POL 1000 Lecture 3 Methodology Sean Clark Lecturer
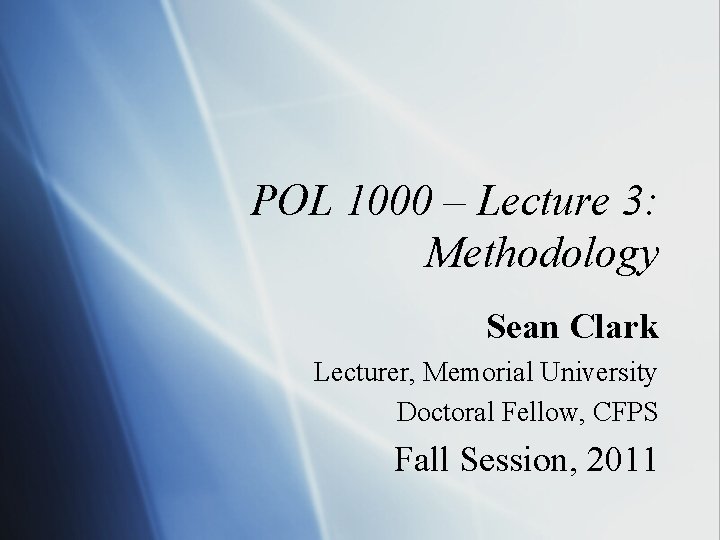
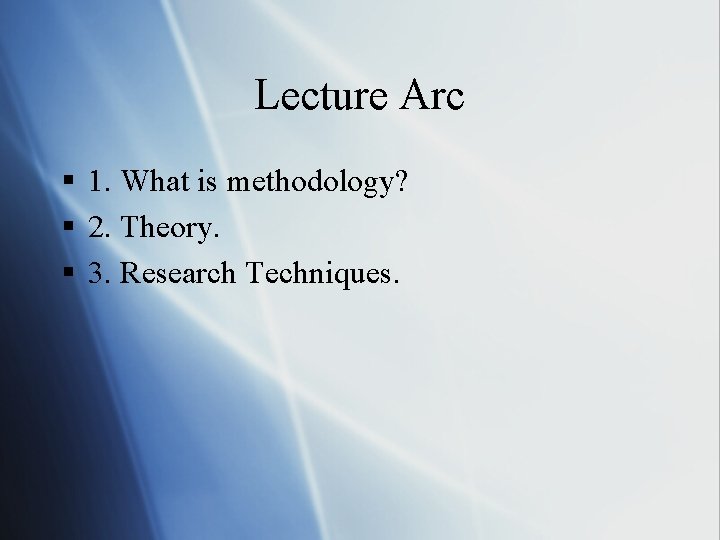
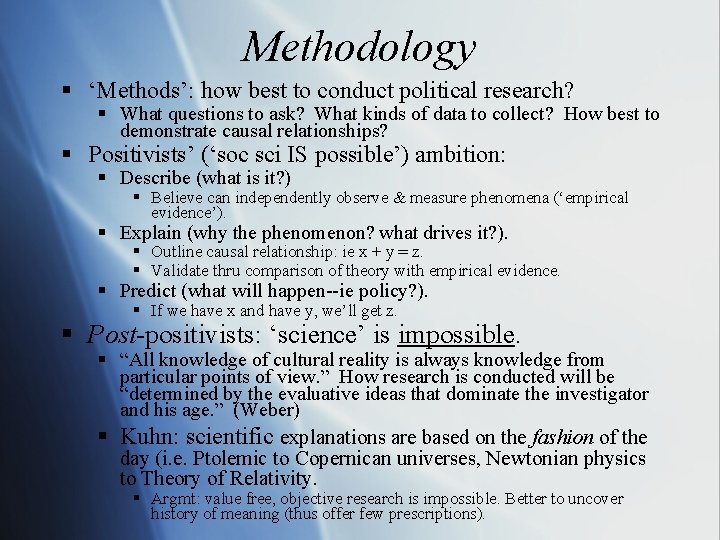
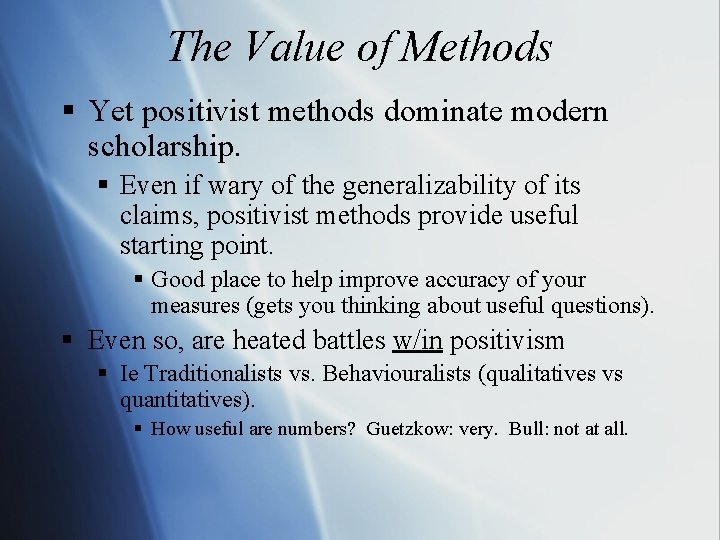
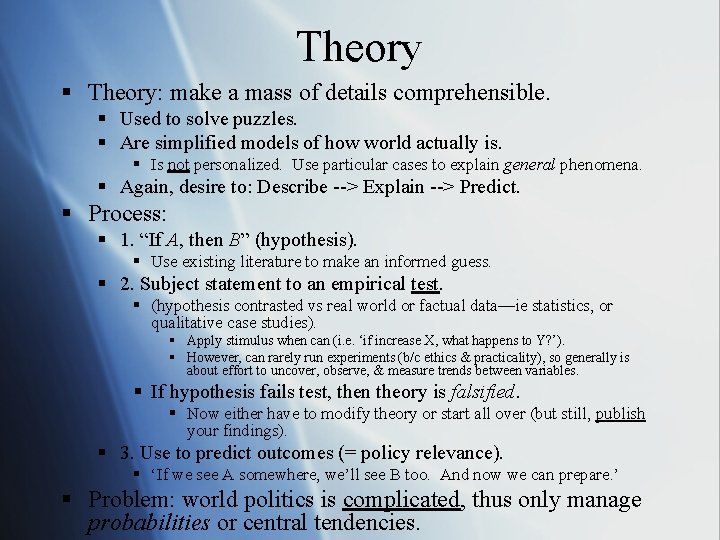
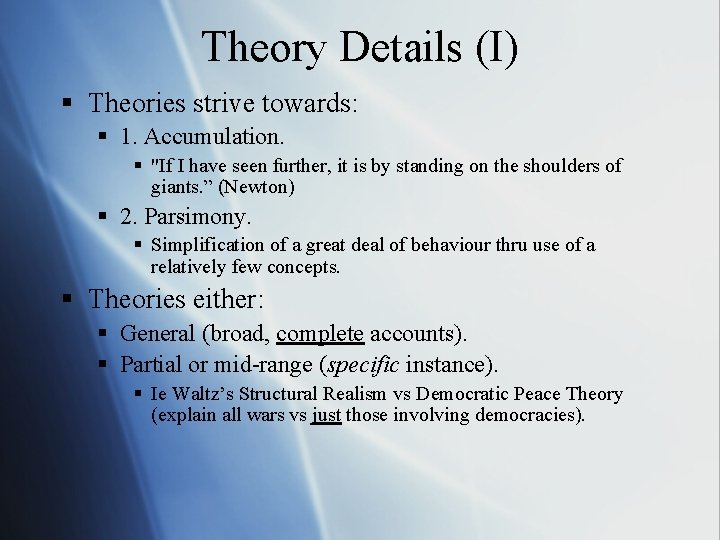
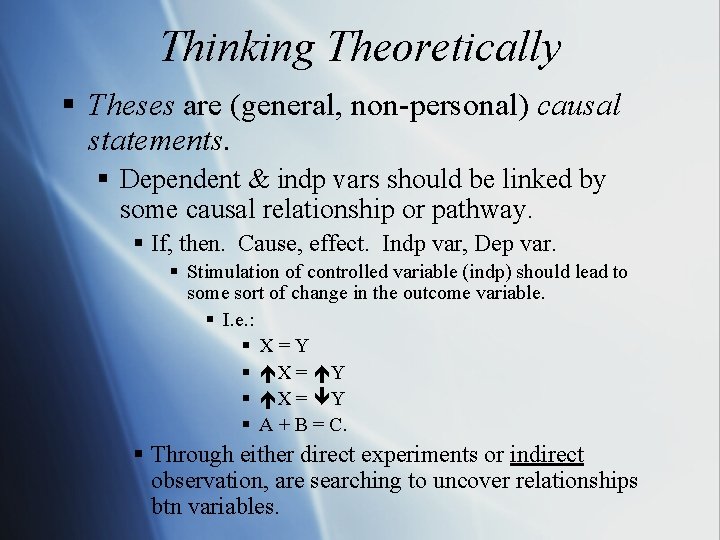
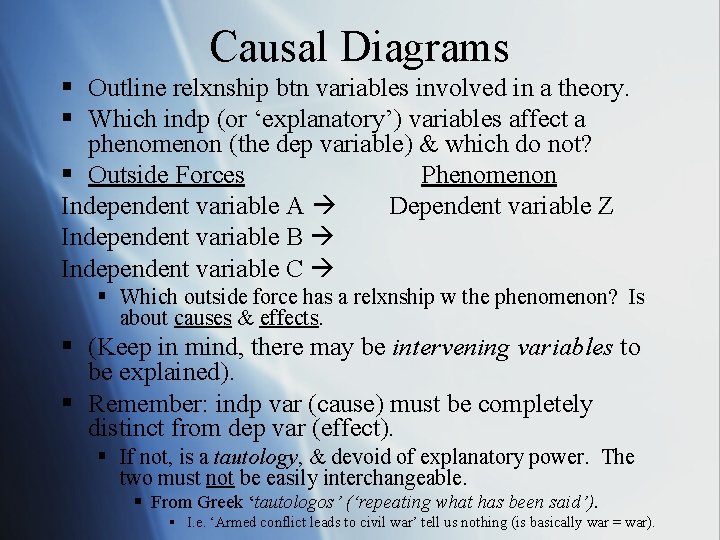
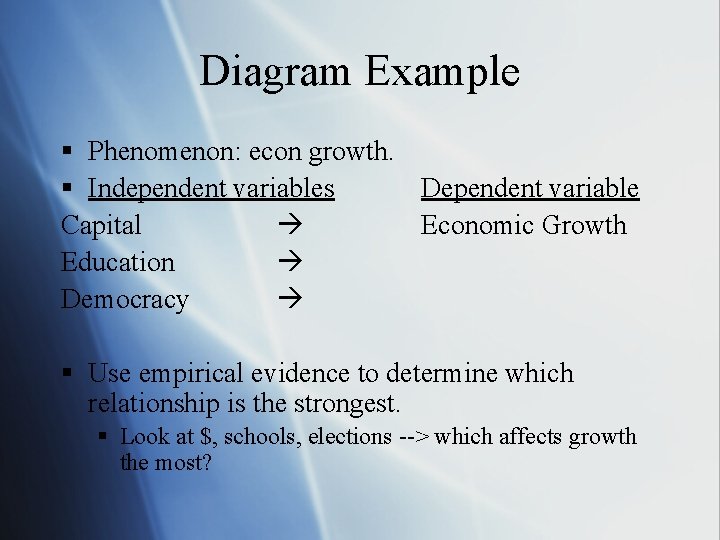
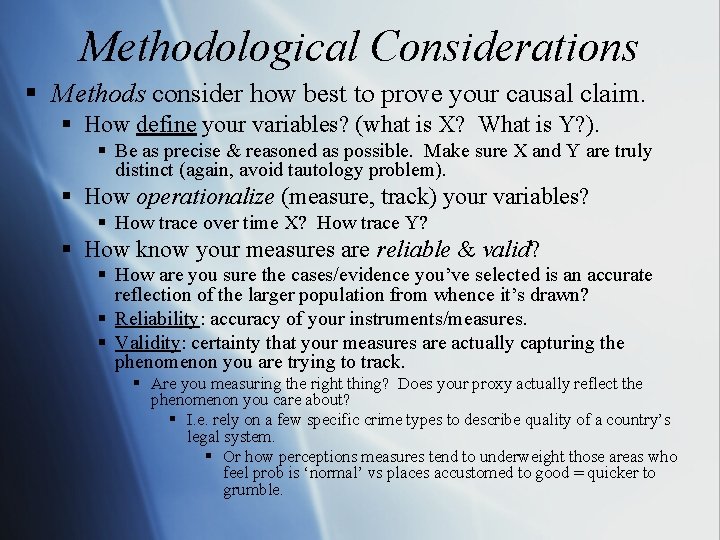
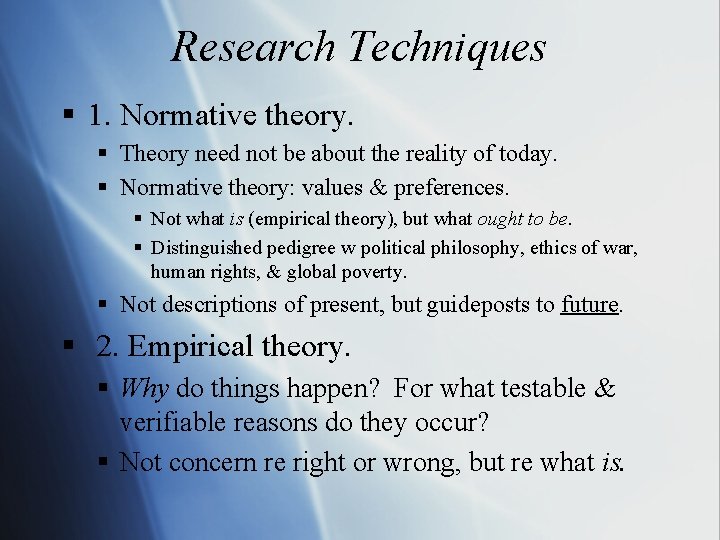
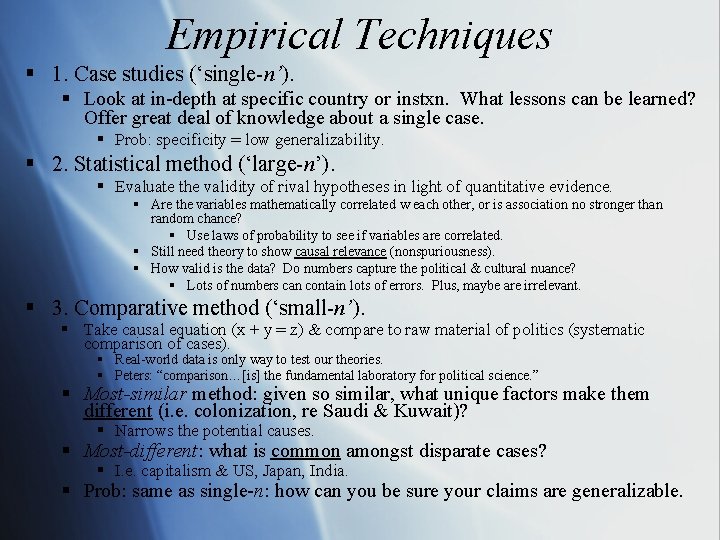
