Integrating local crowdsourced and remotely sensed data to
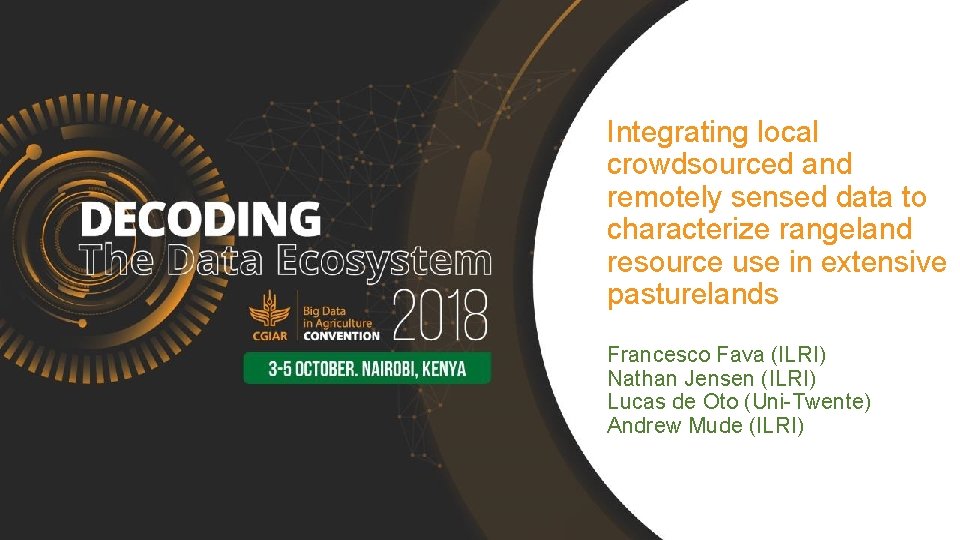
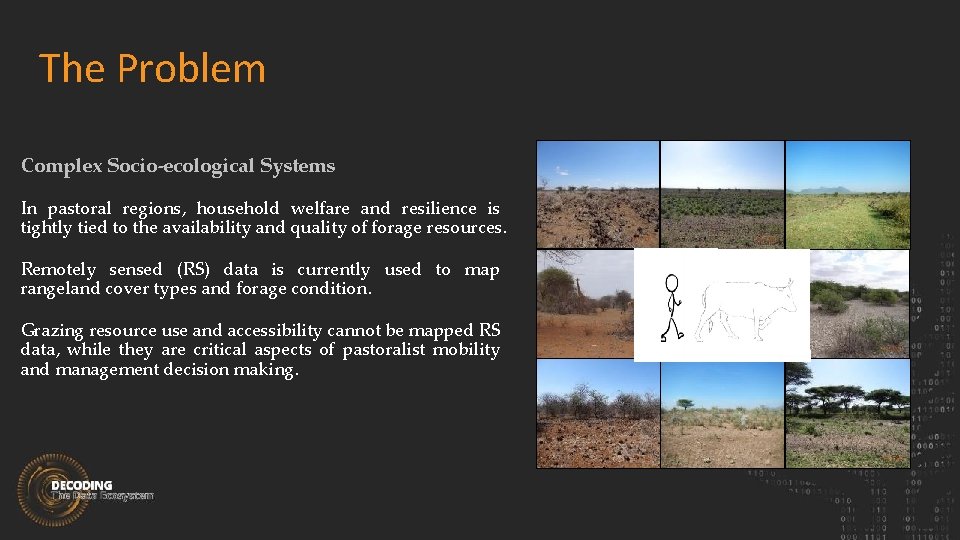
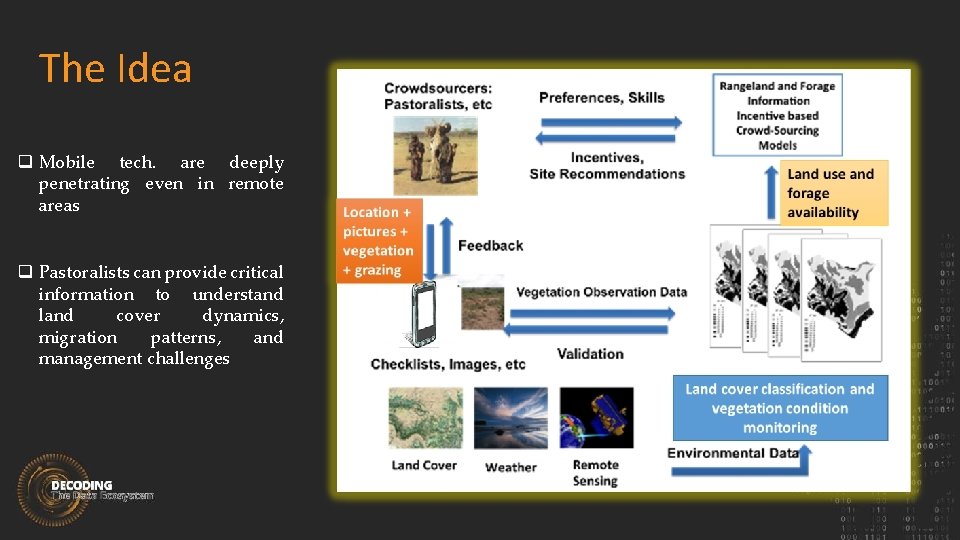
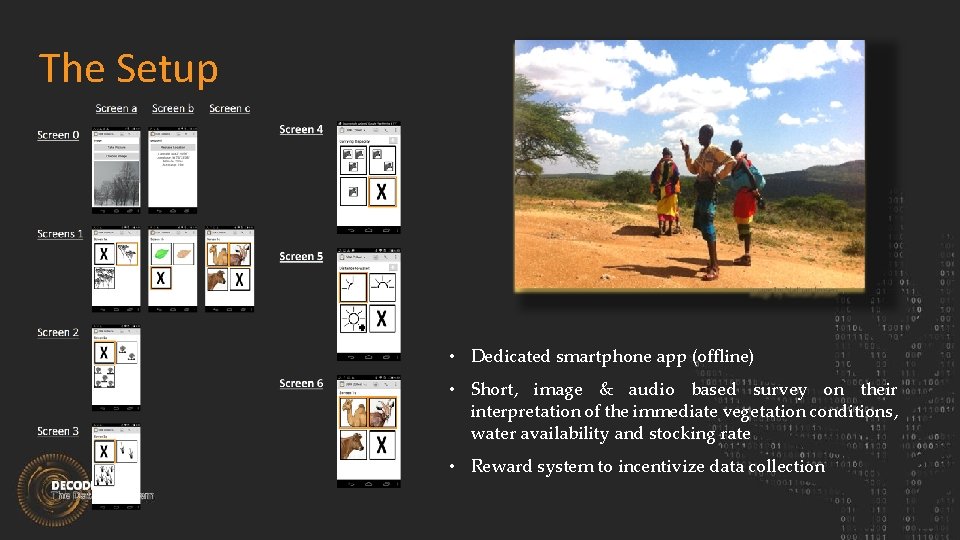
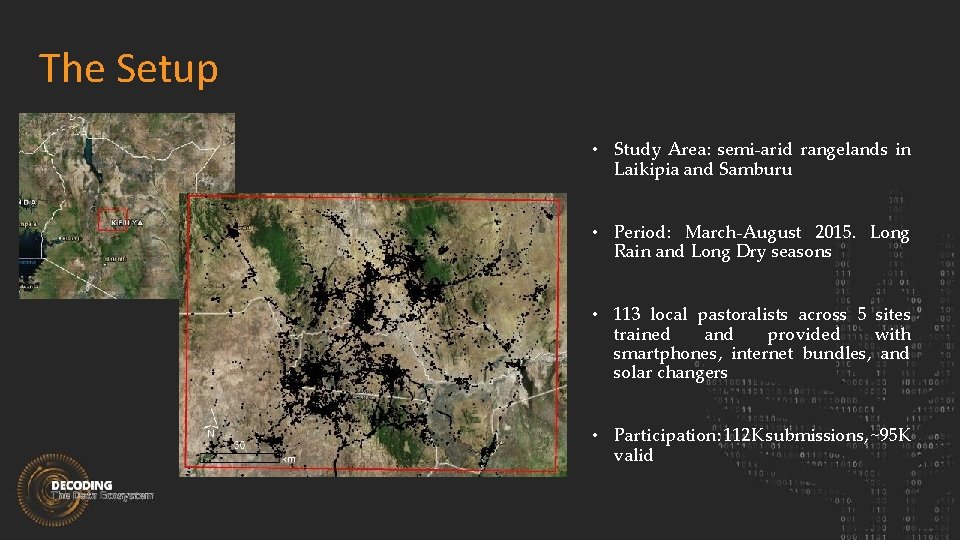
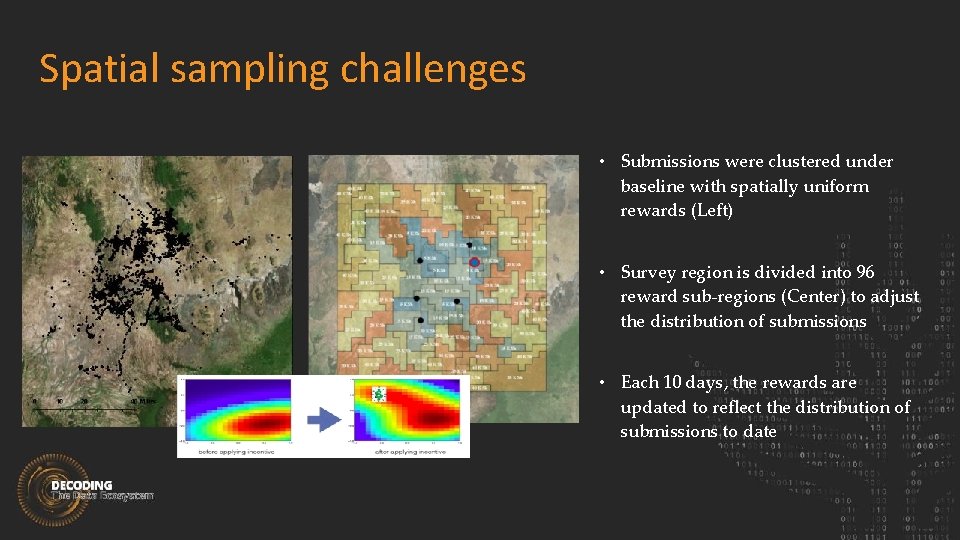
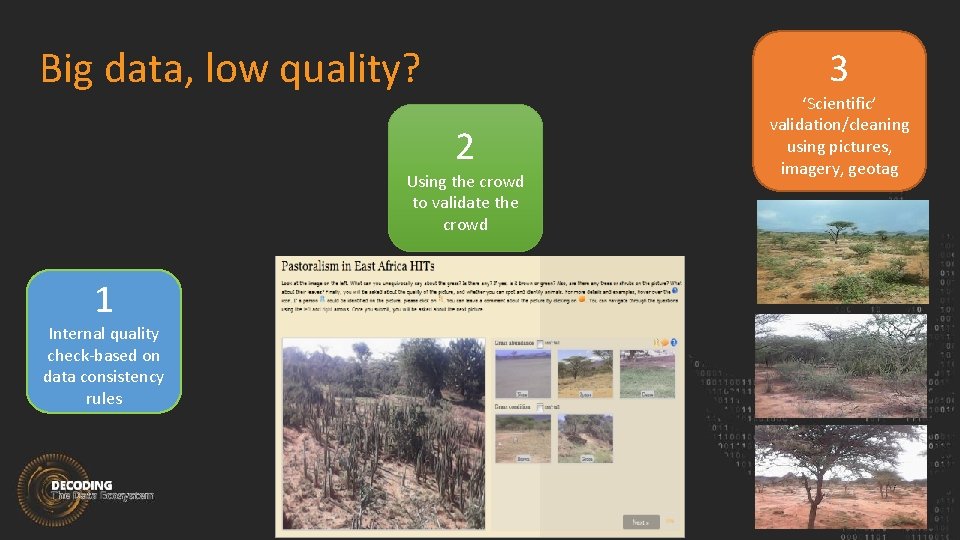
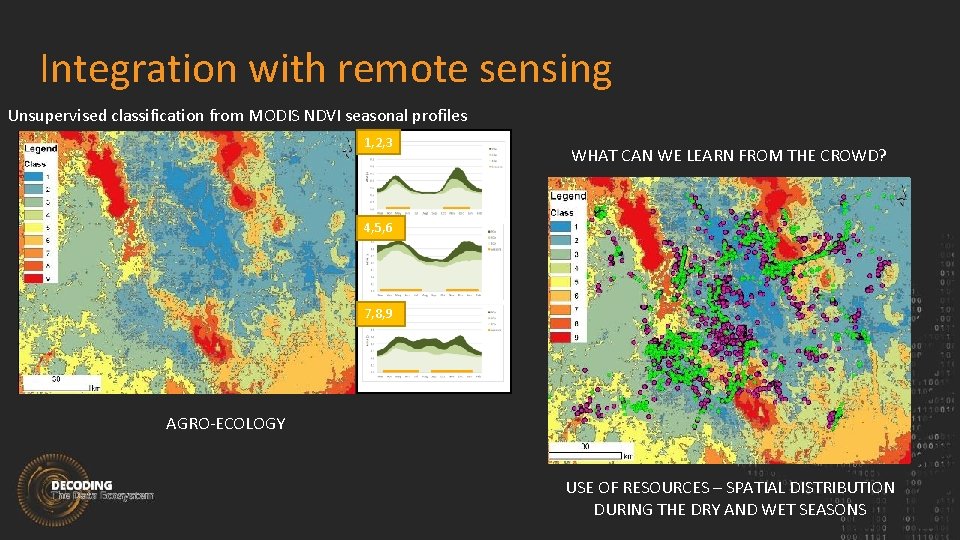
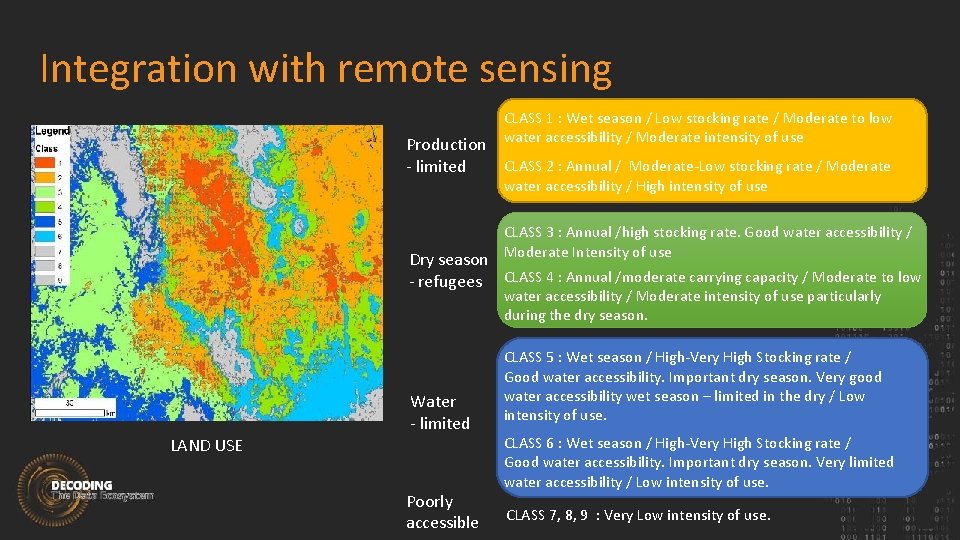
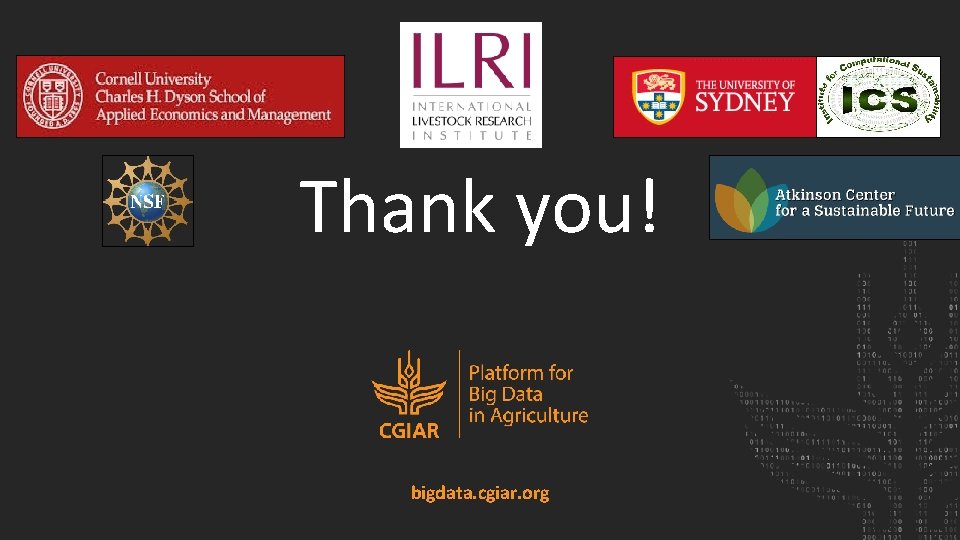
- Slides: 10
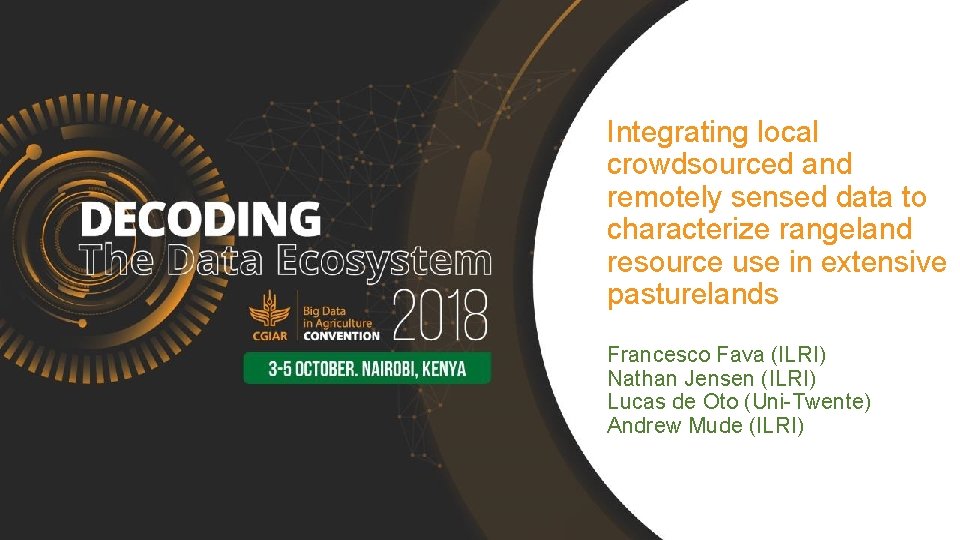
