Neuse Estuary Eutrophication Model Predictions of Water Quality
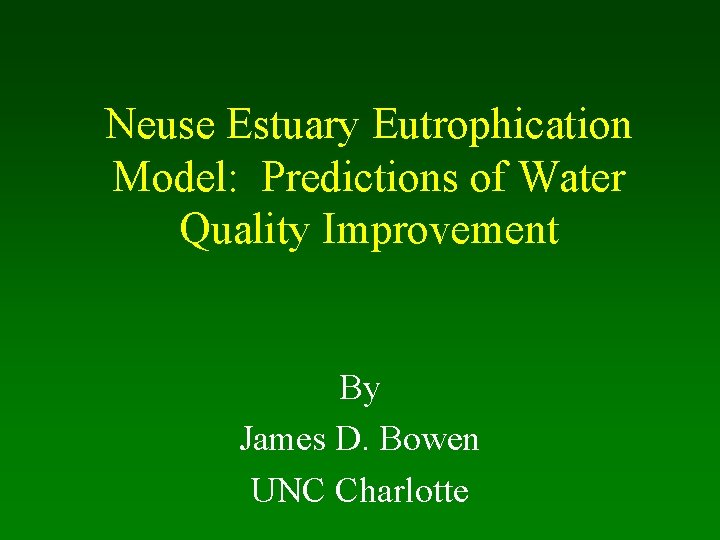
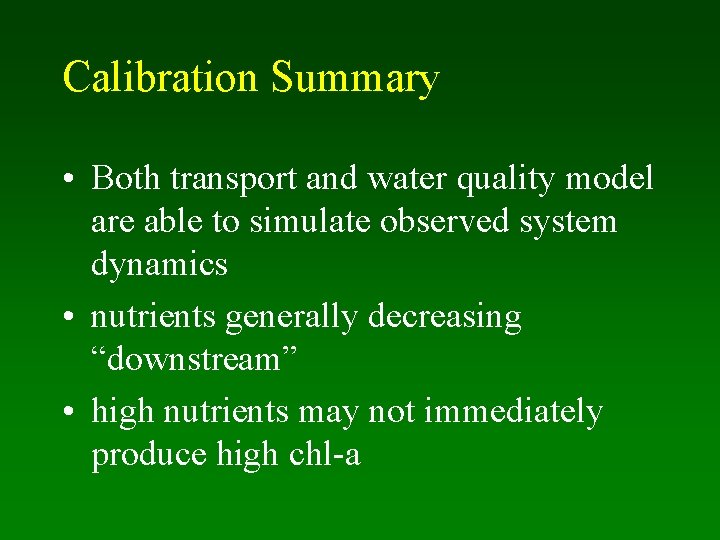
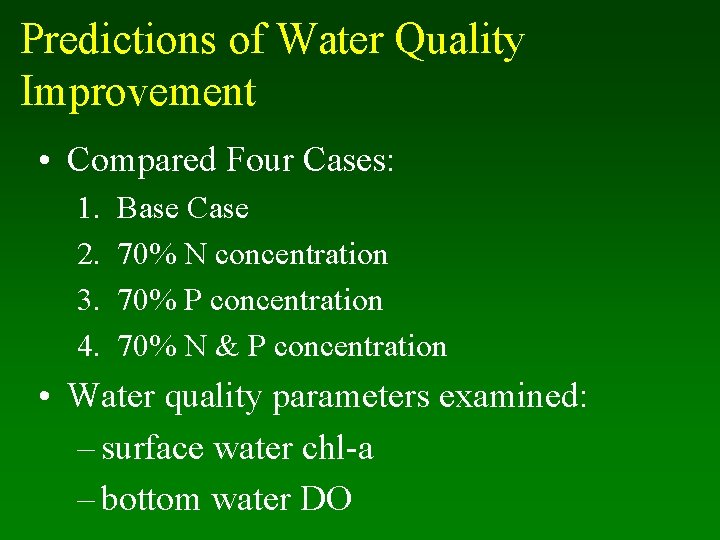
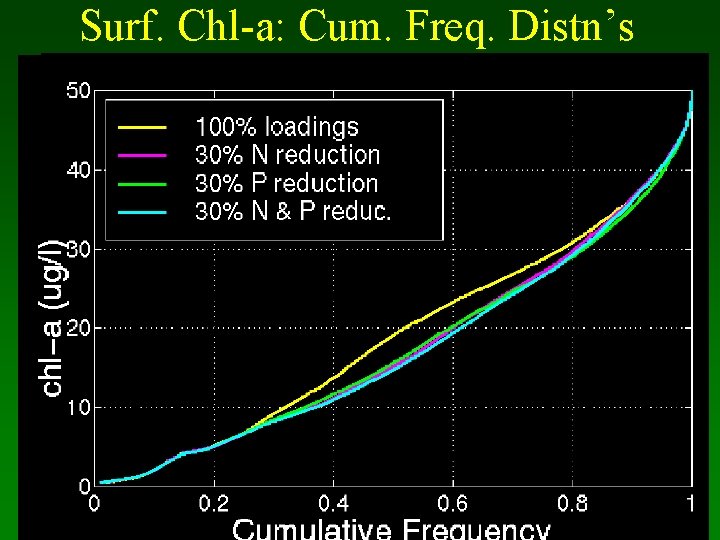
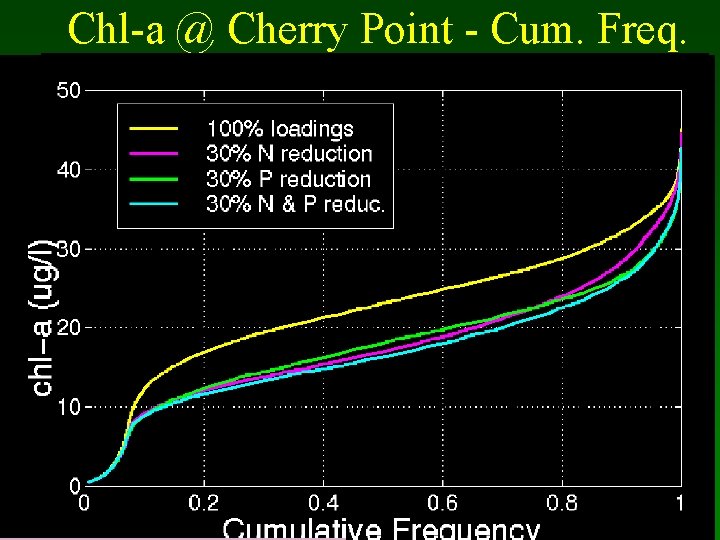
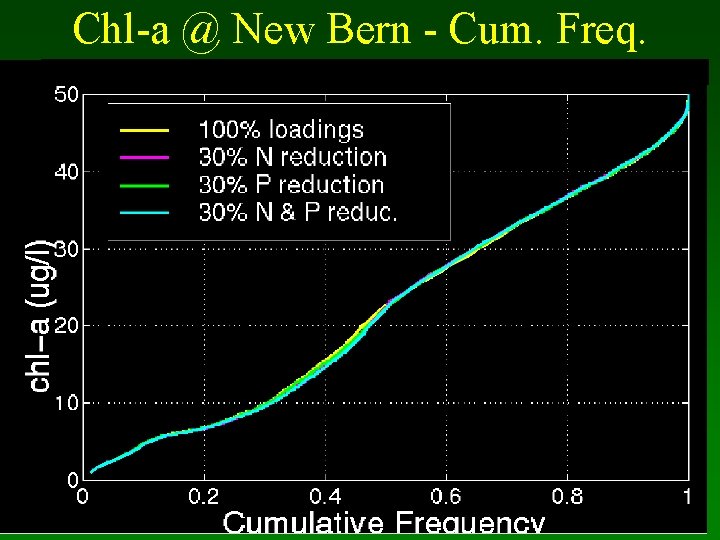
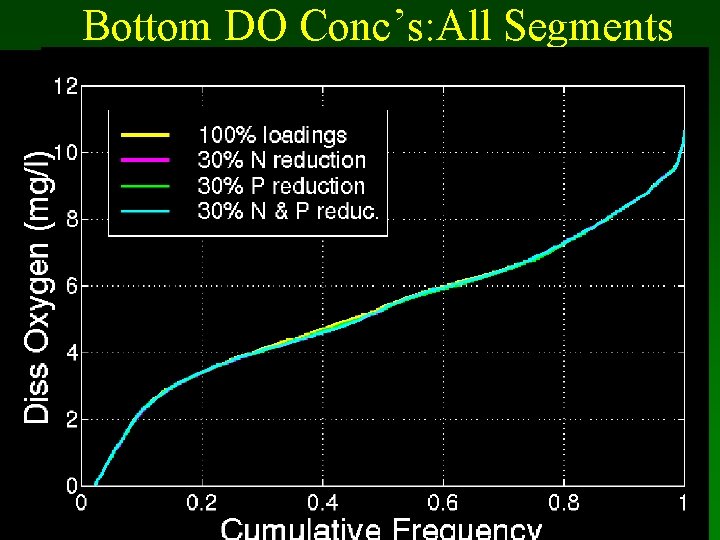
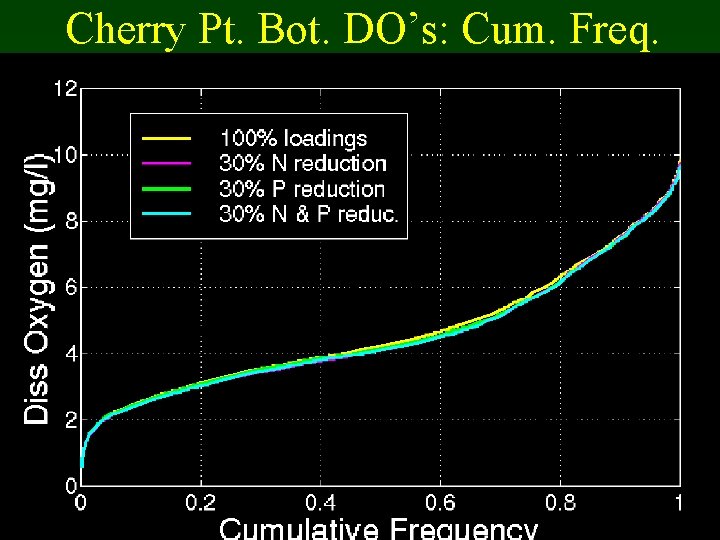
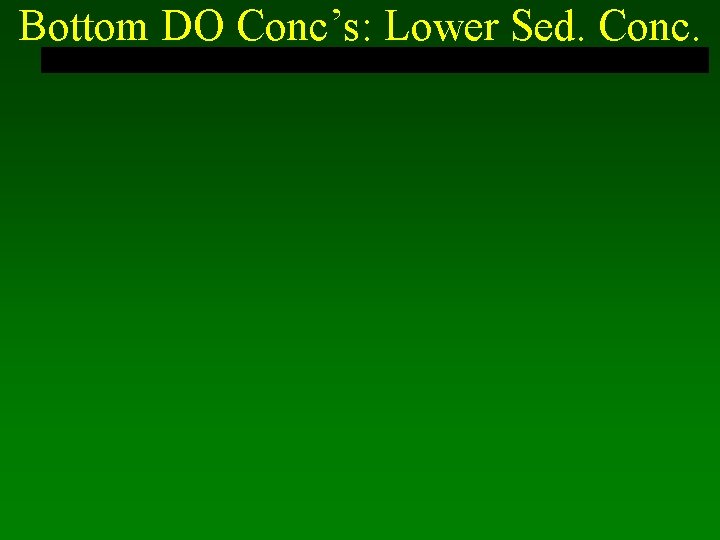
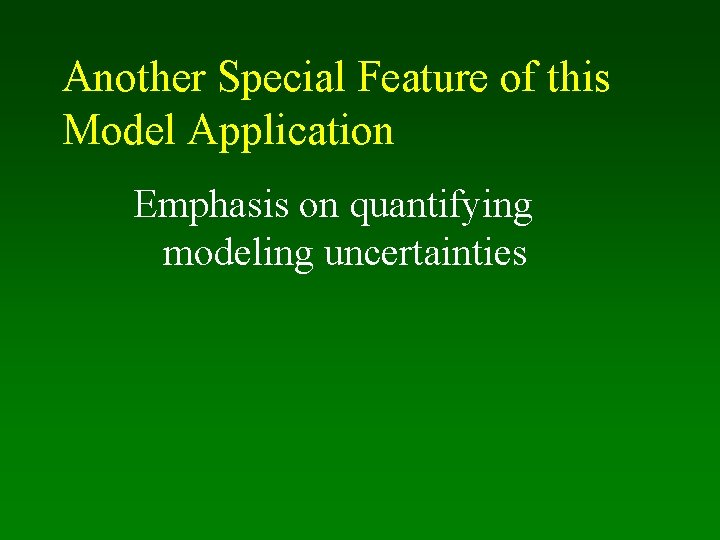
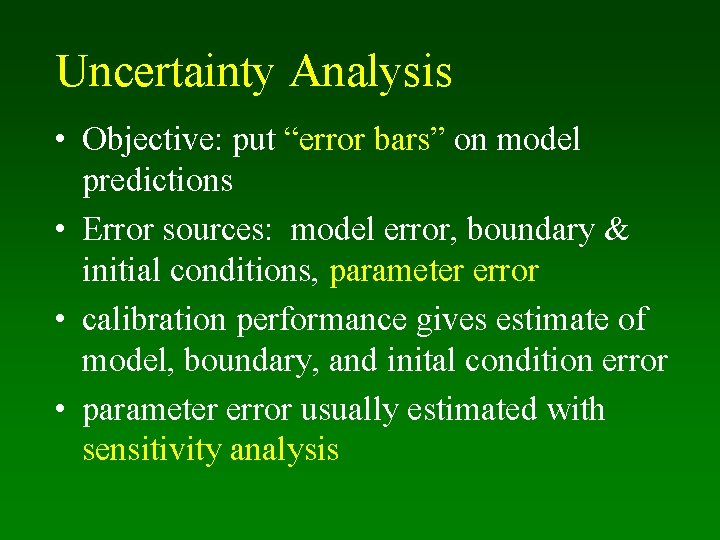
