Southern California Earthquake Center Materials for GTC DC
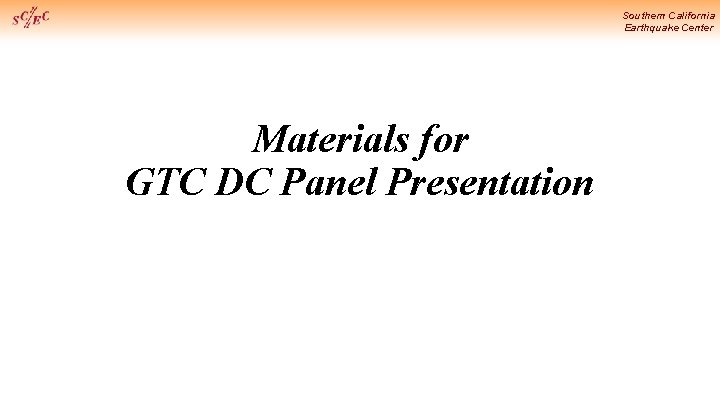
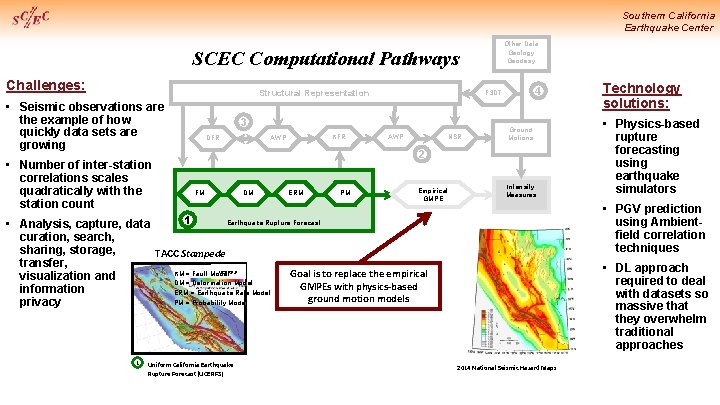
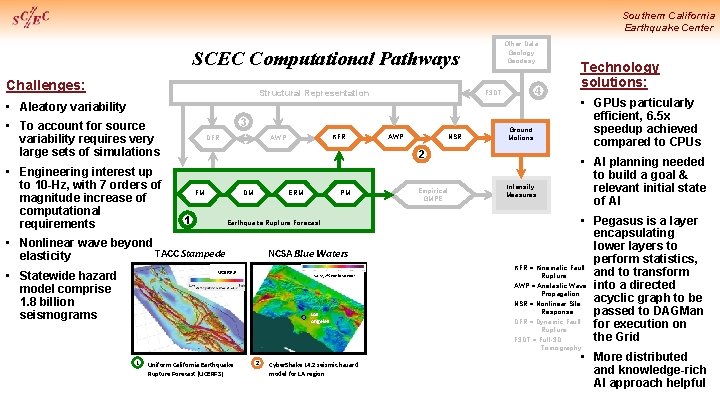
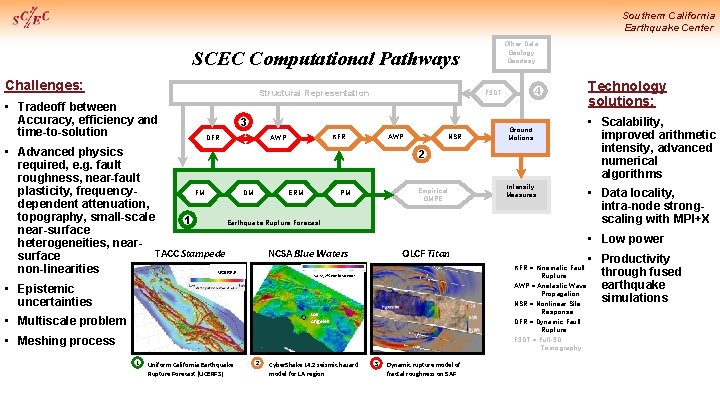
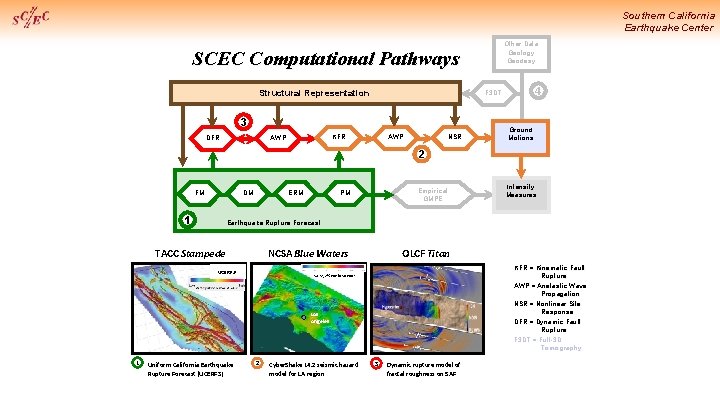
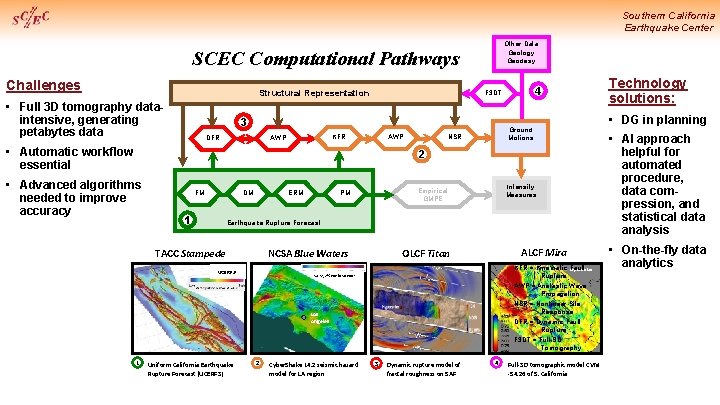
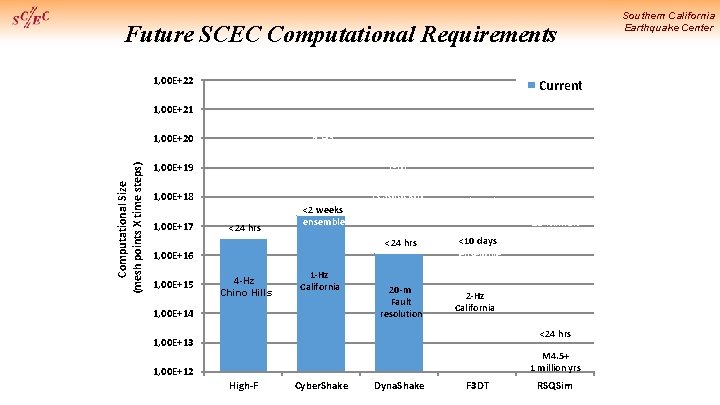
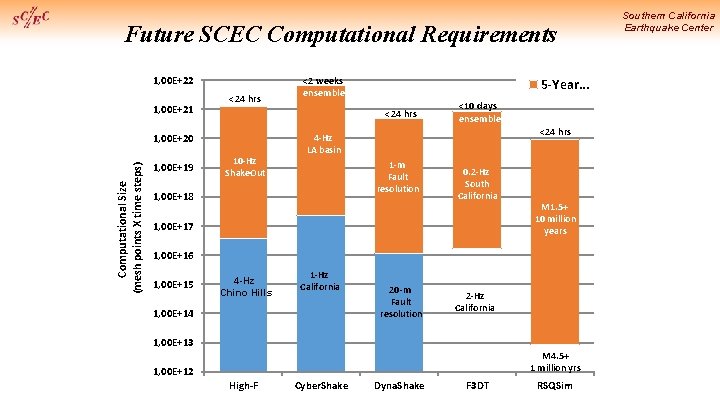
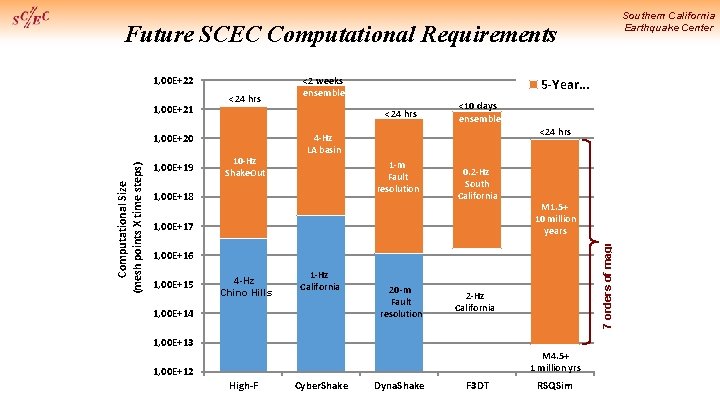
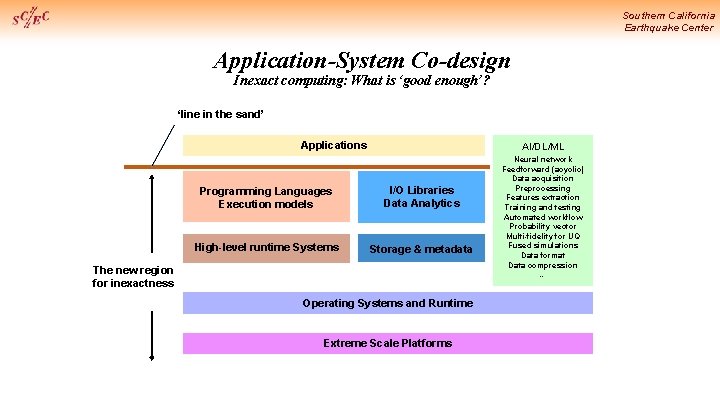
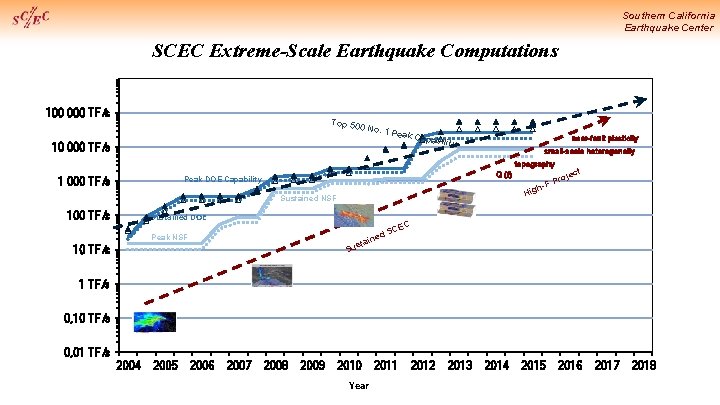
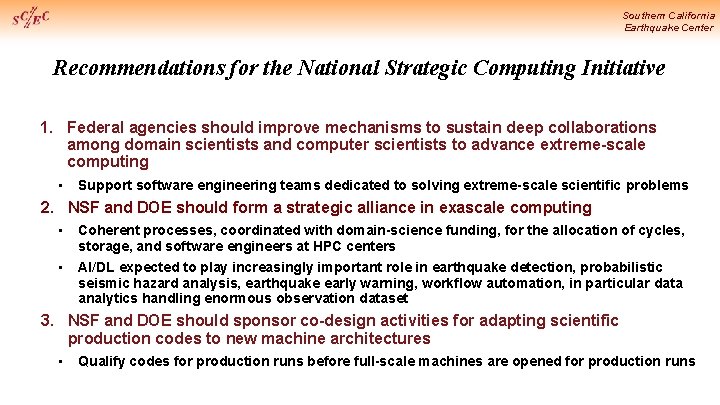
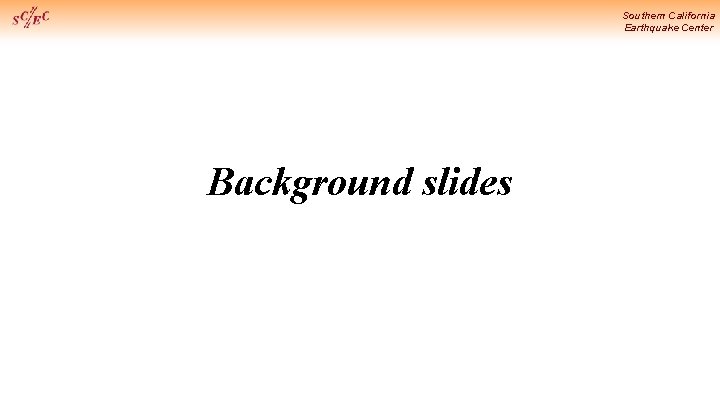
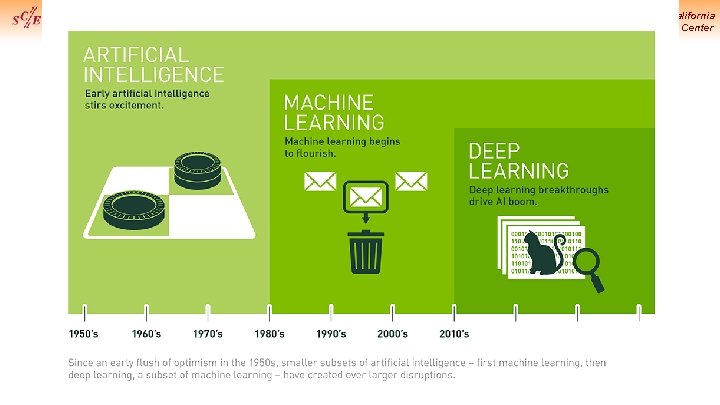
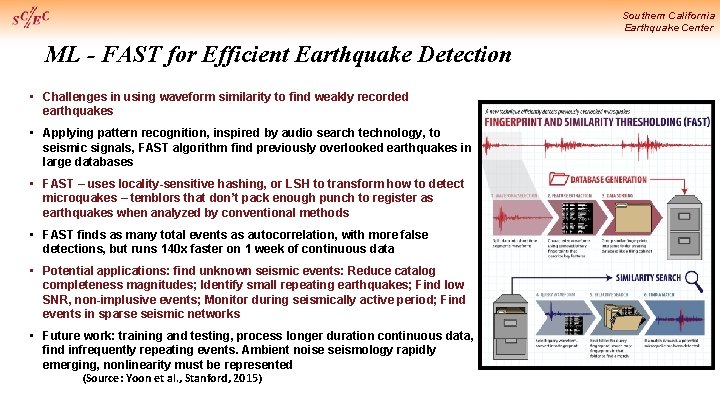
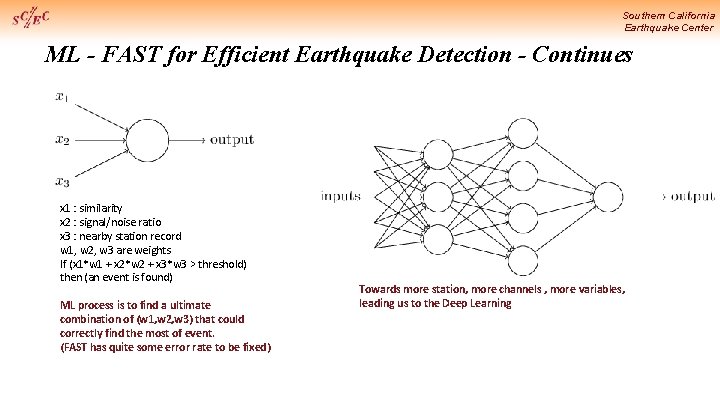
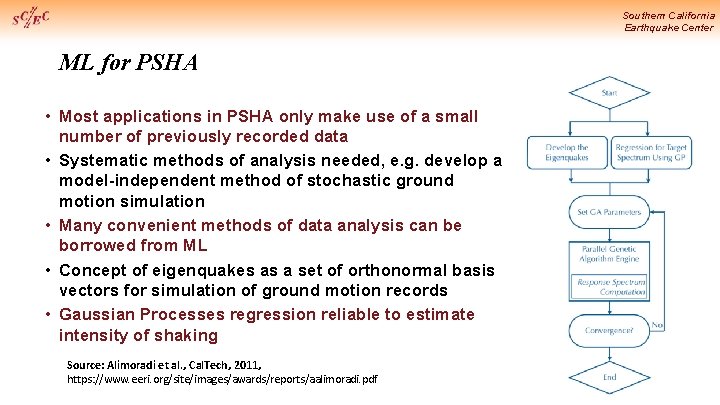
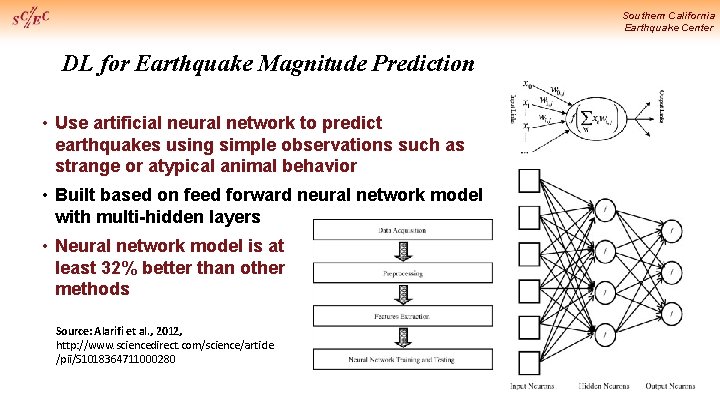
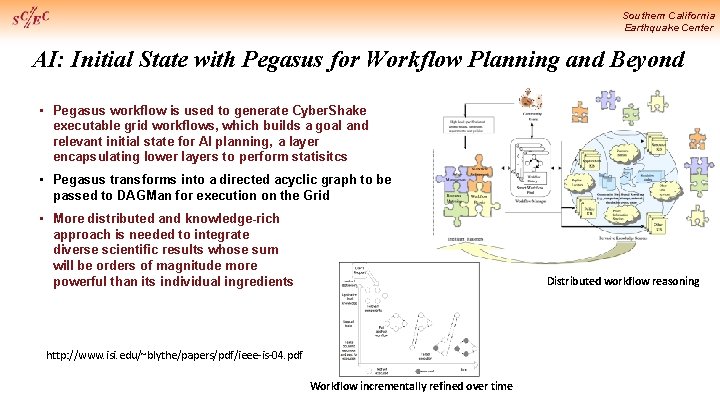
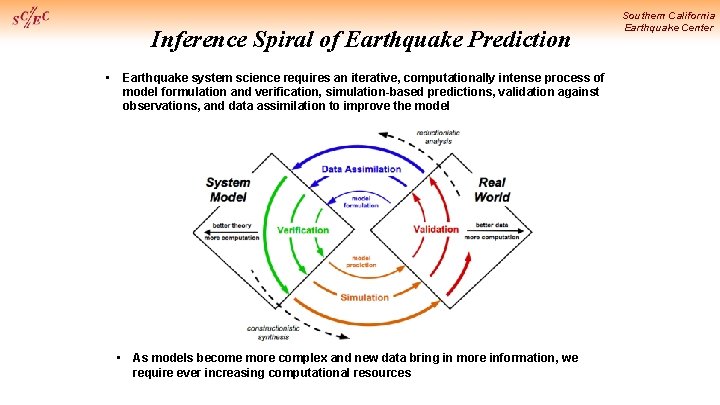
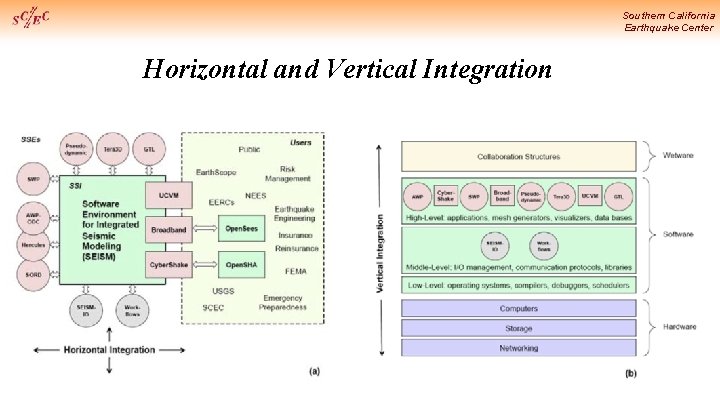
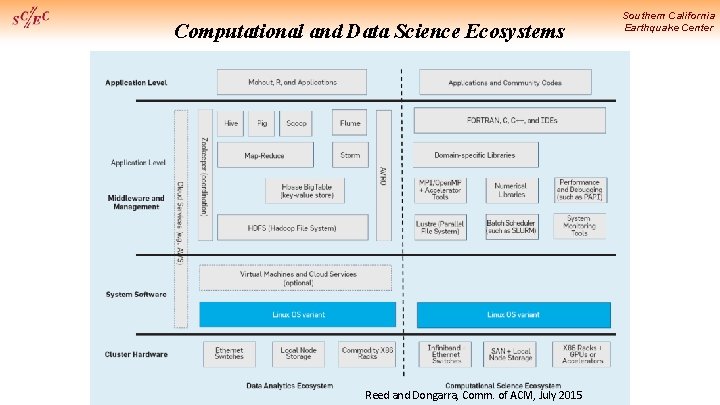
- Slides: 22
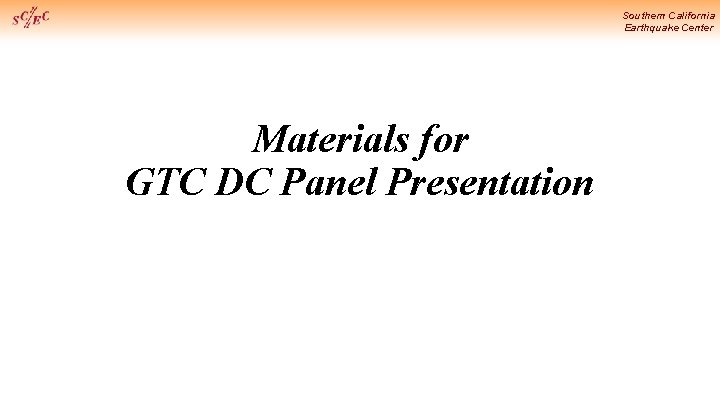
Southern California Earthquake Center Materials for GTC DC Panel Presentation
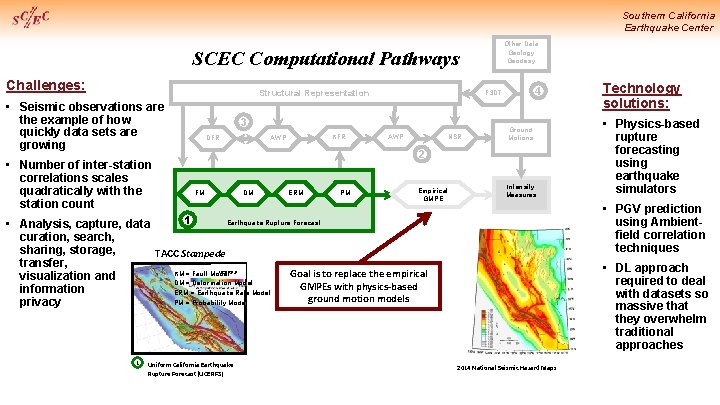
Southern California Earthquake Center Other Data Geology Geodesy SCEC Computational Pathways Challenges: Structural Representation • Seismic observations are the example of how quickly data sets are growing • Number of inter-station correlations scales quadratically with the station count 3 KFR AWP DFR 4 F 3 DT NSR AWP Ground Motions 2 FM DM ERM PM Empirical GMPE Intensity Measures Uniform California Earthquake Rupture Forecast (UCERF 3) • Physics-based rupture forecasting using earthquake simulators • PGV prediction using Ambientfield correlation techniques 1 Earthquake Rupture Forecast • Analysis, capture, data curation, search, sharing, storage, TACC Stampede transfer, UCERF 3 KM = Fault Model Goal is to replace the empirical visualization and DM = Deformation Model GMPEs with physics-based information ERM = Earthquake Rate Model ground motion models PM = Probability Model privacy 1 Technology solutions: • DL approach required to deal with datasets so massive that they overwhelm traditional approaches 2014 National Seismic Hazard Maps
