Strengthening statistical capacity in ACP countries Lessons learned
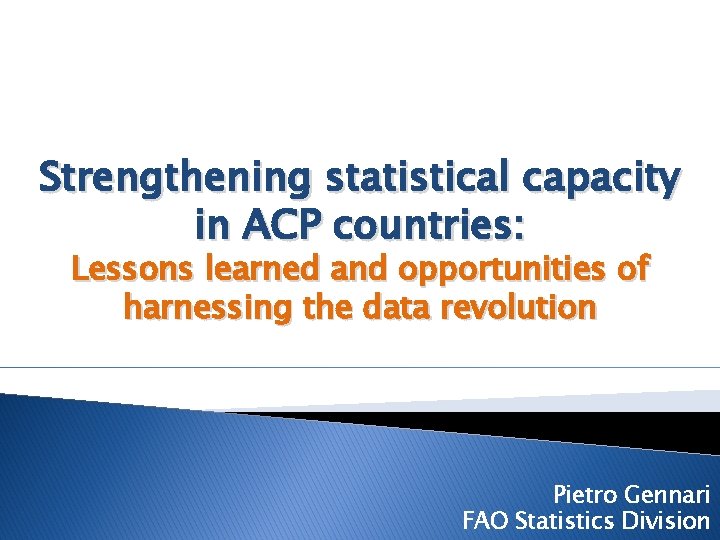
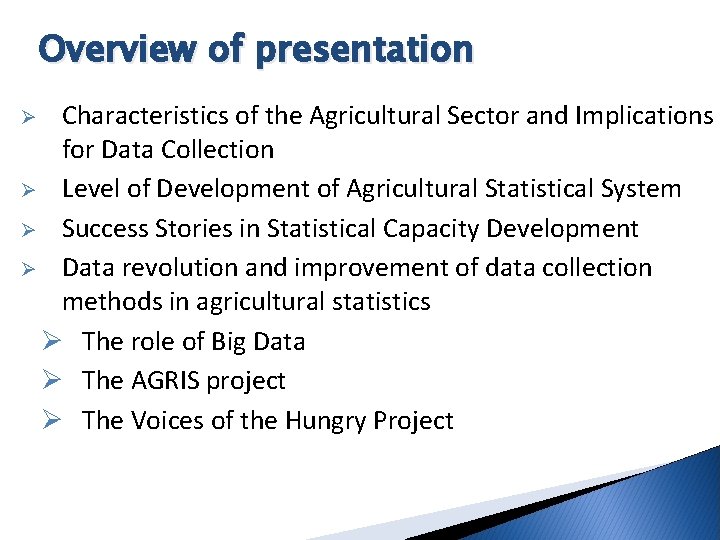
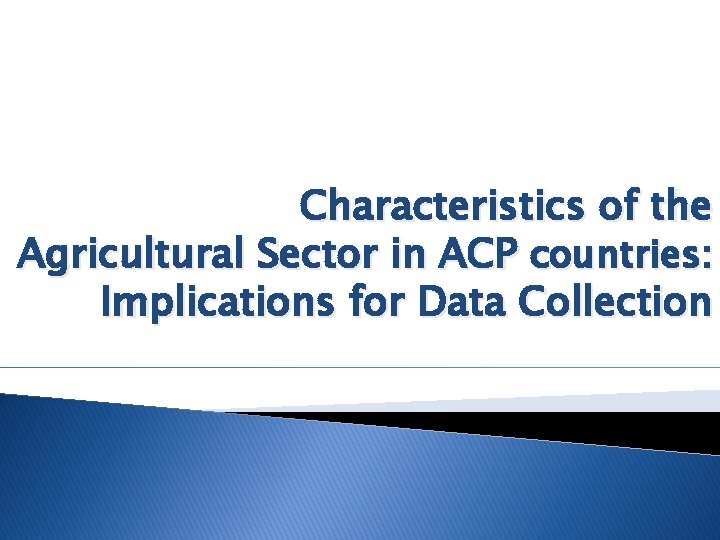
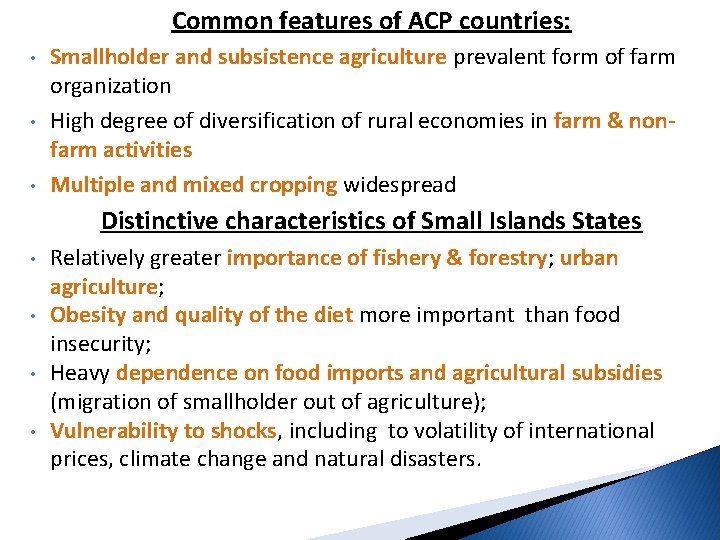
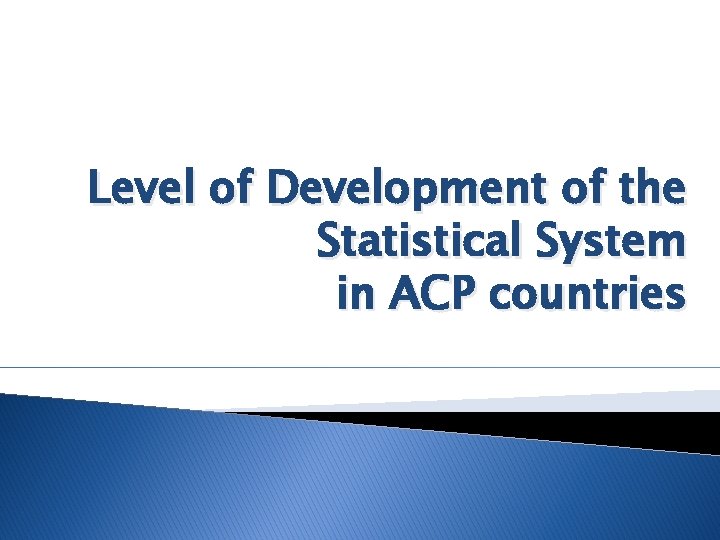
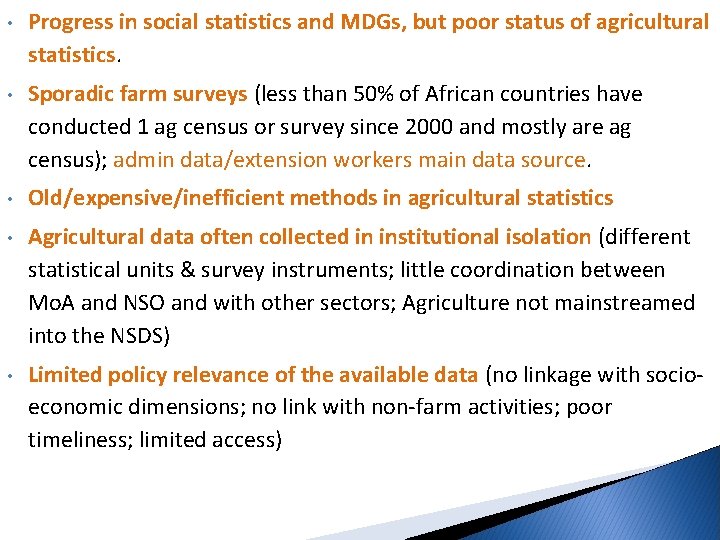
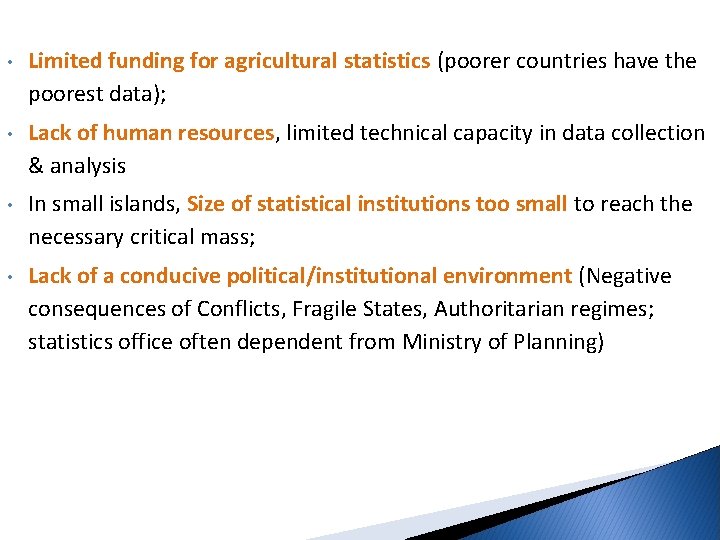
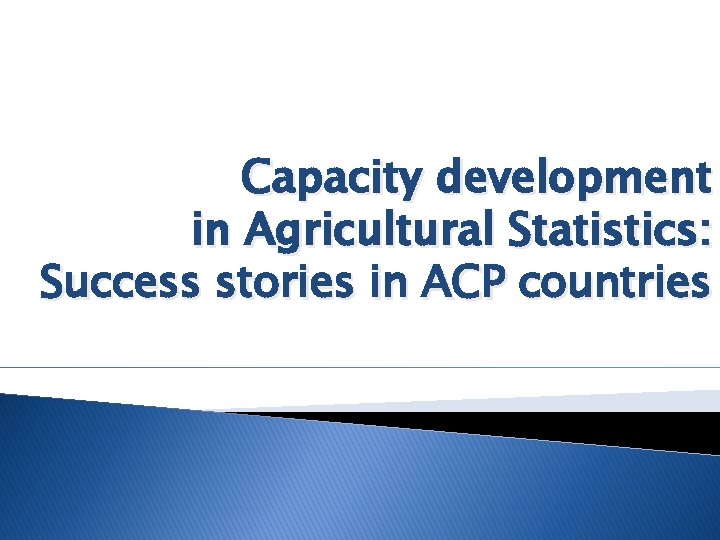
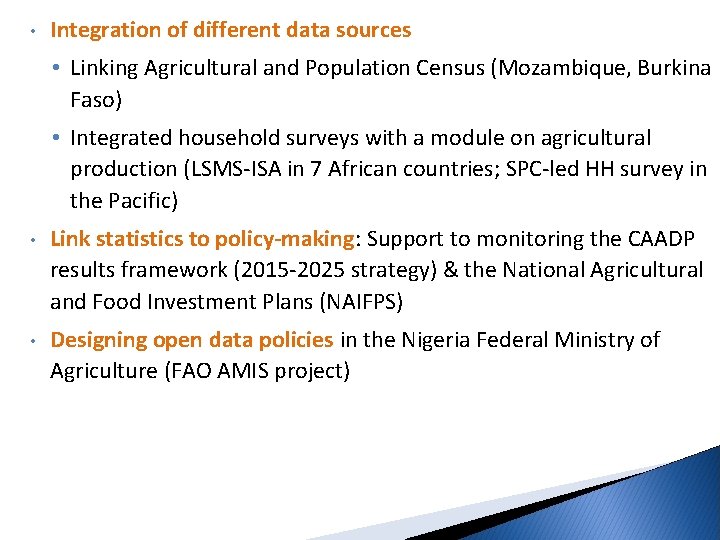
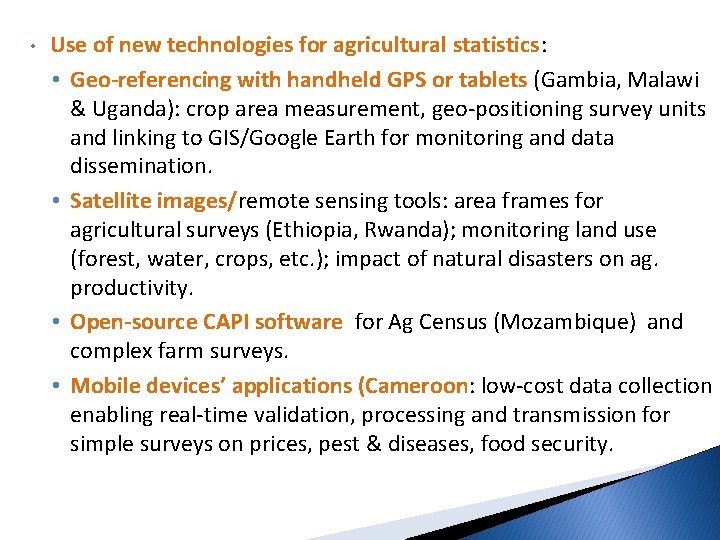
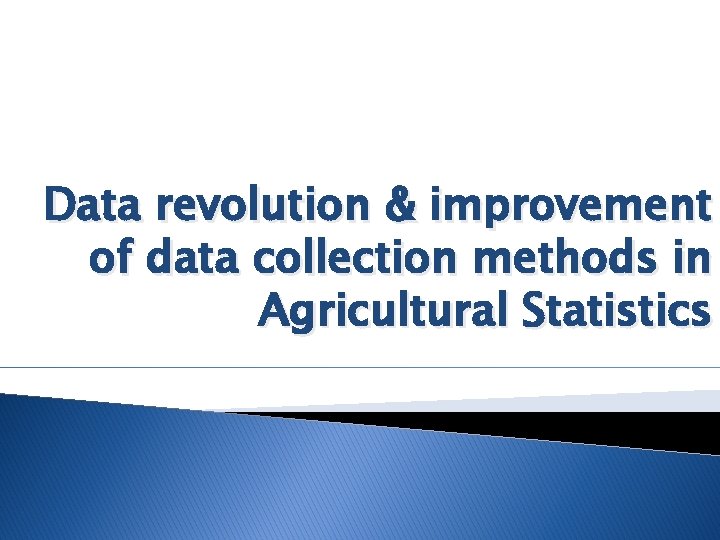
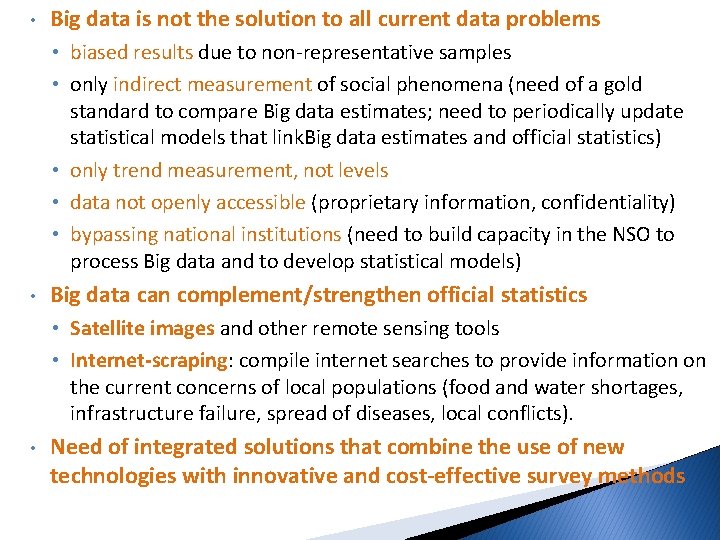
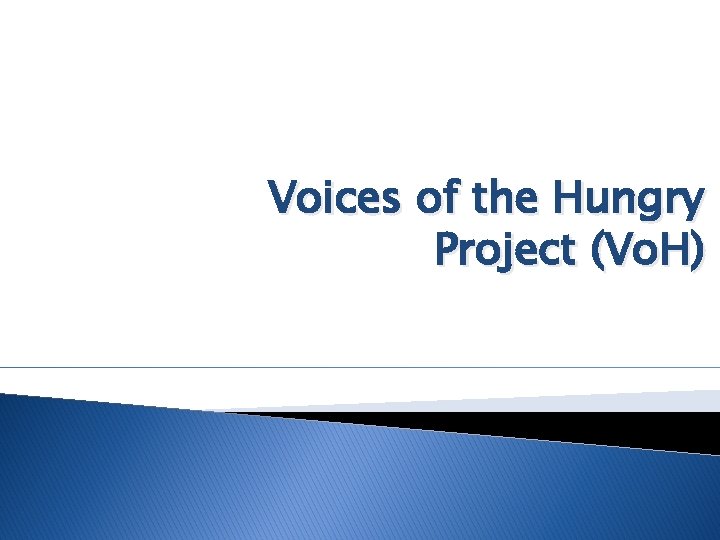
