Precipitation Estimation from Remotely Sensed Information using Artificial
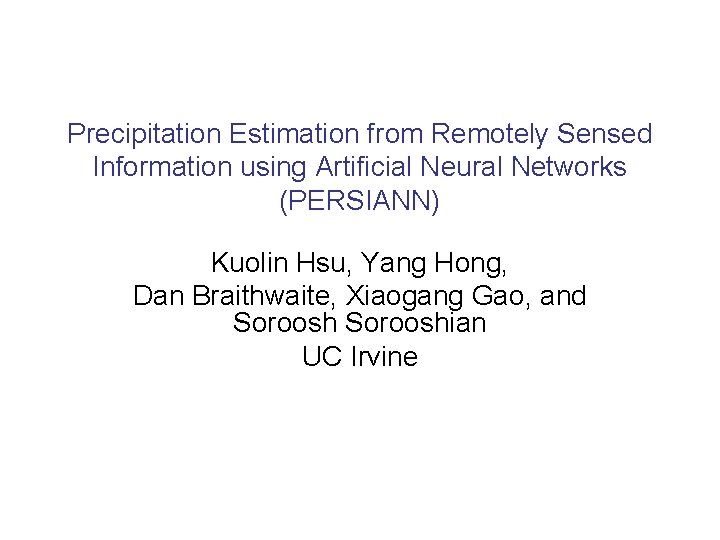
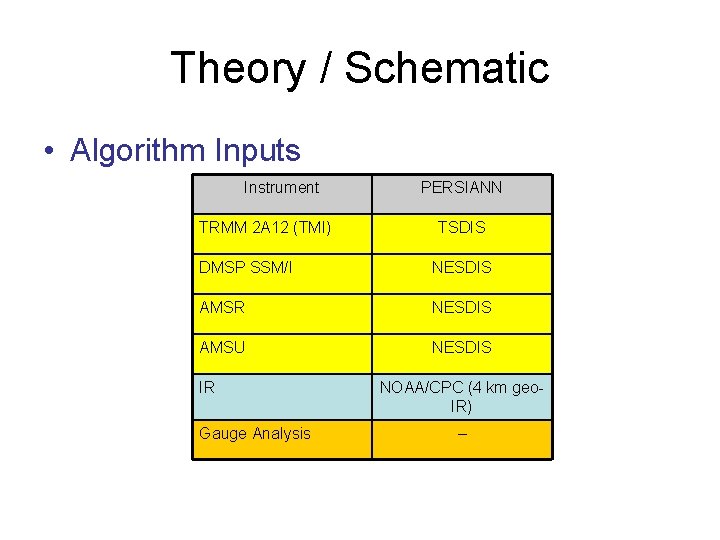
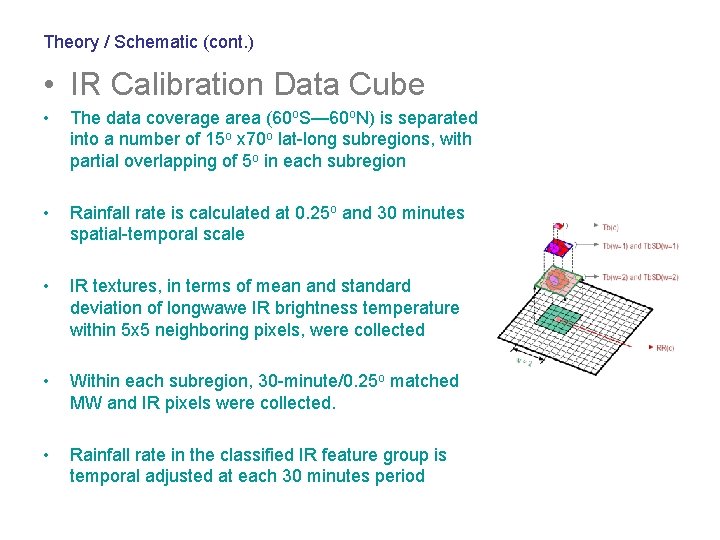
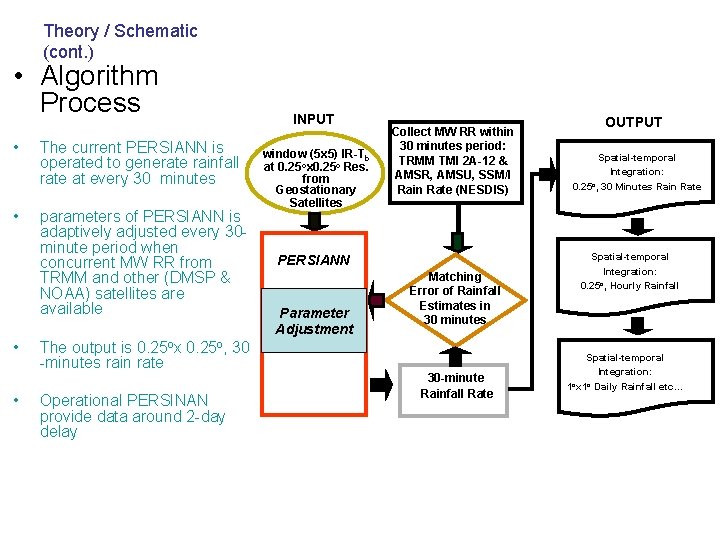
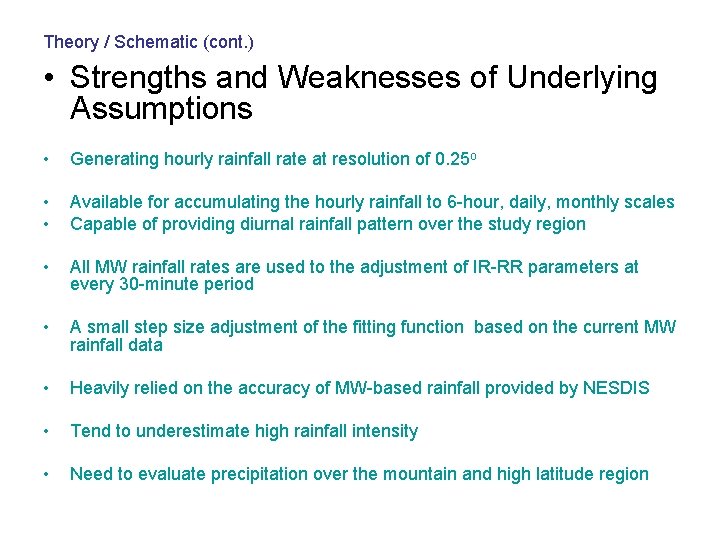
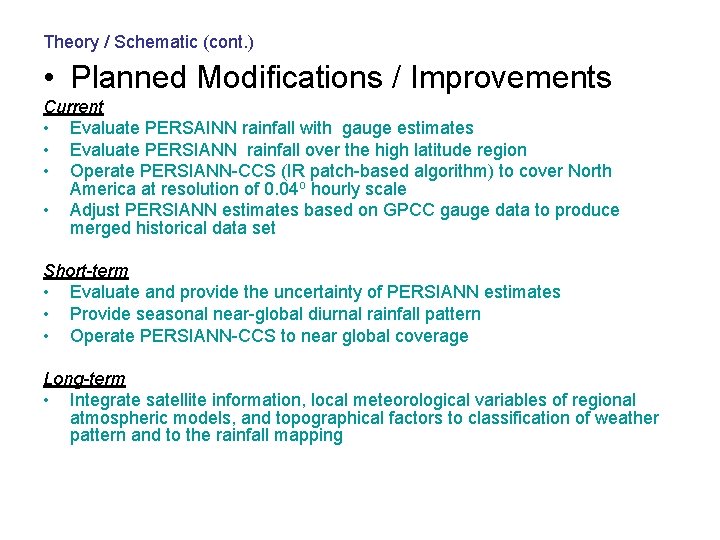
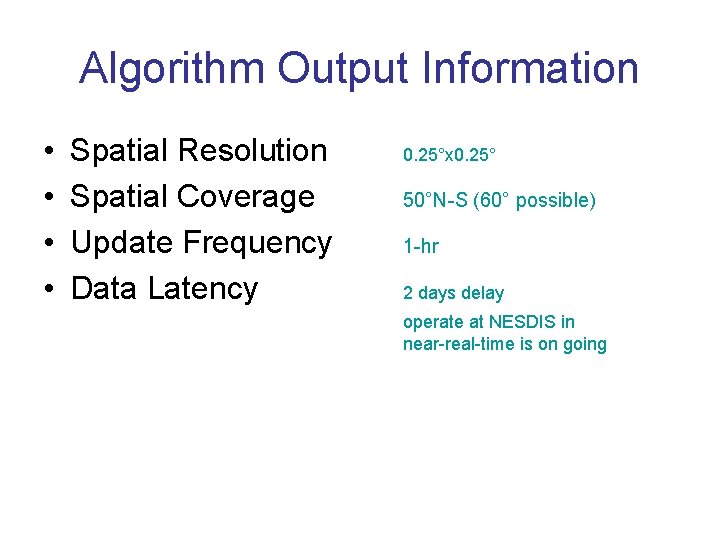
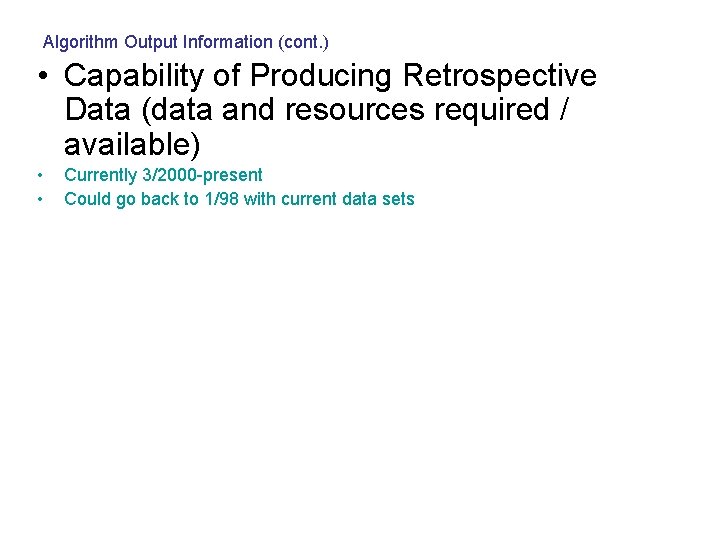
- Slides: 8
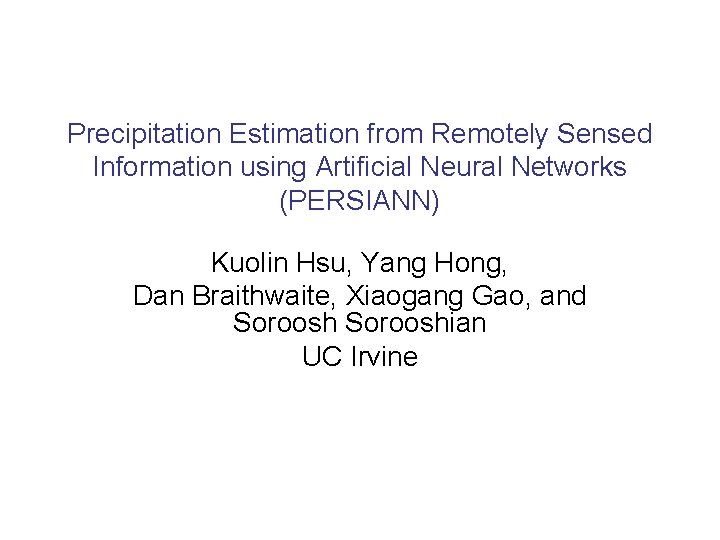
Precipitation Estimation from Remotely Sensed Information using Artificial Neural Networks (PERSIANN) Kuolin Hsu, Yang Hong, Dan Braithwaite, Xiaogang Gao, and Sorooshian UC Irvine
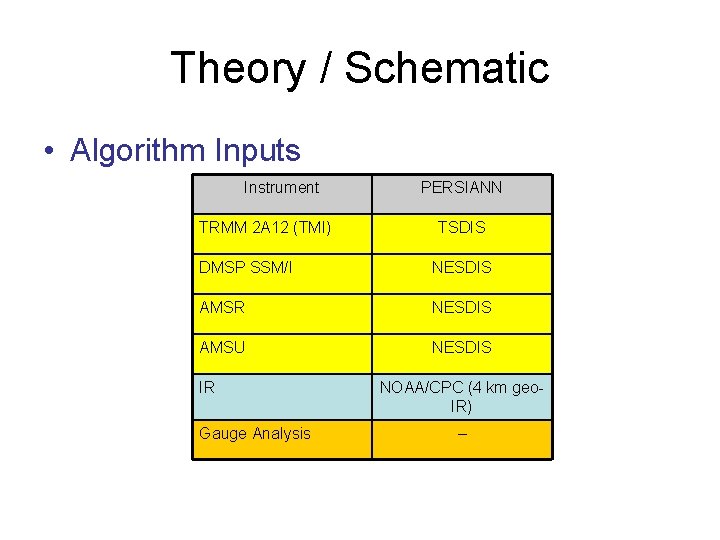