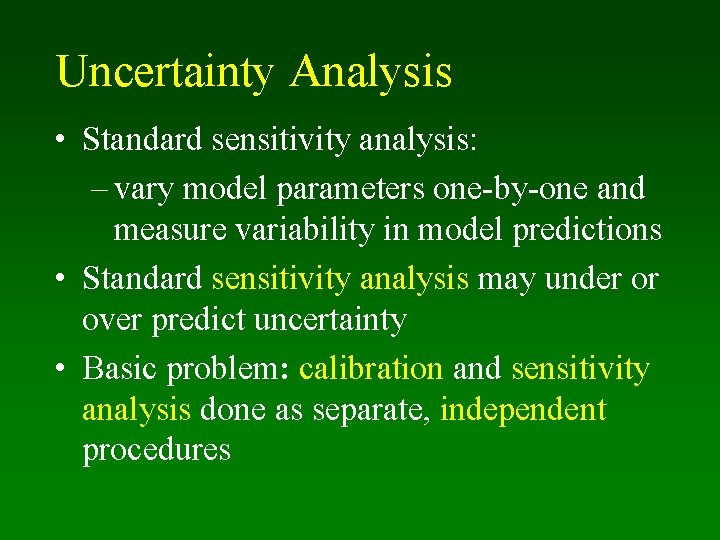
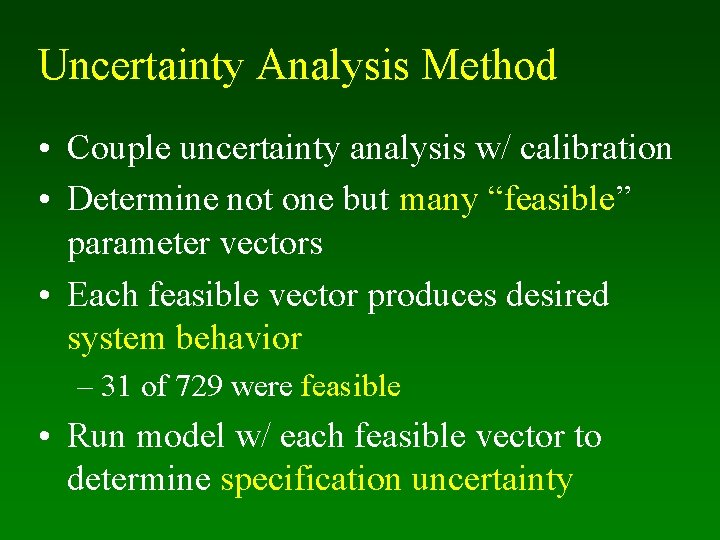
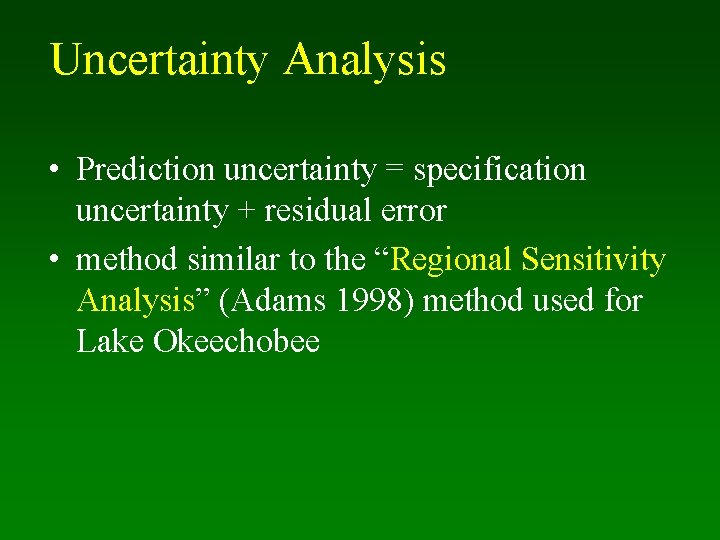
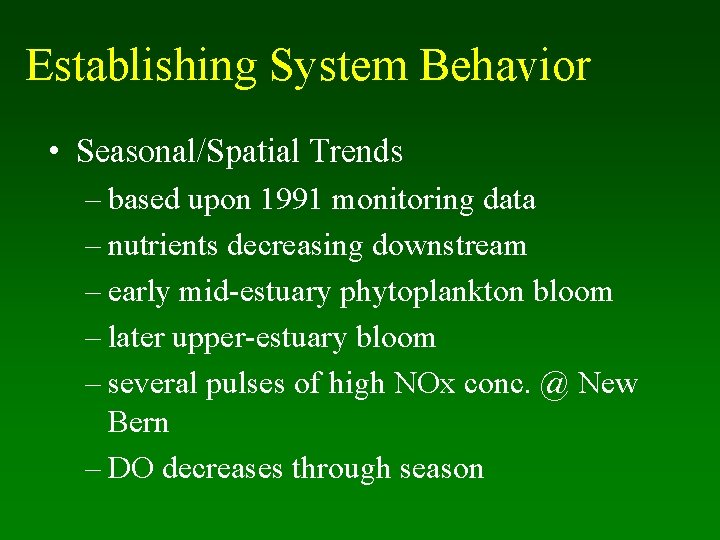
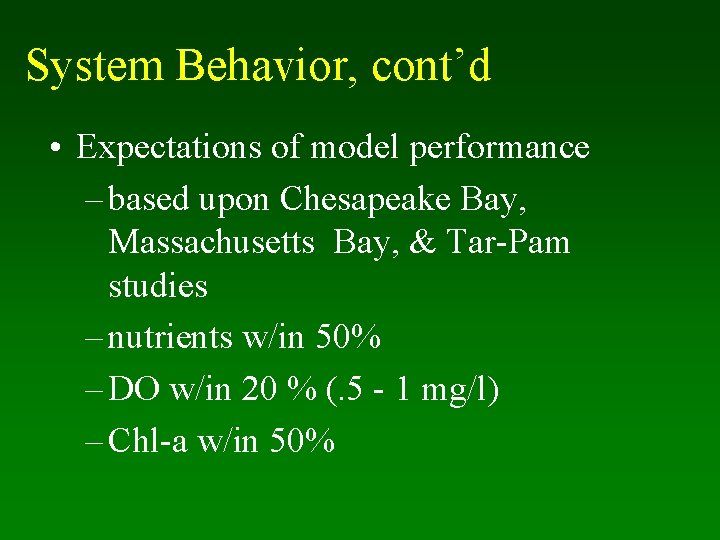
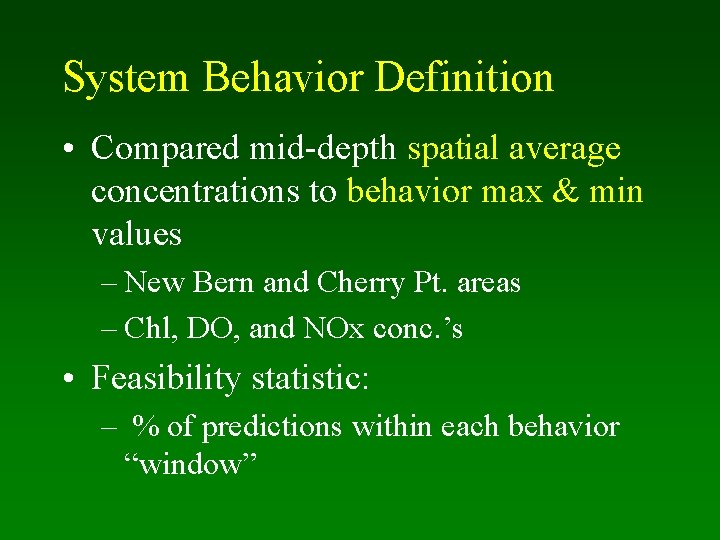
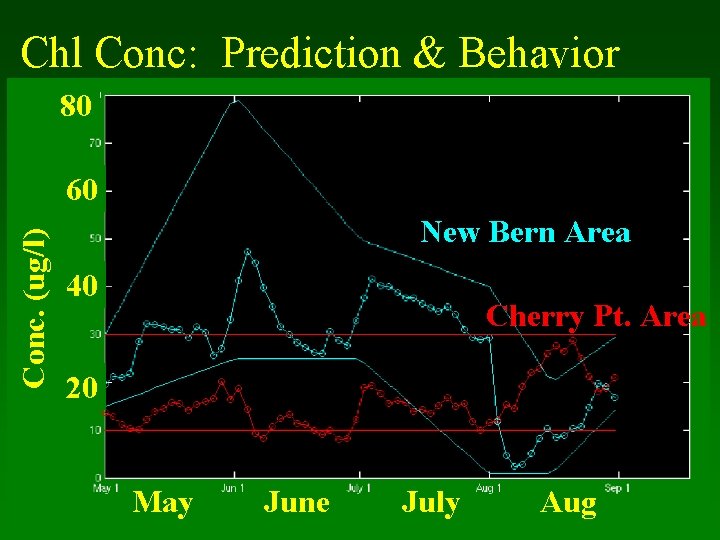
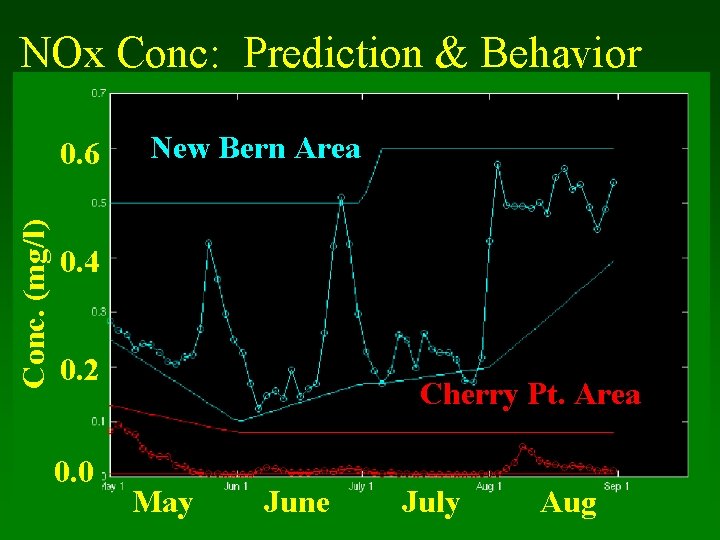
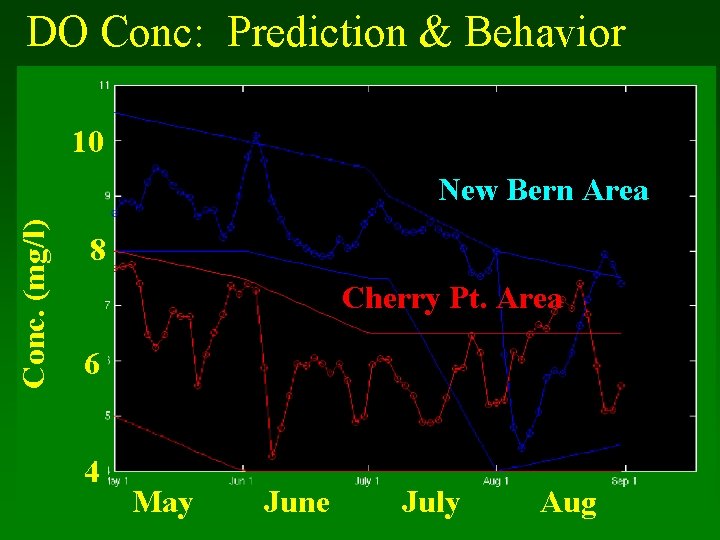
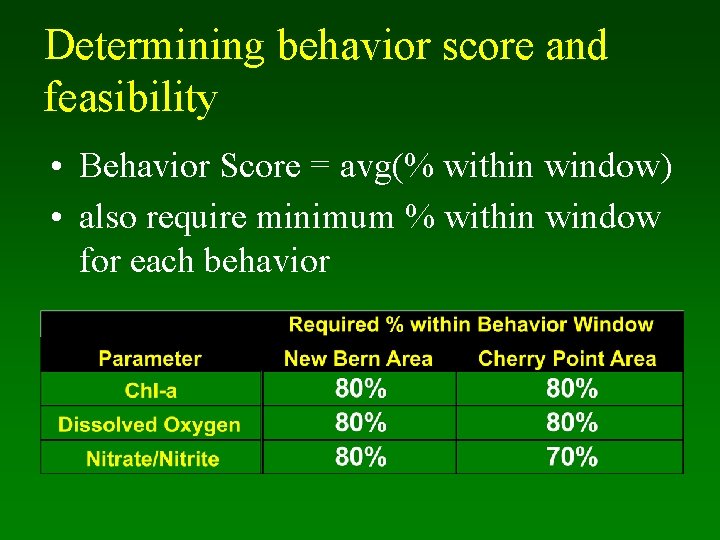
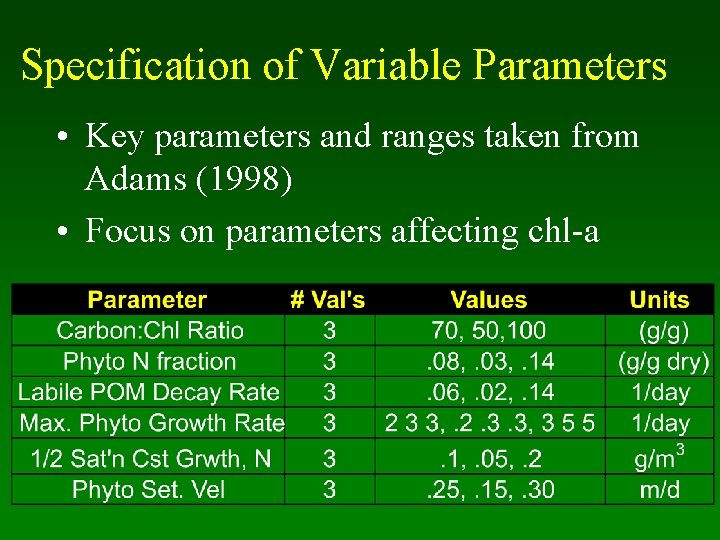
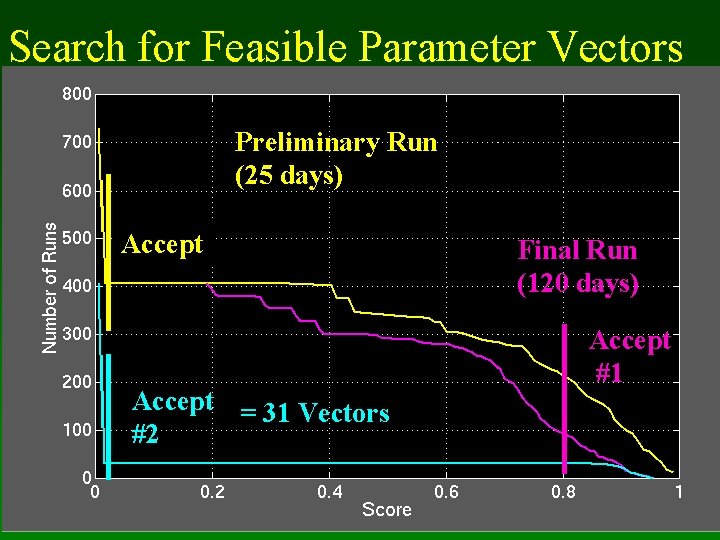
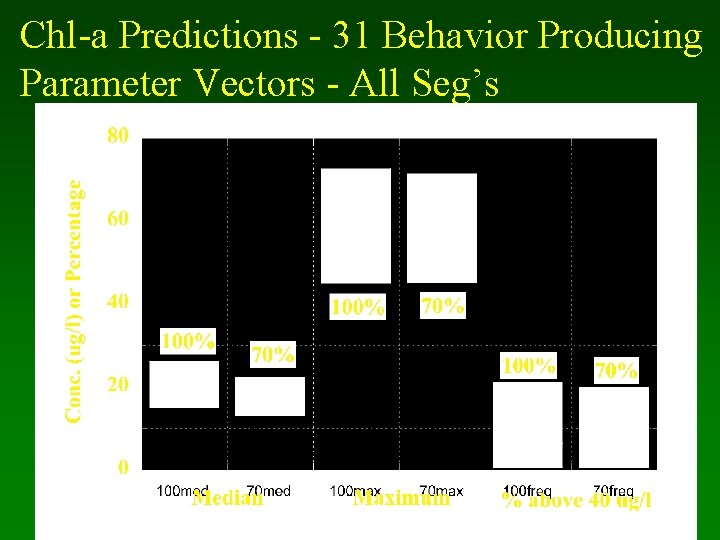