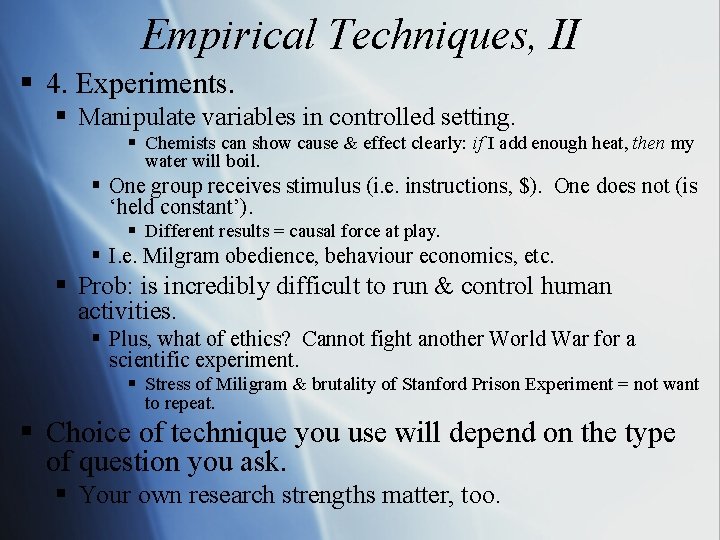
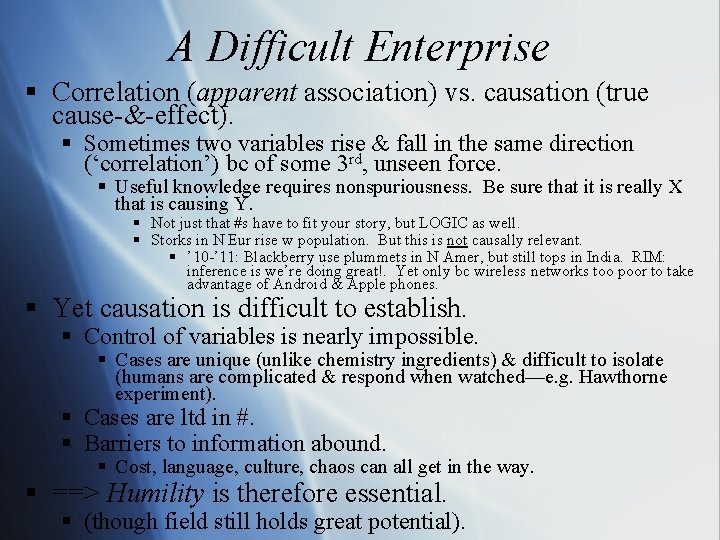
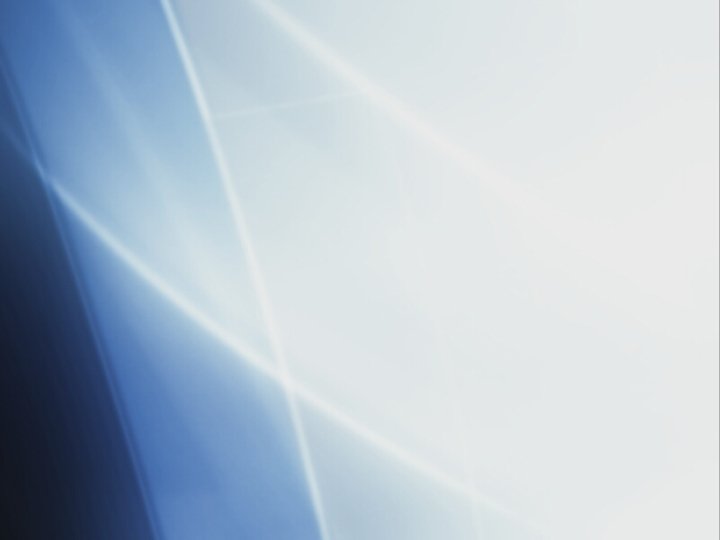
- Slides: 15
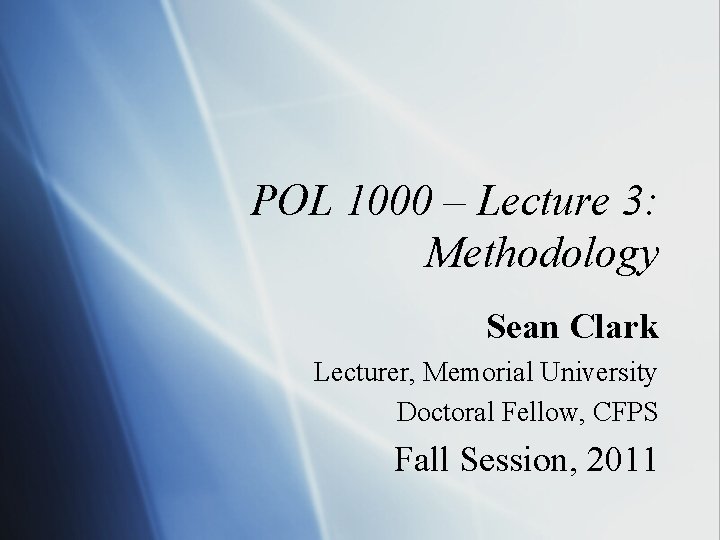
POL 1000 – Lecture 3: Methodology Sean Clark Lecturer, Memorial University Doctoral Fellow, CFPS Fall Session, 2011
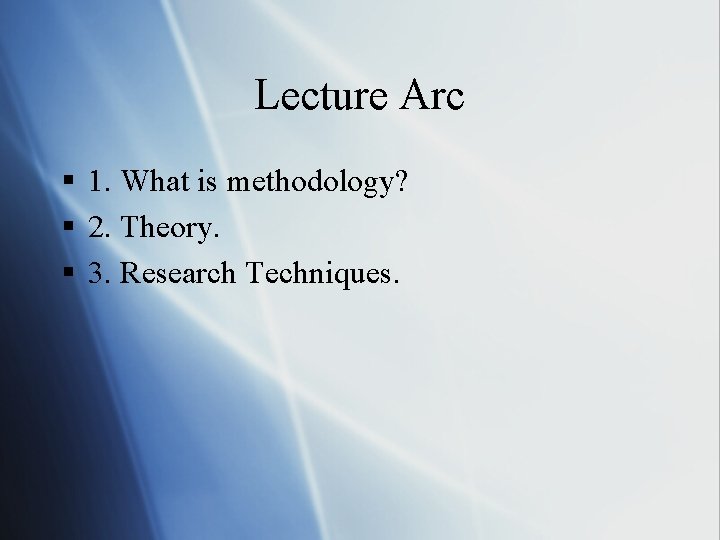
Lecture Arc § 1. What is methodology? § 2. Theory. § 3. Research Techniques.
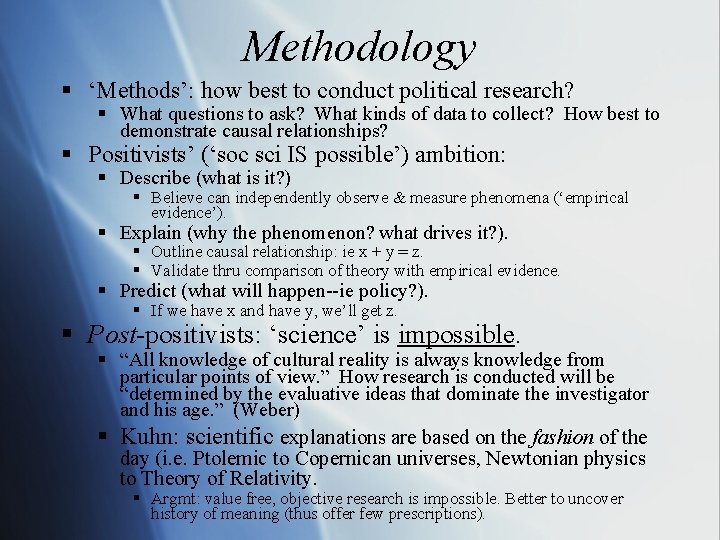
Methodology § ‘Methods’: how best to conduct political research? § What questions to ask? What kinds of data to collect? How best to demonstrate causal relationships? § Positivists’ (‘soc sci IS possible’) ambition: § Describe (what is it? ) § Believe can independently observe & measure phenomena (‘empirical evidence’). § Explain (why the phenomenon? what drives it? ). § Outline causal relationship: ie x + y = z. § Validate thru comparison of theory with empirical evidence. § Predict (what will happen--ie policy? ). § If we have x and have y, we’ll get z. § Post-positivists: ‘science’ is impossible. § “All knowledge of cultural reality is always knowledge from particular points of view. ” How research is conducted will be “determined by the evaluative ideas that dominate the investigator and his age. ” (Weber) § Kuhn: scientific explanations are based on the fashion of the day (i. e. Ptolemic to Copernican universes, Newtonian physics to Theory of Relativity. § Argmt: value free, objective research is impossible. Better to uncover history of meaning (thus offer few prescriptions).