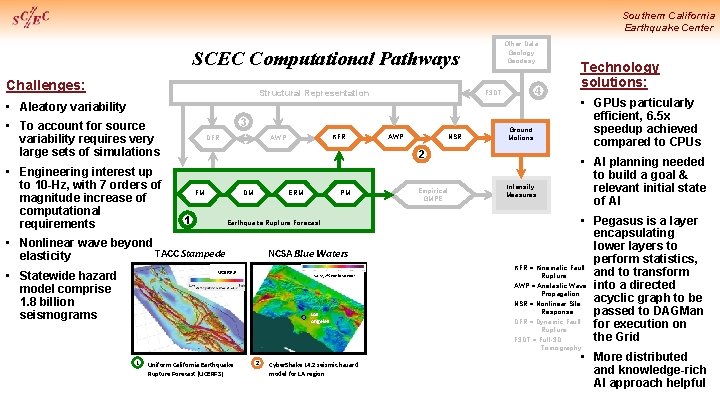
Southern California Earthquake Center Other Data Geology Geodesy SCEC Computational Pathways Challenges: Structural Representation 4 F 3 DT • Aleatory variability 3 • To account for source variability requires very large sets of simulations • Engineering interest up to 10 -Hz, with 7 orders of magnitude increase of computational requirements NSR AWP Ground Motions 2 FM DM 1 ERM PM Earthquake Rupture Forecast • Nonlinear wave beyond TACC Stampede elasticity NCSA Blue Waters UCERF 3 • Statewide hazard model comprise 1. 8 billion seismograms KFR AWP DFR SA-3 s, 2% Po. E in 50 years Los Angeles Empirical GMPE Intensity Measures Technology solutions: • GPUs particularly efficient, 6. 5 x speedup achieved compared to CPUs • AI planning needed to build a goal & relevant initial state of AI • Pegasus is a layer encapsulating lower layers to perform statistics, KFR = Kinematic Fault and to transform Rupture AWP = Anelastic Wave into a directed Propagation acyclic graph to be NSR = Nonlinear Site Response passed to DAGMan DFR = Dynamic Fault for execution on Rupture the Grid F 3 DT = Full-3 D Tomography 1 Uniform California Earthquake Rupture Forecast (UCERF 3) 2 Cyber. Shake 14. 2 seismic hazard model for LA region • More distributed and knowledge-rich AI approach helpful
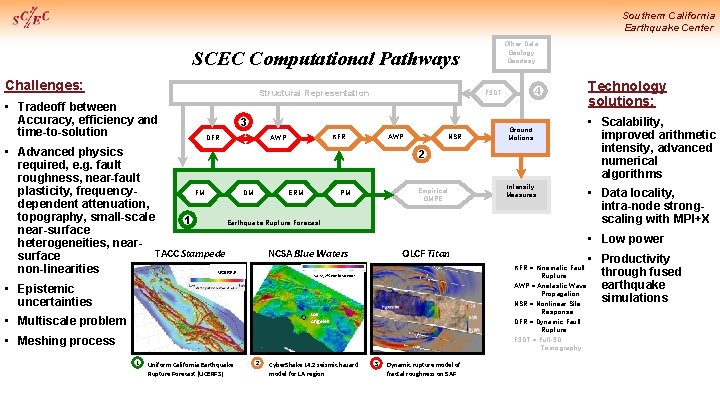
Southern California Earthquake Center Other Data Geology Geodesy SCEC Computational Pathways Challenges: Structural Representation • Tradeoff between Accuracy, efficiency and time-to-solution 3 NSR AWP KFR AWP DFR • Advanced physics required, e. g. fault roughness, near-fault plasticity, frequency. FM DM ERM PM dependent attenuation, topography, small-scale 