ONTOLOGIES COP Practical examples of developing ontologies in
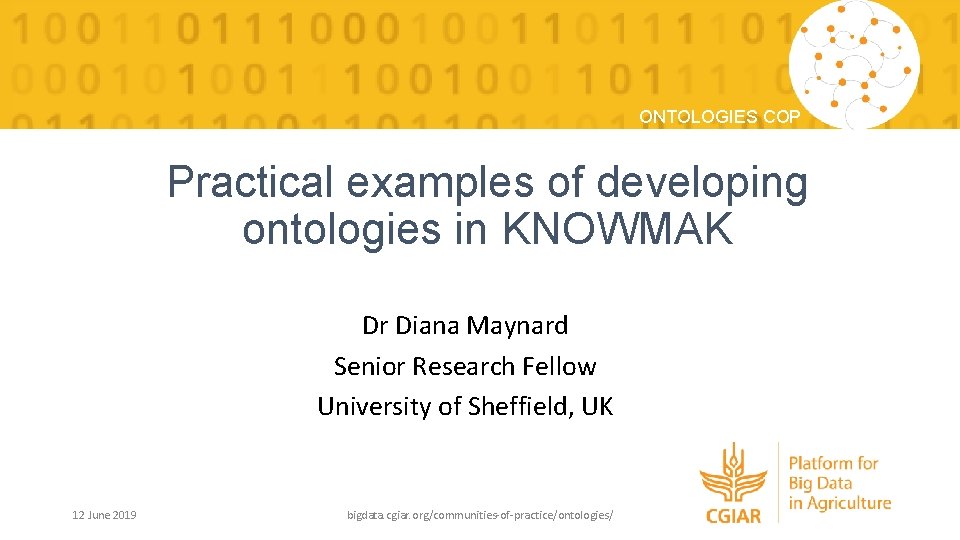
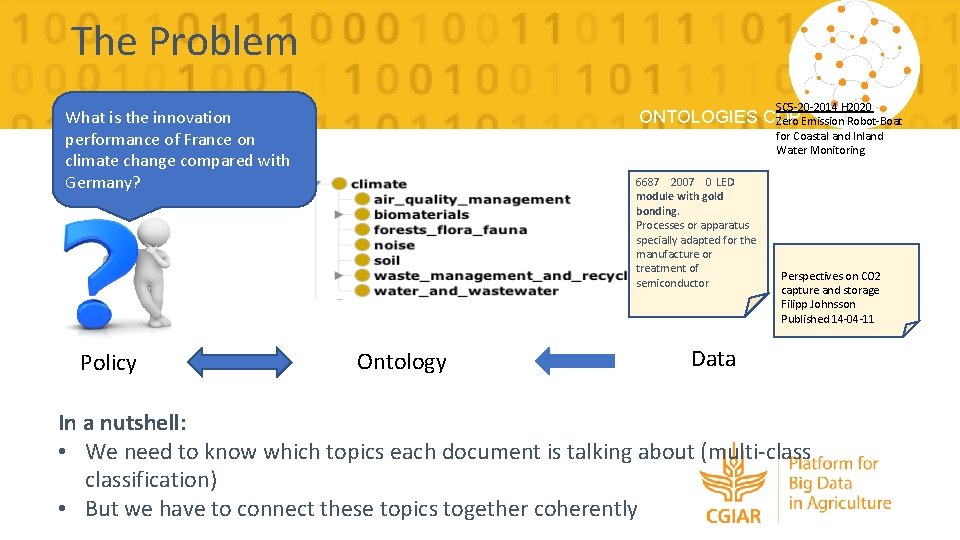
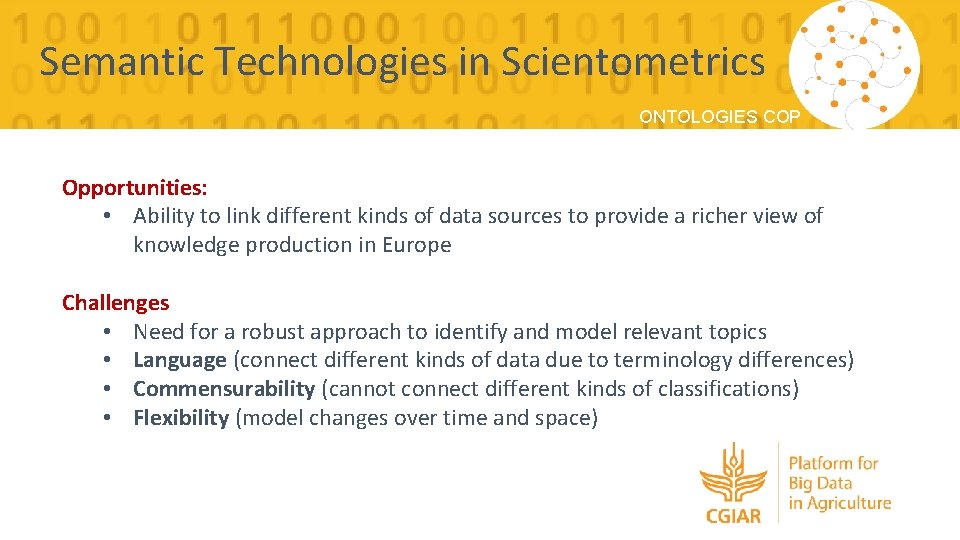
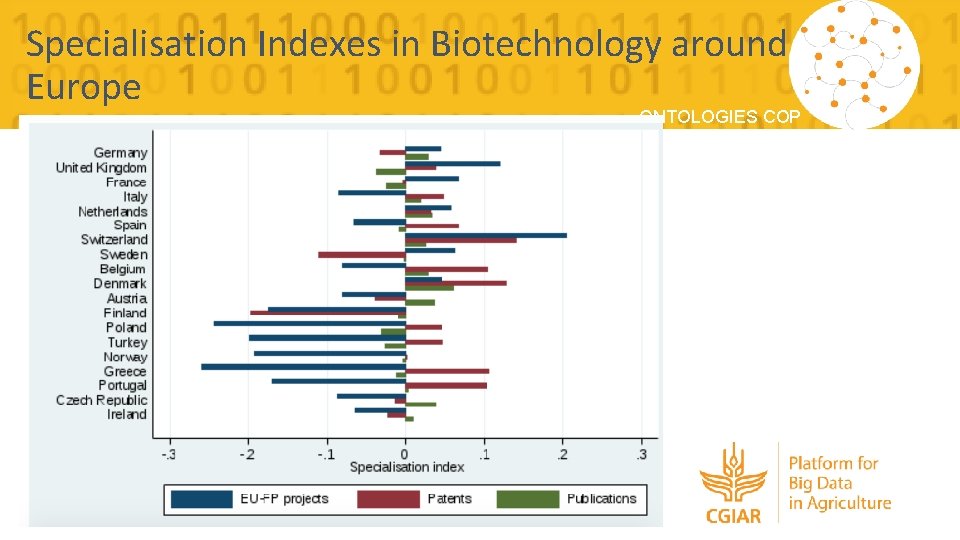
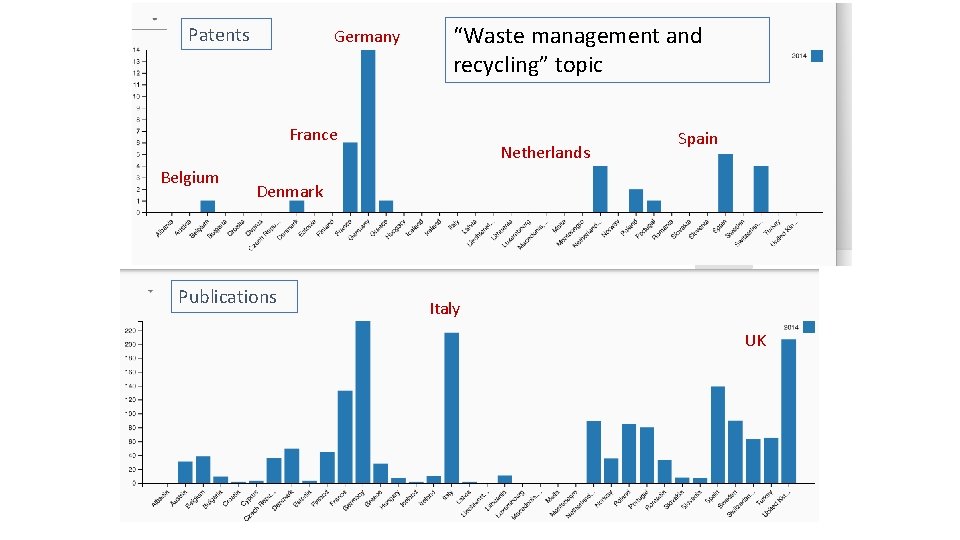
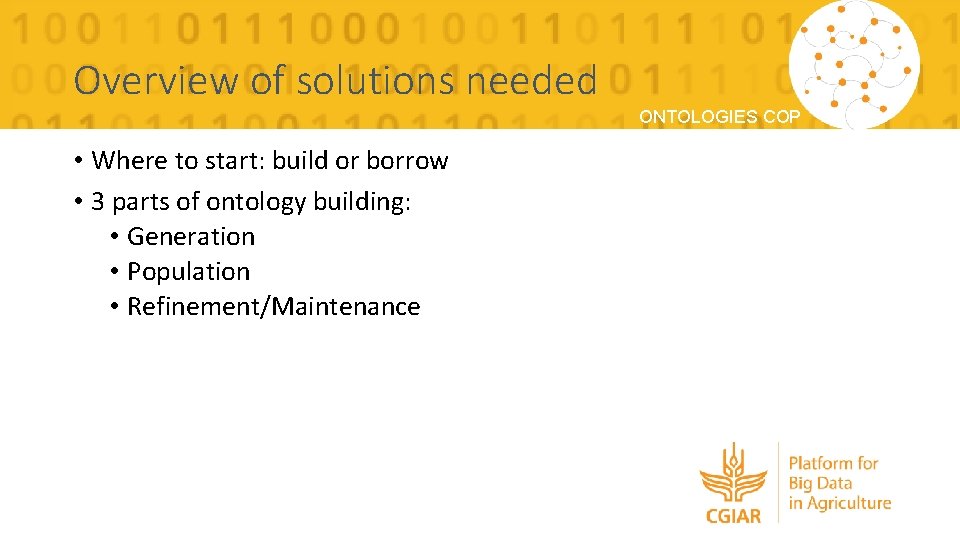
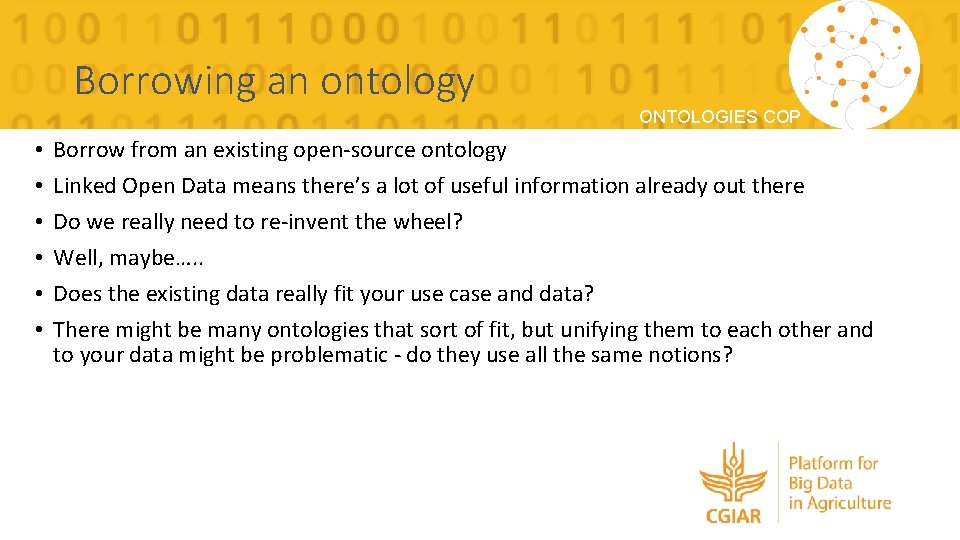
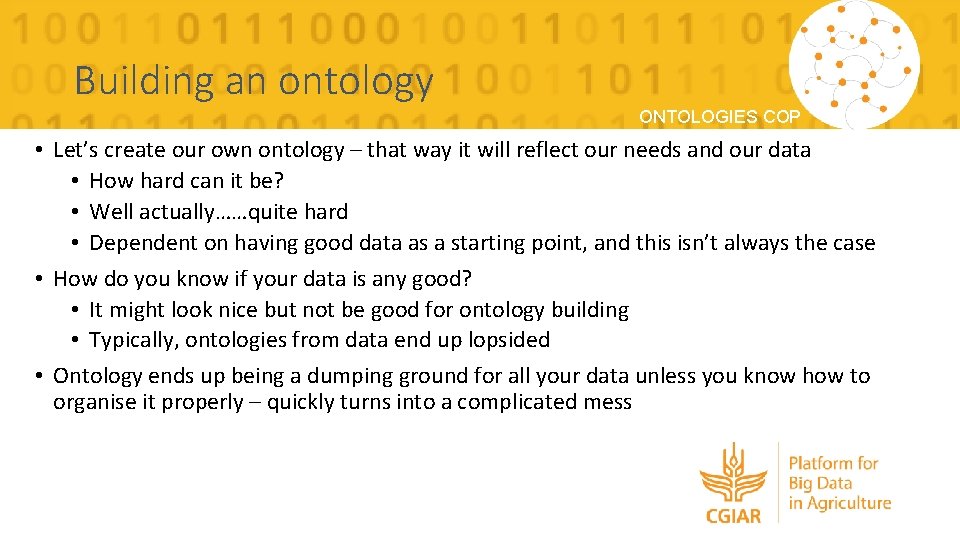
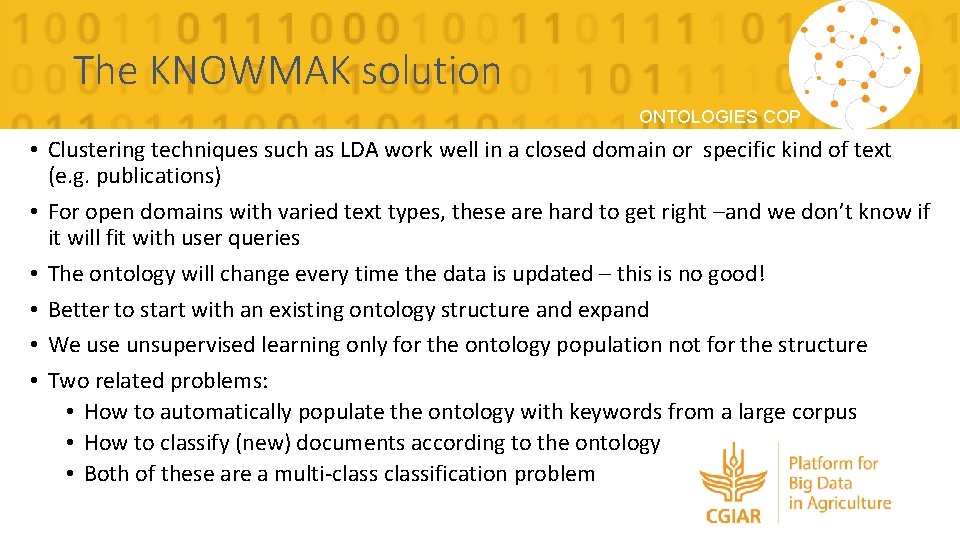
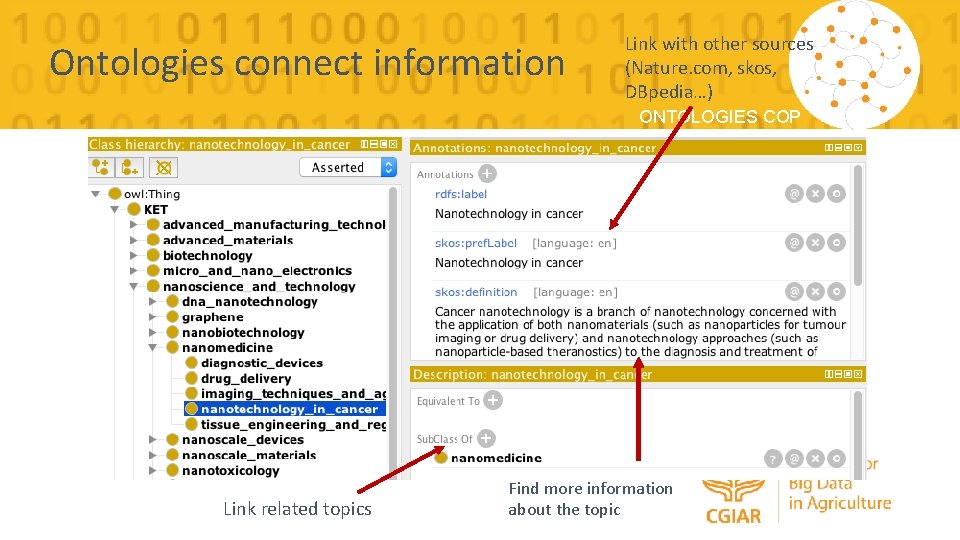
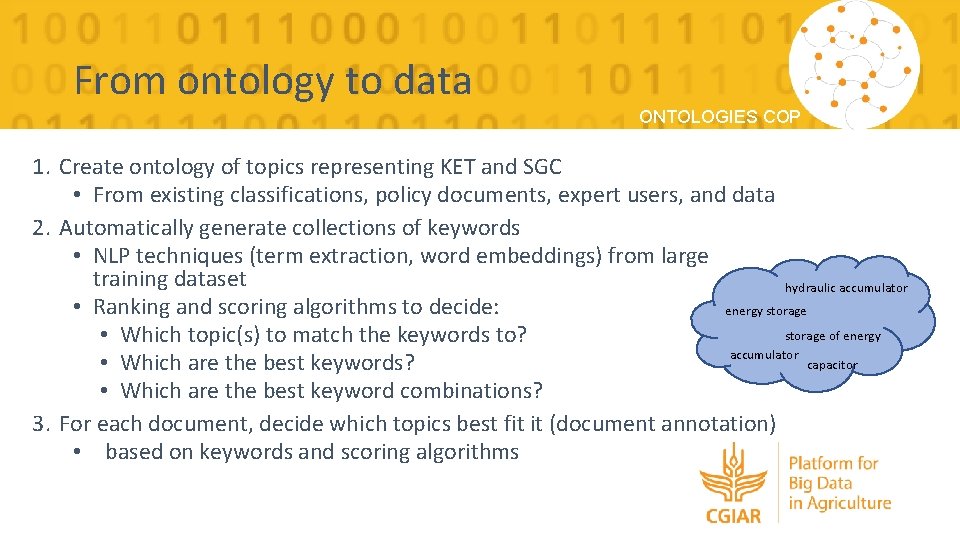
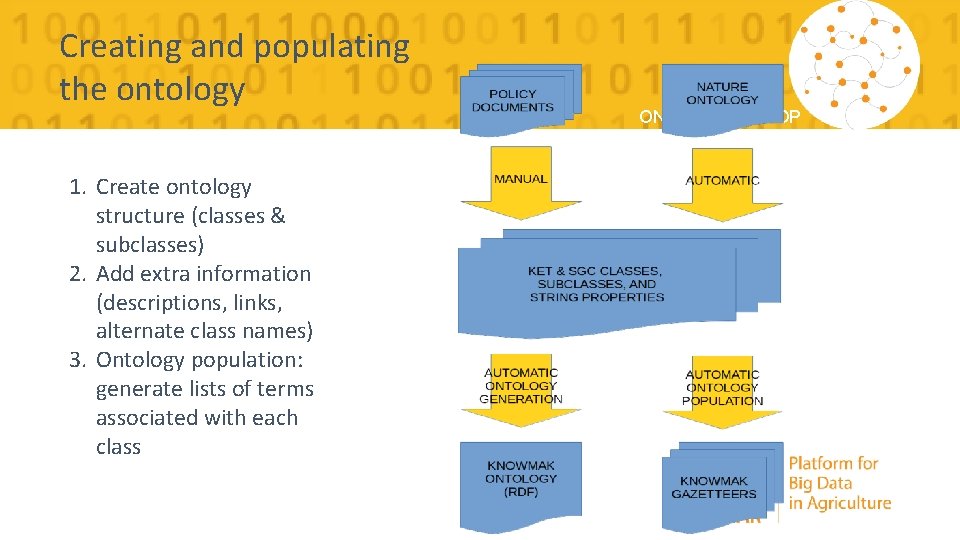
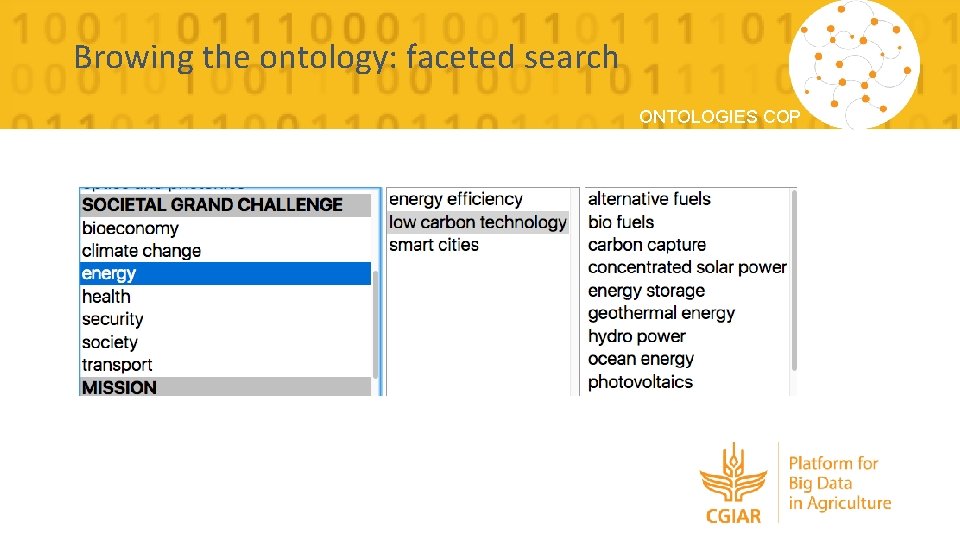
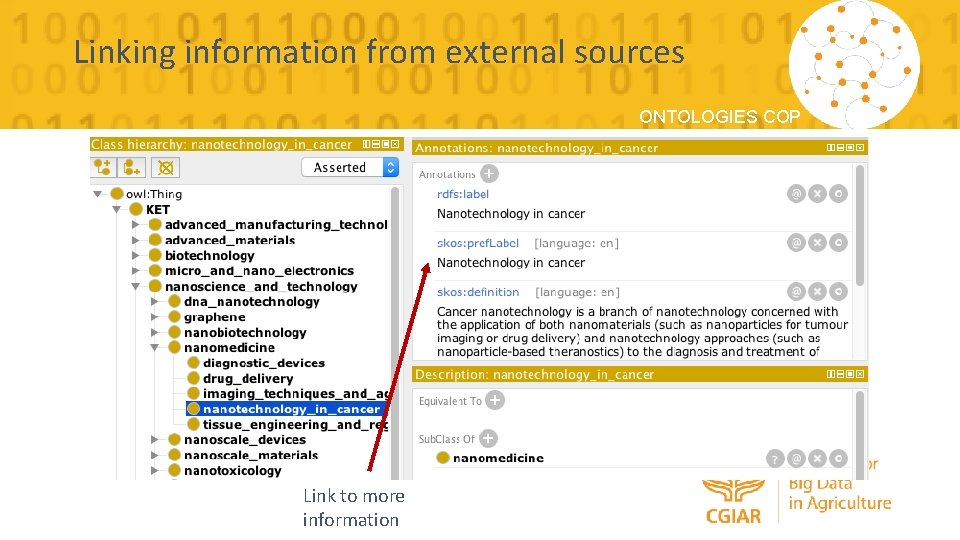
