Stylistics and Stylometry CSC 4598 Machine Translation Dr
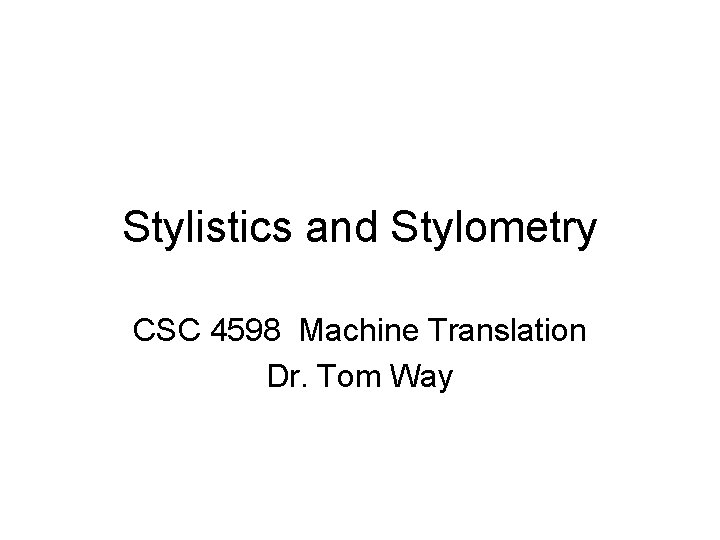
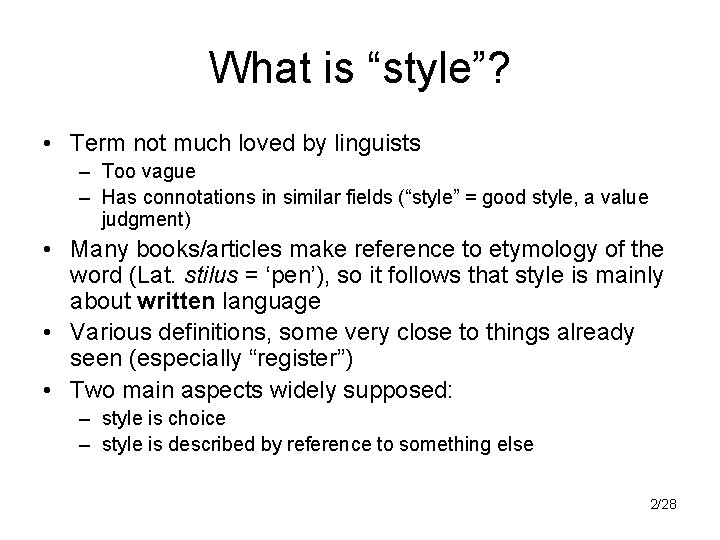
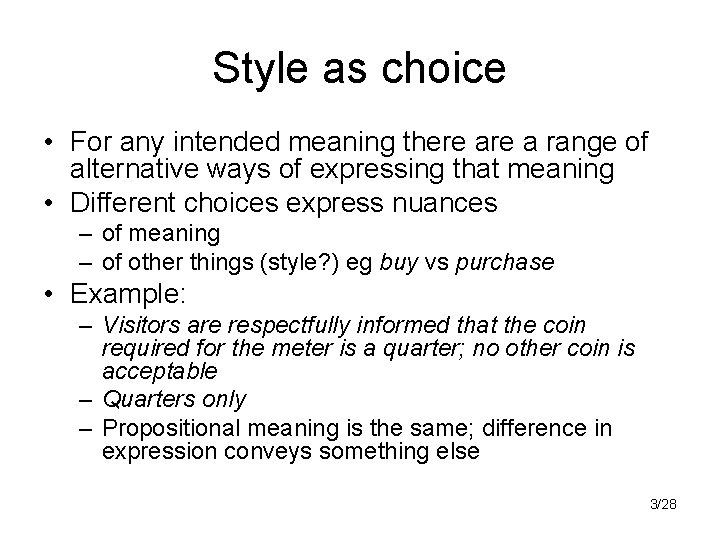
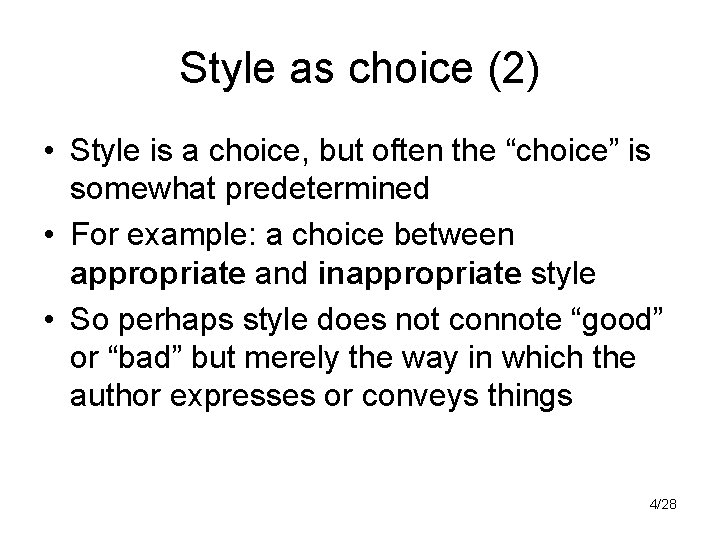
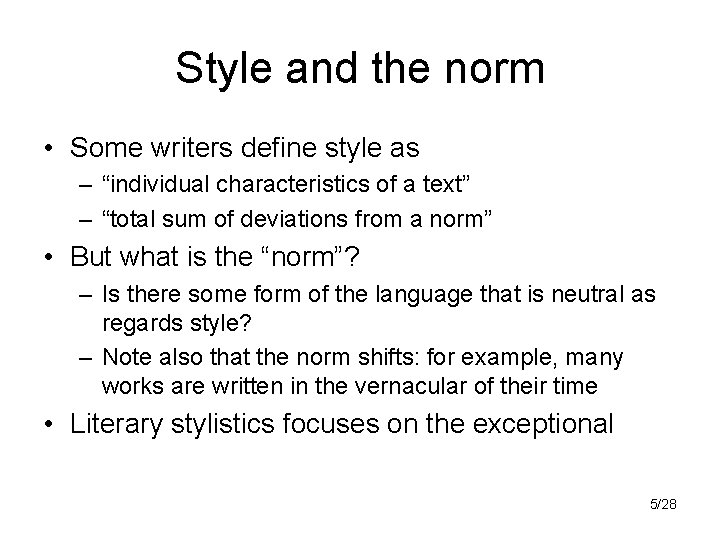
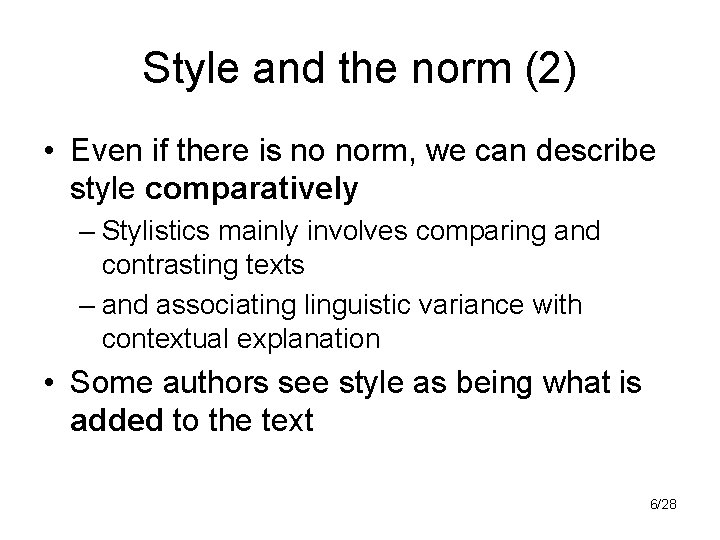
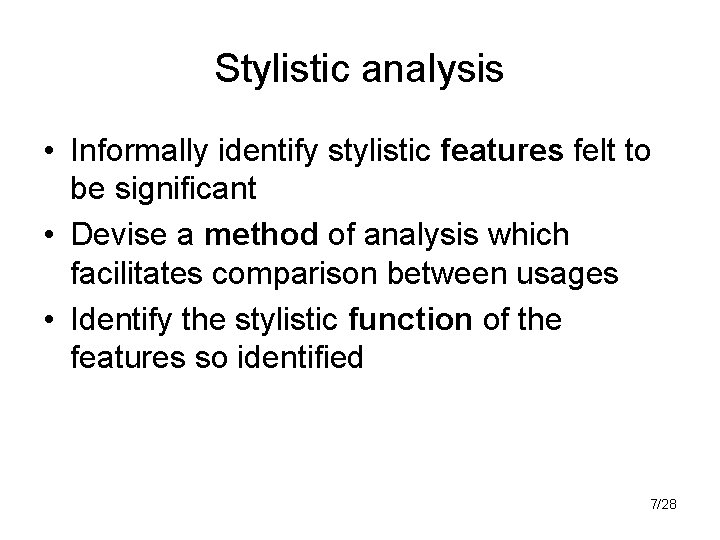
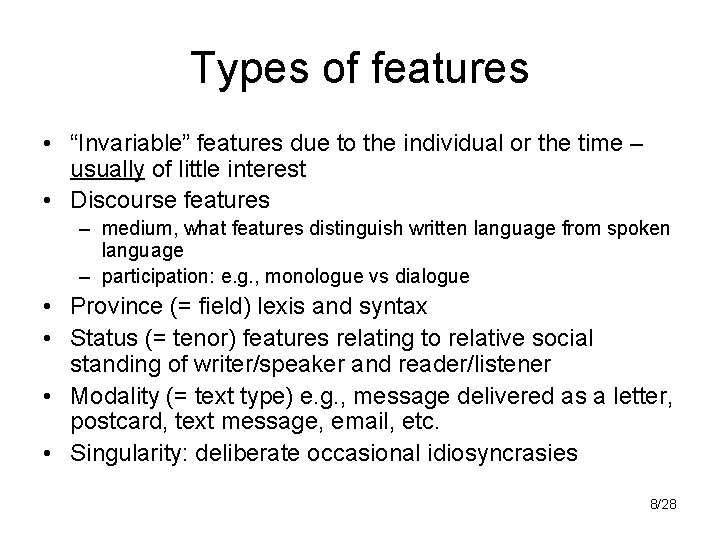
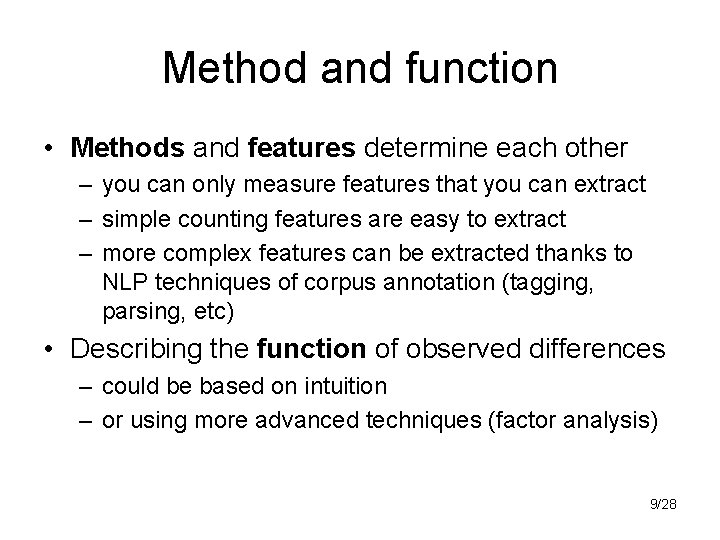
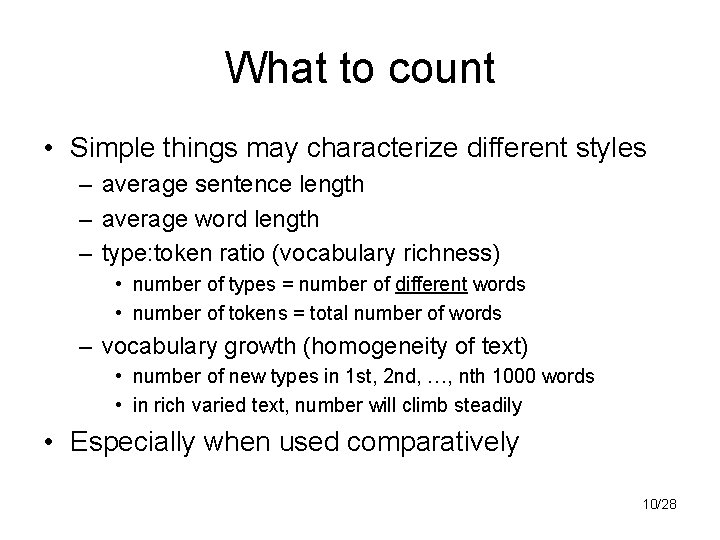
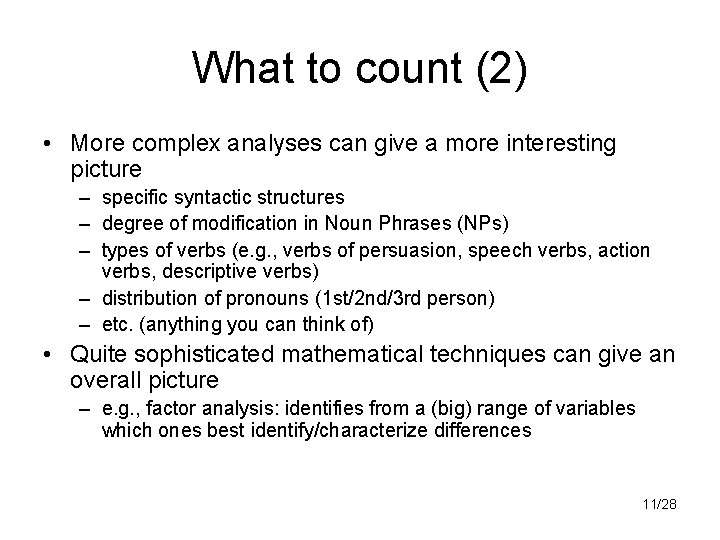
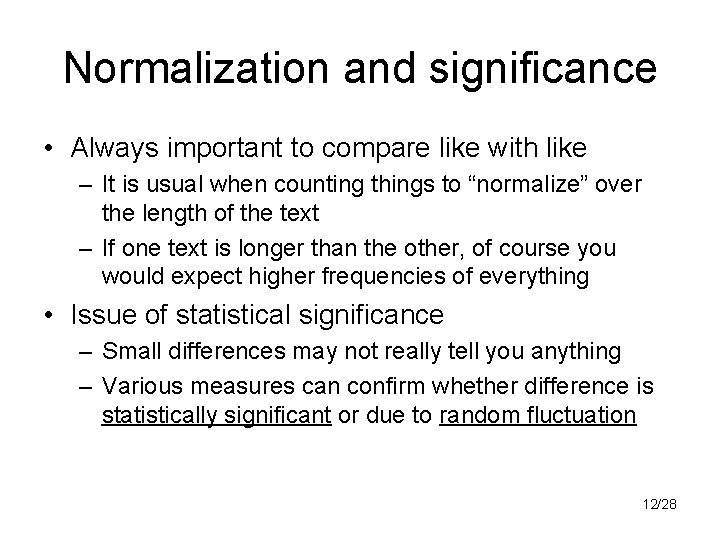
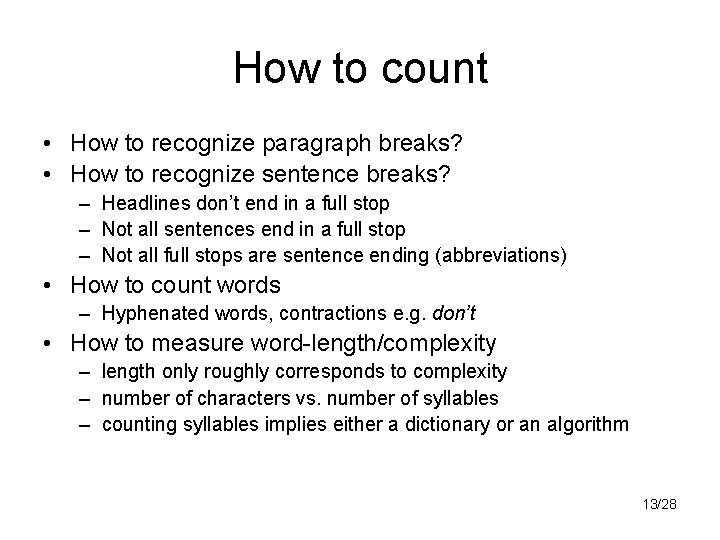
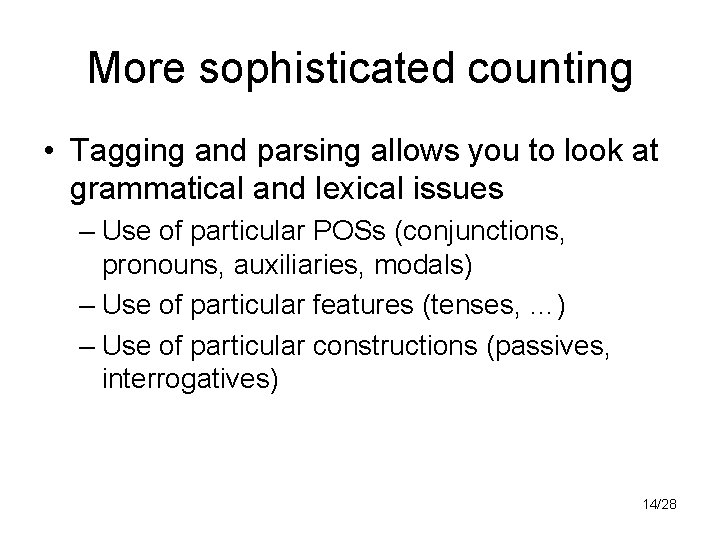
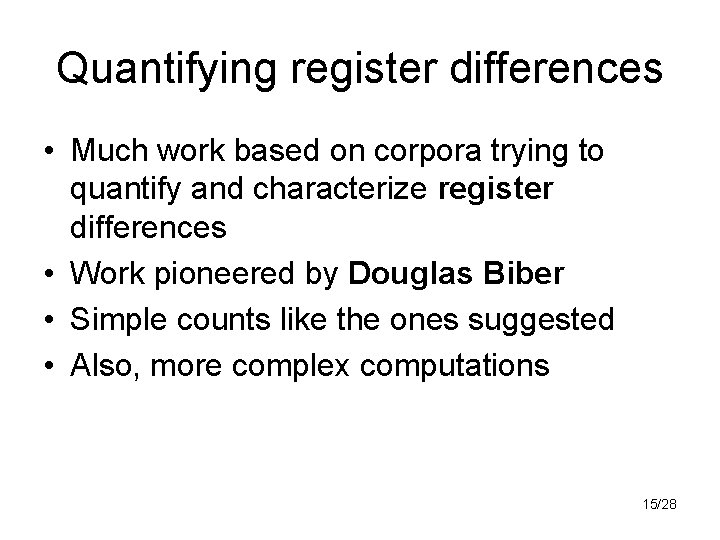
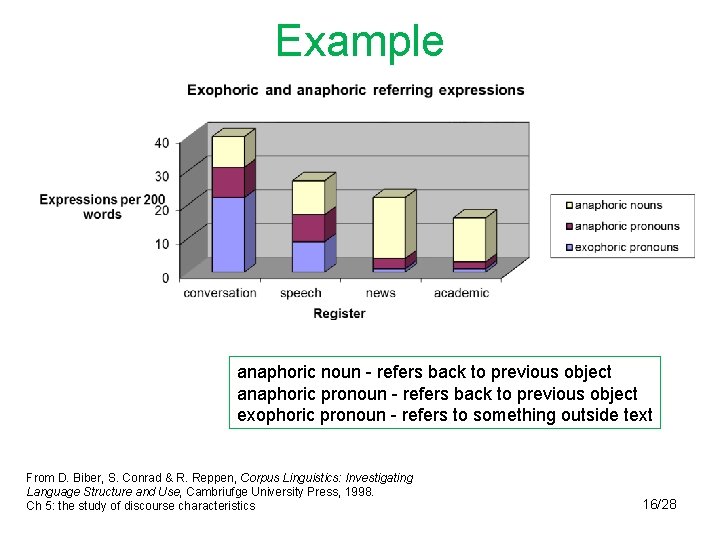
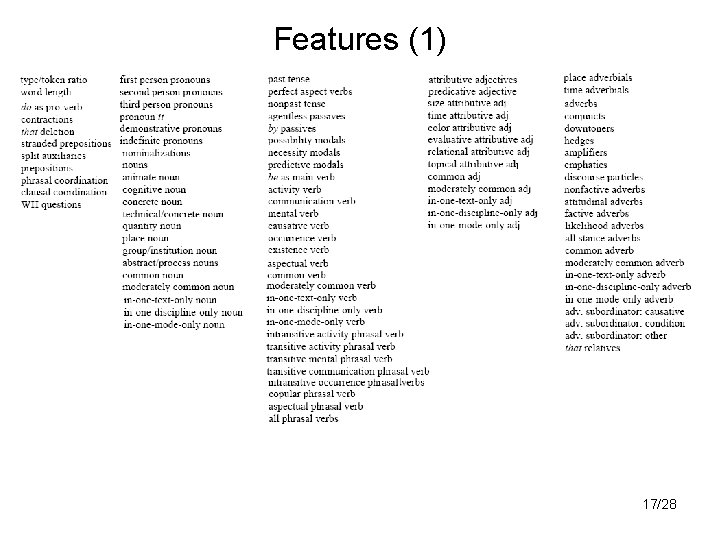
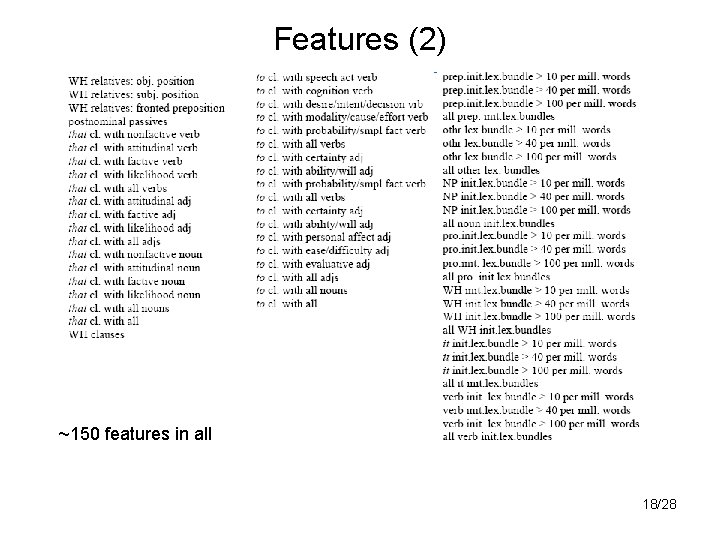
- Slides: 18
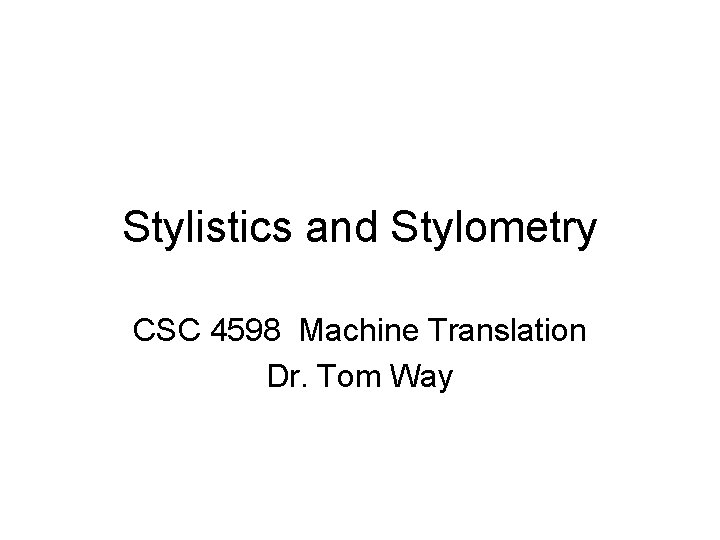
Stylistics and Stylometry CSC 4598 Machine Translation Dr. Tom Way
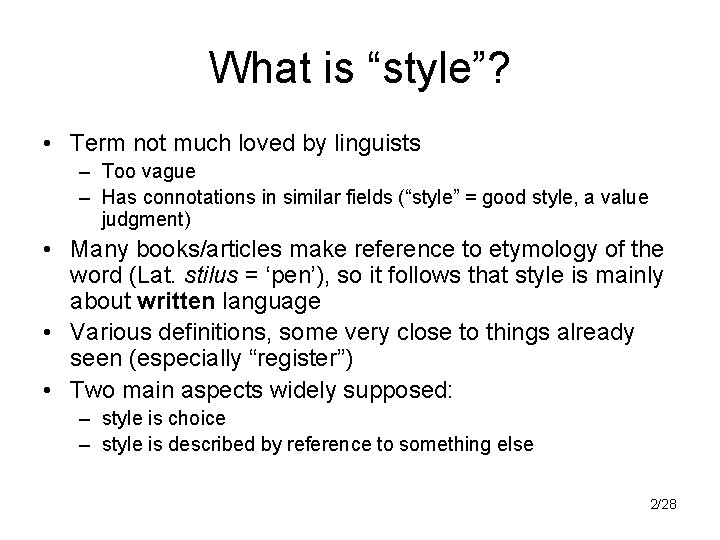