1 Earthquake Rupture Forecast near-surface heterogeneities, near. NCSA Blue Waters TACC Stampede surface non-linearities UCERF 3 Empirical GMPE OLCF Titan KFR = Kinematic Fault Rupture AWP = Anelastic Wave Propagation NSR = Nonlinear Site Response DFR = Dynamic Fault Rupture F 3 DT = Full-3 D Tomography Los Angeles • Meshing process Uniform California Earthquake Rupture Forecast (UCERF 3) 2 Cyber. Shake 14. 2 seismic hazard model for LA region Intensity Measures Technology solutions: • Scalability, improved arithmetic intensity, advanced numerical algorithms • Data locality, intra-node strongscaling with MPI+X • Low power • Epistemic uncertainties 1 Ground Motions 2 SA-3 s, 2% Po. E in 50 years • Multiscale problem 4 F 3 DT 3 Dynamic rupture model of fractal roughness on SAF • Productivity through fused earthquake simulations
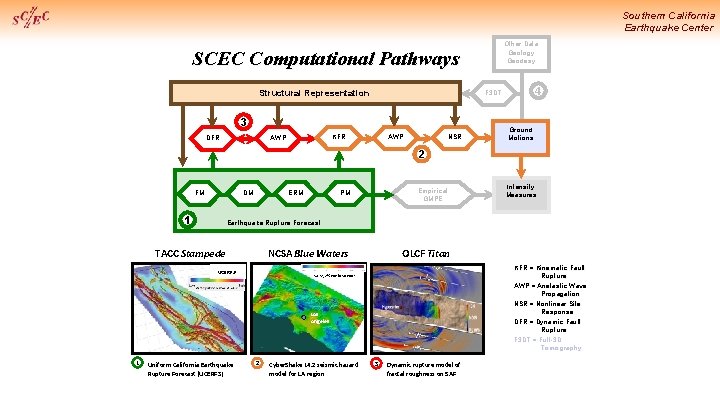
Southern California Earthquake Center Other Data Geology Geodesy SCEC Computational Pathways Structural Representation 3 NSR AWP KFR AWP DFR 4 F 3 DT Ground Motions 2 FM DM 1 PM Empirical GMPE NCSA Blue Waters OLCF Titan ERM Earthquake Rupture Forecast TACC Stampede UCERF 3 KFR = Kinematic Fault Rupture AWP = Anelastic Wave Propagation NSR = Nonlinear Site Response DFR = Dynamic Fault Rupture F 3 DT = Full-3 D Tomography SA-3 s, 2% Po. E in 50 years Los Angeles 1 Intensity Measures Uniform California Earthquake Rupture Forecast (UCERF 3) 2 Cyber. Shake 14. 2 seismic hazard model for LA region 3 Dynamic rupture model of fractal roughness on SAF
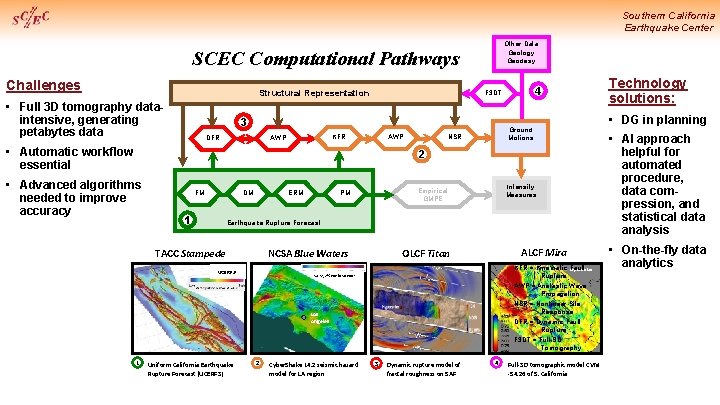
Southern California Earthquake Center Other Data Geology Geodesy SCEC Computational Pathways Challenges Structural Representation • Full 3 D tomography dataintensive, generating petabytes data 3 • Automatic workflow essential Ground Motions NSR AWP KFR AWP DFR 4 F 3 DT 2 • Advanced algorithms needed to improve accuracy FM DM 1 PM Empirical GMPE NCSA Blue Waters OLCF Titan ERM