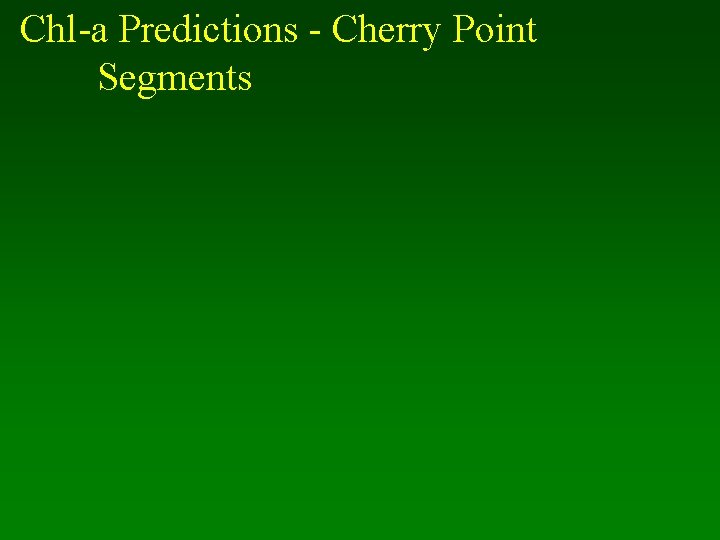
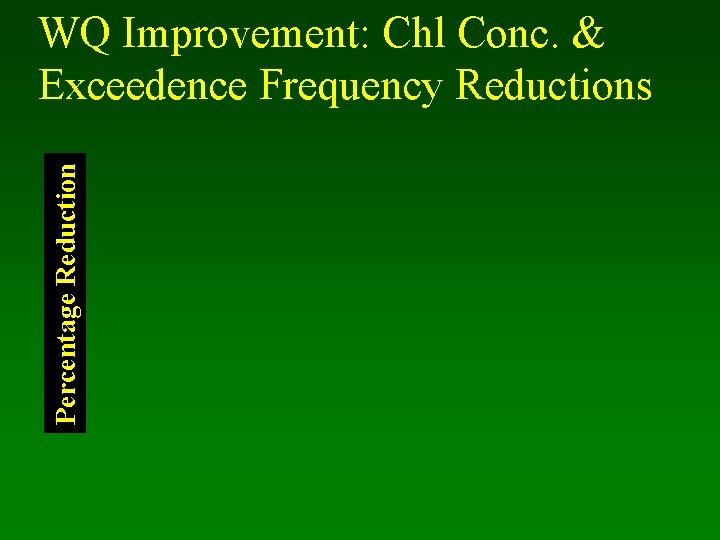
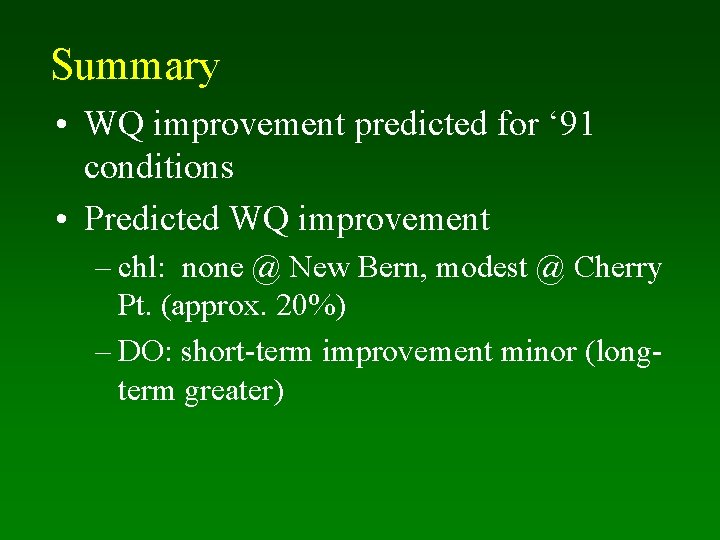
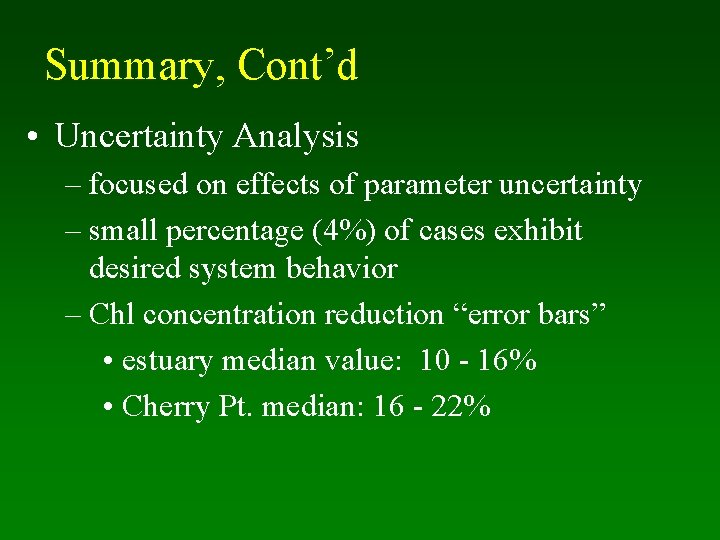
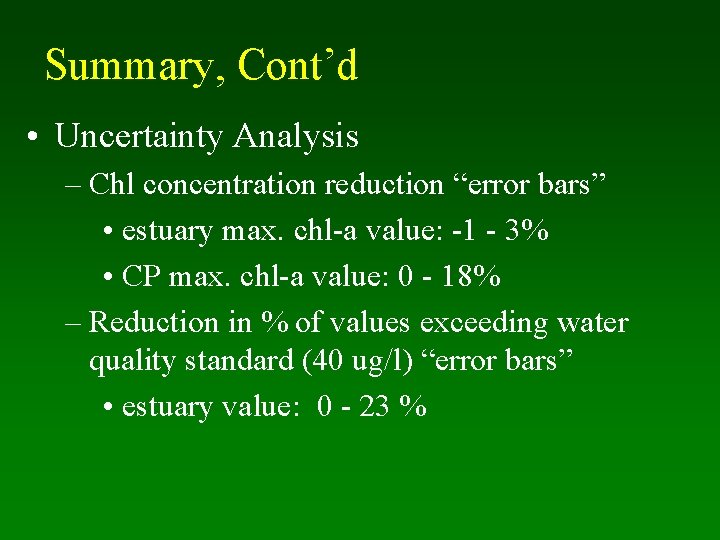
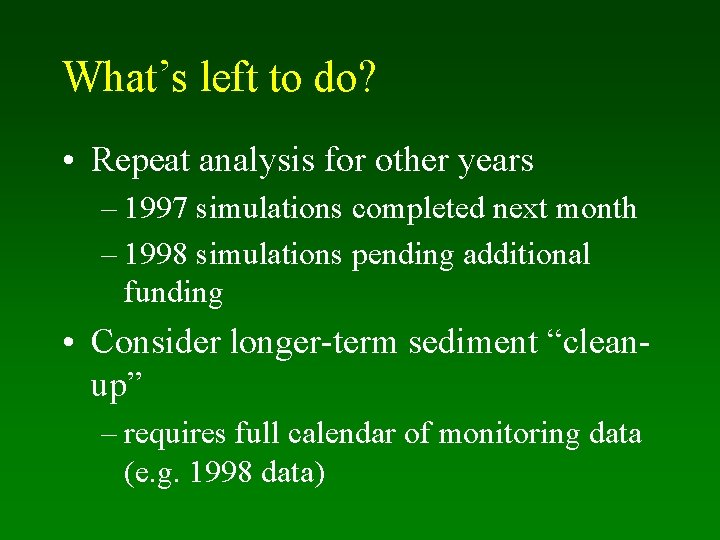
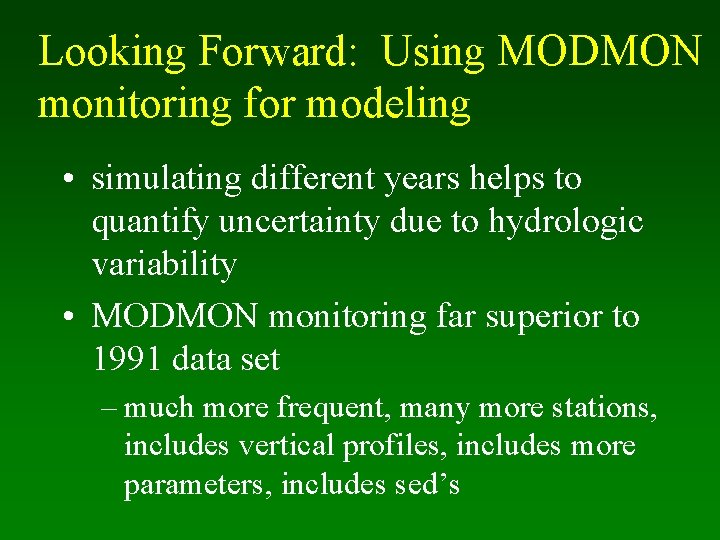
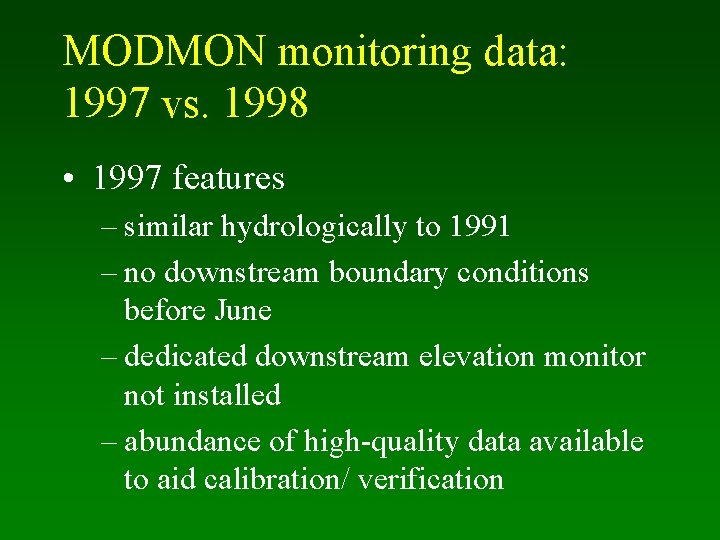
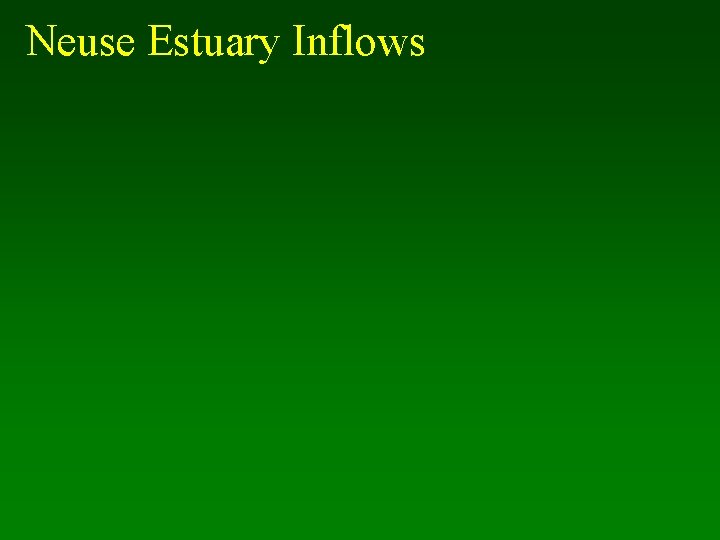
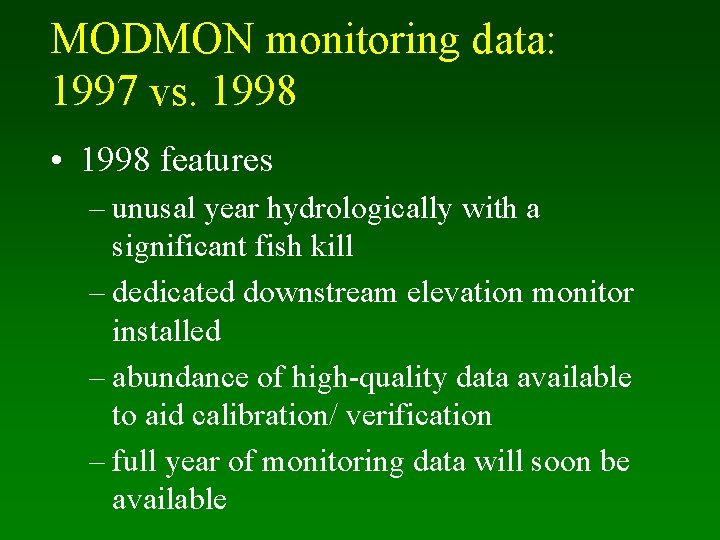
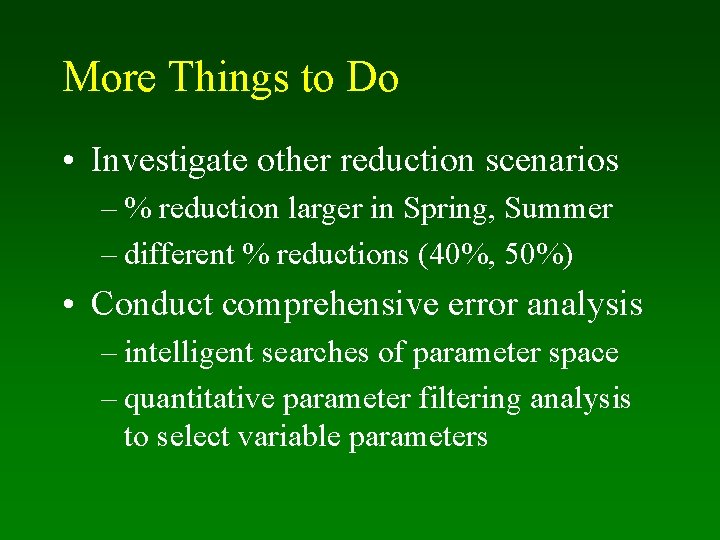
- Slides: 35
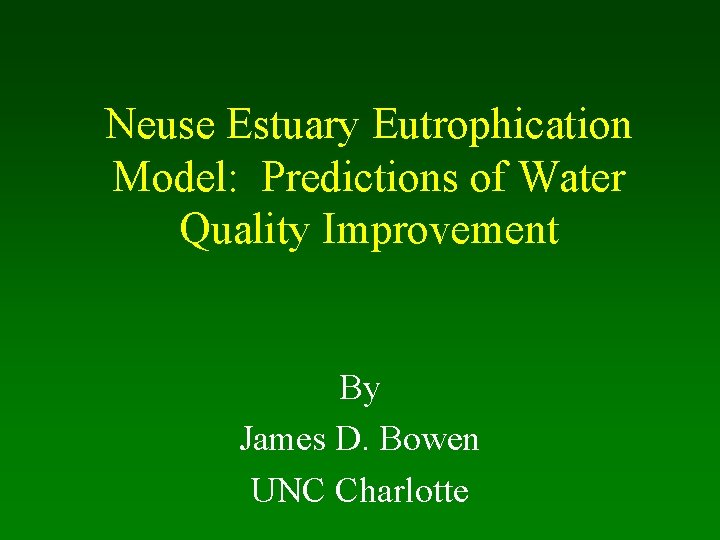