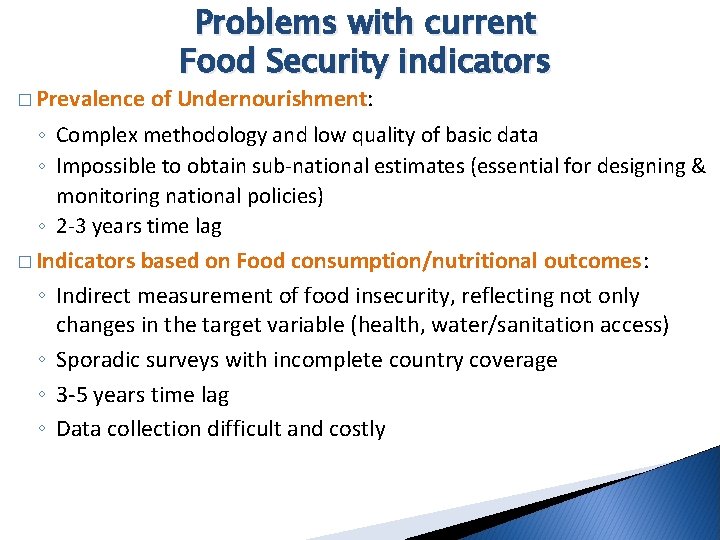
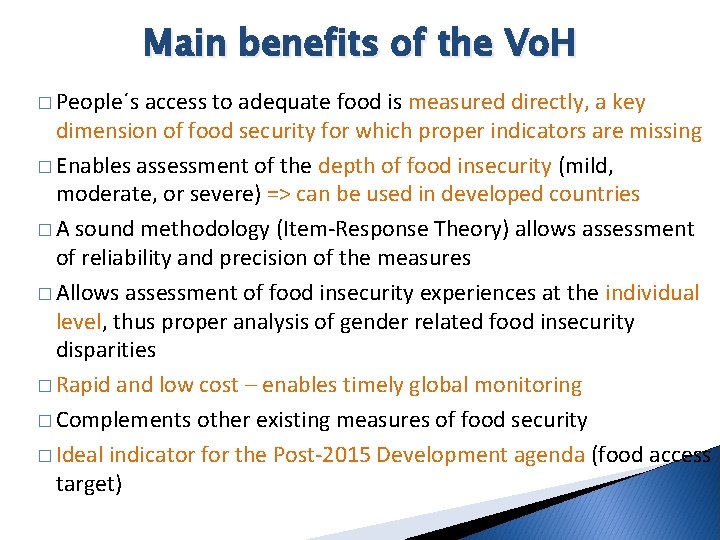
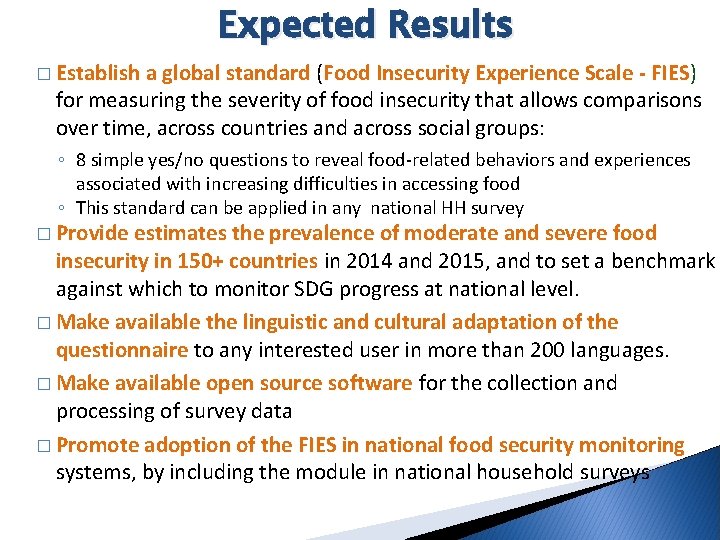
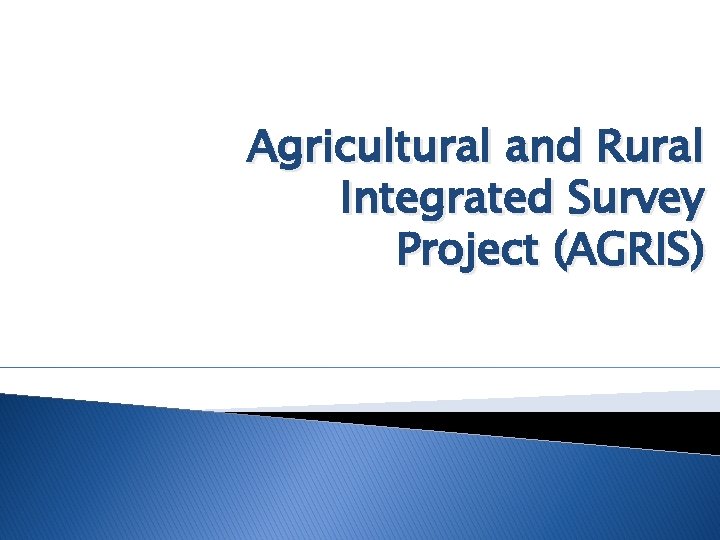
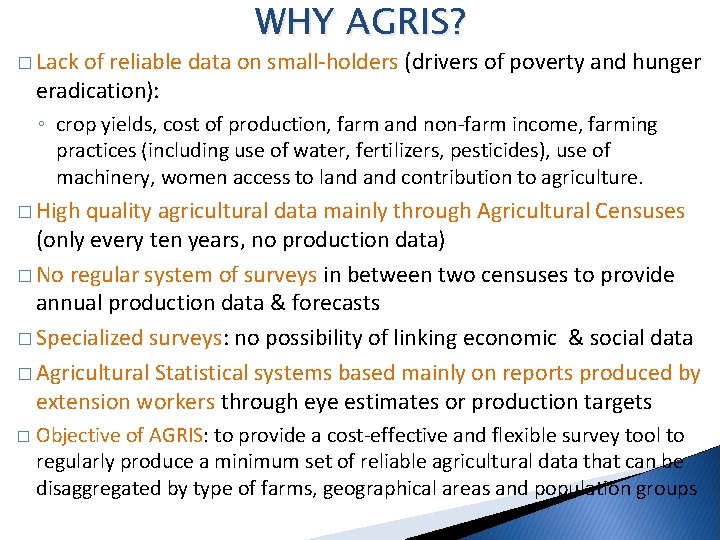
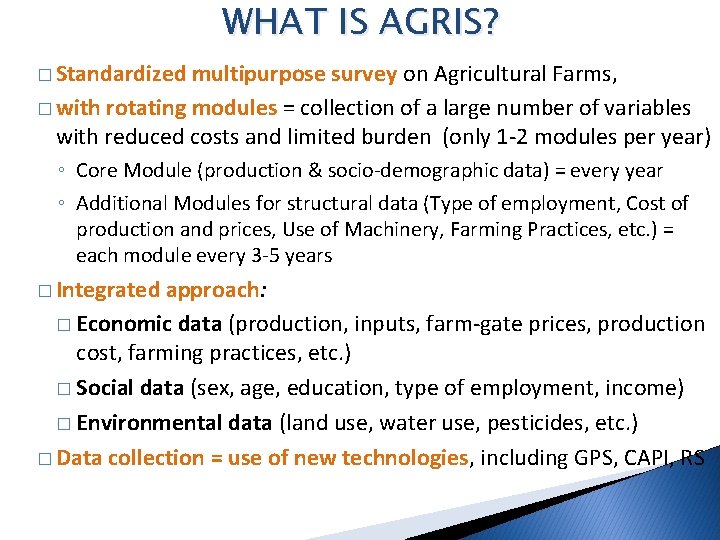
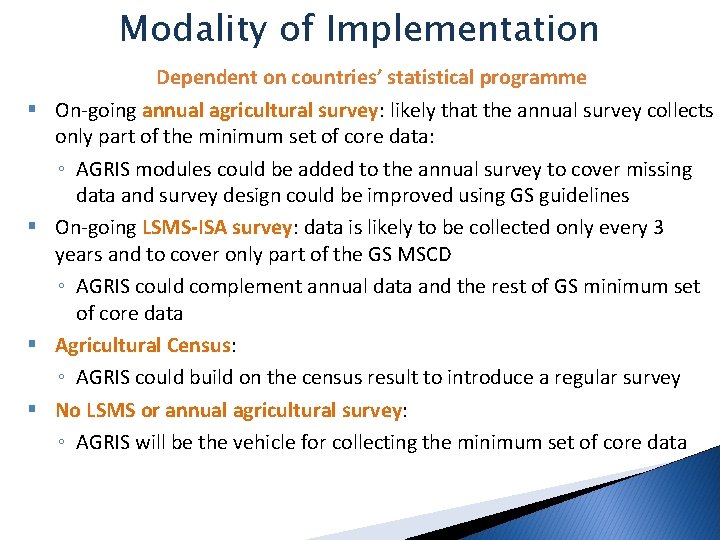
- Slides: 20
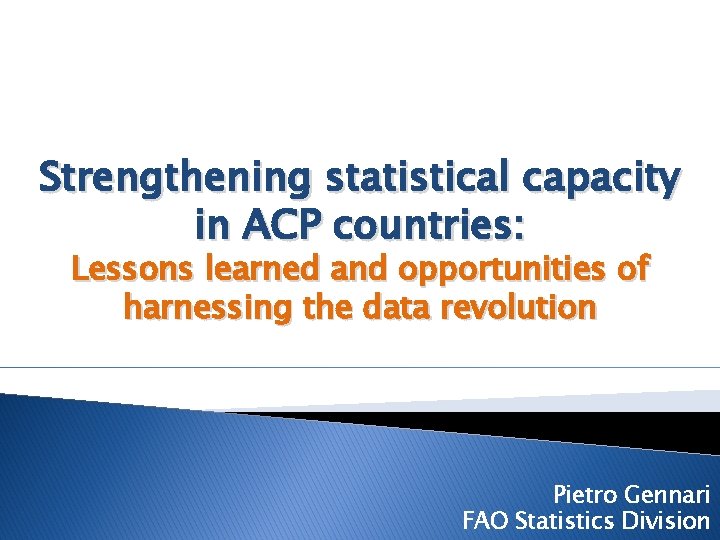
Strengthening statistical capacity in ACP countries: Lessons learned and opportunities of harnessing the data revolution Pietro Gennari FAO Statistics Division
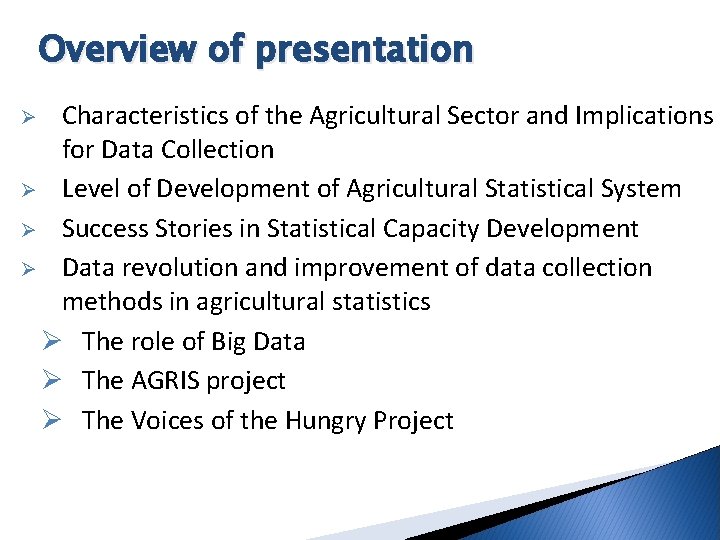