Intensity Measures Earthquake Rupture Forecast TACC Stampede UCERF 3 ALCF Mira KFR = Kinematic Fault depth = 6 km Rupture AWP = Anelastic Wave Propagation NSR = Nonlinear Site Response DFR = Dynamic Fault Rupture F 3 DT = Full-3 D Tomography SA-3 s, 2% Po. E in 50 years Los Angeles 1 Uniform California Earthquake Rupture Forecast (UCERF 3) 2 Cyber. Shake 14. 2 seismic hazard model for LA region 3 Dynamic rupture model of fractal roughness on SAF 4 Full-3 D tomographic model CVM -S 4. 26 of S. California Technology solutions: • DG in planning • AI approach helpful for automated procedure, data compression, and statistical data analysis • On-the-fly data analytics
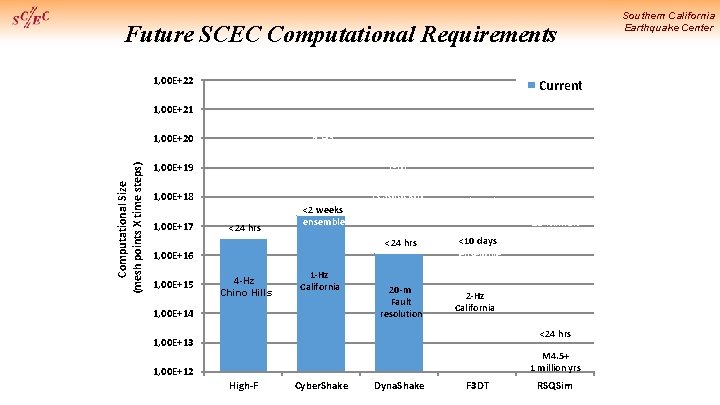
Future SCEC Computational Requirements 1, 00 E+22 Current 1, 00 E+21 Computational Size (mesh points X time steps) 1, 00 E+20 1, 00 E+19 10 -Hz Shake. Out 1, 00 E+18 1, 00 E+17 <24 hrs 4 -Hz LA basin <2 weeks ensemble <24 hrs 1, 00 E+16 1, 00 E+15 1 -m Fault resolution 4 -Hz Chino Hills 1 -Hz California 1, 00 E+14 20 -m Fault resolution 0. 2 -Hz South California <10 days ensemble M 1. 5+ 10 million years 2 -Hz California <24 hrs 1, 00 E+13 M 4. 5+ 1 million yrs 1, 00 E+12 High-F Cyber. Shake Dyna. Shake F 3 DT RSQSim Southern California Earthquake Center
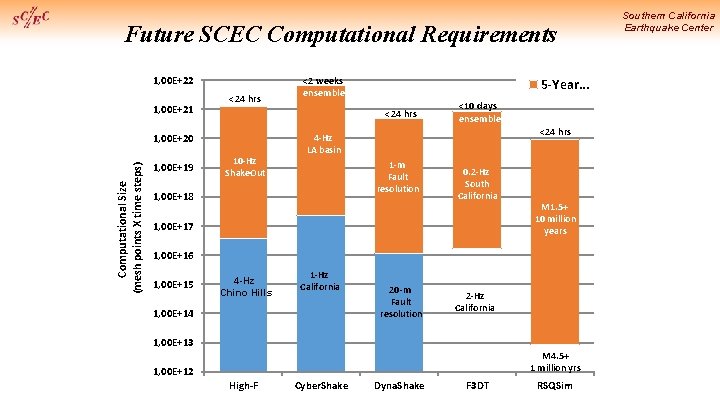
Future SCEC Computational Requirements 1, 00 E+22 1, 00 E+21 <24 hrs Computational Size (mesh points X time steps) 5 -Year. . . <24 hrs 1, 00 E+20 1, 00 E+19 <2 weeks ensemble 10 -Hz Shake. Out <10 days ensemble <24 hrs 4 -Hz LA basin 1 -m Fault resolution 1, 00 E+18 0. 2 -Hz South California 1, 00 E+17 M 1. 5+ 10 million years 1, 00 E+16 1, 00 E+15 4 -Hz Chino Hills 1 -Hz California 1, 00 E+14 20 -m Fault resolution 2 -Hz California 1, 00 E+13 M 4. 5+ 1 million yrs 1, 00 E+12 High-F Cyber. Shake Dyna. Shake F 3 DT RSQSim Southern California Earthquake Center
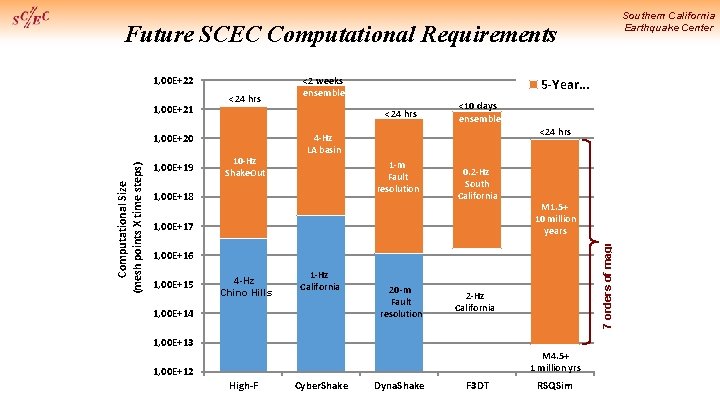
