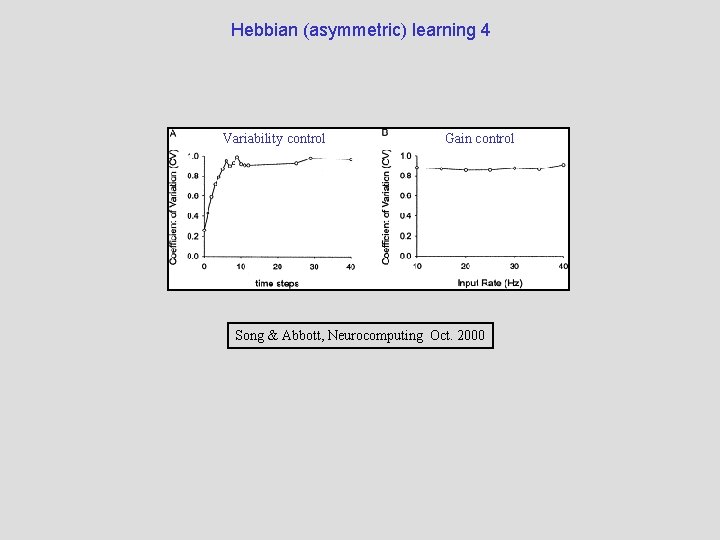
Hebbian (asymmetric) learning 4 Variability control Gain control Song & Abbott, Neurocomputing Oct. 2000
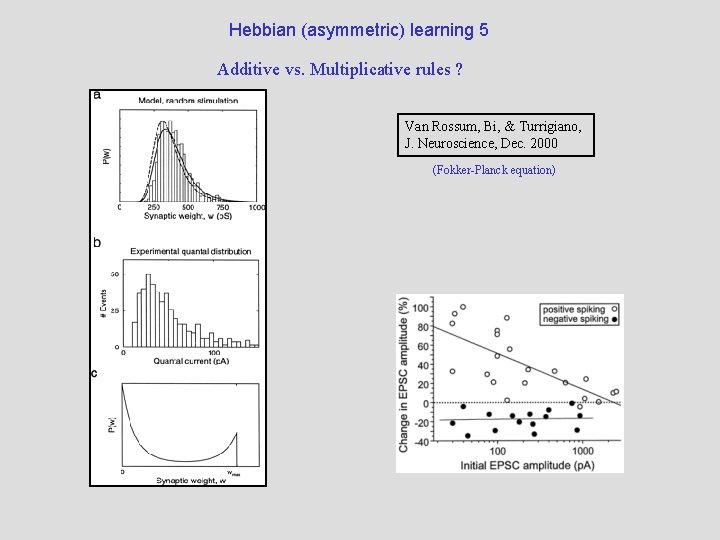
Hebbian (asymmetric) learning 5 Additive vs. Multiplicative rules ? Van Rossum, Bi, & Turrigiano, J. Neuroscience, Dec. 2000 (Fokker-Planck equation)
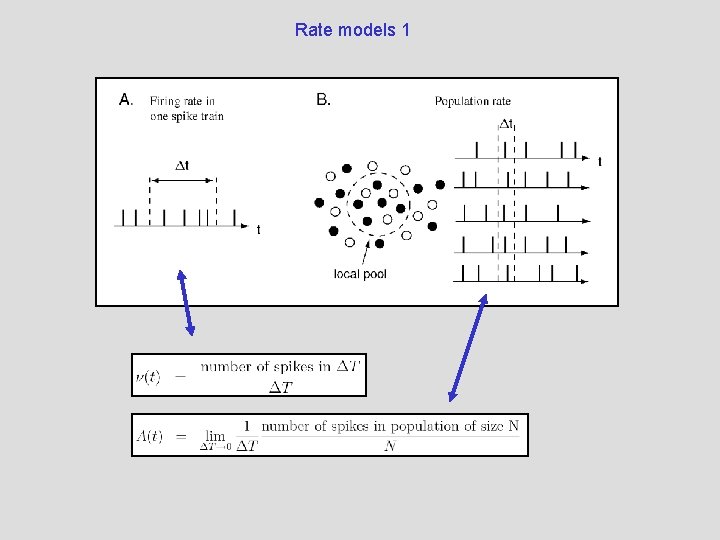
Rate models 1
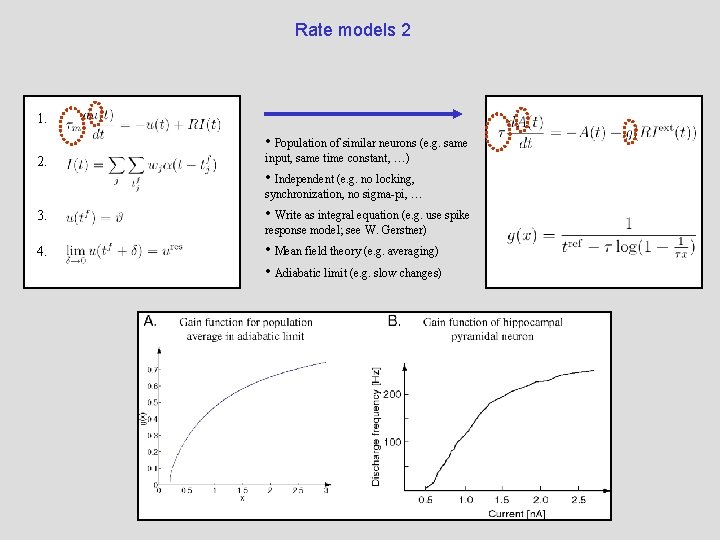
Rate models 2 1. • Population of similar neurons (e. g. same 2. input, same time constant, …) • Independent (e. g. no locking, synchronization, no sigma-pi, … 3. • Write as integral equation (e. g. use spike 4. • Mean field theory (e. g. averaging) • Adiabatic limit (e. g. slow changes) response model; see W. Gerstner)
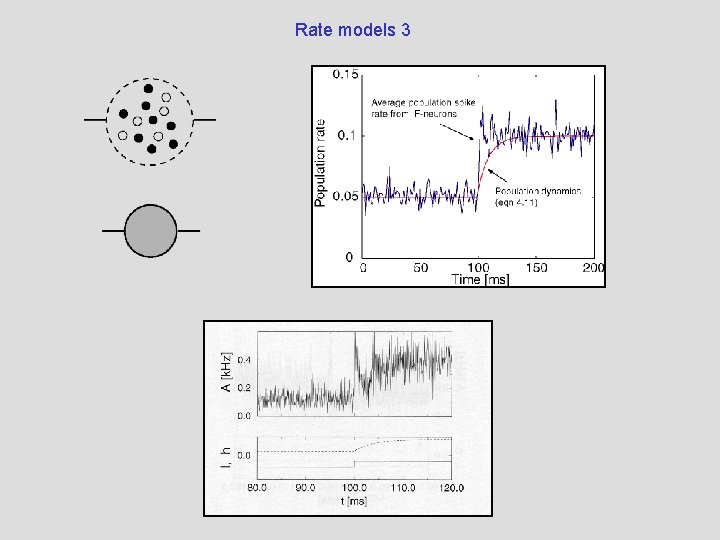
Rate models 3
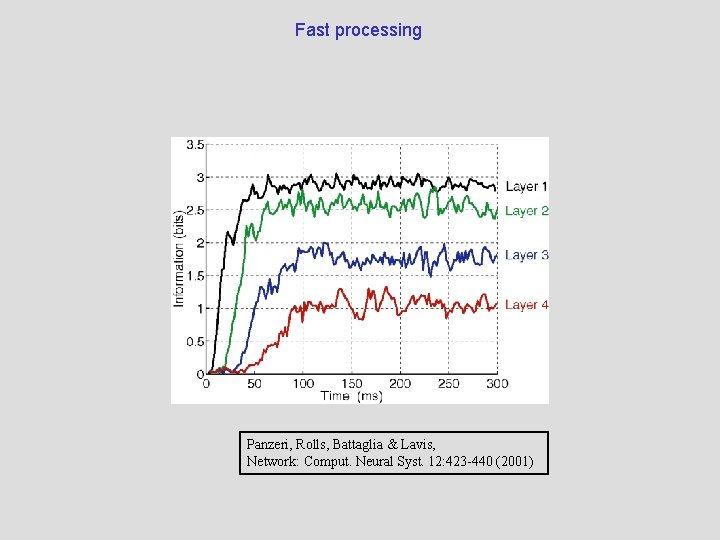