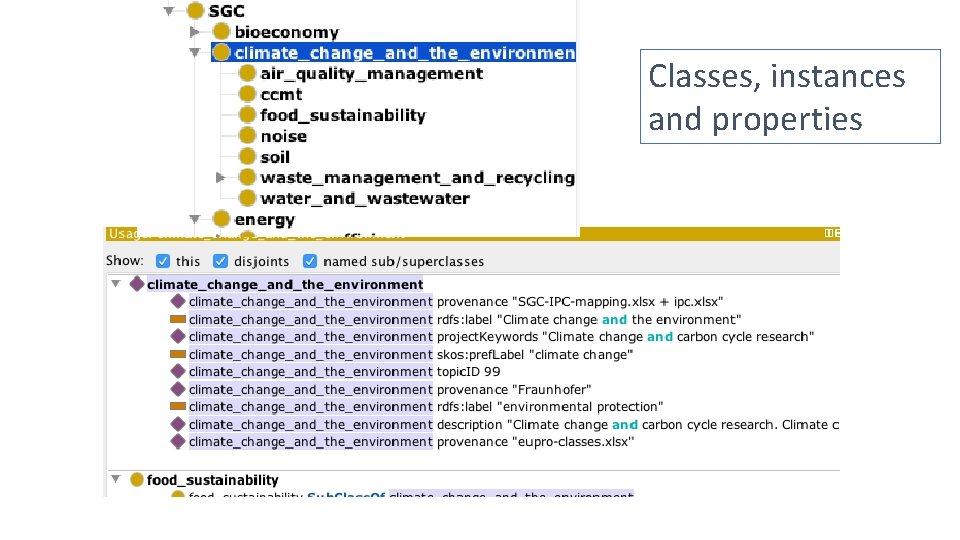
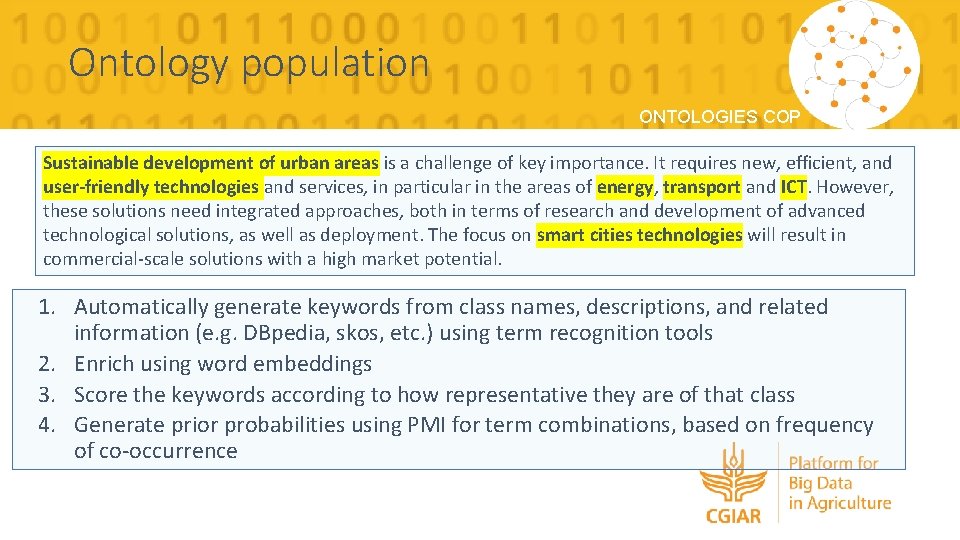
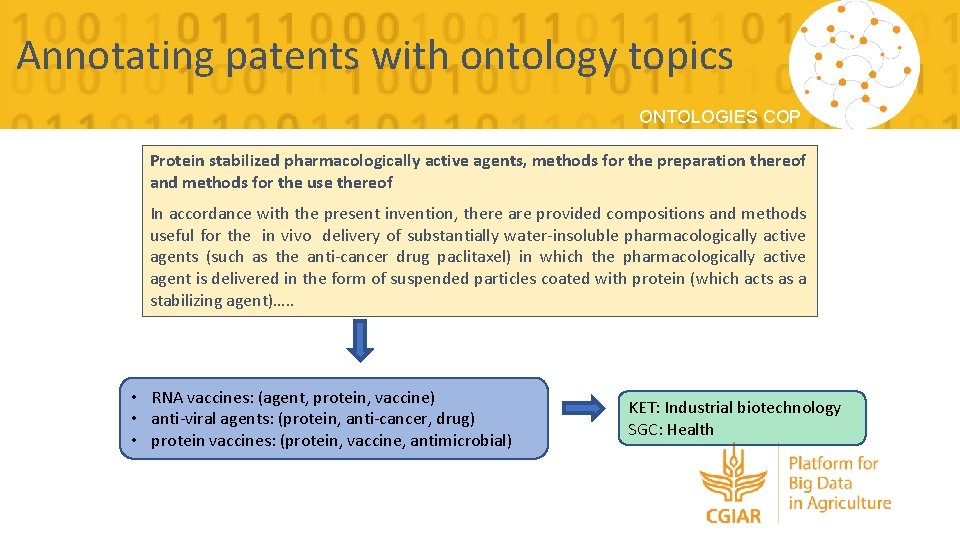
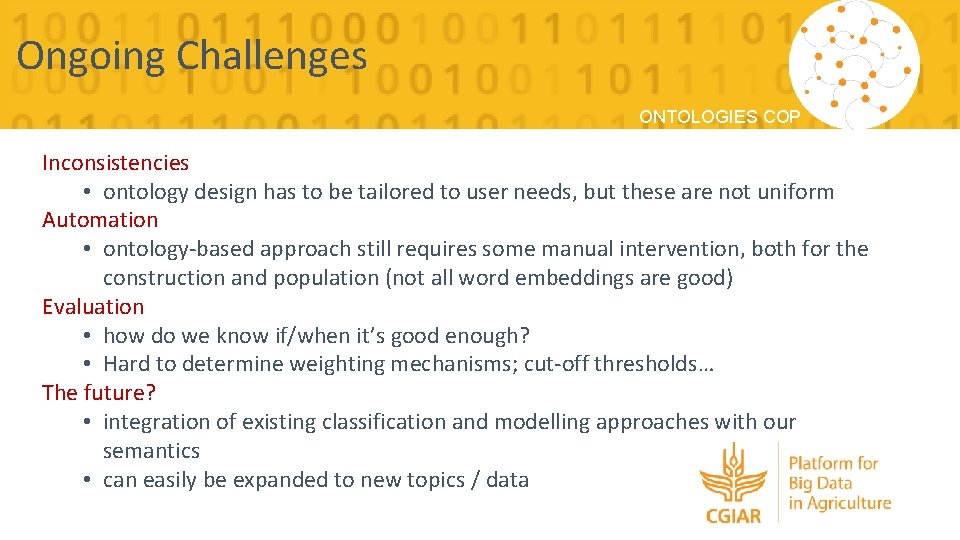
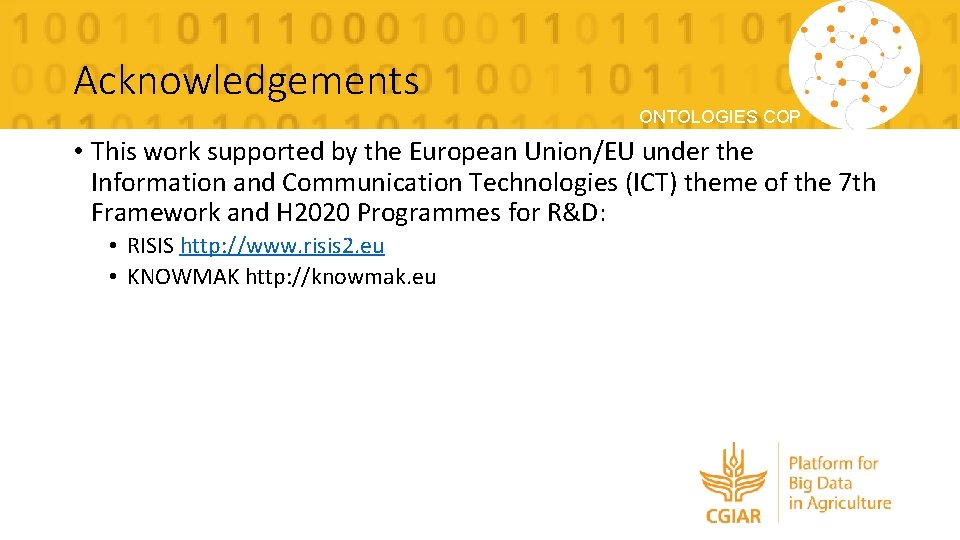
- Slides: 19
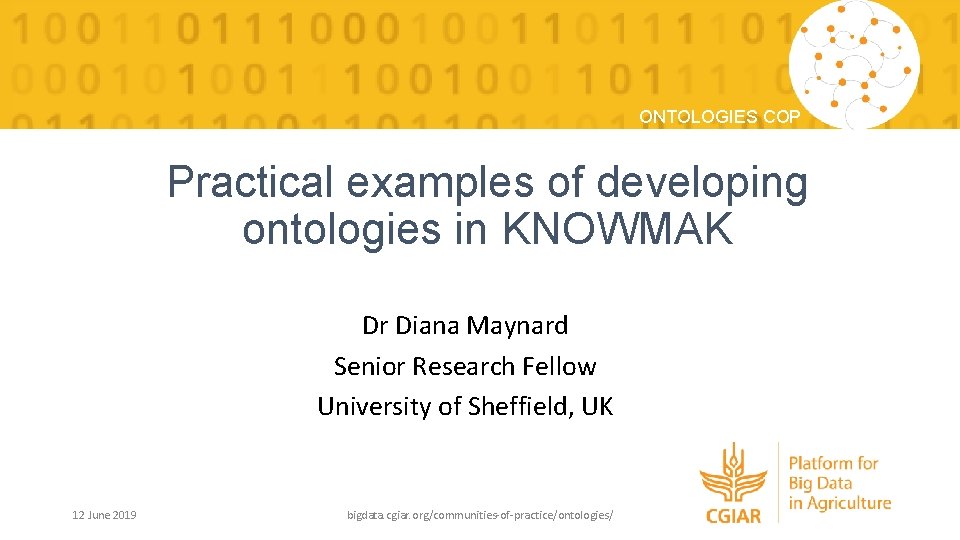
ONTOLOGIES COP Practical examples of developing ontologies in KNOWMAK Dr Diana Maynard Senior Research Fellow University of Sheffield, UK 12 June 2019 bigdata. cgiar. org/communities-of-practice/ontologies/
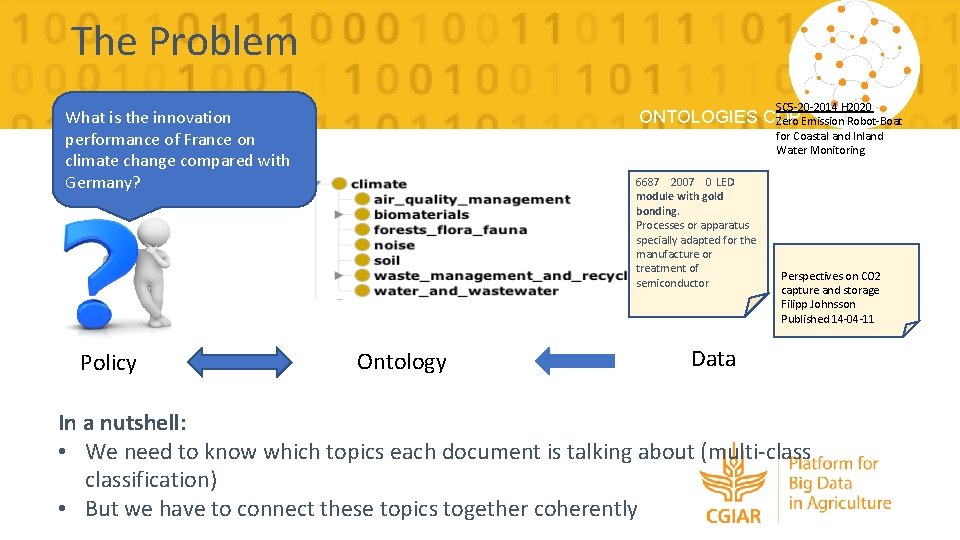
The Problem SC 5 -20 -2014 H 2020 What is the innovation performance of France on climate change compared with Germany? Policy