Text Analytics Workshop Development Tom Reamy Chief Knowledge
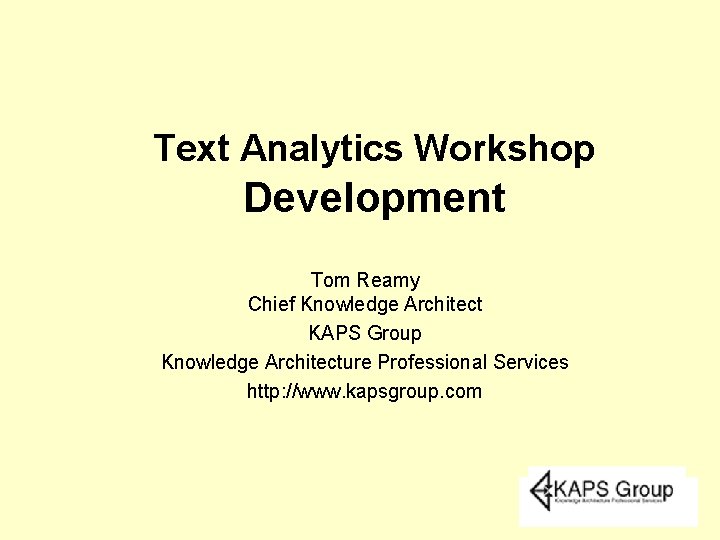
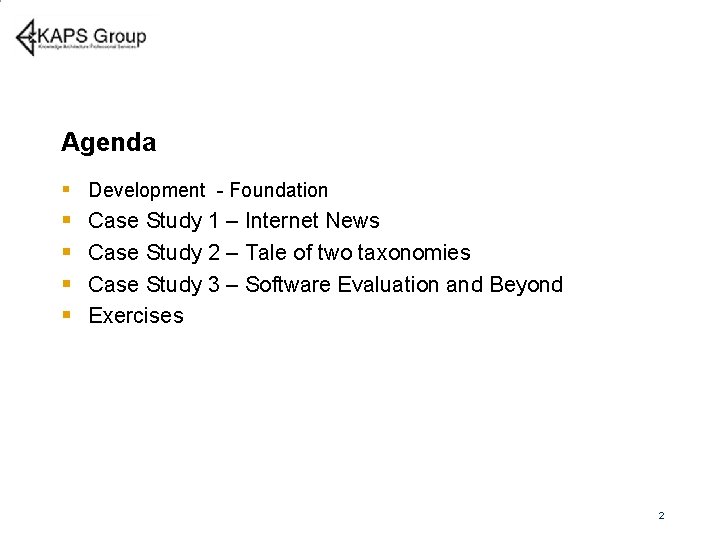
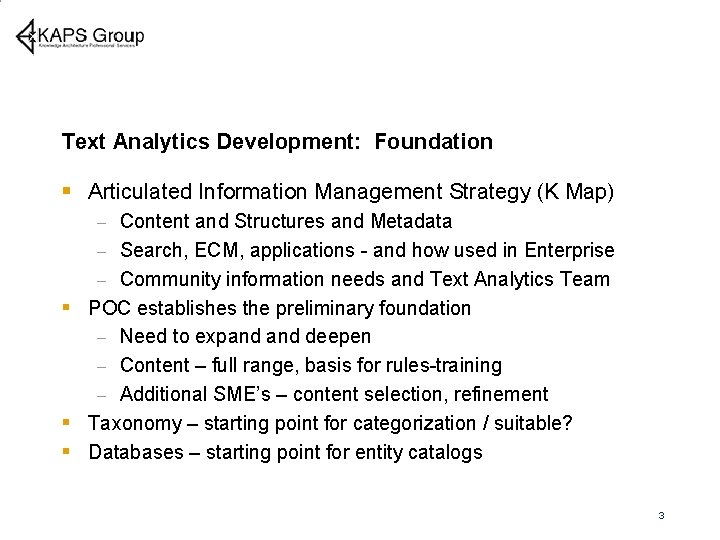
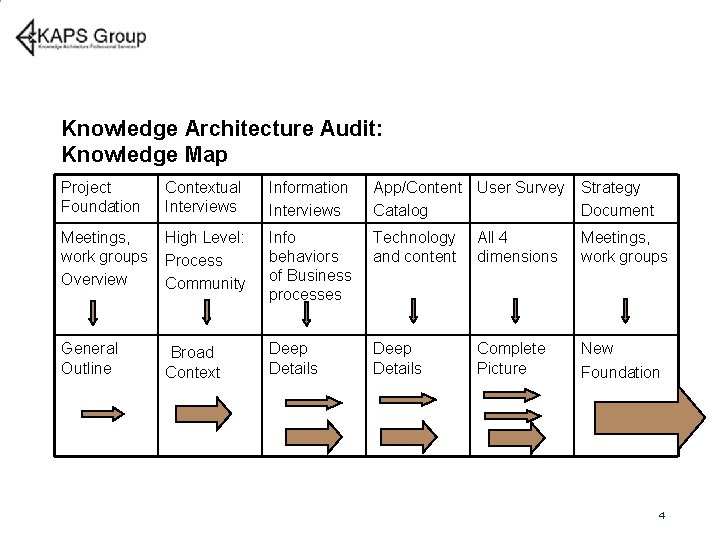
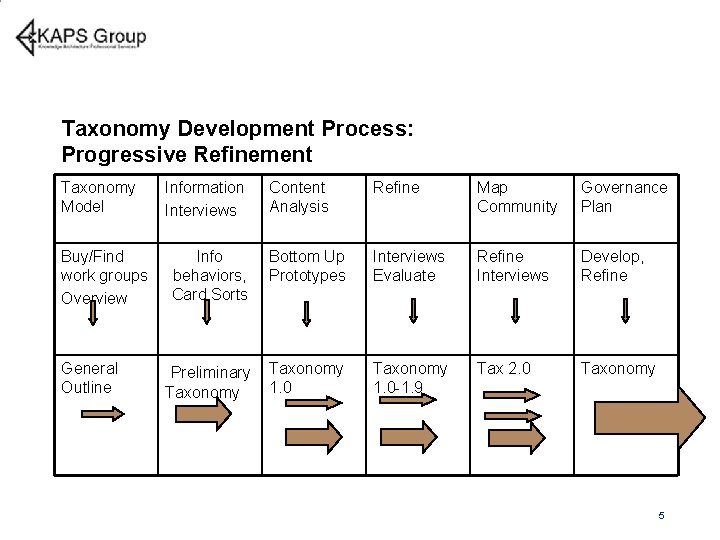
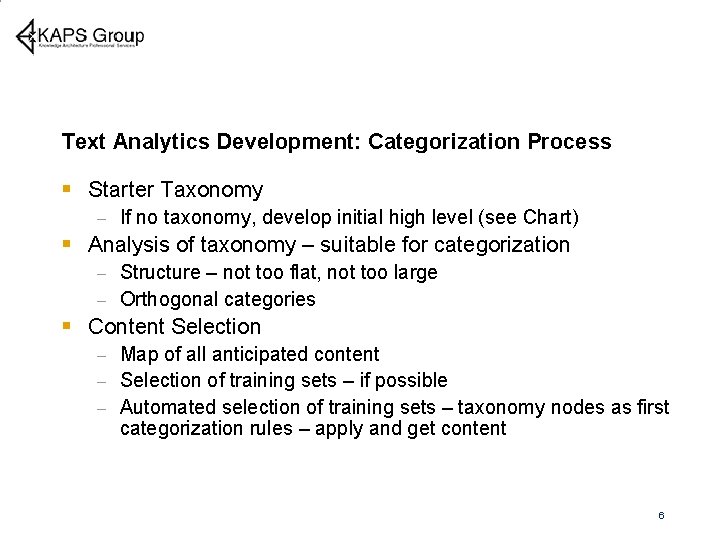
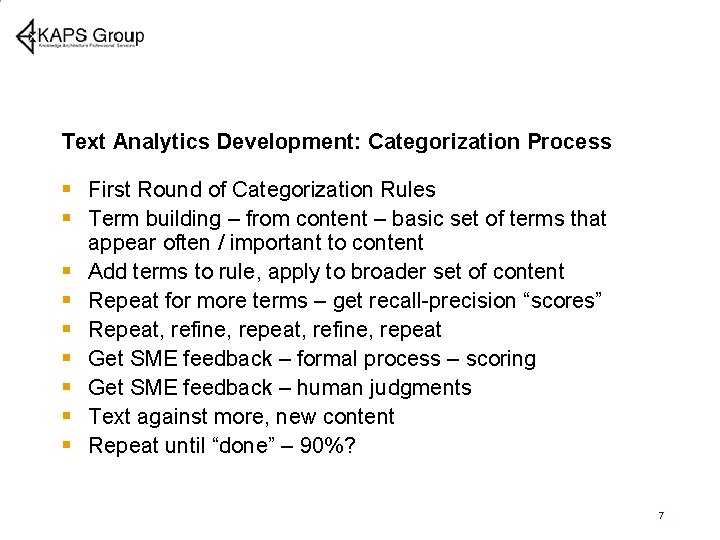
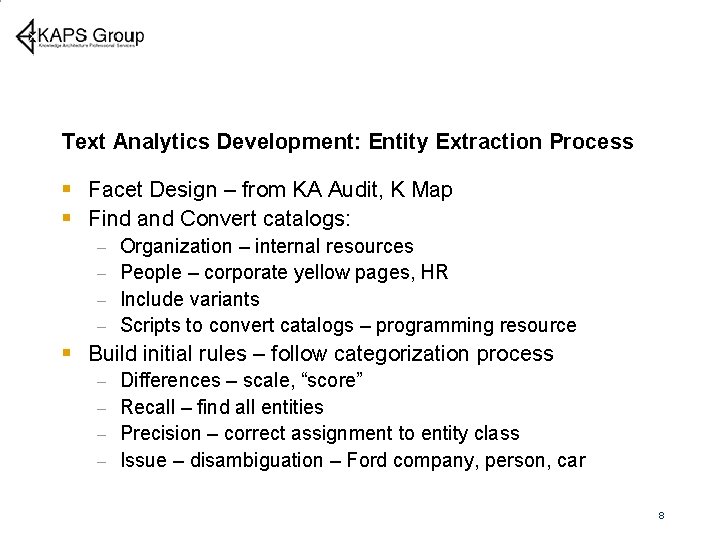
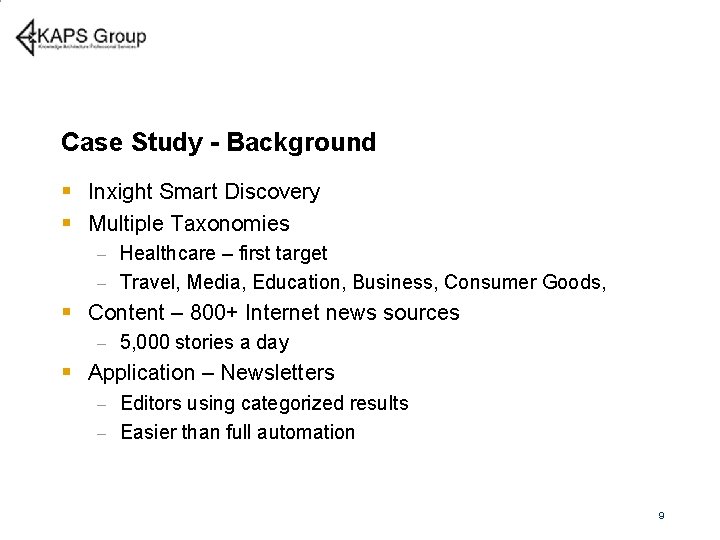
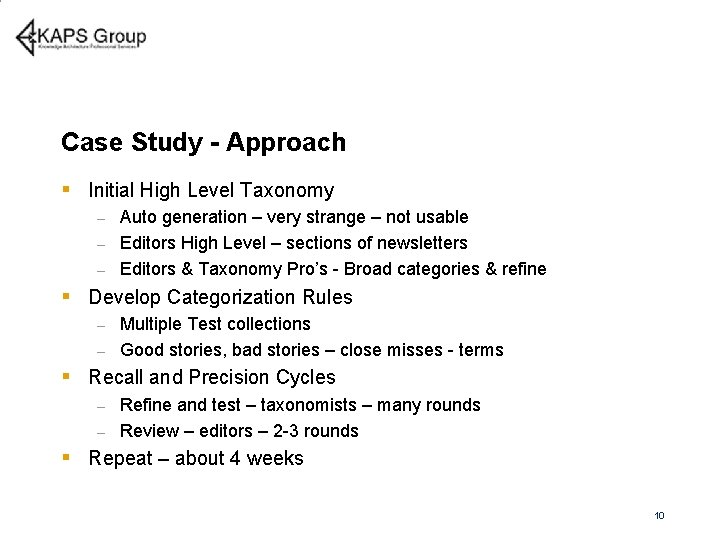
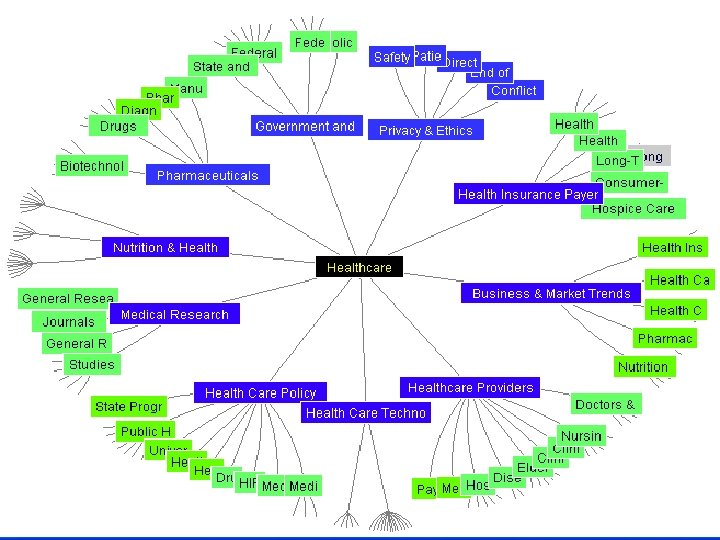
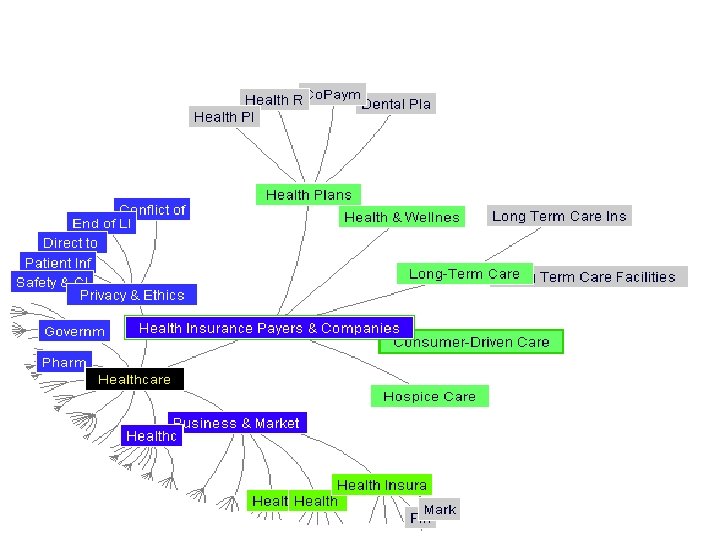
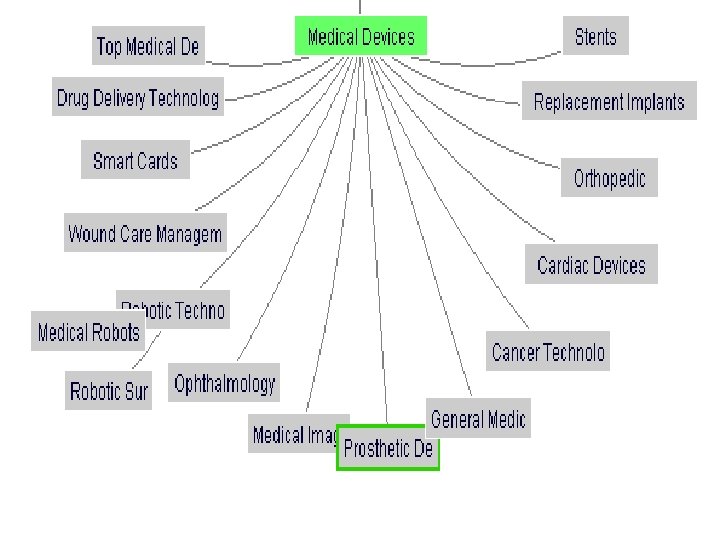
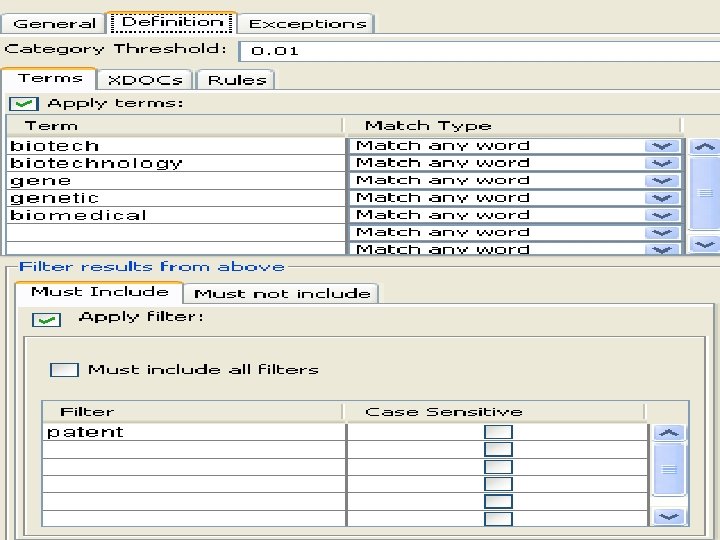
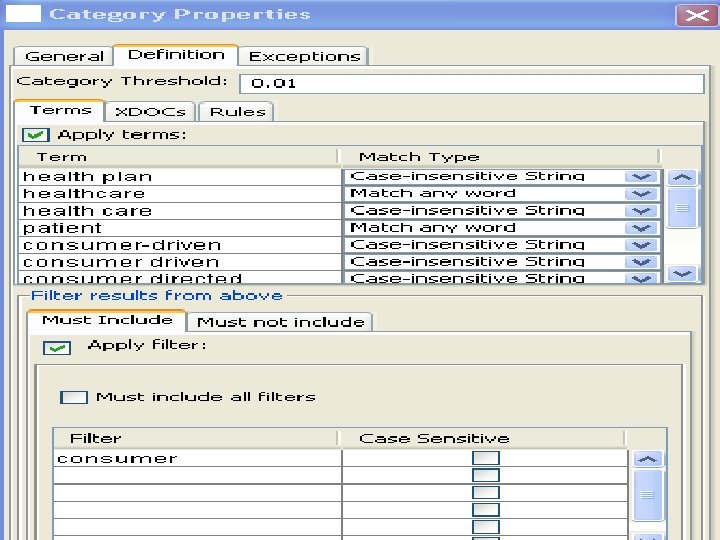
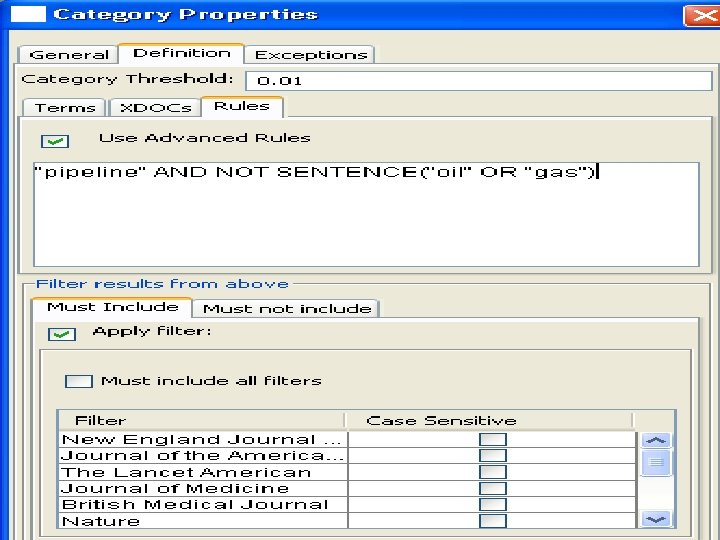
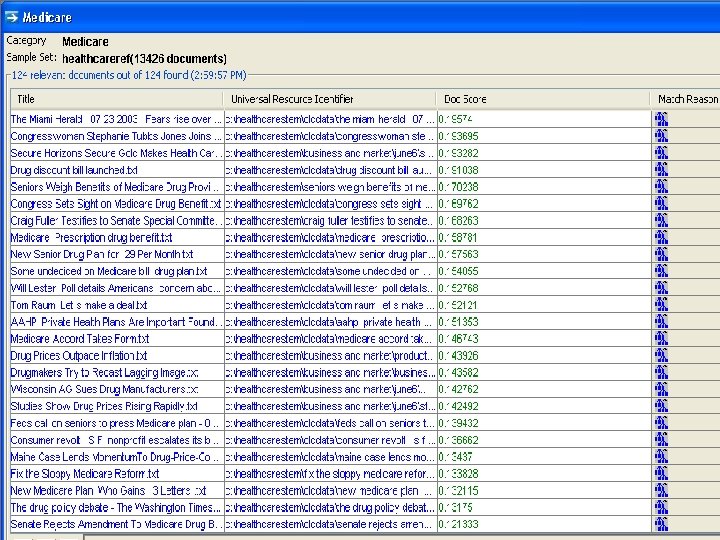
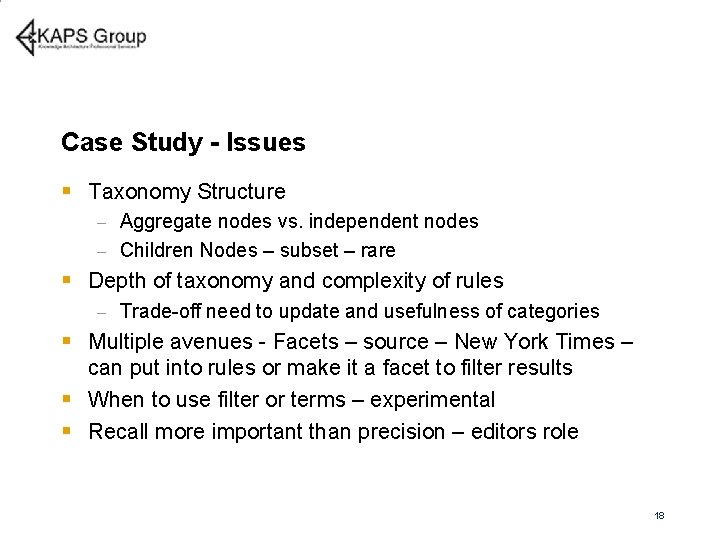
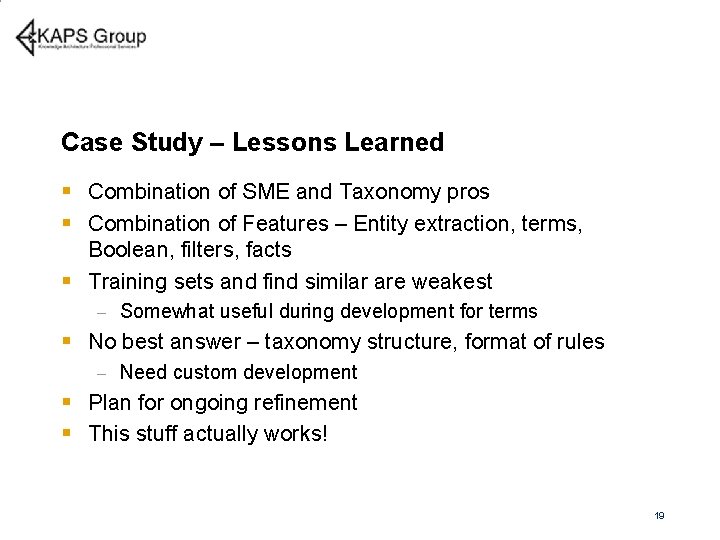
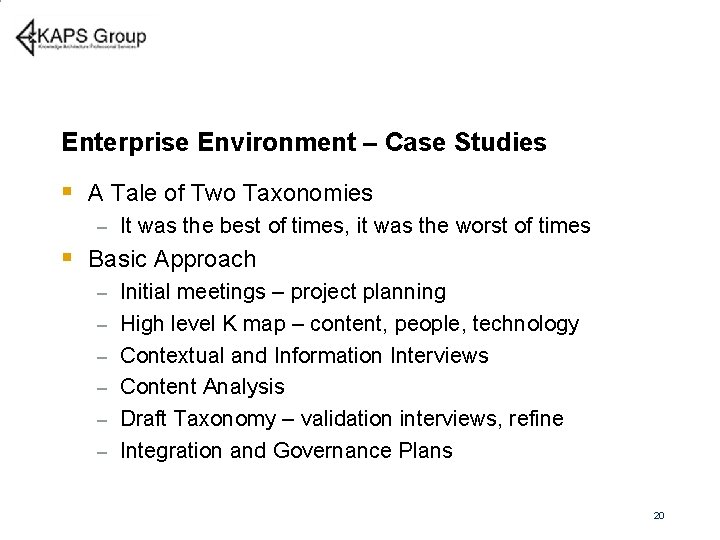
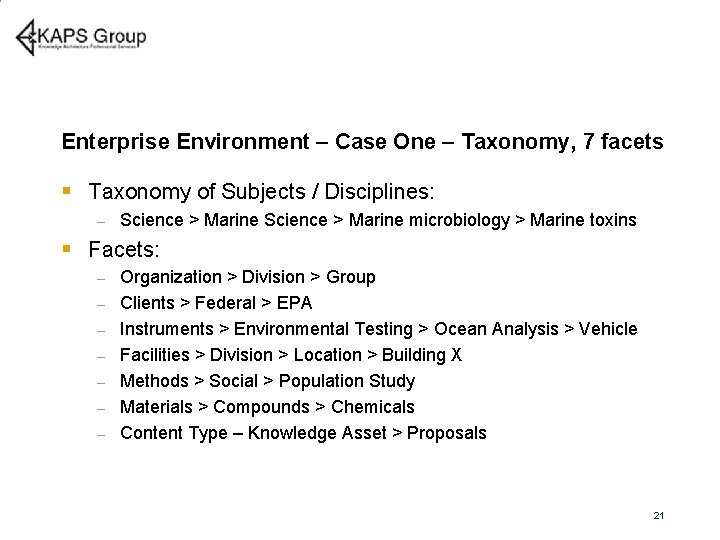
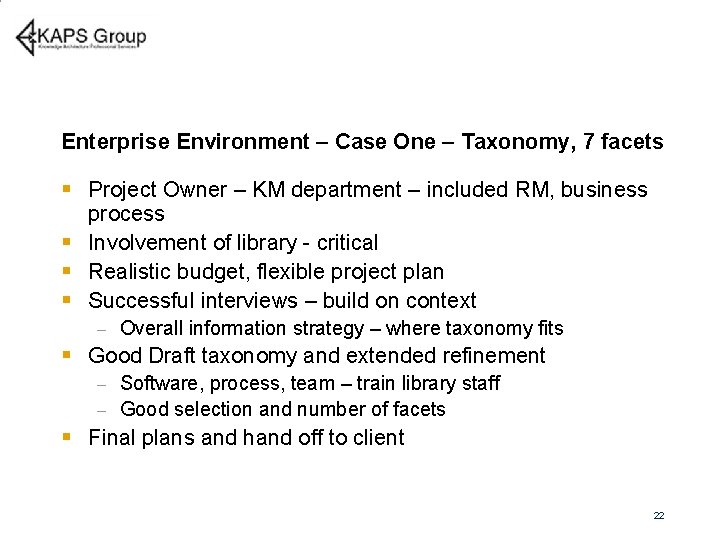
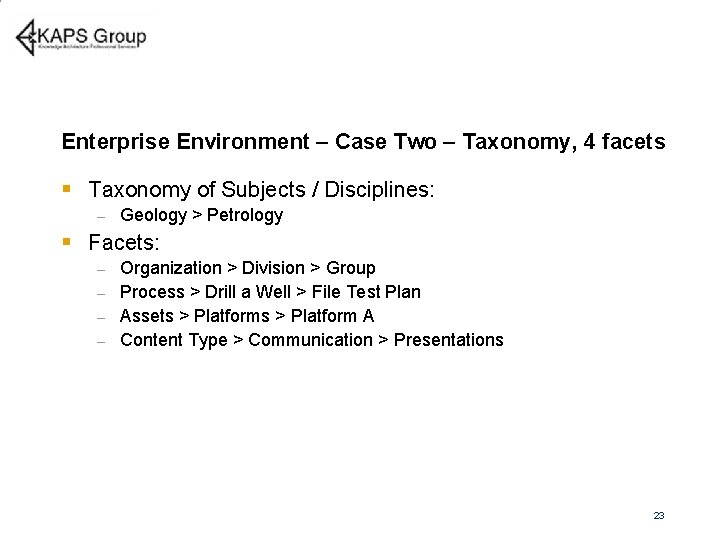
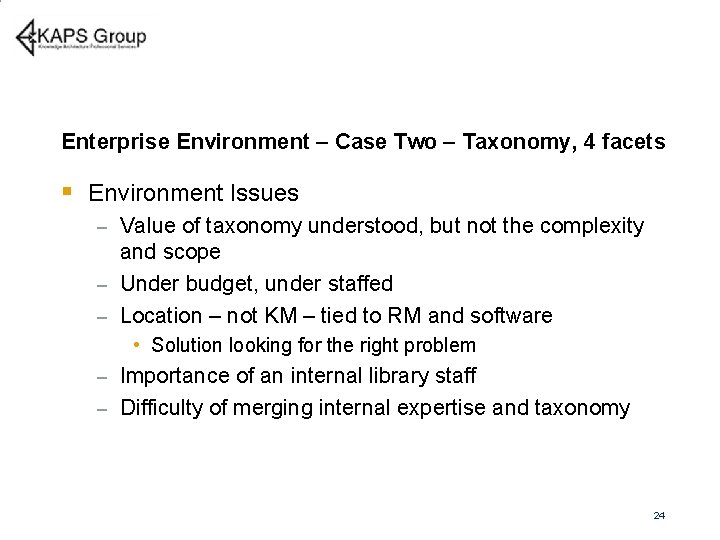
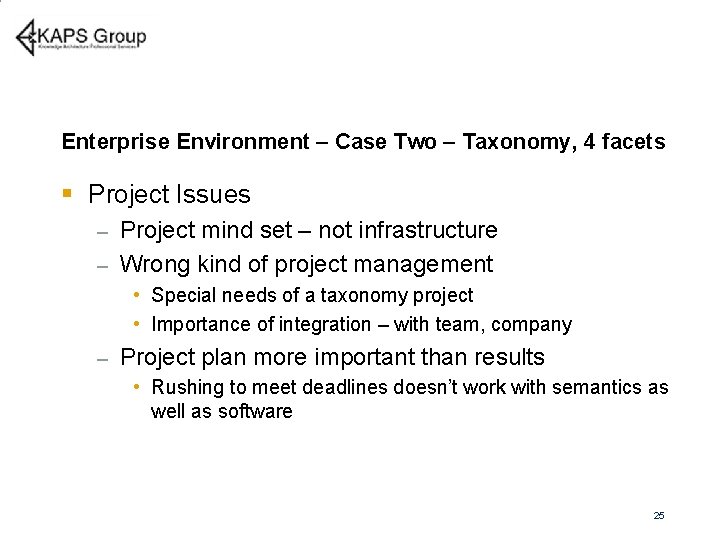
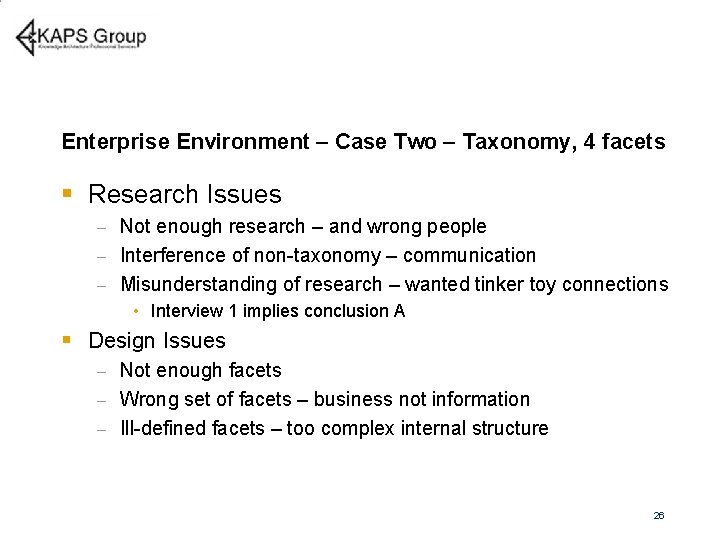
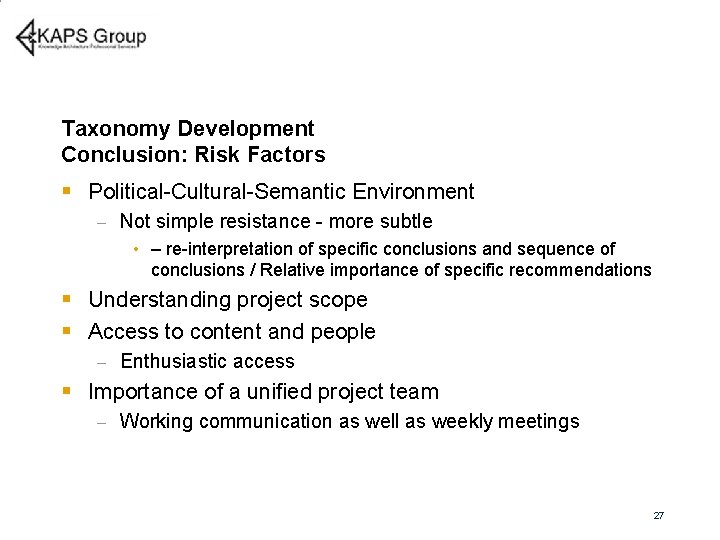
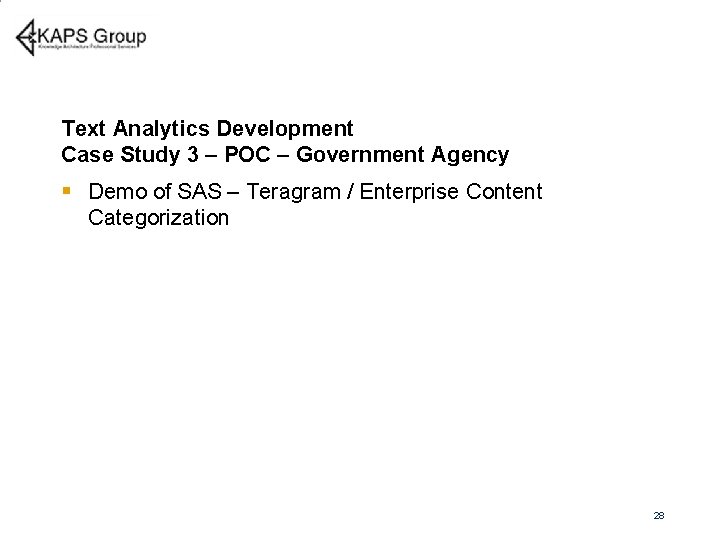
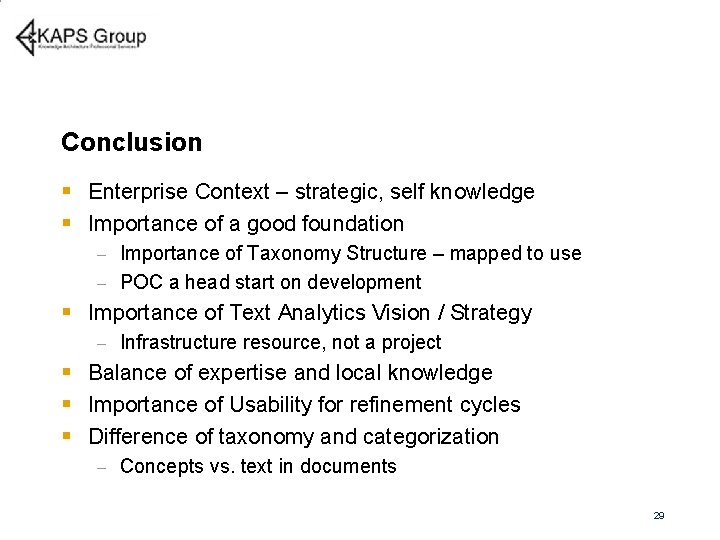
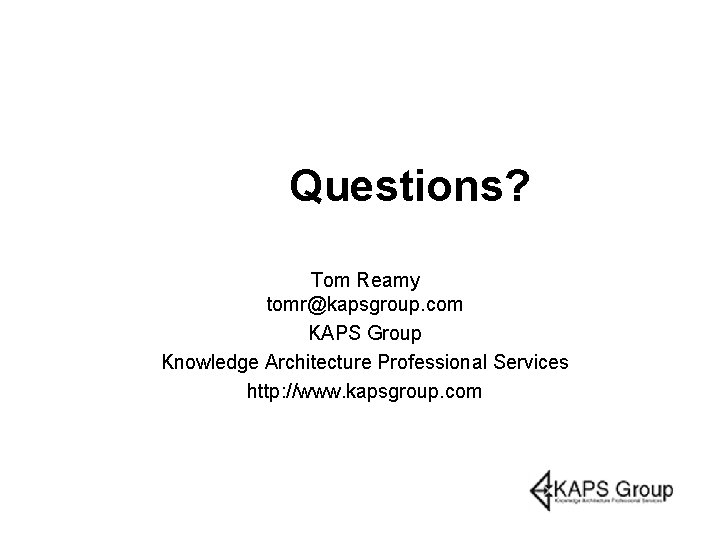
- Slides: 30
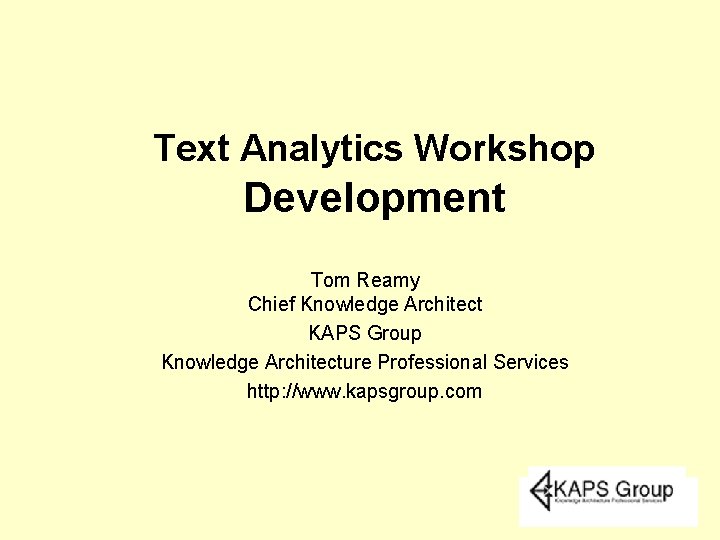
Text Analytics Workshop Development Tom Reamy Chief Knowledge Architect KAPS Group Knowledge Architecture Professional Services http: //www. kapsgroup. com