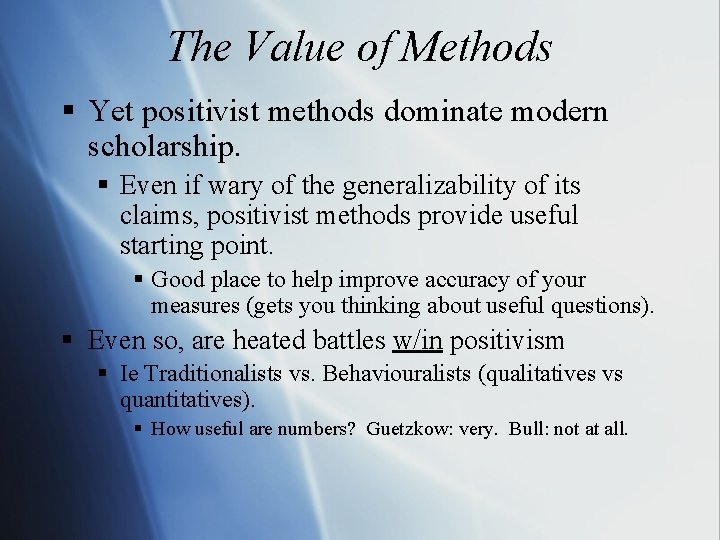
The Value of Methods § Yet positivist methods dominate modern scholarship. § Even if wary of the generalizability of its claims, positivist methods provide useful starting point. § Good place to help improve accuracy of your measures (gets you thinking about useful questions). § Even so, are heated battles w/in positivism § Ie Traditionalists vs. Behaviouralists (qualitatives vs quantitatives). § How useful are numbers? Guetzkow: very. Bull: not at all.
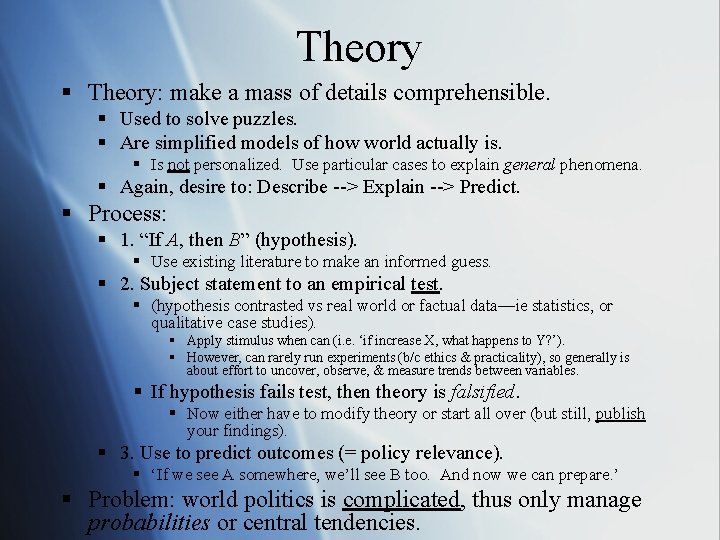
Theory § Theory: make a mass of details comprehensible. § Used to solve puzzles. § Are simplified models of how world actually is. § Is not personalized. Use particular cases to explain general phenomena. § Again, desire to: Describe --> Explain --> Predict. § Process: § 1. “If A, then B” (hypothesis). § Use existing literature to make an informed guess. § 2. Subject statement to an empirical test. § (hypothesis contrasted vs real world or factual data—ie statistics, or qualitative case studies). § Apply stimulus when can (i. e. ‘if increase X, what happens to Y? ’). § However, can rarely run experiments (b/c ethics & practicality), so generally is about effort to uncover, observe, & measure trends between variables. § If hypothesis fails test, then theory is falsified. § Now either have to modify theory or start all over (but still, publish your findings). § 3. Use to predict outcomes (= policy relevance). § ‘If we see A somewhere, we’ll see B too. And now we can prepare. ’ § Problem: world politics is complicated, thus only manage probabilities or central tendencies.
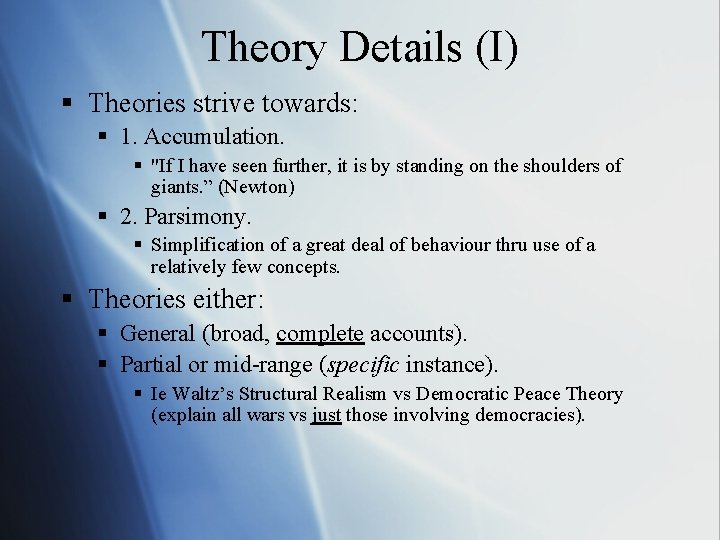
Theory Details (I) § Theories strive towards: § 1. Accumulation. § "If I have seen further, it is by standing on the shoulders of giants. ” (Newton) § 2. Parsimony. § Simplification of a great deal of behaviour thru use of a relatively few concepts. § Theories either: § General (broad, complete accounts). § Partial or mid-range (specific instance). § Ie Waltz’s Structural Realism vs Democratic Peace Theory (explain all wars vs just those involving democracies).