Southern California Earthquake Center 1, 00 E+22 1, 00 E+21 <24 hrs Computational Size (mesh points X time steps) 5 -Year. . . <24 hrs 1, 00 E+20 1, 00 E+19 <2 weeks ensemble 10 -Hz Shake. Out <10 days ensemble <24 hrs 4 -Hz LA basin 1 -m Fault resolution 1, 00 E+18 0. 2 -Hz South California 1, 00 E+17 M 1. 5+ 10 million years 1, 00 E+16 1, 00 E+15 4 -Hz Chino Hills 1 -Hz California 1, 00 E+14 20 -m Fault resolution 2 -Hz California 1, 00 E+13 M 4. 5+ 1 million yrs 1, 00 E+12 High-F Cyber. Shake Dyna. Shake F 3 DT RSQSim 7 orders of magnitude more computation required Future SCEC Computational Requirements
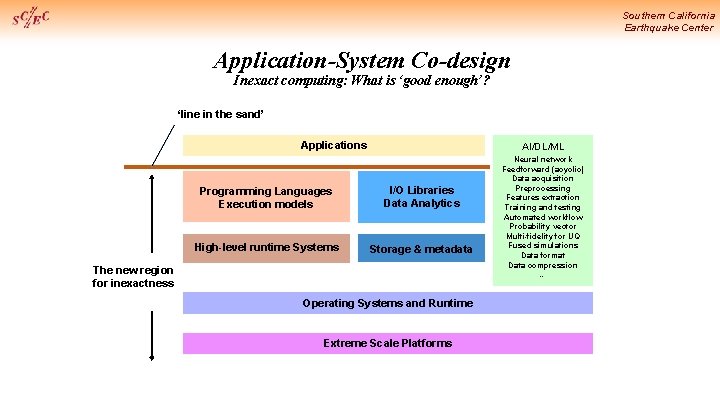
Southern California Earthquake Center Application-System Co-design Inexact computing: What is ‘good enough’? ‘line in the sand’ Applications AI/DL/ML Programming Languages Execution models I/O Libraries Data Analytics High-level runtime Systems Storage & metadata The new region for inexactness Operating Systems and Runtime Extreme Scale Platforms Neural network Feedforward (acyclic) Data acquisition Preprocessing Features extraction Training and testing Automated workflow Probability vector Multi-fidelity for UQ Fused simulations Data format Data compression …
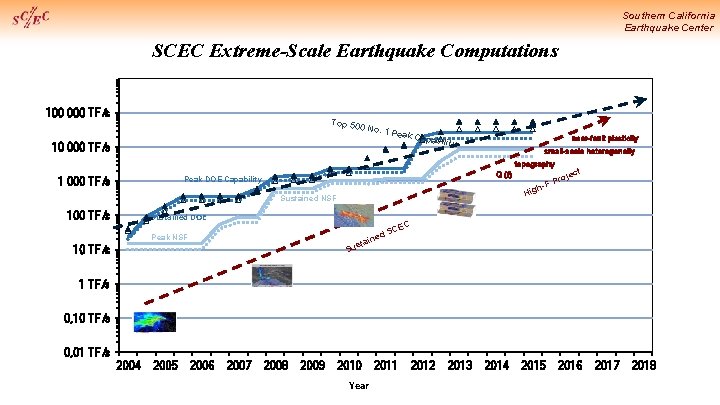
Southern California Earthquake Center SCEC Extreme-Scale Earthquake Computations 100 000 TF/s Top 50 0 No. 1 10 000 TF/s Peak C apabil near-fault plasticity small-scale heterogeneity topography Peak DOE Capability 1 000 TF/s Hig Sustained NSF 100 TF/s ct je Pro h-F Q (f) Sustained DOE C CE d. S e tain Peak NSF Sus 10 TF/s 1 TF/s 0, 10 TF/s 0, 01 TF/s 2004 2005 2006 2007 2008 2009 2010 Year 2011 2012 2013 2014 2015 2016 2017 2018
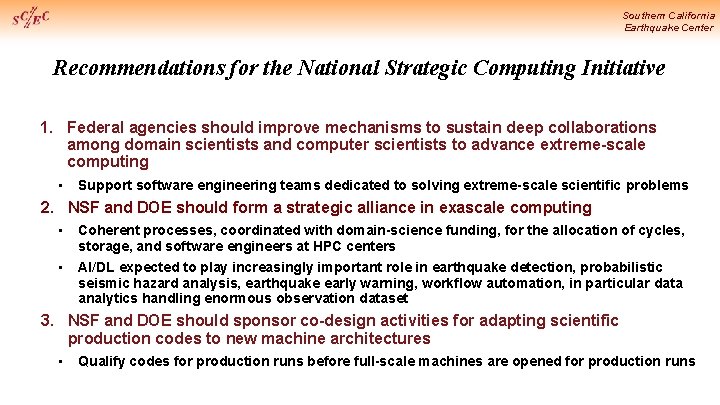
