PLANNING UNDER UNCERTAINTY REGRET THEORY MINIMAX REGRET ANALYSIS
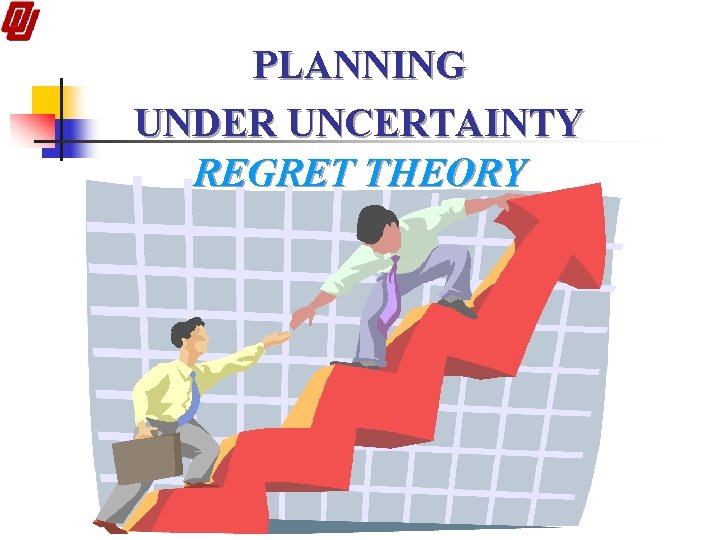
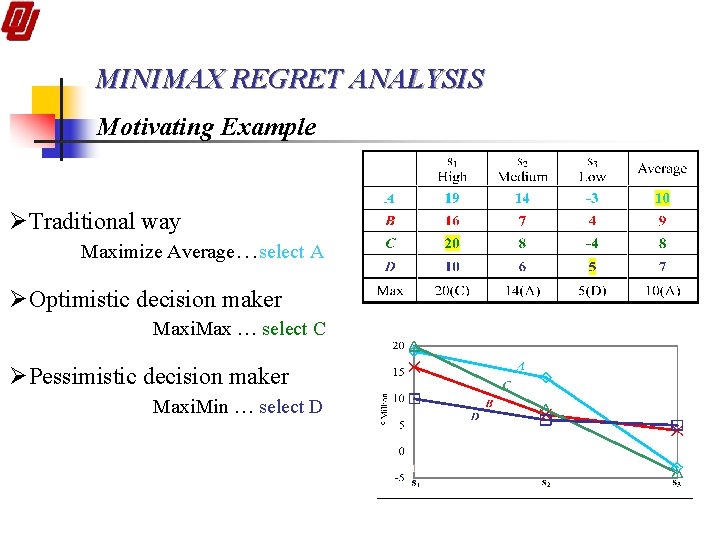
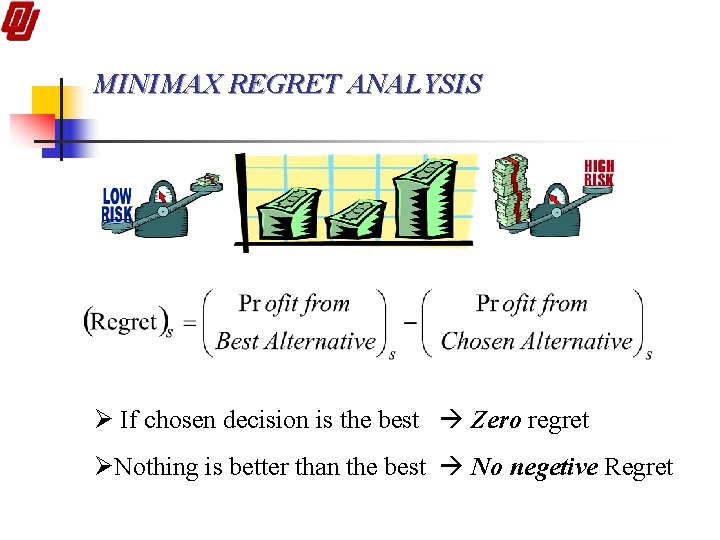
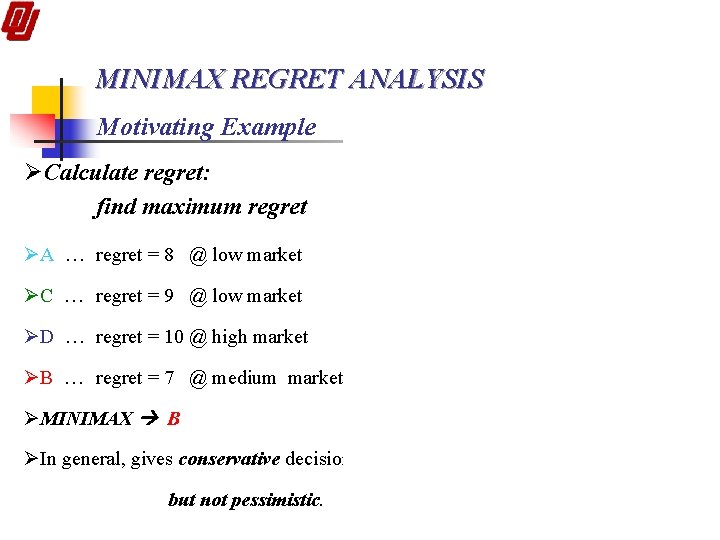
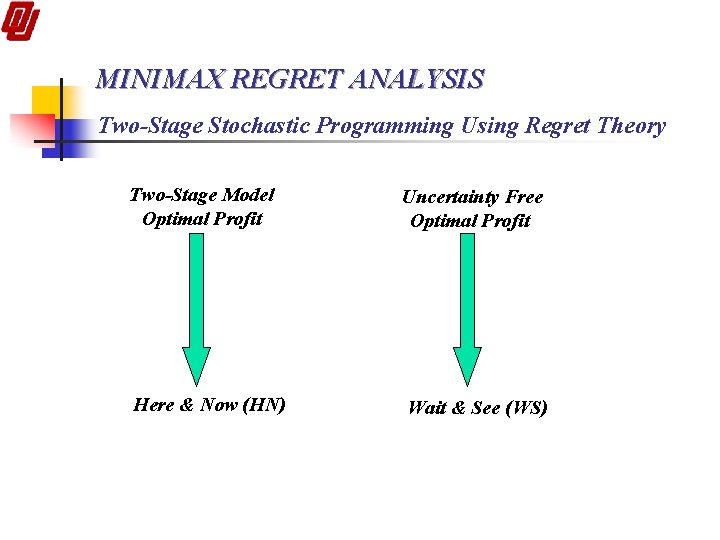
