New Kaon Neural Net Selectors Kalanand Mishra University
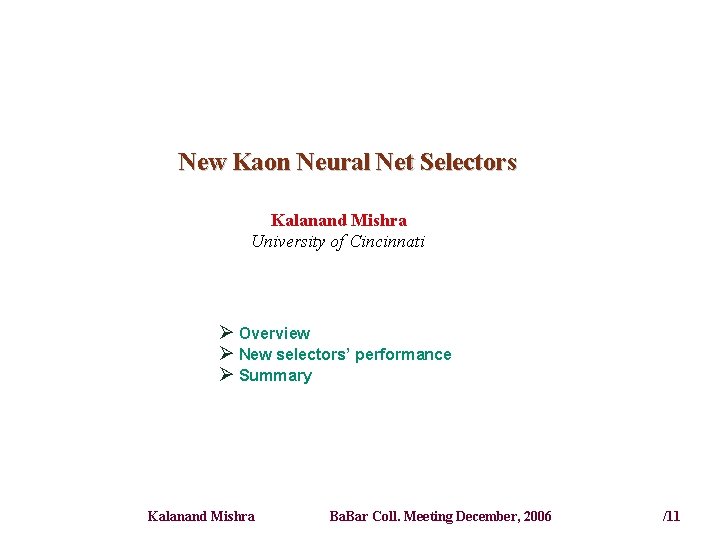
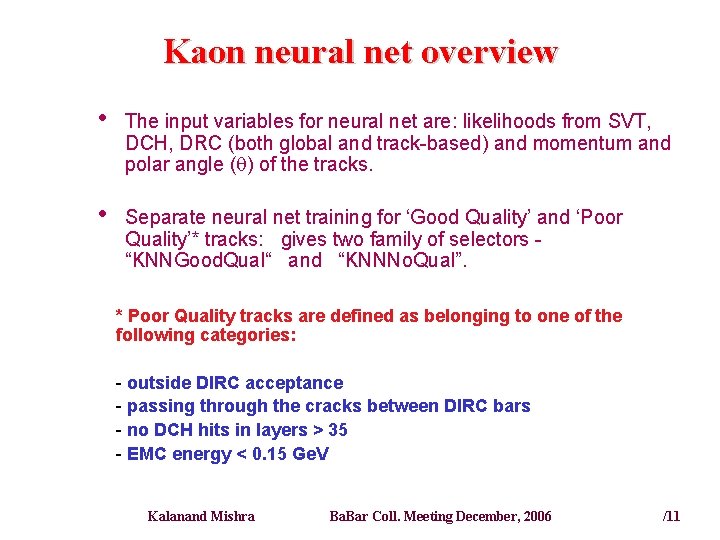
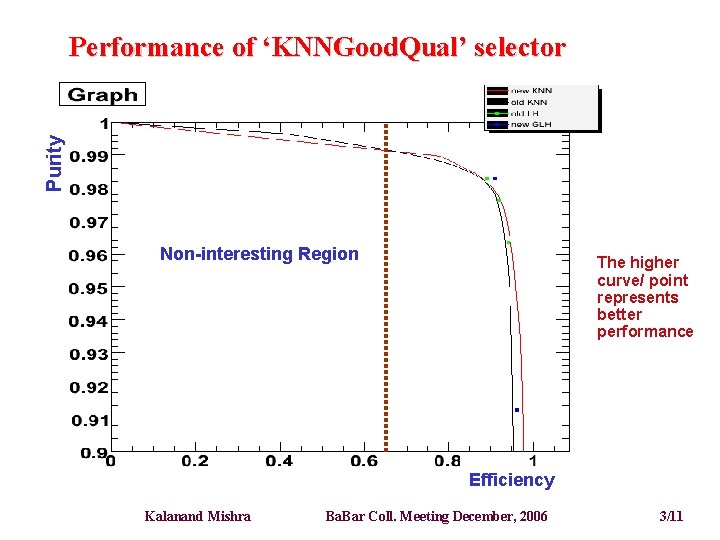
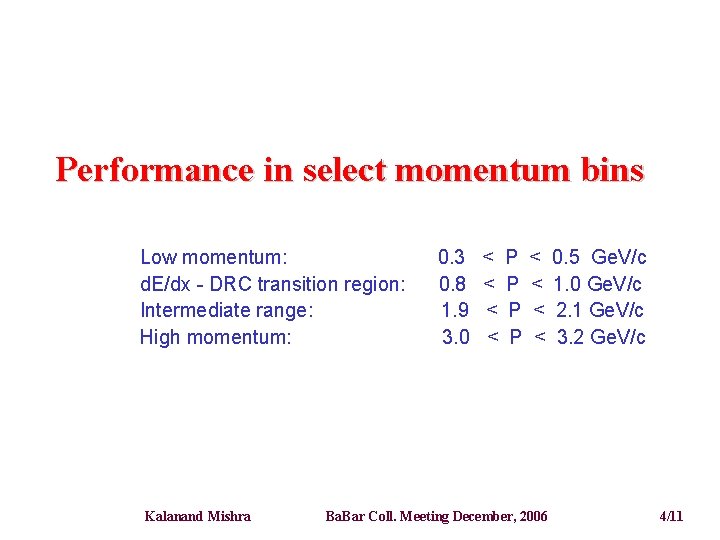
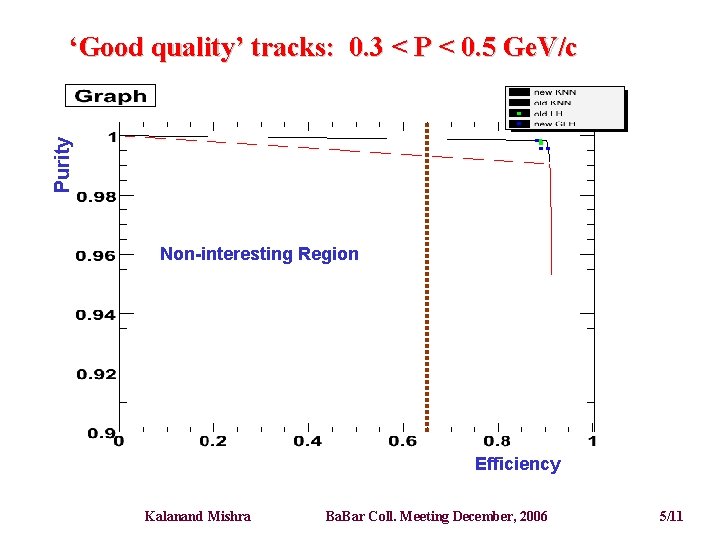
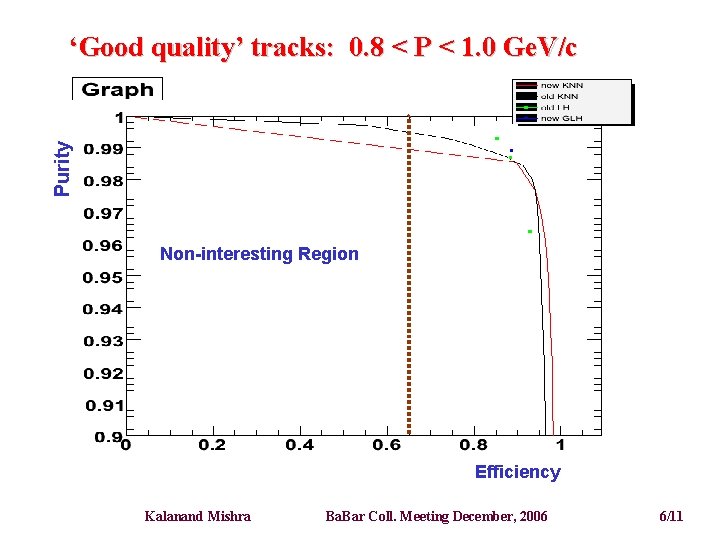
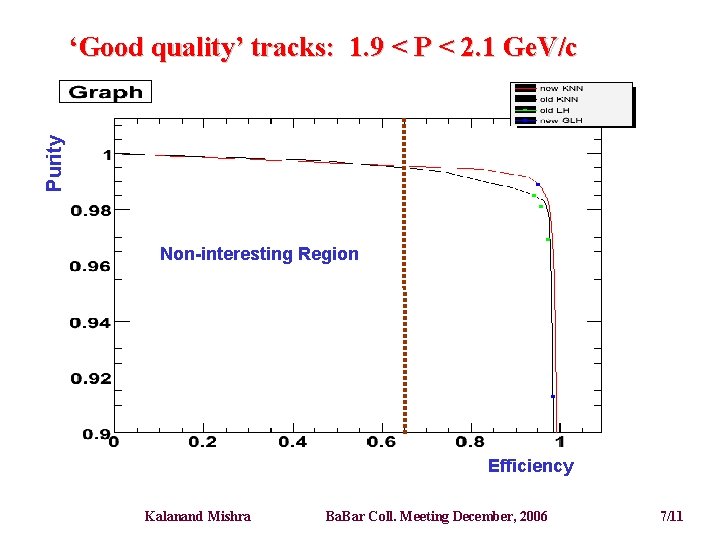
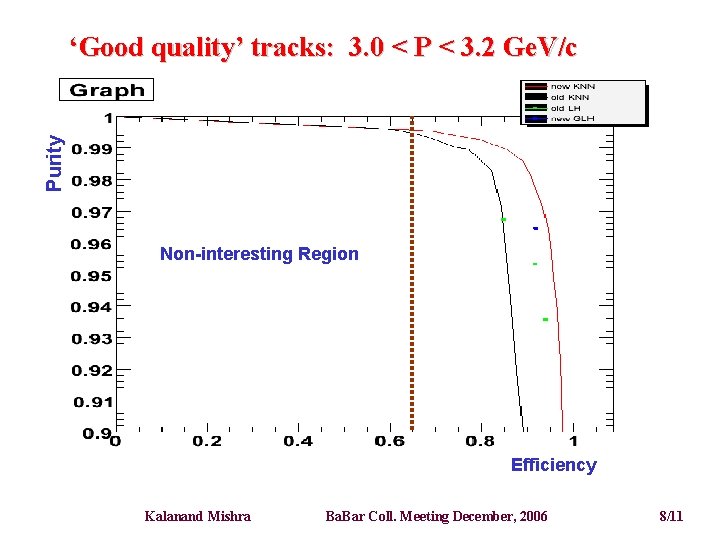
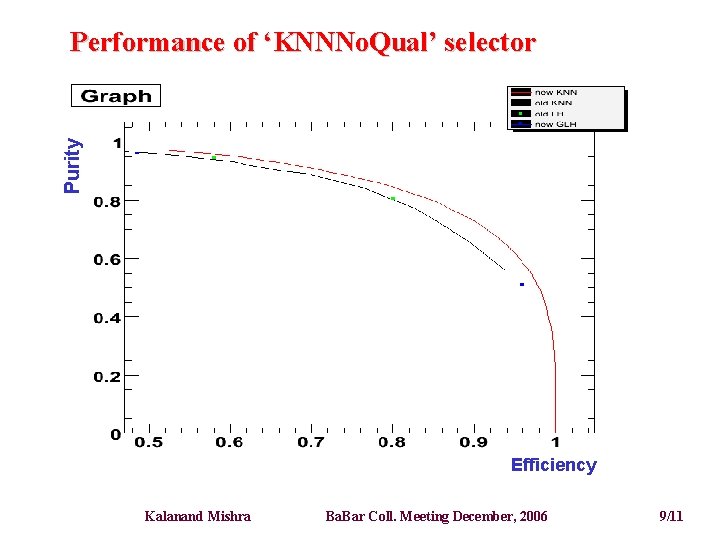
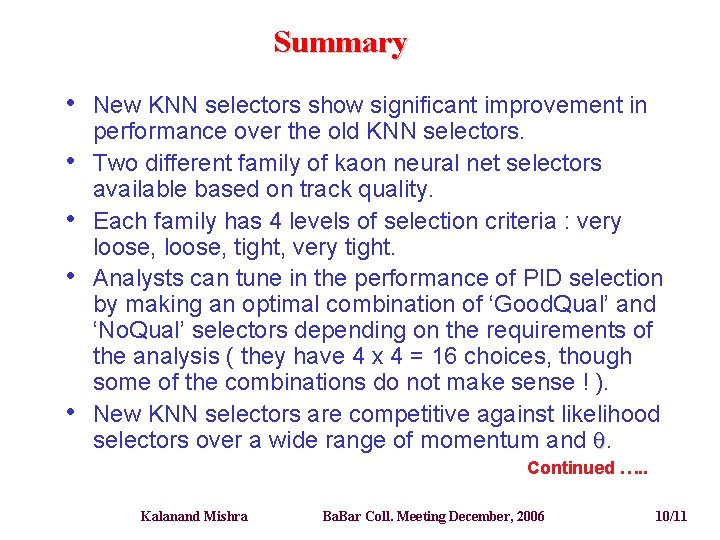
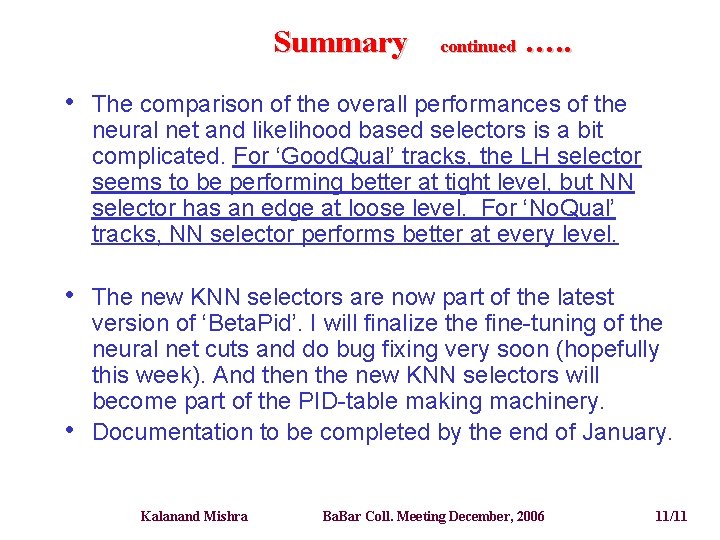
- Slides: 11