ONTOLOGIES COP Zero Emission Robot-Boat for Coastal and Inland Water Monitoring 6687 2007 0 LED module with gold bonding. Processes or apparatus specially adapted for the manufacture or treatment of semiconductor Ontology Perspectives on CO 2 capture and storage Filipp Johnsson Published 14 -04 -11 Data In a nutshell: • We need to know which topics each document is talking about (multi-classification) • But we have to connect these topics together coherently
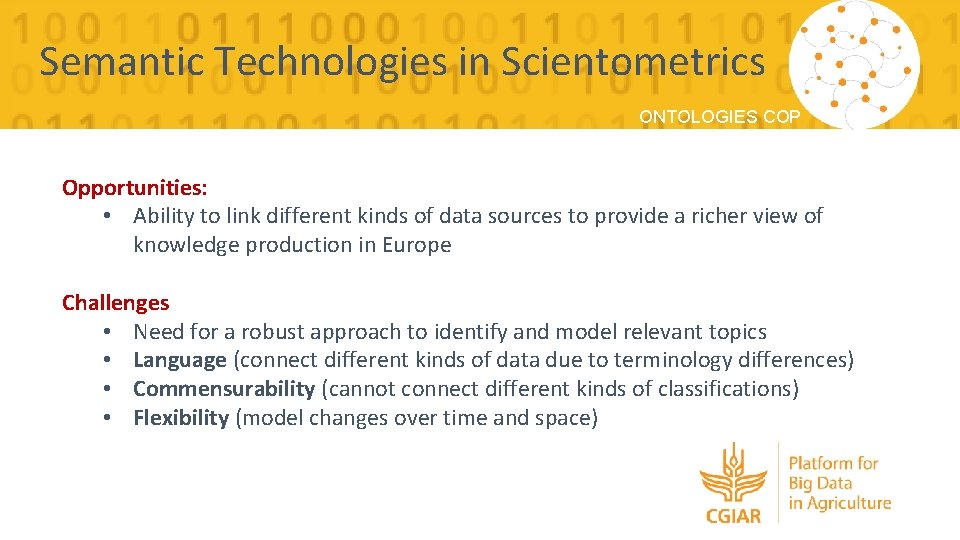
Semantic Technologies in Scientometrics ONTOLOGIES COP Opportunities: • Ability to link different kinds of data sources to provide