What is “style”? • Term not much loved by linguists – Too vague – Has connotations in similar fields (“style” = good style, a value judgment) • Many books/articles make reference to etymology of the word (Lat. stilus = ‘pen’), so it follows that style is mainly about written language • Various definitions, some very close to things already seen (especially “register”) • Two main aspects widely supposed: – style is choice – style is described by reference to something else 2/28
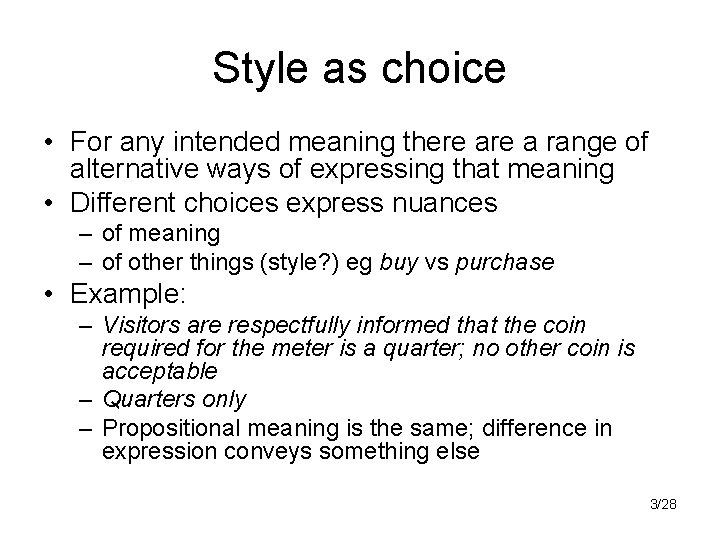
Style as choice • For any intended meaning there a range of alternative ways of expressing that meaning • Different choices express nuances – of meaning – of other things (style? ) eg buy vs purchase • Example: – Visitors are respectfully informed that the coin required for the meter is a quarter; no other coin is acceptable – Quarters only – Propositional meaning is the same; difference in expression conveys something else 3/28
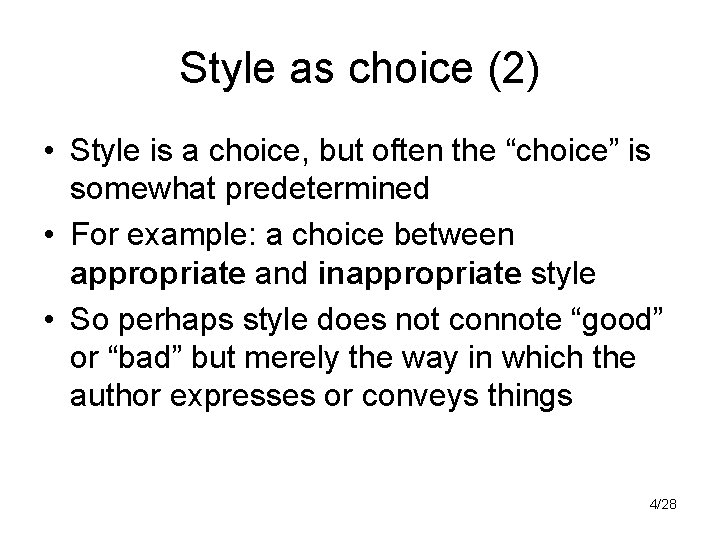