Overview of presentation Characteristics of the Agricultural Sector and Implications for Data Collection Ø Level of Development of Agricultural Statistical System Ø Success Stories in Statistical Capacity Development Ø Data revolution and improvement of data collection methods in agricultural statistics Ø The role of Big Data Ø The AGRIS project Ø The Voices of the Hungry Project Ø
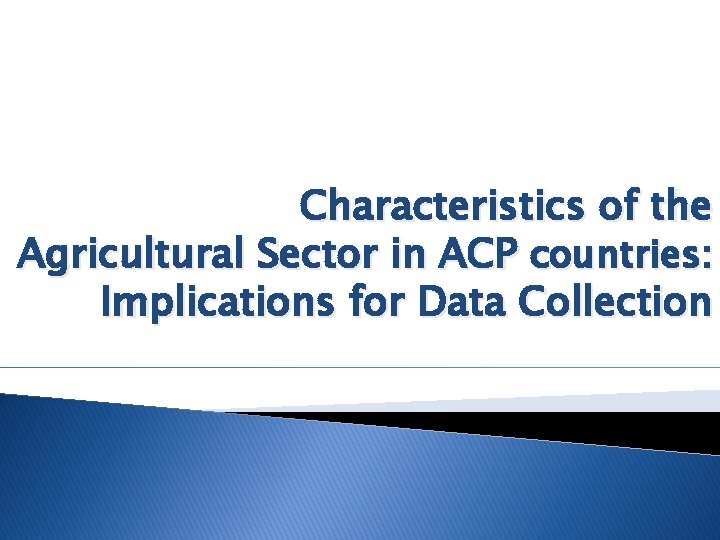
Characteristics of the Agricultural Sector in ACP countries: Implications for Data Collection
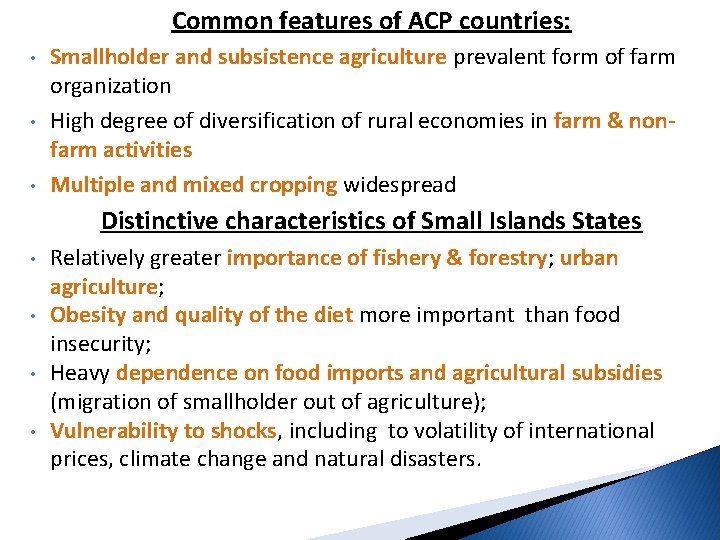
Common features of ACP countries: • • • Smallholder and subsistence agriculture prevalent form of farm organization High degree of diversification of rural economies in farm & nonfarm activities Multiple and mixed cropping widespread Distinctive characteristics of Small Islands States • • Relatively greater importance of fishery & forestry; urban agriculture; Obesity and quality of the diet more important than food insecurity; Heavy dependence on food imports and agricultural subsidies (migration of smallholder out of agriculture); Vulnerability to shocks, including to volatility of international prices, climate change and natural disasters.