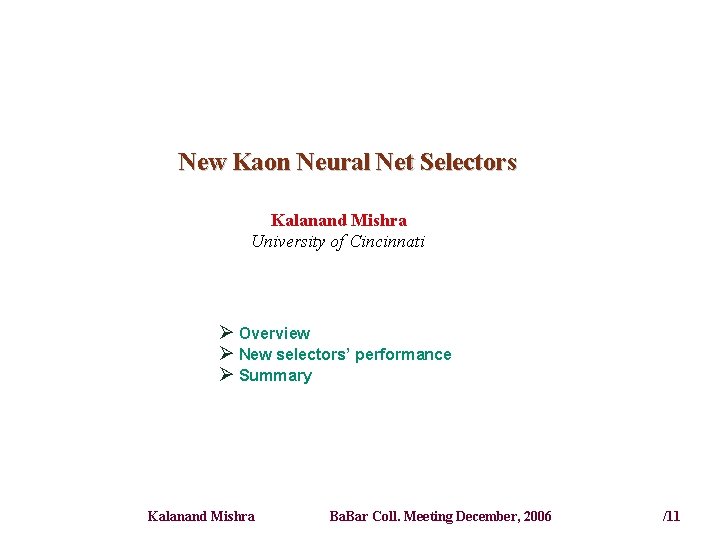
New Kaon Neural Net Selectors Kalanand Mishra University of Cincinnati Ø Overview Ø New selectors’ performance Ø Summary Kalanand Mishra Ba. Bar Coll. Meeting December, 2006 /11
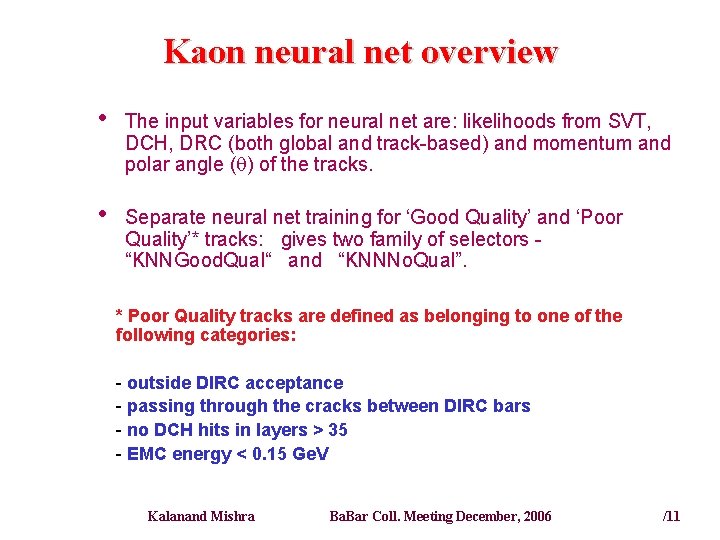
Kaon neural net overview • The input variables for neural net are: likelihoods from SVT, DCH, DRC (both global and track-based) and momentum and polar angle ( ) of the tracks. • Separate neural net training for ‘Good Quality’ and ‘Poor Quality’* tracks: gives two family of selectors “KNNGood. Qual“ and “KNNNo. Qual”. * Poor Quality tracks are defined as belonging to one of the following categories: - outside DIRC acceptance - passing through the cracks between DIRC bars - no DCH hits in layers > 35 - EMC energy < 0. 15 Ge. V Kalanand Mishra Ba. Bar Coll. Meeting December, 2006 /11
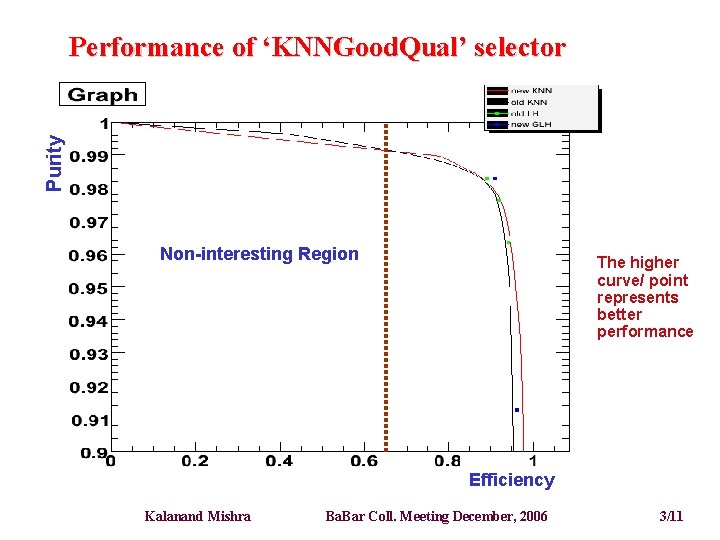
Purity Performance of ‘KNNGood. Qual’ selector Non-interesting Region The higher curve/ point represents better performance Efficiency Kalanand Mishra Ba. Bar Coll. Meeting December, 2006 3/11