Style as choice (2) • Style is a choice, but often the “choice” is somewhat predetermined • For example: a choice between appropriate and inappropriate style • So perhaps style does not connote “good” or “bad” but merely the way in which the author expresses or conveys things 4/28
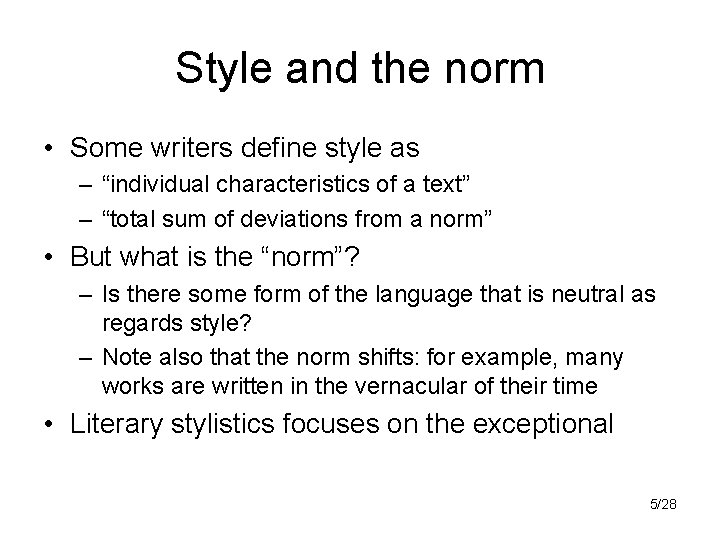
Style and the norm • Some writers define style as – “individual characteristics of a text” – “total sum of deviations from a norm” • But what is the “norm”? – Is there some form of the language that is neutral as regards style? – Note also that the norm shifts: for example, many works are written in the vernacular of their time • Literary stylistics focuses on the exceptional 5/28
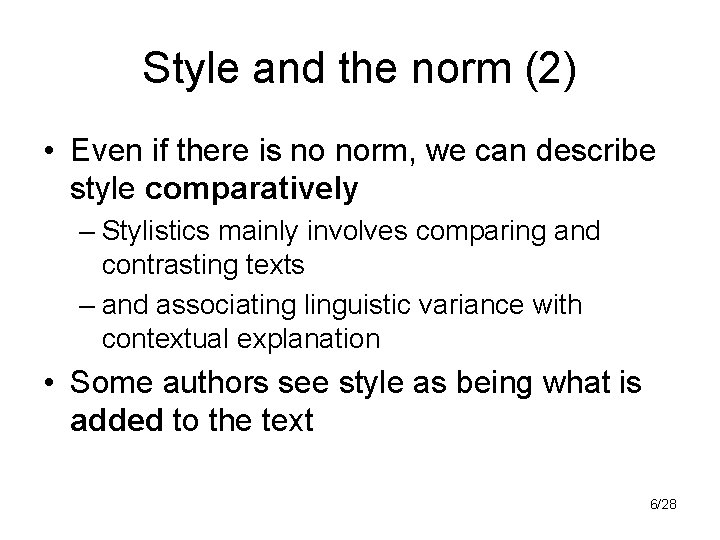
Style and the norm (2) • Even if there is no norm, we can describe style comparatively – Stylistics mainly involves comparing and contrasting texts – and associating linguistic variance with contextual explanation • Some authors see style as being what is added to the text 6/28
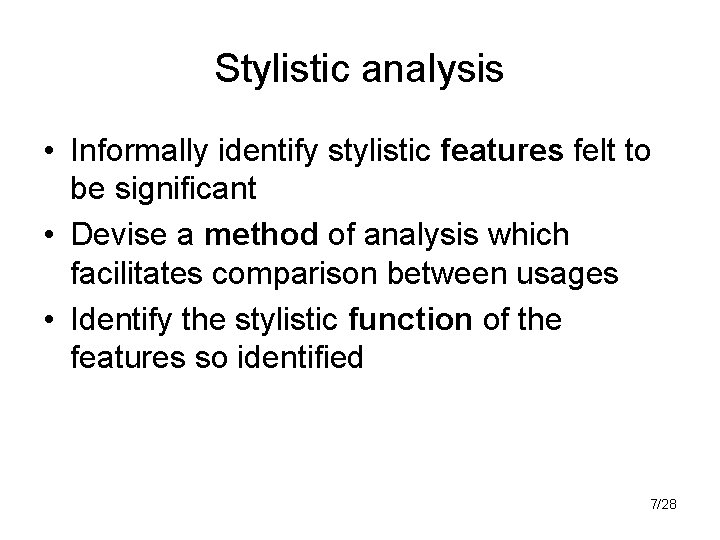