Theory / Schematic • Algorithm Inputs Instrument TRMM 2 A 12 (TMI) PERSIANN TSDIS DMSP SSM/I NESDIS AMSR NESDIS AMSU NESDIS IR Gauge Analysis NOAA/CPC (4 km geo. IR) –
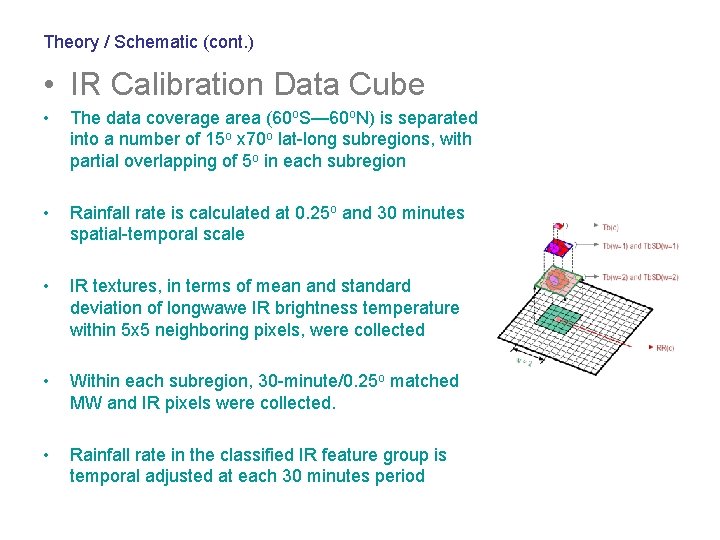
Theory / Schematic (cont. ) • IR Calibration Data Cube • The data coverage area (60 o. S— 60 o. N) is separated into a number of 15 o x 70 o lat-long subregions, with partial overlapping of 5 o in each subregion • Rainfall rate is calculated at 0. 25 o and 30 minutes spatial-temporal scale • IR textures, in terms of mean and standard deviation of longwawe IR brightness temperature within 5 x 5 neighboring pixels, were collected • Within each subregion, 30 -minute/0. 25 o matched MW and IR pixels were collected. • Rainfall rate in the classified IR feature group is temporal adjusted at each 30 minutes period