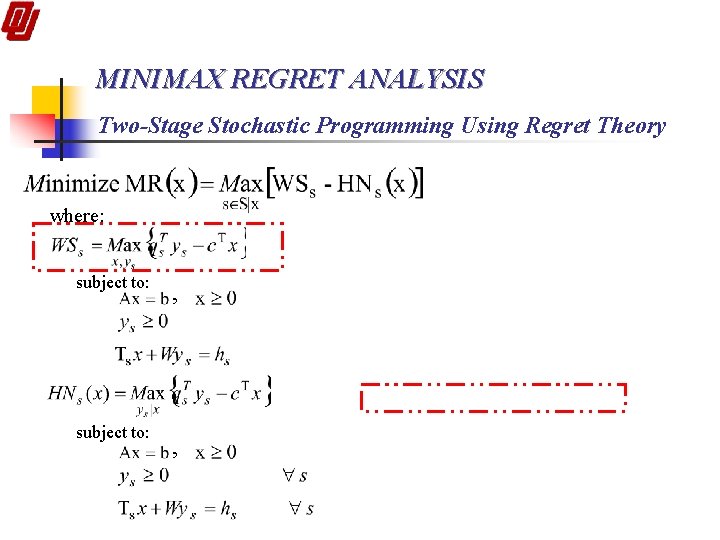
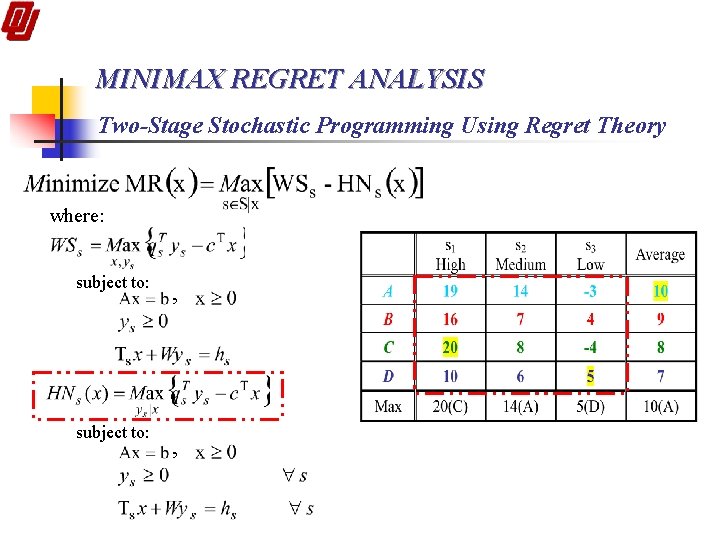
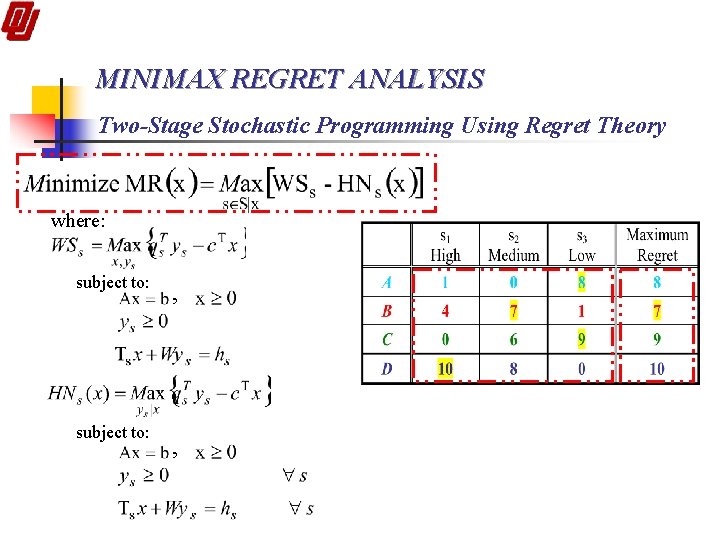
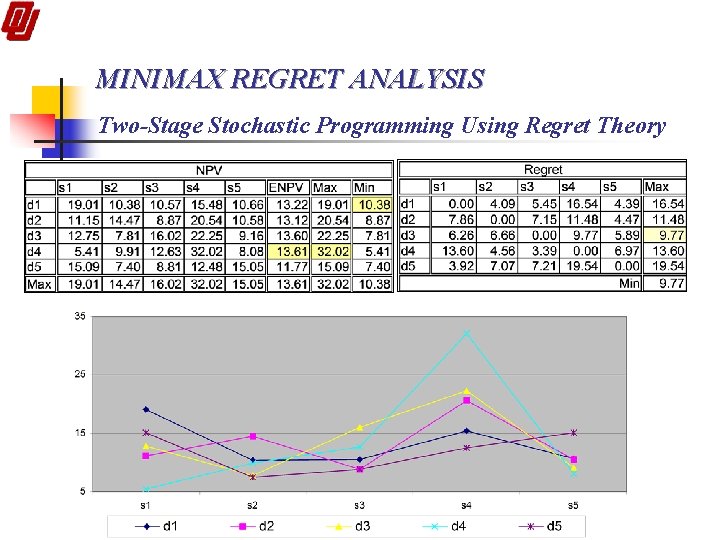
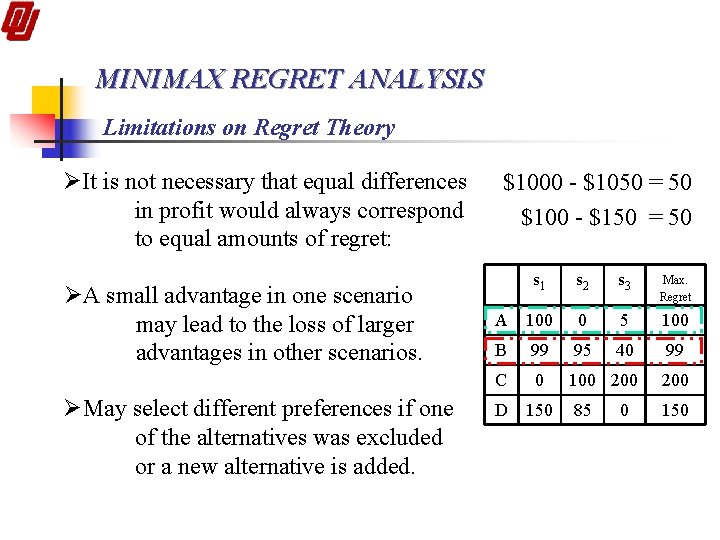
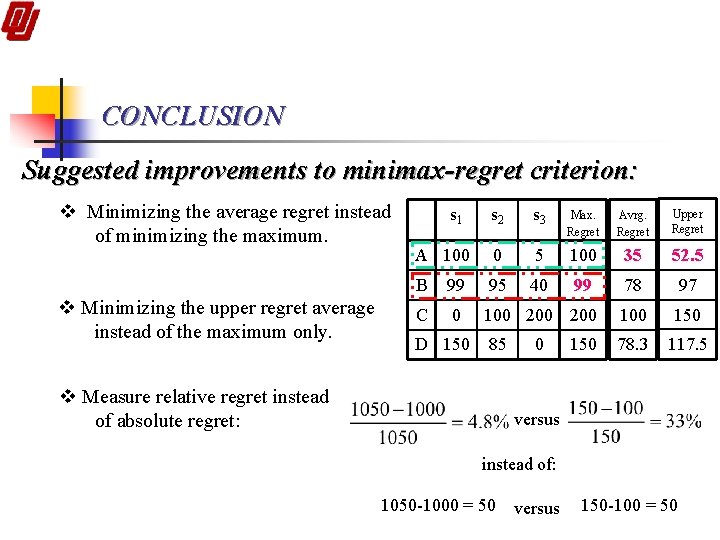
- Slides: 11