Stylistic analysis • Informally identify stylistic features felt to be significant • Devise a method of analysis which facilitates comparison between usages • Identify the stylistic function of the features so identified 7/28
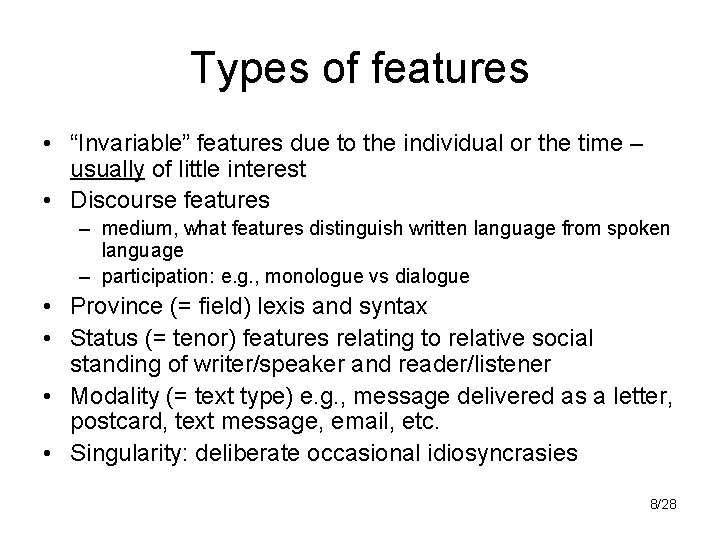
Types of features • “Invariable” features due to the individual or the time – usually of little interest • Discourse features – medium, what features distinguish written language from spoken language – participation: e. g. , monologue vs dialogue • Province (= field) lexis and syntax • Status (= tenor) features relating to relative social standing of writer/speaker and reader/listener • Modality (= text type) e. g. , message delivered as a letter, postcard, text message, email, etc. • Singularity: deliberate occasional idiosyncrasies 8/28
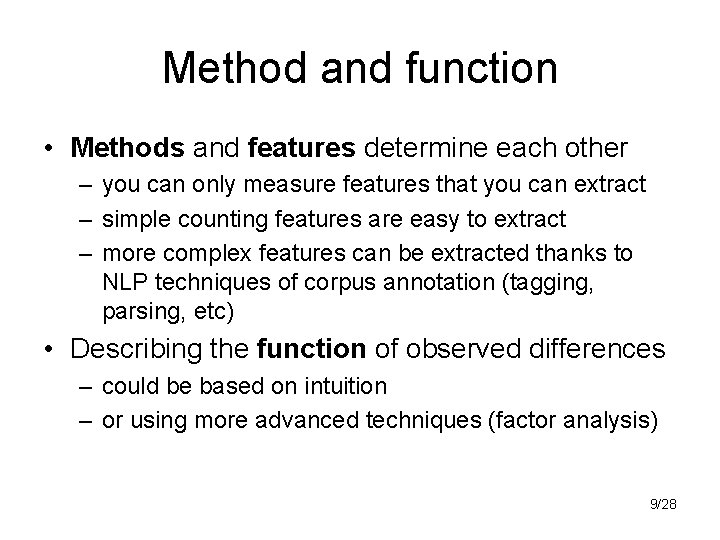
Method and function • Methods and features determine each other – you can only measure features that you can extract – simple counting features are easy to