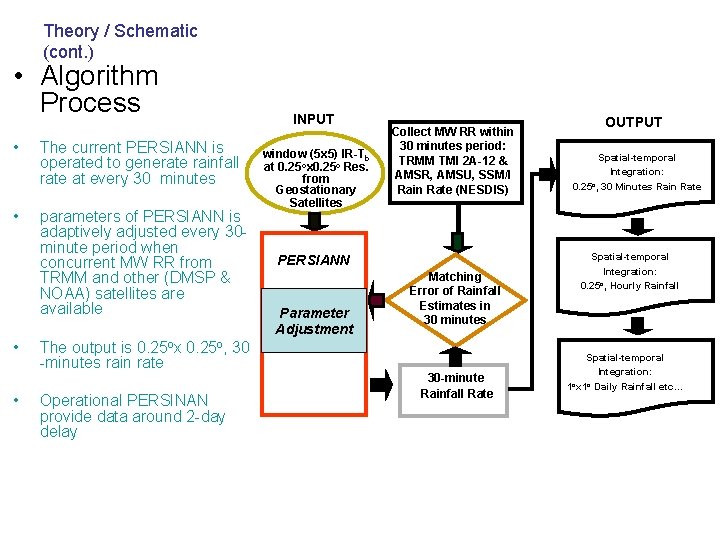
Theory / Schematic (cont. ) • Algorithm Process • The current PERSIANN is operated to generate rainfall rate at every 30 minutes • parameters of PERSIANN is adaptively adjusted every 30 minute period when concurrent MW RR from TRMM and other (DMSP & NOAA) satellites are available • • INPUT window (5 x 5) IR-Tb at 0. 25 ox 0. 25 o Res. from Geostationary Satellites Collect MW RR within 30 minutes period: TRMM TMI 2 A-12 & AMSR, AMSU, SSM/I Rain Rate (NESDIS) PERSIANN Parameter Adjustment Matching Error of Rainfall Estimates in 30 minutes The output is 0. 25 ox 0. 25 o, 30 -minutes rain rate Operational PERSINAN provide data around 2 -day delay 30 -minute Rainfall Rate OUTPUT Spatial-temporal Integration: o 0. 