a richer view of knowledge production in Europe Challenges • Need for a robust approach to identify and model relevant topics • Language (connect different kinds of data due to terminology differences) • Commensurability (cannot connect different kinds of classifications) • Flexibility (model changes over time and space)
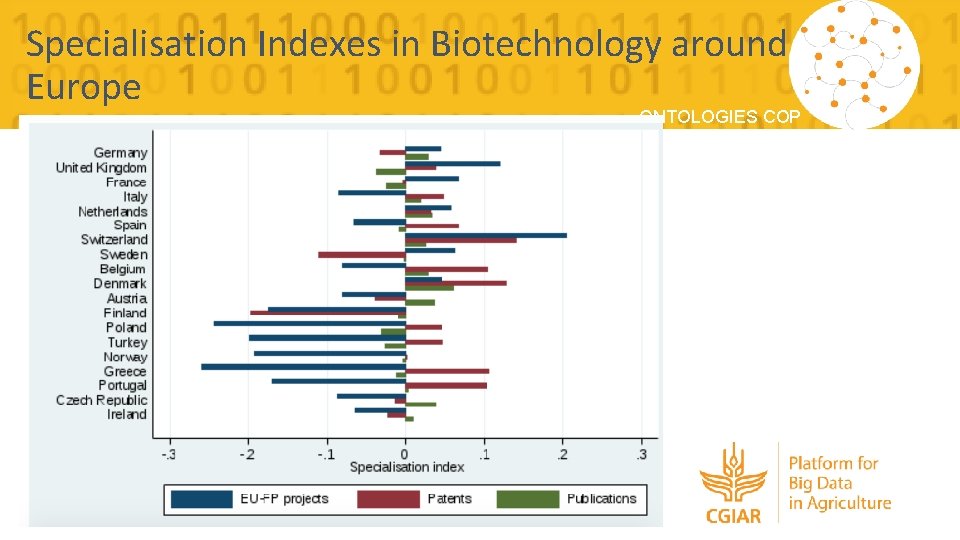
Specialisation Indexes in Biotechnology around Europe ONTOLOGIES COP
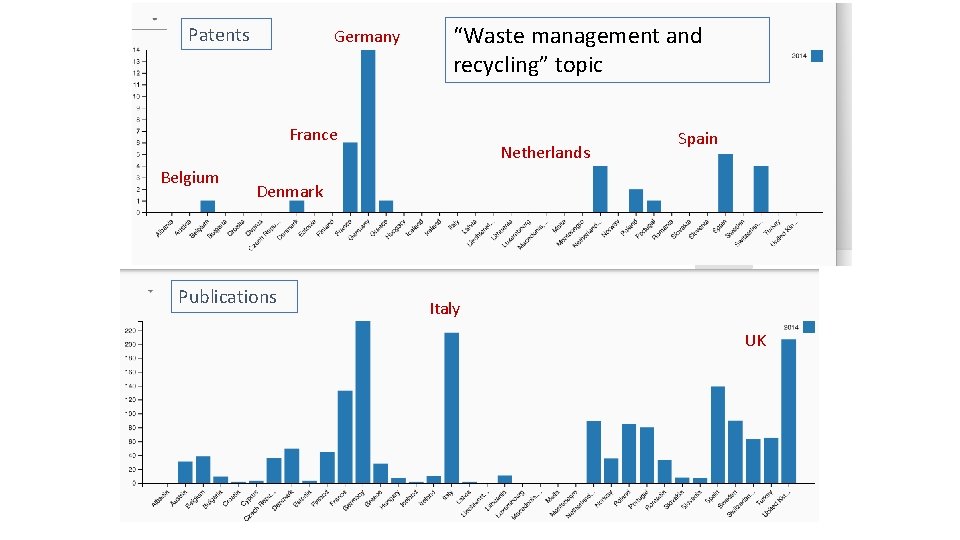
Patents Germany “Waste management and recycling” topic France Belgium Netherlands Spain Denmark Publications Italy UK
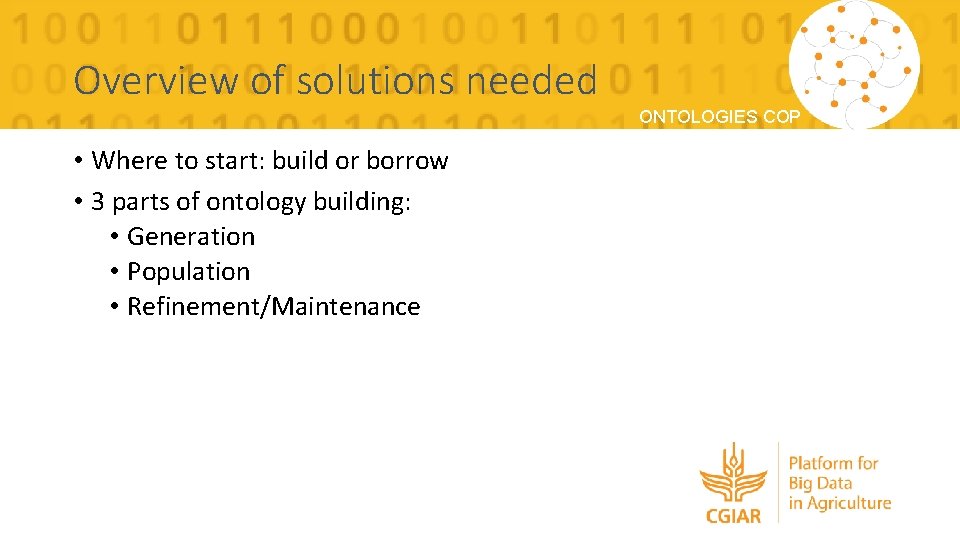
