Update on Kaon Neural Net Training Kalanand Mishra
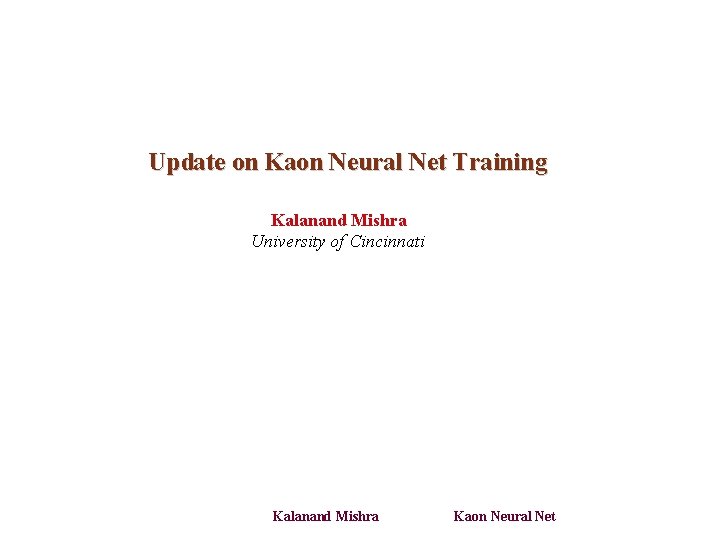
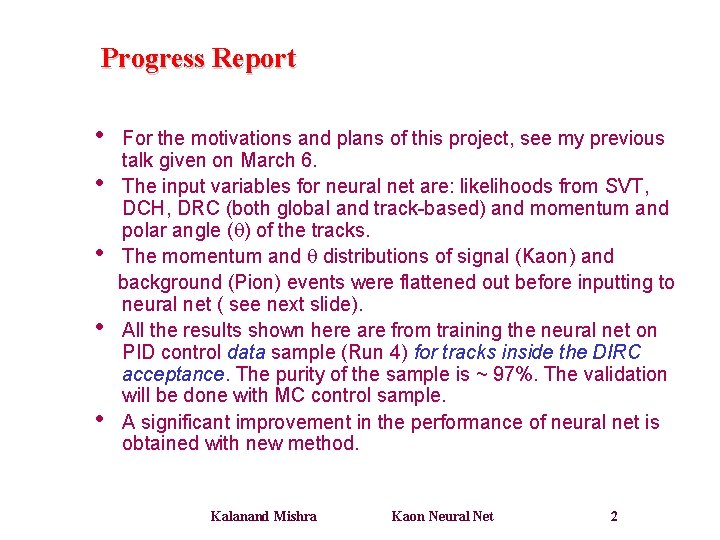
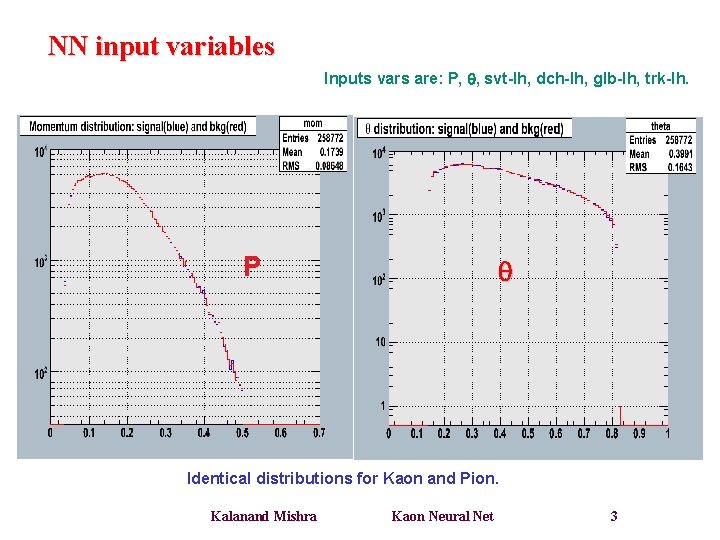
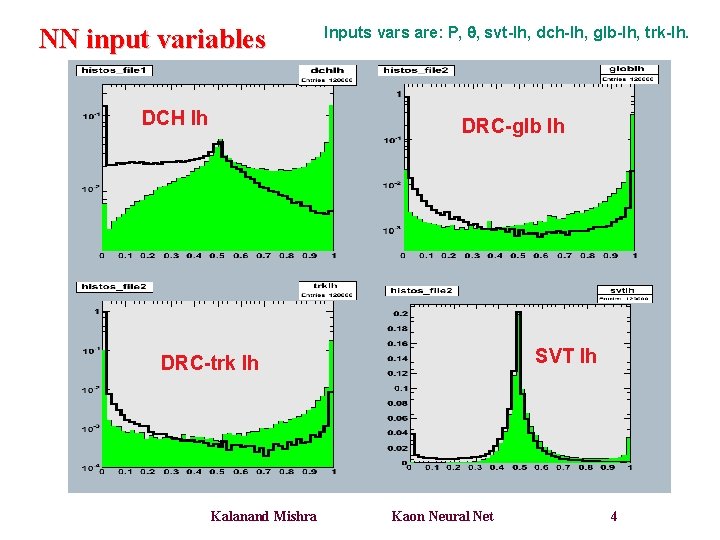
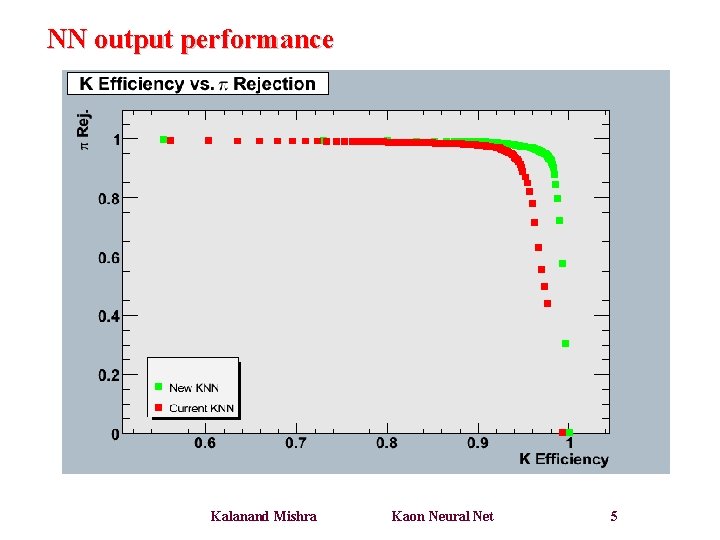
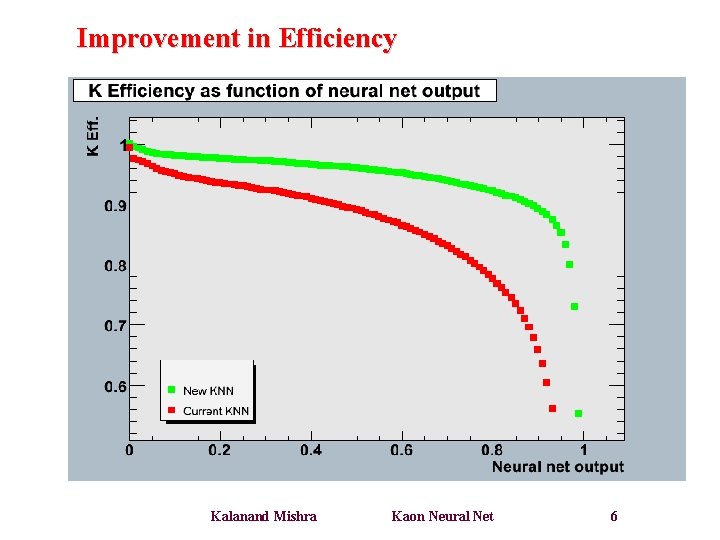
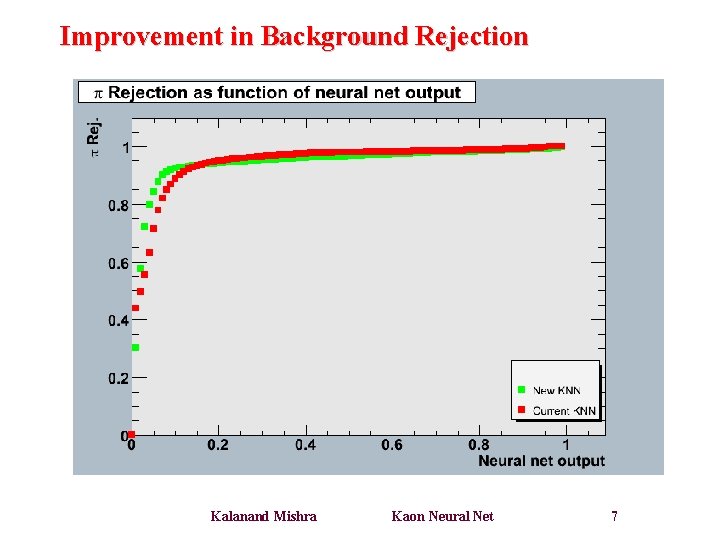
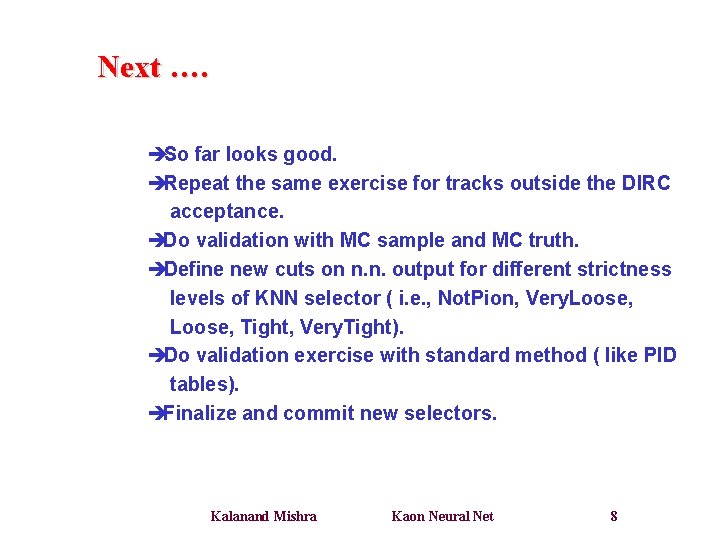
- Slides: 8
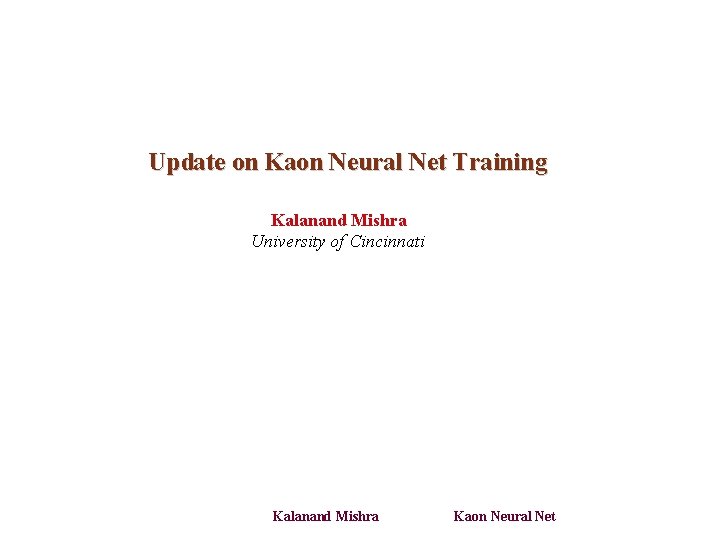
Update on Kaon Neural Net Training Kalanand Mishra University of Cincinnati Kalanand Mishra Kaon Neural Net