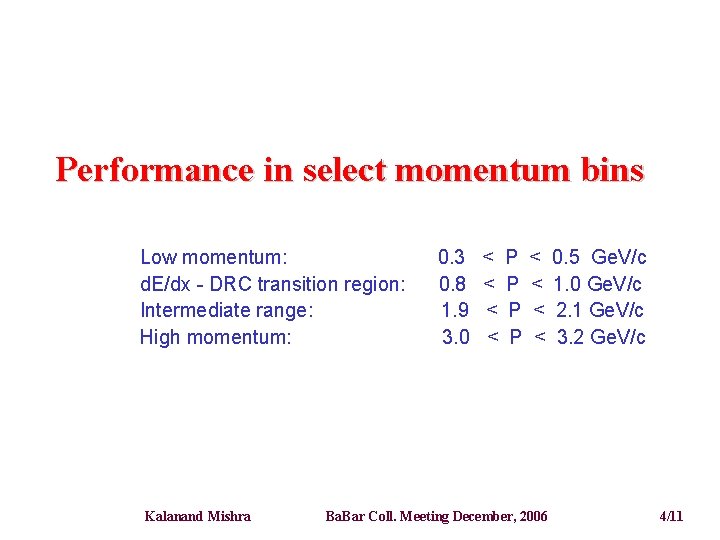
Performance in select momentum bins Low momentum: d. E/dx - DRC transition region: Intermediate range: High momentum: Kalanand Mishra 0. 3 0. 8 1. 9 3. 0 < < P P < < Ba. Bar Coll. Meeting December, 2006 0. 5 Ge. V/c 1. 0 Ge. V/c 2. 1 Ge. V/c 3. 2 Ge. V/c 4/11
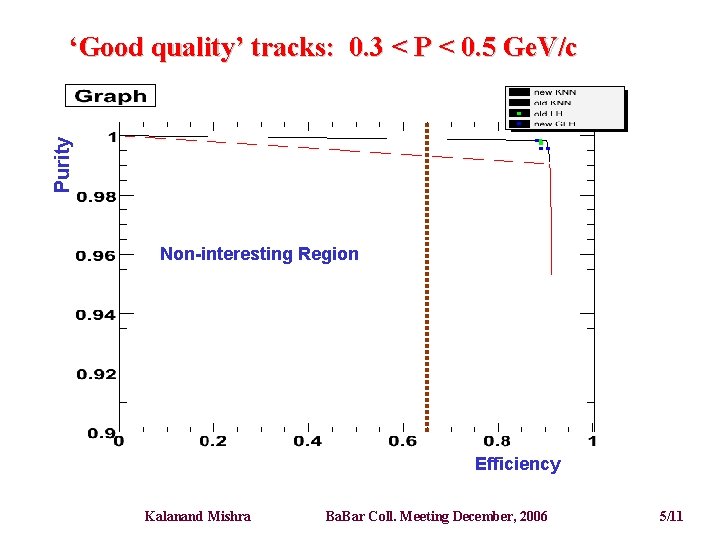
Purity ‘Good quality’ tracks: 0. 3 < P < 0. 5 Ge. V/c Non-interesting Region Efficiency Kalanand Mishra Ba. Bar Coll. Meeting December, 2006 5/11
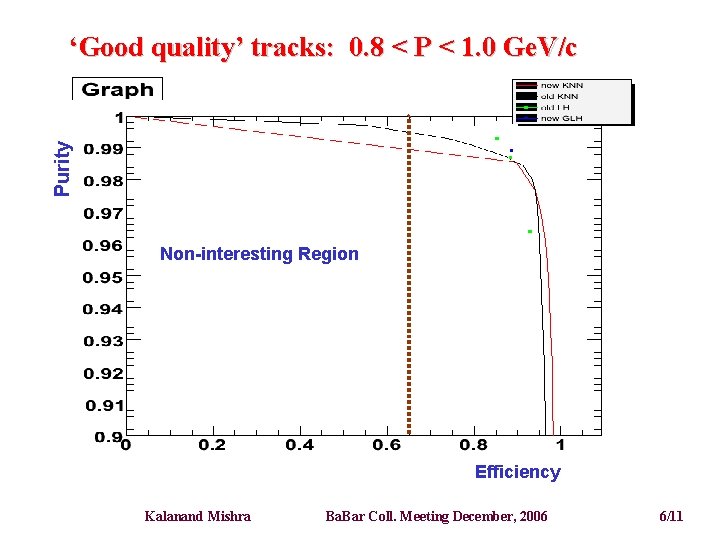