Neuse Estuary Eutrophication Model: Predictions of Water Quality Improvement By James D. Bowen UNC Charlotte
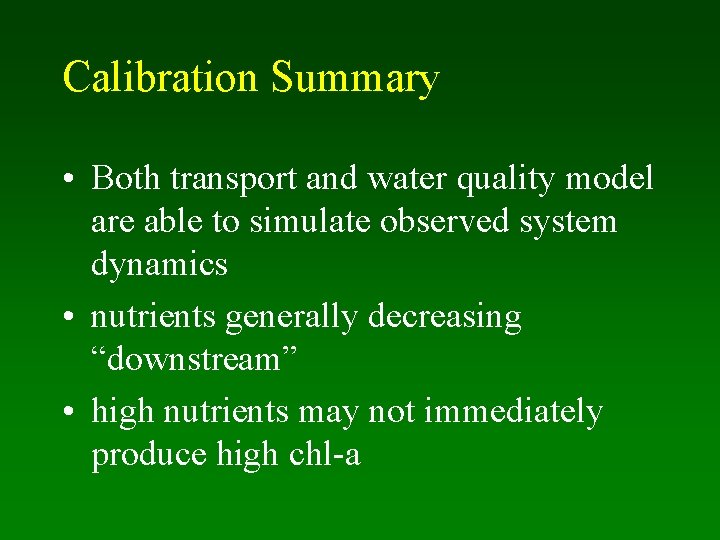
Calibration Summary • Both transport and water quality model are able to simulate observed system dynamics • nutrients generally decreasing “downstream” • high nutrients may not immediately produce high chl-a
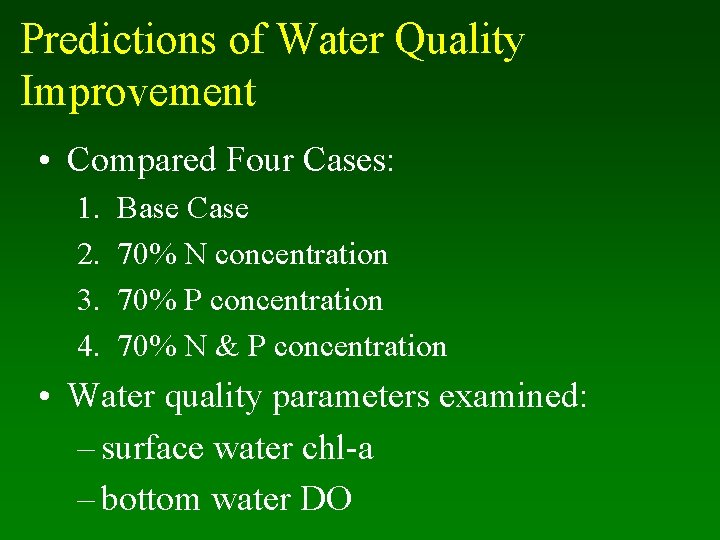
Predictions of Water Quality Improvement • Compared Four Cases: 1. 2. 3. 