Southern California Earthquake Center Recommendations for the National Strategic Computing Initiative 1. Federal agencies should improve mechanisms to sustain deep collaborations among domain scientists and computer scientists to advance extreme-scale computing • Support software engineering teams dedicated to solving extreme-scale scientific problems 2. NSF and DOE should form a strategic alliance in exascale computing • Coherent processes, coordinated with domain-science funding, for the allocation of cycles, storage, and software engineers at HPC centers • AI/DL expected to play increasingly important role in earthquake detection, probabilistic seismic hazard analysis, earthquake early warning, workflow automation, in particular data analytics handling enormous observation dataset 3. NSF and DOE should sponsor co-design activities for adapting scientific production codes to new machine architectures • Qualify codes for production runs before full-scale machines are opened for production runs
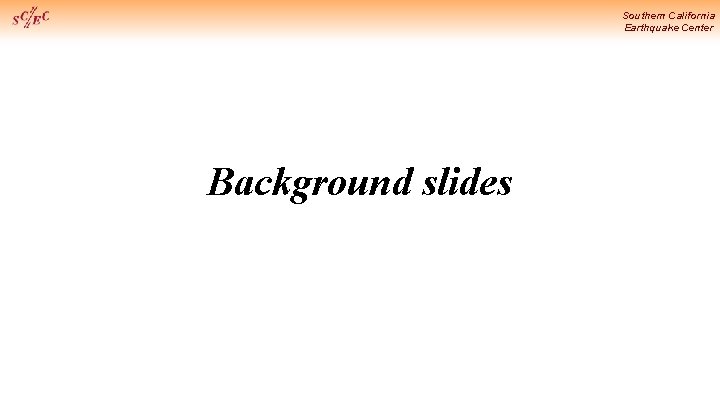
Southern California Earthquake Center Background slides
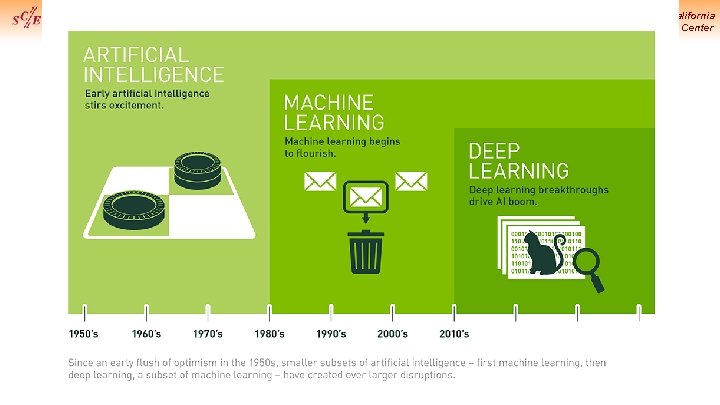
Southern California Earthquake Center
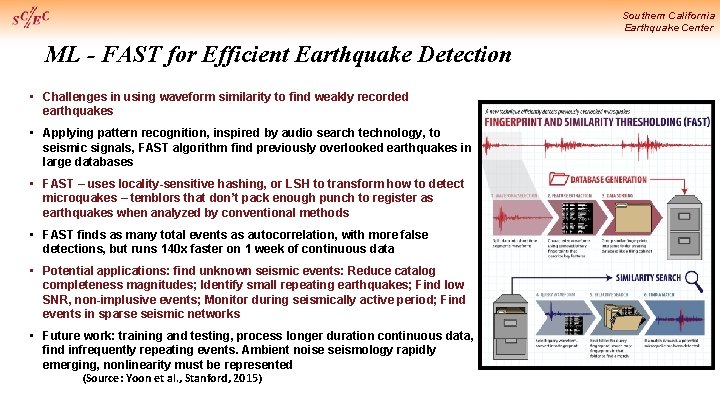
Southern California Earthquake Center ML - FAST for Efficient Earthquake Detection • Challenges in using waveform similarity to find weakly recorded earthquakes • Applying pattern recognition, inspired by audio search technology, to seismic signals, FAST algorithm find previously overlooked earthquakes in large databases • FAST – uses locality-sensitive hashing, or LSH to transform how to detect microquakes – temblors that don’t pack enough punch to register as earthquakes when analyzed by conventional methods • FAST finds as many total events as autocorrelation, with more false detections, but runs 140 x faster on 1 week of continuous data • Potential applications: find unknown seismic events: Reduce catalog completeness magnitudes; Identify small repeating earthquakes; Find low SNR, non-implusive events; Monitor during seismically active period; Find events in sparse seismic networks • Future work: training and testing, process longer duration continuous data, find infrequently repeating events. Ambient noise seismology rapidly emerging, nonlinearity must be represented (Source: Yoon et al. , Stanford, 2015)
