Smart Virtual Power Plants for the Future Electric
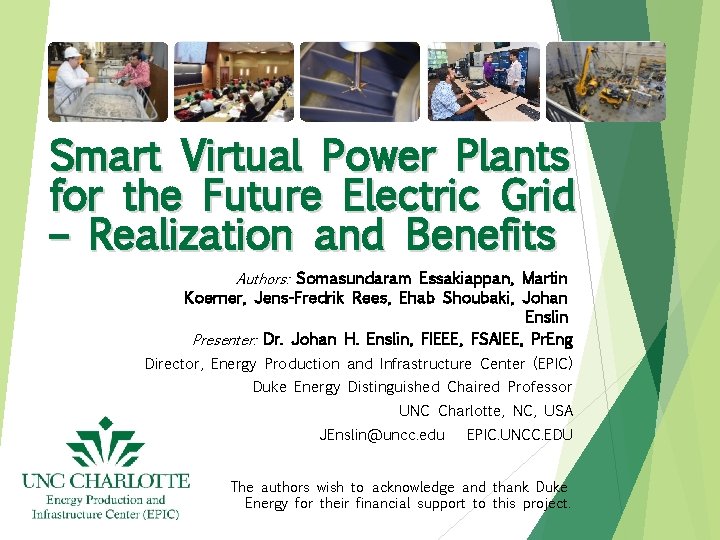
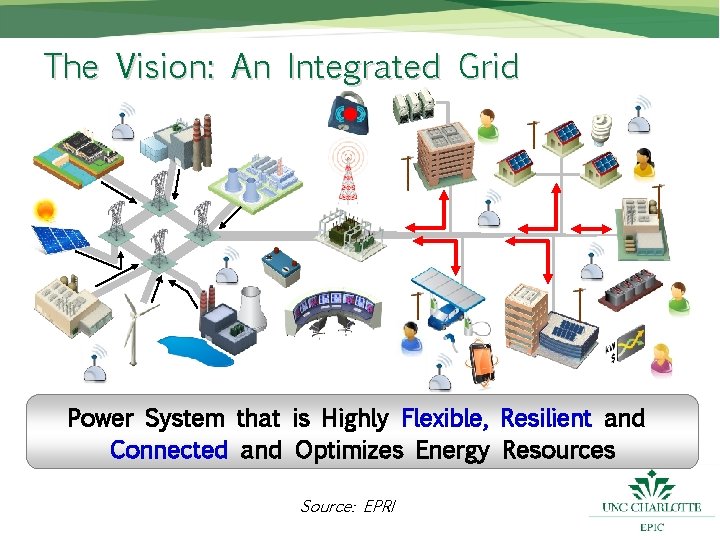
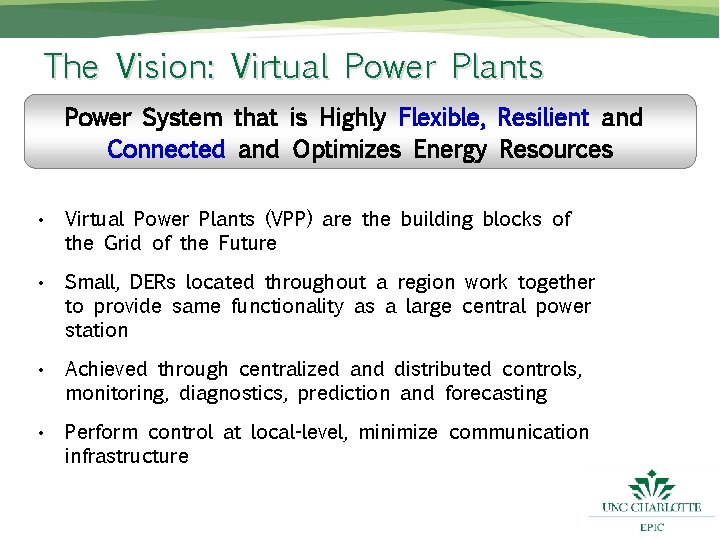
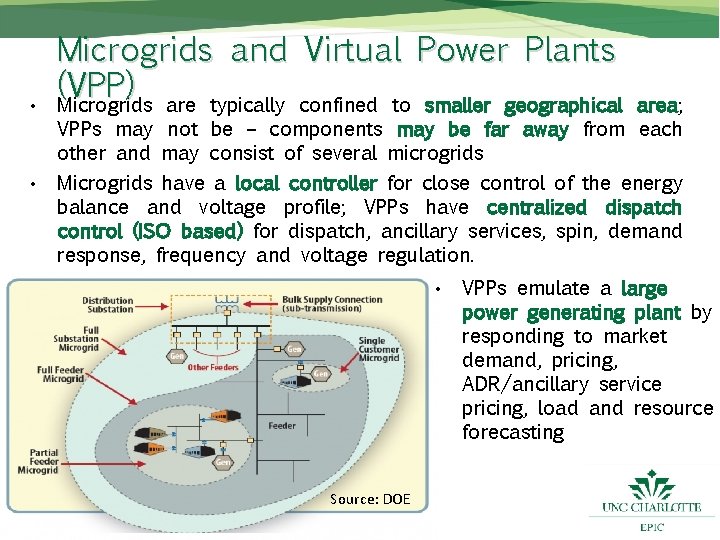
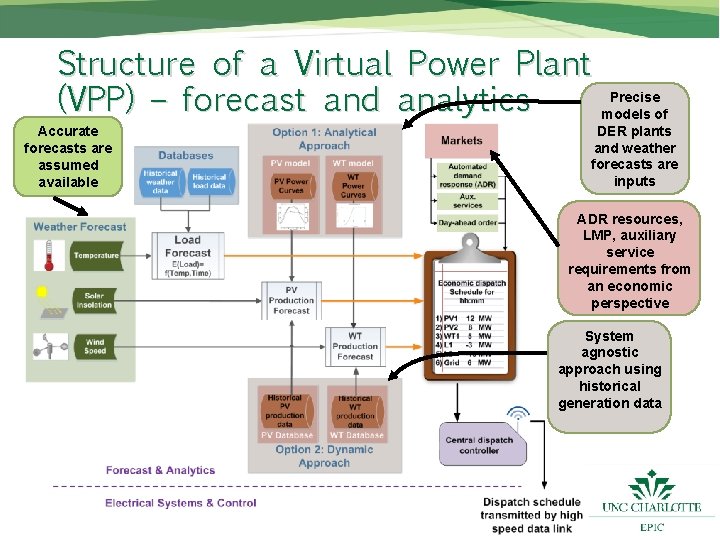
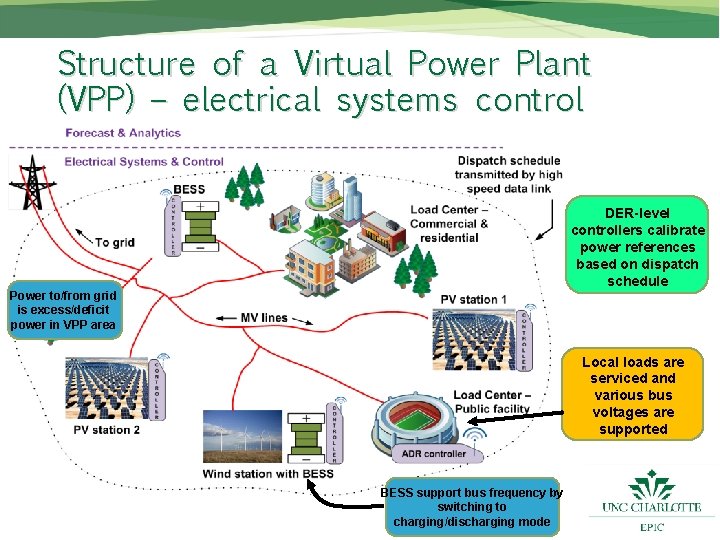
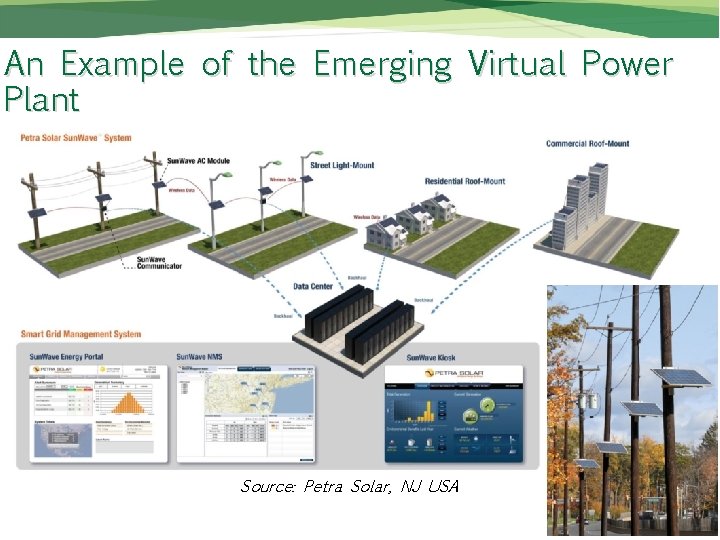