Purity ‘Good quality’ tracks: 0. 8 < P < 1. 0 Ge. V/c Non-interesting Region Efficiency Kalanand Mishra Ba. Bar Coll. Meeting December, 2006 6/11
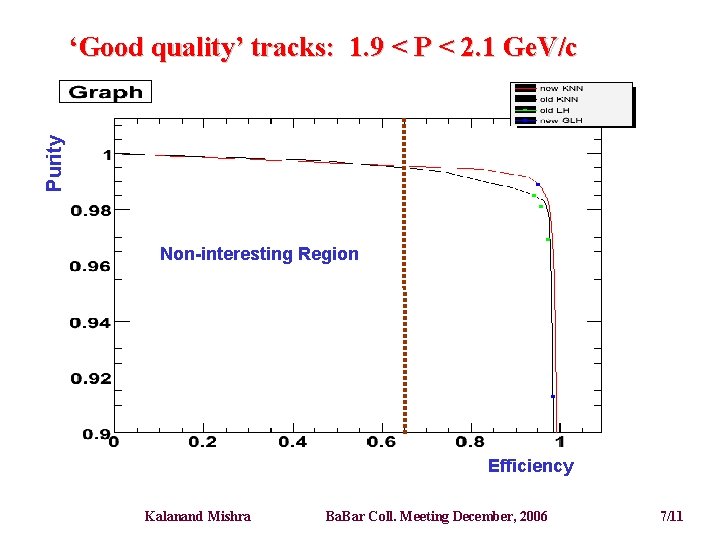
Purity ‘Good quality’ tracks: 1. 9 < P < 2. 1 Ge. V/c Non-interesting Region Efficiency Kalanand Mishra Ba. Bar Coll. Meeting December, 2006 7/11
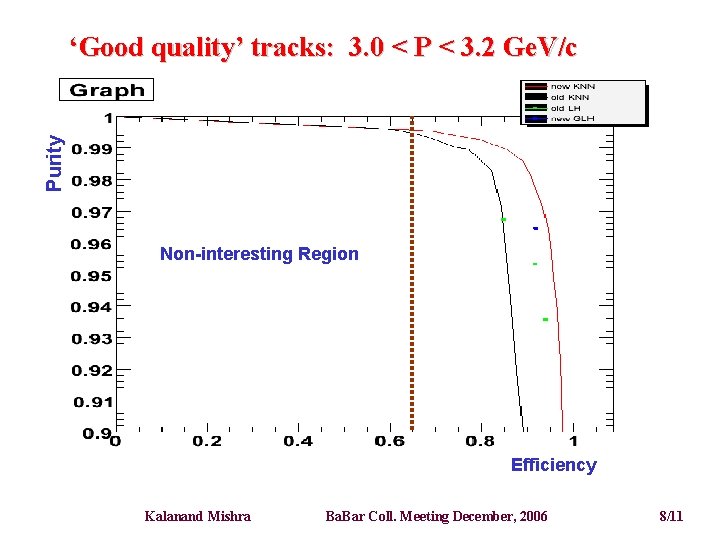
Purity ‘Good quality’ tracks: 3. 0 < P < 3. 2 Ge. V/c Non-interesting Region Efficiency Kalanand Mishra Ba. Bar Coll. Meeting December, 2006 8/11
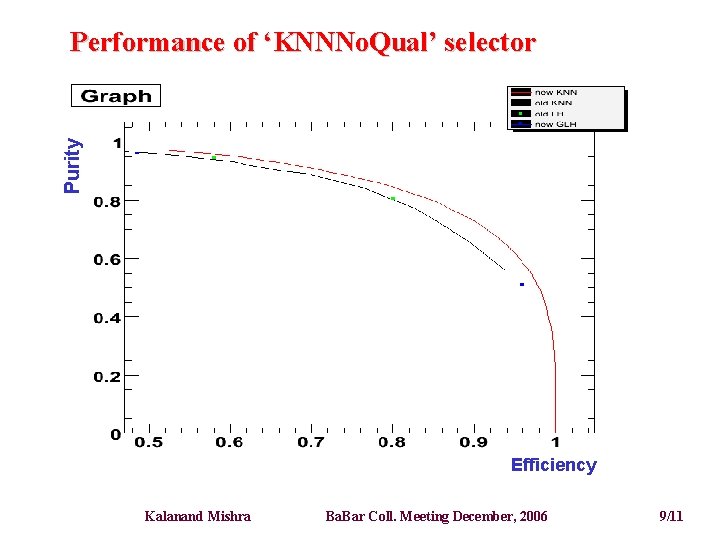