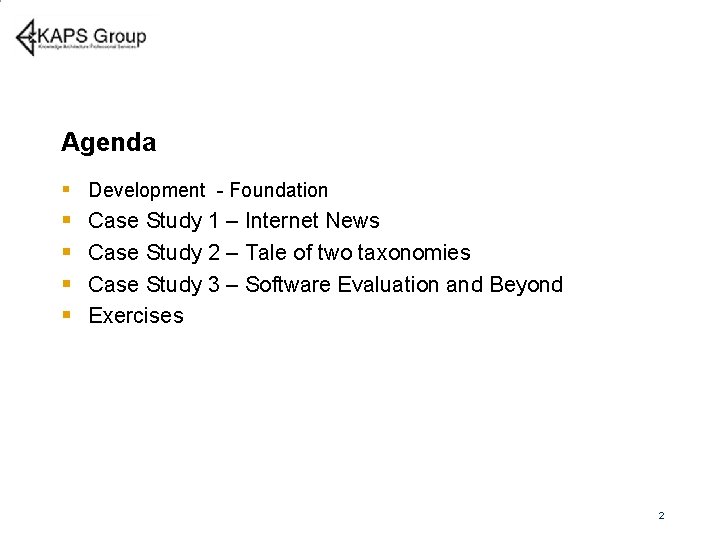
Agenda § Development - Foundation § Case Study 1 – Internet News § Case Study 2 – Tale of two taxonomies § Case Study 3 – Software Evaluation and Beyond § Exercises 2
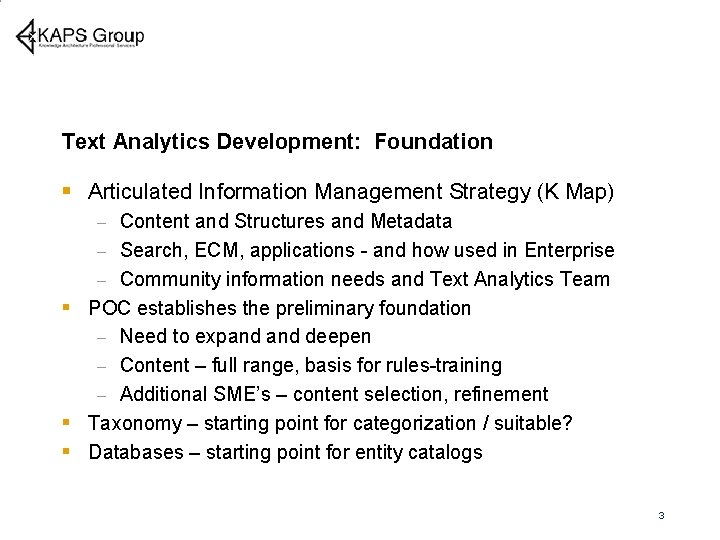
Text Analytics Development: Foundation § Articulated Information Management Strategy (K Map) Content and Structures and Metadata – Search, ECM, applications - and how used in Enterprise – Community information needs and Text Analytics Team § POC establishes the preliminary foundation – Need to expand deepen – Content – full range, basis for rules-training – Additional SME’s – content selection, refinement § Taxonomy – starting point for categorization / suitable? § Databases – starting point for entity catalogs – 3
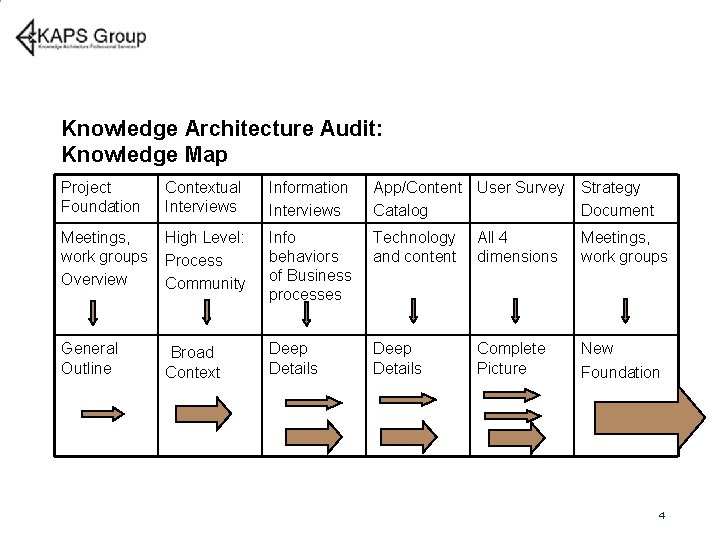
Knowledge Architecture Audit: Knowledge Map Project Foundation Contextual Interviews Information Interviews App/Content User Survey Catalog Strategy Document Meetings, work groups Overview High Level: Process Community Info behaviors of Business processes Technology and content All 4 dimensions Meetings, work groups General Outline Broad Context Deep Details Complete Picture New Foundation 4
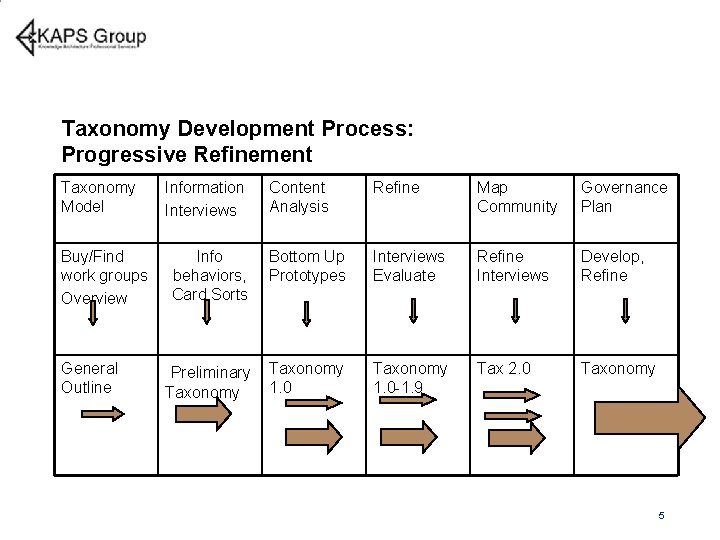