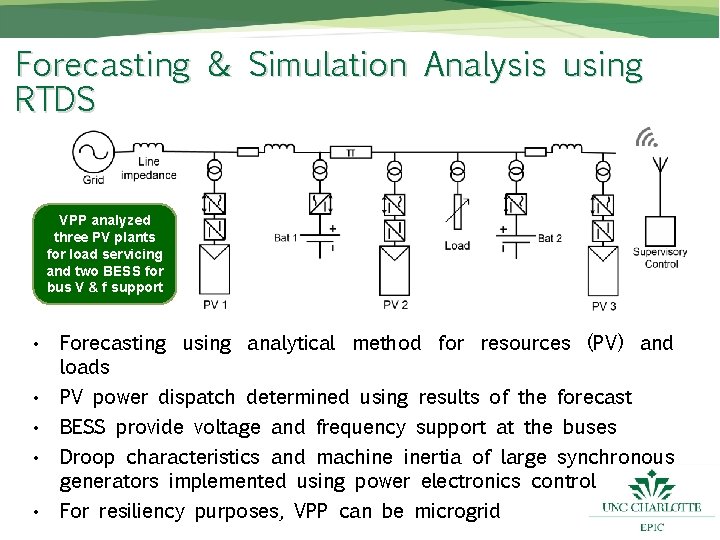
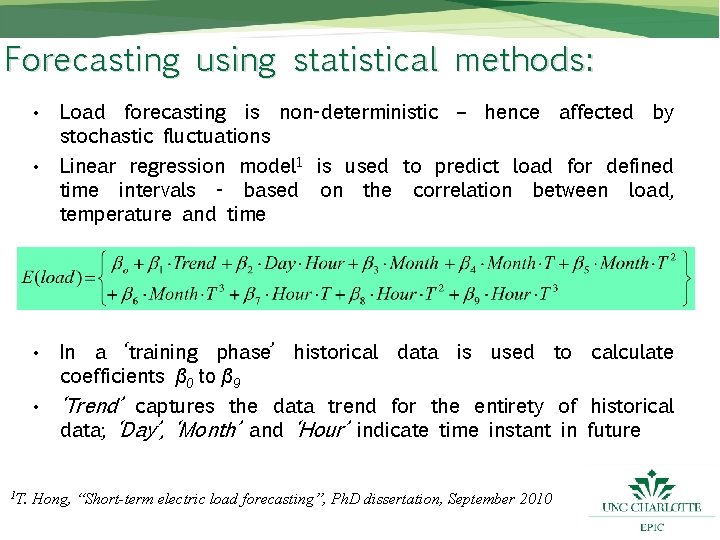
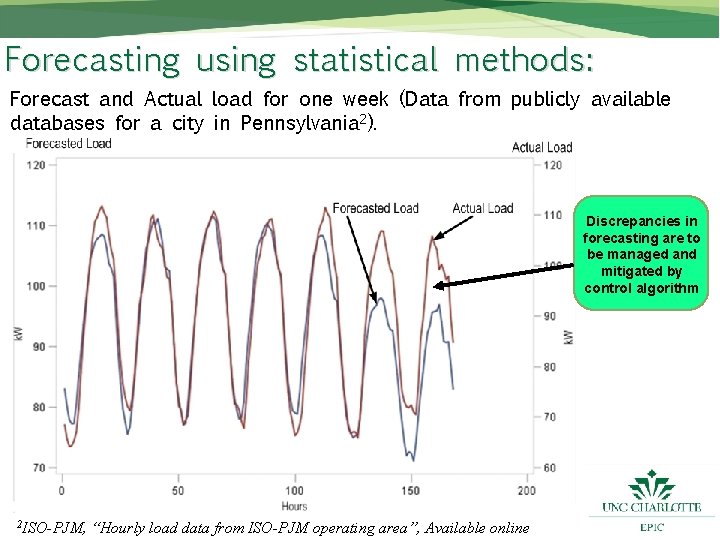
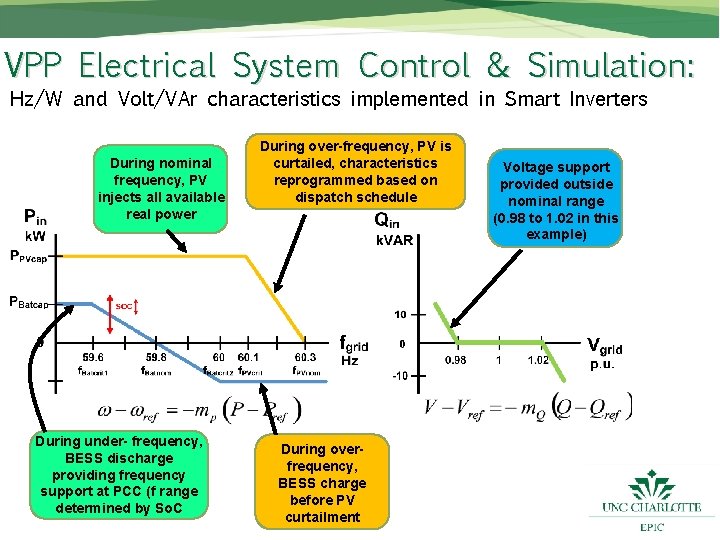
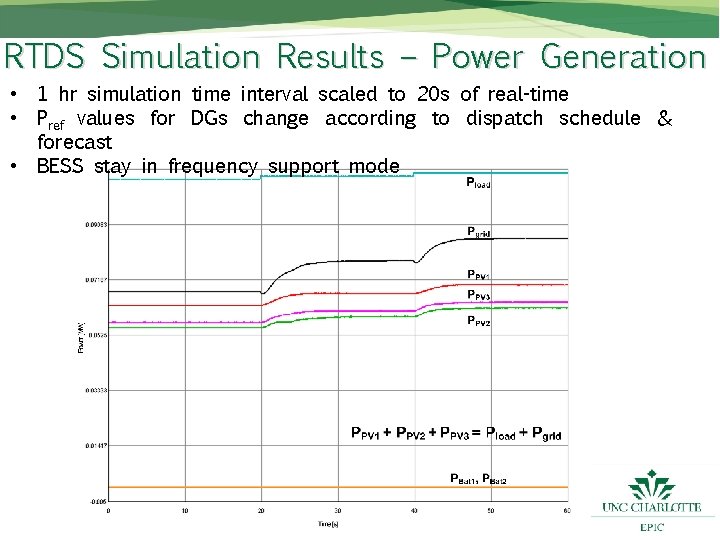
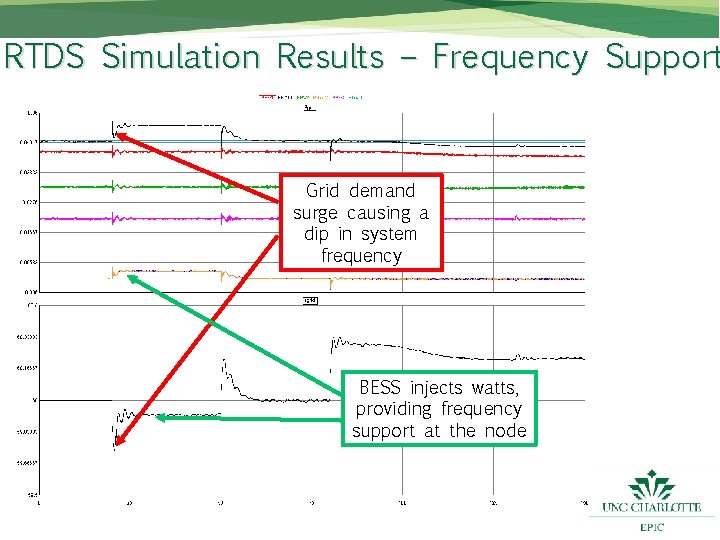
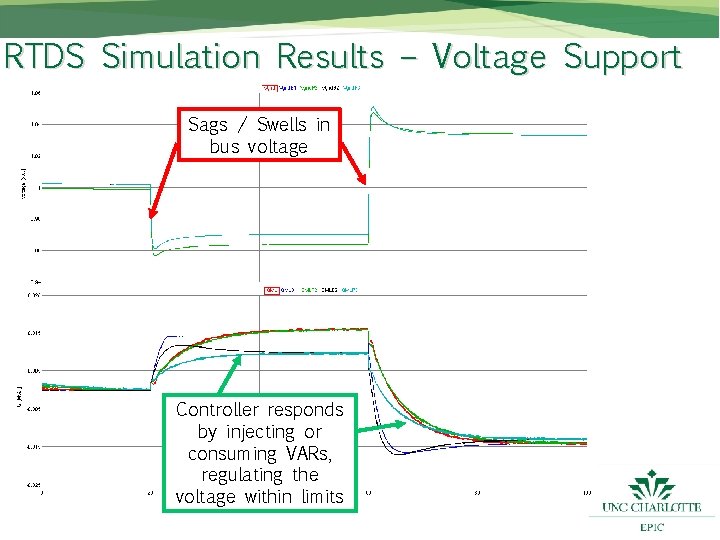
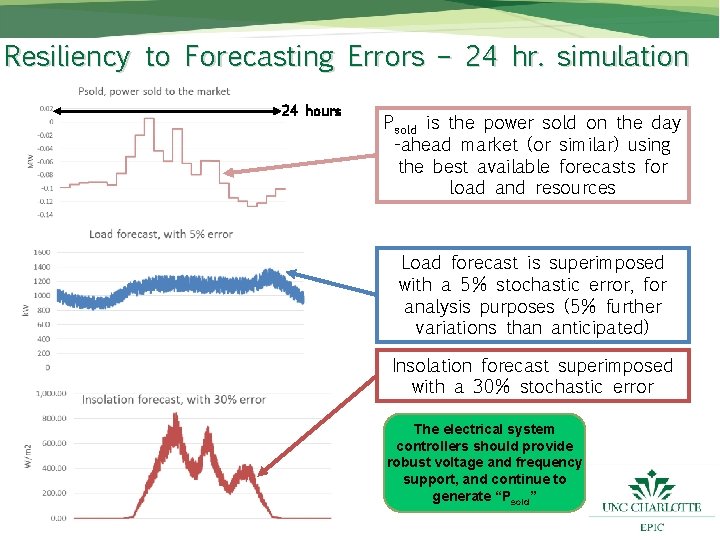