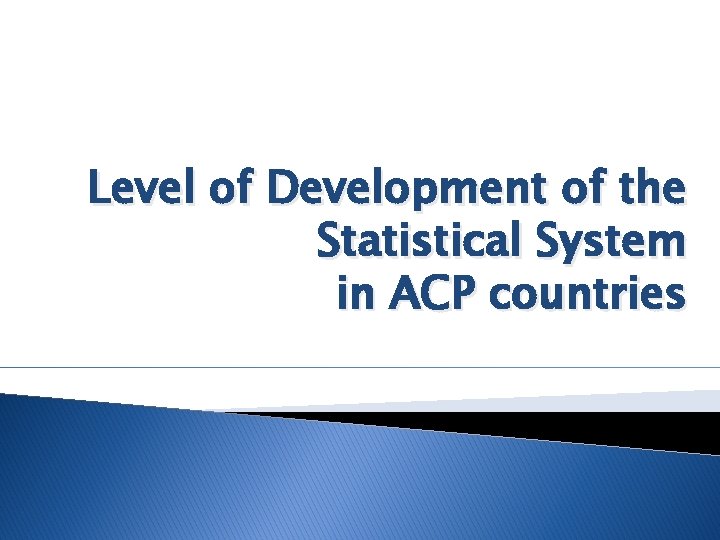
Level of Development of the Statistical System in ACP countries
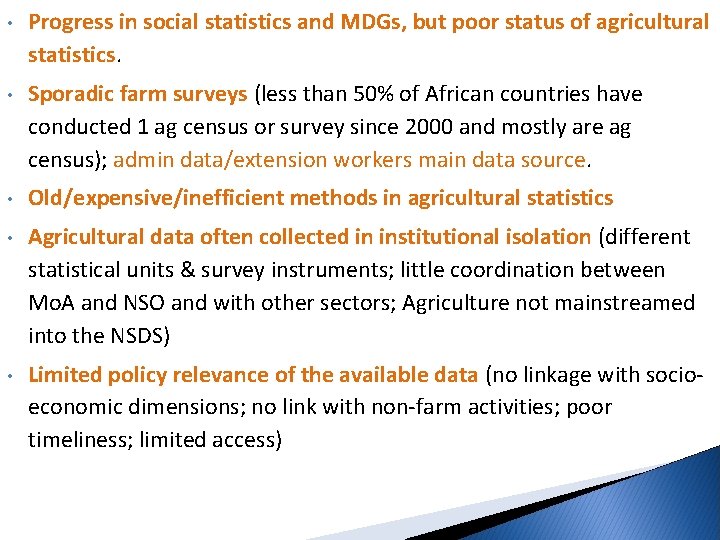
• Progress in social statistics and MDGs, but poor status of agricultural statistics. • Sporadic farm surveys (less than 50% of African countries have conducted 1 ag census or survey since 2000 and mostly are ag census); admin data/extension workers main data source. • Old/expensive/inefficient methods in agricultural statistics • Agricultural data often collected in institutional isolation (different statistical units & survey instruments; little coordination between Mo. A and NSO and with other sectors; Agriculture not mainstreamed into the NSDS) • Limited policy relevance of the available data (no linkage with socioeconomic dimensions; no link with non-farm activities; poor timeliness; limited access)
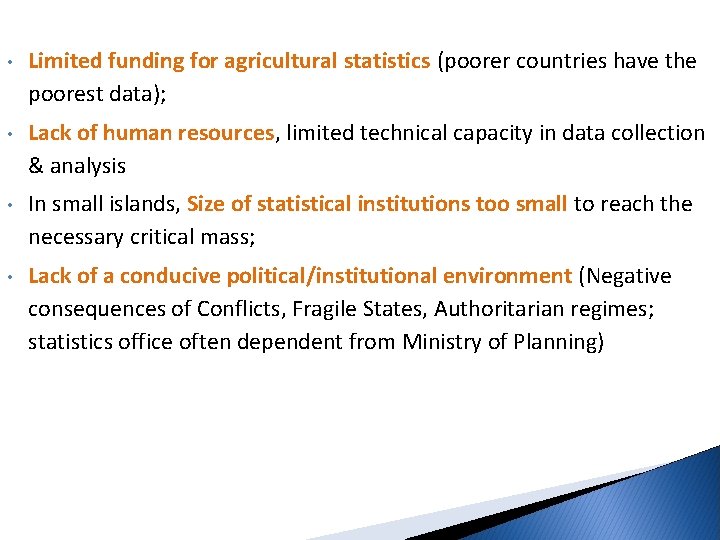
• Limited funding for agricultural statistics (poorer countries have the poorest data); • Lack of human resources, limited technical capacity in data collection & analysis • In small islands, Size of statistical institutions too small to reach the necessary critical mass; • Lack of a conducive political/institutional environment (Negative consequences of Conflicts, Fragile States, Authoritarian regimes; statistics office often dependent from Ministry of Planning)
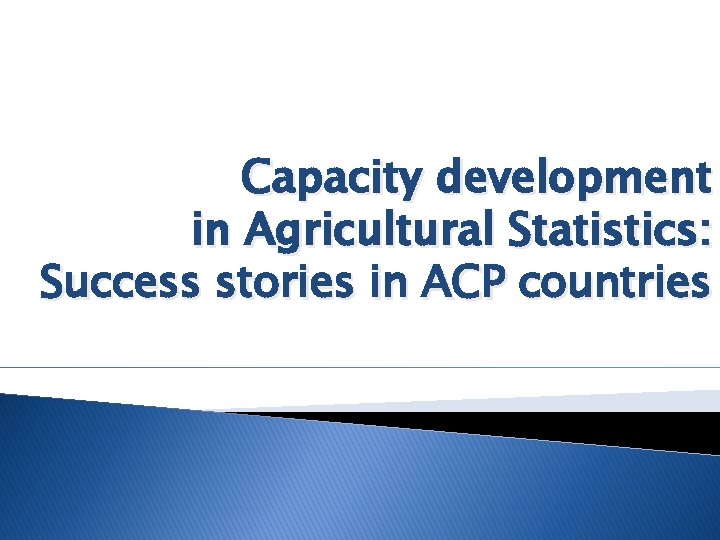
Capacity development in Agricultural Statistics: Success stories in ACP countries
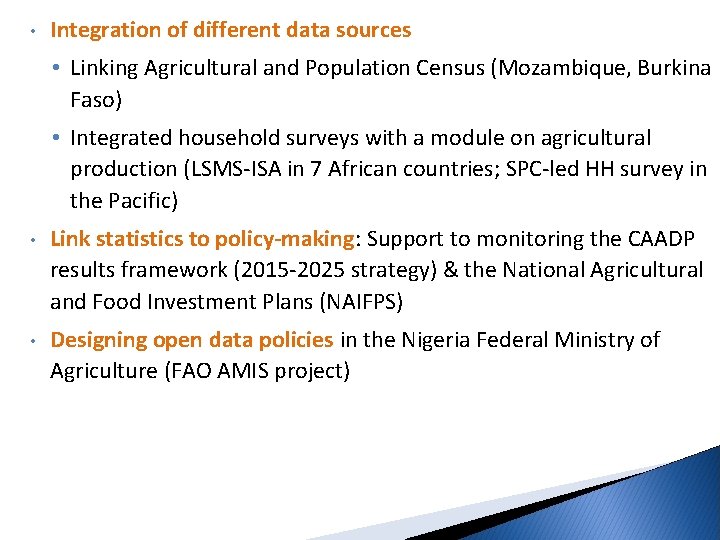
• Integration of different data sources • Linking Agricultural and Population Census (Mozambique, Burkina Faso) • Integrated household surveys with a module on agricultural production (LSMS-ISA in 7 African countries; SPC-led HH survey in the Pacific) • Link statistics to policy-making: Support to monitoring the CAADP results framework (2015 -2025 strategy) & the National Agricultural and Food Investment Plans (NAIFPS) • Designing open data policies in the Nigeria Federal Ministry of Agriculture (FAO AMIS project)
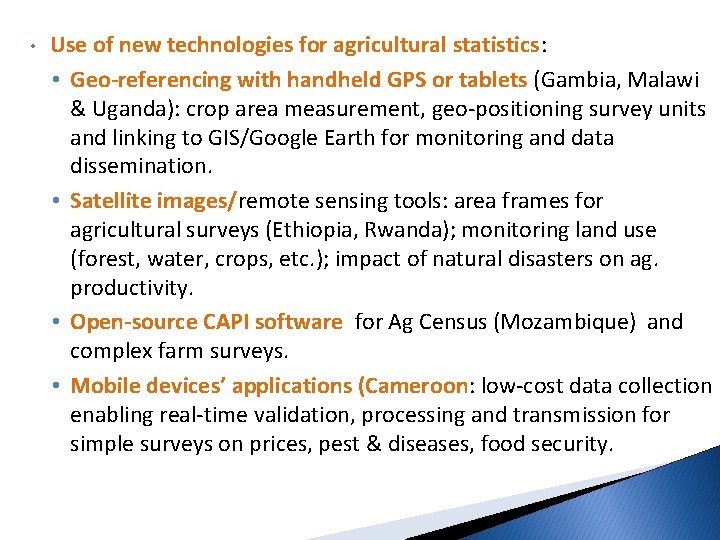