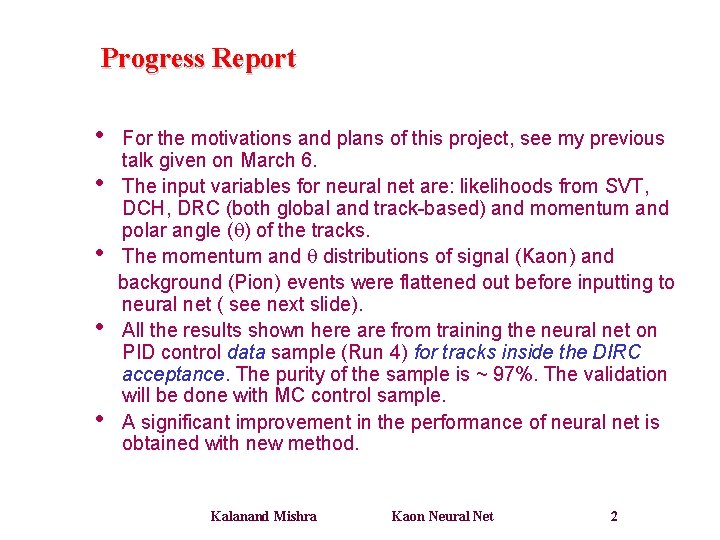
Progress Report • • • For the motivations and plans of this project, see my previous talk given on March 6. The input variables for neural net are: likelihoods from SVT, DCH, DRC (both global and track-based) and momentum and polar angle ( ) of the tracks. The momentum and distributions of signal (Kaon) and background (Pion) events were flattened out before inputting to neural net ( see next slide). All