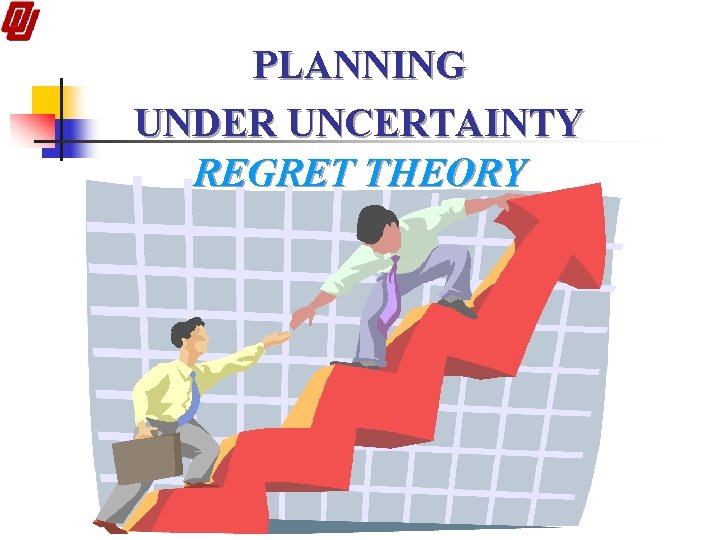
PLANNING UNDER UNCERTAINTY REGRET THEORY
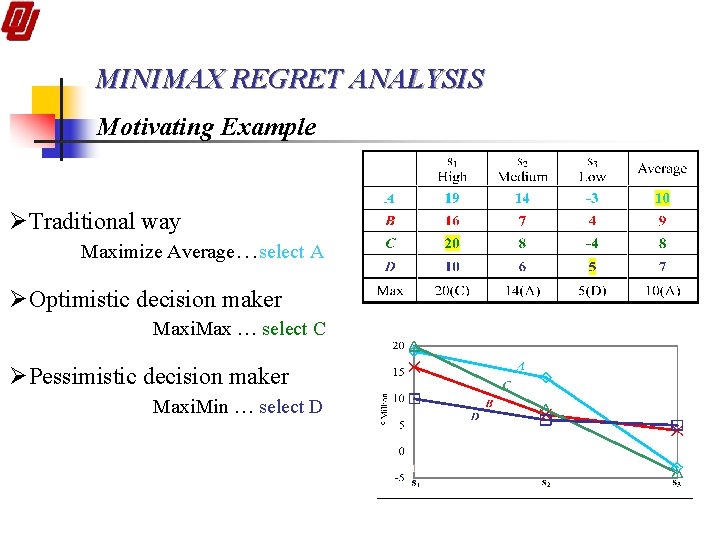
MINIMAX REGRET ANALYSIS Motivating Example ØTraditional way Maximize Average…select A ØOptimistic decision maker Maxi. Max … select C