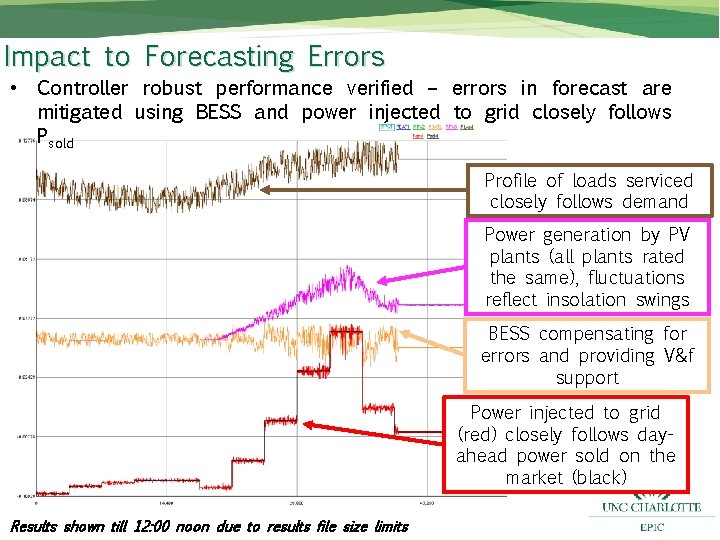
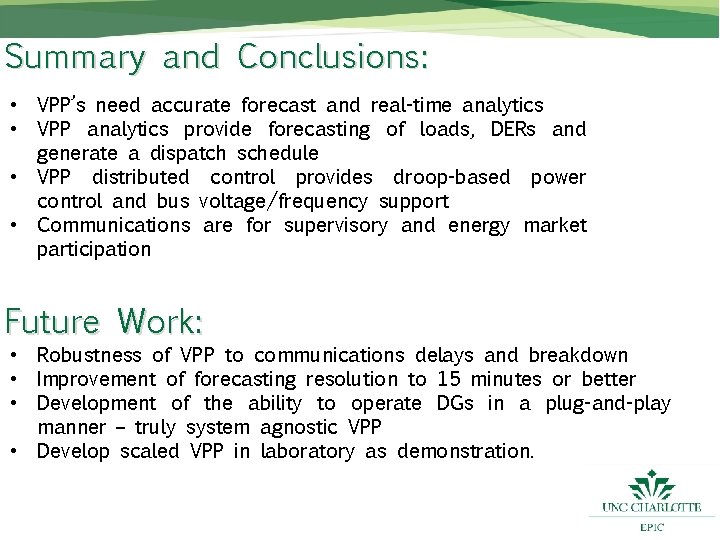
- Slides: 17
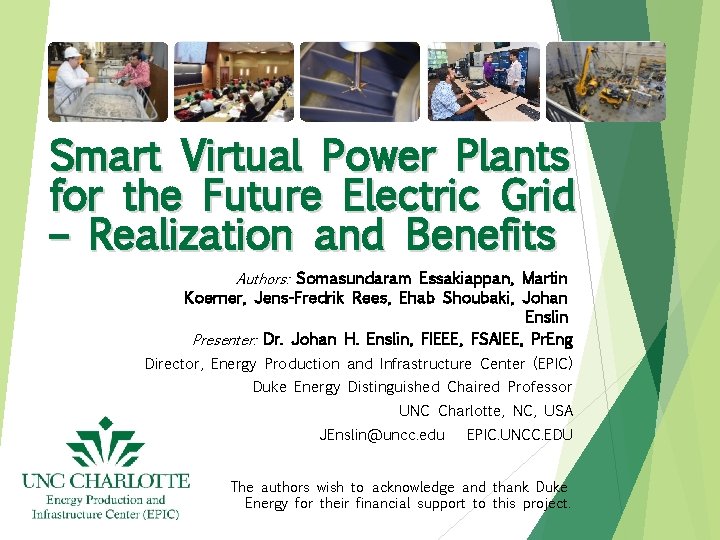
Smart Virtual Power Plants for the Future Electric Grid – Realization and Benefits Authors: Somasundaram Essakiappan, Martin Koerner, Jens-Fredrik Rees, Ehab Shoubaki, Johan Enslin Presenter: Dr. Johan H. Enslin, FIEEE, FSAIEE, Pr. Eng Director, Energy Production and Infrastructure Center (EPIC) Duke Energy Distinguished Chaired Professor UNC Charlotte, NC, USA JEnslin@uncc. edu EPIC. UNCC. EDU The authors wish to acknowledge and thank Duke Energy for their financial support to this project.