Integrating local crowdsourced and remotely sensed data to characterize rangeland resource use in extensive pasturelands Francesco Fava (ILRI) Nathan Jensen (ILRI) Lucas de Oto (Uni-Twente) Andrew Mude (ILRI)
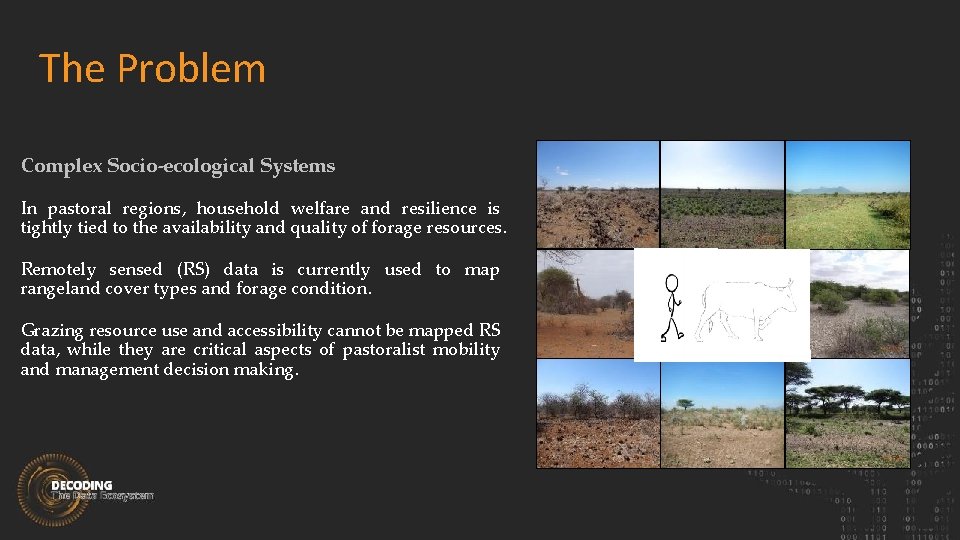
The Problem Complex Socio-ecological Systems In pastoral regions, household welfare and resilience is tightly tied to the availability and quality of forage resources. Remotely sensed (RS) data is currently used to map rangeland cover types and forage condition. Grazing resource use and accessibility cannot be mapped