Research Methods Review Advanced Cognitive Psychology PSY 421
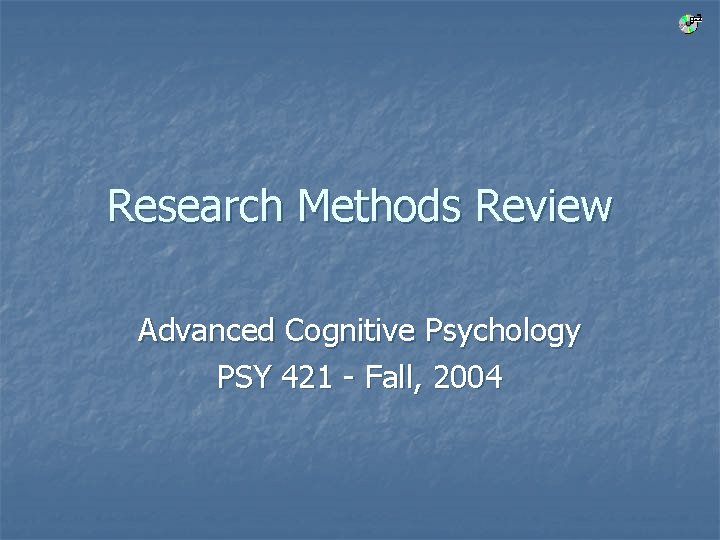
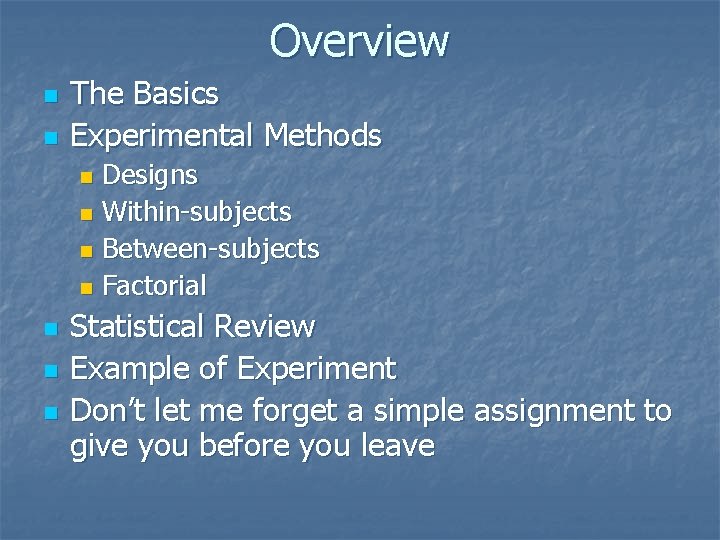
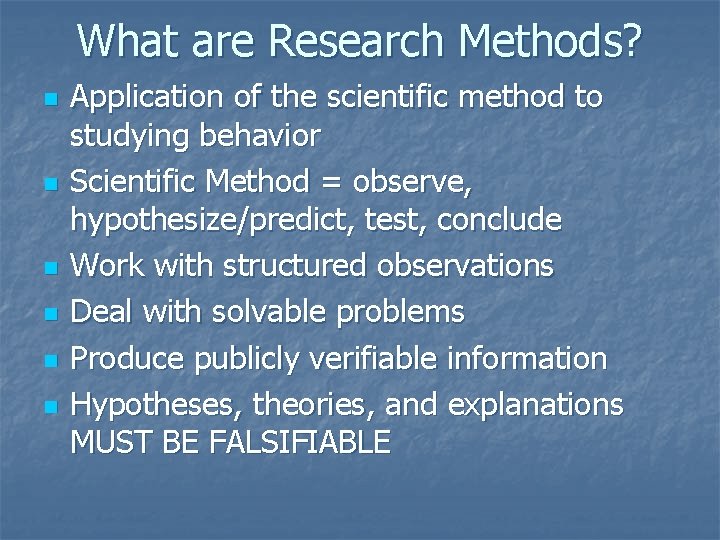
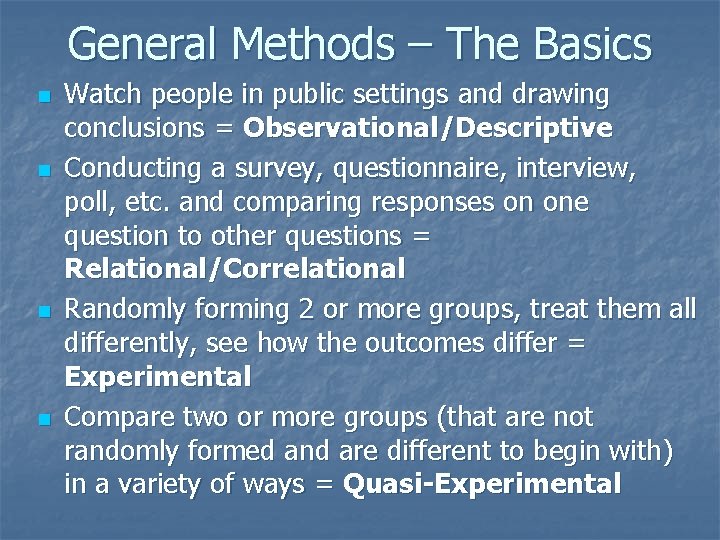
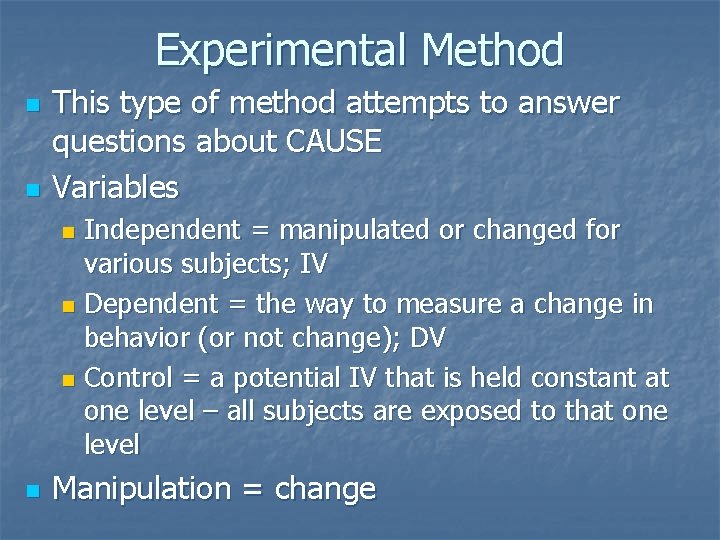
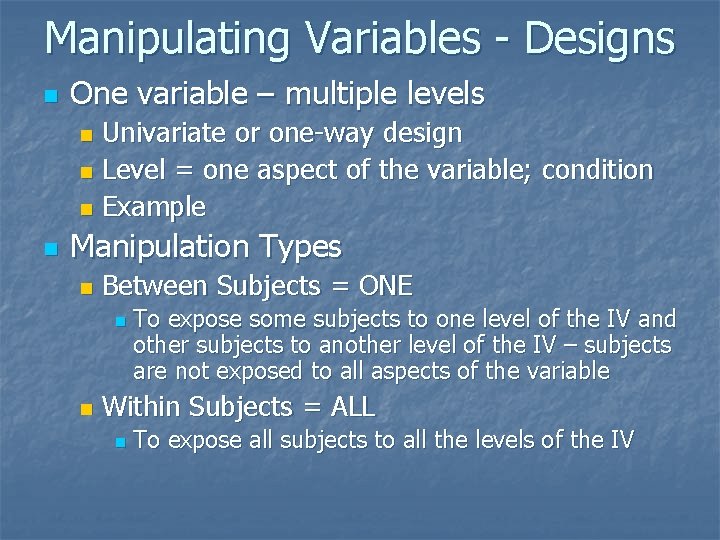
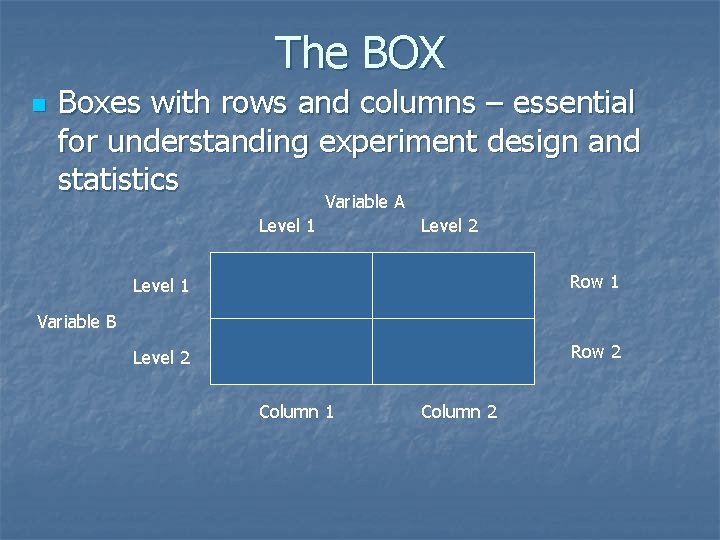
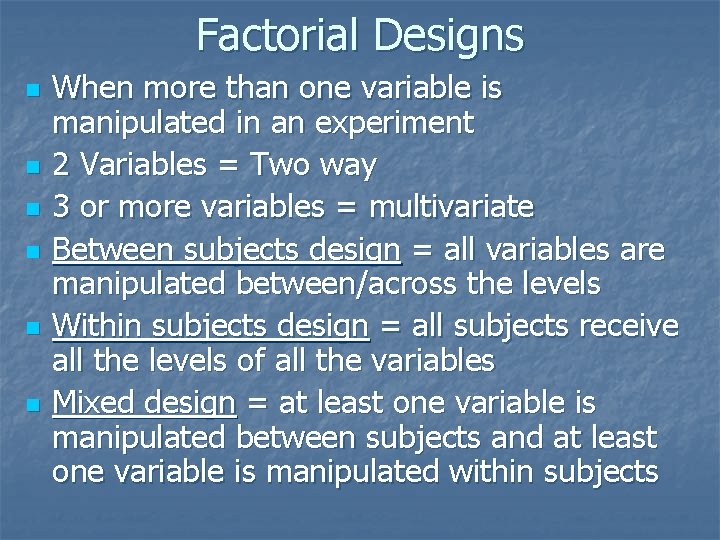
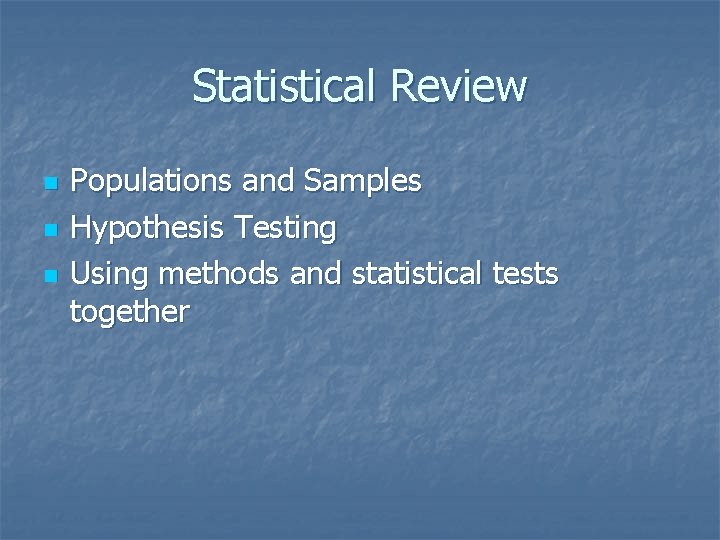
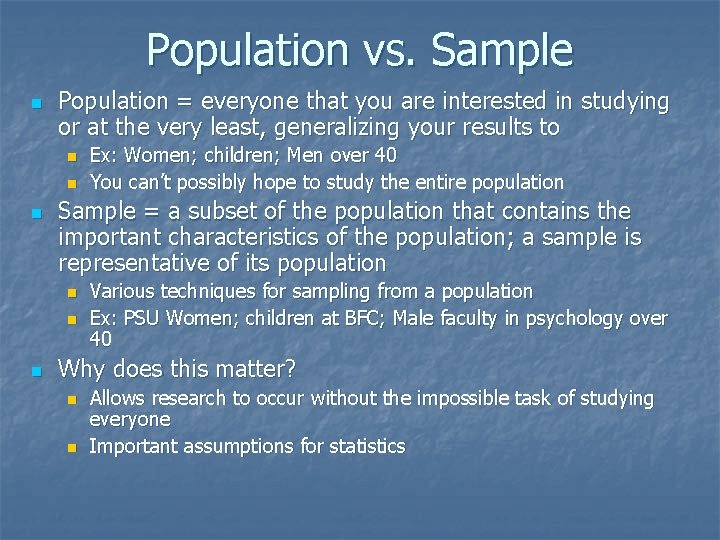
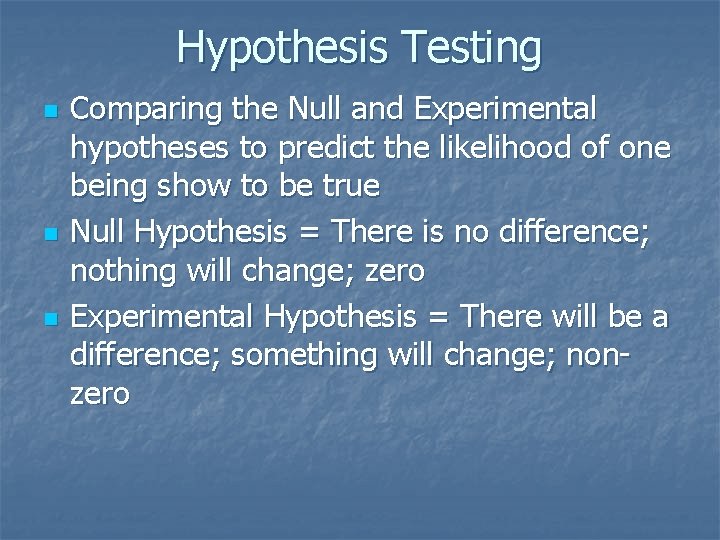
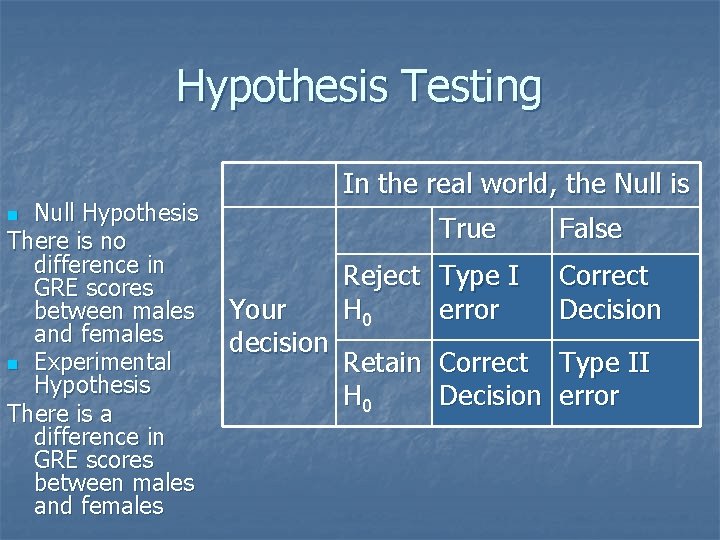
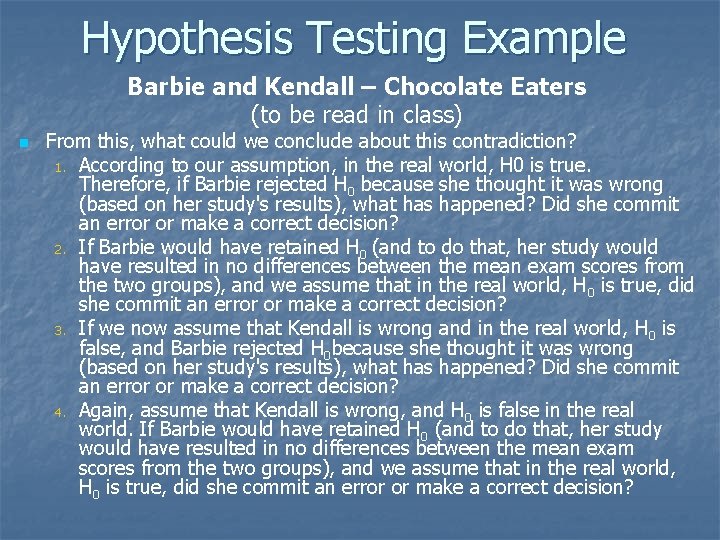
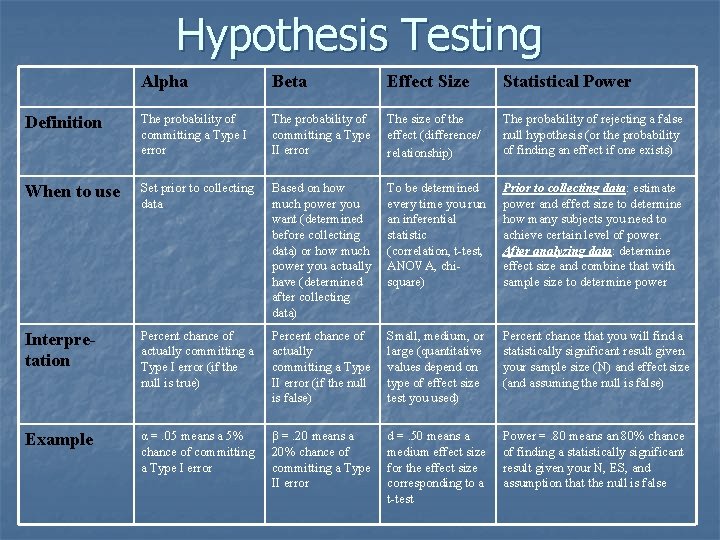
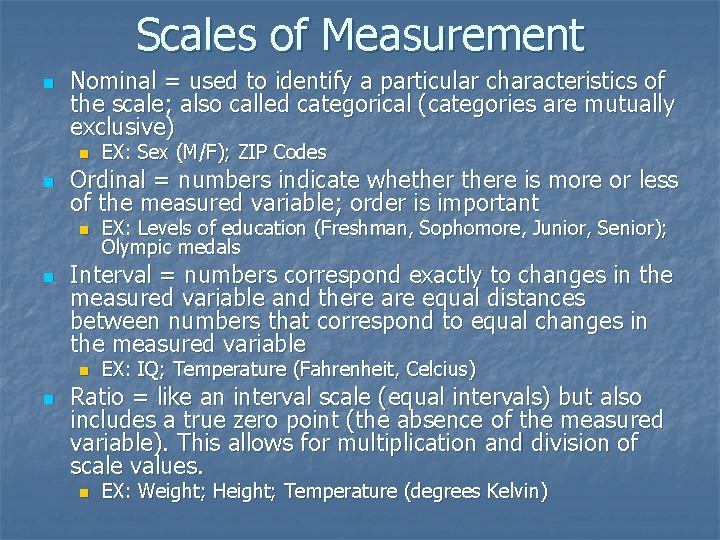
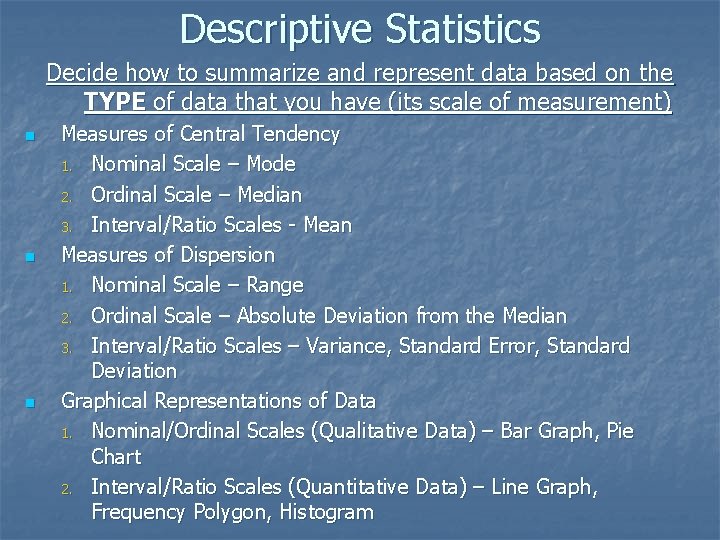
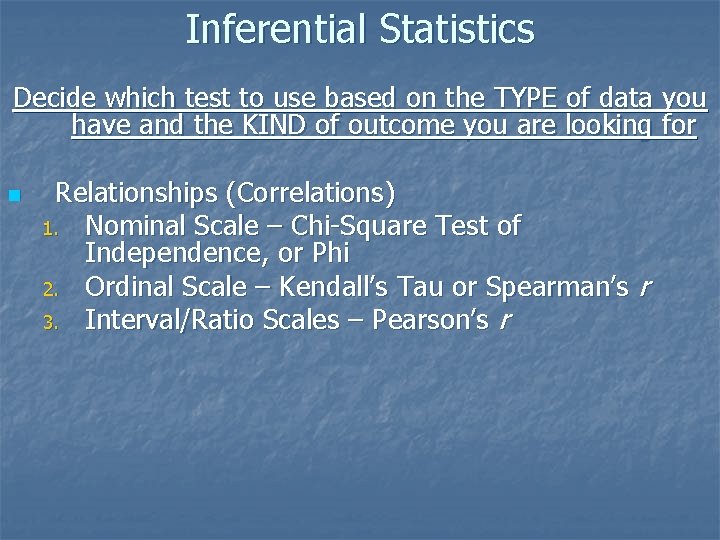
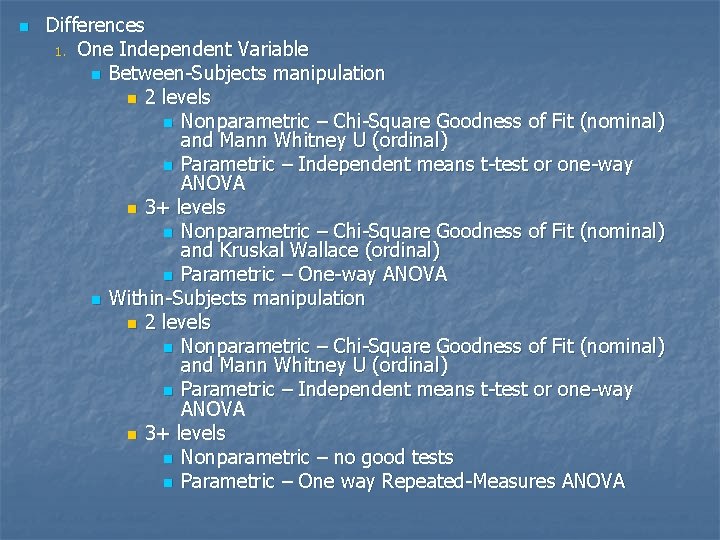
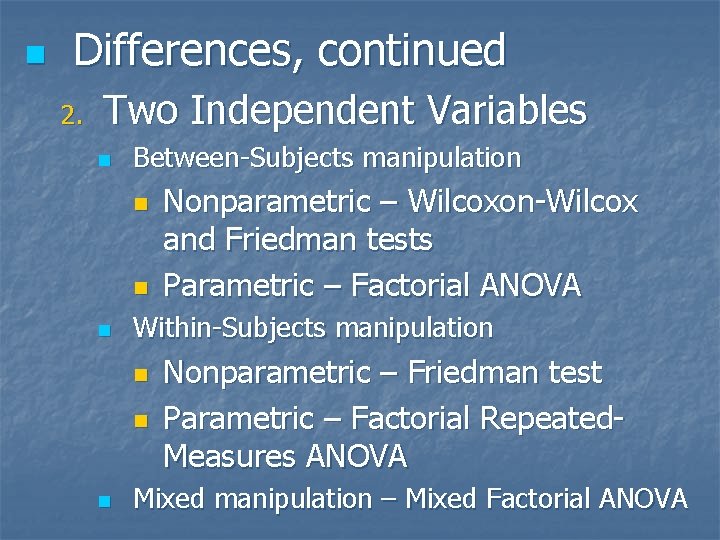
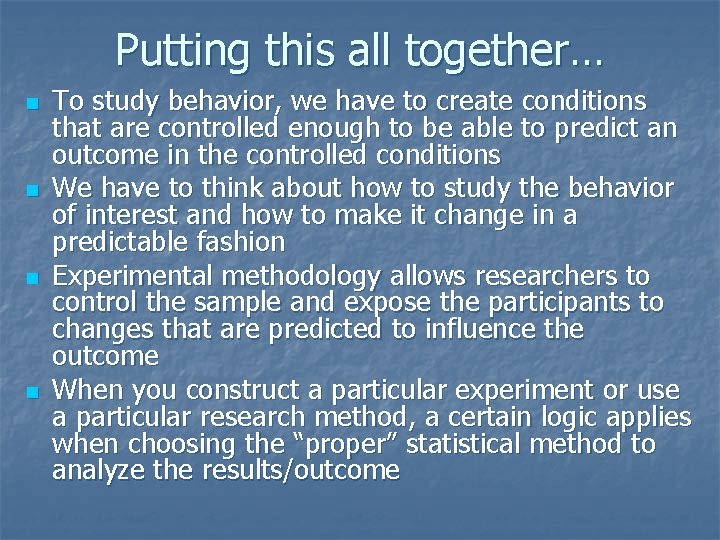
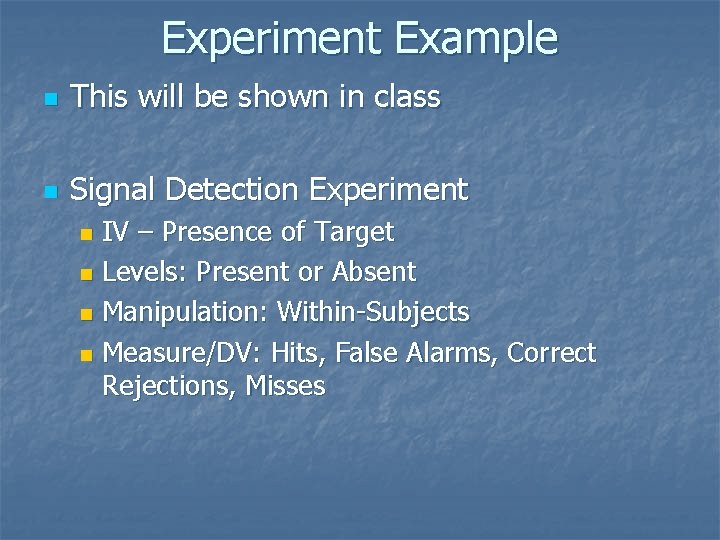
- Slides: 21
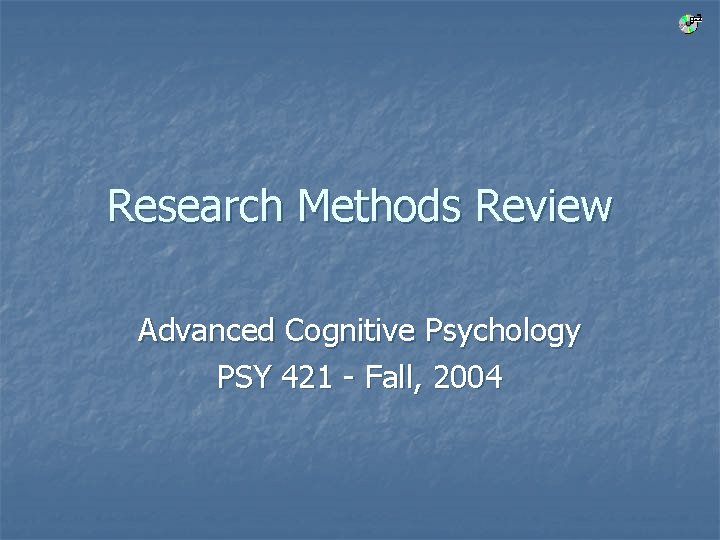
Research Methods Review Advanced Cognitive Psychology PSY 421 - Fall, 2004
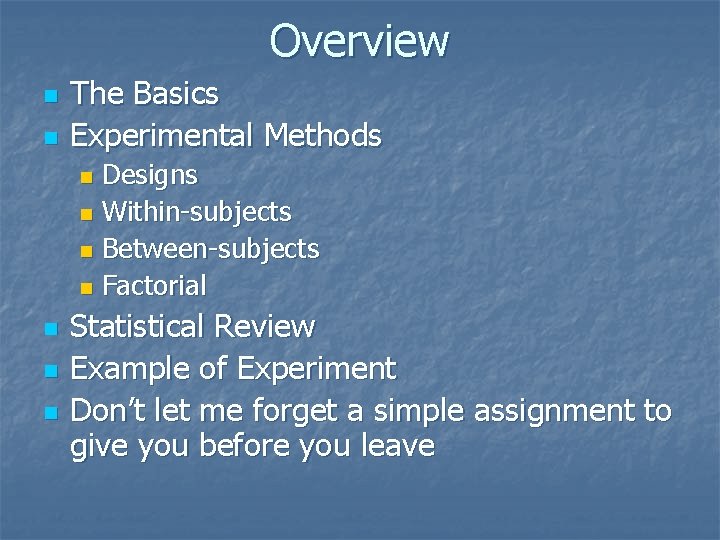