extract – more complex features can be extracted thanks to NLP techniques of corpus annotation (tagging, parsing, etc) • Describing the function of observed differences – could be based on intuition – or using more advanced techniques (factor analysis) 9/28
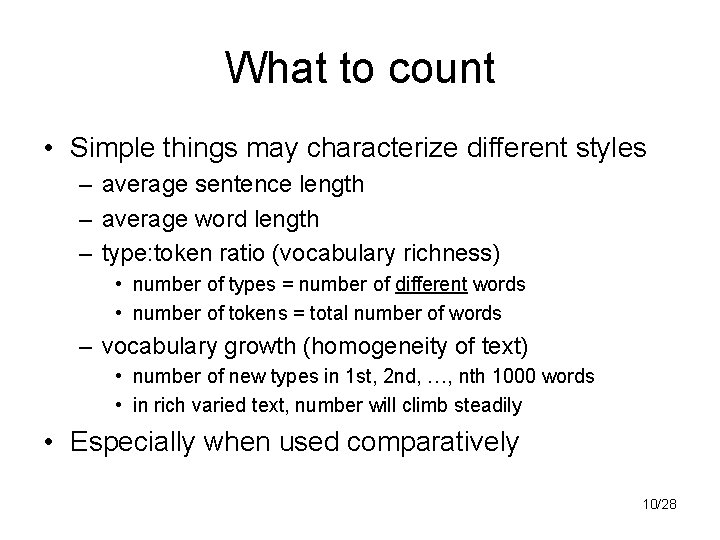
What to count • Simple things may characterize different styles – average sentence length – average word length – type: token ratio (vocabulary richness) • number of types = number of different words • number of tokens = total number of words – vocabulary growth (homogeneity of text) • number of new types in 1 st, 2 nd, …, nth 1000 words • in rich varied text, number will climb steadily • Especially when used comparatively 10/28
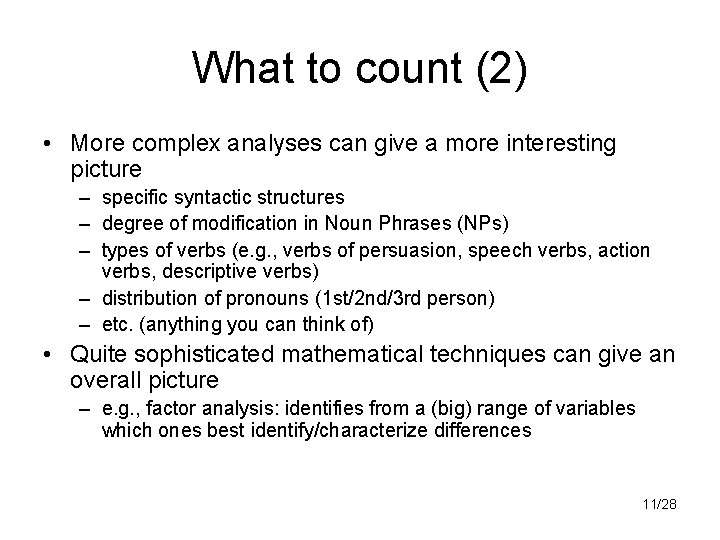
What to count (2) • More complex analyses can give a more interesting picture – specific syntactic structures – degree of modification in Noun Phrases (NPs) – types of verbs (e. g. , verbs of persuasion, speech verbs, action verbs, descriptive