the results shown here are from training the neural net on PID control data sample (Run 4) for tracks inside the DIRC acceptance. The purity of the sample is ~ 97%. The validation will be done with MC control sample. A significant improvement in the performance of neural net is obtained with new method. Kalanand Mishra Kaon Neural Net 2
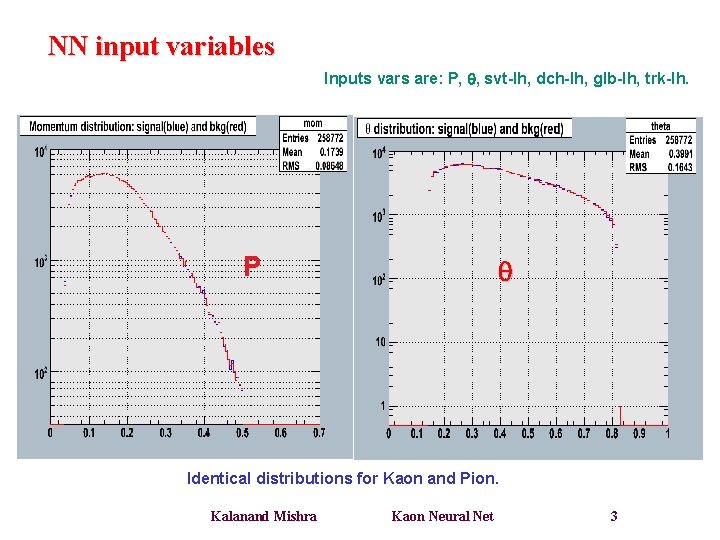
NN input variables Inputs vars are: P, , svt-lh, dch-lh, glb-lh, trk-lh. P Identical distributions for Kaon and Pion. Kalanand Mishra Kaon Neural Net 3