• Use of new technologies for agricultural statistics: • Geo-referencing with handheld GPS or tablets (Gambia, Malawi & Uganda): crop area measurement, geo-positioning survey units and linking to GIS/Google Earth for monitoring and data dissemination. • Satellite images/remote sensing tools: area frames for agricultural surveys (Ethiopia, Rwanda); monitoring land use (forest, water, crops, etc. ); impact of natural disasters on ag. productivity. • Open-source CAPI software for Ag Census (Mozambique) and complex farm surveys. • Mobile devices’ applications (Cameroon: low-cost data collection enabling real-time validation, processing and transmission for simple surveys on prices, pest & diseases, food security.
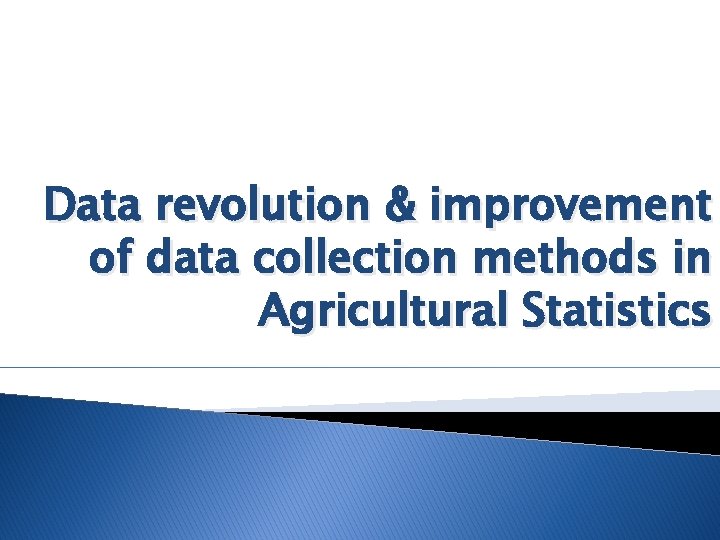
Data revolution & improvement of data collection methods in Agricultural Statistics
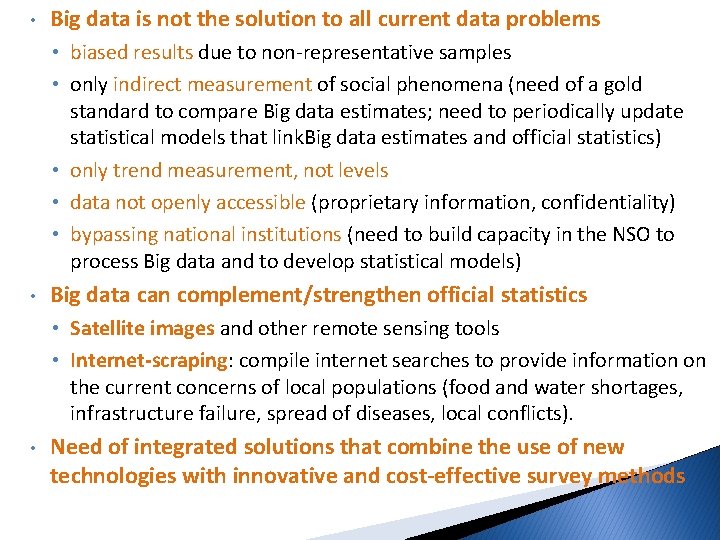
• Big data is not the solution to all current data problems • biased results due to non-representative samples • only indirect measurement of social phenomena (need of a gold standard to compare Big data estimates; need to periodically update statistical models that link. Big data estimates and official statistics) • only trend measurement, not levels • data not openly accessible (proprietary information, confidentiality) • bypassing national institutions (need to build capacity in the NSO to process Big data and to develop statistical models) • Big data can complement/strengthen official statistics • Satellite images and other remote sensing tools • Internet-scraping: compile internet searches to provide information on the current concerns of local populations (food and water shortages, infrastructure failure, spread of diseases, local conflicts). • Need of integrated solutions that combine the use of new technologies with innovative and cost-effective survey methods
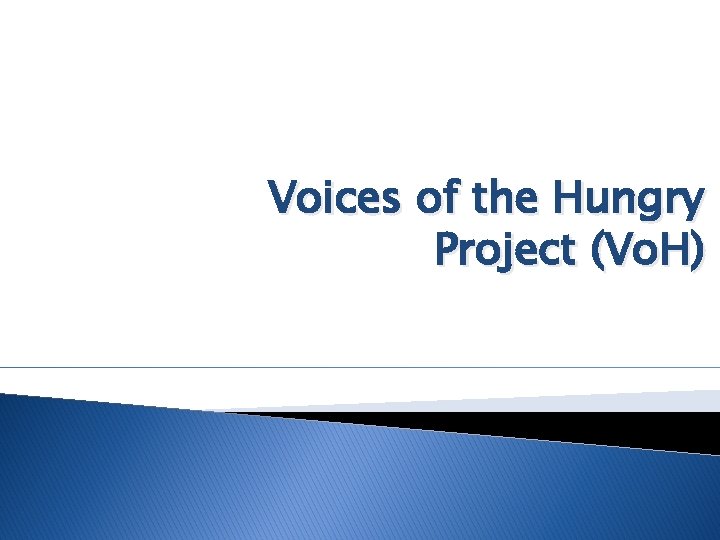
Voices of the Hungry Project (Vo. H)
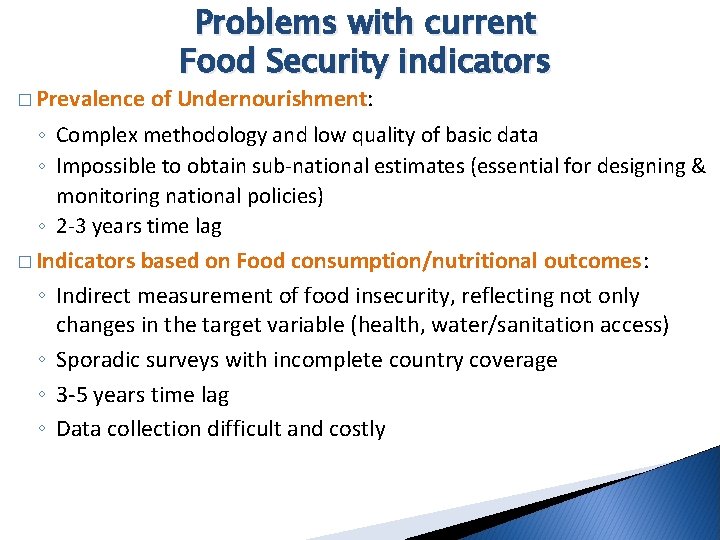
� Prevalence Problems with current Food Security indicators of Undernourishment: ◦ Complex methodology and low quality of basic data ◦ Impossible to obtain sub-national estimates (essential for designing & monitoring national policies) ◦ 2 -3 years time lag � Indicators based on Food consumption/nutritional outcomes: ◦ Indirect measurement of food insecurity, reflecting not only changes in the target variable (health, water/sanitation access) ◦ Sporadic surveys with incomplete country coverage ◦ 3 -5 years time lag ◦ Data collection difficult and costly
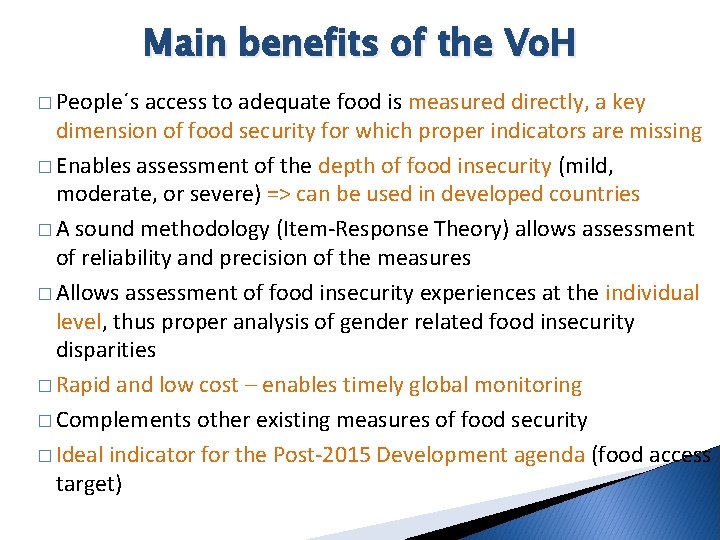