Taxonomy Development Process: Progressive Refinement Taxonomy Model Buy/Find work groups Overview General Outline Information Interviews Content Analysis Refine Map Community Governance Plan Info behaviors, Card Sorts Bottom Up Prototypes Interviews Evaluate Refine Interviews Develop, Refine Preliminary Taxonomy 1. 0 -1. 9 Tax 2. 0 Taxonomy 5
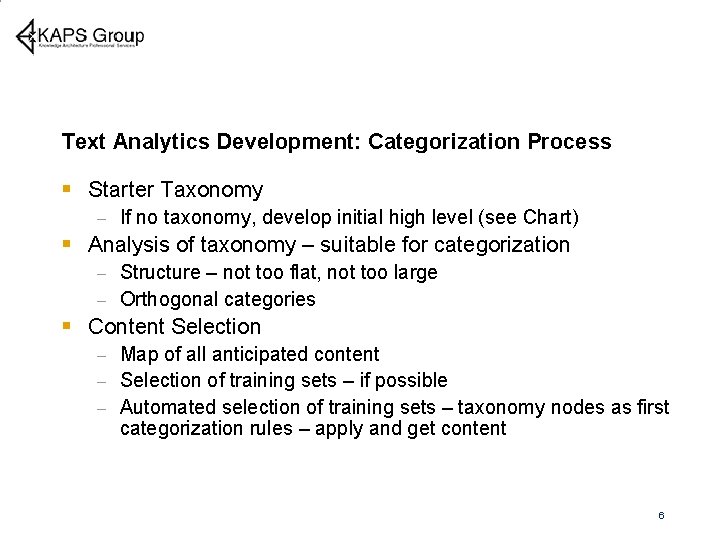
Text Analytics Development: Categorization Process § Starter Taxonomy – If no taxonomy, develop initial high level (see Chart) § Analysis of taxonomy – suitable for categorization Structure – not too flat, not too large – Orthogonal categories – § Content Selection Map of all anticipated content – Selection of training sets – if possible – Automated selection of training sets – taxonomy nodes as first categorization rules – apply and get content – 6
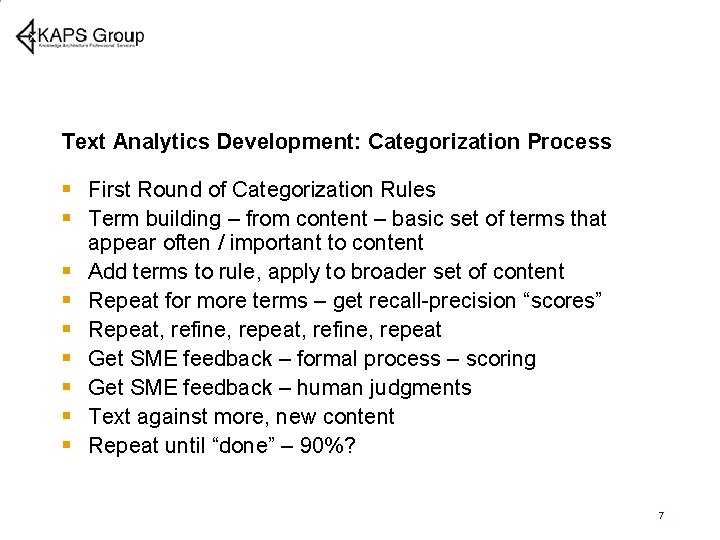
Text Analytics Development: Categorization Process § First Round of Categorization Rules § Term building – from content – basic set of terms that § § § § appear often / important to content Add terms to rule, apply to broader set of content Repeat for more terms – get recall-precision “scores” Repeat, refine, repeat Get SME feedback – formal process – scoring Get SME feedback – human judgments Text against more, new content Repeat until “done” – 90%? 7
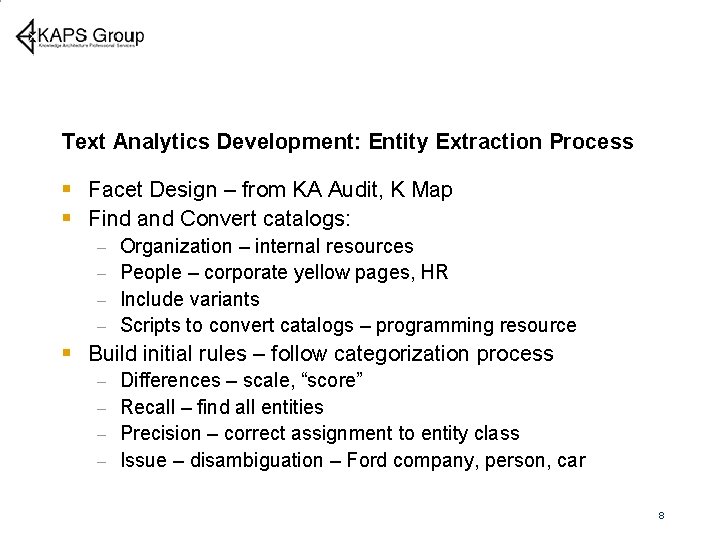
Text Analytics Development: Entity Extraction Process § Facet Design – from KA Audit, K Map § Find and Convert catalogs: Organization – internal resources – People – corporate yellow pages, HR – Include variants – Scripts to convert catalogs – programming