Overview n n The Basics Experimental Methods Designs n Within-subjects n Between-subjects n Factorial n n Statistical Review Example of Experiment Don’t let me forget a simple assignment to give you before you leave
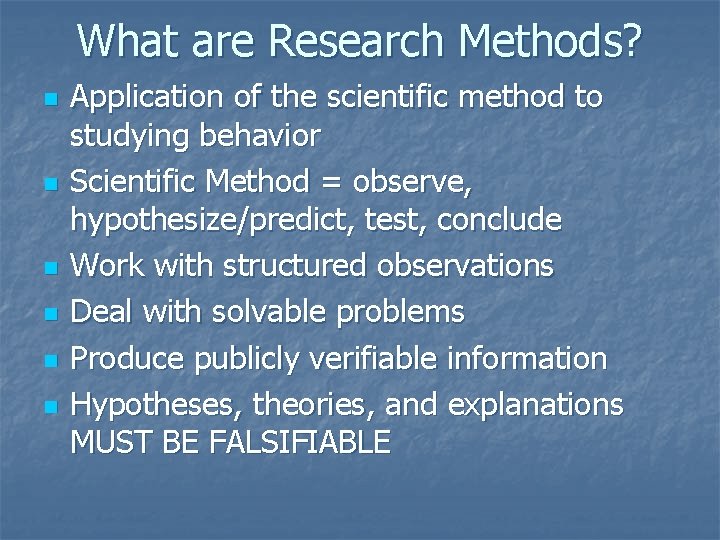
What are Research Methods? n n n Application of the scientific method to studying behavior Scientific Method = observe, hypothesize/predict, test, conclude Work with structured observations Deal with solvable problems Produce publicly verifiable information Hypotheses, theories, and explanations MUST BE FALSIFIABLE
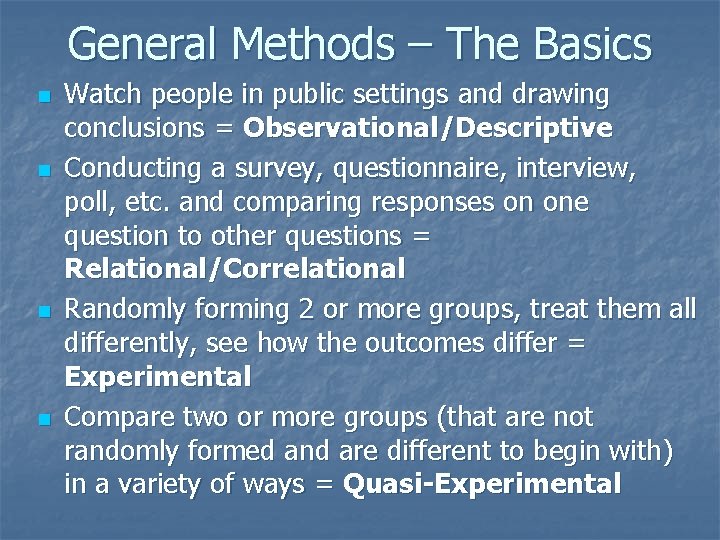
General Methods – The Basics n n Watch people in public settings and drawing conclusions = Observational/Descriptive Conducting a survey, questionnaire, interview, poll, etc. and comparing responses on one question to other questions = Relational/Correlational Randomly forming 2 or more groups, treat them all differently, see how the outcomes differ = Experimental Compare two or more groups (that are not randomly formed and are different to begin with) in a variety of ways = Quasi-Experimental