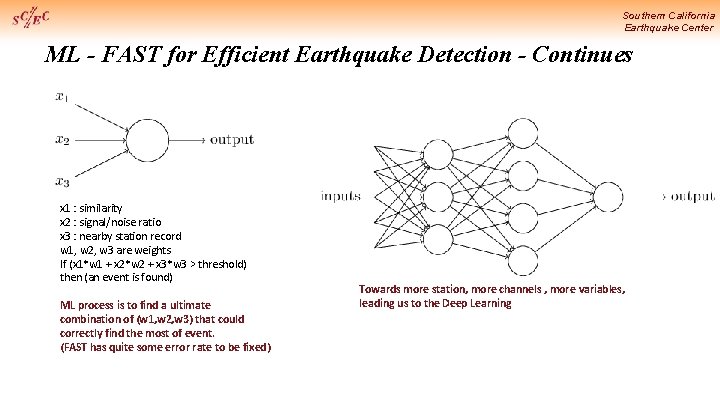
Southern California Earthquake Center ML - FAST for Efficient Earthquake Detection - Continues x 1 : similarity x 2 : signal/noise ratio x 3 : nearby station record w 1, w 2, w 3 are weights If (x 1*w 1 + x 2*w 2 + x 3*w 3 > threshold) then (an event is found) ML process is to find a ultimate combination of (w 1, w 2, w 3) that could correctly find the most of event. (FAST has quite some error rate to be fixed) Towards more station, more channels , more variables, leading us to the Deep Learning
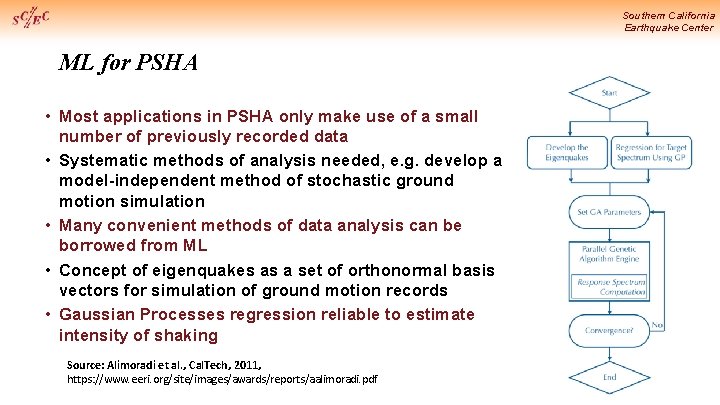
Southern California Earthquake Center ML for PSHA • Most applications in PSHA only make use of a small number of previously recorded data • Systematic methods of analysis needed, e. g. develop a model-independent method of stochastic ground motion simulation • Many convenient methods of data analysis can be borrowed from ML • Concept of eigenquakes as a set of orthonormal basis vectors for simulation of ground motion records • Gaussian Processes regression reliable to estimate intensity of shaking Source: Alimoradi et al. , Cal. Tech, 2011, https: //www. eeri. org/site/images/awards/reports/aalimoradi. pdf
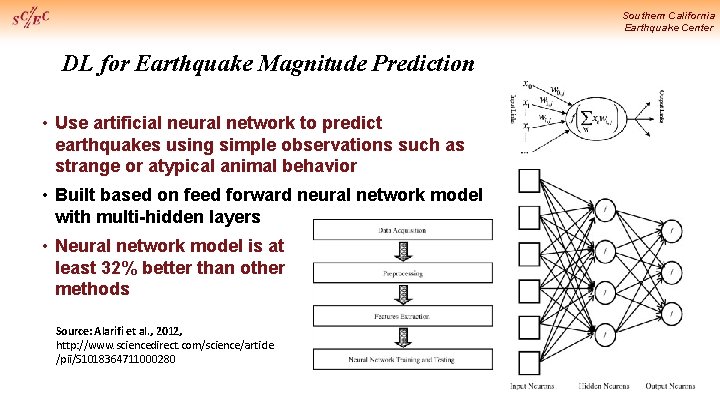
Southern California Earthquake Center DL for Earthquake Magnitude Prediction • Use artificial neural network to predict earthquakes using simple observations such as strange or atypical animal behavior • Built based on feed forward neural network model with multi-hidden layers • Neural network model is at least 32% better than other methods Source: Alarifi et al. , 2012, http: //www. sciencedirect. com/science/article /pii/S 1018364711000280
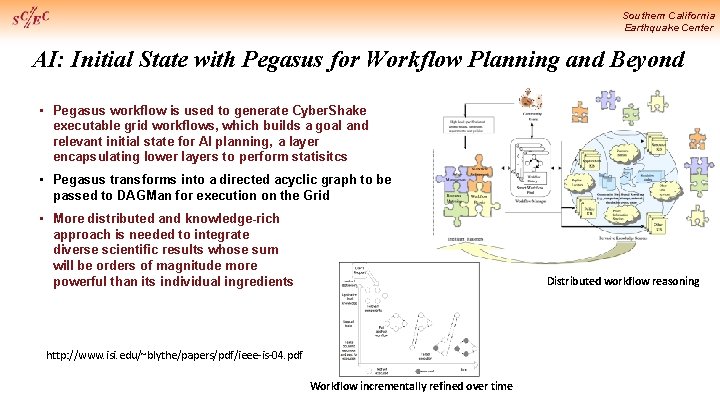