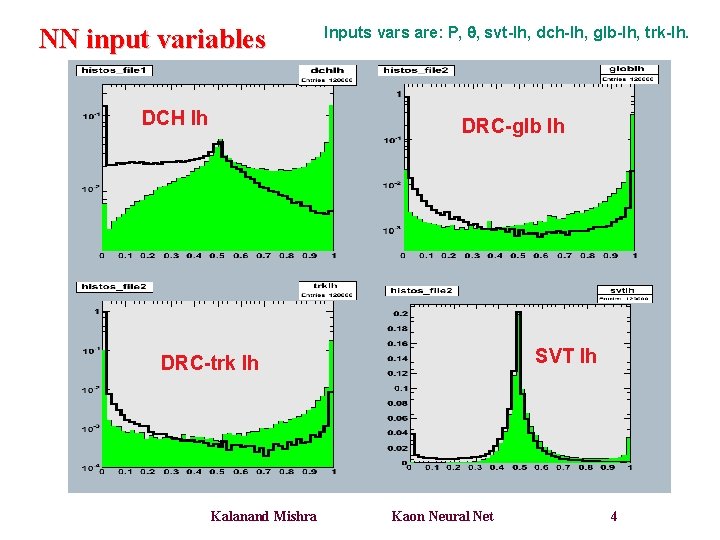
NN input variables DCH lh Inputs vars are: P, , svt-lh, dch-lh, glb-lh, trk-lh. DRC-glb lh SVT lh DRC-trk lh Kalanand Mishra Kaon Neural Net 4
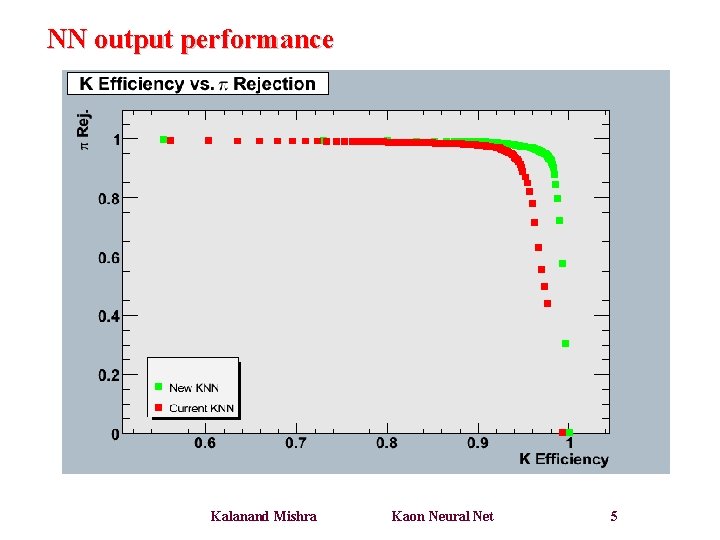
NN output performance Kalanand Mishra Kaon Neural Net 5
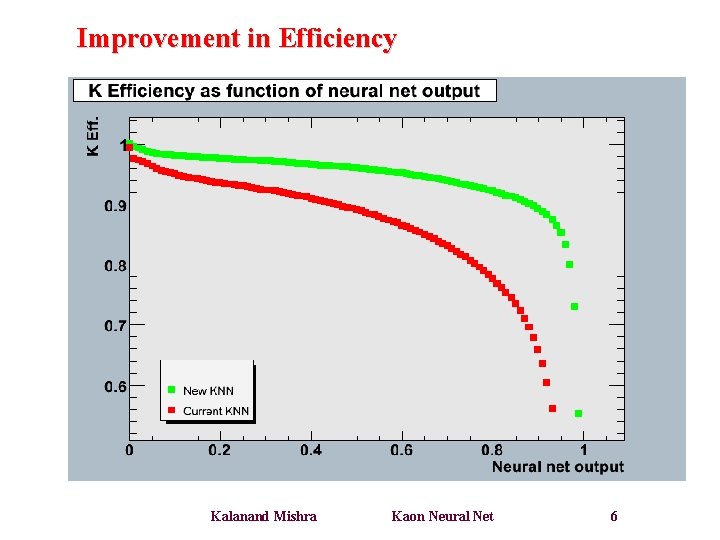
Improvement in Efficiency Kalanand Mishra Kaon Neural Net 6
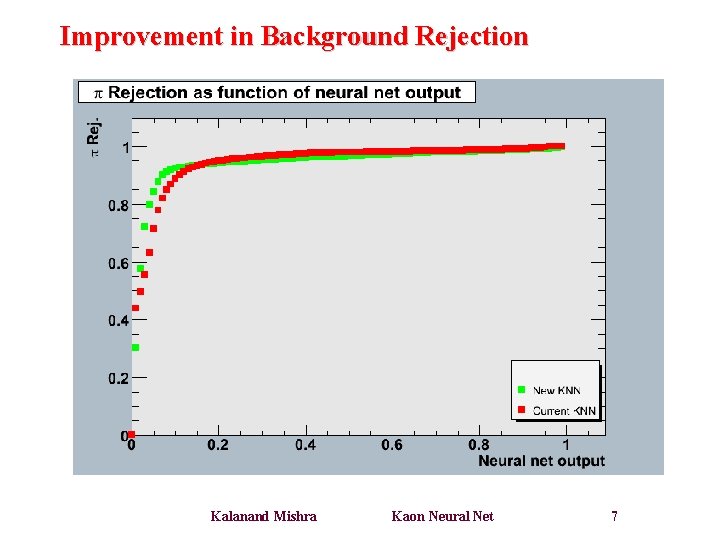