25 , 30 Minutes Rain Rate Spatial-temporal Integration: o 0. 25 , Hourly Rainfall Spatial-temporal Integration: o o 1 x 1 Daily Rainfall etc…
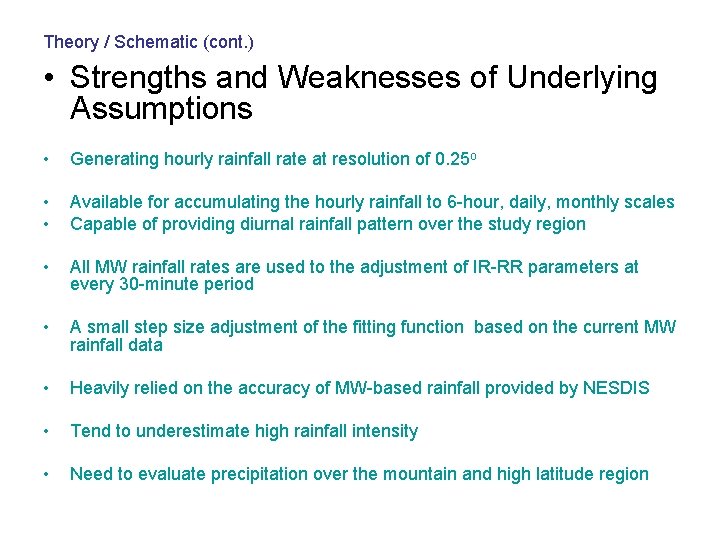
Theory / Schematic (cont. ) • Strengths and Weaknesses of Underlying Assumptions • Generating hourly rainfall rate at resolution of 0. 25 o • • Available for accumulating the hourly rainfall to 6 -hour, daily, monthly scales Capable of providing diurnal rainfall pattern over the study region • All MW rainfall rates are used to the adjustment of IR-RR parameters at every 30 -minute period • A small step size adjustment of the fitting function based on the current MW rainfall data • Heavily