resource – § Build initial rules – follow categorization process Differences – scale, “score” – Recall – find all entities – Precision – correct assignment to entity class – Issue – disambiguation – Ford company, person, car – 8
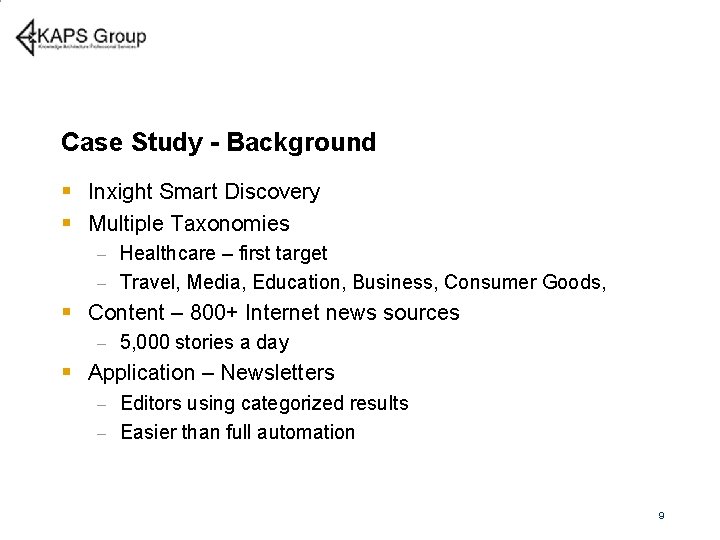
Case Study - Background § Inxight Smart Discovery § Multiple Taxonomies Healthcare – first target – Travel, Media, Education, Business, Consumer Goods, – § Content – 800+ Internet news sources – 5, 000 stories a day § Application – Newsletters Editors using categorized results – Easier than full automation – 9
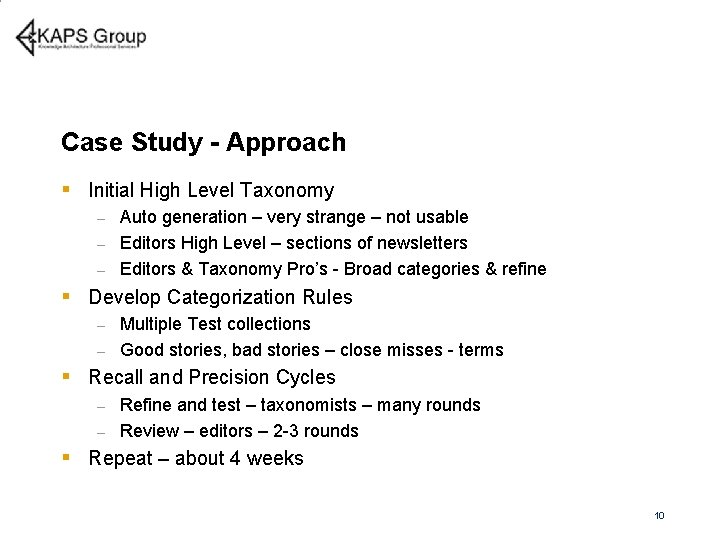
Case Study - Approach § Initial High Level Taxonomy Auto generation – very strange – not usable – Editors High Level – sections of newsletters – Editors & Taxonomy Pro’s - Broad categories & refine – § Develop Categorization Rules Multiple Test collections – Good stories, bad stories – close misses - terms – § Recall and Precision Cycles Refine and test – taxonomists – many rounds – Review – editors – 2 -3 rounds – § Repeat – about 4 weeks 10
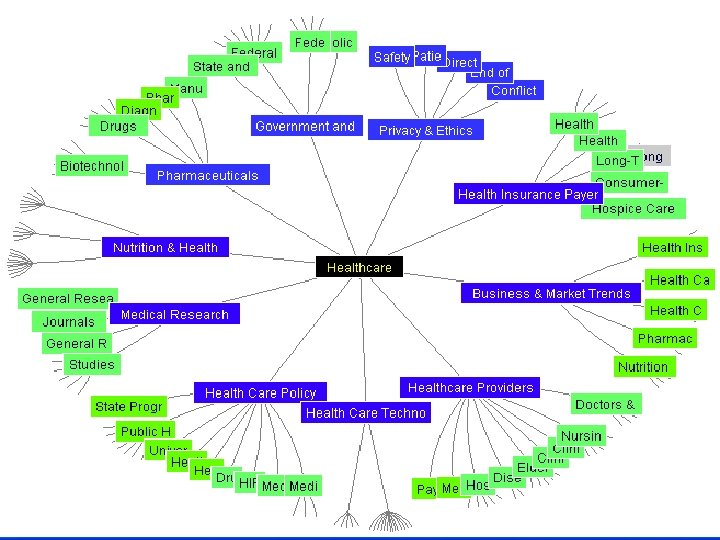
11
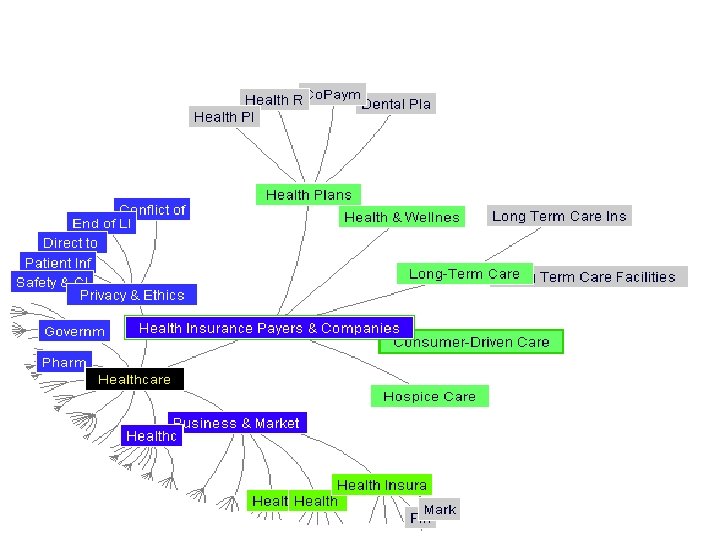
12
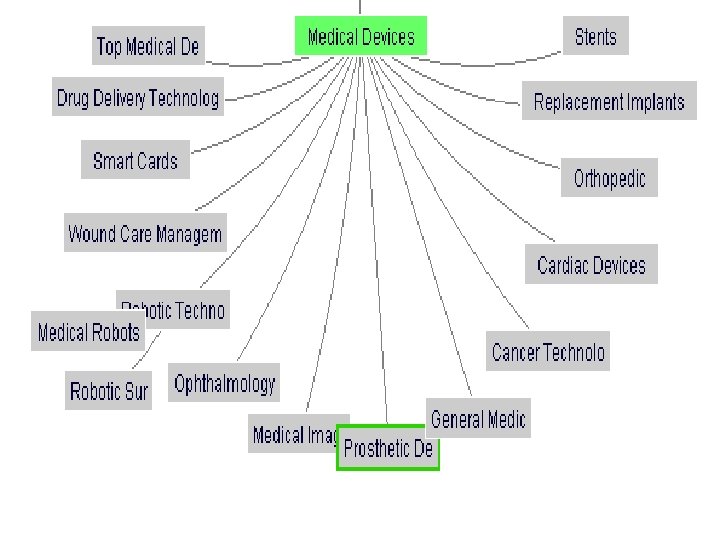
13
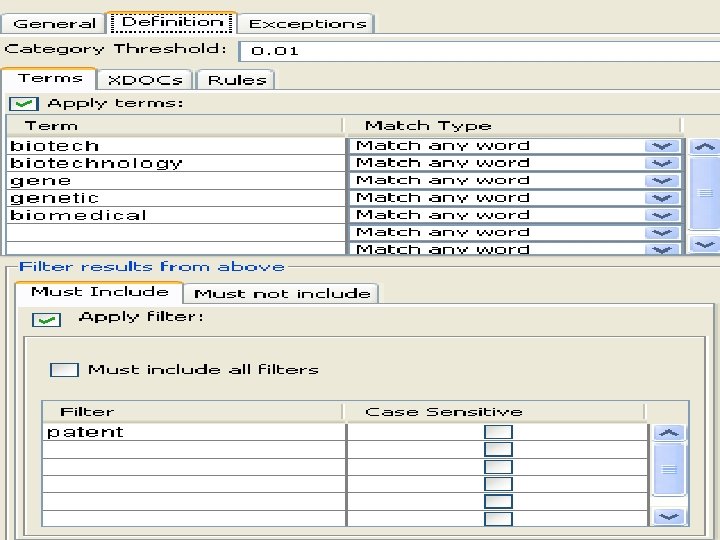
14
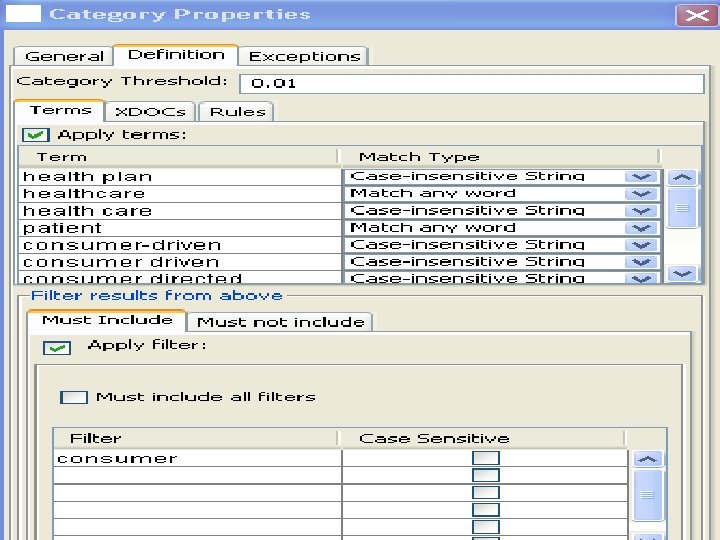
15
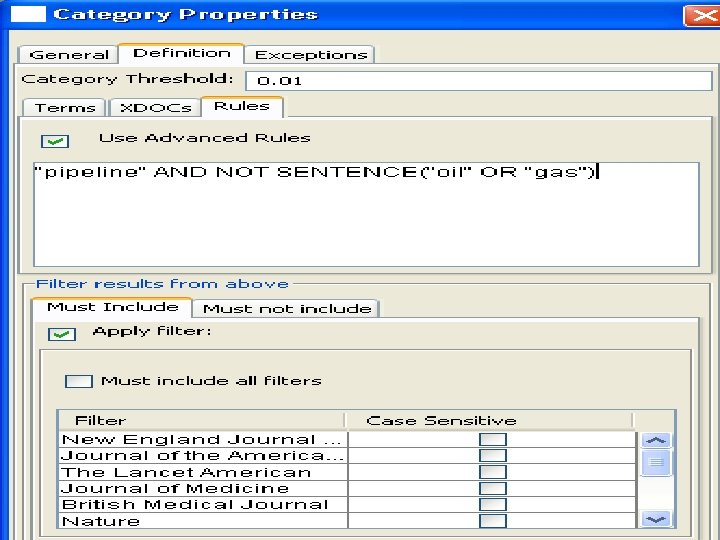
16
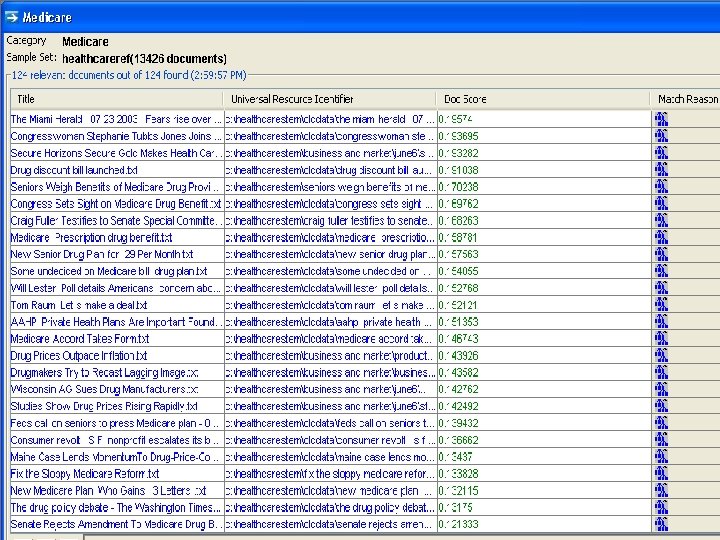
17
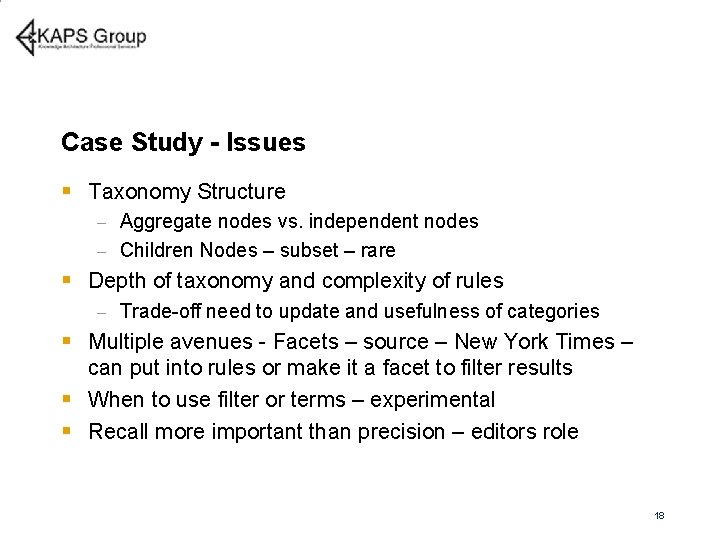