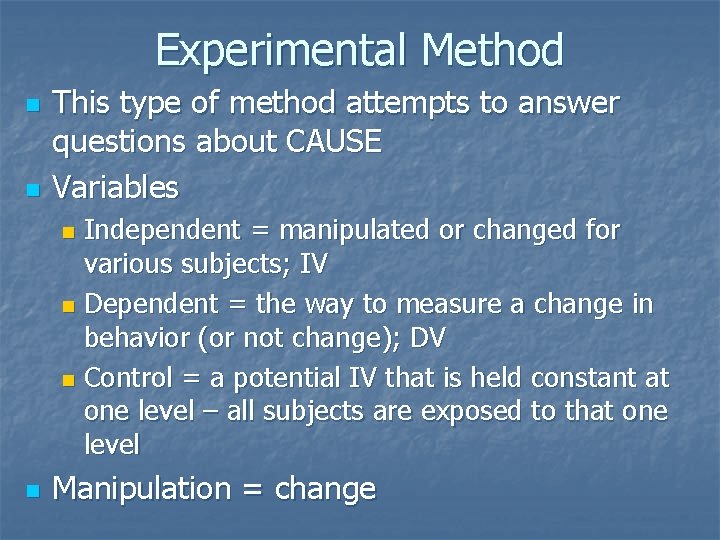
Experimental Method n n This type of method attempts to answer questions about CAUSE Variables Independent = manipulated or changed for various subjects; IV n Dependent = the way to measure a change in behavior (or not change); DV n Control = a potential IV that is held constant at one level – all subjects are exposed to that one level n n Manipulation = change
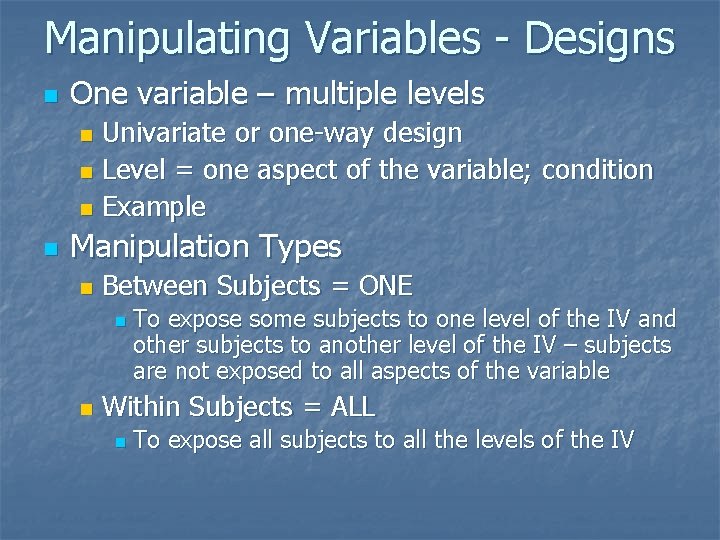
Manipulating Variables - Designs n One variable – multiple levels Univariate or one-way design n Level = one aspect of the variable; condition n Example n n Manipulation Types n Between Subjects = ONE n n To expose some subjects to one level of the IV and other subjects to another level of the IV – subjects are not exposed to all aspects of the variable Within Subjects = ALL n To expose all subjects to all the levels of the IV
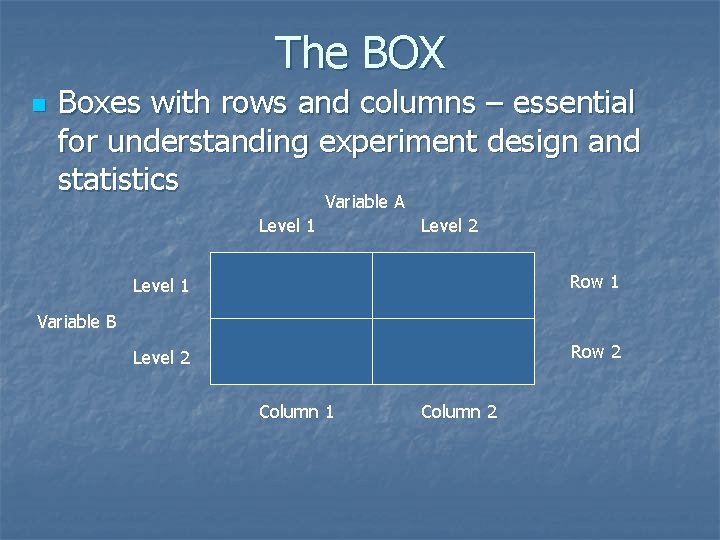
The BOX n Boxes with rows and columns – essential for understanding experiment design and statistics Variable A Level 1 Level 2 Level 1 Row 1 Level 2 Row 2 Variable B Column 1 Column 2