RS data, while they are critical aspects of pastoralist mobility and management decision making.
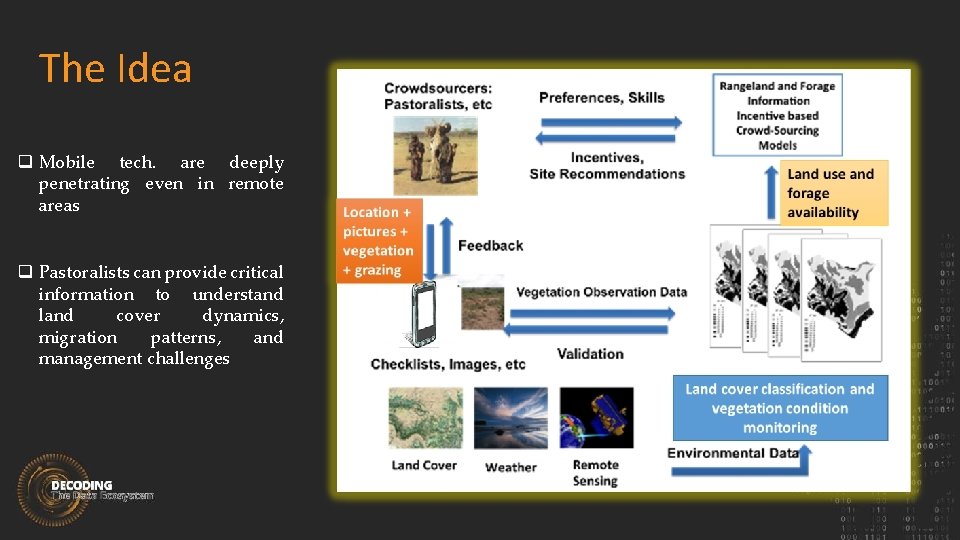
The Idea q Mobile tech. are deeply penetrating even in remote areas q Pastoralists can provide critical information to understand land cover dynamics, migration patterns, and management challenges
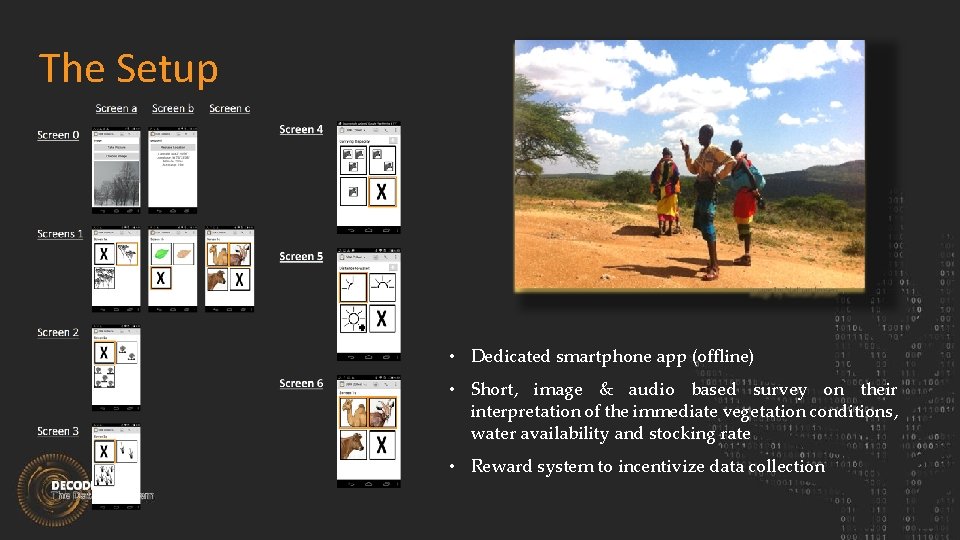
The Setup • Dedicated smartphone app (offline) • Short, image & audio based survey on their interpretation of the immediate vegetation conditions, water availability and stocking rate • Reward system to incentivize data collection