ØPessimistic decision maker Maxi. Min … select D
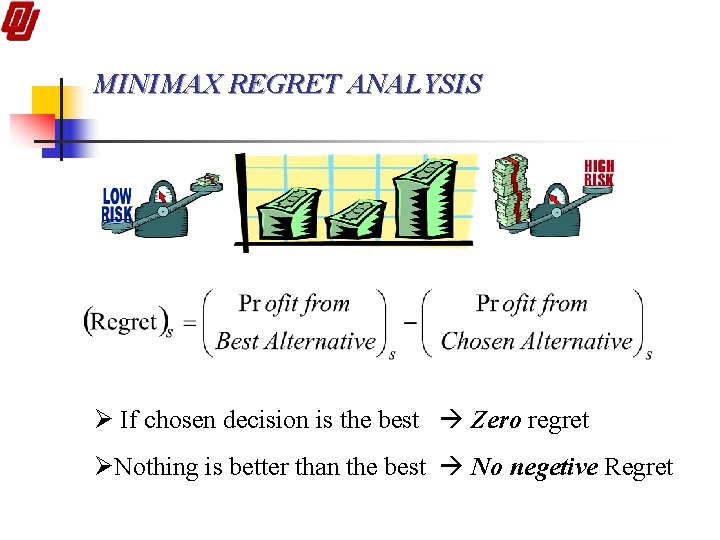
MINIMAX REGRET ANALYSIS Ø If chosen decision is the best Zero regret ØNothing is better than the best No negetive Regret
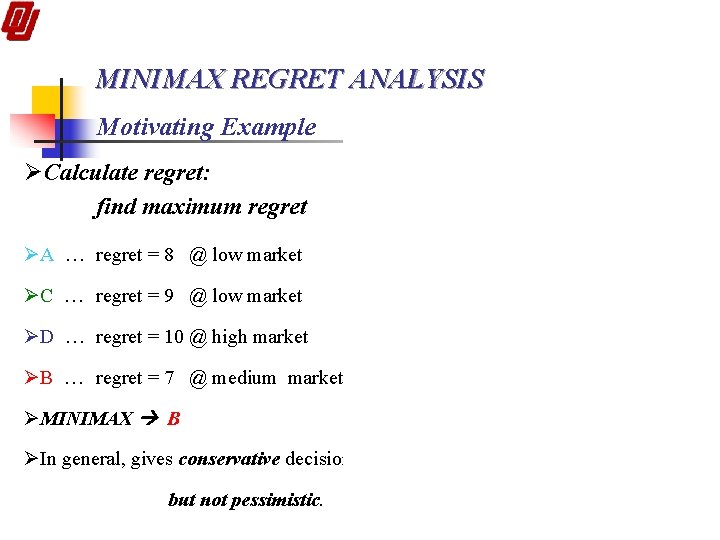
MINIMAX REGRET ANALYSIS Motivating Example ØCalculate regret: find maximum regret ØA … regret = 8 @ low market ØC … regret = 9 @ low market ØD … regret = 10 @ high market ØB … regret = 7 @ medium market ØMINIMAX B ØIn general, gives conservative decision but not pessimistic.