verbs) – distribution of pronouns (1 st/2 nd/3 rd person) – etc. (anything you can think of) • Quite sophisticated mathematical techniques can give an overall picture – e. g. , factor analysis: identifies from a (big) range of variables which ones best identify/characterize differences 11/28
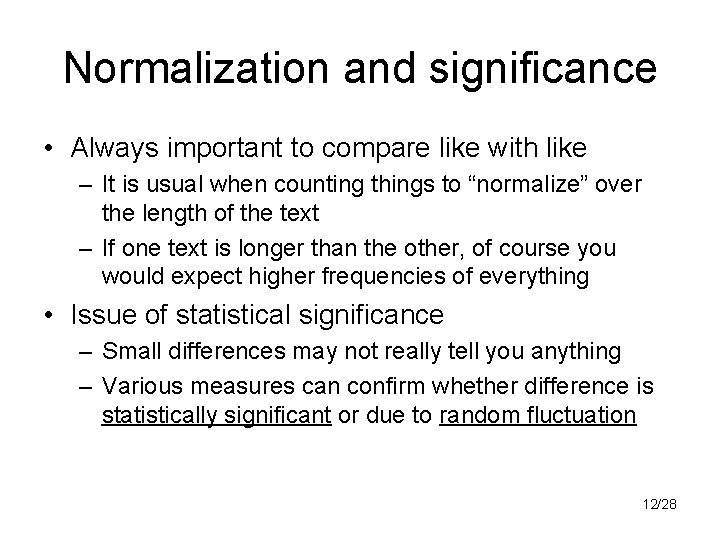
Normalization and significance • Always important to compare like with like – It is usual when counting things to “normalize” over the length of the text – If one text is longer than the other, of course you would expect higher frequencies of everything • Issue of statistical significance – Small differences may not really tell you anything – Various measures can confirm whether difference is statistically significant or due to random fluctuation 12/28
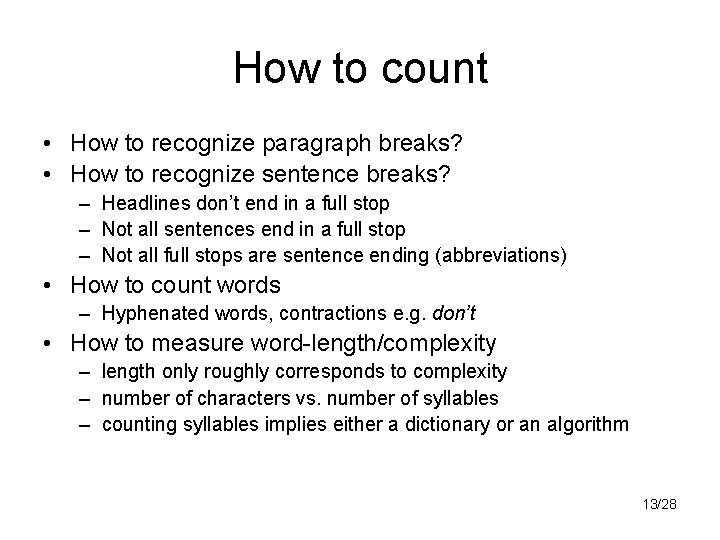
How to count • How to recognize paragraph breaks? • How to recognize sentence breaks? – Headlines don’t end in a full stop – Not all sentences end in a full stop – Not