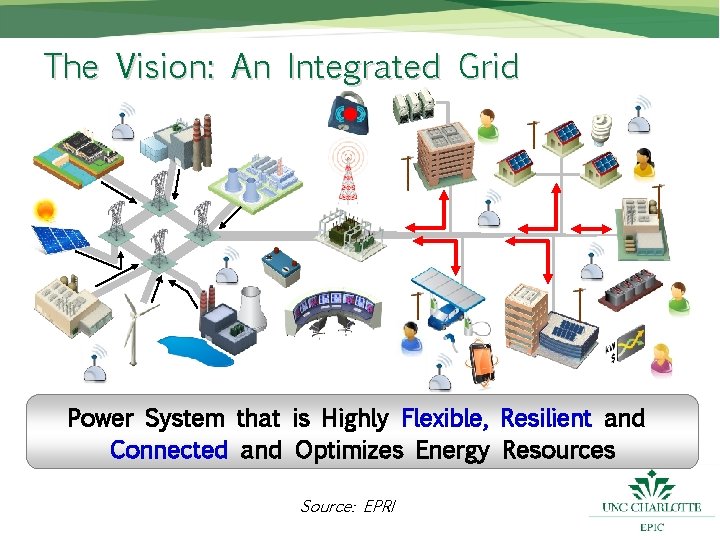
The Vision: An Integrated Grid Power System that is Highly Flexible, Resilient and Connected and Optimizes Energy Resources Source: EPRI
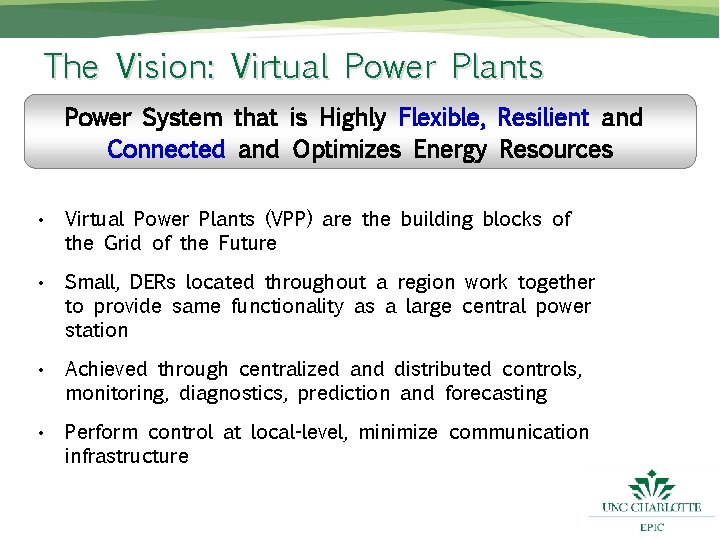
The Vision: Virtual Power Plants Power System that is Highly Flexible, Resilient and Connected and Optimizes Energy Resources • Virtual Power Plants (VPP) are the building blocks of the Grid of the Future • Small, DERs located throughout a region work together to provide same functionality as a large central power station • Achieved through centralized and distributed controls, monitoring, diagnostics, prediction and forecasting • Perform control at local-level, minimize communication infrastructure
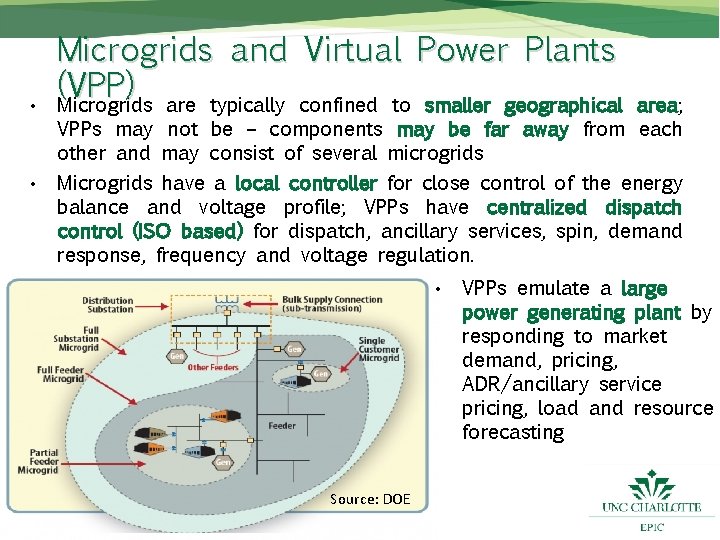
• • Microgrids and Virtual Power Plants (VPP) Microgrids are typically confined to smaller geographical area; VPPs may not be – components may be far away from each other and may consist of several microgrids Microgrids have a local controller for close control of the energy balance and voltage profile; VPPs have centralized dispatch control (ISO based) for dispatch, ancillary services, spin, demand response, frequency and voltage regulation. • Source: DOE VPPs emulate a large power generating plant by responding to market demand, pricing, ADR/ancillary service pricing, load and resource forecasting
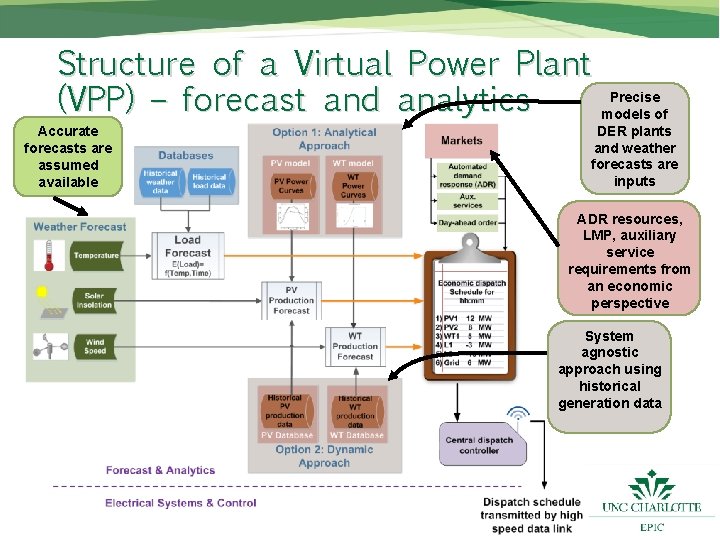
Structure of a Virtual Power Plant Precise (VPP) – forecast and analytics models of Accurate forecasts are assumed available DER plants and weather forecasts are inputs ADR resources, LMP, auxiliary service requirements from an economic perspective System agnostic approach using historical generation data
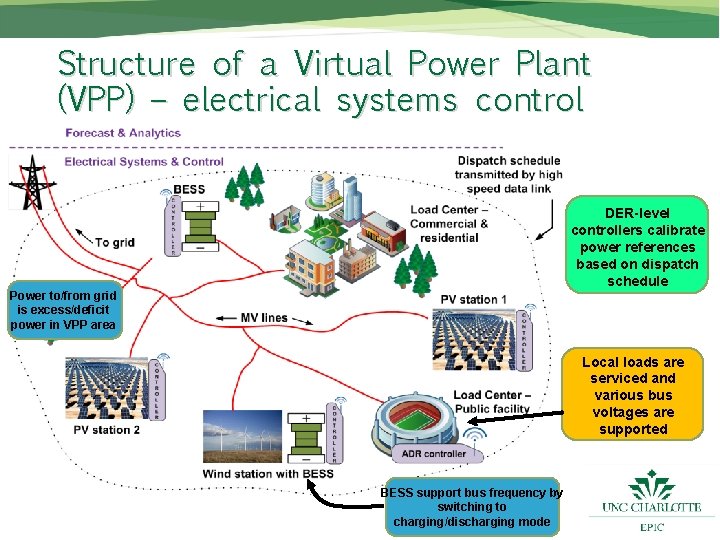