Main benefits of the Vo. H � People´s access to adequate food is measured directly, a key dimension of food security for which proper indicators are missing � Enables assessment of the depth of food insecurity (mild, moderate, or severe) => can be used in developed countries � A sound methodology (Item-Response Theory) allows assessment of reliability and precision of the measures � Allows assessment of food insecurity experiences at the individual level, thus proper analysis of gender related food insecurity disparities � Rapid and low cost – enables timely global monitoring � Complements other existing measures of food security � Ideal indicator for the Post-2015 Development agenda (food access target)
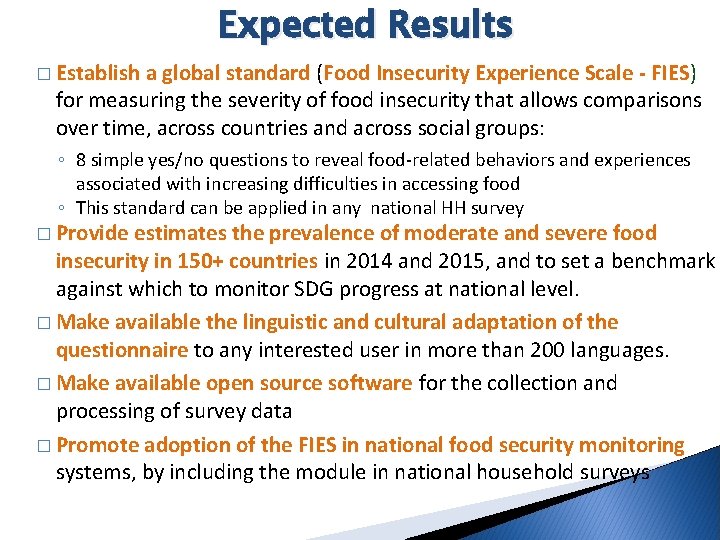
Expected Results � Establish a global standard (Food Insecurity Experience Scale - FIES) for measuring the severity of food insecurity that allows comparisons over time, across countries and across social groups: ◦ 8 simple yes/no questions to reveal food-related behaviors and experiences associated with increasing difficulties in accessing food ◦ This standard can be applied in any national HH survey � Provide estimates the prevalence of moderate and severe food insecurity in 150+ countries in 2014 and 2015, and to set a benchmark against which to monitor SDG progress at national level. � Make available the linguistic and cultural adaptation of the questionnaire to any interested user in more than 200 languages. � Make available open source software for the collection and processing of survey data � Promote adoption of the FIES in national food security monitoring systems, by including the module in national household surveys
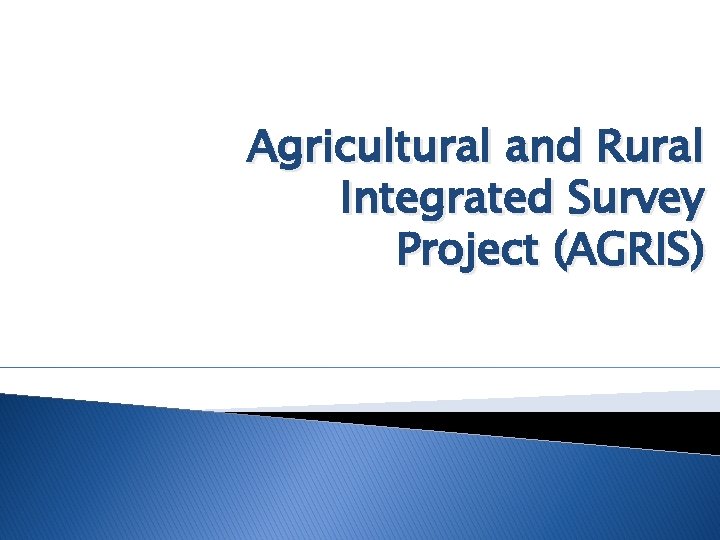
Agricultural and Rural Integrated Survey Project (AGRIS)
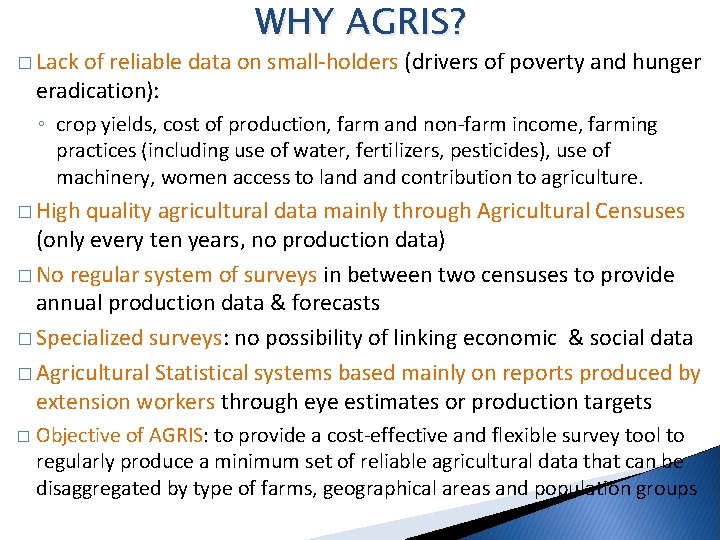
� Lack WHY AGRIS? of reliable data on small-holders (drivers of poverty and hunger eradication): ◦ crop yields, cost of production, farm and non-farm income, farming practices (including use of water, fertilizers, pesticides), use of machinery, women access to land contribution to agriculture. � High quality agricultural data mainly through Agricultural Censuses (only every ten years, no production data) � No regular system of surveys in between two censuses to provide annual production data & forecasts � Specialized surveys: no possibility of linking economic & social data � Agricultural Statistical systems based mainly on reports produced by extension workers through eye estimates or production targets � Objective of AGRIS: to provide a cost-effective and flexible survey tool to regularly produce a minimum set of reliable agricultural data that can be disaggregated by type of farms, geographical areas and population groups