4. Base Case 70% N concentration 70% P concentration 70% N & P concentration • Water quality parameters examined: – surface water chl-a – bottom water DO
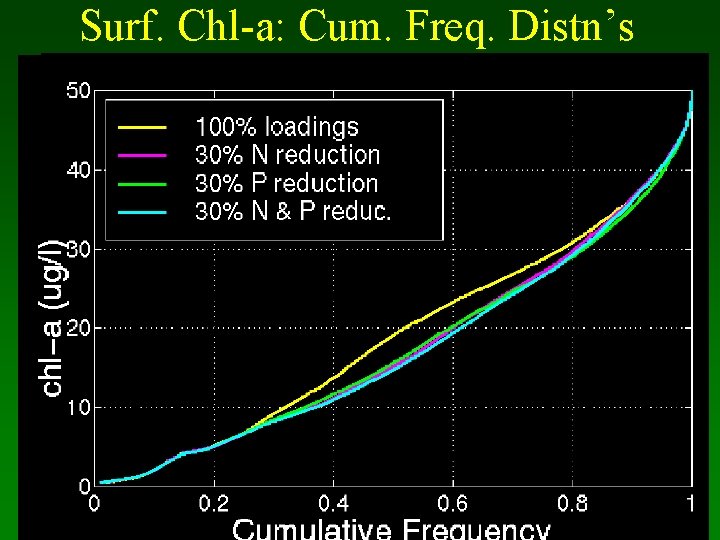
Surf. Chl-a: Cum. Freq. Distn’s
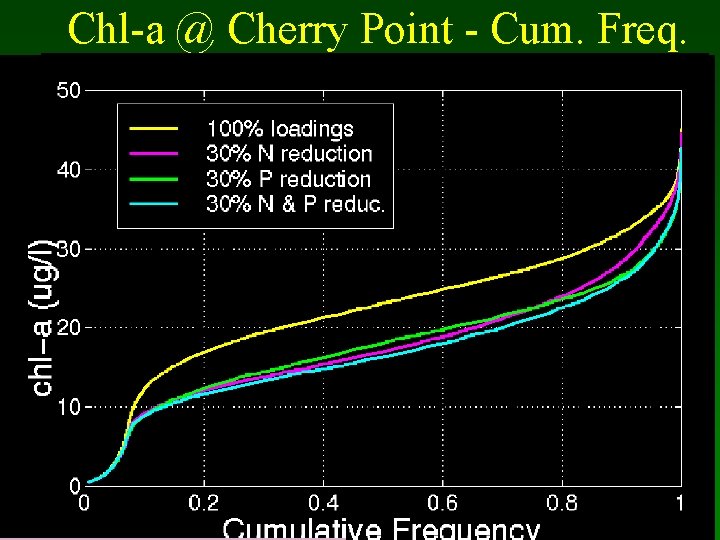
Chl-a @ Cherry Point - Cum. Freq.
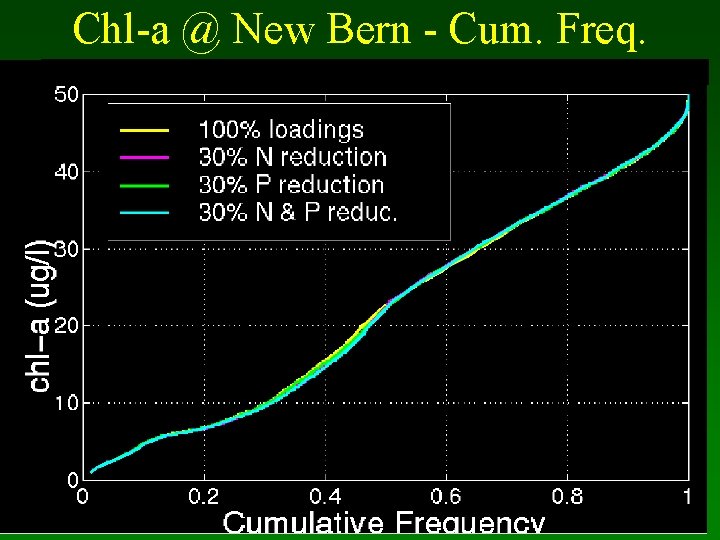
Chl-a @ New Bern - Cum. Freq.
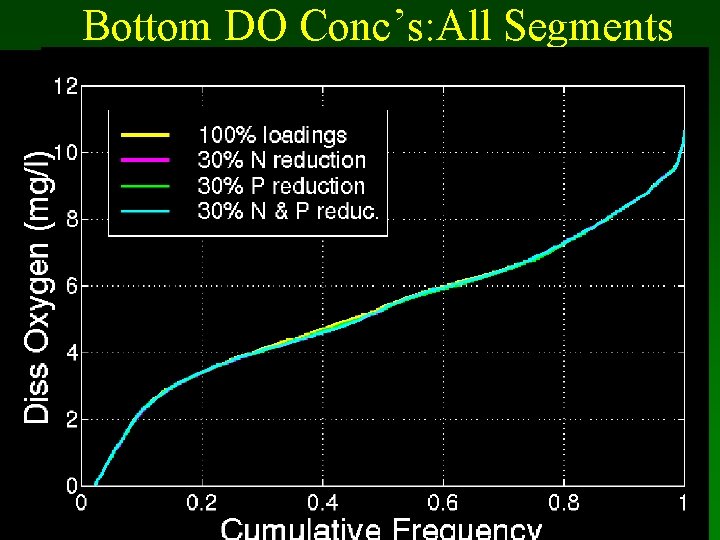
Bottom DO Conc’s: All Segments
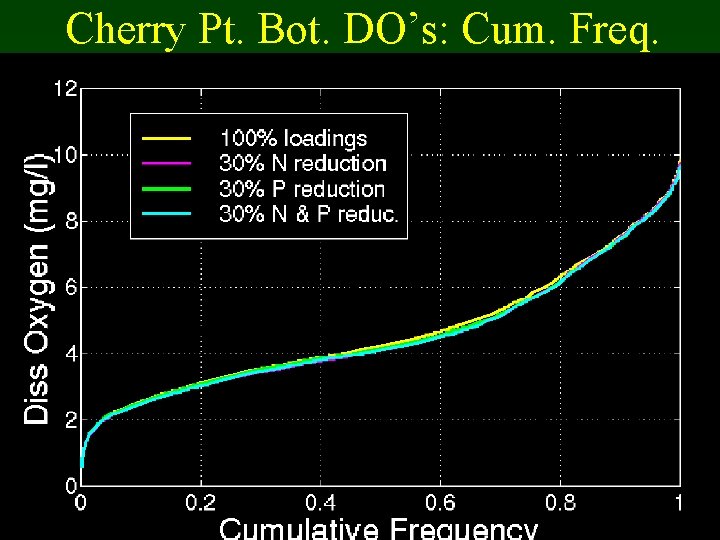
Cherry Pt. Bot. DO’s: Cum. Freq.
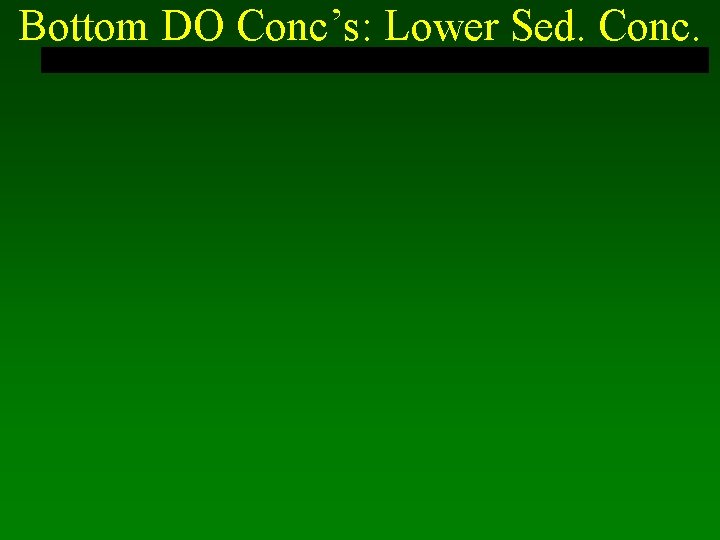
Bottom DO Conc’s: Lower Sed. Conc.