relied on the accuracy of MW-based rainfall provided by NESDIS • Tend to underestimate high rainfall intensity • Need to evaluate precipitation over the mountain and high latitude region
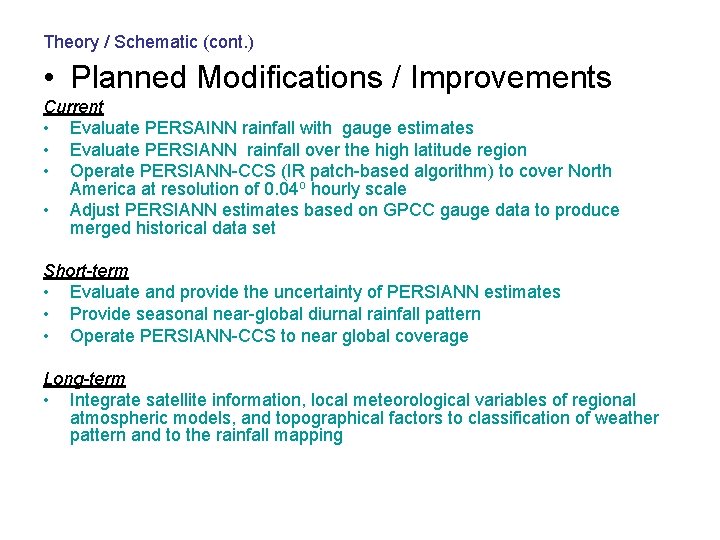
Theory / Schematic (cont. ) • Planned Modifications / Improvements Current • Evaluate PERSAINN rainfall with gauge estimates • Evaluate PERSIANN rainfall over the high latitude region • Operate PERSIANN-CCS (IR patch-based algorithm) to cover North America at resolution of 0. 04 o hourly scale • Adjust PERSIANN estimates based on GPCC gauge data to produce merged historical data set Short-term • Evaluate and provide the uncertainty of PERSIANN estimates • Provide seasonal near-global diurnal rainfall