Purity Performance of ‘KNNNo. Qual’ selector Efficiency Kalanand Mishra Ba. Bar Coll. Meeting December, 2006 9/11
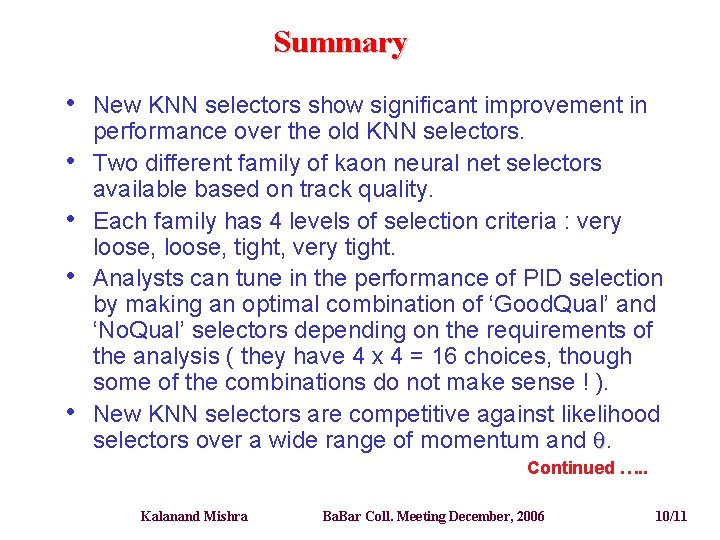
Summary • • • New KNN selectors show significant improvement in performance over the old KNN selectors. Two different family of kaon neural net selectors available based on track quality. Each family has 4 levels of selection criteria : very loose, tight, very tight. Analysts can tune in the performance of PID selection by making an optimal combination of ‘Good. Qual’ and ‘No. Qual’ selectors depending on the requirements of the analysis ( they have 4 x 4 = 16 choices, though some of the combinations do not make sense ! ). New KNN selectors are competitive against likelihood selectors over a wide range of momentum and . Continued …. . Kalanand Mishra Ba. Bar Coll. Meeting December, 2006 10/11
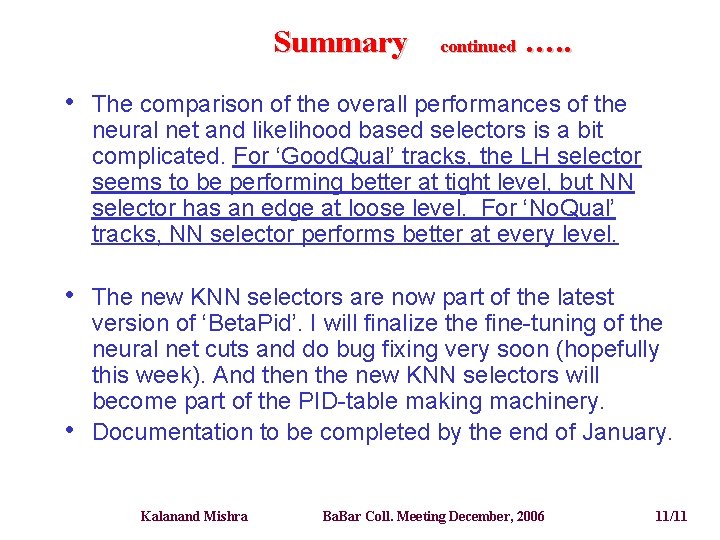
Summary continued …. . • The comparison of the overall performances of the neural net and likelihood based selectors is a bit complicated. For ‘Good. Qual’ tracks, the LH selector seems to be performing better at tight level, but NN selector has an edge at loose level. For ‘No. Qual’ tracks, NN selector performs better at every level. • The new KNN selectors are now part of the latest version of ‘Beta. Pid’. I will finalize the fine-tuning of the neural net cuts and do bug fixing very soon (hopefully this week). And then the new KNN selectors will become part of the PID-table making machinery. Documentation to be completed by the end of January. • Kalanand Mishra Ba. Bar Coll. Meeting December, 2006 11/11