Southern California Earthquake Center AI: Initial State with Pegasus for Workflow Planning and Beyond • Pegasus workflow is used to generate Cyber. Shake executable grid workflows, which builds a goal and relevant initial state for AI planning, a layer encapsulating lower layers to perform statisitcs • Pegasus transforms into a directed acyclic graph to be passed to DAGMan for execution on the Grid • More distributed and knowledge-rich approach is needed to integrate diverse scientific results whose sum will be orders of magnitude more powerful than its individual ingredients Distributed workflow reasoning http: //www. isi. edu/~blythe/papers/pdf/ieee-is-04. pdf Workflow incrementally refined over time
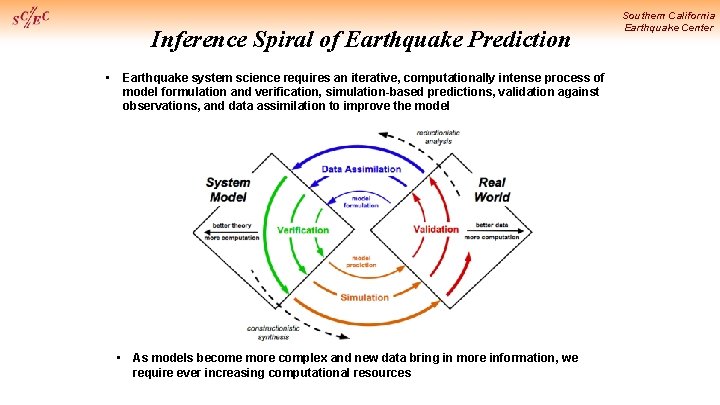
Inference Spiral of Earthquake Prediction • Earthquake system science requires an iterative, computationally intense process of model formulation and verification, simulation-based predictions, validation against observations, and data assimilation to improve the model • As models become more complex and new data bring in more information, we require ever increasing computational resources Southern California Earthquake Center
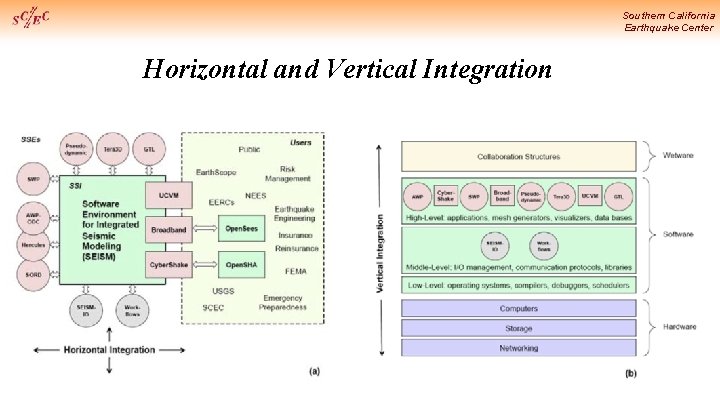
Southern California Earthquake Center Horizontal and Vertical Integration
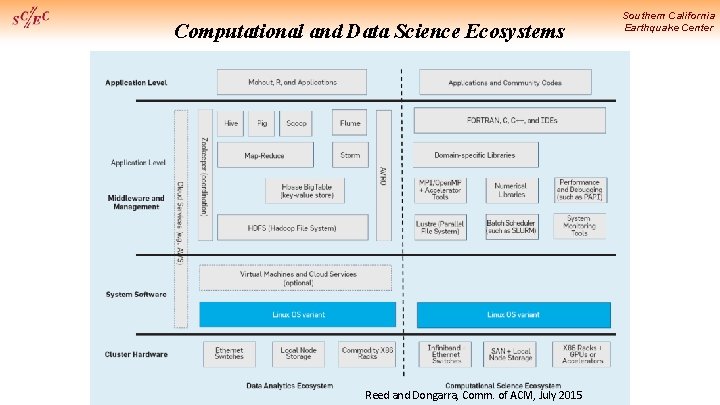
Computational and Data Science Ecosystems Reed and Dongarra, Comm. of ACM, July 2015 Southern California Earthquake Center
Open cures
What is this
Map of the california missions
Cmaa southern california
Marine exchange los angeles
University of southern california
Usc undergraduate symposium
Protein modeling scioly
"automobile club of southern california"
Age cka
Sc acis
Jain center of northern california
California service center laguna niguel
Cant stop the feeling trolls go noodle
Household materials useful
Natural man made
What is adopting materials
Direct materials budget with multiple materials
Cuadro de peligros gtc 45
Certificado norma gtc 185
Gtc 45 actualizada 2021
Rtm
Gtc definition