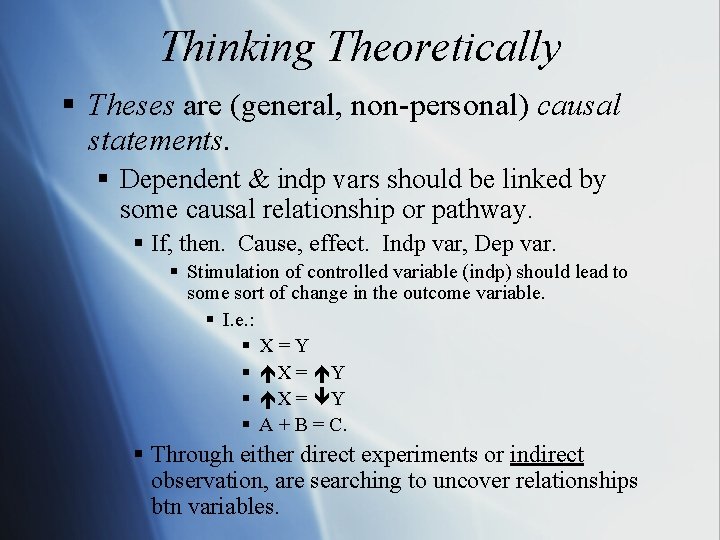
Thinking Theoretically § Theses are (general, non-personal) causal statements. § Dependent & indp vars should be linked by some causal relationship or pathway. § If, then. Cause, effect. Indp var, Dep var. § Stimulation of controlled variable (indp) should lead to some sort of change in the outcome variable. § I. e. : § X=Y § X = Y § A + B = C. § Through either direct experiments or indirect observation, are searching to uncover relationships btn variables.
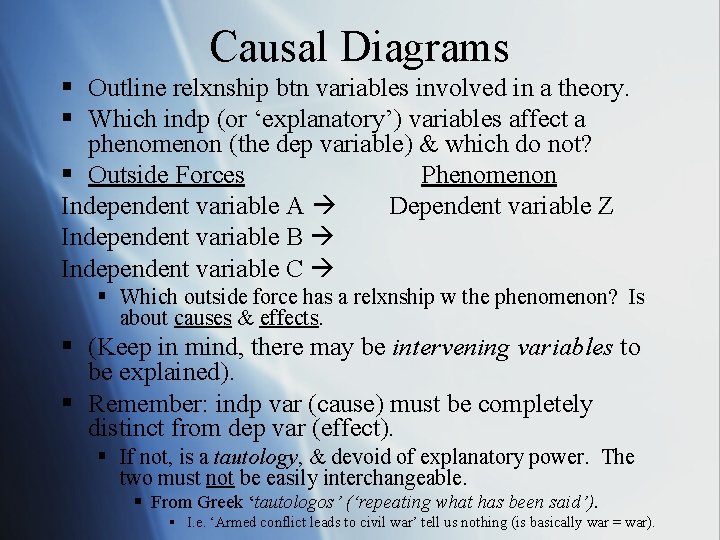
Causal Diagrams § Outline relxnship btn variables involved in a theory. § Which indp (or ‘explanatory’) variables affect a phenomenon (the dep variable) & which do not? § Outside Forces Phenomenon Independent variable A Dependent variable Z Independent variable B Independent variable C § Which outside force has a relxnship w the phenomenon? Is about causes & effects. § (Keep in mind, there may be intervening variables to be explained). § Remember: indp var (cause) must be completely distinct from dep var (effect). § If not, is a tautology, & devoid of explanatory power. The two must not be easily interchangeable. § From Greek ‘tautologos’ (‘repeating what has been said’). § I. e. ‘Armed conflict leads to civil war’ tell us nothing (is basically war = war).
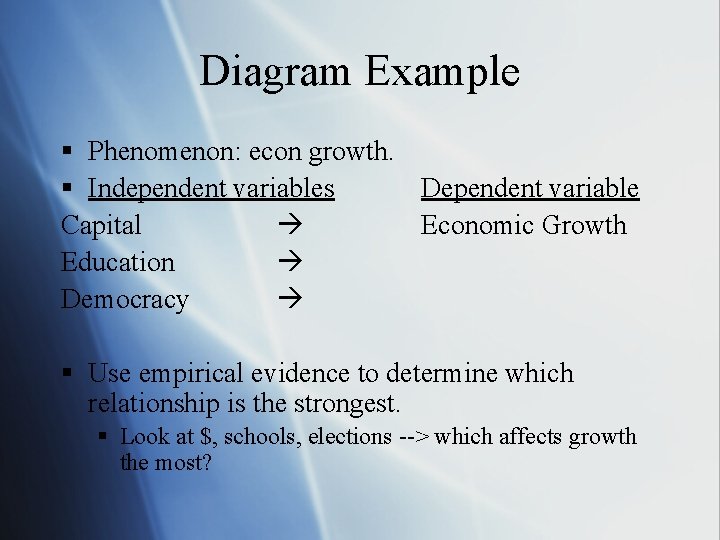
Diagram Example § Phenomenon: econ growth. § Independent variables Dependent variable Capital Economic Growth Education Democracy § Use empirical evidence to determine which relationship is the strongest. § Look at $, schools, elections --> which affects growth the most?