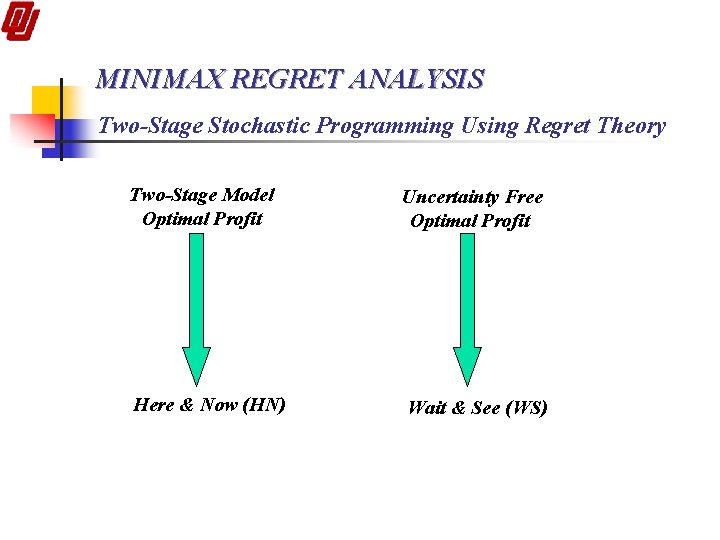
MINIMAX REGRET ANALYSIS Two-Stage Stochastic Programming Using Regret Theory Two-Stage Model Optimal Profit Uncertainty Free Optimal Profit Here & Now (HN) Wait & See (WS)
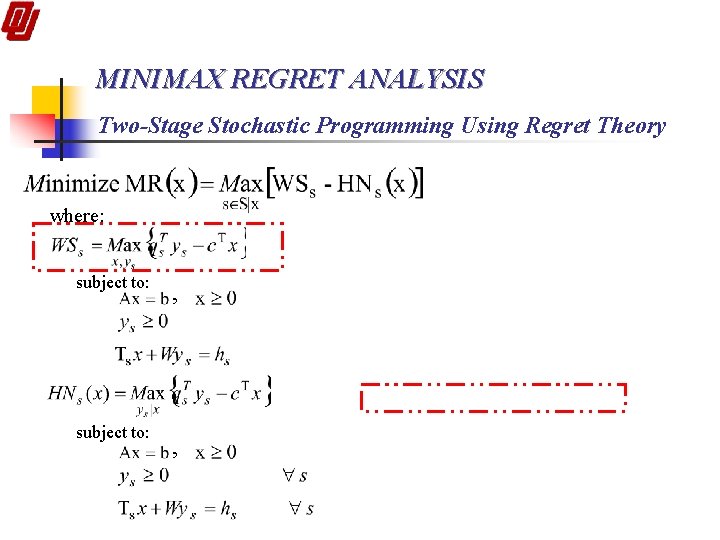
MINIMAX REGRET ANALYSIS Two-Stage Stochastic Programming Using Regret Theory where: subject to: , ,
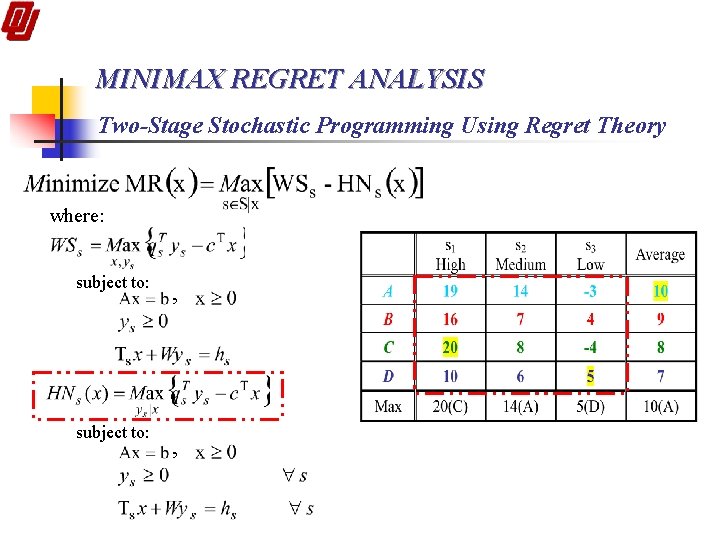
MINIMAX REGRET ANALYSIS Two-Stage Stochastic Programming Using Regret Theory where: subject to: , ,
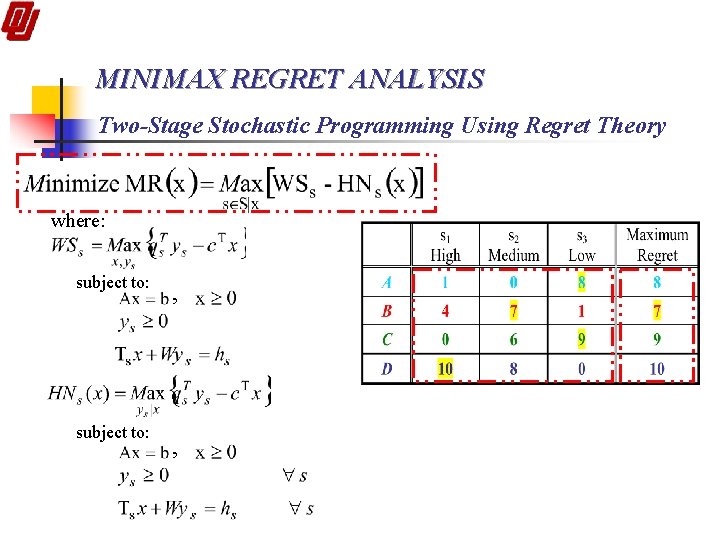
MINIMAX REGRET ANALYSIS Two-Stage Stochastic Programming Using Regret Theory where: subject to: , ,
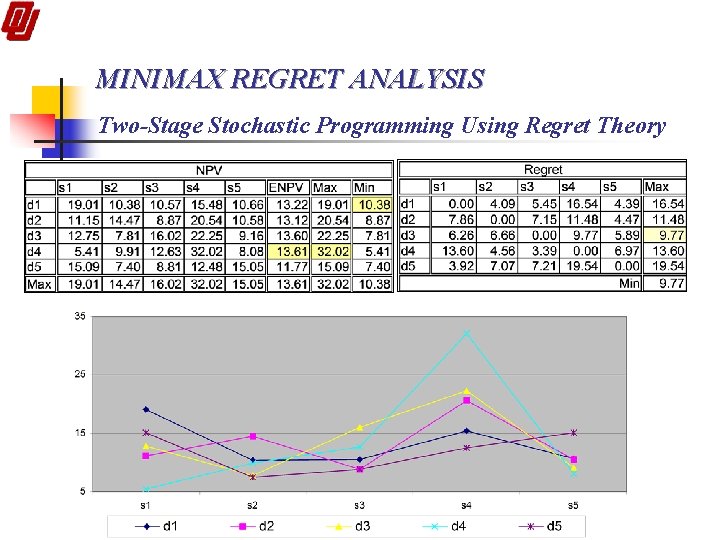