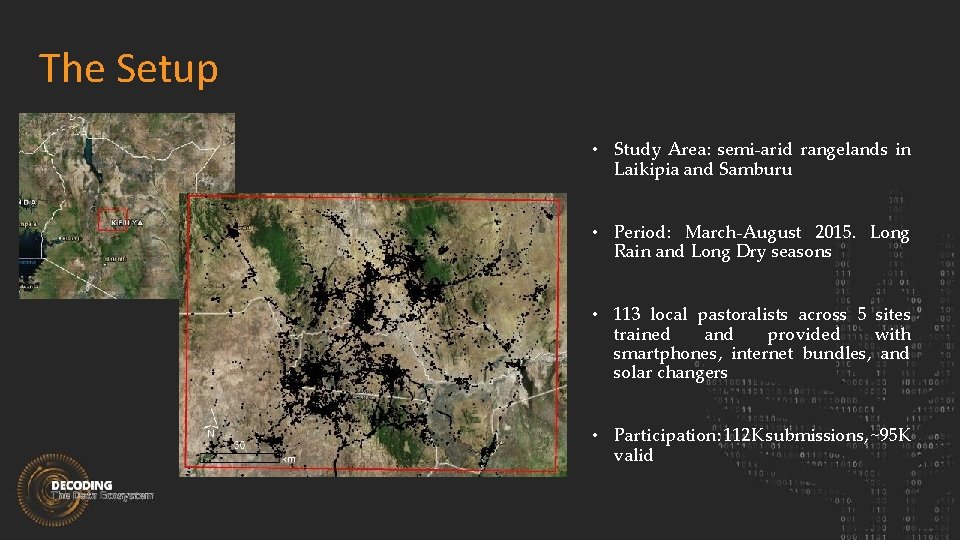
The Setup • Study Area: semi-arid rangelands in Laikipia and Samburu • Period: March-August 2015. Long Rain and Long Dry seasons • 113 local pastoralists across 5 sites trained and provided with smartphones, internet bundles, and solar changers • Participation: 112 K submissions, ~95 K valid
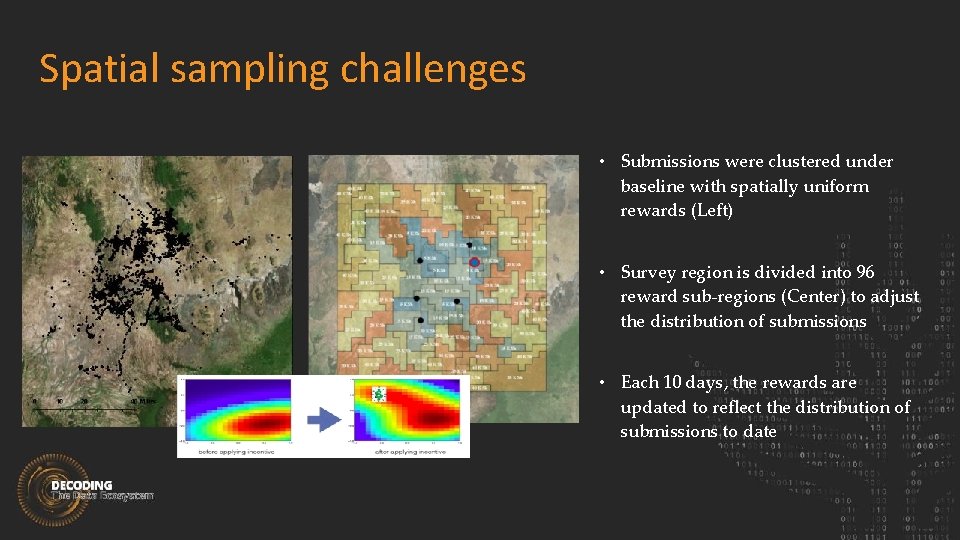
Spatial sampling challenges • Submissions were clustered under baseline with spatially uniform rewards (Left) • Survey region is divided into 96 reward sub-regions (Center) to adjust the distribution of submissions • Each 10 days, the rewards are updated to reflect the distribution of submissions to date
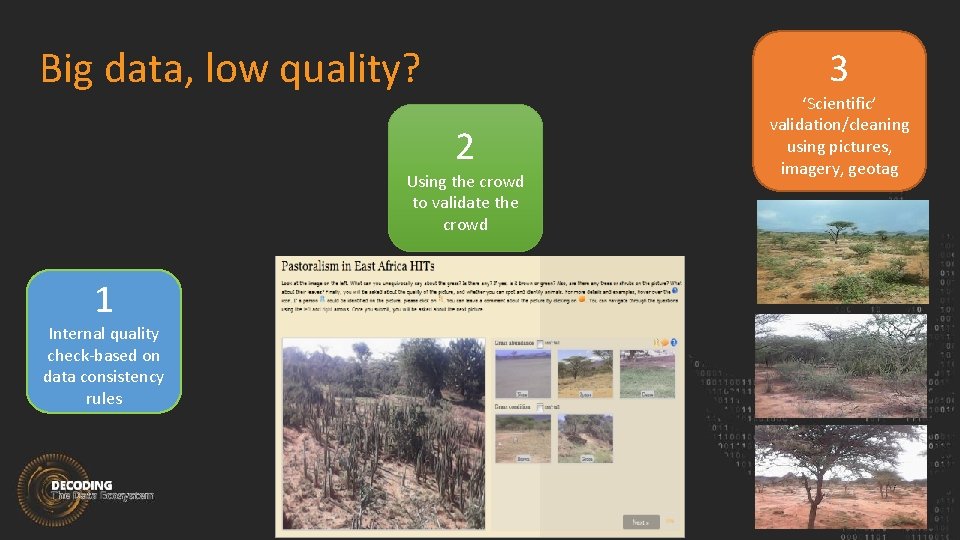
Big data, low quality? 3 2 Using the crowd to validate the crowd 1 Internal quality check-based on data consistency rules ‘Scientific’ validation/cleaning using pictures, imagery, geotag
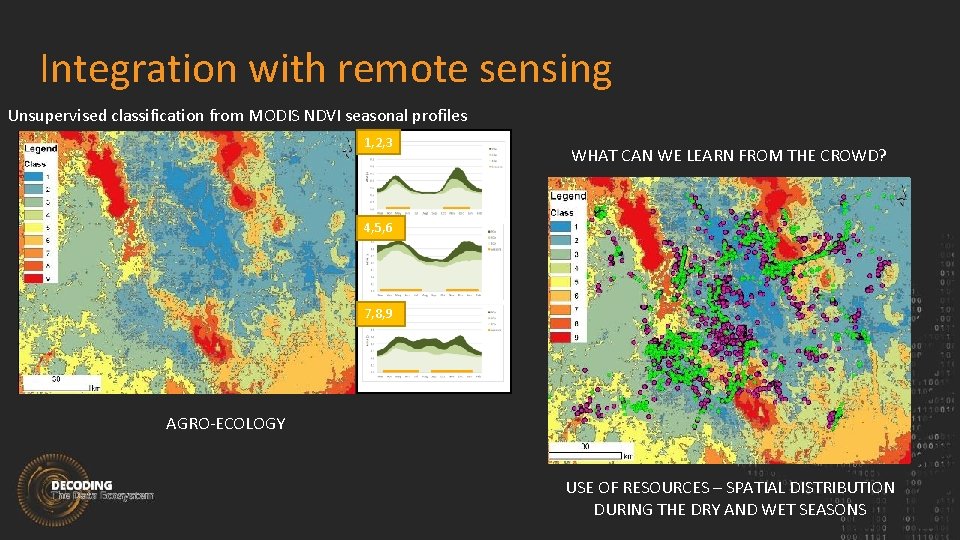