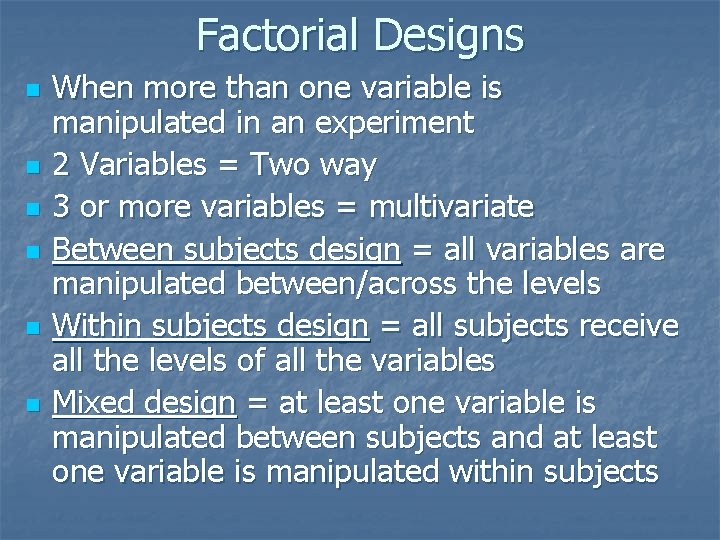
Factorial Designs n n n When more than one variable is manipulated in an experiment 2 Variables = Two way 3 or more variables = multivariate Between subjects design = all variables are manipulated between/across the levels Within subjects design = all subjects receive all the levels of all the variables Mixed design = at least one variable is manipulated between subjects and at least one variable is manipulated within subjects
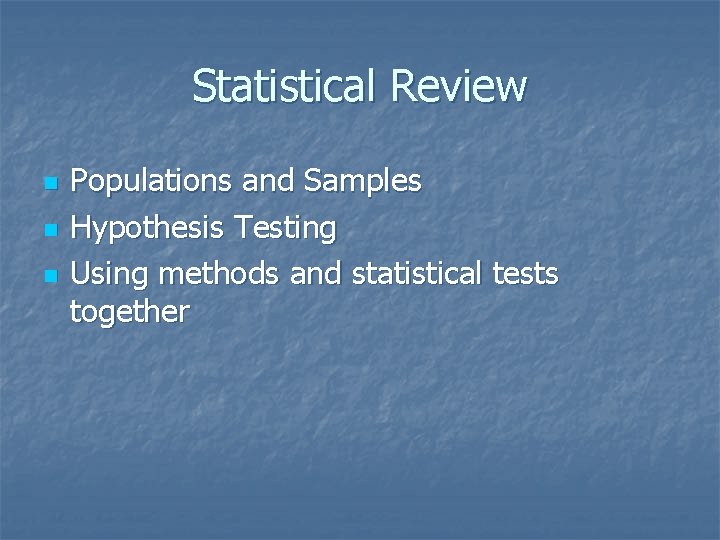
Statistical Review n n n Populations and Samples Hypothesis Testing Using methods and statistical tests together
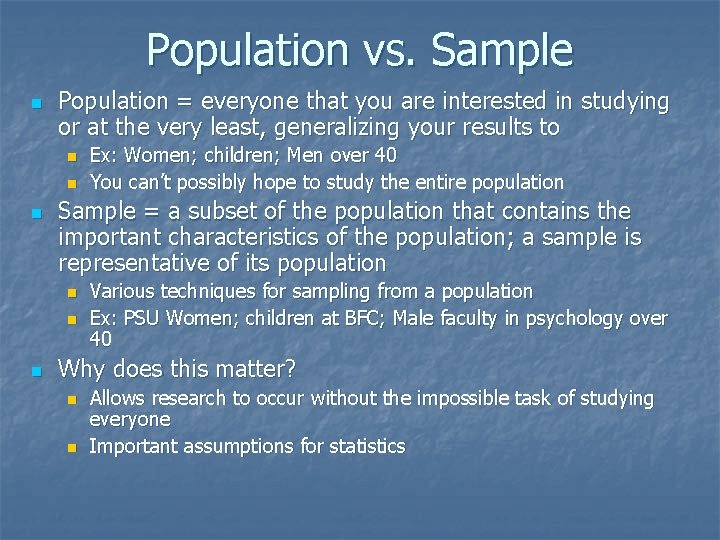
Population vs. Sample n Population = everyone that you are interested in studying or at the very least, generalizing your results to n n n Sample = a subset of the population that contains the important characteristics of the population; a sample is representative of its population n Ex: Women; children; Men over 40 You can’t possibly hope to study the entire population Various