Structure of a Virtual Power Plant (VPP) – electrical systems control DER-level controllers calibrate power references based on dispatch schedule Power to/from grid is excess/deficit power in VPP area Local loads are serviced and various bus voltages are supported BESS support bus frequency by switching to charging/discharging mode
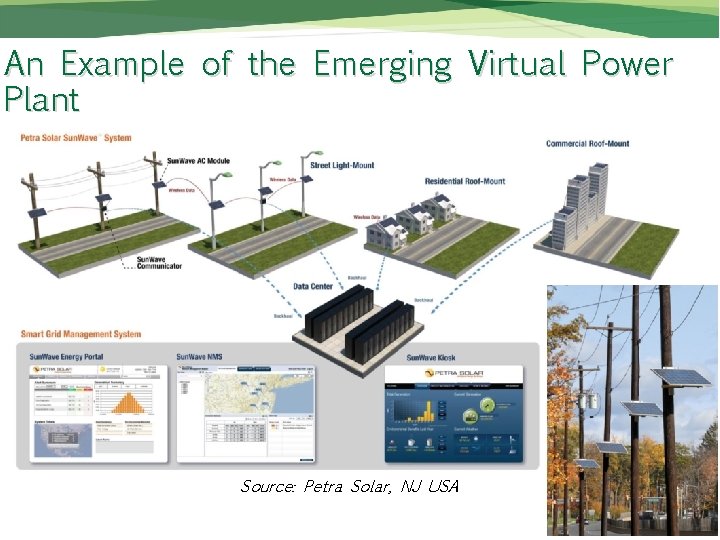
An Example of the Emerging Virtual Power Plant Source: Petra Solar, NJ USA
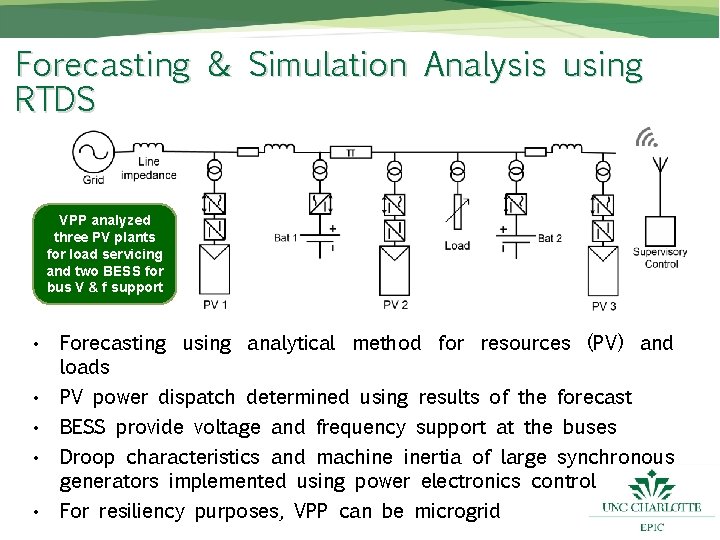
Forecasting & Simulation Analysis using RTDS VPP analyzed three PV plants for load servicing and two BESS for bus V & f support • • • Forecasting using analytical method for resources (PV) and loads PV power dispatch determined using results of the forecast BESS provide voltage and frequency support at the buses Droop characteristics and machine inertia of large synchronous generators implemented using power electronics control For resiliency purposes, VPP can be microgrid
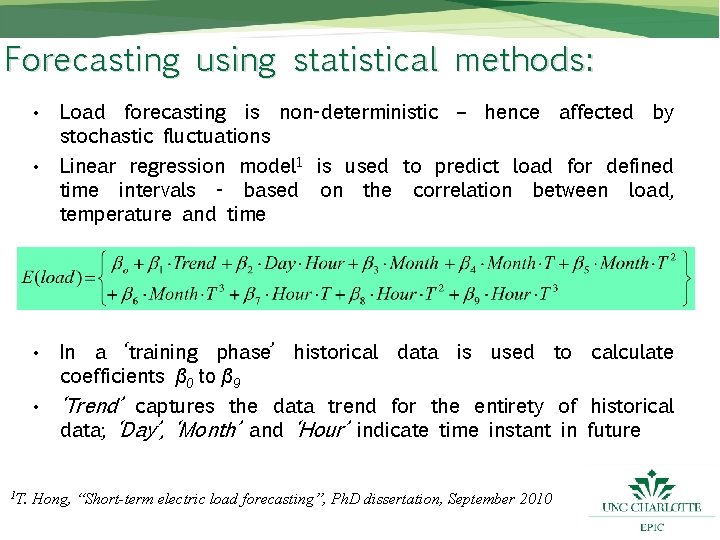
Forecasting using statistical methods: • • 1 T. Load forecasting is non-deterministic – hence affected by stochastic fluctuations Linear regression model 1 is used to predict load for defined time intervals - based on the correlation between load, temperature and time In a ‘training phase’ historical data is used to calculate coefficients β 0 to β 9 ‘Trend’ captures the data trend for the entirety of historical data; ‘Day’, ‘Month’ and ‘Hour’ indicate time instant in future Hong, “Short-term electric load forecasting”, Ph. D dissertation, September 2010
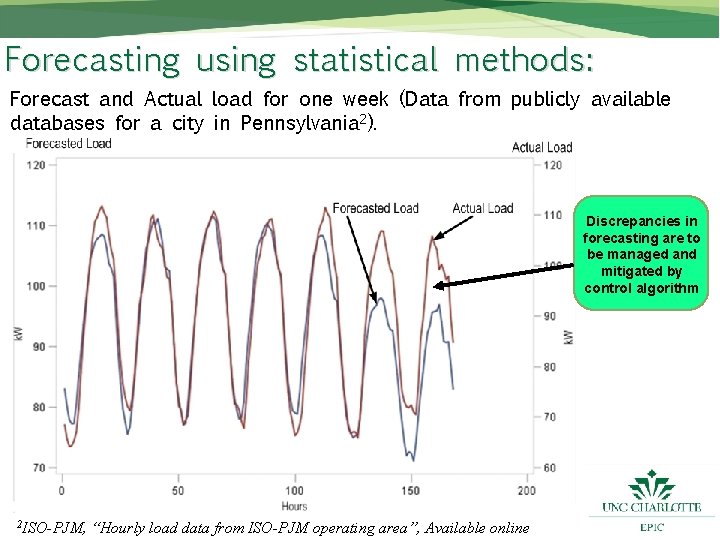
Forecasting using statistical methods: Forecast and Actual load for one week (Data from publicly available databases for a city in Pennsylvania 2). Discrepancies in forecasting are to be managed and mitigated by control algorithm 2 ISO-PJM, “Hourly load data from ISO-PJM operating area”, Available online