Case Study - Issues § Taxonomy Structure Aggregate nodes vs. independent nodes – Children Nodes – subset – rare – § Depth of taxonomy and complexity of rules – Trade-off need to update and usefulness of categories § Multiple avenues - Facets – source – New York Times – can put into rules or make it a facet to filter results § When to use filter or terms – experimental § Recall more important than precision – editors role 18
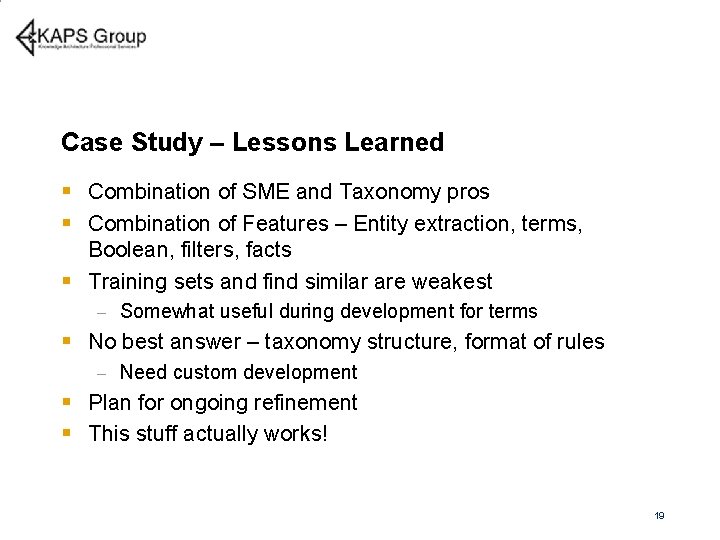
Case Study – Lessons Learned § Combination of SME and Taxonomy pros § Combination of Features – Entity extraction, terms, Boolean, filters, facts § Training sets and find similar are weakest – Somewhat useful during development for terms § No best answer – taxonomy structure, format of rules – Need custom development § Plan for ongoing refinement § This stuff actually works! 19
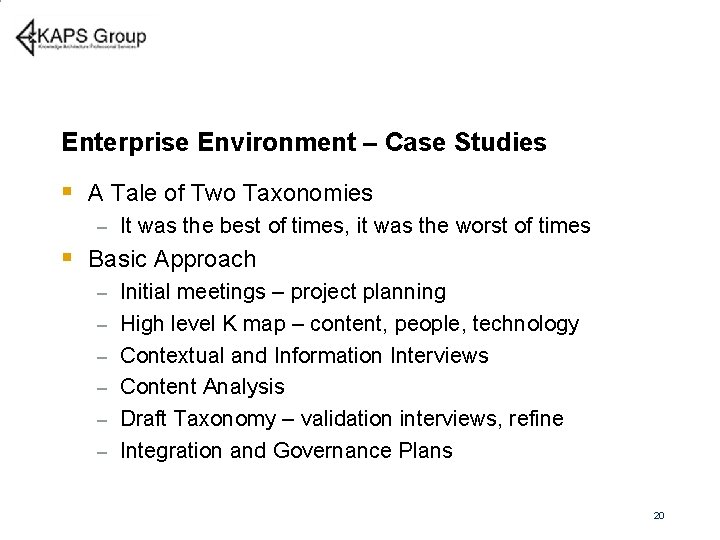
Enterprise Environment – Case Studies § A Tale of Two Taxonomies – It was the best of times, it was the worst of times § Basic Approach – – – Initial meetings – project planning High level K map – content, people, technology Contextual and Information Interviews Content Analysis Draft Taxonomy – validation interviews, refine Integration and Governance Plans 20
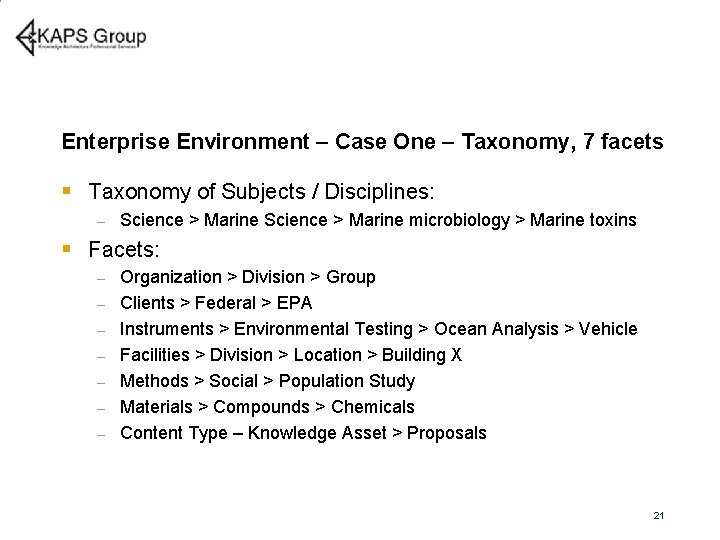