MINIMAX REGRET ANALYSIS Two-Stage Stochastic Programming Using Regret Theory
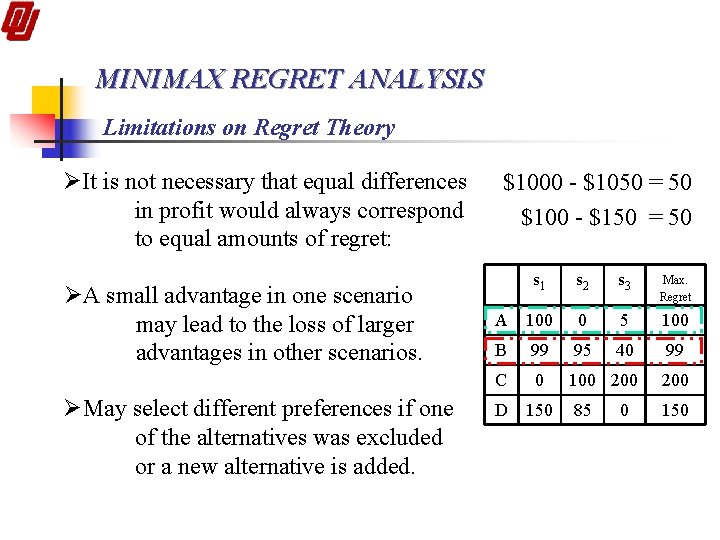
MINIMAX REGRET ANALYSIS Limitations on Regret Theory ØIt is not necessary that equal differences in profit would always correspond to equal amounts of regret: ØA small advantage in one scenario may lead to the loss of larger advantages in other scenarios. ØMay select different preferences if one of the alternatives was excluded or a new alternative is added. $1000 - $1050 = 50 $100 - $150 = 50 s 1 s 2 s 3 Max. Regret A 100 0 5 100 B 99 95 40 99 C 0 100 200 D 150 85 150 0
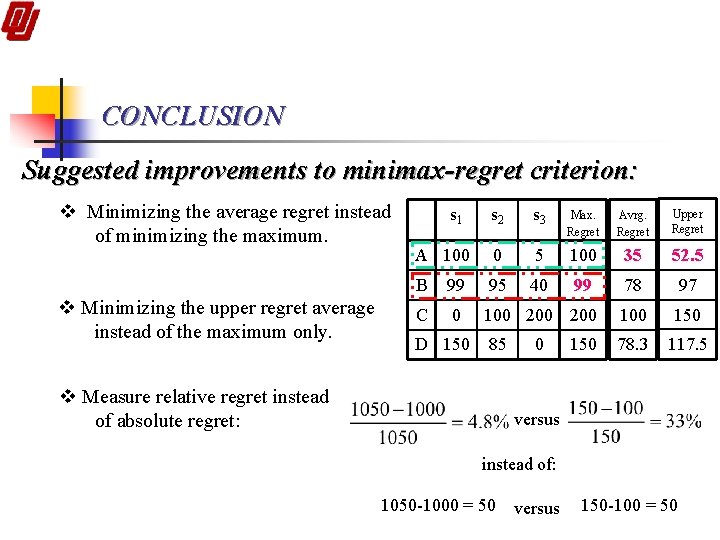
CONCLUSION Suggested improvements to minimax-regret criterion: v Minimizing the average regret instead of minimizing the maximum. v Minimizing the upper regret average instead of the maximum only. s 1 s 2 s 3 Max. Regret Avrg. Regret Upper Regret A 100 0 5 100 35 52. 5 B 99 95 40 99 78 97 C 0 100 200 150 85 78. 3 117. 5 D 150 v Measure relative regret instead of absolute regret: 0 150 versus instead of: 1050 -1000 = 50 versus 150 -100 = 50
Minmax regret
Contoh soal decision making
Zero sum game
Minimax and maximin principle in game theory
Minimalist reasoning
Uncertainity in decision making
Decision-making under uncertainty
Capital budgeting under uncertainty
Expected profit under uncertainty
Regret analysis
Cl alt relationships
Algorytm minimax