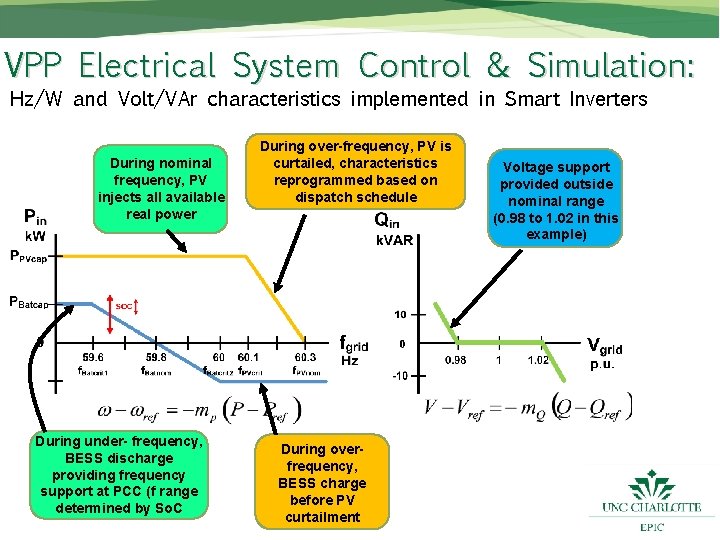
VPP Electrical System Control & Simulation: Hz/W and Volt/VAr characteristics implemented in Smart Inverters During nominal frequency, PV injects all available real power During under- frequency, BESS discharge providing frequency support at PCC (f range determined by So. C During over-frequency, PV is curtailed, characteristics reprogrammed based on dispatch schedule During overfrequency, BESS charge before PV curtailment Voltage support provided outside nominal range (0. 98 to 1. 02 in this example)
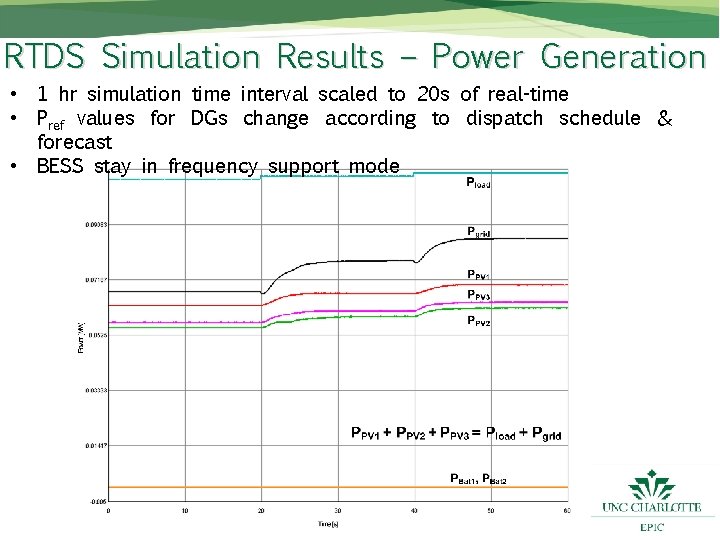
RTDS Simulation Results – Power Generation • 1 hr simulation time interval scaled to 20 s of real-time • Pref values for DGs change according to dispatch schedule & forecast • BESS stay in frequency support mode
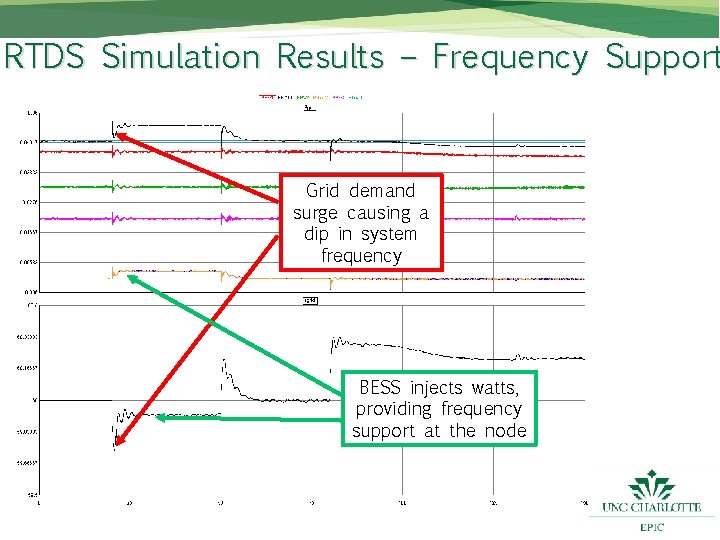
RTDS Simulation Results – Frequency Support Grid demand surge causing a dip in system frequency BESS injects watts, providing frequency support at the node
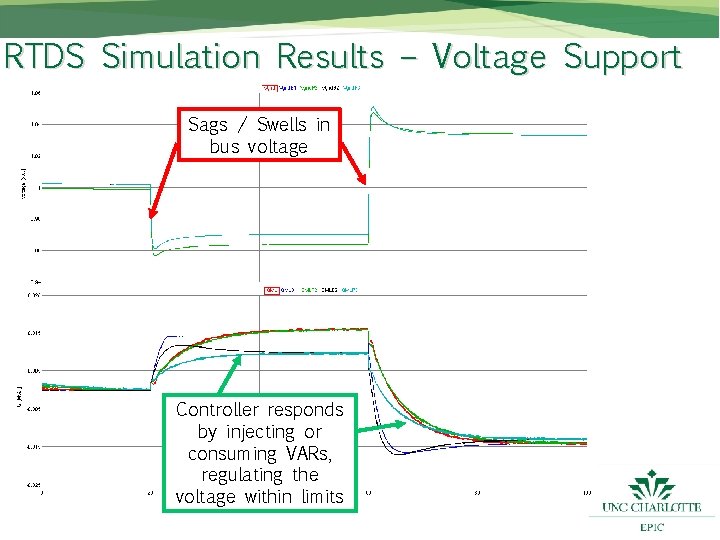
RTDS Simulation Results – Voltage Support Sags / Swells in bus voltage Controller responds by injecting or consuming VARs, regulating the voltage within limits
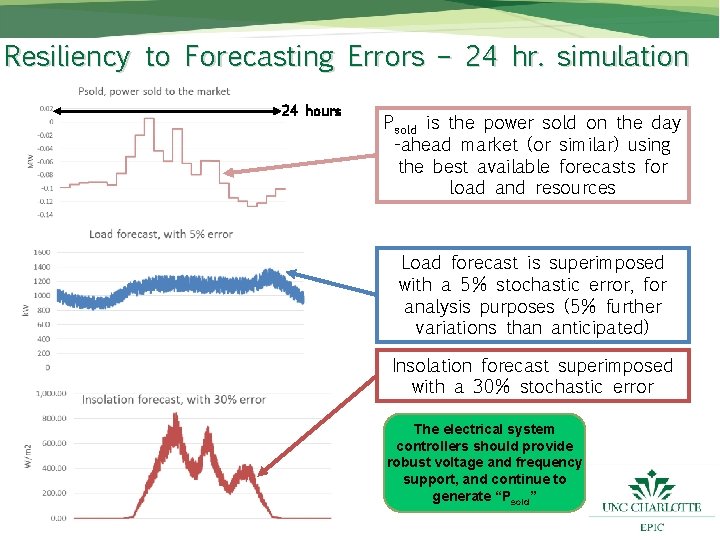
Resiliency to Forecasting Errors – 24 hr. simulation 24 hours Psold is the power sold on the day -ahead market (or similar) using the best available forecasts for load and resources Load forecast is superimposed with a 5% stochastic error, for analysis purposes (5% further variations than anticipated) Insolation forecast superimposed with a 30% stochastic error The electrical system controllers should provide robust voltage and frequency support, and continue to generate “Psold”