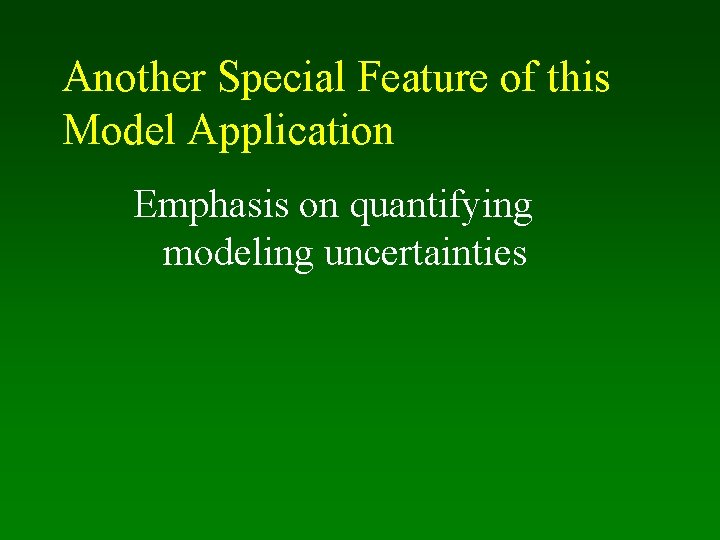
Another Special Feature of this Model Application Emphasis on quantifying modeling uncertainties
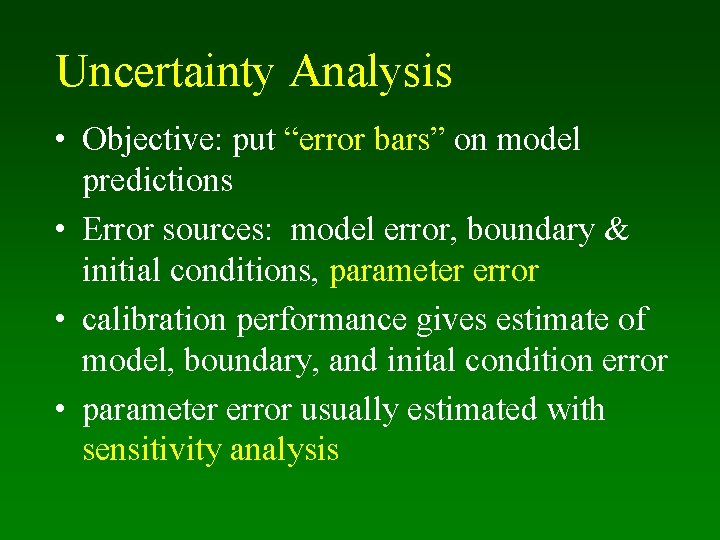
Uncertainty Analysis • Objective: put “error bars” on model predictions • Error sources: model error, boundary & initial conditions, parameter error • calibration performance gives estimate of model, boundary, and inital condition error • parameter error usually estimated with sensitivity analysis
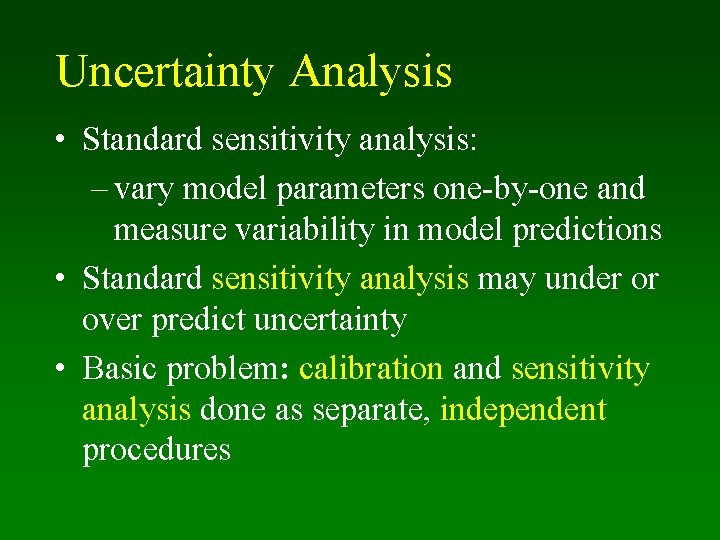
Uncertainty Analysis • Standard sensitivity analysis: – vary model parameters one-by-one and measure variability in model predictions • Standard sensitivity analysis may under or over predict uncertainty • Basic problem: calibration and sensitivity analysis done as separate, independent procedures
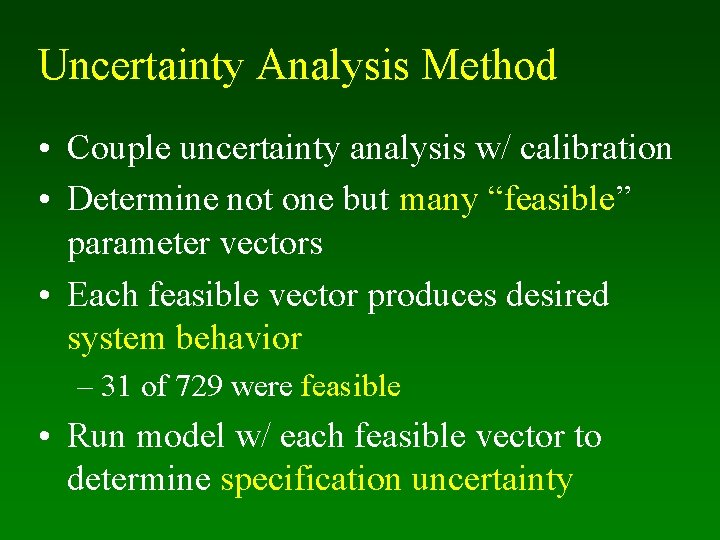
Uncertainty Analysis Method • Couple uncertainty analysis w/ calibration • Determine not one but many “feasible” parameter vectors • Each feasible vector produces desired system behavior – 31 of 729 were feasible • Run model w/ each feasible vector to determine specification uncertainty
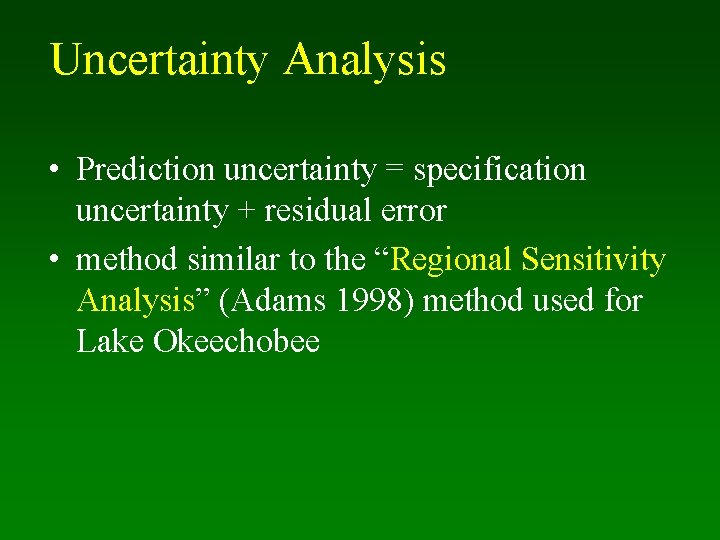
Uncertainty Analysis • Prediction uncertainty = specification uncertainty + residual error • method similar to the “Regional Sensitivity Analysis” (Adams 1998) method used for Lake Okeechobee
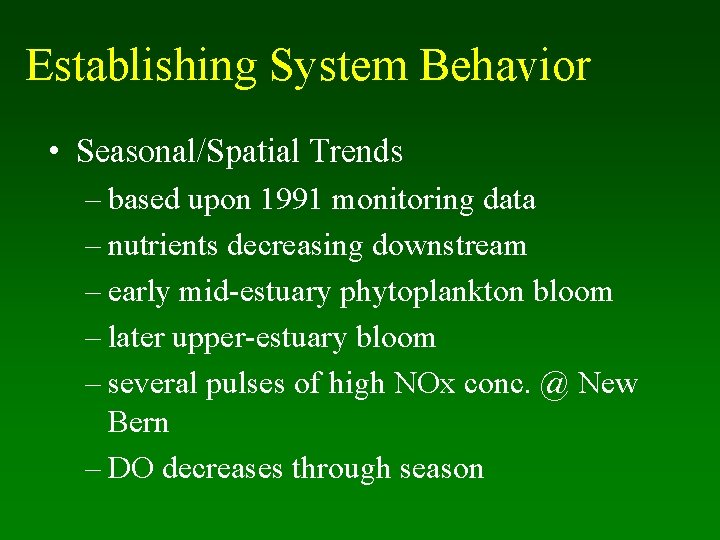
Establishing System Behavior • Seasonal/Spatial Trends – based upon 1991 monitoring data – nutrients decreasing downstream – early mid-estuary phytoplankton bloom – later upper-estuary bloom – several pulses of high NOx conc. @ New Bern – DO decreases through season
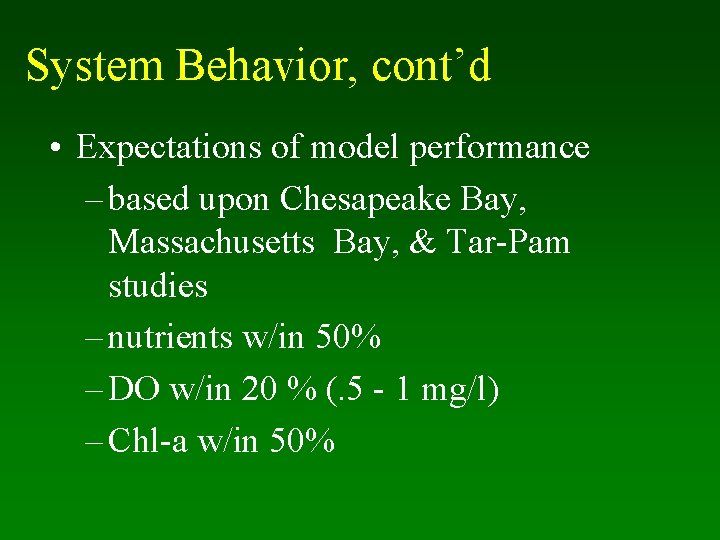
System Behavior, cont’d • Expectations of model performance – based upon Chesapeake Bay, Massachusetts Bay, & Tar-Pam studies – nutrients w/in 50% – DO w/in 20 % (. 5 - 1 mg/l) – Chl-a w/in 50%
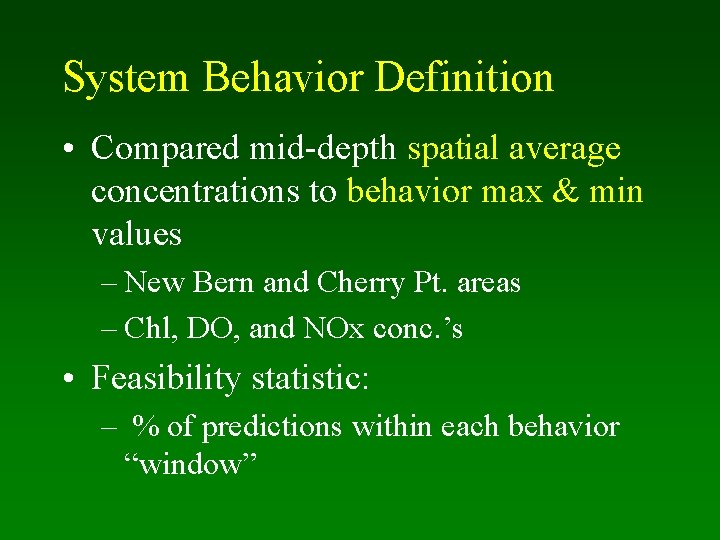
System Behavior Definition • Compared mid-depth spatial average concentrations to behavior max & min values – New Bern and Cherry Pt. areas – Chl, DO, and NOx conc. ’s • Feasibility statistic: – % of predictions within each behavior “window”
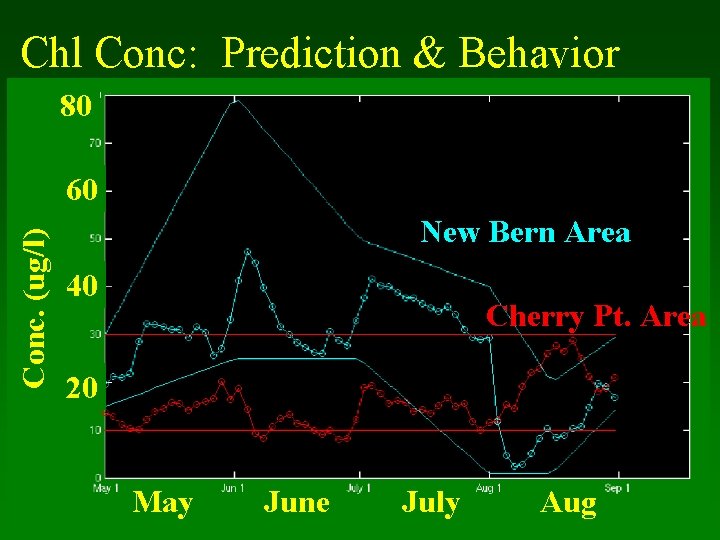