pattern • Operate PERSIANN-CCS to near global coverage Long-term • Integrate satellite information, local meteorological variables of regional atmospheric models, and topographical factors to classification of weather pattern and to the rainfall mapping
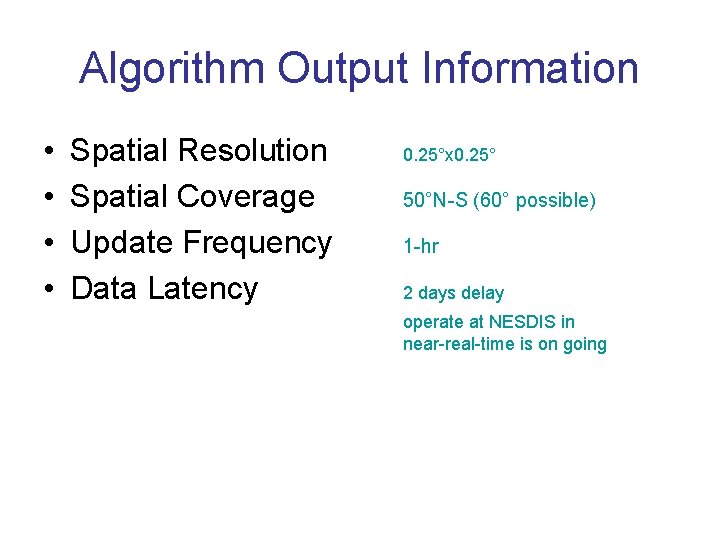
Algorithm Output Information • • Spatial Resolution Spatial Coverage Update Frequency Data Latency 0. 25°x 0. 25° 50°N-S (60° possible) 1 -hr 2 days delay operate at NESDIS in near-real-time is on going
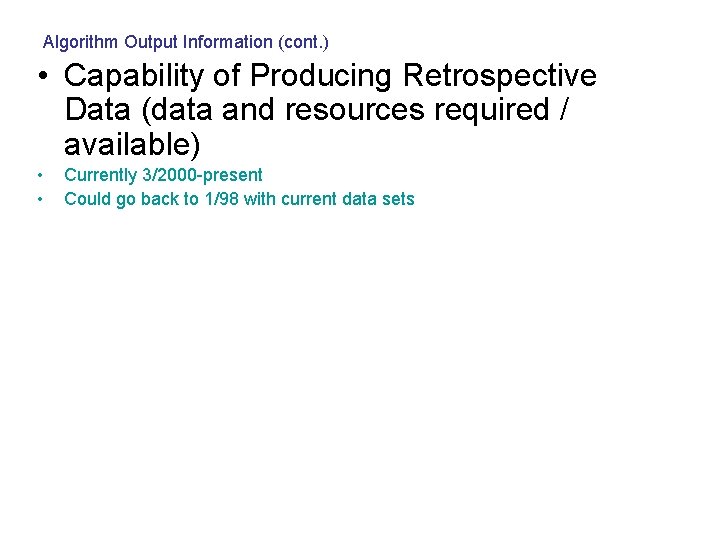
Algorithm Output Information (cont. ) • Capability of Producing Retrospective Data (data and resources required / available) • • Currently 3/2000 -present Could go back to 1/98 with current data sets