techniques for sampling from a population Ex: PSU Women; children at BFC; Male faculty in psychology over 40 Why does this matter? n n Allows research to occur without the impossible task of studying everyone Important assumptions for statistics
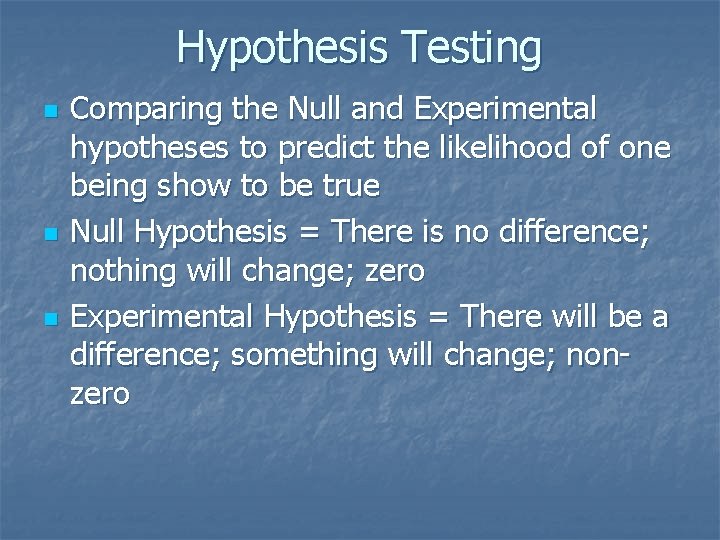
Hypothesis Testing n n n Comparing the Null and Experimental hypotheses to predict the likelihood of one being show to be true Null Hypothesis = There is no difference; nothing will change; zero Experimental Hypothesis = There will be a difference; something will change; nonzero
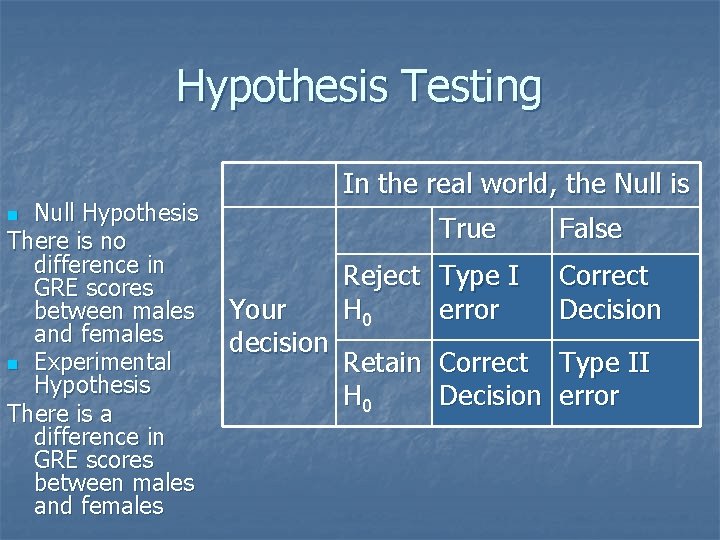
Hypothesis Testing Null Hypothesis There is no difference in GRE scores between males and females n Experimental Hypothesis There is a difference in GRE scores between males and females In the real world, the Null is n True Your decision Reject Type I H 0 error False Correct Decision Retain Correct Type II H 0 Decision error
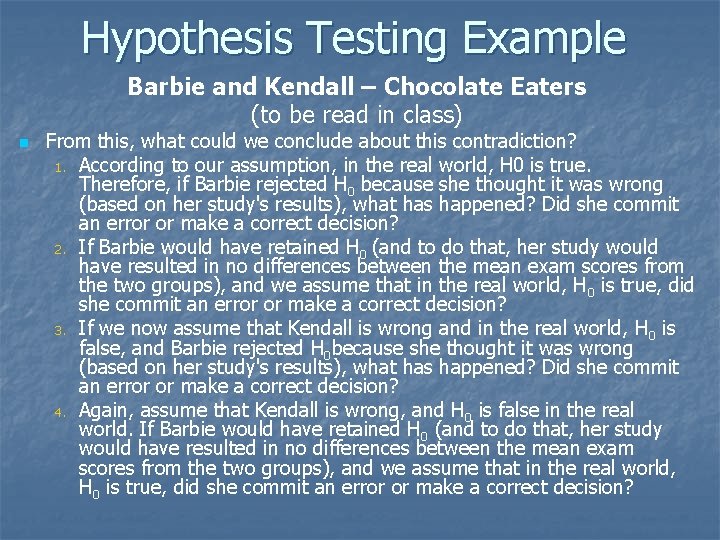
Hypothesis Testing Example Barbie and Kendall – Chocolate Eaters (to be read in class) n From this, what could we conclude about this contradiction? 1. According to our assumption, in the real world, H 0 is true. Therefore, if Barbie rejected H 0 because she thought it was wrong (based on her study's results), what has happened? Did she commit an error or make a correct decision? 2. If Barbie would have retained H 0 (and to do that, her study would have resulted in no differences between the mean exam scores from the two groups), and we assume that in the real world, H 0 is true, did she commit an error or make a correct decision? 3. If we now assume that Kendall is wrong and in the real world, H 0 is false, and Barbie rejected H 0 because she thought it was wrong (based on her study's results), what has happened? Did she commit an error or make a correct decision? 4. Again, assume that Kendall is wrong, and H 0 is false in the real world. If Barbie would have retained H 0 (and to do that, her study would have resulted in no differences between the mean exam scores from the two groups), and we assume that in the real world, H 0 is true, did she commit an error or make a correct decision?