Fast processing Panzeri, Rolls, Battaglia & Lavis, Network: Comput. Neural Syst. 12: 423 -440 (2001)
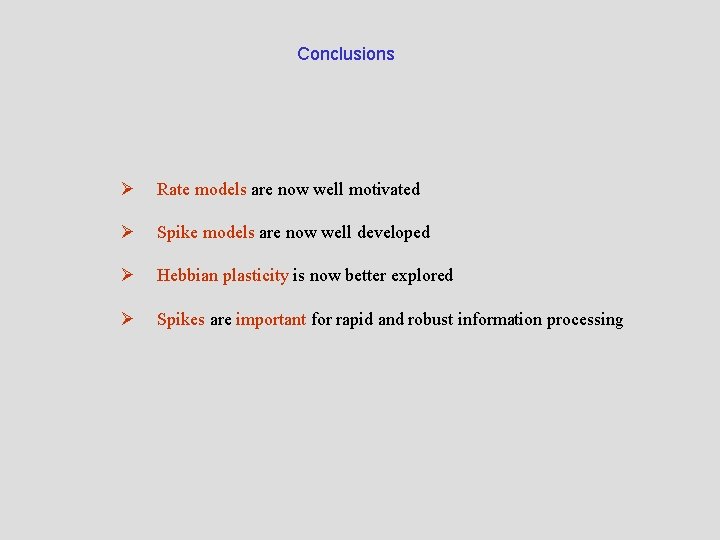
Conclusions Ø Rate models are now well motivated Ø Spike models are now well developed Ø Hebbian plasticity is now better explored Ø Spikes are important for rapid and robust information processing
Becker
Spike timing dependent plasticity
Arash ashari
Adaptive plasticity
Stdp
Plastic limit formula
Plasticity in earth's layers
Plasticity
Plasticity in earth's layers
Distance formula speed time
Thomas mocker and thomas stewart
Grey matter reliaquest
Dural septa
Gray matter and white matter
Ncl caudatus
Passengers can help a driver better manage emotions by
Speed detection of moving vehicle
Spikes mnemonic
Ne regional epilepsy group
Seizure
Florina nechita
Darius power spikes