Integration with remote sensing Unsupervised classification from MODIS NDVI seasonal profiles 1, 2, 3 WHAT CAN WE LEARN FROM THE CROWD? 4, 5, 6 7, 8, 9 AGRO-ECOLOGY WET DRY SEASON USE OF RESOURCES – SPATIAL DISTRIBUTION DURING THE DRY AND WET SEASONS
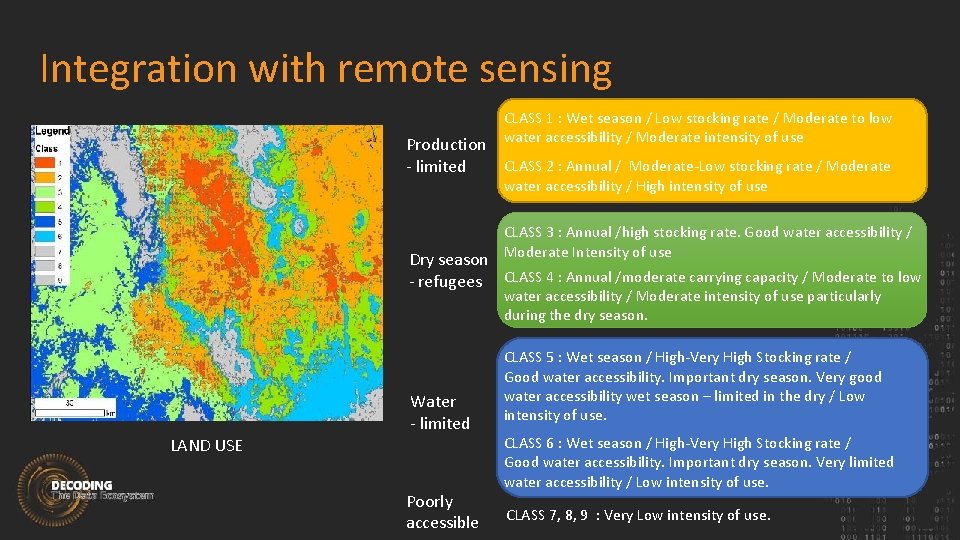
Integration with remote sensing CLASS 1 : Wet season / Low stocking rate / Moderate to low water accessibility / Moderate intensity of use Production CLASS 2 : Annual / Moderate-Low stocking rate / Moderate - limited water accessibility / High intensity of use CLASS 3 : Annual /high stocking rate. Good water accessibility / Moderate