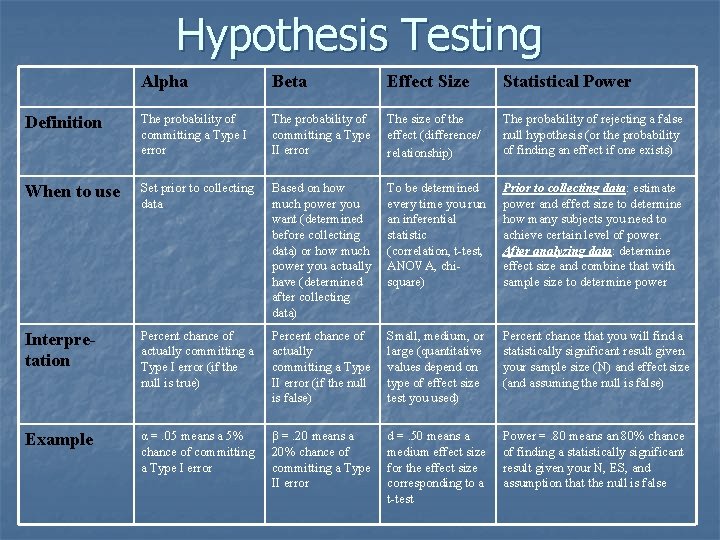
Hypothesis Testing Alpha Beta Effect Size Statistical Power Definition The probability of committing a Type I error The probability of committing a Type II error The size of the effect (difference/ relationship) The probability of rejecting a false null hypothesis (or the probability of finding an effect if one exists) When to use Set prior to collecting data Based on how much power you want (determined before collecting data) or how much power you actually have (determined after collecting data) To be determined every time you run an inferential statistic (correlation, t-test, ANOVA, chisquare) Prior to collecting data: estimate power and effect size to determine how many subjects you need to achieve certain level of power. After analyzing data: determine effect size and combine that with sample size to determine power Interpretation Percent chance of actually committing a Type I error (if the null is true) Percent chance of actually committing a Type II error (if the null is false) Small, medium, or large (quantitative values depend on type of effect size test you used) Percent chance that you will find a statistically significant result given your sample size (N) and effect size (and assuming the null is false) Example α =. 05 means a 5% chance of committing a Type I error β =. 20 means a 20% chance of committing a Type II error d =. 50 means a medium effect size for the effect size corresponding to a t-test Power =. 80 means an 80% chance of finding a statistically significant result given your N, ES, and assumption that the null is false
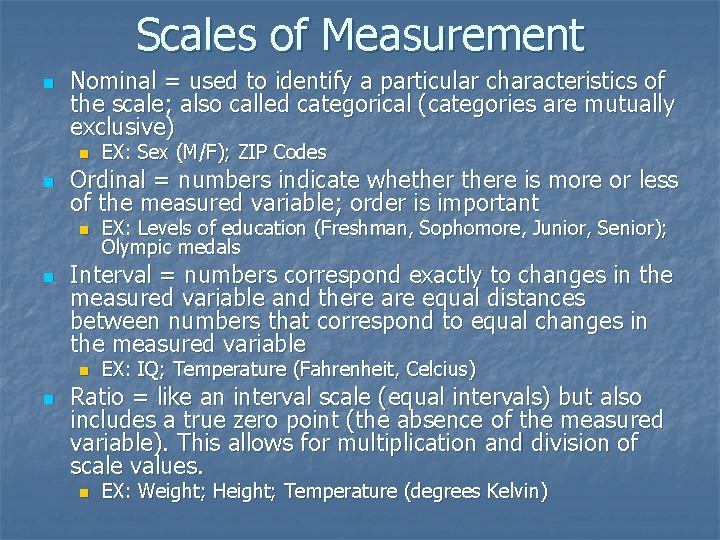
Scales of Measurement n Nominal = used to identify a particular characteristics of the scale; also called categorical (categories are mutually exclusive) n n Ordinal = numbers indicate whethere is more or less of the measured variable; order is important n n EX: Levels of education (Freshman, Sophomore, Junior, Senior); Olympic medals Interval = numbers correspond exactly to changes in the measured variable and there are equal distances between numbers that correspond to equal changes in the measured variable n n EX: Sex (M/F); ZIP Codes EX: IQ; Temperature (Fahrenheit, Celcius) Ratio = like an interval scale (equal intervals) but also includes a true zero point (the absence of the measured variable). This allows for multiplication and division of scale values. n EX: Weight; Height; Temperature (degrees Kelvin)
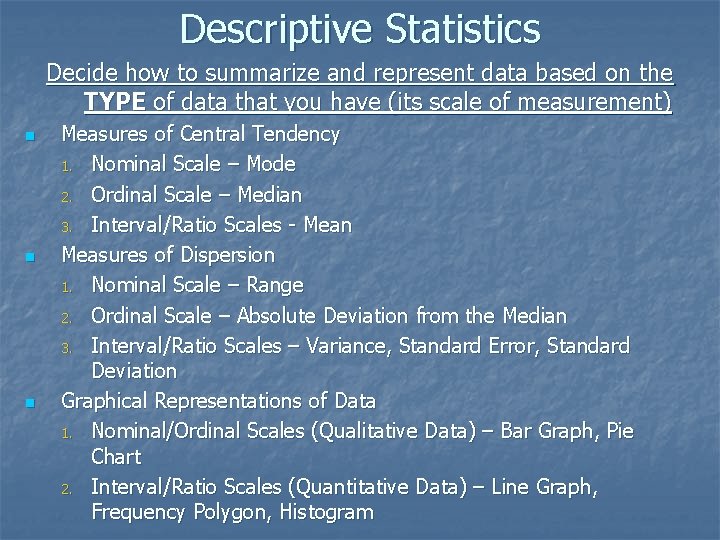
Descriptive Statistics Decide how to summarize and represent data based on the TYPE of data that you have (its scale of measurement) n n n Measures of Central Tendency 1. Nominal Scale – Mode 2. Ordinal Scale – Median 3. Interval/Ratio Scales - Mean Measures of Dispersion 1. Nominal Scale – Range 2. Ordinal Scale – Absolute Deviation from the Median 3. Interval/Ratio Scales – Variance, Standard Error, Standard Deviation Graphical Representations of Data 1. Nominal/Ordinal Scales (Qualitative Data) – Bar Graph, Pie Chart 2. Interval/Ratio Scales (Quantitative Data) – Line Graph, Frequency Polygon, Histogram