Overview of solutions needed ONTOLOGIES COP • Where to start: build or borrow • 3 parts of ontology building: • Generation • Population • Refinement/Maintenance
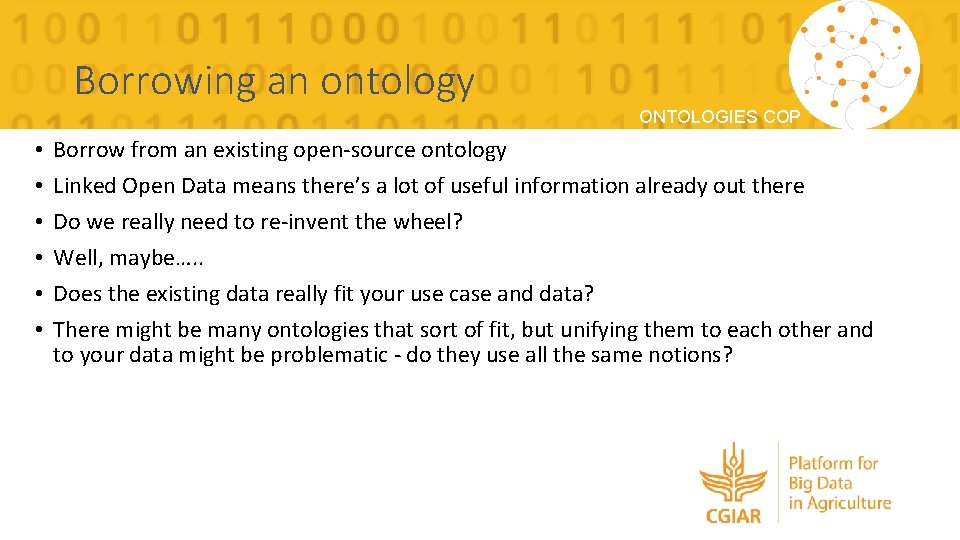
Borrowing an ontology ONTOLOGIES COP • • • Borrow from an existing open-source ontology Linked Open Data means there’s a lot of useful information already out there Do we really need to re-invent the wheel? Well, maybe…. . Does the existing data really fit your use case and data? There might be many ontologies that sort of fit, but unifying them to each other and to your data might be problematic - do they use all the same notions?