Intensity of use Dry season - refugees CLASS 4 : Annual /moderate carrying capacity / Moderate to low water accessibility / Moderate intensity of use particularly during the dry season. Water - limited LAND USE Poorly accessible CLASS 5 : Wet season / High-Very High Stocking rate / Good water accessibility. Important dry season. Very good water accessibility wet season – limited in the dry / Low intensity of use. CLASS 6 : Wet season / High-Very High Stocking rate / Good water accessibility. Important dry season. Very limited water accessibility / Low intensity of use. CLASS 7, 8, 9 : Very Low intensity of use.
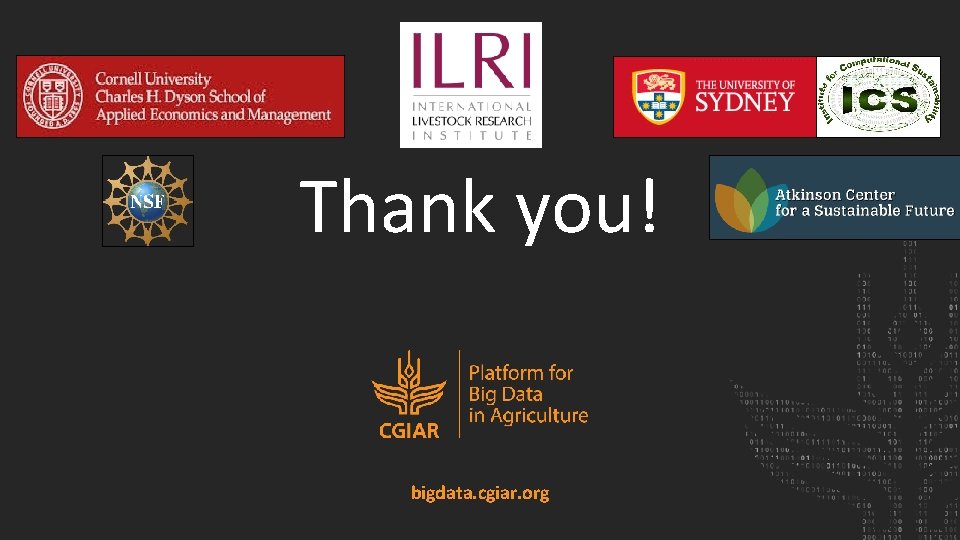
Thank you! bigdata. cgiar. org
Remotely sensed data
Element of dance
Tally remote access
Crowdsourced learning
Crowdsourced funding
Local vertical local horizontal frame
A think local act local multicountry type of strategy
A "think local, act local" multidomestic type of strategy
Two drawbacks of a think local act local
Integrating classification and association rule mining
Integrating strategy and culture in internal assessment