all full stops are sentence ending (abbreviations) • How to count words – Hyphenated words, contractions e. g. don’t • How to measure word-length/complexity – length only roughly corresponds to complexity – number of characters vs. number of syllables – counting syllables implies either a dictionary or an algorithm 13/28
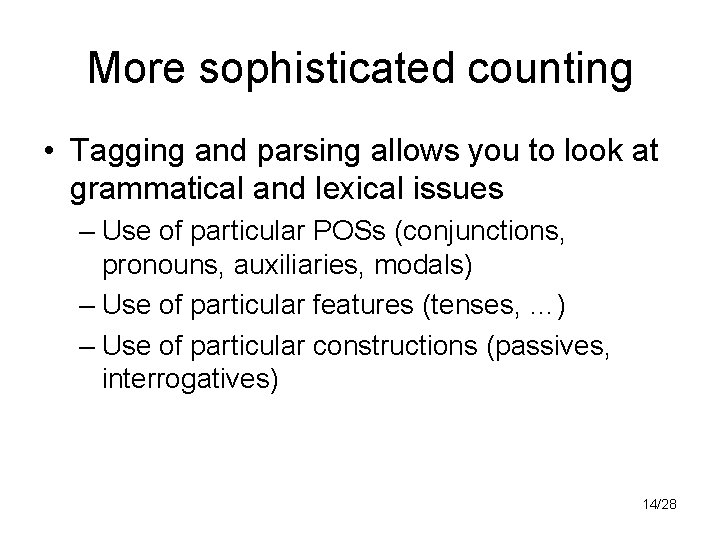
More sophisticated counting • Tagging and parsing allows you to look at grammatical and lexical issues – Use of particular POSs (conjunctions, pronouns, auxiliaries, modals) – Use of particular features (tenses, …) – Use of particular constructions (passives, interrogatives) 14/28
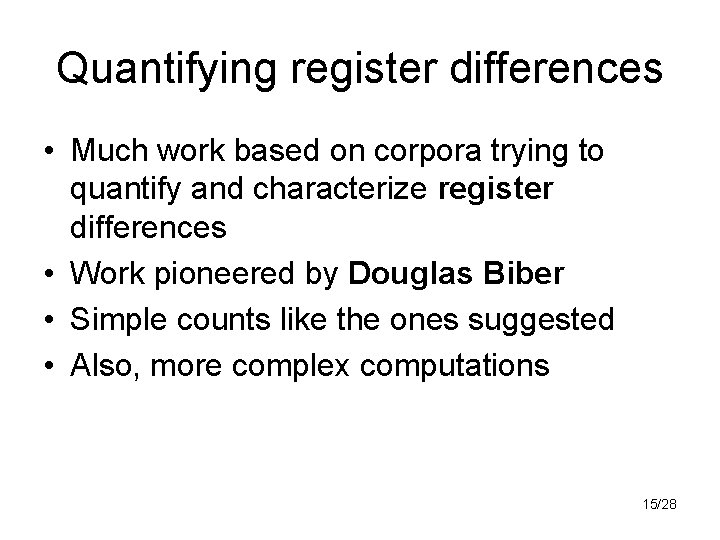
Quantifying register differences • Much work based on corpora trying to quantify and characterize register differences • Work pioneered by Douglas Biber • Simple counts like the ones suggested • Also, more complex computations 15/28
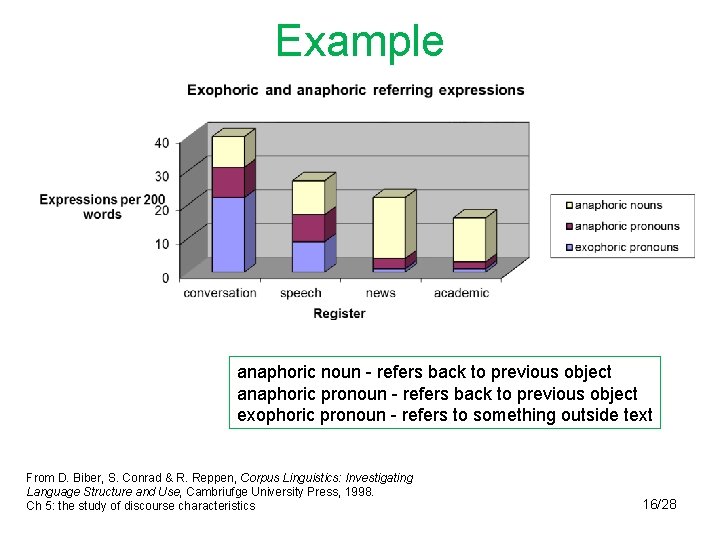