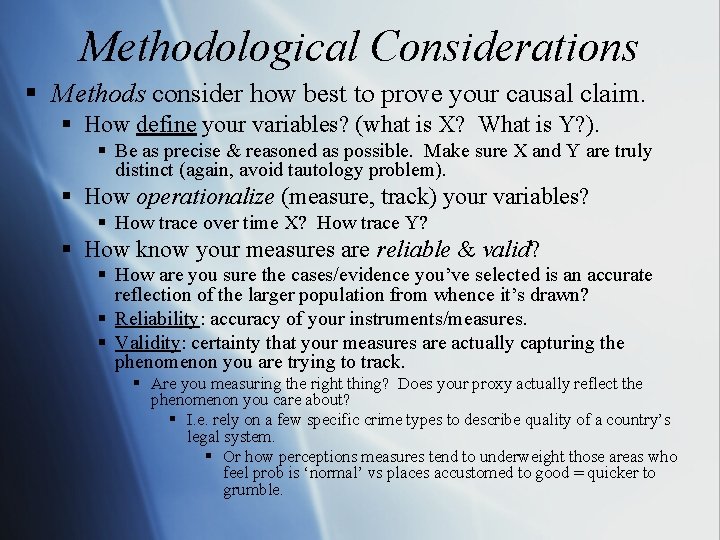
Methodological Considerations § Methods consider how best to prove your causal claim. § How define your variables? (what is X? What is Y? ). § Be as precise & reasoned as possible. Make sure X and Y are truly distinct (again, avoid tautology problem). § How operationalize (measure, track) your variables? § How trace over time X? How trace Y? § How know your measures are reliable & valid? § How are you sure the cases/evidence you’ve selected is an accurate reflection of the larger population from whence it’s drawn? § Reliability: accuracy of your instruments/measures. § Validity: certainty that your measures are actually capturing the phenomenon you are trying to track. § Are you measuring the right thing? Does your proxy actually reflect the phenomenon you care about? § I. e. rely on a few specific crime types to describe quality of a country’s legal system. § Or how perceptions measures tend to underweight those areas who feel prob is ‘normal’ vs places accustomed to good = quicker to grumble.
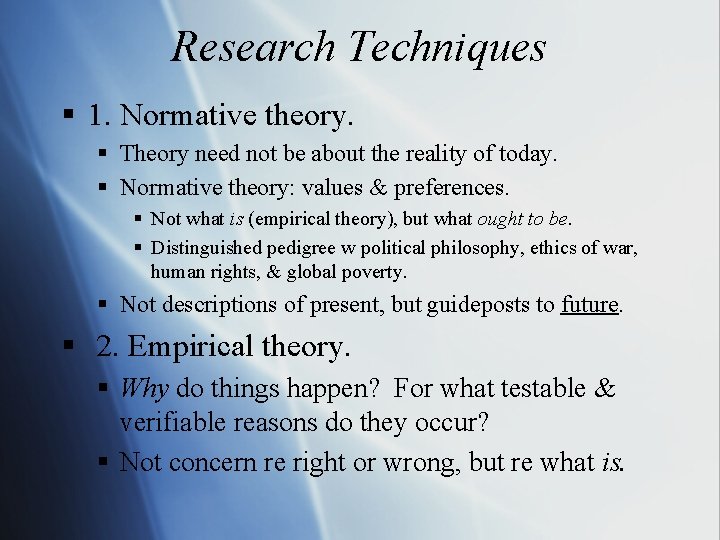
Research Techniques § 1. Normative theory. § Theory need not be about the reality of today. § Normative theory: values & preferences. § Not what is (empirical theory), but what ought to be. § Distinguished pedigree w political philosophy, ethics of war, human rights, & global poverty. § Not descriptions of present, but guideposts to future. § 2. Empirical theory. § Why do things happen? For what testable & verifiable reasons do they occur? § Not concern re right or wrong, but re what is.
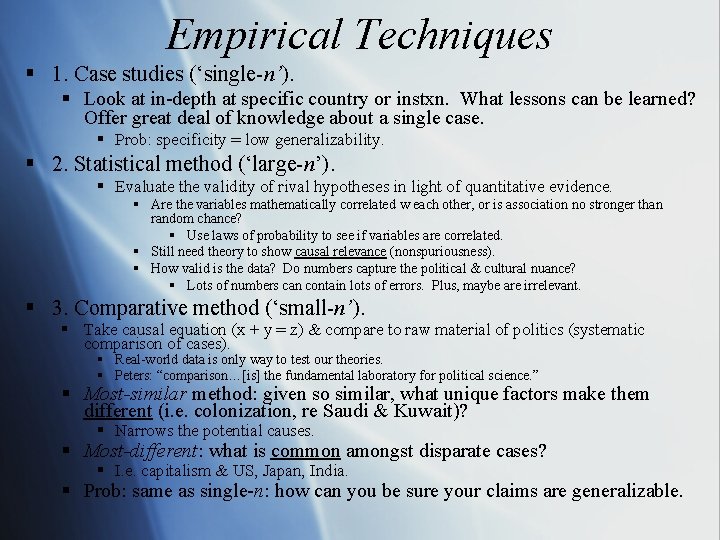
Empirical Techniques § 1. Case studies (‘single-n’). § Look at in-depth at specific country or instxn. What lessons can be learned? Offer great deal of knowledge about a single case. § Prob: specificity = low generalizability. § 2. Statistical method (‘large-n’). § Evaluate the validity of rival hypotheses in light of quantitative evidence. § Are the variables mathematically correlated w each other, or is association no stronger than random chance? § Use laws of probability to see if variables are correlated. § Still need theory to show causal relevance (nonspuriousness). § How valid is the data? Do numbers capture the political & cultural nuance? § Lots of numbers can contain lots of errors. Plus, maybe are irrelevant. § 3. Comparative method (‘small-n’). § Take causal equation (x + y = z) & compare to raw material of politics (systematic comparison of cases). § Real-world data is only way to test our theories. § Peters: “comparison…[is] the fundamental laboratory for political science. ” § Most-similar method: given so similar, what unique factors make them different (i. e. colonization, re Saudi & Kuwait)? § Narrows the potential causes. § Most-different: what is common amongst disparate cases? § I. e. capitalism & US, Japan, India. § Prob: same as single-n: how can you be sure your claims are generalizable.