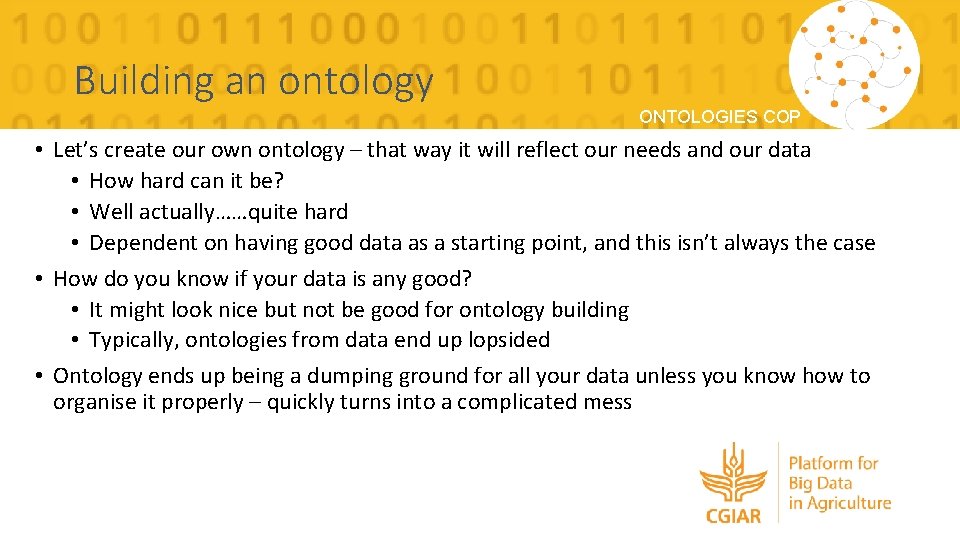
Building an ontology ONTOLOGIES COP • Let’s create our own ontology – that way it will reflect our needs and our data • How hard can it be? • Well actually……quite hard • Dependent on having good data as a starting point, and this isn’t always the case • How do you know if your data is any good? • It might look nice but not be good for ontology building • Typically, ontologies from data end up lopsided • Ontology ends up being a dumping ground for all your data unless you know how to organise it properly – quickly turns into a complicated mess
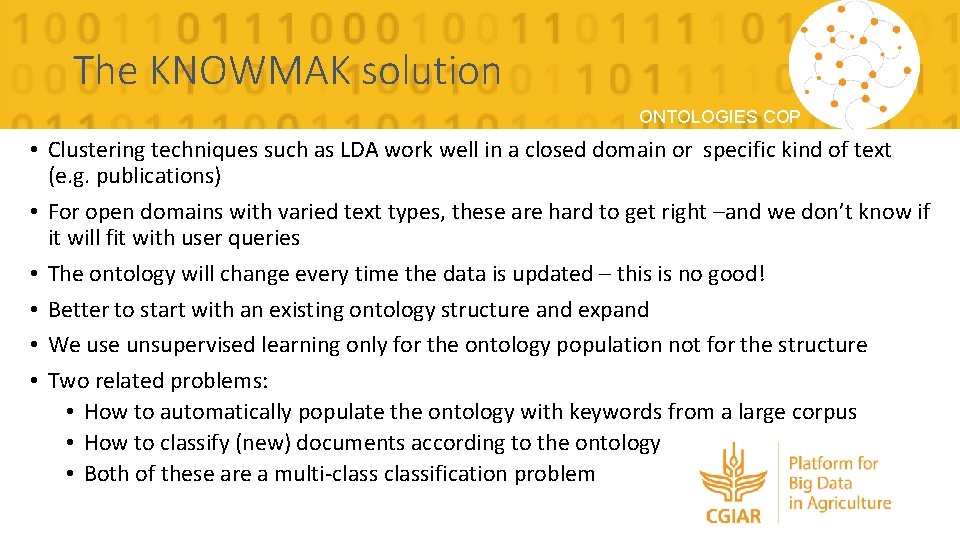
The KNOWMAK solution ONTOLOGIES COP • Clustering techniques such as LDA work well in a closed domain or specific kind of text (e. g. publications) • For open domains with varied text types, these are hard to get right –and we don’t know if it will fit with user queries • The ontology will change every time the data is updated – this is no good! • Better to start with an existing ontology structure and expand • We use unsupervised learning only for the ontology population not for the structure • Two related problems: • How to automatically populate the ontology with keywords from a large corpus • How to classify (new) documents according to the ontology • Both of these are a multi-classification problem
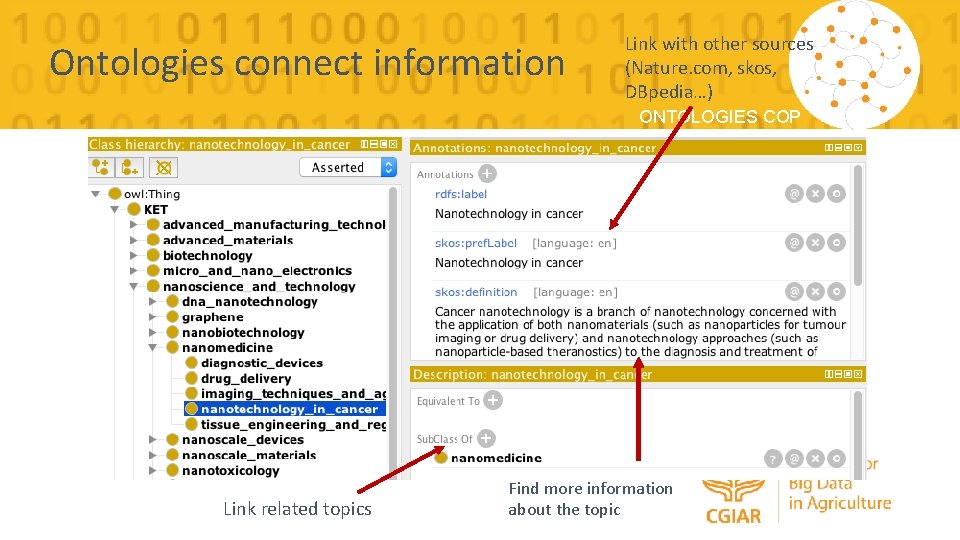
Ontologies connect information Link with other sources (Nature. com, skos, DBpedia…) ONTOLOGIES COP Link related topics Find more information about the topic
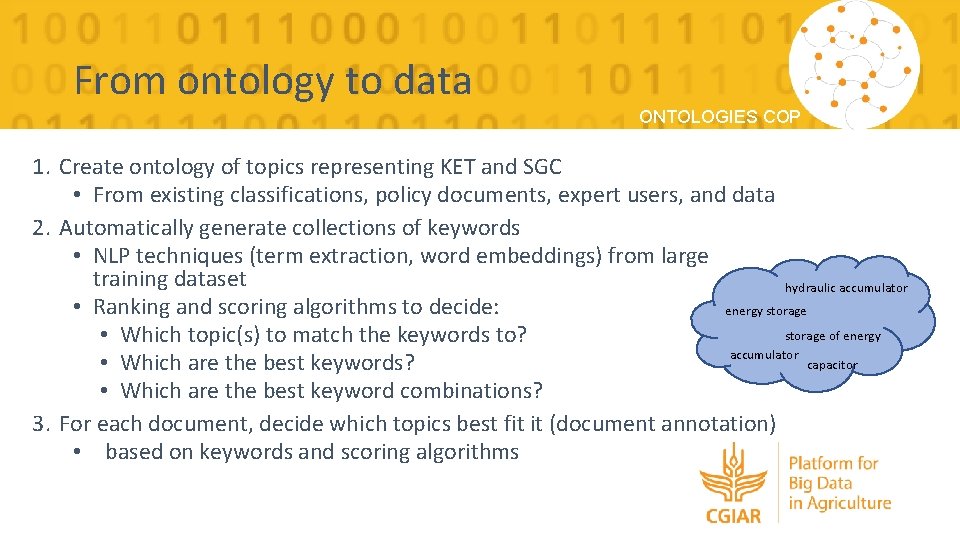
From ontology to data ONTOLOGIES COP 1. Create ontology of topics representing KET and SGC • From existing classifications, policy documents, expert users, and data 2. Automatically generate collections of keywords • NLP techniques (term extraction, word embeddings) from large training dataset hydraulic accumulator • Ranking and scoring algorithms to decide: energy storage of energy • Which topic(s) to match the keywords to? accumulator capacitor • Which are the best keywords? • Which are the best keyword combinations? 