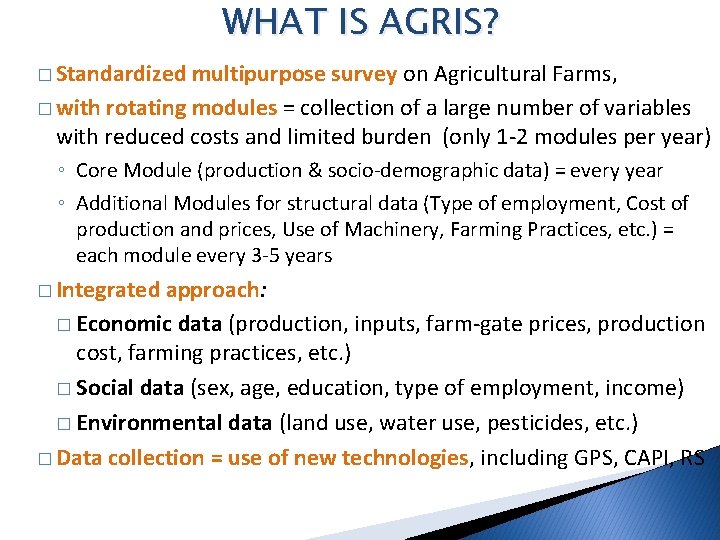
WHAT IS AGRIS? � Standardized multipurpose survey on Agricultural Farms, � with rotating modules = collection of a large number of variables with reduced costs and limited burden (only 1 -2 modules per year) ◦ Core Module (production & socio-demographic data) = every year ◦ Additional Modules for structural data (Type of employment, Cost of production and prices, Use of Machinery, Farming Practices, etc. ) = each module every 3 -5 years � Integrated approach: � Economic data (production, inputs, farm-gate prices, production cost, farming practices, etc. ) � Social data (sex, age, education, type of employment, income) � Environmental data (land use, water use, pesticides, etc. ) � Data collection = use of new technologies, including GPS, CAPI, RS
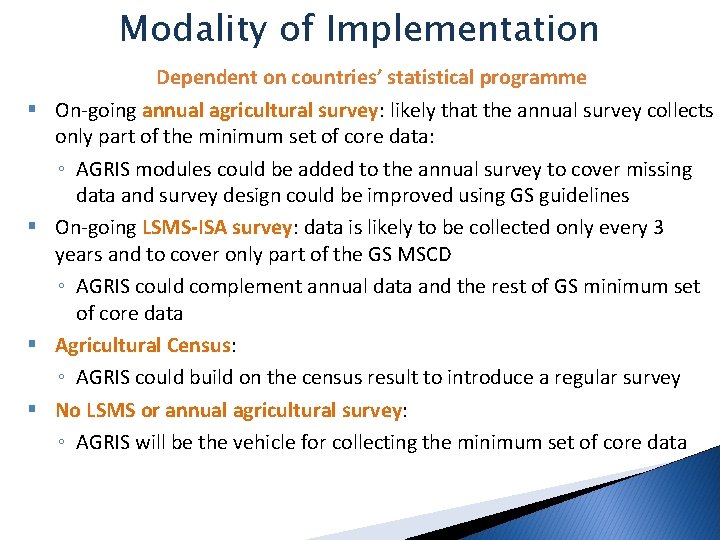
Modality of Implementation § § Dependent on countries’ statistical programme On-going annual agricultural survey: likely that the annual survey collects only part of the minimum set of core data: ◦ AGRIS modules could be added to the annual survey to cover missing data and survey design could be improved using GS guidelines On-going LSMS-ISA survey: data is likely to be collected only every 3 years and to cover only part of the GS MSCD ◦ AGRIS could complement annual data and the rest of GS minimum set of core data Agricultural Census: ◦ AGRIS could build on the census result to introduce a regular survey No LSMS or annual agricultural survey: ◦ AGRIS will be the vehicle for collecting the minimum set of core data
Lessons learned from king josiah
Lessons learned ground rules
Lessons not learned in blood
Change management lessons learned
Risk management lessons learned
Lessons learned repository
Pmbok lessons learned
What are the things we can learn from ants?
Claremont workday
Obadiah in the book of kings
Lessons learned purpose
Lessons learned suomeksi
Six sigma lessons learned
Lessons learned faa
Lessons in life of pi
Contest at mt carmel
Lessons learned from king josiah
Lesson learned in typhoon yolanda
Nerc lessons learned
Hyatt regency walkway collapse lessons learned
Tac lessons learned