Improvement in Background Rejection Kalanand Mishra Kaon Neural Net 7
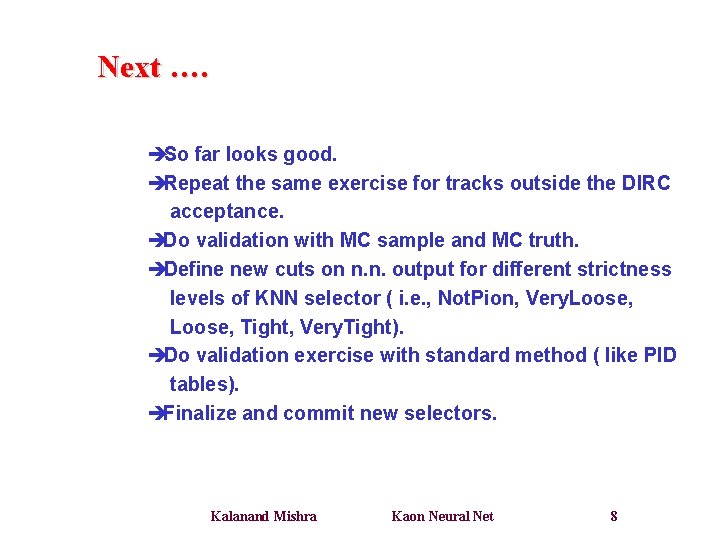
Next …. èSo far looks good. èRepeat the same exercise for tracks outside the DIRC acceptance. èDo validation with MC sample and MC truth. èDefine new cuts on n. n. output for different strictness levels of KNN selector ( i. e. , Not. Pion, Very. Loose, Tight, Very. Tight). èDo validation exercise with standard method ( like PID tables). èFinalize and commit new selectors. Kalanand Mishra Kaon Neural Net 8