Enterprise Environment – Case One – Taxonomy, 7 facets § Taxonomy of Subjects / Disciplines: – Science > Marine microbiology > Marine toxins § Facets: – – – – Organization > Division > Group Clients > Federal > EPA Instruments > Environmental Testing > Ocean Analysis > Vehicle Facilities > Division > Location > Building X Methods > Social > Population Study Materials > Compounds > Chemicals Content Type – Knowledge Asset > Proposals 21
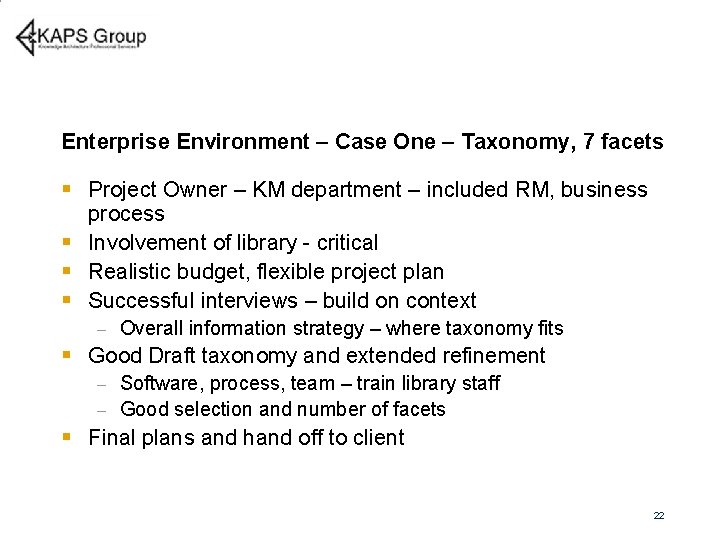
Enterprise Environment – Case One – Taxonomy, 7 facets § Project Owner – KM department – included RM, business process § Involvement of library - critical § Realistic budget, flexible project plan § Successful interviews – build on context – Overall information strategy – where taxonomy fits § Good Draft taxonomy and extended refinement Software, process, team – train library staff – Good selection and number of facets – § Final plans and hand off to client 22
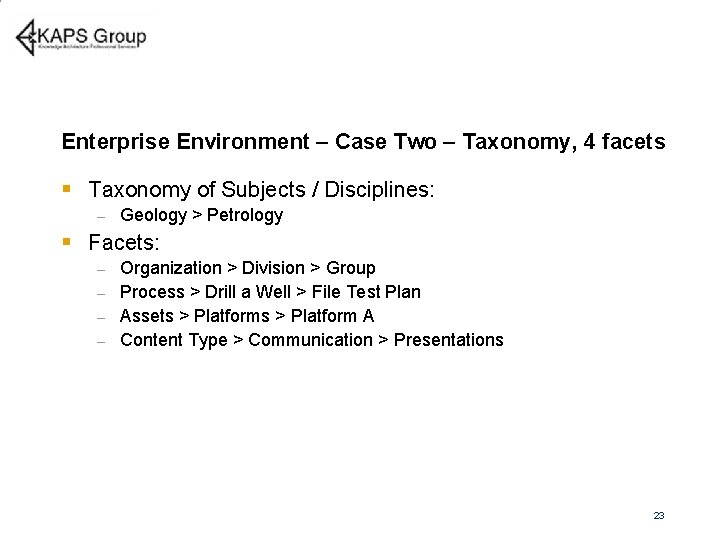
Enterprise Environment – Case Two – Taxonomy, 4 facets § Taxonomy of Subjects / Disciplines: – Geology > Petrology § Facets: Organization > Division > Group – Process > Drill a Well > File Test Plan – Assets > Platform A – Content Type > Communication > Presentations – 23
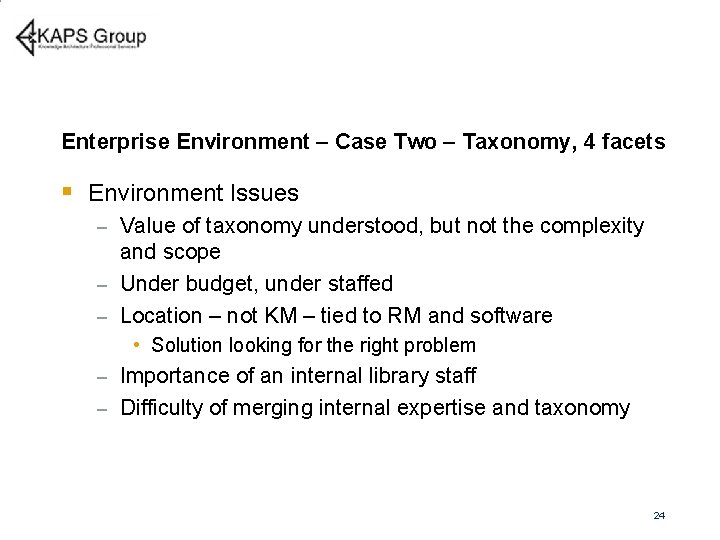
Enterprise Environment – Case Two – Taxonomy, 4 facets § Environment Issues Value of taxonomy understood, but not the complexity and scope – Under budget, under staffed – Location – not KM – tied to RM and software – • Solution looking for the right problem Importance of an internal library staff – Difficulty of merging internal expertise and taxonomy – 24