Chl Conc: Prediction & Behavior 80 Conc. (ug/l) 60 New Bern Area 40 Cherry Pt. Area 20 May June July Aug
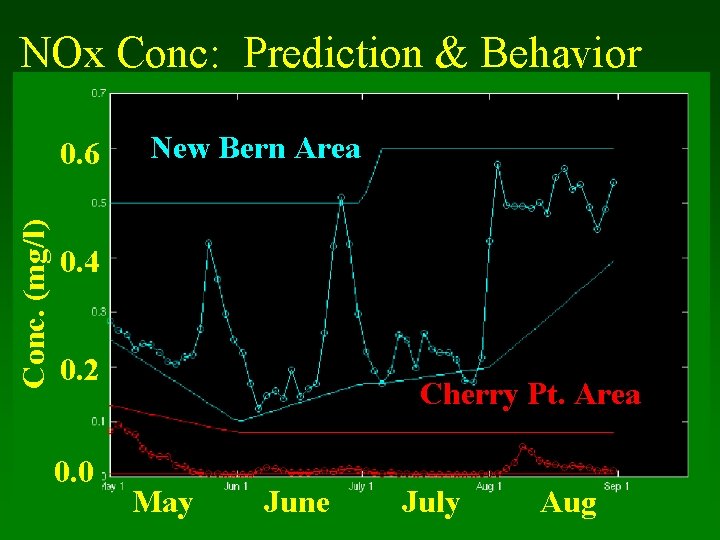
NOx Conc: Prediction & Behavior Conc. (mg/l) 0. 6 New Bern Area 0. 4 0. 2 0. 0 Cherry Pt. Area May June July Aug
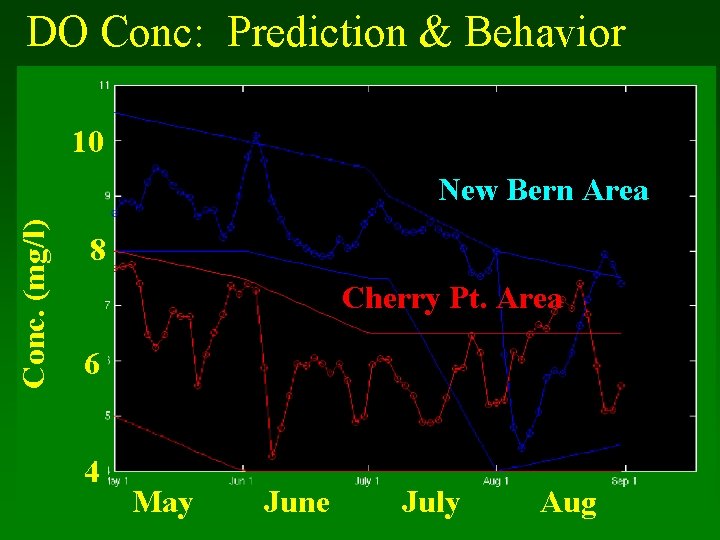
DO Conc: Prediction & Behavior 10 Conc. (mg/l) New Bern Area 8 Cherry Pt. Area 6 4 May June July Aug
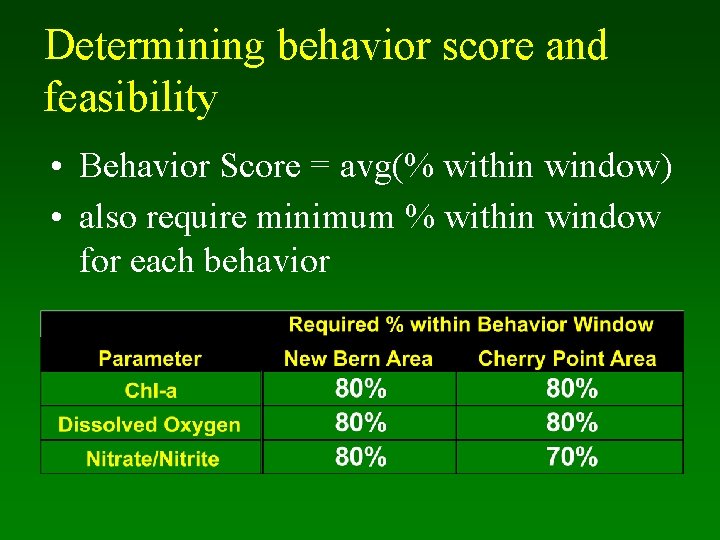
Determining behavior score and feasibility • Behavior Score = avg(% within window) • also require minimum % within window for each behavior
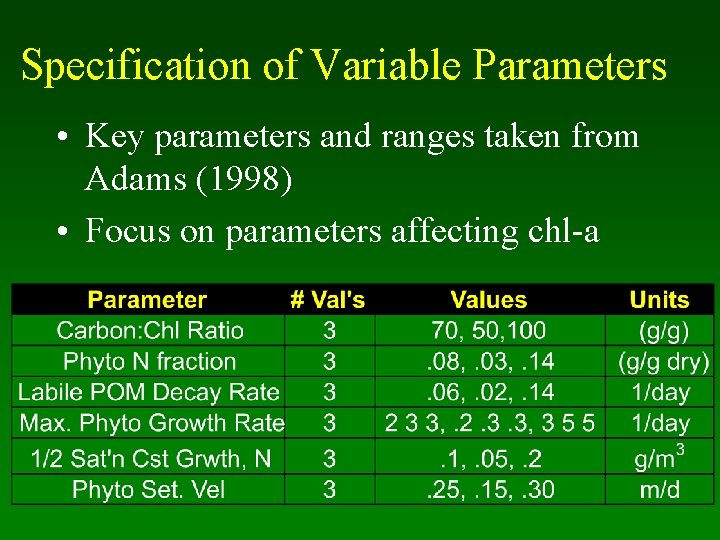
Specification of Variable Parameters • Key parameters and ranges taken from Adams (1998) • Focus on parameters affecting chl-a
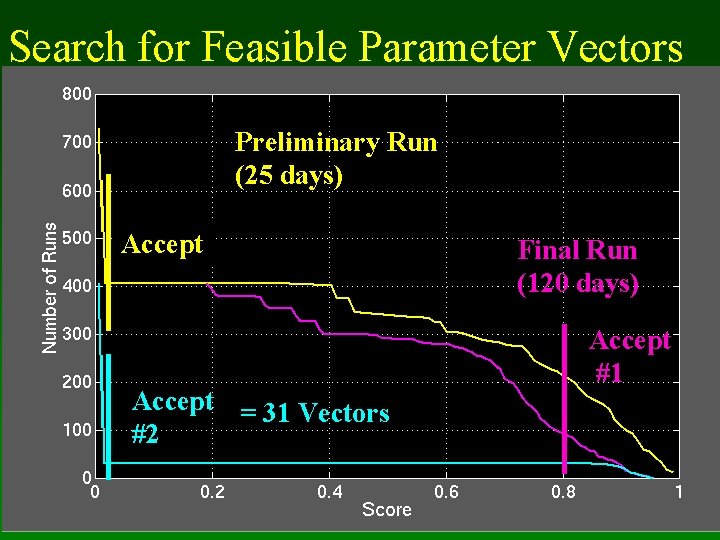
Search for Feasible Parameter Vectors Preliminary Run (25 days) Accept = 31 Vectors #2 Final Run (120 days) Accept #1
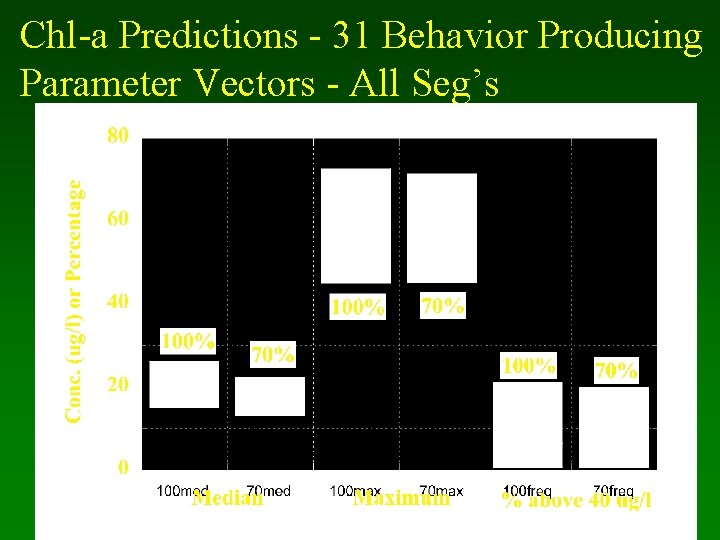
Chl-a Predictions - 31 Behavior Producing Parameter Vectors - All Seg’s
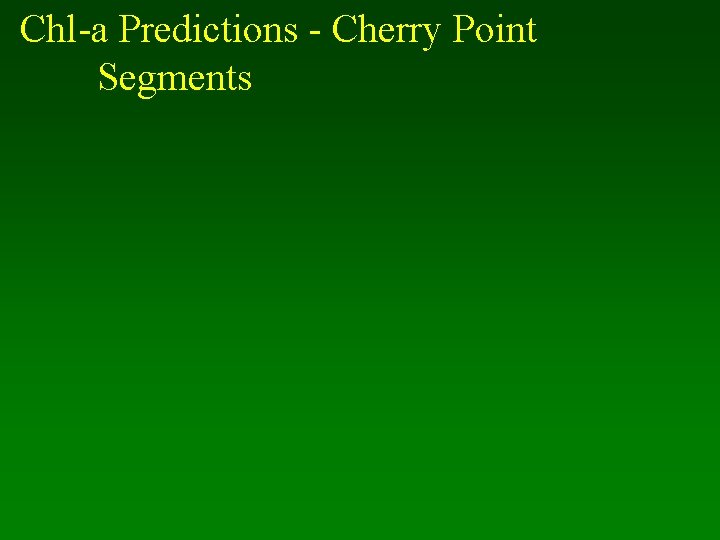
Chl-a Predictions - Cherry Point Segments
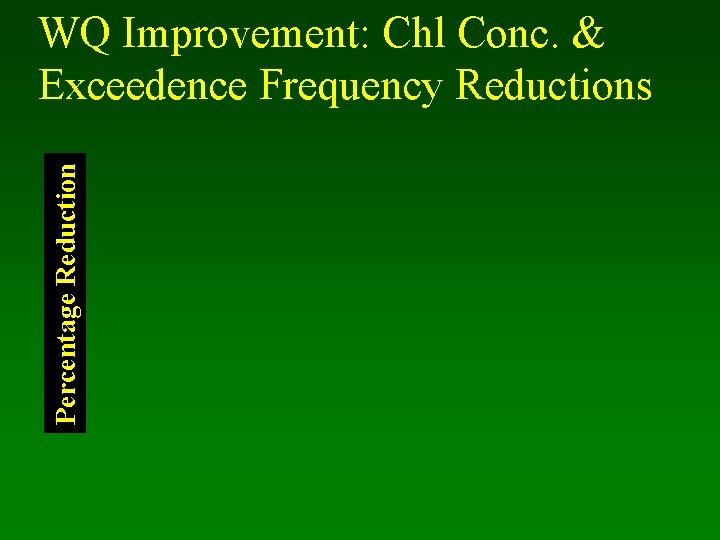
Percentage Reduction WQ Improvement: Chl Conc. & Exceedence Frequency Reductions
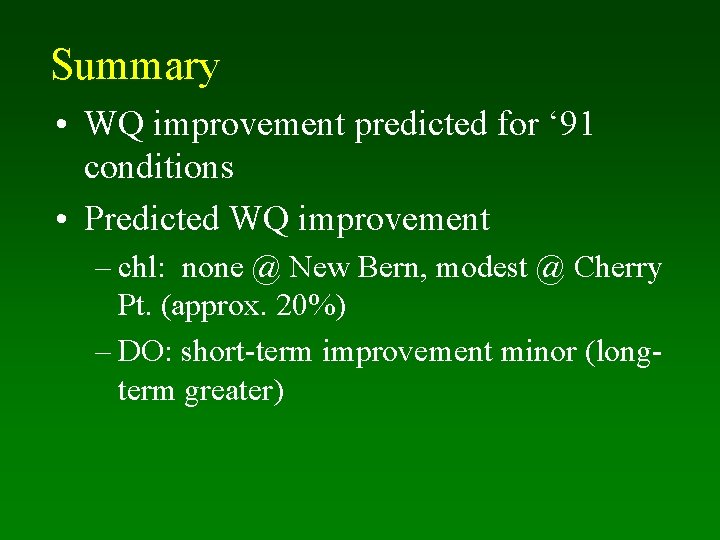
Summary • WQ improvement predicted for ‘ 91 conditions • Predicted WQ improvement – chl: none @ New Bern, modest @ Cherry Pt. (approx. 20%) – DO: short-term improvement minor (longterm greater)
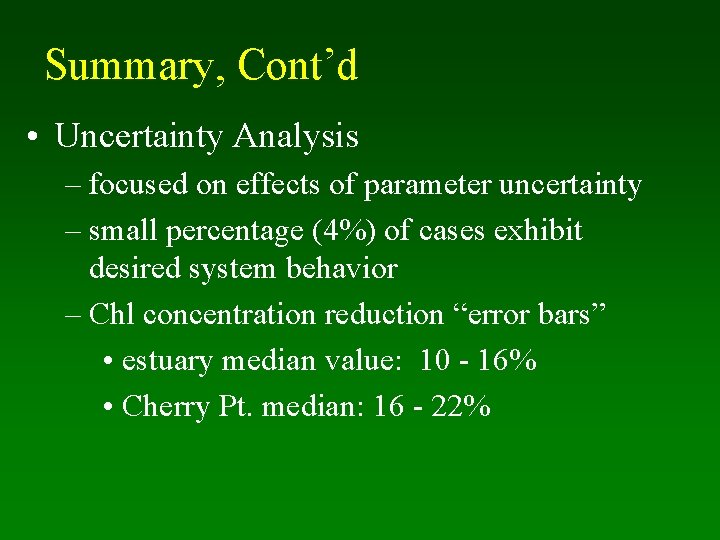
Summary, Cont’d • Uncertainty Analysis – focused on effects of parameter uncertainty – small percentage (4%) of cases exhibit desired system behavior – Chl concentration reduction “error bars” • estuary median value: 10 - 16% • Cherry Pt. median: 16 - 22%
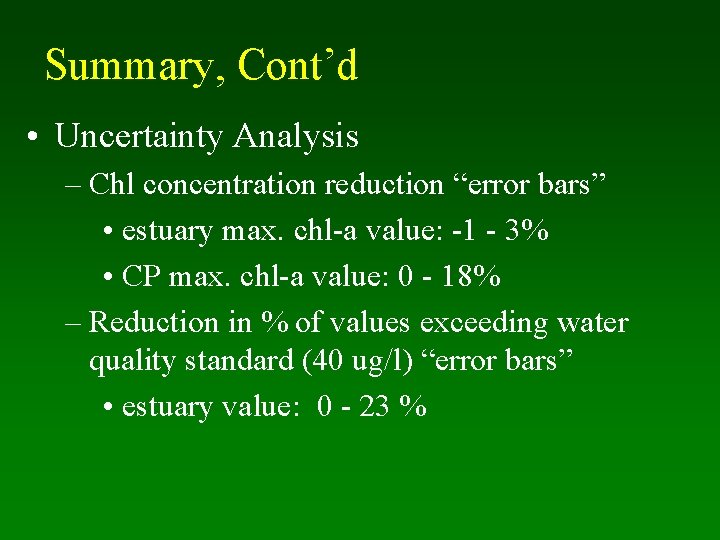
Summary, Cont’d • Uncertainty Analysis – Chl concentration reduction “error bars” • estuary max. chl-a value: -1 - 3% • CP max. chl-a value: 0 - 18% – Reduction in % of values exceeding water quality standard (40 ug/l) “error bars” • estuary value: 0 - 23 %
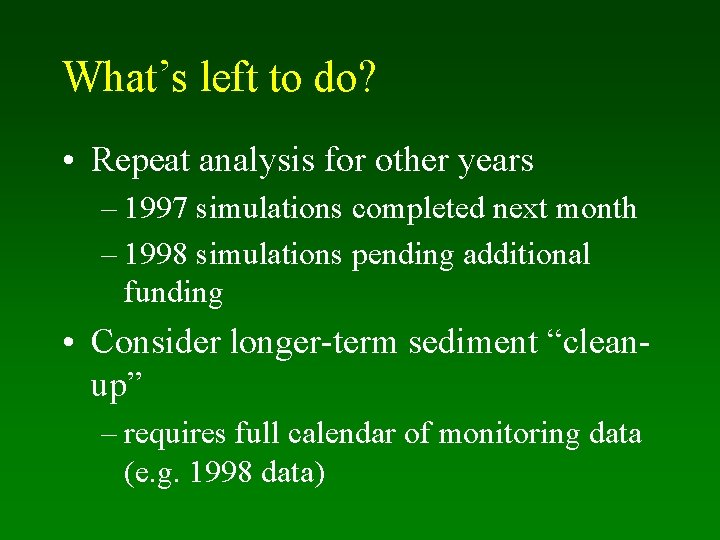
What’s left to do? • Repeat analysis for other years – 1997 simulations completed next month – 1998 simulations pending additional funding • Consider longer-term sediment “cleanup” – requires full calendar of monitoring data (e. g. 1998 data)