Example anaphoric noun - refers back to previous object anaphoric pronoun - refers back to previous object exophoric pronoun - refers to something outside text From D. Biber, S. Conrad & R. Reppen, Corpus Linguistics: Investigating Language Structure and Use, Cambriufge University Press, 1998. Ch 5: the study of discourse characteristics 16/28
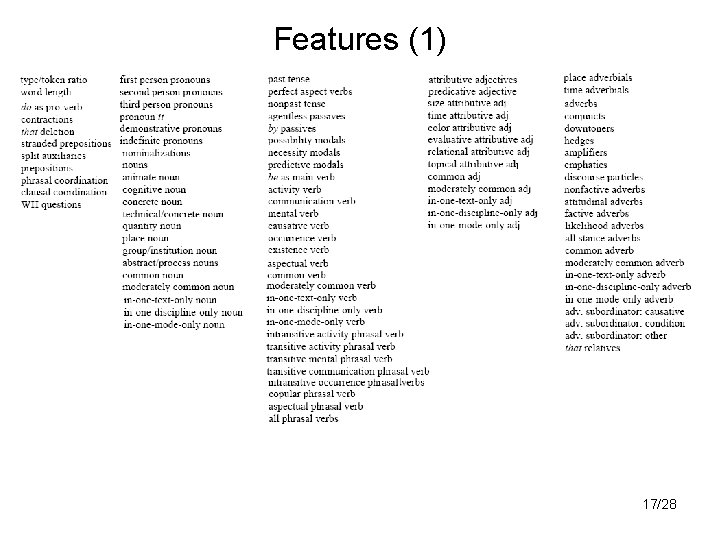
Features (1) 17/28
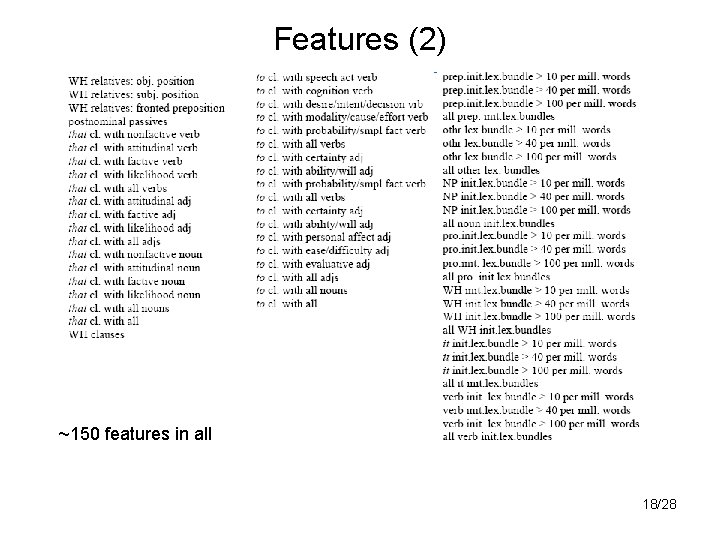
Features (2) ~150 features in all 18/28
Duke of venice othello
Csc translation
What is semantic translation?
Vertical translation and horizontal translation
Visualizing and understanding neural machine translation
Number translation using voice translation profiles
Semantic translation và communicative translation
Types of speech style
What's stylistics
Three basic principles of stylistics
Defamiliarization
Moore machine
Energy work and simple machines chapter 10 answers
Interactive machine translation
Lms machine translation
Google translate
Machine translation
Machine translation
Machine translation