3. For each document, decide which topics best fit it (document annotation) • based on keywords and scoring algorithms
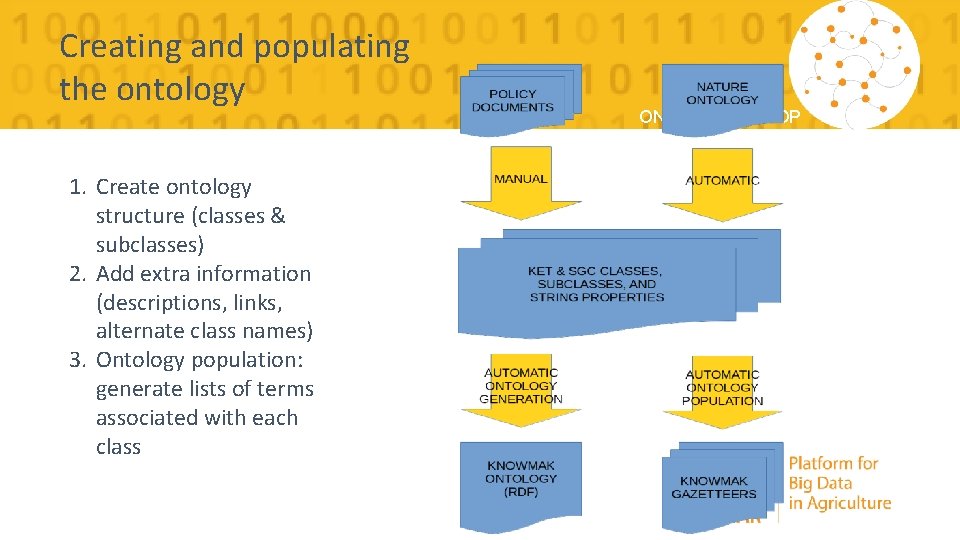
Creating and populating the ontology 1. Create ontology structure (classes & subclasses) 2. Add extra information (descriptions, links, alternate class names) 3. Ontology population: generate lists of terms associated with each class ONTOLOGIES COP
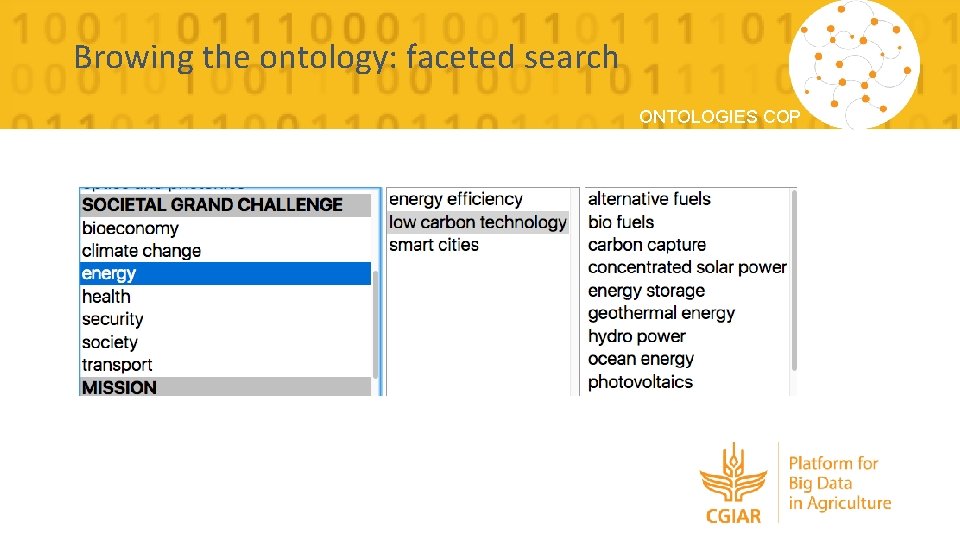
Browing the ontology: faceted search ONTOLOGIES COP
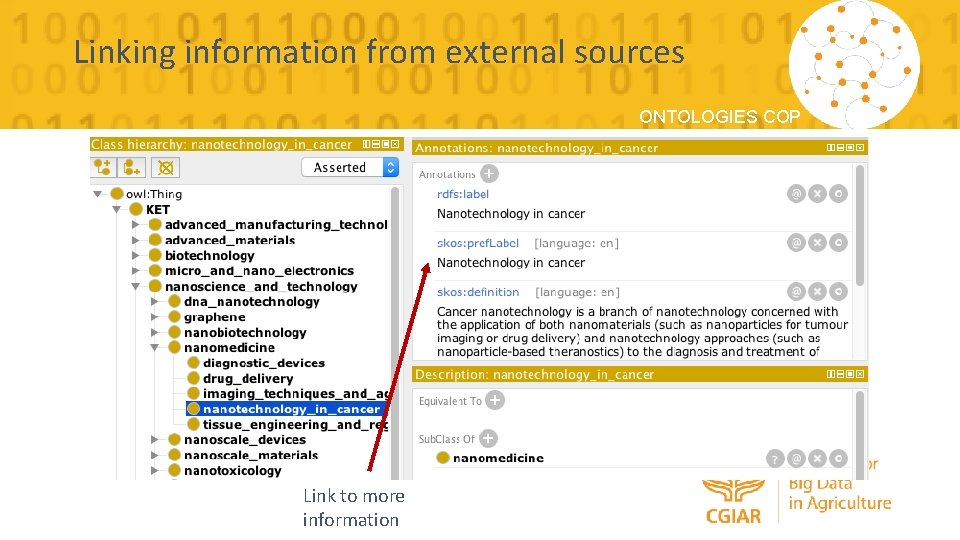
Linking information from external sources ONTOLOGIES COP Link to more information
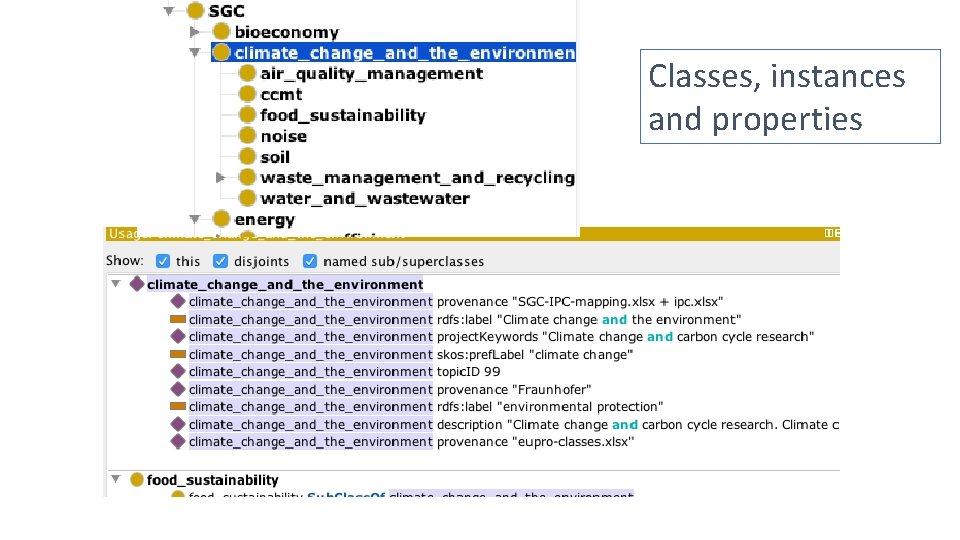
Classes, instances and properties
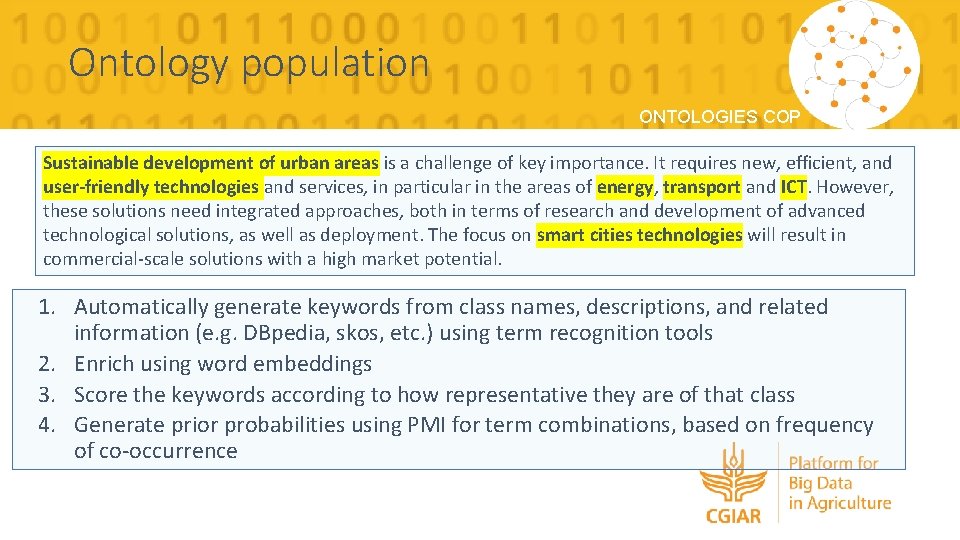
Ontology population ONTOLOGIES COP Sustainable development of urban areas is a challenge of key importance. It requires new, efficient, and user-friendly technologies and services, in particular in the areas of energy, transport and ICT. However, these solutions need integrated approaches, both in terms of research and development of advanced technological solutions, as well as deployment. The focus on smart cities technologies will result in commercial-scale solutions with a high market potential. 1. Automatically generate keywords from class names, descriptions, and related information (e. g. DBpedia, skos, etc. ) using term recognition tools 2. Enrich using word embeddings 3. Score the keywords according to how representative they are of that class 4. Generate prior probabilities using PMI for term combinations, based on frequency of co-occurrence
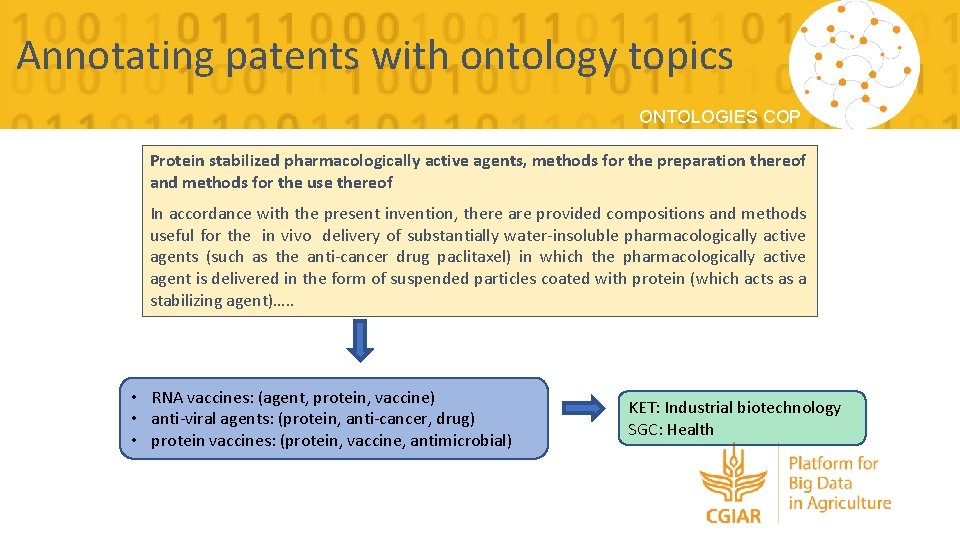