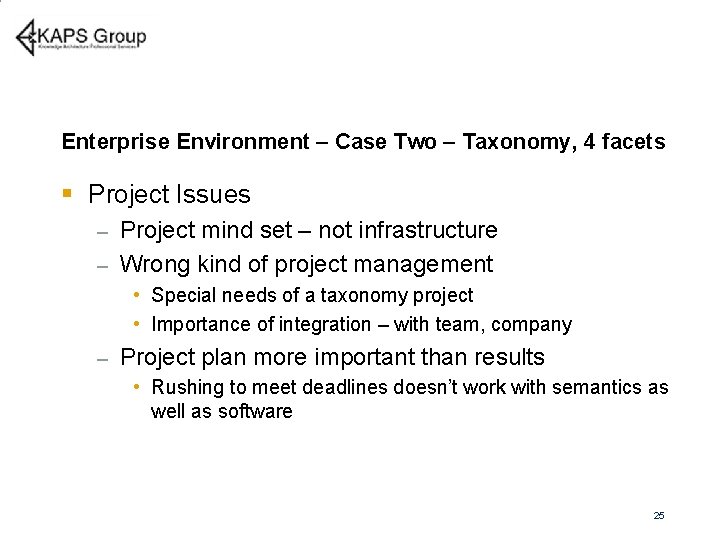
Enterprise Environment – Case Two – Taxonomy, 4 facets § Project Issues Project mind set – not infrastructure – Wrong kind of project management – • Special needs of a taxonomy project • Importance of integration – with team, company – Project plan more important than results • Rushing to meet deadlines doesn’t work with semantics as well as software 25
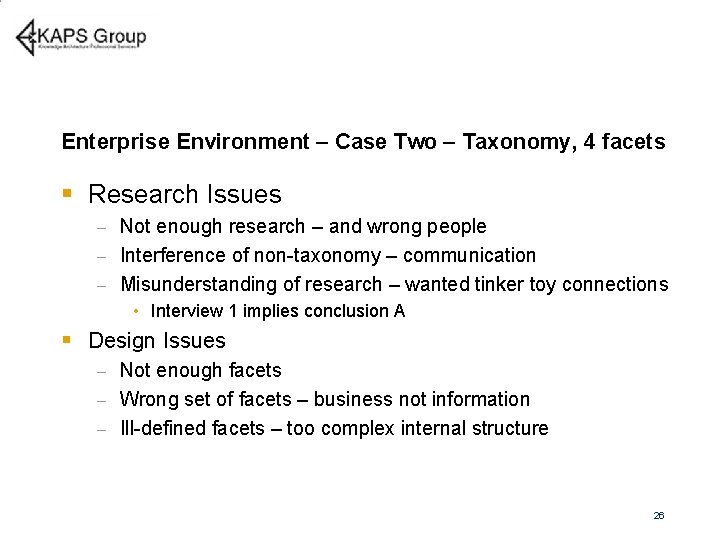
Enterprise Environment – Case Two – Taxonomy, 4 facets § Research Issues Not enough research – and wrong people – Interference of non-taxonomy – communication – Misunderstanding of research – wanted tinker toy connections – • Interview 1 implies conclusion A § Design Issues Not enough facets – Wrong set of facets – business not information – Ill-defined facets – too complex internal structure – 26
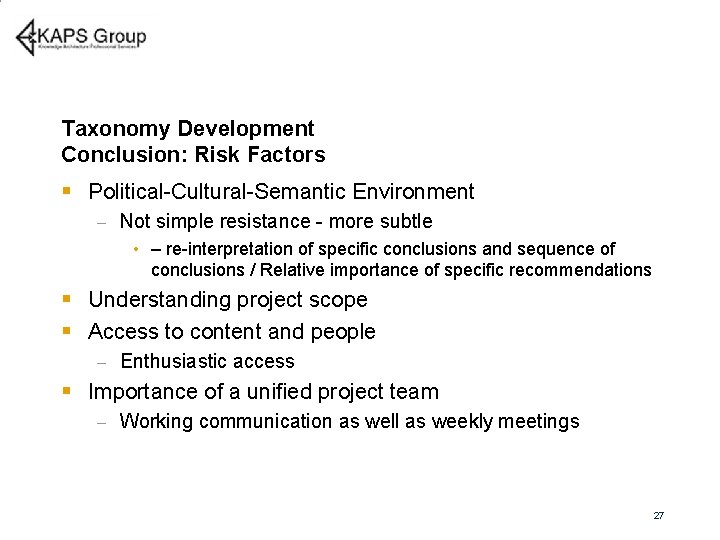
Taxonomy Development Conclusion: Risk Factors § Political-Cultural-Semantic Environment – Not simple resistance - more subtle • – re-interpretation of specific conclusions and sequence of conclusions / Relative importance of specific recommendations § Understanding project scope § Access to content and people – Enthusiastic access § Importance of a unified project team – Working communication as well as weekly meetings 27
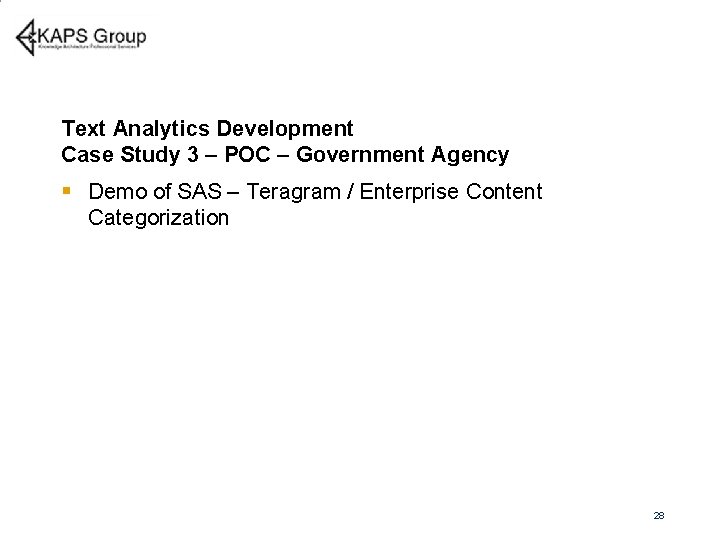
Text Analytics Development Case Study 3 – POC – Government Agency § Demo of SAS – Teragram / Enterprise Content Categorization 28