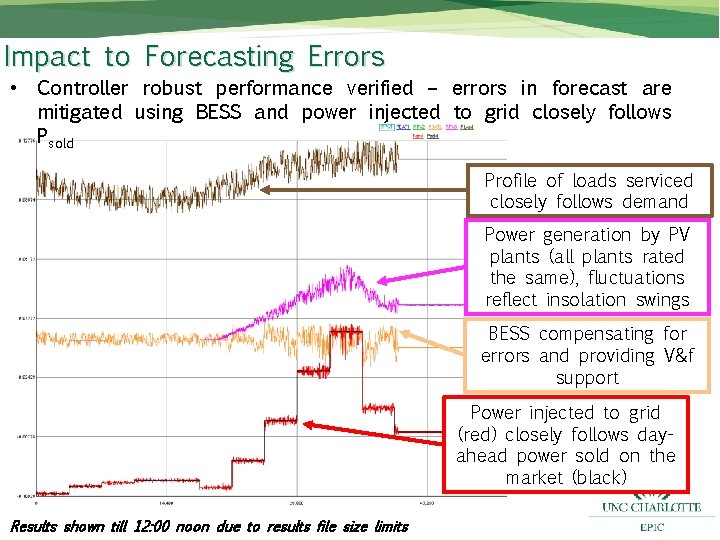
Impact to Forecasting Errors • Controller robust performance verified – errors in forecast are mitigated using BESS and power injected to grid closely follows Psold Profile of loads serviced closely follows demand Power generation by PV plants (all plants rated the same), fluctuations reflect insolation swings BESS compensating for errors and providing V&f support Power injected to grid (red) closely follows dayahead power sold on the market (black) Results shown till 12: 00 noon due to results file size limits
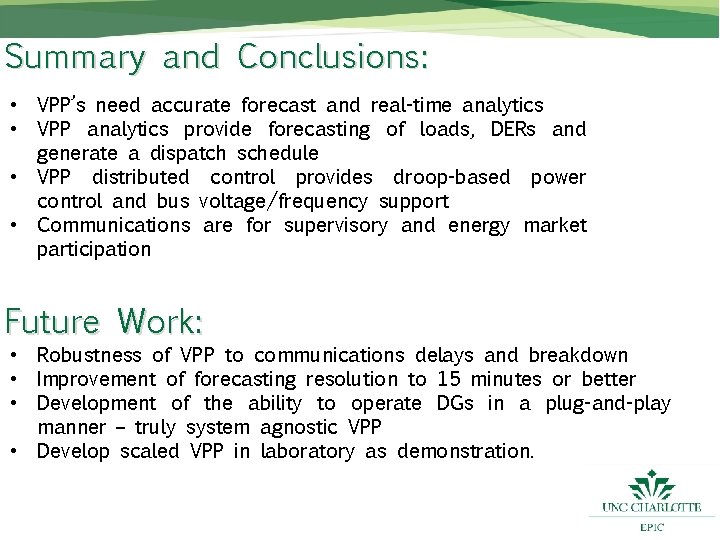
Summary and Conclusions: • VPP’s need accurate forecast and real-time analytics • VPP analytics provide forecasting of loads, DERs and generate a dispatch schedule • VPP distributed control provides droop-based power control and bus voltage/frequency support • Communications are for supervisory and energy market participation Future Work: • Robustness of VPP to communications delays and breakdown • Improvement of forecasting resolution to 15 minutes or better • Development of the ability to operate DGs in a plug-and-play manner – truly system agnostic VPP • Develop scaled VPP in laboratory as demonstration.
Future smart ways to pay answers
Perfect future progressive
Future perfect simple vs future perfect continuous
Draw the power triangle
What non vascular plants
Non vascular plant reproduction
Classification of non flowering plants
C3 plant
Energy and electric field
Potential difference
Electrical potential energy
Electric potential electric field
A suitable electric pump in an electric circuit is a
Chapter 21 electric charge and electric field
Chapter 21 electric charge and electric field
Electric force
Units of a charge
Electric charges and electric forces lesson outline