Annotating patents with ontology topics ONTOLOGIES COP Protein stabilized pharmacologically active agents, methods for the preparation thereof and methods for the use thereof In accordance with the present invention, there are provided compositions and methods useful for the in vivo delivery of substantially water-insoluble pharmacologically active agents (such as the anti-cancer drug paclitaxel) in which the pharmacologically active agent is delivered in the form of suspended particles coated with protein (which acts as a stabilizing agent)…. . • RNA vaccines: (agent, protein, vaccine) • anti-viral agents: (protein, anti-cancer, drug) • protein vaccines: (protein, vaccine, antimicrobial) KET: Industrial biotechnology SGC: Health
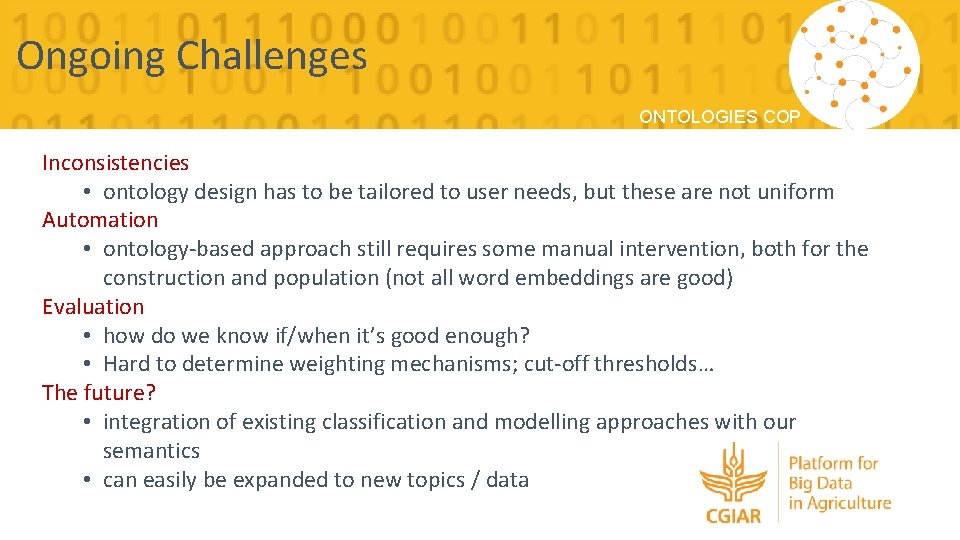
Ongoing Challenges ONTOLOGIES COP Inconsistencies • ontology design has to be tailored to user needs, but these are not uniform Automation • ontology-based approach still requires some manual intervention, both for the construction and population (not all word embeddings are good) Evaluation • how do we know if/when it’s good enough? • Hard to determine weighting mechanisms; cut-off thresholds… The future? • integration of existing classification and modelling approaches with our semantics • can easily be expanded to new topics / data
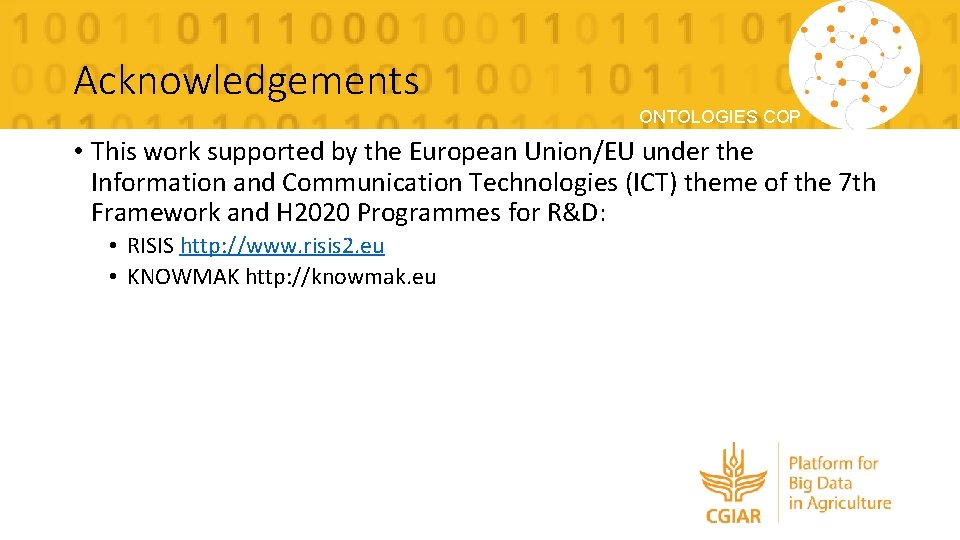
Acknowledgements ONTOLOGIES COP • This work supported by the European Union/EU under the Information and Communication Technologies (ICT) theme of the 7 th Framework and H 2020 Programmes for R&D: • RISIS http: //www. risis 2. eu • KNOWMAK http: //knowmak. eu
Obo
Good cop bad cop interrogation
Cop 1 cop 2
Multipurpose refrigeration systems with a single compressor
Brayton cycle refrigeration
Isppia
Refrigeration cycle ts diagram
Dikdörtgene benzeyen cisimler
Les polly cop
Cop 4910
Cop 4710
Cop 4710 ucf
Montagne ucf
Cop 2500
Cop 2500
Anima assoclima
Cop2500
Brooklyn cop
Audit cop
Cop 16