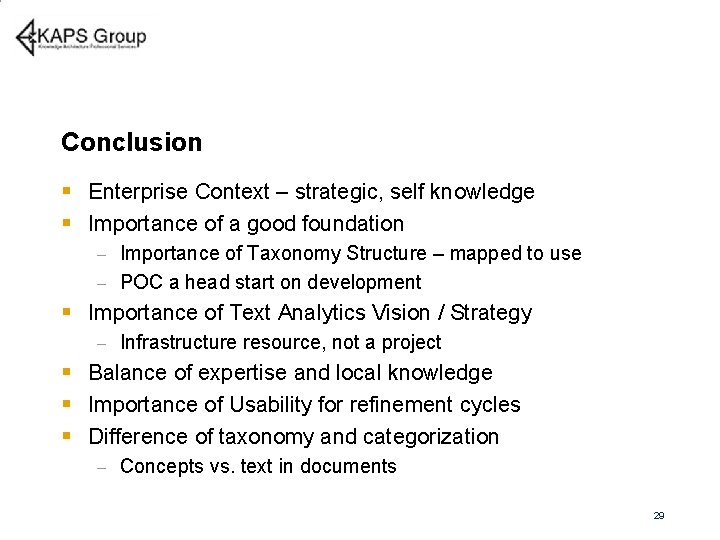
Conclusion § Enterprise Context – strategic, self knowledge § Importance of a good foundation Importance of Taxonomy Structure – mapped to use – POC a head start on development – § Importance of Text Analytics Vision / Strategy – Infrastructure resource, not a project § Balance of expertise and local knowledge § Importance of Usability for refinement cycles § Difference of taxonomy and categorization – Concepts vs. text in documents 29
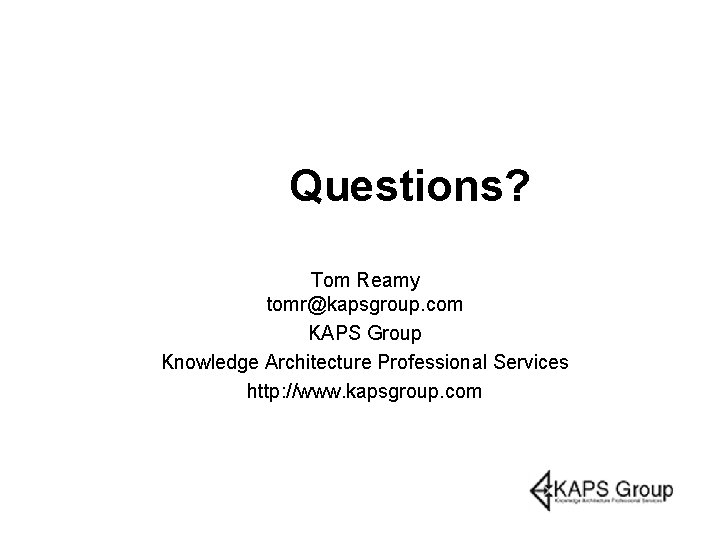
Questions? Tom Reamy tomr@kapsgroup. com KAPS Group Knowledge Architecture Professional Services http: //www. kapsgroup. com
Lesson 3 commander in chief and chief diplomat
Making connections
Text analytics and text mining
Text analytics and text mining
Teramond
Chief knowledge officer responsibilities
Icef toronto
Go 910
The devil and tom walker symbols
Knowledge analytics inc
Power bi qualitative data
Text analytics summit
Idol
Text analytics world
Text analytics forum 2019
Wziu hub
Jmp text explorer
Pptmoban
Text analytics unipi
What is shared knowledge
Knowledge shared is knowledge squared meaning
Knowledge shared is knowledge multiplied
Knowledge creation and knowledge architecture
Contoh shallow knowledge dan deep knowledge
Posteriori knowledge
Street smart vs book smart
Shared knowledge vs personal knowledge
Gertler econ
Strategy development workshop
Strategy development workshop
Business development workshop