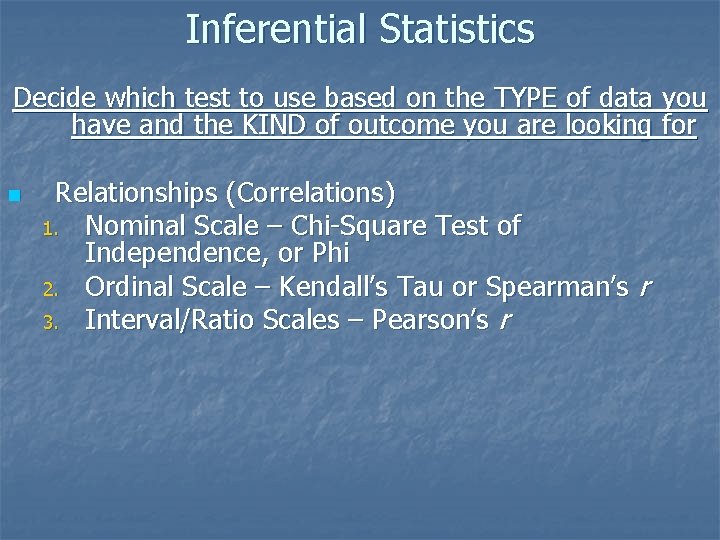
Inferential Statistics Decide which test to use based on the TYPE of data you have and the KIND of outcome you are looking for n Relationships (Correlations) 1. Nominal Scale – Chi-Square Test of Independence, or Phi 2. Ordinal Scale – Kendall’s Tau or Spearman’s r 3. Interval/Ratio Scales – Pearson’s r
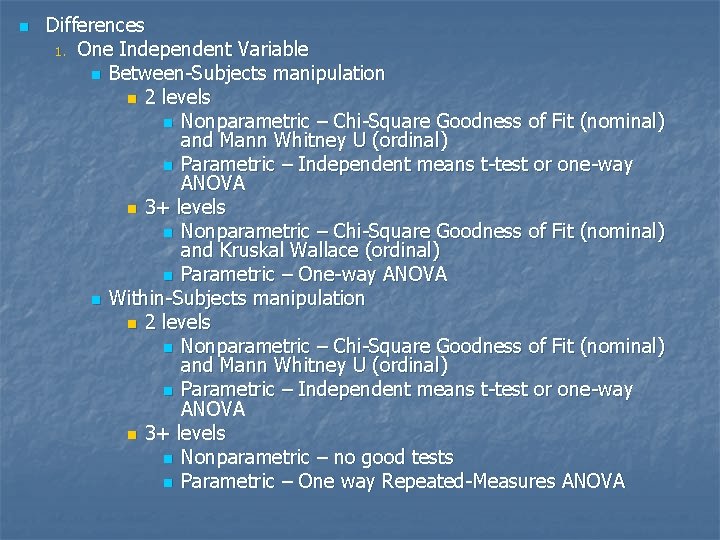
n Differences 1. One Independent Variable n Between-Subjects manipulation n 2 levels n Nonparametric – Chi-Square Goodness of Fit (nominal) and Mann Whitney U (ordinal) n Parametric – Independent means t-test or one-way ANOVA n 3+ levels n Nonparametric – Chi-Square Goodness of Fit (nominal) and Kruskal Wallace (ordinal) n Parametric – One-way ANOVA n Within-Subjects manipulation n 2 levels n Nonparametric – Chi-Square Goodness of Fit (nominal) and Mann Whitney U (ordinal) n Parametric – Independent means t-test or one-way ANOVA n 3+ levels n Nonparametric – no good tests n Parametric – One way Repeated-Measures ANOVA
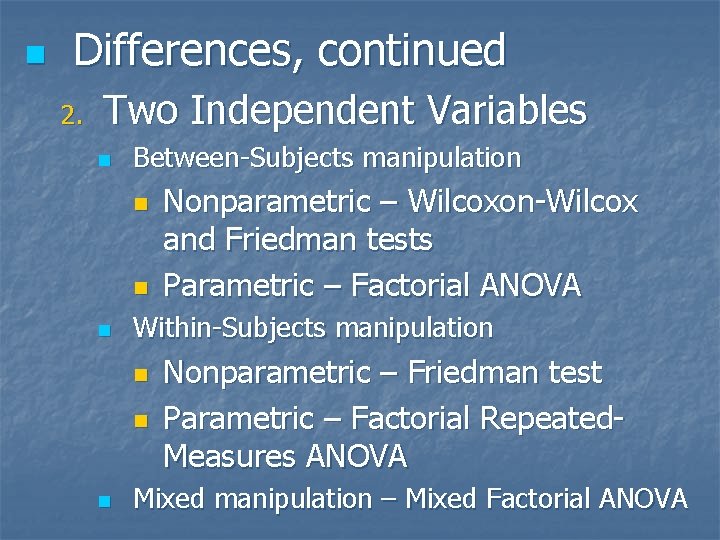
n Differences, continued 2. Two Independent Variables n Between-Subjects manipulation n Within-Subjects manipulation n Nonparametric – Wilcoxon-Wilcox and Friedman tests Parametric – Factorial ANOVA Nonparametric – Friedman test Parametric – Factorial Repeated. Measures ANOVA Mixed manipulation – Mixed Factorial ANOVA
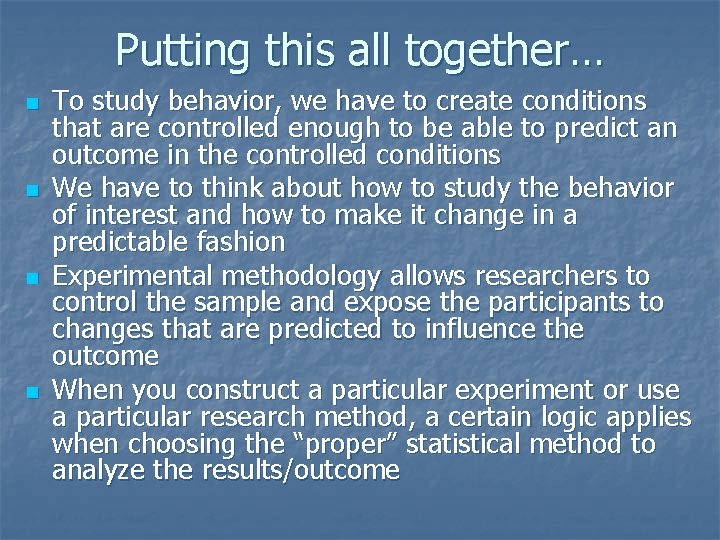
Putting this all together… n n To study behavior, we have to create conditions that are controlled enough to be able to predict an outcome in the controlled conditions We have to think about how to study the behavior of interest and how to make it change in a predictable fashion Experimental methodology allows researchers to control the sample and expose the participants to changes that are predicted to influence the outcome When you construct a particular experiment or use a particular research method, a certain logic applies when choosing the “proper” statistical method to analyze the results/outcome
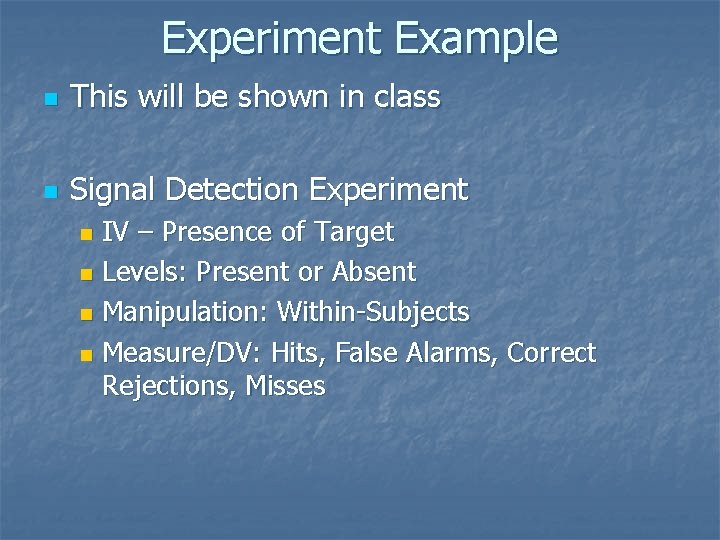
Experiment Example n This will be shown in class n Signal Detection Experiment IV – Presence of Target n Levels: Present or Absent n Manipulation: Within-Subjects n Measure/DV: Hits, False Alarms, Correct Rejections, Misses n
Psy
Advanced business research
Advanced higher modern studies research methods
Methods of social psychology
Research methods in developmental psychology
Research methods in abnormal psychology
Ocr psychology research methods
Chapter 2 critical thinking answers
Cognitive and non cognitive religious language
Euro psy
Ucf psychology requirements
Psy 2055
Psy 2055
Xxxxblue
Psy
Psy sanin
Psy
Psy
Psy
Psy 226
Maria took a drink from a container marked milk
Alla kulikova