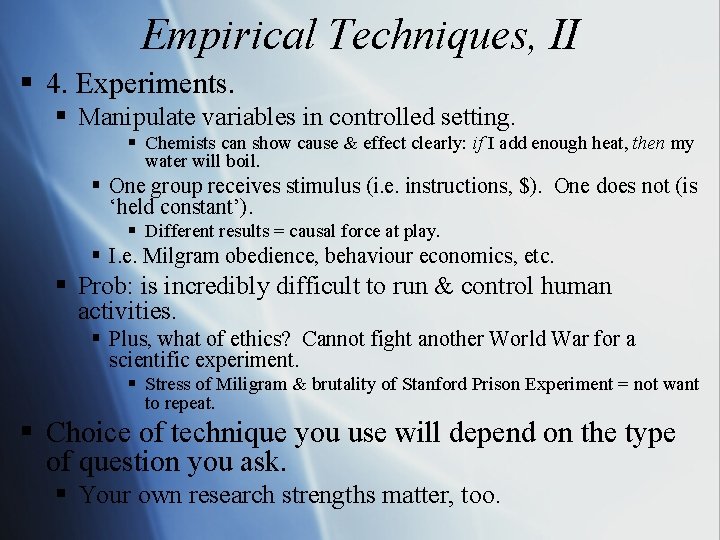
Empirical Techniques, II § 4. Experiments. § Manipulate variables in controlled setting. § Chemists can show cause & effect clearly: if I add enough heat, then my water will boil. § One group receives stimulus (i. e. instructions, $). One does not (is ‘held constant’). § Different results = causal force at play. § I. e. Milgram obedience, behaviour economics, etc. § Prob: is incredibly difficult to run & control human activities. § Plus, what of ethics? Cannot fight another World War for a scientific experiment. § Stress of Miligram & brutality of Stanford Prison Experiment = not want to repeat. § Choice of technique you use will depend on the type of question you ask. § Your own research strengths matter, too.
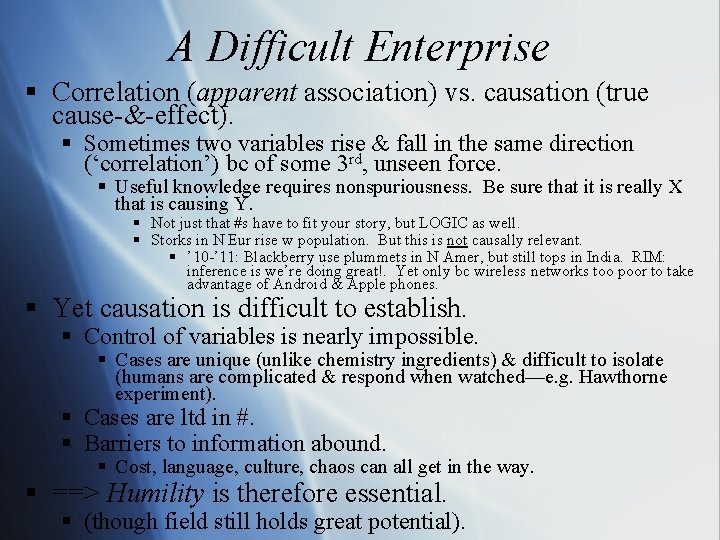
A Difficult Enterprise § Correlation (apparent association) vs. causation (true cause-&-effect). § Sometimes two variables rise & fall in the same direction (‘correlation’) bc of some 3 rd, unseen force. § Useful knowledge requires nonspuriousness. Be sure that it is really X that is causing Y. § Not just that #s have to fit your story, but LOGIC as well. § Storks in N Eur rise w population. But this is not causally relevant. § ’ 10 -’ 11: Blackberry use plummets in N Amer, but still tops in India. RIM: inference is we’re doing great!. Yet only bc wireless networks too poor to take advantage of Android & Apple phones. § Yet causation is difficult to establish. § Control of variables is nearly impossible. § Cases are unique (unlike chemistry ingredients) & difficult to isolate (humans are complicated & respond when watched—e. g. Hawthorne experiment). § Cases are ltd in #. § Barriers to information abound. § Cost, language, culture, chaos can all get in the way. § ==> Humility is therefore essential. § (though field still holds great potential).
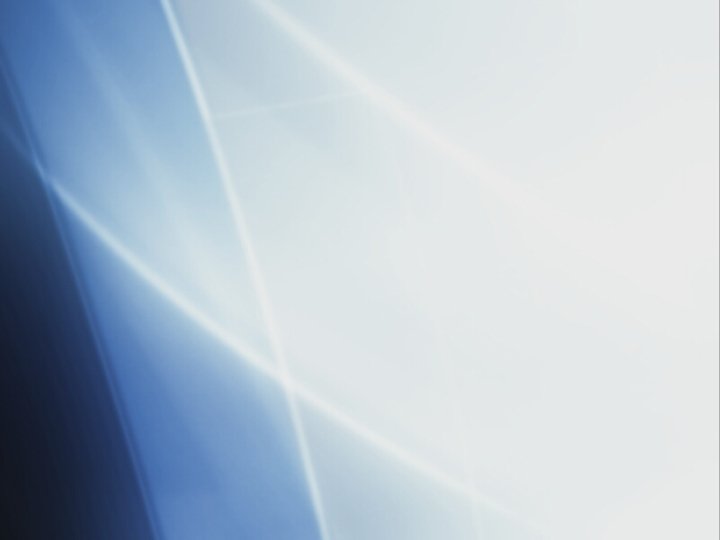
Lecturer's name
Pol 1000
Functions of marketing by clark and clark
Hugh et leifson
Research methodology lecture notes doc
Significance of research methodology
01:640:244 lecture notes - lecture 15: plat, idah, farad
Lecturer name
Spe distinguished lecturer
Lecturer in charge
Designation lecturer
Pearson lecturer resources
Good morning teacher
Cfa lecturer handbook
Designation of lecturer
Spe distinguished lecturer