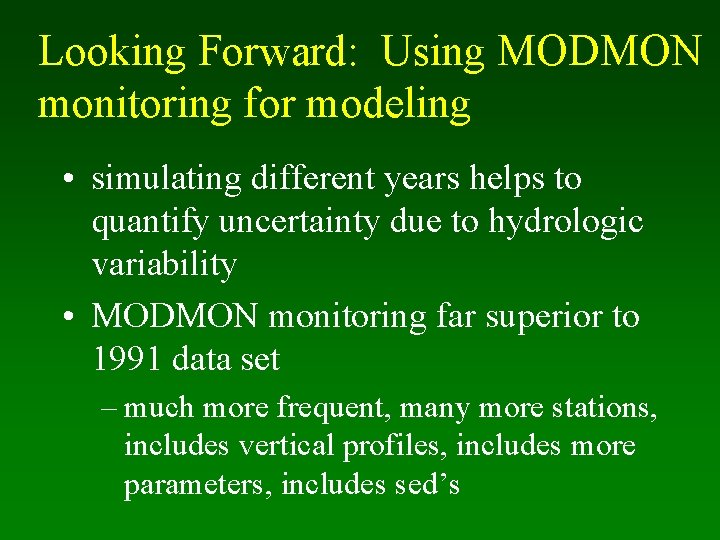
Looking Forward: Using MODMON monitoring for modeling • simulating different years helps to quantify uncertainty due to hydrologic variability • MODMON monitoring far superior to 1991 data set – much more frequent, many more stations, includes vertical profiles, includes more parameters, includes sed’s
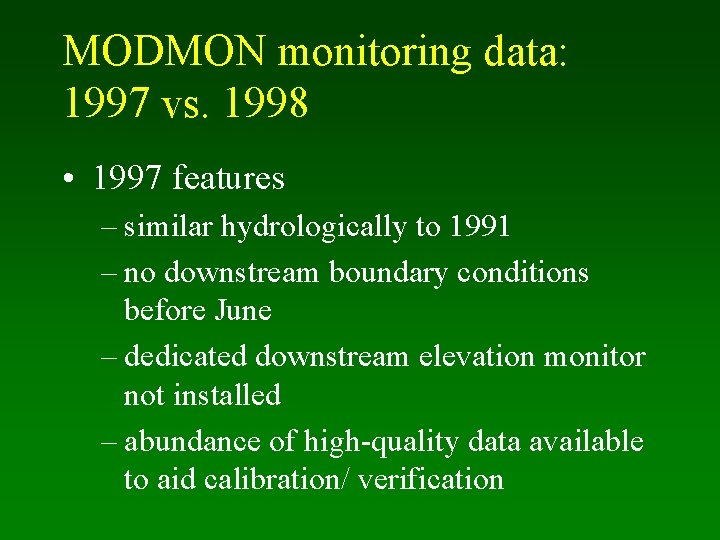
MODMON monitoring data: 1997 vs. 1998 • 1997 features – similar hydrologically to 1991 – no downstream boundary conditions before June – dedicated downstream elevation monitor not installed – abundance of high-quality data available to aid calibration/ verification
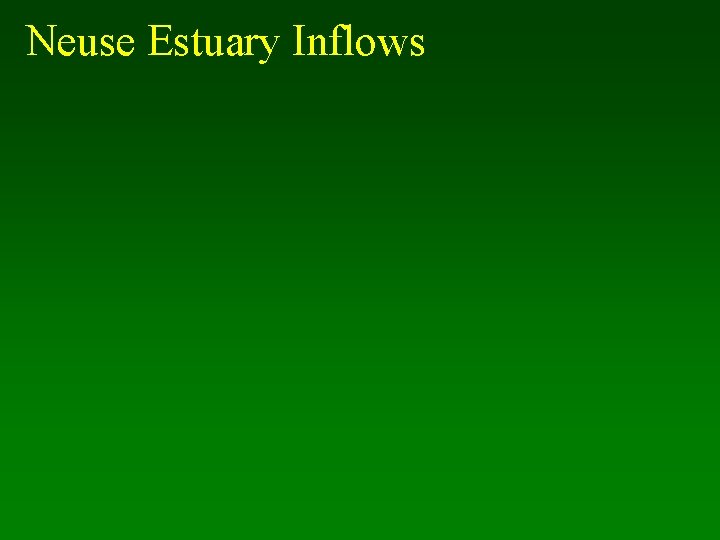
Neuse Estuary Inflows
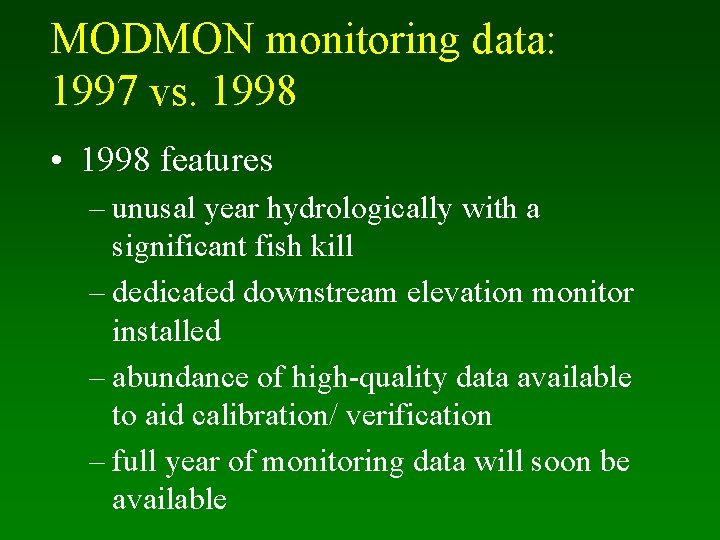
MODMON monitoring data: 1997 vs. 1998 • 1998 features – unusal year hydrologically with a significant fish kill – dedicated downstream elevation monitor installed – abundance of high-quality data available to aid calibration/ verification – full year of monitoring data will soon be available
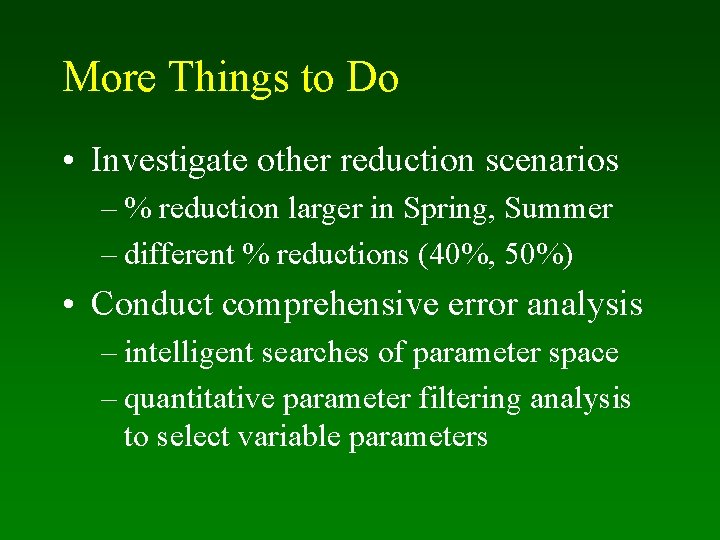
More Things to Do • Investigate other reduction scenarios – % reduction larger in Spring, Summer – different % reductions (40%, 50%) • Conduct comprehensive error analysis – intelligent searches of parameter space – quantitative parameter filtering analysis to select variable parameters
Water and water and water water
Eutrophication steps
Eutrophication bbc
Point source pollution
Eutrophication
Primary succession comic strip
Eutrophication
Eutrophication
Eutrophication
Eutrophication
Eutrophication
Eutrophication
Estuary biome
What is a salt wedge estuary
Estuary circulation
Ecosystem foldable
Estuary circulation
Drowned river estuary
Pavis humber
Questions about estuaries
Estuary
Draw and label an energy pyramid
Will and might for predictions
Romeo and juliet nicknames
Prediction vs inference venn diagram
Good readers making prediction by
Making predictions ppt
Make a prediction
Making predictions with experimental probability
Assumptions generalizations predictions vs. conclusion
The future simple predictions
Making predictions practice
Can you make predictions about tech devices
Animal farm predictions
Making predictions quiz
Making predictions reading strategy